- 1Department of Medical and Surgical Science Nutrition Unit, University Magna Grecia, Catanzaro, Italy
- 2Department of Biomedicine and Prevention, University of Tor Vergata, Rome, Italy
- 3Department of Experimental Medicine, University La Sapienza, Rome, Italy
- 4Department of Clinical and Experimental Medicine, Nutrition Unit, University Magna Grecia, Catanzaro, Italy
In recent times, it has become evident that there are individuals who, from a metabolic point of view, are affected by obesity but have a normal body mass index. There are also metabolically healthy individuals with a high body mass index who are thus are considered as to be affected by obesity obese. Understanding that individuals with obesity are phenotypically heterogeneous is a relatively novel concept which, although present in the scientific literature, unfortunately has not yet had an impact in clinical practice. However, common dietary approaches are not effective in treating large numbers of obese patients with obesity. This narrative review, based on the material searched via PubMed and the Web of Science up to October 2021, proposes a downsizing of the role of the body mass index in identifying the individual with “true obesity” since it is only partially useful, and suggests a new approach which also integrates the body composition and assessment of metabolic parameters. This approach leads to personalized therapies that work best for each obesity phenotype in reducing the risk of non-communicable diseases.
Introduction
Worldwide obesity has nearly tripled over the past 50 years (1). In this period, the prevalence of obesity, and especially severe obesity, has increased in adults by 42% (2), thus currently over 650 million adults are affected by obesity, while more than 1.9 billion adults are overweight (1). By 2030, the respective number of adults who are overweight and affected by obesity is projected to be 2.16 billion and 1.12 billion, respectively (3).
The global deaths and disability-adjusted life years (DALYs) attributable to a high body mass index (BMI) were analyzed in the Global Burden of Disease (GBD) 2017 study (4). Cardiovascular disease (CVD) was found to be the leading cause of high BMI-related DALYs, followed by diabetes, kidney disease, and neoplasms. These conditions together accounted for 89.3% of all high-BMI-related DALYs (4). The GBD 2017 study demonstrated that a poor diet is responsible for more deaths than any other risk factor in the world, leading to one in five deaths being linked to unhealthy diets (4, 5). Obesity is thus an urgent problem that needs to be properly addressed. Physicians, public and global health policy decision-makers need timely, up-to-date scientific information to develop new interventions aimed at counteracting the burden generated by obesity. The importance of this has been magnified by the current COVID-19 pandemic, as obesity and the related chronic diseases are among the strongest predictors of COVID-19 severity and mortality (6).
Interest in the association between body shape and health outcomes date back over a century (7–9). However, it is now well recognized that more efficacious anthropometric biomarkers are required to better predict the onset of non-communicable diseases (NCDs) than BMI as well as new, well-functioning and tailored dietary/nutritional interventions for individuals affected by obesity (7, 8).
The benefits of this new approach are supported by three factors. First, we now have several advanced technologies that can identify the changes in body composition that precede the onset of NCDs. Second, it is now known that individuals with obesity are only apparently similar, whereas in fact they are phenotypically (and genotypically) heterogeneous. Third, no single management strategy is suitable for each individual patient and novel therapeutic approaches for treating particular obesity phenotypes and tailoring the treatment are already being generated in response to the new biological drivers.
This narrative review is based on the material searched for and obtained via PubMed and the Web of Science up to October 2021. The search terms used were: “obesity, obesity phenotypes, obesity paradox, adipose tissue, fat mass, lean mass, muscle mass, sarcopenia, DXA, body composition” in combination with “non-communicable disease, chronic diseases, cardiovascular, coronary heart disease, mortality, dietary pattern.”
Anthropometric Parameters as Biomarkers of Chronic Disease
The BMI, which is calculated by a person's weight in kilograms divided by the square of his or her height in meters (kg/m2), was initially used as a good indicator of obesity and correlated well with the development of several NCDs such as type 2 diabetes (T2DM), several types of cancer, osteoarthritis, asthma, and all-cause mortality (10–16). The BMI is widely used primarily because it is a simple, non-invasive and inexpensive test, which can be used at the population level to generate models that span geographical regions.
However, the BMI has several limitations. In fact, it cannot be used as a proxy of body fat content in individuals who tend to have a high lean body mass (LBM) (17). One meta-analysis suggested the protective effect of a high BMI on mortality and found that only severe obesity increased the risk of CVDs (18), thus, highlighting the BMI's limitation in clarifying the diverse body compartments (fat mass-FM, muscle mass-MM, etc.). In addition, in the Münster Heart Study (PROCAM), the risk of coronary heart disease (CHD) was mediated via other risk factors (19). All this evidence has generated uncertainty regarding the risks associated with obesity.
Are waist circumference (WC) or the waist-to-hip ratio (WHR) better biomarkers than the BMI? In 1947, the French physician Vague observed that his patients with both obesity and diabetes or clinical signs of a CVD had a central distribution of body fat and that the gynoid fat accumulation was rarely associated with these complications (20). However, very recently the medical community has recognized that WC and WHR are more strongly correlated to metabolic complications and cardiovascular outcomes than the BMI (21–23).
Other measures of body fat, and particularly visceral fat, seemed to be better indicators of the risk of obesity-related health issues (24, 25). In the INTERHEART study, the WHR was the strongest anthropometric predictor of myocardial infarction in both genders, across all age and ethnic groups, in smokers and non-smokers, and in those with or without the classical CV risk factors that are the consequences of obesity (26). A meta-analysis reported the superiority of centralized obesity measures, especially the waist-to-height ratio (WHtR) over the BMI for CVD risk detection (27). The association of the conventional BMI parameter with myocardial infarction was found to be weaker and less consistent across ethnic and other subgroups (26). In addition, neck circumference (NC) has been proposed as a quicker, more reliable and easier-to-apply anthropometric marker of central obesity (28). NC also predicts cardio-metabolic risk factors (28).
Results from the EPIC (European Prospective Investigation into Cancer and Nutrition) highlighted the use of the central obesity index in predicting the risk of death (29). In addition, the scientific literature continues to confirm the association between abdominal obesity and the risk of cancer (30–32). However, there could be considerable differences in the percentage of fat and LBM or MM between individuals with similar WC, WHR, NC, or BMI especially when these indices are compared across different ethnic groups (33).
In general, none of these anthropometric parameters differentiate between FM and MM, which have opposite health impacts. The main drawback of WC (and WHR) is also an inability to differentiate subcutaneous from visceral fat deposition. Even with the same WC value, a larger subcutaneous adipose tissue is observed in the gynoid region of an older female compared to a younger female (34). WHR and WC are, thus, not accurate indicators of abdominal visceral fat accumulation (35). Furthermore, WHR and WC measurements need standardization and training as there are several methods described for assessing these parameters using non-elastic flexible tape.
Novel Nutritional Biomarkers of Non-communicable Diseases
Biomarkers play an important role in the evaluation of the onset of chronic diseases as well as in the development of drug treatments for these conditions (36). Biomarkers may also be able to reflect the pathophysiological process of a specific disease and may be able to predict the prognosis and guide clinical decision making (37). Accuracy, precision, high sensitivity and specificity and low intra-individual variability are important characteristics of an ideal biomarker (36).
Because of the great advantages offered by imaging tools in research and clinics, the focus of clinicians is now moving to powerful imaging techniques such as computed tomography (CT), magnetic resonance (MR) imaging, dual-energy X-ray absorptiometry (DXA), or other techniques such as bioimpedance analysis (BIA) and ultrasound (US) to more accurately measure FM (38). Although some of the above methods can be expensive, in some cases invasive, and not readily accessible, they are more accurate at measuring body fat, and thus better at predicting the risk of obesity-related health issues. One limitation of using these tools is the need for specialized equipment and trained staff, which can be challenging in routine clinical practice.
In two large prospective cohort studies, a strong and linear association was found between FM, assessed by radiological imaging techniques, and mortality from all causes (39, 40), CVD, and cancer (40). In a cohort of postmenopausal women from the Women's Health Initiative (WHI) cohort, DXA-derived FM measures were positively associated with breast cancer risk (41). Among postmenopausal women with a normal BMI, both elevated trunk fat and reduced leg fat, assessed by DXA, were associated with an increased risk of CVD (42). However, FM should be normalized for body size, precisely to eliminate the differences in the %FM associated with one's height (43). The fat mass index (FMI), which is calculated by dividing FM by the square of height, could be therefore, a useful measure of obesity, better than FM alone.
However, the relationship between the risk of FM and NCDs is also influenced by the presence of sarcopenia, a clinical condition in which low muscle function is associated with a low quantity or quality of MM (44). In fact, in a large prospective cohort study, free-fat mass (FFM), rather than FM, was a stronger predictor of overall cancer risk (45). The discovery of the role of LBM in the mortality risk in patients with cardiac disease (46, 47), suggested that other body composition parameters, such as MM and appendicular skeletal muscle mass (ASMM), could be more appropriate biomarkers of NCDs. The prevalence of sarcopenia is significantly higher in individuals with different type of NCDs, with the highest prevalence in individuals affected by T2DM, CVD, dementia, and respiratory disease (48).
There is a particular phenotype of individual in whom, despite an excess of FM and a high BMI, the CV risk is low or not increased due to a preserved or high MM. Some authors have defined this phenomenon as “the obesity paradox,” in which obesity seemed to protect against CV diseases (47, 49, 50). The study of body composition and MM has clarified that obesity is not protective against NCDs unlike that maintaining MM and, thus there is a “BMI paradox,” rather than an “obesity paradox” (50). On the other hand, sarcopenia could worsen the effects of obesity, especially in older adults, resulting in a particular phenotype defined as “sarcopenic obesity” which was found to have a higher risk of all-cause mortality than obesity or sarcopenia alone (51).
Overall, all these studies highlight that, although clinically valuable, classical, old anthropometric measures have a limited sensitivity and specificity as screening modalities and are poorly predictive of clinical outcome. Understanding that individuals with obesity are phenotypically heterogeneous is a relatively novel concept which, although present in the scientific literature, unfortunately has not yet had an impact in clinical practice.
Progress in the Identification of Different Obesity Phenotypes
On the basis of the current scientific data, it is clear that individuals with obesity are a heterogenous group, probably requiring specific treatments.
It has become evident that there are individuals who are affected by obesity but have a BMI in the normal range, as well as metabolically healthy individuals with a high BMI, thus considered with obesity. We propose a downsizing of the role of the BMI in identifying individuals with “true obesity” which should be used if integrated with body composition and metabolic assessment.
A screening strategy in phenotyping individuals with obesity is thus urgently needed in the global strategy aimed at preventing NCDs. Image diagnostics, supported by technological innovations, provides an accurate and repeatable body composition assessment. Among the number of available technologies, bioimpedance analysis (BIA) and DXA could play a primary role as they are minimally or non-invasive, cost effective, easy to perform and, of course, accurate, as previously described (38).
Here we thus suggest that, on the basis on body composition assessment and metabolic status, and only in part on BMI, individuals with “true obesity” could be better identified. The term “obesity” therefore assumes a new and different connotation with respect to the meaning assumed up to now. Specifically, based on the integration of all these parameters, people could be categorized as having:
1. metabolically healthy normal-weight (MHN) (healthy individuals);
2. metabolically unhealthy non-obesity [MUN, which includes two different sub-phenotypes, previously identified as normal-weight obesity, NWO (46) and metabolic obesity normal-weight, MONW (52)];
3. metabolically healthy obesity (MHO);
4. metabolically unhealthy obesity [(MUO) (53), also defined as “complicated obesity” and which includes sarcopenic obesity-SO (54)];
5. lipodystrophic phenotype (LP), (Figure 1).
Based on BMI, only MHO and MUO would be defined as having obesity, while based on body composition assessment and metabolic characteristics, MUN (which include overweight individuals or those with a cluster of metabolic features as dyslipidemia and hyperglycemia) and LP (who have an abnormal fat accumulation) are also considered individuals with “true obesity.”
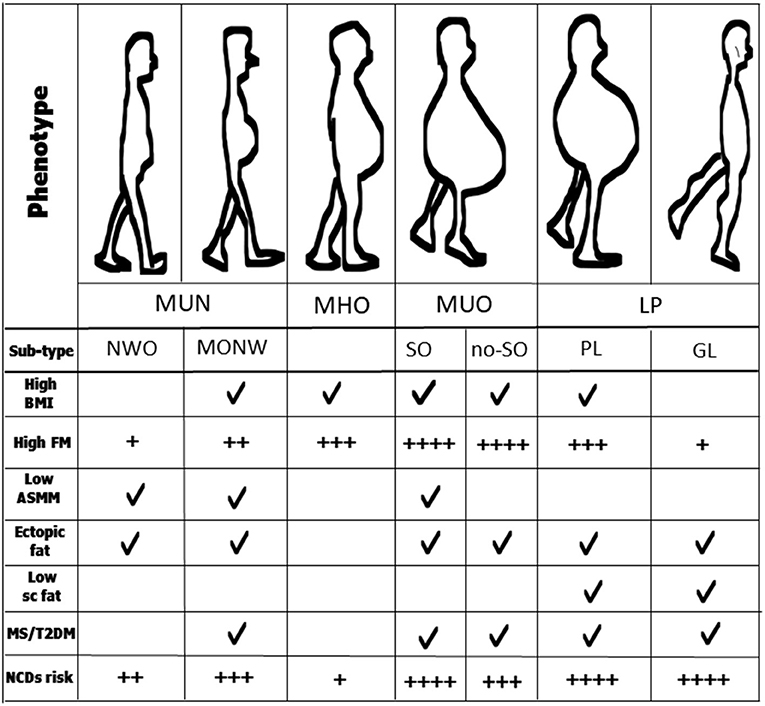
Figure 1. Anthropometrics and other clinical characteristics of obesity phenotypes and sub-types. MUN, normal-weight obesity; MHO, metabolically healthy obesity; MUO, metabolically unhealthy obesity; LP, lipodystrophic phenotype; NOW, normal-weight obesity; MONW, metabolic obesity normal-weight; SO, sarcopenic obesity; no-SO, no sarcopenic obesity; GL, generalized lipodystrophy; PL, partial lipodystrophy; BMI, body mass index; FM, fat mass; ASMM, appendicular skeletal muscle mass; MS, metabolic syndrome; T2DM, type 2 diabetes; NCDs, non-communicable diseases.
Epidemiology and Risk of Chronic Diseases Among Obesity Phenotypes
The real prevalence of these phenotypes around the world is not entirely known. The prevalence of MHO is estimated overall to be 6.5-10%, while it is about 30% in individuals with obesity. The prevalence of MUN is 20-30% in the general population with the NWO prevalence ranging from 4.5 to 23.5% and MONW from 10 to 37% among individuals with a BMI of <25 kg/m2 (44, 55, 56). LPs occur very infrequently, and thus are considered rare diseases.
Do these phenotypes have a different risk in terms of chronic diseases? Overall studies suggest a different degree in the risk of NCDs between the various obesity phenotypes, thus individuals with MUO are at higher risk than those with MUN, and individuals with MUN are at higher risk than those with MHO (53, 55, 57–59).
The cardiometabolic risk associated with MHO is still an open issue (60). A recent population-based prospective cohort study of 381,363 UK Biobank participants with a median follow-up of 11.2 years demonstrated that people with MUN, MHO and MUO were at a substantially higher risk of diabetes, atherosclerotic cardiovascular diseases (ASCVD), heart failure (HF), respiratory diseases and all-cause mortality compared with people with MHN (53). It is worth noting that people with MHO were at an even higher risk of HF and respiratory disease than those with MHN and MUN (53, 55). A multi-national European study found that those with MHO had a higher CVD risk than those with MHN but lower than those with MUN and MUO (56). Among people with baseline MHO who remained affected by obesity, over one-third became metabolically unhealthy within 3-5 years (53, 55, 61). These people acquired an even higher risk of ASCVD (53). Due to the presence of several metabolic alterations, including hyperglycemia and insulin resistance, high blood pressure and hyperlipidemia (46, 59, 62), people with MUN also have a high risk of NCD (53).
Major causes of mortality for LP include heart disease (particularly heart failure and myocardial infarction), liver and kidney failure, and acute pancreatitis (63).
How to Differentiate Between the Phenotypes?
Recent studies highlight an important phenotypical difference between individuals with obesity. While individuals with MHO seem to have higher physical activity levels than those with MUO (64, 65), individuals with MUN (both NWO and MONW) have a poorer MM, in terms of quality and quantity than MHO and MHN (44, 56, 65–68). Furthermore, since they do not manifest the metabolic syndrome (MS) despite a cluster of metabolic and genetic features, individuals with NWO differ from those with MONW, who in contrast, might have MS (69). In addition, individuals with NWO have a normal BMI, while those with MONW may be overweight (69, 70). However, an important common feature is that both are at risk of sarcopenia (46, 70). Figure 1 presents all these characteristics.
Lipodystrophies constitute a rare group of heterogenous genetic or acquired disorders, which are mainly characterized by partial or total loss of adipose tissue, especially in the subcutaneous adipose depots, of individuals with a wide range of BMIs and, as a result of the inability to store energy, with ectopic fat accumulation (63). Limited lipid storage capacity in subcutaneous fat depots results in the near total lack of adipocyte expandability in patients with generalized lipodystrophy, in which subcutaneous fat is absent on the face, arms, legs and buttocks and who also express acromegaloid features (63, 71). In individuals affected by partial lipodystrophy, the inability to store energy in the subcutaneous depots is partial and leads to an increased WHR due to a low ratio of the lower limb to truncal fat or in contrast, in certain forms, an increased subcutaneous fat deposition in the lower extremities (63, 71).
Metabolic Characteristics of the Different Obesity Phenotypes
It has been now recognized that excessive fat mass alone does not increase the risk of T2DM. Various recent studies have shown a link between a low MM, especially appendicular muscle mass (ASMM), and the development of T2DM (72–74), which may partially explain the high risk of CVD and cancer in NWO and MONW phenotypes.
Central adiposity is one of the principal characteristics of MUO, which provides the foundation for the increase in the flow of free fatty acids (FFAs) and the inhibition of insulin release. The large number of FFAs contributes to reducing the glucose up-take by skeletal muscle and stimulates the hepatic production of very low-density lipoproteins (VLDLs) and glucose. FFAs also have a lipotoxic effect in the pancreatic beta cells, leading to the development of T2DM.
In MUO, the risk of T2DM rises more than 10-fold compared to healthy individuals and the CV risk is twice in comparison with MHN (53, 75). In MUO associated with multiple risk factors, the risk of developing the disease is greater than the sum of the risks attributable to each individual factor (73). The association between visceral fat and metabolic and cardiovascular disorders is also related to the accumulation of ectopic fat that accompanies visceral adiposity (76, 77). Furthermore, insulin and inflammation are the main actors in the pathogenesis of muscle loss in those with obesity (78), which mainly occurs in the early stages of the aging process (54). Therefore, in this review, SO is considered as a subtype of the MUO.
If obesity is defined as abnormal fat accumulation that presents a risk to health, individuals with lipodystrophy have a particular obesity phenotype. Individuals with LP are also affected by insulin resistance, severe hypertriglyceridemia, diabetes, and liver steatosis (63, 71, 79, 80). Figure 1 summarizes all these metabolic alterations.
Dietary, Physical Exercise and Pharmacological Treatments Tailored to the Different Obesity Phenotypes
Although it is well accepted that the BMI does not capture the large heterogeneity in the risk of NCDs observed across individuals, only a few studies have tested the effects of lifestyle interventions in relation to the different obesity phenotypes.
Dietary, Physical Exercise, and Pharmacological Treatments in MUN
It is intuitive that a significant weight loss may only be partially practical, or not practical at all, for individuals with MUN. Individuals with MUN (both NWO and MONW) have less lean mass (44, 56, 65–68) than other phenotypes. One study demonstrated that individuals with NWO have similar diet quality scores but lower physical fitness levels than lean individuals and, thus, a higher quality of diet than those who are overweight or suffer from obesity (81). Physical exercise prevents the development of an NWO phenotype (82). A program of resistance exercise combined with a dairy supplement significantly decreases FM and improves the metabolic parameters better than a standard supplement in overweight individuals with low MM (83). A 6-month soy-enriched high protein snack meal in women with NWO increases ASMM and reduces FM and appetite compared to an isocaloric low protein snack (84). Twelve weeks of a high protein diet results in no significant weight loss in women with NWO, but in a significant improvement in LBM and a reduction in FM with respect to a standard protein diet (85). Taken together these studies suggest that, in terms of body composition and metabolic parameters, physical exercise and improvement in MM might be more important than a dietary approach in MUN.
In the MONW phenotype, a high quality diet score, linked to a high consumption of fruit and vegetables, is significantly associated with a reduction in the risk of all-cause mortality (22%; HR, 0.78; 95% CI, 0.68-0.90) and CV mortality (HR 0.79; 95% CI, 0.65-0.97) (86), especially in young adults (87). Healthy dietary patterns alone may thus be effective in reducing the cardiometabolic risk among younger adults with MONW, but in older adults, physical activity might be more important than a dietary approach (88) (Figure 2).
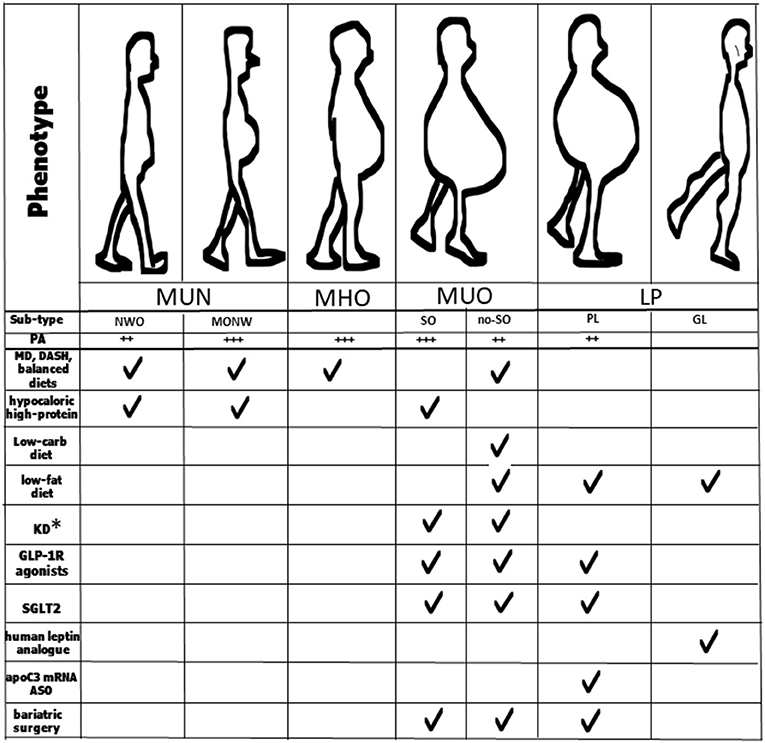
Figure 2. Key strategies for management of the different obesity phenotypes and sub-types. *Patients taking SGLT2 inhibitors should avoid KD; MUN, normal-weight obesity; MHO, metabolically healthy obesity; MUO, metabolically unhealthy obesity; LP, lipodystrophic phenotype; NOW, normal-weight obesity; MONW, metabolic obesity normal-weight; SO, sarcopenic obesity; no-SO, no sarcopenic obesity; GL, generalized lipodystrophy; PL, partial lipodystrophy; MD, Mediterranean diet; DASH, Dietary Approaches to Stop Hypertension; KD, ketogenic diet; GLP-1 R, glucagon-like peptide-1 receptor; ASO, antisense therapeutic oligonucleotide.
Dietary, Physical Exercise, and Pharmacological Treatment in MHO
As discussed above, MHO is a transient phenotype and over one-third of individuals become metabolically unhealthy overtime (53, 58, 89). MHO individuals with an impaired fat oxidation rapidly develop MS/diabetes (90), gain body weight, and suffer co-morbidities (91–94). Skeletal muscle contributes significantly to the overall utilization of fat (95), and after several sessions of vigorous intensity exercise (96, 97) or whole-body electromyostimulation (WB-EMS), fat oxidation significantly improves (98). In MHO, exercise training is necessary to improve fat oxidation (99). Furthermore, several studies have demonstrated that certain dietary bioactive components (such as catechins, capsaicin, and L-carnitine) significantly improve fat oxidation, with a consequent reduction in BMI and FM (100–102).
In one study, following a Mediterranean diet (MD), which is rich in antioxidants, was positively associated with the MHO phenotype, but not in the older age group (87). A five-point increase in the adherence to MD in MHO individuals was associated with a 41% reduction in the risk of all-cause mortality (HR, 0.59; 95% CI, 0.37-0.94) (86) (Figure 2). All these studies seem to indicate that it might be possible to prevent the transition from MHO to MUO through intense physical activity programs as well as through the consumption of foods that contain bioactive molecules that stimulate fat utilization as in the MD, or through nutraceuticals.
Dietary, Physical Exercise, and Pharmacological Treatment in MUO
Although specific features of a diet, such as a low glycemic index, might slightly lower the risks of CVD (103), a dietary approach alone (even an MD), does not reduce mortality in the MUO phenotype (86). A limited body weight reduction (5-20%) has several beneficial metabolic effects on serum glucose and LDL and blood pressure (104), and thus on CVD risk.
A recent meta-analysis, based on quantitative estimates of the relative effect of different diets, demonstrated that both MD and DASH result in slightly less weight loss (~3 vs. ~5 kg, at 6 months) and blood pressure reductions (~3 vs. ~ 6 mmHg) than low-carbohydrate or low-fat dietary patterns (105, 106) (Figure 2). A diet relatively low in carbohydrates may have positive effects on muscle protein turnover and prevent glucose abnormalities, especially in the elderly (107). One variation of a carbohydrate restriction diet is the ketogenic diet (KD) which consists of up to 70% of fat and below 50 g of carbohydrate of the total daily calorie intake. There is evidence that the use of the KD in MUO patients improves insulin sensitivity and glycemic control and is successfully used as part of the treatment for T2DM, obesity and metabolic syndrome (108–110) (Figure 2). From a weight loss perspective and in the short term, a low-carbohydrate diet thus appears to be more effective than other diets in MUO.
However, low-carbohydrate diets result in reduced effects compared to low-fat diets, MD and DASH on LDL cholesterol reduction (105). On the other hand, in several studies LDL-C was found to slightly increase as a consequence of a low-carbohydrate diet (105, 106). Two cohort studies seem to deny any maintainable benefit from a low-carbohydrate diet in the general population (111). Randomized clinical trials and prospective cohorts studies have demonstrated that carbohydrate quality may be relevant for health in the long-term, and a high consumption of whole carbohydrate foods have been shown to reduce NCD incidence (112–114). A meta-analysis demonstrated that there is no difference in changes in HbA1c between low-carbohydrates and balanced weight loss diets at 3–6 months and 1–2 years (115), and glycaemia depends on the degree of weight loss in overweight adults and those with obesity rather than the type of diet (104). A prospective cohort study using data from the US National Health and Nutrition Examination Survey (NHANES) from 1999 to 2014, suggested that total mortality depends on the quality and food sources of macronutrients (116) as already suggested from studies on the glycemic index (103).
Taking together, these studies suggest that, in order to obtain rapid effects on body weight loss and metabolic parameters, a low-carbohydrate diet is useful for a short period, and then patients should switch to a balanced and high carbohydrate (whole grains, fiber) long-term diet to prevent NCDs.
However, most individuals with severe obesity, i.e., those with MUO, often fail to maintain long-term weight loss with the associated metabolic improvement (117), thus pharmacological agents are needed for these individuals. Newer pharmacological treatments have shown promising results in terms of weight loss and the prevention of obesity-related CV complications in MUO.
Liraglutide, a GLP-1(glucagon-like peptide-1) receptor agonist induces and maintains weight loss by promoting satiety and reducing energy intake in patients with obesity affected by T2DM, hypertension and dyslipidemia (Figure 2). Liraglutide is thus now approved for treating obesity at an increased 3.0 mg daily dose (118–120). In a large, multicenter, double blind trial carried out in patients with T2DM and high cardiovascular risk, at a daily dose of 1.8 mg, liraglutide significantly reduced the primary composite outcome, which included death from cardiovascular causes, non-fatal myocardial infarction, or non-fatal stroke, compared to a placebo over 3.8 years (13.0 vs. 14.9%; HR 0.87, 95% CI 0.78-0.97), with fewer CV deaths in the treated group compared with the placebo (hazard ratio, 0.78; 95% CI, 0.66-0.93) (121).
Semaglutide is an oral GLP-1 receptor agonist approved for the treatment of T2DM which reduces HbA1c by ~0.9%, body weight by ~3.0 kg, systolic blood pressure by 3.2 mmHg as well as all-cause and cardiovascular mortality compared with placebo (122). However, it is associated with an increased incidence of adverse gastrointestinal events (122).
SGLT2 inhibitors are a new group of oral medications for treating T2DM which have demonstrated efficacy for weight loss and the reduction of cardiovascular risk in individuals with obesity (123, 124). However, due to the risk of euglycemic diabetic ketoacidosis in the setting of SGLT2 inhibitor use and KD, patients taking SGLT2 inhibitors should be advised to avoid this type of diet (Figure 2).
Fixed-dose combination of Naltrexone-Bupropion became available in 2014 after FDA approval for use as an adjunct to a reduced-calorie diet and increased physical activity in long-term weight management in individuals with obesity (125). Bupropion inhibits the presynaptic reuptake of both dopamine and noradrenaline (key neurotransmitters in the reward pathway associated with addiction) leading to increased levels of both these neurotransmitters in the synaptic cleft. Naltrexone belongs to a group of drugs known as opioid antagonists and regulates satiety. In several studies, Naltrexone-Bupropion demonstrated a placebo-adjusted weight loss of 2.5-5.2% at target doses (104). However, the FDA initially rejected this new drug application for Bupropion because of the rise in blood pressure and heart rate. The cardiovascular safety of this combination remains uncertain (126).
Metabolic/bariatric surgery is the most effective strategy to accomplish a significant (≥30%) and durable (at ≥5 years) weight loss in patients with obesity (104) and is recommended for patients with a BMI ≥ 40 kg/m2 or ≥ 35 kg/m2 with obesity-related comorbidities.
In addition, among patients with T2DM and a BMI of 30 or greater, compared with usual care, metabolic surgery (which included Roux-en-Y gastric bypass, sleeve gastrectomy, adjustable gastric banding, and duodenal switch) was associated with a significantly lower risk of major adverse cardiac events (MACE) and diabetic nephropathy (127). On the other hand, there is only a transient positive effect of abdominal lipectomy in reducing FM and body weight in women, which disappears a few months after the procedure (128, 129).
Regarding sarcopenic obesity, this syndrome is predominantly observed in the aging population which is thus at risk of several complications from both sarcopenia and obesity (130). The most effective lifestyle intervention for treating sarcopenic obesity should include both diet-induced weight loss and regular exercise (aerobic and resistance exercises) (131, 132). In older adults with both obesity and sarcopenia, a 20% reduction in body weight results in a greater reduction in FM than LBM, leading to an increase in MM (133). A hypocaloric high-protein diet (1.2-1.4 g/kg body weight reference/day) or KD preserve LBM compared to a low-calorie diet (134). An ingestion of at least 25 grams of protein per meal ensures an optimal muscle protein synthesis, especially in the elderly (135) (Figure 2).
Dietary and Pharmacological Treatment in LP
There are few studies on specific diets in lipodystrophy. Diets high in fat should be avoided in patients with LP due to the possible development of metabolic sequelae while an energy-restricted diet and the consumption of medium-chain triglyceride oil formulas can improve metabolic abnormalities in these individuals (71). Metreleptin, a synthetic analog of human leptin that binds to and activates the leptin receptor, was first approved by the U.S. Food and Drug Administration (FDA) in 2014 for the treatment of metabolic complications in patients with congenital or acquired generalized lipodystrophies (136). Metreleptin was then approved by the European Medicines Agency (EMA) for adults and children aged ≥ 12 years affected by partial lipodystrophy who are non-responders to standard treatments (137). However, metreleptin is also effective in treating partial lipodystrophies (138). Volanesorsen is a second-generation chimeric antisense therapeutic oligonucleotide (ASO) which selectively reduces apoC3 mRNA, thereby lowering the levels of triglycerides and FM in particular genetic syndromes such as familial partial lipodystrophy (139, 140). It was approved in the European Union in May 2019 (Figure 2).
Implications for Clinical Practice and End Social Stigma
Current knowledge and technologies enable detailed assessments of the body composition of individuals so that their treatments can be tailored. Although these technologies have come down in cost, their availability is limited, especially in peripheral hospitals. Moreover, there is often a scarcity of skilled personnel in this area, which thus preclude phenotyping obesity in routine clinical practice. Getting a DXA in some countries is really challenging and the cost of the scan may be prohibitive (141, 142).
BIA and DXA are currently mostly used in research, rather than in general hospitals (141), and sarcopenia is still a relatively new concept (141). Governments seem more likely to carry on with the old strategies and policies for patients with obesity rather than implementing innovative policies. Consequently, some of the possible approaches outlined in this review may take time to be accepted by decision makers in public health and the impact of this review in clinical practice is thus difficult to predict.
According to the WHO (143), weight bias is defined as a negative attitude toward, and belief about, others because of their weight. Obesity stigma is a result of weight bias and an individual with high body weight is victim of prejudice. Obesity stigma can affect an individual's everyday life. Increasing academic and public education regarding the complex causes of obesity and its phenotypes can help end obesity stigma. This narrative review highlights that the diagnosis of obesity is complex and goes beyond body weight. Even a lean individual could be metabolically obese. It is therefore better not to judge other individuals (or patients) by weight.
Open Questions
In the elderly, a combination of aerobic and resistance exercises appears to be the most effective treatment strategy in helping to decrease body weight and improve MM function and fitness. However, how we can really make a positive impact on their daily behavior is not yet clear.
Further research is also needed to understand the magnitude of the reduction in the burden of NCDs by addressing the different phenotypes of obesity.
Conclusions
Obesity is a clinical condition associated with metabolic derangements resulting in severe comorbidities as well as the risk of the development of NCDs that depend on the phenotype and subtype of obesity. Many complications of obesity are secondary to an excess of adipose mass resulting in ectopic lipid storage in other organs and causing insulin resistance. In fact, insulin resistance leads to diabetes, hypertriglyceridemia, and non-alcoholic fatty liver disease and cardiovascular mortality. Other complications are secondary to the loss of MM, especially of ASMM, as with most endocrine diseases, as well as physical disability and even mortality.
Common dietary approaches are not effective in treating large numbers of patients affected by obesity. These individuals thus need to undergo precise phenotyping using nuclear magnetic resonance, BIA or DXA. In fact, DXA is the reference method for body composition in terms of accuracy, non-invasiveness and cost (144). This approach leads to a tailor-made prevention and treatment strategy in order to reduce their risk of NCDs.
Author Contributions
TM and RP: conceptualization. TM, AP, AL, and AD: supervision and review. RP, MT, and FA: original draft preparation and editing. All authors contributed to the article and approved the submitted version.
Conflict of Interest
The authors declare that the research was conducted in the absence of any commercial or financial relationships that could be construed as a potential conflict of interest.
Publisher's Note
All claims expressed in this article are solely those of the authors and do not necessarily represent those of their affiliated organizations, or those of the publisher, the editors and the reviewers. Any product that may be evaluated in this article, or claim that may be made by its manufacturer, is not guaranteed or endorsed by the publisher.
References
1. NCD Risk Factor Collaboration (NCD-RisC). Worldwide trends in body-mass index, underweight, overweight, and obesity from 1975 to 2016: a pooled analysis of 2416 population-based measurement studies in 128·9 million children, adolescents, and adults. Lancet. (2017) 390:2627–42. doi: 10.1016/S0140-6736(17)32129-3
2. Hales CM, Carroll MD, Fryar CD, Ogden CL. Prevalence of obesity and severe obesity among adults: United States, 2017-2018. NCHS Data Brief. (2020) 360:1–8.
3. Kelly T, Yang W, Chen CS, Reynolds K, He J. Global burden of obesity in 2005 and projections to 2030. Int J Obes. (2008) 32:1431–7. doi: 10.1038/ijo.2008.102
4. GBD 2017 Diet Collaborators. Health effects of dietary risks in 195 countries, 1990-2017: a systematic analysis for the Global Burden of Disease Study 2017. Lancet. (2019) 393:1958–72. doi: 10.1016/S0140-6736(19)30041-8
5. Guh DP, Zhang W, Bansback N, Amarsi Z, Birmingham CL, Anis AH. The incidence of co-morbidities related to obesity and overweight: a systematic review and meta-analysis. BMC Public Health. (2009) 9:88. doi: 10.1186/1471-2458-9-88
6. De Lorenzo A, Tarsitano MG, Falcone C, Di Renzo L, Romano L, Macheda S, et al. Fat mass affects nutritional status of ICU COVID-19 patients. J Transl Med. (2020) 3:299. doi: 10.1186/s12967-020-02464-z
7. Biomarkers Definitions Working Group. Biomarkers and surrogate endpoints: preferred definitions and conceptual framework. Clin Pharmacol Ther. (2001) 69:89–95. doi: 10.1067/mcp.2001.113989
8. Hlatky MA, Greenland P, Arnett DK, Ballantyne CM, Criqui MH, Elkind MS, et al. Criteria for evaluation of novel markers of cardiovascular risk: a scientific statement from the American Heart Association. Circulation. (2009) 119:2408–16. doi: 10.1161/CIRCULATIONAHA.109.192278
9. Burchard EML. Physique and psychosis: an analysis of the postulated relationship between bodily constitution and mental disease syndrome. Comparative Psychol Monogr. (1936) 13:73.
10. Huang Z, Willett WC, Manson JE, Rosner B, Stampfer MJ, Speizer FE, et al. Body weight, weight change, and risk for hypertension in women. Ann Intern Med. (1998) 128:81–8. doi: 10.7326/0003-4819-128-2-199801150-00001
11. Willett WC, Manson JE, Stampfer MJ, Colditz GA, Rosner B, Speizer FE, et al. Weight, weight change, and coronary heart disease in women. Risk within the normal weight range. JAMA. (1995) 273:461–5. doi: 10.1001/jama.273.6.461
12. Curb JD, Marcus EB. Body fat, coronary heart disease, and stroke in Japanese men. Am J Clin Nutr. (1991) 53:1612S−5S. doi: 10.1093/ajcn/53.6.1612S
13. Fraser GE, Strahan TM, Sabaté J, Beeson WL, Kissinger D. Effects of traditional coronary risk factors on rates of incident coronary events in a low-risk population. The Adventist Health Study. Circulation. (1992) 86:406–13. doi: 10.1161/01.CIR.86.2.406
14. Aune D, Sen A, Prasad M, Norat T, Janszky I, Tonstad S, et al. BMI and all cause mortality: systematic review and non-linear dose-response meta-analysis of 230 cohort studies with 3.74 million deaths among 30.3 million participants. BMJ. (2016) 353:i2156. doi: 10.1136/bmj.i2156
15. Teucher B, Rohrmann S, Kaaks R. Obesity: focus on all-cause mortality and cancer. Maturitas. (2010) 65:112–6. doi: 10.1016/j.maturitas.2009.11.018
16. Gilmore J. Body mass index and health. Health Rep. (1999) 11:31–47. doi: 10.1016/S0168-8510(99)00033-0
17. Garrido-Chamorro RP, Sirvent-Belando JE, Gonzalez-Lorenzo M, Martin-Carratala ML, Roche E. Correlation between body mass index and body composition in elite athletes. J Sports Med Phys Fitness. (2009) 49:278–84.
18. Flegal KM, Kit BK, Orpana H, Graubard BI. Association of all-cause mortality with overweight and obesity using standard body mass index categories: a systematic review and meta-analysis. JAMA. (2013) 309:71–82. doi: 10.1001/jama.2012.113905
19. Schulte H, Cullen P, Assmann G. Obesity, mortality and cardiovascular disease in the Münster Heart Study (PROCAM). Atherosclerosis. (1999) 144:199–209. doi: 10.1016/S0021-9150(99)00055-6
20. Vague J. La différenciation sexuelle; facteur déterminant des formes de l'obésité [Sexual differentiation; Factor determining forms of obesity]. Presse Med. (1947) 55:339.
21. Kissebah AH, Vydelingum N, Murray R, Evans DJ, Hartz AJ, Kalkhoff RK, et al. Relation of body fat distribution to metabolic complications of obesity. J Clin Endocrinol Metab. (1982) 54:254–60. doi: 10.1210/jcem-54-2-254
22. Ohlson LO, Larsson B, Svärdsudd K, Welin L, Eriksson H, Wilhelmsen L, et al. The influence of body fat distribution on the incidence of diabetes mellitus. 13.5 years of follow-up of the participants in the study of men born in 1913. Diabetes. (1985) 34:1055–8. doi: 10.2337/diab.34.10.1055
23. Lapidus L, Bengtsson C, Larsson B, Pennert K, Rybo E, Sjöström L. Distribution of adipose tissue and risk of cardiovascular disease and death: a 12 year follow up of participants in the population study of women in Gothenburg, Sweden. Br Med J. (Clin Res Ed.). (1984) 289:1257–61. doi: 10.1136/bmj.289.6454.1257
24. Rexrode KM, Carey VJ, Hennekens CH, Walters EE, Colditz GA, Stampfer MJ, et al. Abdominal adiposity and coronary heart disease in women. JAMA. (1998) 280:1843–8. doi: 10.1001/jama.280.21.1843
25. Barbagallo CM, Cavera G, Sapienza M, Noto D, Cefalù AB, Pagano M, et al. Prevalence of overweight and obesity in a rural southern Italy population and relationships with total and cardiovascular mortality: the Ventimiglia di Sicilia project. Int J Obes Relat Metab Disord. (2001) 25:185–90. doi: 10.1038/sj.ijo.0801321
26. Yusuf S, Hawken S, Ounpuu S, Bautista L, Franzosi MG, Commerford P, et al. Obesity and the risk of myocardial infarction in 27,000 participants from 52 countries: a case-control study. Lancet. (2005) 366:1640–9. doi: 10.1016/S0140-6736(05)67663-5
27. De Simone G, Chinali M. Is central obesity a better discriminator of the risk of hypertension than body mass index in ethnically diverse populations? J Hypertens. (2008) 26:169–81. doi: 10.1097/HJH.0b013e3282f16ad3
28. Zhou JY, Ge H, Zhu MF, Wang LJ, Chen L, et al. Neck circumference as an independent predictive contributor to cardio-metabolic syndrome. Cardiovasc Diabetol. (2013) 12:76. doi: 10.1186/1475-2840-12-76
29. Pischon T, Boeing H, Hoffmann K, Bergmann M, Schulze MB, Overvad K, et al. General and abdominal adiposity and risk of death in Europe. N Engl J Med. (2008) 359:2105–20. doi: 10.1056/NEJMoa0801891
30. Moore LL, Bradlee ML, Singer MR, Splansky GL, Proctor MH, Ellison RC, et al. BMI and waist circumference as predictors of lifetime colon cancer risk in Framingham Study adults. Int J Obes Relat Metab Disord. (2004) 28:559–67. doi: 10.1038/sj.ijo.0802606
31. Schlesinger S, Aleksandrova K, Pischon T, Fedirko V, Jenab M, Trepo E, et al. Abdominal obesity, weight gain during adulthood and risk of liver and biliary tract cancer in a European cohort. Int J Cancer. (2013) 132:645–57. doi: 10.1002/ijc.27645
32. Stolzenberg-Solomon RZ, Adams K, Leitzmann M, Schairer C, Michaud DS, Hollenbeck A, et al. Adiposity, physical activity, and pancreatic cancer in the National Institutes of Health-AARP Diet and Health Cohort. Am J Epidemiol. (2008) 167:586–97. doi: 10.1093/aje/kwm361
33. Deurenberg P, Yap M, van Staveren WA. Body mass index and percent body fat: a meta analysis among different ethnic groups. Int J Obes Relat Metab Disord. (1998) 22:1164–71. doi: 10.1038/sj.ijo.0800741
34. Ponti F, Santoro A, Mercatelli D, Gasperini C, Conte M, Martucci M, et al. Aging and imaging assessment of body composition: from fat to facts. Front Endocrinol. (2020) 10:861. doi: 10.3389/fendo.2019.00861
35. Pouliot MC, Després JP, Lemieux S, Moorjani S, Bouchard C, Tremblay A, et al. Waist circumference and abdominal sagittal diameter: best simple anthropometric indexes of abdominal visceral adipose tissue accumulation and related cardiovascular risk in men and women. Am J Cardiol. (1994) 73:460–8. doi: 10.1016/0002-9149(94)90676-9
36. Naylor S. Biomarkers: current perspectives and future prospects. Expert Rev Mol Diagn. (2003) 3:525–9. doi: 10.1586/14737159.3.5.525
37. Mitchell T, Lehéricy S, Chiu SY, Strafella AP, Stoessl AJ, Vaillancourt DE. Emerging neuroimaging biomarkers across disease stage in Parkinson Disease: a review. JAMA Neurol. (2021) 78:1262–72. doi: 10.1001/jamaneurol.2021.1312
38. Montalcini T, Pujia A, Donini LM, Frittitta L, Galvano F, Natali A, et al. A call to action: now is the time to screen elderly and treat osteosarcopenia, a position paper of the Italian college of academic nutritionists MED/49 (ICAN-49). Nutrients. (2020) 12:2662. doi: 10.3390/nu12092662
39. Padwal R, Leslie WD, Lix LM, Majumdar SR. Relationship Among body fat percentage, body mass index, and all-cause mortality: a cohort study. Ann Intern Med. (2016) 164:532–41. doi: 10.7326/M15-1181
40. Lee DH, Keum N, Hu FB, Orav EJ, Rimm EB, Willett WC, et al. Predicted lean body mass, fat mass, and all cause and cause specific mortality in men: prospective US cohort study. BMJ. (2018) 362:k2575. doi: 10.1136/bmj.k2575
41. Arthur RS, Xue X, Kamensky V, Chlebowski RT, Simon M, Luo J, et al. The association between DXA-derived body fat measures and breast cancer risk among postmenopausal women in the Women's Health Initiative. Cancer Med. (2020) 9:1581–99. doi: 10.1002/cam4.2690
42. Chen GC, Arthur R, Iyengar NM, Kamensky V, Xue X, Wassertheil-Smoller S, et al. Association between regional body fat and cardiovascular disease risk among postmenopausal women with normal body mass index. Eur Heart J. (2019) 40:2849–55. doi: 10.1093/eurheartj/ehz391
43. Kyle UG, Schutz Y, Dupertuis YM, Pichard C: Body composition interpretation. Contributions of the fat-free mass index and the body fat mass index. Nutrition. (2003) 19:597-604. doi: 10.1016/S0899-9007(03)00061-3
44. Cruz-Jentoft AJ, Bahat G, Bauer J, Boirie Y, Bruyère O, Cederholm T, et al. Sarcopenia: revised European consensus on definition and diagnosis. Age Ageing. (2019) 48:16–31. doi: 10.1093/ageing/afz046
45. He Q, Xia B, Liu A, Li M, Zhou Z, Cheung EC, et al. Association of body composition with risk of overall and site-specific cancers: a population-based prospective cohort study. Int J Cancer. (2021) 149:435–1447. doi: 10.1002/ijc.33697
46. De Lorenzo A, Martinoli R, Vaia F, Di Renzo L. Normal weight obese (NWO) women: an evaluation of a candidate new syndrome. Nutr Metab Cardiovasc Dis. (2006) 16:513–23. doi: 10.1016/j.numecd.2005.10.010
47. Srikanthan P, Horwich TB, Tseng CH. Relation of muscle mass and fat mass to cardiovascular disease mortality. Am J Cardiol. (2016) 117:1355–60. doi: 10.1016/j.amjcard.2016.01.033
48. Mijnarends DM, Schols JM, Meijers JM, Tan FE, Verlaan S, Luiking YC, et al. Instruments to assess sarcopenia and physical frailty in older people living in a community (care) setting: similarities and discrepancies. J Am Med Dir Assoc. (2015) 16:301–8. doi: 10.1016/j.jamda.2014.11.011
49. Romero-Corral A, Montori VM, Somers VK, Korinek J, Thomas RJ, Allison TG, et al. Association of bodyweight with total mortality and with cardiovascular events in coronary artery disease: a systematic review of cohort studies. Lancet. (2006) 368:666–78. doi: 10.1016/S0140-6736(06)69251-9
50. Donini LM, Pinto A, Giusti AM, Lenzi A, Poggiogalle E. Obesity or BMI paradox? Beneath the Tip of the Iceberg. Front Nutr. (2020) 7:53. doi: 10.3389/fnut.2020.00053
51. Tian S, Xu Y. Association of sarcopenic obesity with the risk of all-cause mortality: a meta-analysis of prospective cohort studies. Geriatr Gerontol Int. (2016) 16:155–66. doi: 10.1111/ggi.12579
52. Ruderman N, Chisholm D, Pi-Sunyer X, Schneider S. The metabolically obese, normal-weight individual revisited. Diabetes. (1998) 47:699–713. doi: 10.2337/diabetes.47.5.699
53. Zhou Z, Macpherson J, Gray SR, Gill J, Welsh P, Celis-Morales C, et al. Are people with metabolically healthy obesity really healthy? A prospective cohort study of 381,363 UK Biobank participants. Diabetologia. (2021) 64:1963–72. doi: 10.1007/s00125-021-05484-6
54. Poggiogalle E, Lubrano C, Sergi G, Coin A, Gnessi L, Mariani S, et al. Sarcopenic obesity and metabolic syndrome in adult caucasian subjects. J Nutr Health Aging. (2016) 20:958–63. doi: 10.1007/s12603-015-0638-1
55. Lopez-Garcia E, Guallar-Castillon P, Leon-Muñoz L, Rodriguez-Artalejo F. Prevalence and determinants of metabolically healthy obesity in Spain. Atherosclerosis. (2013) 231:152–7. doi: 10.1016/j.atherosclerosis.2013.09.003
56. Badoud F, Perreault M, Zulyniak MA, Mutch DM. Molecular insights into the role of white adipose tissue in metabolically unhealthy normal weight and metabolically healthy obese individuals. FASEB J. (2015) 29:748–58. doi: 10.1096/fj.14-263913
57. Correa-Rodríguez M, González-Ruíz K, Rincón-Pabón D, Izquierdo M, García-Hermoso A, Agostinis-Sobrinho C, et al. Normal-weight obesity is associated with increased cardiometabolic risk in young adults. Nutrients. (2020) 12:1106. doi: 10.3390/nu12041106
58. Kim NH, Kim KJ, Choi J, Kim SG. Metabolically unhealthy individuals, either with obesity or not, have a higher risk of critical coronavirus disease 2019 outcomes than metabolically healthy individuals without obesity. Metabolism. (2021) 30:154894. doi: 10.1016/j.metabol.2021.154894
59. Kapoor N, Lotfaliany M, Sathish T, Thankappan KR, Thomas N, Furler J, et al. Prevalence of normal weight obesity and its associated cardio-metabolic risk factors - Results from the baseline data of the Kerala Diabetes Prevention Program (KDPP). PLoS ONE. (2020) 15:e0237974. doi: 10.1371/journal.pone.0237974
60. Donini LM, Merola G, Poggiogalle E, Lubrano C, Gnessi L, Mariani S, et al. Disability, physical inactivity, and impaired health-related quality of life are not different in metabolically healthy vs. unhealthy obese subjects. Nutrients. (2016) 25:759. doi: 10.3390/nu8120759
61. Mongraw-Chaffin M, Foster MC, Anderson C, Burke GL, Haq N, Kalyani RR, et al. Metabolically healthy obesity, transition to metabolic syndrome, cardiovascular risk. J Am Coll Cardiol. (2018) 71:1857–65. doi: 10.1016/j.jacc.2018.02.055
62. Ding WQ, Yan YK, Zhang MX, Cheng H, Zhao XY, Hou DQ, Mi J. Hypertension outcomes in metabolically unhealthy normal-weight and metabolically healthy obese children and adolescents. J Hum Hypertens. (2015) 29:548–54. doi: 10.1038/jhh.2014.124
63. Garg A. Acquired inherited lipodystrophies. N Engl J Med. (2004) 350:1220–34. doi: 10.1056/NEJMra025261
64. Hinnouho GM, Czernichow S, Dugravot A, Batty GD, Kivimaki M, Singh-Manoux A. Metabolically healthy obesity and risk of mortality: does the definition of metabolic health matter? Diabetes Care. (2013) 36:2294-300. doi: 10.2337/dc12-1654
65. Arnlöv J, Ingelsson E, Sundström J, Lind L. Impact of body mass index and the metabolic syndrome on the risk of cardiovascular disease and death in middle-aged men. Circulation. (2010) 121:230–6. doi: 10.1161/CIRCULATIONAHA.110.960849
66. Batsis JA, Sahakyan KR, Rodriguez-Escudero JP, Bartels SJ, Somers VK, Lopez-Jimenez F. Normal weight obesity and mortality in United States subjects ≥60 years of age (from the Third National Health and Nutrition Examination Survey). Am J Cardiol. (2013) 112:1592–8. doi: 10.1016/j.amjcard.2013.07.014
67. Kim MK, Han K, Kwon HS, Song KH, Yim HW, Lee WC, et al. Normal weight obesity in Korean adults. Clin Endocrinol. (2014) 80:214–20. doi: 10.1111/cen.12162
68. Hayes L, Pearce MS, Firbank MJ, Walker M, Taylor R, Unwin NC. Do obese but metabolically normal women differ in intra-abdominal fat and physical activity levels from those with the expected metabolic abnormalities? A cross-sectional study. BMC Public Health. (2010) 10:723. doi: 10.1186/1471-2458-10-723
69. Phillips CM, Dillon C, Harrington JM, McCarthy VJ, Kearney PM, Fitzgerald AP, et al. Defining metabolically healthy obesity: role of dietary and lifestyle factors. PLoS ONE. (2013) 8:e76188. doi: 10.1371/journal.pone.0076188
70. Wijayatunga NN, Dhurandhar EJ. Normal weight obesity and unaddressed cardiometabolic health risk-a narrative review. Int J Obes. (2021) 45:2141–55. doi: 10.1038/s41366-021-00858-7
71. Brown RJ, Araujo-Vilar D, Cheung PT, Dunger D, Garg A, Jack M, el al. The diagnosis and management of lipodystrophy syndromes: a multi-society practice Guideline. J Clin Endocrinol Metab. (2016) 101:4500–11. doi: 10.1210/jc.2016-2466
72. De Lorenzo A, Soldati L, Sarlo F, Calvani M, Di Lorenzo N, Di Renzo L. New obesity classification criteria as a tool for bariatric surgery indication. World J Gastroenterol. (2016) 22:681–703. doi: 10.3748/wjg.v22.i2.681
73. Conus F, Allison DB, Rabasa-Lhoret R, St-Onge M, St-Pierre DH, Tremblay-Lebeau A, et al. Metabolic and behavioral characteristics of metabolically obese but normal-weight women. J Clin Endocrinol Metab. (2004) 89:5013–20. doi: 10.1210/jc.2004-0265
74. Buscemi C, Ferro Y, Pujia R, Mazza E, Boragina G, Sciacqua A, et al. Sarcopenia and appendicular muscle mass as predictors of impaired fasting glucose/type 2 diabetes in elderly women. Nutrients. (2021) 13:1909. doi: 10.3390/nu13061909
75. Karpe F, Pinnick KE. Biology of upper-body and lower-body adipose tissue–link to whole-body phenotypes. Nat Rev Endocrinol. (2015) 11:90–100. doi: 10.1038/nrendo.2014.185
76. Jung UJ, Choi MS. Obesity and its metabolic complications: the role of adipokines and the relationship between obesity, inflammation, insulin resistance, dyslipidemia and nonalcoholic fatty liver disease. Int J Mol Sci. (2014) 15:6184–223. doi: 10.3390/ijms15046184
77. Després JP, Lemieux I, Bergeron J, Pibarot P, Mathieu P, Larose E, et al. Abdominal obesity and the metabolic syndrome: contribution to global cardiometabolic risk. Arterioscler Thromb Vasc Biol. (2008) 28:1039–49. doi: 10.1161/ATVBAHA.107.159228
78. Levine ME, Crimmins EM. The impact of insulin resistance and inflammation on the association between sarcopenic obesity and physical functioning. Obesity. (2012) 20:2101–6. doi: 10.1038/oby.2012.20
79. Semple RK, Savage DB, Cochran EK, Gorden P, O'Rahilly S. Genetic syndromes of severe insulin resistance. Endocr Rev. (2011) 32:498–514. doi: 10.1210/er.2010-0020
80. Chan JL, Oral EA. Clinical classification and treatment of congenital and acquired lipodystrophy. Endocr Pract. (2010) 16:310–23. doi: 10.4158/EP09154.RA
81. Bellissimo MP, Bettermann EL, Tran PH, Crain BH, Ferranti EP, Binongo JN, et al. Physical fitness but not diet quality distinguishes lean and normal weight obese adults. J Acad Nutr Diet. (2020) 120:1963–73.e2. doi: 10.1016/j.jand.2020.07.020
82. Maitiniyazi G, Chen Y, Qiu YY, Xie ZX, He JY, Xia SF. Characteristics of body composition and lifestyle in Chinese university students with normal-weight obesity: a cross-sectional study. Diabetes Metab Syndr Obes. (2021) 14:3427–36. doi: 10.2147/DMSO.S325115
83. Maltais ML, Perreault K, Courchesne-Loyer A, Lagacé JC, Barsalani R, Dionne IJ. Effect of resistance training and various sources of protein supplementation on body fat mass and metabolic profile in sarcopenic overweight older adult men: a pilot study. Int J Sport Nutr Exerc Metab. (2016) 26:71–7. doi: 10.1123/ijsnem.2015-0160
84. Haghighat N, Ashtary-Larky D, Bagheri R, Wong A, Cheraghloo N, Moradpour G, et al. Effects of 6 months of soy-enriched high protein compared to eucaloric low protein snack replacement on appetite, dietary intake, and body composition in normal-weight obese women: a randomized controlled trial. Nutrients. (2021) 13:2266. doi: 10.3390/nu13072266
85. Haghighat N, Ashtary-Larky D, Bagheri R, Mahmoodi M, Rajaei M, Alipour M, et al. The effect of 12 weeks of euenergetic high-protein diet in regulating appetite and body composition of women with normal-weight obesity: a randomised controlled trial. Br J Nutr. (2020) 124:1044–51. doi: 10.1017/S0007114520002019
86. Park YM, Fung TT, Steck SE, Zhang J, Hazlett LJ, Han K, et al. Diet quality and mortality risk in metabolically obese normal-weight adults. Mayo Clin Proc. (2016) 91:1372–83. doi: 10.1016/j.mayocp.2016.06.022
87. Park YM, Steck SE, Fung TT, Zhang J, Hazlett LJ, Han K, et al. Mediterranean diet. Dietary Approaches to Stop Hypertension (DASH) style diet, and metabolic health in US adults. Clin Nutr. (2017) 36:1301–9. doi: 10.1016/j.clnu.2016.08.018
88. Lee SW, Youm Y, Lee WJ, Choi W, Chu SH, Park YR, et al. Appendicular skeletal muscle mass and insulin resistance in an elderly korean population: the Korean social life, health and aging project-health examination cohort. Diabetes Metab J. (2015) 39:37–45. doi: 10.4093/dmj.2015.39.1.37
89. Pujia A, Gazzaruso C, Ferro Y, Mazza E, Maurotti S, Russo C, et al. Individuals with metabolically healthy overweight/obesity have higher fat utilization than metabolically unhealthy individuals. Nutrients. (2016) 8:2. doi: 10.3390/nu8010002
90. Pujia A, Mazza E, Ferro Y, Gazzaruso C, Coppola A, Doldo P, et al. Lipid oxidation assessed by indirect calorimetry predicts metabolic syndrome and type 2 diabetes. Front Endocrinol. (2019) 9:806. doi: 10.3389/fendo.2018.00806
91. Zurlo F, Lillioja S, Esposito-Del Puente A, Nyomba BL, Raz I, Saad MF, et al. Low ratio of fat to carbohydrate oxidation as predictor of weight gain: study of 24-h RQ. Am J Physiol. (1990) 259:E650–7. doi: 10.1152/ajpendo.1990.259.5.E650
92. Montalcini T, Lamprinoudi T, Morrone A, Mazza E, Gazzaruso C, Romeo S, et al. Nutrients utilization in obese individuals with and without hypertriglyceridemia. Nutrients. (2014) 6:790–8. doi: 10.3390/nu6020790
93. Montalcini T, Lamprinoudi T, Gorgone G, Ferro Y, Romeo S, Pujia A. Subclinical cardiovascular damage and fat utilization in overweight/obese individuals receiving the same dietary and pharmacological interventions. Nutrients. (2014) 6:5560–71. doi: 10.3390/nu6125560
94. Ferro Y, Gazzaruso C, Coppola A, Romeo S, Migliaccio V, Giustina A, et al. Fat utilization and arterial hypertension in overweight/obese subjects. J Transl Med. (2013) 11:159. doi: 10.1186/1479-5876-11-159
95. Dériaz O, Dumont M, Bergeron N, Després JP, Brochu M, Prud'homme D. Skeletal muscle low attenuation area and maximal fat oxidation rate during submaximal exercise in male obese individuals. Int J Obes Relat Metab Disord. (2001) 25:1579–84. doi: 10.1038/sj.ijo.0801809
96. Talanian JL, Galloway SD, Heigenhauser GJ, Bonen A, Spriet LL. Two weeks of high-intensity aerobic interval training increases the capacity for fat oxidation during exercise in women. J Appl Physiol. (2007) 102:1439–47. doi: 10.1152/japplphysiol.01098.2006
97. Perry CG, Heigenhauser GJ, Bonen A, Spriet LL. High-intensity aerobic interval training increases fat and carbohydrate metabolic capacities in human skeletal muscle. Appl Physiol Nutr Metab. (2008) 33:1112–23. doi: 10.1139/H08-097
98. Amaro-Gahete FJ, De-la-O A, Jurado-Fasoli L, Sanchez-Delgado G, Ruiz JR, Castillo MJ. Metabolic rate in sedentary adults, following different exercise training interventions: The FIT-AGEING randomized controlled trial. Clin Nutr. (2020) 39:3230–40. doi: 10.1016/j.clnu.2020.02.001
99. Ryan AS, Ortmeyer HK. Insulin suppression of fatty acid skeletal muscle enzyme activity in postmenopausal women, and improvements in metabolic flexibility and lipoprotein lipase with aerobic exercise and weight loss. Int J Obes. (2019) 43:276–84. doi: 10.1038/s41366-018-0068-3
100. Rondanelli M, Opizzi A, Perna S, Faliva M, Solerte SB, Fioravanti M, et al. Improvement in insulin resistance and favourable changes in plasma inflammatory adipokines after weight loss associated with two months' consumption of a combination of bioactive food ingredients in overweight subjects. Endocrine. (2013) 44:391–401. doi: 10.1007/s12020-012-9863-0
101. Kapoor MP, Sugita M, Fukuzawa Y, Okubo T. Physiological effects of epigallocatechin-3-gallate (EGCG) on energy expenditure for prospective fat oxidation in humans: a systematic review and meta-analysis. J Nutr Biochem. (2017) 43:1–10. doi: 10.1016/j.jnutbio.2016.10.013
102. Rondanelli M, Riva A, Petrangolini G, Allegrini P, Perna S, Faliva MA, et al. Effect of acute and chronic dietary supplementation with green tea catechins on resting metabolic rate, energy expenditure and respiratory quotient: a systematic review. Nutrients. (2021) 13:644. doi: 10.3390/nu13020644
103. Jenkins DJA, Dehghan M, Mente A, Bangdiwala SI, Rangarajan S, Srichaikul K, et al. Glycemic Index, Glycemic Load, and Cardiovascular Disease and Mortality. N Engl J Med. (2021) 384:1312–22. doi: 10.1056/NEJMoa2007123
104. Heffron SP, Parham JS, Pendse J, Alemán JO. Treatment of obesity in mitigating metabolic risk. Circ Res. (2020) 126:1646–65. doi: 10.1161/CIRCRESAHA.119.315897
105. Ge L, Sadeghirad B, Ball G, da Costa BR, Hitchcock CL, Svendrovski A, et al. Comparison of dietary macronutrient patterns of 14 popular named dietary programmes for weight and cardiovascular risk factor reduction in adults: systematic review and network meta-analysis of randomised trials. BMJ. (2020) 369:m696. doi: 10.1136/bmj.m696
106. Dong T, Guo M, Zhang P, Sun G, Chen B. The effects of low-carbohydrate diets on cardiovascular risk factors: a meta-analysis. PLoS ONE. (2020) 15:e0225348. doi: 10.1371/journal.pone.0225348
107. Volpi E, Mittendorfer B, Rasmussen BB, Wolfe RR. The response of muscle protein anabolism to combined hyperaminoacidemia and glucose-induced hyperinsulinemia is impaired in the elderly. J Clin Endocrinol Metab. (2000) 85:4481–90. doi: 10.1210/jc.85.12.4481
108. Volek JS, Phinney S, Forsythe CE, Quann EE, Wood RJ, Puglisi MJ, et al. Carbohydrate restriction has a more favorable impact on the metabolic syndrome than a low fat diet. Lipids. (2008) 44:297–309. doi: 10.1007/s11745-008-3274-2
109. Goday A, Bellido D, Sajoux I, Crujeiras AB, Burguera B, García-Luna PP, et al. Short-term safety, tolerability and efficacy of a very low-calorie-ketogenic diet interventional weight loss program versus hypocaloric diet in patients with type 2 diabetes mellitus. Nutr Diabetes. (2016) 6:e230. doi: 10.1038/nutd.2016.36
110. Romano L, Marchetti M, Gualtieri P, Di Renzo L, Belcastro M, De Santis, et al. Effects of a personalized VLCKD on body composition and resting energy expenditure in the reversal of diabetes to prevent complications. Nutrients. (2019) 11:1526. doi: 10.3390/nu11071526
111. Fung TT, van Dam RM, Hankinson SE, Stampfer M, Willett WC, Hu FB. Low-carbohydrate diets and all-cause and cause-specific mortality: two cohort studies. Ann Intern Med. (2010) 153:289–98. doi: 10.7326/0003-4819-153-5-201009070-00003
112. Aune D, Keum N, Giovannucci E, Fadnes LT, Boffetta P, Greenwood DC, et al. Whole grain consumption and risk of cardiovascular disease, cancer, and all cause and cause specific mortality: systematic review and dose-response meta-analysis of prospective studies. BMJ. (2016) 353:i2716. doi: 10.1136/bmj.i2716
113. Hebden L, O'Leary F, Rangan A, Singgih Lie E, Hirani V, Allman-Farinelli M. Fruit consumption and adiposity status in adults: a systematic review of current evidence. Crit Rev Food Sci Nutr. (2017) 57:2526–40 doi: 10.1080/10408398.2015.1012290
114. Zhan J, Liu YJ, Cai LB, Xu FR, Xie T, He QQ. Fruit and vegetable consumption and risk of cardiovascular disease: A meta-analysis of prospective cohort studies. Crit Rev Food Sci Nutr. (2017) 57:1650–63. doi: 10.1080/10408398.2015.1008980
115. Naude CE, Schoonees A, Senekal M, Young T, Garner P, Volmink J. Low carbohydrate versus isoenergetic balanced diets for reducing weight and cardiovascular risk: a systematic review and meta-analysis. PLoS ONE. (2014) 9:e100652. doi: 10.1371/journal.pone.0100652
116. Shan Z, Guo Y, Hu FB, Liu L, Qi Q. Association of low-carbohydrate and low-fat diets with mortality among US adults. JAMA Internal Med. (2020) 180:513–23. doi: 10.1001/jamainternmed.2019.6980
117. Unick JL, Beavers D, Bond DS, Clark JM, Jakicic JM, Kitabchi AE, et al. The long-term effectiveness of a lifestyle intervention in severely obese individuals. Am J Med. (2013) 126:236-42. doi: 10.1016/j.amjmed.2012.10.010
118. Davies MJ, Bergenstal R, Bode B, Kushner RF, Lewin A, Skjøth TV, et al. Efficacy of liraglutide for weight loss among patients with type 2 diabetes: the SCALE diabetes randomized clinical trial. JAMA. (2015) 314:687–99. doi: 10.1001/jama.2015.9676
119. Pi-Sunyer X, Astrup A, Fujioka K, Greenway F, Halpern A, Krempf M, et al. A randomized, controlled trial of 3.0 mg of liraglutide in weight management. N Engl J Med. 373:11–22. doi: 10.1056/NEJMoa1411892
120. Wadden TA, Hollander P, Klein S, Niswender K, Woo V, Hale PM, et al. Weight maintenance and additional weight loss with liraglutide after low-calorie-diet-induced weight loss: the SCALE Maintenance randomized study. Int J Obes. (2013) 37:1443–51. doi: 10.1038/ijo.2013.120
121. Marso SP, Daniels GH, Brown-Frandsen K, Kristensen P, Mann JF, Nauck MA, et al. Liraglutide and cardiovascular outcomes in type 2 diabetes. N Engl J Med. 375:311-22. doi: 10.1056/NEJMoa1603827
122. Avgerinos I, Michailidis T, Liakos A, Karagiannis T, Matthews DR, Tsapas A, et al. Oral semaglutide for type 2 diabetes: a systematic review and meta-analysis. Diabetes Obes Metab. (2020) 22:335–45. doi: 10.1111/dom.13899
123. Zelniker TA, Wiviott SD, Raz I, Im K, Goodrich EL, Bonaca MP, et al. SGLT2 inhibitors for primary and secondary prevention of cardiovascular and renal outcomes in type 2 diabetes: a systematic review and meta-analysis of cardiovascular outcome trials. Lancet. (2019) 393:31–9. doi: 10.1016/S0140-6736(18)32590-X
124. Lee PC, Ganguly S, Goh SY. Weight loss associated with sodium-glucose cotransporter-2 inhibition: a review of evidence and underlying mechanisms. Obes Rev. (2018) 19:1630–41. doi: 10.1111/obr.12755
125. Center for Drug Evaluation and Research, US Food and Drug Administration. Summary Review of Application Number 2000063Orig1s000. Available online at: http://www.accessdata.fda.gov/drugsatfda_docs/nda/2014/200063Orig1s000SumR.pdf (accessed February 10, 2016).
126. Nissen SE, Wolski KE, Prcela L, Wadden T, Buse JB, Bakris G, et al. Effect of naltrexone-bupropion on major adverse cardiovascular events in overweight and obese patients with cardiovascular risk factors: a randomized clinical trial. JAMA. (2016) 315:990–1004. doi: 10.1001/jama.2016.1558
127. Aminian A, Zajichek A, Arterburn DE, Wolski KE, Brethauer SA, Schauer PR, et al. Association of metabolic surgery with major adverse cardiovascular outcomes in patients with type 2 diabetes and obesity. JAMA. (2019) 322:1271–82. doi: 10.1001/jama.2019.14231
128. Seretis K, Goulis DG, Koliakos G, Demiri E. Short- and long-term effects of abdominal lipectomy on weight and fat mass in females: a systematic review. Obes Surg. (2015) 25:1950–8. doi: 10.1007/s11695-015-1797-1
129. Merra G, Gualtieri P, Cioccoloni G, Falco S, Bigioni G, Tarsitano MG, et al. FTO rs9939609 influence on adipose tissue localization in the Italian population. Eur Rev Med Pharmacol Sci. (2020) 24:3223–35. doi: 10.26355/eurrev_202003_20689
130. Donini LM, Busetto L, Bauer JM, Bischoff S, Boirie Y, Cederholm T, et al. Critical appraisal of definitions and diagnostic criteria for sarcopenic obesity based on a systematic review. Clin Nutr. (2020) 39:2368–88. doi: 10.1016/j.clnu.2019.11.024
131. Guo SS, Zeller C, Chumlea WC, Siervogel RM. Aging, body composition, and lifestyle: the Fels Longitudinal Study. Am J Clin Nutr. (1999) 70:405–11. doi: 10.1093/ajcn/70.3.405
132. Bouchonville MF, Villareal DT. Sarcopenic obesity: how do we treat it?. Curr Opin Endocrinol Diabetes Obes. (2013) 20:412–9. doi: 10.1097/01.med.0000433071.11466.7f
133. Batsis JA, Villareal DT. Sarcopenic obesity in older adults: aetiology, epidemiology and treatment strategies. Nat Rev Endocrinol. (2018) 14:513–37. doi: 10.1038/s41574-018-0062-9
134. Sammarco R, Marra M, Di Guglielmo ML, Naccarato M, Contaldo F, Poggiogalle E, et al. Evaluation of hypocaloric diet with protein supplementation in middle-aged sarcopenic obese women: a pilot study. Obes Facts. (2017) 10:160–7. doi: 10.1159/000468153
135. Cuthbertson DJ, Babraj J, Smith K, Wilkes E, Fedele MJ, Esser K, et al. Anabolic signaling and protein synthesis in human skeletal muscle after dynamic shortening or lengthening exercise. Am J Physiol Endocrinol Metab. (2006) 290:E731–8. doi: 10.1152/ajpendo.00415.2005
136. Aegerion Pharmaceuticals Inc. MYALEPT (Metreleptin): US Prescribing Information. Cambridge: Aegerion Pharmaceuticals Inc (2015).
137. Aegerion Pharmaceuticals Ltd. Myalepta (Metreleptin) 3, 5.8 and 11.3 Mg Powder for Solution for Injection: EU Summary of Product Characteristics. Windsor: Aegerion Pharmaceuticals Ltd (2018).
138. Oral EA, Gorden P, Cochran E, Araújo-Vilar D, Savage DB, Long A, et al. Long-term effectiveness and safety of metreleptin in the treatment of patients with partial lipodystrophy. Endocrine. (2019) 64:500–11. doi: 10.1007/s12020-019-01862-8
139. Aslesh T, Yokota T. Development of antisense oligonucleotide gapmers for the treatment of dyslipidemia and lipodystrophy. Methods Mol Biol. (2020) 2176:69–85. doi: 10.1007/978-1-0716-0771-8_5
140. Digenio A, Dunbar RL, Alexander VJ, Hompesch M, Morrow L, Lee RG, et al. Antisense-mediated lowering of plasma apolipoprotein C-III by volanesorsen improves dyslipidemia and insulin sensitivity in type 2 diabetes. Diabetes Care. (2016) 39:1408–15. doi: 10.2337/dc16-0126
141. De Lorenzo A, Romano L, Di Renzo L, Di Lorenzo N, Cenname G, Gualtieri P. Obesity: a preventable, treatable, but relapsing disease. Nutrition. (2020) 71:110615. doi: 10.1016/j.nut.2019.110615
142. Treviño-Aguirre E, López-Teros T, Gutiérrez-Robledo L, Vandewoude M, Pérez-Zepeda M. Availability and use of dual energy X-ray absorptiometry (DXA) and bio-impedance analysis (BIA) for the evaluation of sarcopenia by Belgian and Latin American geriatricians. J Cachexia Sarcopenia Muscle. (2014) 5:79–81. doi: 10.1007/s13539-013-0126-6
143. Weight Bias and Obesity Stigma: Considerations for the WHO European Region. (2017). Available online at: http://www.euro.who.int/en/health-topics/noncommunicable-diseases/obesity/ (accessed December 5, 2017).
Keywords: obesity, obesity phenotypes, sarcopenia, body composition, fat mass, muscle mass, dietary treatment
Citation: Pujia R, Tarsitano MG, Arturi F, De Lorenzo A, Lenzi A, Pujia A and Montalcini T (2022) Advances in Phenotyping Obesity and in Its Dietary and Pharmacological Treatment: A Narrative Review. Front. Nutr. 9:804719. doi: 10.3389/fnut.2022.804719
Received: 29 October 2021; Accepted: 21 January 2022;
Published: 15 February 2022.
Edited by:
Caterina Conte, Università telematica San Raffaele, ItalyReviewed by:
Justyna Godos, University of Catania, ItalyLuisa Lampignano, National Institute of Gastroenterology S. de Bellis Research Hospital (IRCCS), Italy
Copyright © 2022 Pujia, Tarsitano, Arturi, De Lorenzo, Lenzi, Pujia and Montalcini. This is an open-access article distributed under the terms of the Creative Commons Attribution License (CC BY). The use, distribution or reproduction in other forums is permitted, provided the original author(s) and the copyright owner(s) are credited and that the original publication in this journal is cited, in accordance with accepted academic practice. No use, distribution or reproduction is permitted which does not comply with these terms.
*Correspondence: Tiziana Montalcini, dG1vbnRhbGNpbmlAdW5pY3ouaXQ=