- 1Jiangsu Key Laboratory of New Drug Research and Clinical Pharmacy, School of Pharmacy, Xuzhou Medical University, Xuzhou, China
- 2Department of Pharmacy, Feng Xian People’s Hospital, Xuzhou, China
- 3School Infirmary, Jiangsu Normal University, Xuzhou, China
- 4Department of Pharmacy, Suzhou Science & Technology Town Hospital, Gusu School, Nanjing Medical University, Suzhou, China
- 5Department of Endocrinology, Huaian Hospital of Huaian City, Huaian, China
The present study aimed to explore the effect of carnitine supplementation on body weight in patients with polycystic ovary syndrome (PCOS) and predict an appropriate dosage schedule using a machine-learning approach. Data were obtained from literature mining and the rates of body weight change from the initial values were selected as the therapeutic index. The maximal effect (Emax) model was built up as the machine-learning model. A total of 242 patients with PCOS were included for analysis. In the machine-learning model, the Emax of carnitine supplementation on body weight was −3.92%, the ET50 was 3.6 weeks, and the treatment times to realize 25%, 50%, 75%, and 80% (plateau) Emax of carnitine supplementation on body weight were 1.2, 3.6, 10.8, and 14.4 weeks, respectively. In addition, no significant relationship of dose-response was found in the dosage range of carnitine supplementation used in the present study, indicating the lower limit of carnitine supplementation dosage, 250 mg/day, could be used as a suitable dosage. The present study first explored the effect of carnitine supplementation on body weight in patients with PCOS, and in order to realize the optimal therapeutic effect, carnitine supplementation needs 250 mg/day for at least 14.4 weeks.
Introduction
Polycystic ovary syndrome (PCOS) is a common endocrine disease, influencing approximately 5–15% of reproductive-age women (1). The clinical characteristics of PCOS include polycystic ovarian morphology, ovulatory dysfunction, and hyperandrogenism (2, 3). The exact mechanism of PCOS has not been elucidated; however, it is clear that the syndrome is associated with metabolic syndrome, obesity, or other comorbidities (4, 5). In recent years, the incidence of PCOS was increasing (6–8), which could cause ratio imbalance of estrogen, insulin resistance, hyperinsulinemia, hyperandrogenemia, endometrial dysfunction, infertility, cardiovascular disease, and obesity (9). In addition, the hormone secretion imbalance of patients with PCOS was usually along with many clinical symptoms, such as menstrual cycle disorders, hirsutism, and obesity, jeopardizing the daily activities of the patients (10–12). The main worldwide reason for female infertility, PCOS, has attracted more and more attention for its features, instead of simply ovarian disease (9). Therefore, the treatment of PCOS has been placed in an increasingly important position.
Carnitine, an essential nutrient in b-oxidation of fatty acids and found mostly in animal foods, such as meat, fish, milk, and dairy products, is the carrier of fatty acids across the inner mitochondrial membrane (13). Many studies had verified that there were potential roles of carnitine in patients with PCOS, such as losing weight and normalizing metabolic profiles (14). Its function is to accelerate fat burning and decomposition by transporting fat to mitochondria. As is known to everyone that carnitine is not a cure for obesity; however, the regulation of PCOS patients’ body weight from carnitine can promote the progress of PCOS treatment. That was to say, the regulation of the body weight of patients with PCOS by carnitine is beneficial to the treatment of PCOS. However, the quantitative effects of carnitine supplementation on body weight in patients with PCOS and optimal treatment scheme are still unknown. Thus, the present study aimed to explore the effects of carnitine supplementation on body weight in patients with PCOS and predict an appropriate dosage schedule using a machine-learning approach.
Materials and methods
Enrolled patients
To study the effect of carnitine supplementation on body weight in patients with PCOS, patients with PCOS treated with carnitine supplementation were enrolled from the literature report. The search details are shown in Supplementary Table 1, meantime the inclusion criteria were as follows: (i) patients with PCOS, (ii) patients with the treatment of carnitine supplementation, (iii) patients with body weight information, (iv) patients with exact doses and durations of carnitine supplementation, and (v) patients with the control group. In addition, source, group, carnitine dosage, duration of treatment, body weight, number of people, and age were included in a dataset.
For eliminating the potential baseline effect of different studies, the rates of body weight change from the initial values were selected as the therapeutic index. The computational formula is shown in Equation (a):
where Etime is body weight value at a time and Ebase is body weight value at baseline.
Model building
The maximal effect (Emax) model built up via non-linear mixed-effect modeling (NONMEM, edition 7, ICON Development Solutions, Ellicott City, MD, United States) was used for analysis. In addition, in order to acquire the actual effect of carnitine supplementation on body weight in patients with PCOS, the control effect was needed to be removed from the sum effect, which is shown in Equations (b) and (c):
where EA, i, j represents the sum effect of carnitine supplementation on body weight in patients with PCOS, such as actual effect and control effect. EB, i, j represents the actual effect of carnitine supplementation on body weight in patients with PCOS. EC, i, j represents the control effect of carnitine supplementation on body weight in patients with PCOS. i represents different studies and j represents every study’s time point. Emax is the carnitine supplementation’s maximal effect on body weight in patients with PCOS. ET50 represents the treatment time to achieve half of Emax from carnitine supplementation on body weight in patients with PCOS. εi, j represents the study i with j time’s residual error. Ni, j represents the study i with time point j’s sample size. εi, j is weighted by sample size, which is assumed to be distributed normally, and the distribution characteristic is a mean of 0 and variance of σ2/(Ni, j/100).
The exponential error model or additive error model was used for assessing the inter-study variability, which is shown in formulas (d–g):
where η1, i and η2, i represent the variabilities of inter-study, which are added into Emax or ET50 when available and they are assumed to be distributed normally and the distribution characteristics are a mean of 0 and variance of ω1, i2 and ω2, i2, respectively.
The categorical covariates and continuous covariates were assessed using Equations (h) to (j):
Where Ppat represents the patient’s parameter who was with a covariate value of COVar. Pv represents the parameter’s typical value. COVar represents the value of covariate. COVmed represents the covariate’s median value of the population. θcor represents the covariate’s correction coefficient with the model parameter.
The potential covariates included source, carnitine dosage, initial body weight, and age, when the basic model was built up, potential covariates were considered to add into Emax or ET50, respectively. If one or more potential covariables were included in Emax or ET50, they indicated that one or several covariables would affect efficacy through influencing Emax or ET50. If no potential covariable was included, it meant that none of the current potential covariables would affect efficacy. The changes from the objective function value (OFV) were selected for the criteria of covariate inclusion. If the OFV value’s reduction was more than 3.84 (χ2, α = 0.05, degrees of freedom (d.f.) = 1), the corresponding covariable met the inclusion criteria. If the increase of OFV value was more than 6.63 (χ2, α = 0.01, d.f. = 1), the corresponding covariable met the criteria, adding to the final model (15).
Model evaluation
The final model was evaluated using goodness-of-fit plots [individual predictions vs. observations, conditional-weighted residuals (WRES) vs. time] and the distribution of conditional WRES for the model (quantiles of conditional WRES vs. quantiles of normal). The bootstrap’s median values and 2.5–97.5% values were used for comparing to the final model parameter values. In addition, the predictive performance of the final model was assessed by prediction-corrected visual predictive check (VPC) plots.
Model prediction
The final model’s Emax efficacy curve included the time of duration required to achieve 25%, 50%, 75%, and 80% (plateau) Emax of carnitine supplementation on body weight in patients with PCOS were simulated using the Monte Carlo method.
Results
Included patients
A total of 242 patients with PCOS were included for modeling (16–19). The carnitine dosage was from 250 to 1,000 mg/day, and the duration of treatment was 12 weeks. The mean age range of patients with PCOS was from 23.6 to 30.8 years. The change rates of body weight from baseline in the included studies were from −1.97 to −3.88%. Supplementary Figure 1 is a strategy for literature search and Supplementary Table 2 shows the detailed patients included in the analysis. The body weight change of the studied subjects post-carnitine administration is shown in Supplementary Figure 2.
Model building
In the final model of machine learning, the Emax of carnitine supplementation on body weight was −3.92%, and the ET50 was 3.6 weeks, in addition, no covariate was included in the final model.
The final model is shown in formula (k):
Where E represents the effect of carnitine supplementation on body weight in patients with PCOS. The time represents carnitine supplementation treatment duration in patients with PCOS.
Model evaluation
Individual predictions vs. observations and conditional WRES vs. time are shown in Figure 1, and quantiles of conditional WRES vs. quantiles of normal are shown in Figure 2, indicating better correlativity in individual predictions and observations. Figure 3 shows the prediction-corrected VPC plots, where most observed data fell within the 95% confidence interval (CI) generated by model simulations, showing that the final model had good forecasting ability. Table 1 shows the final model’s parameter estimated values and bootstrap, where the 1,000 bootstrap’s median values are near to the corresponding final model’s parameter values, showing that the final model was accurate and reliable.
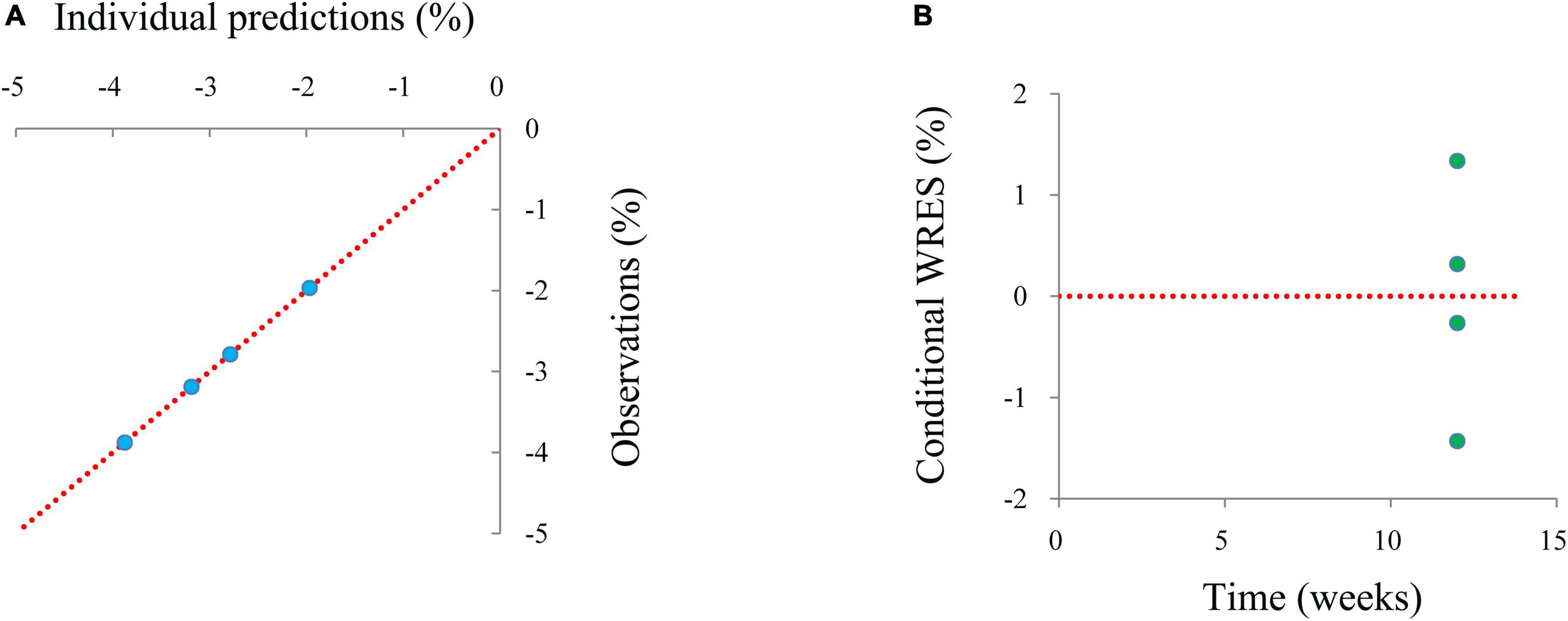
Figure 1. The goodness-of-fit plots of a model. (A) Individual predictions vs. observations, (B) conditional-weighted residuals (WRES) vs. time.
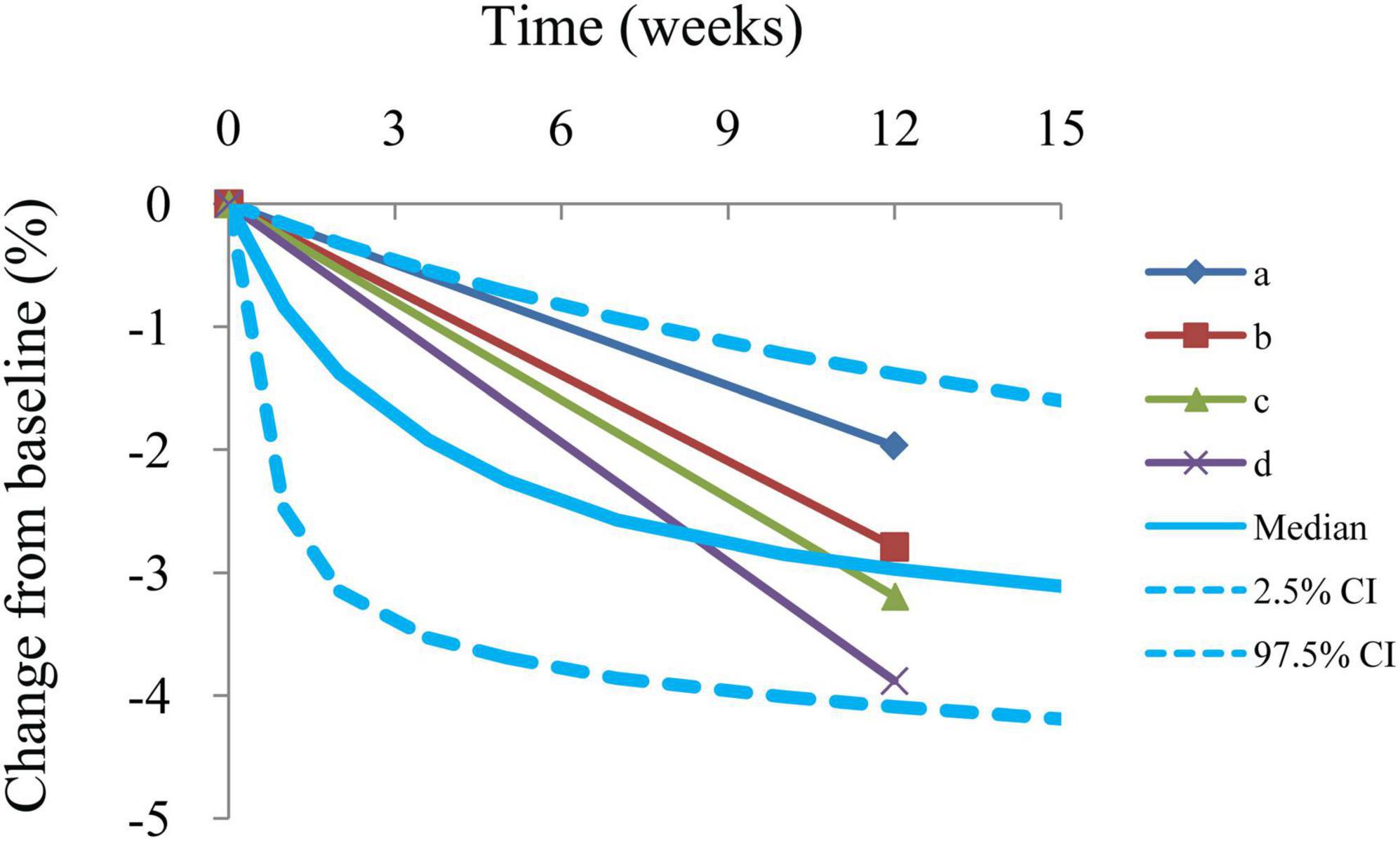
Figure 3. Prediction-corrected visual predictive check plots. Median, 2.5% CI, and 97.5% CI were simulated by Monte Carlo (n = 1,000); CI, confidence interval. a–d: 4 groups from included studies (16–19).
Model prediction
Figure 4 is the efficacy trend from carnitine supplementation on body weight in patients with PCOS, where the treatment times to achieve 25%, 50%, 75%, and 80% (plateau) Emax of carnitine supplementation on body weight are 1.2, 3.6, 10.8, and 14.4 weeks, respectively. In addition, no covariate was included in the model. No significant relationship of dose-response was found in the dosage range of carnitine supplementation used in this study whose carnitine dosage was from 250 to 1,000 mg/day, indicating the lower limit of carnitine supplementation dosage, 250 mg/day, could be used as a suitable dosage. In other words, in order to realize the ideal therapeutic effect, carnitine supplementation needs 250 mg/day for at least 14.4 weeks.
Discussion
Carnitine, whose structural formula is an essential water-soluble molecule (20), is one of the quaternary ammonium cationic complexes with two stereoisomers (bioactive L-carnitine and abiotic enantiomeric isomer D-carnitine) (21), among which the main carnitine available in the medical and biological domain is L-carnitine, which is also called “carnitine” (21), and whose quality in the human body is approximately 300 mg/kg (21, 22). D-carnitine without bioactivities in people, however, has adverse biological effects via suppressing the carnitine acetyltransferase (23), with supplementation of D-carnitine inducing oxidative stress, liver inflammation, and apoptosis in animal studies (24), causing secondary carnitine deficiency, and therefore, almost no human studies of D-carnitine have been conducted (21).
At present, carnitine has many functions, including reducing oxidative stress, increasing expressions of pro-inflammatory cytokines (25–27), improving mitochondrial dysfunction (28) and insulin resistance (29, 30). Therefore, based on these effects, carnitine has an increasingly key role in treating some illnesses. For example, it was found that carnitine as a supplement may be used to treat diabetes mellitus (29, 30), liver cirrhosis (31), dyslipidemia (32), hypertension (33), heart failure (34), coronary artery disease (35), non-alcoholic fatty liver disease (36), Alzheimer’s disease (37), migraine (38), and muscle injury (39). In addition, carnitine treatment of PCOS had been reported in many pieces of literature, for example, according to the current evidence, Maleki et al. found that carnitine might improve weight loss, glycemic status, and oxidative stress (14). Salehpour et al. found that treatment with carnitine in patients with PCOS might decrease the risk of cardiovascular events by normalizing metabolic profiles and body mass index (BMI) using self-controlled study before and after treatment (40). Liao et al. found that carnitine supplementation in women with PCOS had beneficial effects on weight loss and lipid profiles (41). However, the quantitative effects of carnitine supplementation on body weight in patients with PCOS and optimal treatment scheme are still unknown. Therefore, the present study aimed to explore the effect of carnitine supplementation on body weight in patients with PCOS and predict an appropriate dosage schedule using a machine-learning approach.
Machine learning was one of the artificial intelligence fields, which could be used for solving medical problems via building up computational modeling based on data. Compared with traditional statistical analysis, machine learning had better behavior (42, 43). Nowadays, machine learning is widely used in the medical field, for example, Tsang et al. reported a pilot study of machine-learning-based automated planning for primary brain tumors (44). Wang et al. reported predicting the targets of interferon regulatory factor-8 (IRF8) and nuclear factor-activated T cells c1 (NFATc1) during osteoclast differentiation using the machine-learning method framework cohort-based TF target prediction system (cTAP) (45). Ohanyan et al. reported that machine-learning approaches to characterize the obesogenic urban exposome (46). Ku et al. reported machine-learning models for predicting the occurrence of respiratory diseases using climatic and air-pollution factors (47). Zeng et al. reported developing a machine-learning model to predict severe chronic obstructive pulmonary disease exacerbations: a retrospective cohort study (48). Ebrahimi et al. reported predictive determinants of overall survival among re-infected patients with COVID-19 using the elastic-net regularized Cox proportional hazards model: a machine-learning algorithm (49). Together, these studies further supported the feasibility of machine-learning approaches to problem solving.
In the present study, data were obtained from literature mining, and the rates of body weight change from the initial values were selected as the therapeutic index. The Emax model built up via NONMEM was used as the machine-learning model. Weight reduction in kilograms was not taken into account, because the initial body weight of patients with PCOS varied in several of the studies included. Therefore, to eliminate the potential baseline effect of different studies, the rates of body weight change from the initial values were selected as the therapeutic index, which was a better indicator used to assess changes in body weight. In addition, as was known to everyone, the calorie deficit diet, Mediterranean diet, or vegetarian diet may affect weight change. Thus, the carnitine group included in this study had its corresponding control group, so the non-carnitine effect of the control group was deducted from the carnitine treatment group mainly included the effect from the diet, etc. In other words, in order to acquire the actual effect of carnitine supplementation on body weight in patients with PCOS, the control effect needs to be removed from the sum effect. Certainly, this method had been applied in many previous studies (50, 51). Through this scheme, the influence of different diets in different studies could be eliminated. More importantly, the actual effect of carnitine on the body weight of patients with PCOS could be obtained.
In addition, in the four studies included in the analysis, the diagnosis of PCOS was made according to the Rotterdam criteria. A total of 242 patients with PCOS were included for modeling (16–19). In the machine-learning model, the Emax of carnitine supplementation on body weight was − 3.92%, the ET50 was 3.6 weeks, and the treatment times to achieve 25%, 50%, 75%, and 80% (plateau) Emax of carnitine supplementation on body weight were 1.2, 3.6, 10.8, and 14.4 weeks, respectively. The potential covariates included source, carnitine dosage, initial body weight, and age. If one or more potential covariables could be included in Emax or ET50, indicating that one or several covariables would affect efficacy through influencing Emax or ET50. If no potential covariable was included, it meant that none of the current potential covariables would affect efficacy. The final model found that no covariate was included in the final model. No significant relationship of dose-response was found in the dosage range of carnitine supplementation used in this study whose carnitine dosage was from 250 to 1,000 mg/day, indicating the lower limit of carnitine supplementation dosage, 250 mg/day, could be used as a suitable dosage. In other words, in order to realize the ideal therapeutic effect, carnitine supplementation needs 250 mg/day for at least 14.4 weeks.
However, this study also has some limitations. First of all, the study population was mainly from Iran, and the number of participants was small, so it needs to be further verified by increasing the sample size in future studies.
Conclusion
The present study explored the effect of carnitine supplementation on body weight in patients with PCOS using machine learning for the first time, and in order to realize the optimal therapeutic effect, carnitine supplementation needs 250 mg/day for at least 14.4 weeks.
Data availability statement
The original contributions presented in this study are included in the article/Supplementary material, further inquiries can be directed to the corresponding authors.
Author contributions
D-DW, S-MH, PZ, and Q-LW conceived and designed the study. D-DW, Y-FL, Y-ZM, S-MH, PZ, and Q-LW collected the data. D-DW built the model, evaluated the data, and wrote the manuscript. All authors read and approved the manuscript.
Funding
This work was supported by the Xuzhou Special fund for promoting scientific and technological innovation (no. KC21257), the Initializing Fund of Xuzhou Medical University (no. RC20552111), the Fusion Innovation Project of Xuzhou Medical University (no. XYRHCX2021011), the Suzhou Science & Technology Town Hospital pre-research fund project (no. 2019Y01), the Suzhou Science and Technology Development Plan Project (no. SYSD2019076), the Jiangsu Pharmaceutical Society-Tianqing Hospital Pharmaceutical Fund Project (no. Q202024), and Suzhou Key Clinical Specialty Project (2021).
Conflict of interest
The authors declare that the research was conducted in the absence of any commercial or financial relationships that could be construed as a potential conflict of interest.
Publisher’s note
All claims expressed in this article are solely those of the authors and do not necessarily represent those of their affiliated organizations, or those of the publisher, the editors and the reviewers. Any product that may be evaluated in this article, or claim that may be made by its manufacturer, is not guaranteed or endorsed by the publisher.
Supplementary material
The Supplementary Material for this article can be found online at: https://www.frontiersin.org/articles/10.3389/fnut.2022.851275/full#supplementary-material
References
1. Yildiz BO, Bozdag G, Yapici Z, Esinler I, Yarali H. Prevalence, phenotype and cardiometabolic risk of polycystic ovary syndrome under different diagnostic criteria. Hum Reprod. (2012) 27:3067–73. doi: 10.1093/humrep/des232
2. Rotterdam ESHRE/ASRM-Sponsored PCOS consensus workshop group. Revised 2003 consensus on diagnostic criteria and long-term health risks related to polycystic ovary syndrome (PCOS). Hum Reprod. (2004) 19:41–7. doi: 10.1093/humrep/deh098
3. Eyupoglu ND, Ergunay K, Acikgoz A, Akyon Y, Yilmaz E, Yildiz BO. Gut microbiota and oral contraceptive use in overweight and obese patients with polycystic ovary syndrome. J Clin Endocrinol Metab. (2020) 105:dgaa600. doi: 10.1210/clinem/dgaa600
4. Orio F, Muscogiuri G, Nese C, Palomba S, Savastano S, Tafuri D, et al. Obesity, type 2 diabetes mellitus and cardiovascular disease risk: an uptodate in the management of polycystic ovary syndrome. Eur J Obstet Gynecol Reprod Biol. (2016) 207:214–9. doi: 10.1016/j.ejogrb.2016.08.026
5. Chen X, He S, Wang D. Effects of metformin on body weight in polycystic ovary syndrome patients: model-based meta-analysis. Expert Rev Clin Pharmacol. (2021) 14:121–30. doi: 10.1080/17512433.2021.1863788
6. Ganie MA, Rashid A, Sahu D, Nisar S, Wani IA, Khan J. Prevalence of polycystic ovary syndrome (PCOS) among reproductive age women from Kashmir valley: a cross-sectional study. Int J Gynaecol Obstet. (2020) 149:231–6. doi: 10.1002/ijgo.13125
7. Kyrou I, Karteris E, Robbins T, Chatha K, Drenos F, Randeva HS. Polycystic ovary syndrome (PCOS) and COVID-19: an overlooked female patient population at potentially higher risk during the COVID-19 pandemic. BMC Med. (2020) 18:220. doi: 10.1186/s12916-020-01697-5
8. Ozkan S, Yilmaz OC, Yavuz B. Increased masked hypertension prevalence in patients with polycystic ovary syndrome (PCOS). Clin Exp Hypertens. (2020) 42:681–4. doi: 10.1080/10641963.2020.1772815
9. Cao C, Qi Y, Fang D, Yu Y. Clinical study on polycystic ovary syndrome treated with diane-35 and pioglitazone. Am J Transl Res. (2021) 13:12742–9.
10. Ollila MM, West S, Keinanen-Kiukaanniemi S, Jokelainen J, Auvinen J, Puukka K, et al. Overweight and obese but not normal weight women with PCOS are at increased risk of type 2 diabetes mellitus-a prospective, population-based cohort study. Hum Reprod. (2017) 32:423–31. doi: 10.1093/humrep/dew329
11. Ntumy M, Maya E, Lizneva D, Adanu R, Azziz R. The pressing need for standardization in epidemiologic studies of PCOS across the globe. Gynecol Endocrinol. (2019) 35:1–3. doi: 10.1080/09513590.2018.1488958
12. Liu J, Ding J, Qu B, Liu J, Song X, Suo Q, et al. CircPSMC3 alleviates the symptoms of PCOS by sponging miR-296-3p and regulating PTEN expression. J Cell Mol Med. (2020) 24:11001–11. doi: 10.1111/jcmm.15747
13. El-Hattab AW, Scaglia F. Disorders of carnitine biosynthesis and transport. Mol Genet Metab. (2015) 116:107–12. doi: 10.1016/j.ymgme.2015.09.004
14. Maleki V, Jafari-Vayghan H, Kashani A, Moradi F, Vajdi M, Kheirouri S, et al. Potential roles of carnitine in patients with polycystic ovary syndrome: a systematic review. Gynecol Endocrinol. (2019) 35:463–9. doi: 10.1080/09513590.2019.1576616
15. Wang DD, Mao YZ, He SM, Chen X. Analysis of time course and dose effect from metformin on body mass index in children and adolescents. Front Pharmacol. (2021) 12:611480. doi: 10.3389/fphar.2021.611480
16. Samimi M, Jamilian M, Ebrahimi FA, Rahimi M, Tajbakhsh B, Asemi Z. Oral carnitine supplementation reduces body weight and insulin resistance in women with polycystic ovary syndrome: a randomized, double-blind, placebo-controlled trial. Clin Endocrinol (Oxf). (2016) 84:851–7. doi: 10.1111/cen.13003
17. Jamilian H, Jamilian M, Samimi M, Afshar Ebrahimi F, Rahimi M, Bahmani F, et al. Oral carnitine supplementation influences mental health parameters and biomarkers of oxidative stress in women with polycystic ovary syndrome: a randomized, double-blind, placebo-controlled trial. Gynecol Endocrinol. (2017) 33:442–7. doi: 10.1080/09513590.2017.1290071
18. Talari HR, Azad ZJ, Hamidian Y, Samimi M, Gilasi HR, Ebrahimi Afshar F, et al. Effects of carnitine administration on carotid intima-media thickness and inflammatory factors in patients with polycystic ovary syndrome: a randomized, double-blind, placebo-controlled trial. Int J Prev Med. (2019) 10:89. doi: 10.4103/ijpvm.IJPVM_2_18
19. Sangouni AA, Pakravanfar F, Ghadiri-Anari A, Nadjarzadeh A, Fallahzadeh H, Hosseinzadeh M. The effect of L-carnitine supplementation on insulin resistance, sex hormone-binding globulin and lipid profile in overweight/obese women with polycystic ovary syndrome: a randomized clinical trial. Eur J Nutr. (2021) 61:1199–207. doi: 10.1007/s00394-021-02659-0
20. Almannai M, Alfadhel M, El-Hattab AW. Carnitine inborn errors of metabolism. Molecules. (2019) 24:3251. doi: 10.3390/molecules24183251
21. Li N, Zhao H. Role of carnitine in non-alcoholic fatty liver disease and other related diseases: an update. Front Med. (2021) 8:689042. doi: 10.3389/fmed.2021.689042
22. Adeva-Andany MM, Calvo-Castro I, Fernandez-Fernandez C, Donapetry-Garcia C, Pedre-Pineiro AM. Significance of L-carnitine for human health. IUBMB Life. (2017) 69:578–94. doi: 10.1002/iub.1646
23. Vashistha VK, Bhushan R. Bioanalysis and enantioseparation of dl-carnitine in human plasma by the derivatization approach. Bioanalysis. (2015) 7:2477–88. doi: 10.4155/bio.15.155
24. Li JM, Li LY, Zhang YX, Jiang ZY, Limbu SM, Qiao F, et al. Functional differences between l- and d-carnitine in metabolic regulation evaluated using a low-carnitine Nile tilapia model. Br J Nutr. (2019) 122:625–38. doi: 10.1017/S000711451900148X
25. Komlosi K, Havasi V, Bene J, Sule N, Pajor L, Nicolai R, et al. Histopathologic abnormalities of the lymphoreticular tissues in organic cation transporter 2 deficiency: evidence for impaired B cell maturation. J Pediatr. (2007) 150:109–11.e102. doi: 10.1016/j.jpeds.2006.09.042
26. Lee BJ, Lin JS, Lin YC, Lin PT. Effects of L-carnitine supplementation on oxidative stress and antioxidant enzymes activities in patients with coronary artery disease: a randomized, placebo-controlled trial. Nutr J. (2014) 13:79. doi: 10.1186/1475-2891-13-79
27. Ribas GS, Vargas CR, Wajner M. L-carnitine supplementation as a potential antioxidant therapy for inherited neurometabolic disorders. Gene. (2014) 533:469–76. doi: 10.1016/j.gene.2013.10.017
28. Modanloo M, Shokrzadeh M. Analyzing mitochondrial dysfunction, oxidative stress, and apoptosis: potential role of L-carnitine. Iran J Kidney Dis. (2019) 13:74–86.
29. Xu Y, Jiang W, Chen G, Zhu W, Ding W, Ge Z, et al. L-carnitine treatment of insulin resistance: a systematic review and meta-analysis. Adv Clin Exp Med. (2017) 26:333–8. doi: 10.17219/acem/61609
30. Bene J, Hadzsiev K, Melegh B. Role of carnitine and its derivatives in the development and management of type 2 diabetes. Nutr Diabetes. (2018) 8:8. doi: 10.1038/s41387-018-0017-1
31. Hanai T, Shiraki M, Imai K, Suetugu A, Takai K, Shimizu M. Usefulness of carnitine supplementation for the complications of liver cirrhosis. Nutrients. (2020) 12:1915. doi: 10.3390/nu12071915
32. Askarpour M, Hadi A, Symonds ME, Miraghajani M, Omid S, Sheikhi A, et al. Efficacy of L-carnitine supplementation for management of blood lipids: a systematic review and dose-response meta-analysis of randomized controlled trials. Nutr Metab Cardiovasc Dis. (2019) 29:1151–67. doi: 10.1016/j.numecd.2019.07.012
33. Askarpour M, Hadi A, Dehghani Kari Bozorg A, Sadeghi O, Sheikhi A, Kazemi M, et al. Effects of L-carnitine supplementation on blood pressure: a systematic review and meta-analysis of randomized controlled trials. J Hum Hypertens. (2019) 33:725–34. doi: 10.1038/s41371-019-0248-1
34. Kinugasa Y, Sota T, Ishiga N, Nakamura K, Kamitani H, Hirai M, et al. L-carnitine supplementation in heart failure patients with preserved ejection fraction; a pilot study. Geriatr Gerontol Int. (2020) 20:1244–5. doi: 10.1111/ggi.14060
35. Nachvak SM, Shabanpur M, Mostafai R, Heidari Moghaddam R, Moludi J. L-carnitine supplementation reduces biomarkers of inflammatory and oxidative stress in patients with coronary artery disease: a randomised controlled trial. Arch Physiol Biochem. (2020) 20:1–8. doi: 10.1080/13813455.2020.1797102 [Epub ahead of print].
36. Savic D, Hodson L, Neubauer S, Pavlides M. The importance of the fatty acid transporter L-carnitine in non-alcoholic fatty liver disease (NAFLD). Nutrients. (2020) 12:2178. doi: 10.3390/nu12082178
37. Kepka A, Ochocinska A, Borzym-Kluczyk M, Skorupa E, Stasiewicz-Jarocka B, Chojnowska S, et al. Preventive role of L-carnitine and balanced diet in Alzheimer’s disease. Nutrients. (2020) 12:1987. doi: 10.3390/nu12071987
38. Hajihashemi P, Askari G, Khorvash F, Reza Maracy M, Nourian M. The effects of concurrent coenzyme Q10, L-carnitine supplementation in migraine prophylaxis: a randomized, placebo-controlled, double-blind trial. Cephalalgia. (2019) 39:648–54. doi: 10.1177/0333102418821661
39. Yarizadh H, Shab-Bidar S, Zamani B, Vanani AN, Baharlooi H, Djafarian K. The effect of L-carnitine supplementation on exercise-induced muscle damage: a systematic review and meta-analysis of randomized clinical trials. J Am Coll Nutr. (2020) 39:457–68. doi: 10.1080/07315724.2019.1661804
40. Salehpour S, Nazari L, Hoseini S, Moghaddam PB, Gachkar L. Effects of L-carnitine on polycystic ovary syndrome. JBRA Assist Reprod. (2019) 23:392–5. doi: 10.5935/1518-0557.20190033
41. Liao D, Liu X, Yuan X, Feng P, Ouyang Z, Liu Y, et al. Clinical evidence of the effects of carnitine supplementation on body weight, glycemic control and serum lipids in women with polycystic ovary syndrome: a systematic review and meta-analysis. Gynecol Endocrinol. (2022) 38:110–5. doi: 10.1080/09513590.2021.1988559
42. Beam AL, Kohane IS. Big data and machine learning in health care. JAMA. (2018) 319:1317–8. doi: 10.1001/jama.2017.18391
43. Liu LP, Zhao QY, Wu J, Luo YW, Dong H, Chen ZW, et al. Machine learning for the prediction of red blood cell transfusion in patients during or after liver transplantation surgery. Front Med. (2021) 8:632210. doi: 10.3389/fmed.2021.632210
44. Tsang DS, Tsui G, McIntosh C, Purdie T, Bauman G, Dama H, et al. A pilot study of machine-learning based automated planning for primary brain tumours. Radiat Oncol. (2022) 17:3. doi: 10.1186/s13014-021-01967-3
45. Wang H, Joshi P, Hong SH, Maye PF, Rowe DW, Shin DG. Predicting the targets of IRF8 and NFATc1 during osteoclast differentiation using the machine learning method framework cTAP. BMC Genomics. (2022) 23:14. doi: 10.1186/s12864-021-08159-z
46. Ohanyan H, Portengen L, Huss A, Traini E, Beulens JWJ, Hoek G, et al. Machine learning approaches to characterize the obesogenic urban exposome. Environ Int. (2022) 158:107015. doi: 10.1016/j.envint.2021.107015
47. Ku Y, Kwon SB, Yoon JH, Mun SK, Chang M. Machine learning models for predicting the occurrence of respiratory diseases using climatic and air-pollution factors. Clin Exp Otorhinolaryngol. (2022) 15:168–76. doi: 10.21053/ceo.2021.01536
48. Zeng S, Arjomandi M, Tong Y, Liao ZC, Luo G. Developing a machine learning model to predict severe chronic obstructive pulmonary disease exacerbations: retrospective cohort study. J Med Internet Res. (2022) 24:e28953. doi: 10.2196/28953
49. Ebrahimi V, Sharifi M, Mousavi-Roknabadi RS, Sadegh R, Khademian MH, Moghadami M, et al. Predictive determinants of overall survival among re-infected COVID-19 patients using the elastic-net regularized Cox proportional hazards model: a machine-learning algorithm. BMC Public Health. (2022) 22:10. doi: 10.1186/s12889-021-12383-3
50. Wang DD, He SM, Han Y, Wang TY, Wang YM. Quantifying the relationship between dapagliflozin and loss of weight in type 1 diabetes mellitus patients. J Clin Pharm Ther. (2022) 47:237–42. doi: 10.1111/jcpt.13572
Keywords: machine learning, predicting, carnitine supplementation, body weight, polycystic ovary syndrome
Citation: Wang D-D, Li Y-F, Mao Y-Z, He S-M, Zhu P and Wei Q-L (2022) A machine-learning approach for predicting the effect of carnitine supplementation on body weight in patients with polycystic ovary syndrome. Front. Nutr. 9:851275. doi: 10.3389/fnut.2022.851275
Received: 09 January 2022; Accepted: 21 July 2022;
Published: 10 August 2022.
Edited by:
Alessandra Durazzo, Council for Agricultural Research and Economics, ItalyReviewed by:
Mohd Ashraf Ganie, Sher-I-Kashmir Institute of Medical Sciences, IndiaMałgorzata Szczuko, Pomeranian Medical University, Poland
Copyright © 2022 Wang, Li, Mao, He, Zhu and Wei. This is an open-access article distributed under the terms of the Creative Commons Attribution License (CC BY). The use, distribution or reproduction in other forums is permitted, provided the original author(s) and the copyright owner(s) are credited and that the original publication in this journal is cited, in accordance with accepted academic practice. No use, distribution or reproduction is permitted which does not comply with these terms.
*Correspondence: Su-Mei He, aGVoZTgyMDRAMTYzLmNvbQ==; Ping Zhu, enBpbmcxOTgzQDE2My5jb20=; Qun-Li Wei, d2VpcXVubGlAMTI2LmNvbQ==
†These authors have contributed equally to this work and share first authorship