- 1Nutrition and Clinical Trials Unit, GENYAL Platform, IMDEA-Food Institute, CEI UAM + CSIC, Madrid, Spain
- 2Faculty of Health Sciences, Valencian International University (VIU), Valencia, Spain
- 3Departamento de Ciencias Farmaceúticas y de la Salud, Facultad de Farmacia, Universidad San Pablo-CEU, CEU Universities, Madrid, Spain
- 4Nutritional Genomics and Health Unit, GENYAL Platform, IMDEA-Food Institute, CEI UAM + CSIC, Madrid, Spain
- 5GenyalLab, GENYAL Platform, IMDEA-Food Institute, CEI UAM + CSIC, Madrid, Spain
- 6Biostatistics and Bioinformatics Unit, IMDEA-Food Institute, CEI UAM + CSIC, Madrid, Spain
- 7Precision Nutrition and Cardiometabolic Health, IMDEA-Food Institute, CEI UAM + CSIC, Madrid, Spain
- 8IdisNA, Navarra Institute for Health Research, Pamplona, Spain
- 9Center of Biomedical Research in Physiopathology of Obesity and Nutrition, Institute of Health Carlos III, Madrid, Spain
- 10Molecular Oncology and Nutritional Genomics of Cancer, IMDEA-Food Institute, CEI UAM + CSIC, Madrid, Spain
- 11Production and Development of Foods for Health, IMDEA-Food Institute, CEI UAM + CSIC, Madrid, Spain
- 12Department of Production and Characterization of Novel Foods, Institute of Food Science Research (CIAL), CEI UAM+CSIC, Madrid, Spain
- 13Departamento de Nutrición y Ciencia de los Alimentos, Facultad de Farmacia, Universidad Complutense de Madrid, Grupo de Investigación VALORNUT-UCM, Madrid, Spain
Objective: This article describes the methodology and summarizes some preliminary results of the GENYAL study aiming to design and validate a predictive model, considering both environmental and genetic factors, that identifies children who would benefit most from actions aimed at reducing the risk of obesity and its complications.
Design: The study is a cluster randomized clinical trial with 5-year follow-up. The initial evaluation was carried out in 2017. The schools were randomly split into intervention (nutritional education) and control schools. Anthropometric measurements, social and health as well as dietary and physical activity data of schoolchildren and their families are annually collected. A total of 26 single nucleotide polymorphisms (SNPs) were assessed. Machine Learning models are being designed to predict obesity phenotypes after the 5-year follow-up.
Settings: Six schools in Madrid.
Participants: A total of 221 schoolchildren (6–8 years old).
Results: Collected results show that the prevalence of excess weight was 19.0, 25.4, and 32.2% (according to World Health Organization, International Obesity Task Force and Orbegozo Foundation criteria, respectively). Associations between the nutritional state of children with mother BMI [β = 0.21 (0.13–0.3), p (adjusted) <0.001], geographical location of the school [OR = 2.74 (1.24–6.22), p (adjusted) = 0.06], dairy servings per day [OR = 0.48 (0.29–0.75), p (adjusted) = 0.05] and 8 SNPs [rs1260326, rs780094, rs10913469, rs328, rs7647305, rs3101336, rs2568958, rs925946; p (not adjusted) <0.05] were found.
Conclusions: These baseline data support the evidence that environmental and genetic factors play a role in the development of childhood obesity. After 5-year follow-up, the GENYAL study pretends to validate the predictive model as a new strategy to fight against obesity.
Clinical Trial Registration: This study has been registered in ClinicalTrials.gov with the identifier NCT03419520, https://clinicaltrials.gov/ct2/show/NCT03419520.
Introduction
Obesity is a complex, chronic and multifactorial disease, originated as an interaction between genetic and environmental factors (1). The prevalence of overweight and obese children is rising every year. Specifically, in compliance with the WHO, the number of overweight and obese children aged 0–5 years increased from 32 million globally in 1990 to 41 million in 2016. And it is expected to increase to 70 million by 2025 if these trends continue (2). The situation in Spain is also alarming, with a prevalence of 23.2% of overweight (22.4% boys and 23.9% girls), and 18.1% of obesity (20.4% boys and 15.8% girls) according to data from the ALADINO study carried out by the Spanish Agency for Consumer Affairs, Food Safety and Nutrition (3).
Childhood obesity usually leads to adulthood obesity, which increases the risk of developing certain diseases, such as hypertension, type 2 diabetes and cardiovascular diseases, prematurely (4–6). This early age has been identified as a key point for the implementation of healthy dietary and lifestyle patterns. Thus, the home and schools provide a useful environment to develop educational and lifestyle interventions for school-age children (7).
There is no doubt about the multifactorial etiology of obesity in which socio-cultural, dietetic, environmental and genetic factors are involved (8–11). However, current knowledge is still insufficient to determine the relative importance of these different factors, having a complex network of associations between them (12). In this regard, machine learning techniques represent a powerful prediction tool through their great ability to big data analysis. Thus, Machine learning represents a tool based on a set of algorithms that can characterize, adapt, learn, predict, and analyze data, increasing the knowledge of obesity and offering possibilities of predicting the disease with unprecedented precision. These techniques have been proposed as a potential tool to predict a future excess of body weight and its comorbidities. There are several predictive machine learning algorithms such as neural networks, decision tree analysis or random forest. Each of them should be used according to the purpose and nature of the study variables (13).
Considering all the above-mentioned aspects, the main objective of the GENYAL study is to design and validate a machine learning-based predictive model that identifies children who would benefit most from actions aimed at reducing the risk of obesity and its complications, considering both environmental and genetic factors, and applicable at the beginning of the school stage. The nutritional education developed in the intervention's schools will be also evaluated as part of the predictive model. This article describes the methods and analyses that will be applied. In addition, it summarizes some preliminary results obtained after the first year of the data collection.
Methods
Type of Study and Duration
The present study is a cluster randomized clinical trial with 5-year follow-up intervention based on nutritional education, annual anthropometric measurement evaluations and data collection from questionnaires. Saliva samples were collected for all the schoolchildren in the initial evaluation (2017) in order to obtain genetic information. The final evaluation will be carried out 4 years after the initial intervention, which corresponds to the end of the primary school (Figure 1). The study is therefore expected to last 5 years, from 2016 to 2017 academic year to 2021–2022. Table 1 provides a schedule of activities and interventions throughout the study.
Recruitment, Sample Size, and Sample Characteristics
Due to the nature of the study as a clinical trial, the large number of variables necessary for the design of the preventive model with machine learning (each of them of a very different nature), and the duration of 5 years, a statistically robust sample size could not be implemented. Furthermore, the Consejería de Educación e Investigación de la Comunidad de Madrid was responsible for the selection of six representative schools of the Autonomous Community of Madrid (ACM) (Spain) (two in the north, two in the center and two in the city's south zone), considering the number of students per center and the average socioeconomic level of the districts and neighborhoods. Therefore, the selection was representative of the average income of the ACM households (14). All the School Boards approved the participation in the study and included a total of 569 potential children participants from different districts of Madrid: Chamberí, Hortaleza, Carabanchel, Puente de Vallecas, and Moncloa-Aravaca.
Inclusion and Exclusion Criteria
The inclusion criteria to participate in the study were: being in 1st or 2nd grade of primary school and having an informed consent signed by at least one of the parents.
Exclusion criteria were not attending school during the evaluation days or having planned not to stay at the school the following years.
Randomization
In order to avoid cross-contamination between intervention and control subjects, randomization was carried out by school center instead of individually. Thus, participating schools were randomly and proportionally stratified into two groups: intervention schools and control schools, considering the number of participants per center, their geographic area and their socioeconomic status.
The randomization procedure was carried out with the statistical software R version 3.4 (www.r-project.org).
Ethical Aspects and Data Processing
Protocols and methodology used in the present study comply with the ethical principles for research involving human subjects laid down in the Declaration of Helsinki (1964) and its modifications. The study was approved by the Research Ethics Committee of the IMDEA Food Foundation (PI:IM024; Approval date: March 29th, 2016) and it has been registered in ClinicalTrials.gov with the identifier NCT03419520. School centers and families were informed in detail about the different stages of the project both, orally and in writing. Signed informed consent from at least one of the parents were collected by the researchers prior to the first evaluation. This document included a specific consent to DNA extraction and the evaluation of polymorphisms from the saliva samples. In addition, it included a section on the storage of the remaining samples as a collection registered, according to Spanish legislation (Royal Decree 1716/2011, of November 18th).
Data compiled along the study are going to be processed using a web application that applies dissociation criteria making the volunteers' data anonymous, in compliance with the current Spanish legislation (Organic Law 15/1999 of December 13th, on the protection of Personal Data) and may be used for scientific purpose as publications and conferences. Only the researchers directly related to the study will be allowed to access data.
Selection of Single Nucleotide Polymorphisms
A total of 26 single nucleotide polymorphisms (SNPs) associated with a higher risk of early-age onset of obesity and its comorbidities were selected. The selection was made considering the biological activity of each SNP, Caucasian allele frequencies and the scientific evidence that supports the association between the presence of the polymorphism and the risk of developing overweight, obesity or its complications. The sum of the risk alleles will further be used to design a genetic risk score.
Different databases such as 1,000 Genomes, HapMap, Pubmed, GWAS Central, GWAS Catalog or Ensembl were used. Table 2 shows the selected 26 SNPs, which will be included in the predictive model.
Questionnaires
Different questionnaires were designed based on other surveys used in similar studies to facilitate the comparison of the results. All of them are annually sent to families by email or in the paper format according to the parents' preference and are filled by at least one of the parents. The information collected is summarized in Table 3.
Regarding social, health and demographic data, parents annually complete a self-reported questionnaire that includes different personal questions based on the surveys used in the ALADINO and ELOIN studies (3, 112).
Dietary information is gathered using a 48-h food record of 2 non-consecutive days, a weekday and a weekend day, as recommended by the European Food Safety Authority guidelines (113). Afterwards, the data are tabulated and analyzed using the DIAL software (Alce Ingeniería, Madrid, Spain) (114) in order to obtain information about macro and micronutrients.
Moreover, the adherence to the Mediterranean diet pattern is assessed using the “KIDMED Mediterranean Diet Quality Index” in addition to general questions about the dietary habits of the children and their parents. The KIDMED questionnaire consists of a total of 16 dichotomous questions that must be answered affirmatively or negatively to obtain a score (115).
Physical activity and free time data about the children and their parents are gathered using a questionnaire with different sections adapted and modified from the ALADINO (3) and the ELOIN (112) studies. In addition, a 48-h physical activity record is collected, corresponding to 24 h of a weekday and a complete weekend day (116). In the physical activity record, parents had to specify the time that their children spent during 24 h of a week day and 24 h of a weekend day doing different activities, including resting hours and activities with a variable level of intensity (very light, light, moderate and intense). The time spent doing each activity is multiplied by the corresponding activity coefficient defined by the WHO (117), added and divided by 24, obtaining the Individual Physical Activity Coefficient (IPAC). Then, the IPAC corresponding to a weekday is multiplied by 5 and the weekend IPAC by 2, both results are added and divided by 7, thus, obtaining the median physical activity per individual. Afterwards, it is necessary to convert the IPAC into a Physical Activity Coefficient (PAC) according to sex, therefore an equivalence is made between the IPAC and the PAC proposed by the Institute of Medicine (118). Finally, participants are classified into sedentary, lightly active, active and very active in line with their PAC.
All these data and information are collected every year on equal terms.
Anthropometric and Blood Pressure Measurements
These data are collected in the school centers, early in the morning, by previously trained nutritionists, following standardized protocols and WHO international instructions for this age group (117). For the anthropometric measurements, children had to wear a T-shirt and gym shorts. All measures are taken twice, and the average is used for the analyses.
Height is determined using a Leicester height rod with an accuracy of 1 mm (Biological Medical Technology SL, Barcelona, Spain). Body weight and fat mass percentage are assessed using a BF511 Body Composition Monitor (BF511- OMRON HEALTHCARE UK, LT, Kyoto, Japan). Furthermore, fat mass percentage is classified according to the tables offered by OMROM Healthcare (119). Waist and brachial circumferences measurements are taken using a non-elastic tape (KaWe Kirchner & Wilhelm GmbH, Asperg, Germany; range 0–150 cm, 1 mm of precision). The waist circumference measurements obtained are classified by percentiles in compliance with Fernández et al. (120). Triceps skinfolds are taken following the International Society for the Advancement of Kinanthropometry guidelines (121) using a mechanic caliper (HOLTAIN LTD. CRYMYCH UK 10 g/mm2 constant pressure; range 0–39 mm and 0.1 mm of precision) and the results obtained are ranked according to percentiles proposed by Frisancho AR (122).
Using these data, other variables of interest are calculated. In particular, BMI is calculated as the body weight divided by the squared height (kg/m2). There is not a universal technique to classify the BMI values in the pediatric collective, therefore, the results are ranked according to the percentiles of Faustino Orbegozo Eizaguirre Foundation, reviewed in 2011 (120), International Obesity Task Force reviewed in 2000 (123), and WHO reviewed in 2007 (124). The results of overweight and obesity rates are unified as a single category called excess weight (EW). The arm muscular and fat areas are obtained using the equations proposed by Mataix Verdú and López Jurado (125) and López-Sobaler and Quintas Herrero (126), respectively. The protein and caloric reserves are calculated by Frisancho AR equations (122). Waist/height ratio is calculated as waist circumference (cm)/height (cm) and it was classified according to Panjikkaran et al. and Ashwell investigations (127, 128). Height/age index is rated in percentiles according to Fernández et al. (120).
For blood pressure monitoring, an automatic digital monitor is used (OMRON M3-Intellisense) using a cuff suitable for children. The results are classified according to the percentiles established by the Spanish Association of Pediatrics (129).
All these measurements are repeated every year on equal terms.
Compiling Saliva Samples, DNA Extraction and Genotyping
Buccal smears were collected for DNA extraction following standardized protocols. For this purpose, a sterile swab free of human RNAse, DNAse and DNA (300263DNA-Hisopos Deltalab polystyrene and polyester) was used. Children had to have their mouth clean and avoid eating or drinking 30 min prior to collection. Three samples were taken per children, each one identified with the number corresponding to the order of extraction, to ensure traceability. As the samples were collected, they were directly stored in refrigeration until all the children were evaluated. Immediately after, they were frozen at −80°C until their processing.
Genomic DNA was extracted from the buccal swabs using the INVISORB® SPIN TISSUE MINI KIT (Stratec), according to the manufacturer's instructions. Samples were lysed in the presence of proteinase K and a specific lysis buffer. The lysate was then purified and finally, it was eluted in a free EDTA solution.
For genotyping, the DNA samples were loaded in TaqMan® OpenArray® Real-Time PCR plates (Life Technologies Inc., Carlsbad, CA) already configured with the specific selected SNPs with specific waves for each allele, marked with a different fluorophore to determine the genotype. This process was made using the OpenArray® AccuFill™ System (Life Technologies Inc., Carlsbad, CA). Once it was charged, a PCR performed and the chips were read in the QuantStudio® 12K Flex Real-Time PCR Instrument (Life Technologies Inc., Carlsbad, CA). Results were analyzed using the TaqMan® Genotyper software (Life Technologies Inc., Carlsbad, CA), which automatically assigns the genotype to each sample according to the amount of detected signal for each fluorophore.
The duplicate analysis was used to validate the genotyping result.
Design of Educational Tools and Implementation of the Nutritional Education Programme
For the implementation of the nutritional education programme in the “intervention schools”, three different kinds of guides were designed aimed at parents, children and teachers. All this information was developed and adapted to the participants' age by the nutritionists from the IMDEA Food Foundation. This material is sent to parents and educational centers in different modules adapted to parents, students, and teachers. The same modules include different activities and topics each year according to the children's growth. The sending strategy follows a protocol, and it will be maintained until the end of the study, through email or regular delivery, as the receiver may prefer.
Moreover, some workshops are being carried out and are summarized in Table 4.
The validation of this tool is expected to be carried out through the impact generated over the years of the study, measured as the evolution of anthropometric variables and the dietary habits, between control and intervention schools. Moreover, parents and teachers along the study will evaluate all the material.
Statistical Analysis
Descriptive analyses of the baseline data were performed by computing for the categorical variables the class's absolute and relative frequencies, and for the quantitative variables the mean, median, standard deviation, interquartile range, maximum and minimum. To check the homogeneity of the two groups in the case of quantitative variables, t-tests were used for normally-distributed variables, or Mann-Whitney U-test as non-parametric alternative. In the case of categorical variables, Chi-Square or Fischer exact tests were used. The association between anthropometric and dietary, social, health and SNP variables were performed by linear or logistic regressions. The Bonferroni correction was applied for multiple tests. In addition, for the SNPs variables, the Hardy-Weinberg equilibrium condition was tested by means of Chi-Square tests. All analyses were conducted with R Statistical Software version 3.41. Statistical tests used a 0.05 significance level, in two-tailed tests.
Regarding Machine Learning models, they will be derived to predict the BMI from all the analyzed variables after the 5-year follow-up. Both classification (after dichotomization of the BMI) and regression models will be considered, and Random Forest will be applied. It has been observed that the use of Random Forest improves the predictive model's performance, creating a more effective predictive model than the one that could be obtained using decision tree or logistic regression techniques (13). The predictive power of the models will be evaluated and internal cross-validation and external validations with external datasets will be implemented. Variable importance analyses will be performed in order to quantify the relative weights of the different variables in the prediction of BMI. The model will be iteratively improved by refitting with new data along with the successive yearly evaluations during the study.
Results
Parents of 224 children (116 girls and 105 boys) accepted to participate in the study and signed the informed consent. It shows a collaboration rate of 39%. Finally, 221 children were evaluated, since three did not attend the initial evaluation (Figure 2).
Among the total number of students enrolled in the study, 115 belonged to intervention schools while 106 were in control schools. Tables 5, 6 show the basal main characteristics of the study sample according to the corresponding control or intervention group.
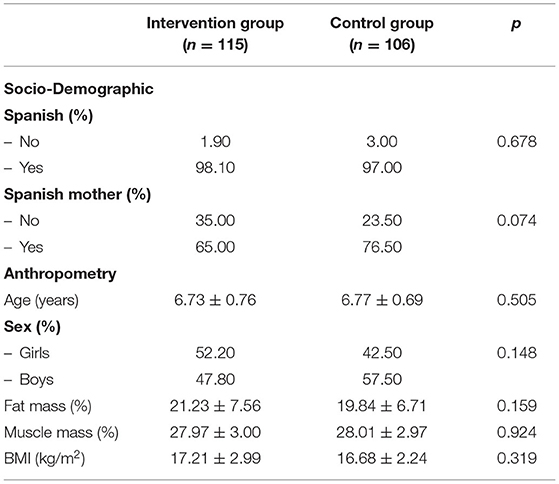
Table 5. Main socio-demographic and anthropometric characteristics of the schoolchildren by groups (intervention schools vs. control schools).
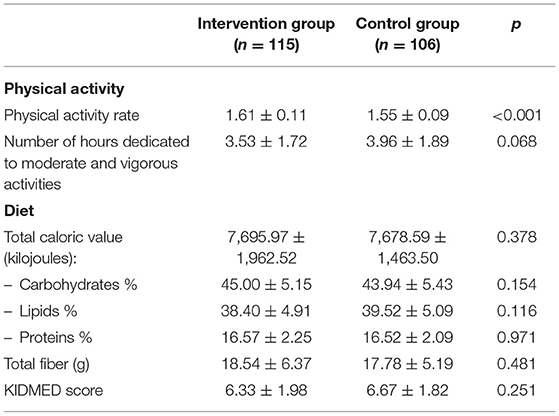
Table 6. Main dietary and physical activity characteristics of the schoolchildren by groups (intervention schools vs. control schools).
According to the preliminary results obtained after the first year of the evaluation, 32.2% of the students presented EW, taking into account the WHO criteria. These figures were higher when IOFT standard (25.4%) or the national criteria of the Orbegozo Foundation (19.0%) were applied.
A significative association between the nutritional state of children and mother BMI [β = 0.21 (0.13–0.3), p (adj) <0.001], geographical location of the school [OR = 2.74 (1.24–6.22), p (adj) = 0.06], dairy servings per day [OR = 0.48 (0.29–0.75), p (adj) = 0.05] and eight of the total SNPs studied [rs1260326, rs780094, rs10913469, rs328, rs7647305, rs3101336, rs2568958, rs925946; p (not adj) <0.05] were found (data adjusted for sex and age).
Discussion
The GENYAL study to prevent child obesity is, to our knowledge, the first interventional trial in Spanish schoolchildren aiming to provide preventive and therapeutic approach based on a high degree of evidence for early obesity through machine learning.
The baseline data and the associations observed after the first analysis support the evidence that environmental and genetic factors play a role in the development of childhood obesity.
According with the results, for each point in the BMI of the mother, the BMI of the child was increased 0.21 kg/m2. It shows that among the multiple risk factors for the development of obesity in children, parental obesity is one of the most impactful as a result of both genetic and environmental interactions. Children imitate their parents, therefore, the parents' dietary habits and PA are more likely to be reproduced by their descendants (130).
With reference to the location of the school, that represent the socioeconomic level, presented a close relationship with the presence of EW, with a decreasing distribution of risk from south to north area. These results are consistent with other studies that have shown how the socioeconomic status of the school correlates with the prevalence of overweight and obesity as it increases the likelihood that schoolchildren will follow a diet rich in energy-dense, low-cost foods, as well as fewer opportunities to practice sport (131).
Regarding dietary aspects, dairy servings per day showed a protective effect against EW. It could be related to several factors such as if this food is a source of calcium, peptides, bioactive compounds, etc. They have been studied due to their relationship in the appetite control and other mechanisms involved in controlling weight (132, 133). These results highlight the important role that this group of foods could have for the prevention of weight overload.
The study of these factors in the child population and the social context of Madrid is minimal, having found a single study with similar sociodemographic characteristics (134). Nevertheless, no intervention was performed in this study, nor was genetic data collection, thus the GENYAL project is shown as a novel study in this regard.
In this study, a significant association (data adjusted for sex and age) between the nutritional status of schoolchildren and 8 SNPs was found (rs1260326, rs780094, rs10913469, rs328, rs7647305, rs3101336, rs2568958, rs925946). In previous studies, these polymorphisms have been associated with adiposity traits and their related comorbidities (Table 2). Genetic factors play an essential role in the development of obesity (135). Thus, knowing which ones are associated with excess weight early in life could contribute to obesity early detection.
Conversely, it is important to note that current knowledge is insufficient to determine the relative importance of these different factors. Therefore, new techniques are needed to be used as predictive instruments (12). Currently, machine learning is considered an extremely valuable tool in the medical field, since it is capable of providing diagnostic and early detection strategies for diseases through the analysis of large datasets (136). Prevention plays a crucial role in controlling the high obesity prevalence, so machine learning techniques have already been used for the prediction of the BMI in children (137). However, the current predictive model would be the first to include obesity-related SNPs as genetic information, as well as anthropometric, social and lifestyle variables.
Regarding the last results from the Commission on Ending Childhood Obesity, the implementation of integral programs that promote healthy environment in schools is recommended with the objective of ensuring that children grow well and develop healthy habits (138). Nevertheless, although Spain is one of the countries where more intervention studies to prevent obesity have been developed (139), the politic strategies to prevent chronic illnesses such as overweight and obesity are not defined, and even show very low evidence of efficiency, according to the last data revised by the Cochrane Database (140).
According to the latest scientific research, the intervention studies in schools which include family and community spheres, implementing actions to promote healthy food and physical activity, are the most effective (5, 46). This study has been designed to elaborate strategies and to work as a multidisciplinary team reinforcing the educational sphere of the participating children and their environment, school, and family.
One of the strong points of our study consists in the implementation, and subsequent validation, of educational tools for students, their parents and teachers, applying a nutritional education method that promotes healthy dietetic habits and physical exercise, both in schools and outside. The importance of the validation of these educational strategies lay on a large number of studies with contradictory results, which might be partly explained by the fact that many researches may have lacked statistical power to detect changes in the results of interest related to adiposity or children's nutritional status (5). In the present study, the educational approaches to the intervention schools will be held for 5 years, in line with the annual anthropometric assessments. Their utility will be evaluated taking into account the evolution of the anthropometric and dietetic results annually collected. Moreover, the presence or absence of the educational program (control and intervention schools) will be included as an input dichotomous variable in the predictive model, evaluating its influence on the predicted BMI. This will allow us to detect differences in the body composition between intervention and control schools, enabling us to assess the impact of educational support.
Another strength of the present study is the selection of 26 SNPs for early prevention of obesity, by making an extensive bibliographic research, as shown in Table 2. The nutritional genomic tools would be very useful in the research and prevention of obesity, and they would be an important support in public health applications. Obesity is a multifactorial illness, where the genetic variants involved are dispersed along the whole genome. Although SNPs have been cataloged as the best indicators to predict obesity risk (141), several studies suggest that much remains to be discovered. There is a lot of interest in predicting the appearance of chronic diseases at an early age (142). According to the last review about precision nutrition (143), the creation of a genetic risk score may let us determine the risk of developing obesity or other chronic pathologies related to the individual genetic component, and even be able to predict the expected weight gain as a consequence of exposure to different variables, such as specific diets.
On the other hand, we consider that the sample size used is one of the weak aspects in our study, pointing to the necessity to include new schools to increase the number of children. Nevertheless, from this first phase of the study, we expect to calculate the sample size needed to increase the statistical power and, consequently, to find solid associations between the studied variables. Furthermore, the classification of schools according to the area and the socioeconomic level widens the scope of the research, making it more representative of the city of Madrid. Similarly, the use of dietary and physical activity questionnaires may lead to reporting bias, but in the absence of better tools with low cost and high throughput, these records can offer valuable information, although it should be interpreted with caution.
After 5 years of follow-up, the GENYAL study aims to validate the machine learning predictive model that considers environmental and genetic factors in the obesity development, as well as the educational tools, to obtain new and potentially valuable data to increase our knowledge of the precipitants of childhood obesity and their relative importance to design preventive protocols at an early age based on machine learning models. With a view to the future perspective of continuity of this study, in addition to increasing the sample size to validate the results obtained, the possibility of implementing personalized nutritional education interventions is proposed to improve adherence and efficacy by applying the novel concepts provided by studies on precision nutrition.
Data Availability Statement
The original contributions presented in the study are included in the article/supplementary materials, further inquiries can be directed to the corresponding author/s.
Ethics Statement
The studies involving human participants were reviewed and approved by Ethics Committee of Fundación IMDEA-Food. Written informed consent to participate in this study was provided by the participants' legal guardian/next of kin.
Author Contributions
VL-K was the principal investigator and responsible for the study and protocol design. HM-P helped designing the protocol and drafting the manuscript. HM-P, EA-A, RI, and IE-S were responsible for data collection. GC conducted statistical analysis of the data. SM contributed to genetic samples management. JM, GR, and AR supervised the final compilation of the manuscript and provided scientific advice and consultation. All authors read and approved the final manuscript.
Funding
This study was supported by Conserjería de Educación, Universidades y Ciencia de la Comunidad de Madrid, Dirección General de Educación Infantil, Primaria y Secundaria.
Conflict of Interest
The authors declare that the research was conducted in the absence of any commercial or financial relationships that could be construed as a potential conflict of interest.
Publisher's Note
All claims expressed in this article are solely those of the authors and do not necessarily represent those of their affiliated organizations, or those of the publisher, the editors and the reviewers. Any product that may be evaluated in this article, or claim that may be made by its manufacturer, is not guaranteed or endorsed by the publisher.
Acknowledgments
The authors would like to acknowledge the help of all the teachers and head teachers of Juan Zaragüeta, Fernando el Católico, Fernández Moratín, La Rioja, Concepción Arenal y Rosa Luxemburgo schools; and, especially, the families and children who participated in the study. We would like to thank all the IMDEA-Food students for their contribution in the nutritional education programme development.
Abbreviations
ACM, Autonomous Community of Madrid; SNPs, Single Nucleotide Polymorphisms; IPAC, Individual Physical Activity Coefficient; PAC, Physical Activity Coefficient; EW, Excess Weight.
References
1. Güngör NK. Overweight and obesity in children and adolescents. J Clin Res Pediatr Endocrinol. (2014) 6:129–43. doi: 10.4274/jcrpe.1471
2. WHO. Facts and Figures on Childhood Obesity. Available online at: http://www.who.int/end-childhood-obesity/facts/en/ (accessed December 22, 2019).
3. Agencia Española de Consumo, Seguridad Alimentaria y Nutrición. Estudio ALADINO 2015: Estudio de Vigilancia del Crecimiento, Alimentación, Actividad Física, Desarrollo Infantil y Obesidad en España 2015. Madrid: Ministerio de Sanidad, Servicios Sociales e Igualdad (2016)
4. Kolotkin RL, Andersen JR. A systematic review of reviews: exploring the relationship between obesity, weight loss and health-related quality of life. Clin Obes. (2017) 7:273–89. doi: 10.1111/cob.12203
5. Bleich SN, Vercammen KA, Zatz LY, Frelier JM, Ebbeling CB, Peeters A. Interventions to prevent global childhood overweight and obesity: a systematic review. Lancet Diabetes Endocrinol. (2017) 4:332–46. doi: 10.1016/S2213-8587(17)30358-3
6. Genoni G, Menegon V, Secco GG, Sonzini M, Martelli M, Castagno M, et al. Insulin resistance, serum uric acid and metabolic syndrome are linked to cardiovascular dysfunction in pediatric obesity. Int J Cardiol. (2017) 249:366–71. doi: 10.1016/j.ijcard.2017.09.031
7. Matwiejczyk L, Mehta K, Scott J, Tonkin E, Coveney J. Characteristics of effective interventions promoting healthy eating for pre-schoolers in childcare settings: an umbrella review. Nutrients. (2018) 10:293. doi: 10.3390/nu10030293
8. Iguacel I, Fernández-Alvira JM, Labayen I, Moreno LA, Samper MP, Rodríguez G. CALINA study. Social vulnerabilities as determinants of overweight in 2-, 4- and 6-year-old Spanish children. Eur J Public Health. (2017) 28:289–95. doi: 10.1093/eurpub/ckx095
9. Paparo L, di Costanzo M, di Scala C, Cosenza L, Leone L, Nocerino R, et al. The influence of early life nutrition on epigenetic regulatory mechanisms of the immune system. Nutrients. (2014) 6:4706–19. doi: 10.3390/nu6114706
10. Kumar S, Kelly AS. Review of childhood obesity: from epidemiology, etiology, and comorbidities to clinical assessment and treatment. Mayo Clin Proc. (2017) 92:251–65. doi: 10.1016/j.mayocp.2016.09.017
11. Kostovski M, Tasic V, Laban N, Polenakovic M, Danilovski D, Gucev Z. Obesity in childhood and adolescence, genetic factors. PRILOZI. (2017) 38:121–33. doi: 10.2478/prilozi-2018-0013
12. Qasim A, Turcotte M, Souza RJ de, Samaan MC, Champredon D, Dushoff J, et al. On the origin of obesity: identifying the biological, environmental and cultural drivers of genetic risk among human populations. Obes Rev. (2018) 19:121–49. doi: 10.1111/obr.12625
13. DeGregory KW, Kuiper P, DeSilvio T, Pleuss JD, Miller R, Roginski JW, et al. A review of machine learning in obesity. Obes Rev. (2018) 19:668–85. doi: 10.1111/obr.12667
14. Renta neta media de los hogares (Urban Audit) - Ayuntamiento de Madrid. Available online at: http://www.madrid.es/portales/munimadrid/es/Inicio/El-Ayuntamiento/Estadistica/Areas-de-informacion-estadistica/Economia/Renta/Renta-neta-media-de-los-hogares-Urban-Audit-?vgnextfmt=default&vgnextoid=65e0c19a1666a510VgnVCM1000001d4a900aRCRD&vgnextchannel=ef863636b44b4210VgnVCM2000000c205a0aRCRD (accessed December 22, 2019).
15. Suárez-Sánchez F, Klunder-Klunder M, Valladares-Salgado A, Gómez-Zamudio J, Peralta-Romero J, Meyre D, et al. APOA5 and APOA1 polymorphisms are associated with triglyceride levels in Mexican children. Pediatr Obes. (2017) 12:330–6. doi: 10.1111/ijpo.12147
16. Zhu W-F, Wang C-L, Liang L, Shen Z, Fu J-F, Liu P-N, et al. Triglyceride-raising APOA5 genetic variants are associated with obesity and non-HDL-C in Chinese children and adolescents. Lipids Health Dis. (2014) 13:93. doi: 10.1186/1476-511X-13-93
17. Au A, Griffiths LR, Irene L, Kooi CW, Wei LK. The impact of APOA5, APOB, APOC3 and ABCA1 gene polymorphisms on ischemic stroke: evidence from a meta-analysis. Atherosclerosis. (2017) 265:60–70. doi: 10.1016/j.atherosclerosis.2017.08.003
18. Ye H, Zhou A, Hong Q, Tang L, Xu X, Xin Y, et al. Positive association between APOA5 rs662799 polymorphism and coronary heart disease: a case-control study and meta-analysis. PLoS ONE. (2015) 10:e0135683. doi: 10.1371/journal.pone.0135683
19. Xu C, Bai R, Zhang D, Li Z, Zhu H, Lai M, et al. Effects of APOA5−1131T>C (rs662799) on fasting plasma lipids and risk of metabolic syndrome: evidence from a case-control study in China and a meta-analysis. PLoS ONE. (2013) 8:e56216. doi: 10.1371/journal.pone.0056216
20. Elks CE, Loos RJF, Sharp SJ, Langenberg C, Ring SM, Timpson NJ, et al. Genetic markers of adult obesity risk are associated with greater early infancy weight gain and growth. PLoS Med. (2010) 7:e1000284. doi: 10.1371/journal.pmed.1000284
21. Dušátková L, Zamrazilová H, Aldhoon-Hainerová I, Sedláčková B, Včelák J, Hlavatý P, et al. common variant near BDNF is associated with dietary calcium intake in adolescents. Nutr Res. (2015) 35:766–73. doi: 10.1016/j.nutres.2015.06.004
22. Dušátková L, Zamrazilová H, Sedláčková B, Včelák J, Hlavatý P, Aldhoon Hainerová I, et al. Association of obesity susceptibility gene variants with metabolic syndrome and related traits in 1,443 Czech adolescents. Folia Biol. (2013) 59:123–33.
23. Kvaløy K, Kulle B, Romundstad P, Holmen TL. Sex-specific effects of weight-affecting gene variants in a life course perspective–The HUNT Study, Norway. Int J Obes. (2013) 37:1221–9. doi: 10.1038/ijo.2012.220
24. Sandholt CH, Vestmar MA, Bille DS, Borglykke A, Almind K, Hansen L, et al. Studies of metabolic phenotypic correlates of 15 obesity associated gene variants. PLoS ONE. (2011) 6:e23531. doi: 10.1371/journal.pone.0023531
25. Akbarian S-A, Salehi-Abargouei A, Pourmasoumi M, Kelishadi R, Nikpour P, Heidari-Beni M. Association of Brain-derived neurotrophic factor gene polymorphisms with body mass index: a systematic review and meta-analysis. Adv Med Sci. (2017) 63:43–56. doi: 10.1016/j.advms.2017.07.002
26. Lv D, Zhou D, Zhang Y, Zhang S, Zhu Y-M. Two obesity susceptibility loci in LYPLAL1 and ETV5 independently associated with childhood hypertension in Chinese population. Gene. (2017) 627:284–9. doi: 10.1016/j.gene.2017.06.030
27. Elks CE, Perry JRB, Sulem P, Chasman DI, Franceschini N, He C, et al. Thirty new loci for age at menarche identified by a meta-analysis of genome-wide association studies. Nat Genet. (2010) 42:1077–85. doi: 10.1038/ng.714
28. Zhao J, Bradfield JP Li M, Wang K, Zhang H, Kim CE, Annaiah K, et al. The role of obesity-associated loci identified in genome-wide association studies in the determination of pediatric BMI. Obesity. (2009) 17:2254–7. doi: 10.1038/oby.2009.159
29. Grant SFA, Li M, Bradfield JP, Kim CE, Annaiah K, Santa E, et al. Association analysis of the FTO gene with obesity in children of Caucasian and African ancestry reveals a common tagging SNP. PLoS ONE. (2008) 3:e1746. doi: 10.1371/journal.pone.0001746
30. Mitchell JA, Hakonarson H, Rebbeck TR, Grant SFA. Obesity-susceptibility loci and the tails of the pediatric BMI distribution. Obesity. (2013) 21:1256–60. doi: 10.1002/oby.20319
31. Qureshi SA, Mumtaz A, Shahid SU, Shabana NA. rs3751812, a common variant in fat mass and obesity-associated (FTO) gene, is associated with serum high- and low-density lipoprotein cholesterol in Pakistani individuals. Nutrition. (2017) 39–40:92–5. doi: 10.1016/j.nut.2016.04.008
32. Wang K, Li W-D, Zhang CK, Wang Z, Glessner JT, Grant SFA, et al. Genome-wide association study on obesity and obesity-related traits. PLoS ONE. (2011) 6:e18939. doi: 10.1371/journal.pone.0018939
33. Namjou B, Keddache M, Marsolo K, Wagner M, Lingren T, Cobb B, et al. EMR-linked GWAS study: investigation of variation landscape of loci for body mass index in children. Front Genet. (2013) 4:268. doi: 10.3389/fgene.2013.00268
34. Dwivedi OP, Tabassum R, Chauhan G, Ghosh S, Marwaha RK, Tandon N, et al. Common variants of FTO are associated with childhood obesity in a cross-sectional study of 3,126 urban Indian children. PLoS ONE. (2012) 7:e47772. doi: 10.1371/journal.pone.0047772
35. Mei H, Chen W, Jiang F, He J, Srinivasan S, Smith EN, et al. Longitudinal replication studies of GWAS risk SNPs influencing body mass index over the course of childhood and adulthood. PLoS ONE. (2012) 7:e31470. doi: 10.1371/journal.pone.0031470
36. Yang Y, Liu B, Xia W, Yan J, Liu H-Y, Hu L, Liu S-M. FTO Genotype and Type 2 diabetes mellitus: spatial analysis and meta-analysis of 62 case-control studies from different regions. Genes. (2017) 8:70. doi: 10.3390/genes8020070
37. Hunt SC, Stone S, Xin Y, Scherer CA, Magness CL, Iadonato SP, et al. Association of the FTO gene with BMI. Obesity. (2008) 16:902–4. doi: 10.1038/oby.2007.126
38. Quan L-L, Wang H, Tian Y, Mu X, Zhang Y, Tao K. Association of fat-mass and obesity-associated gene FTO rs9939609 polymorphism with the risk of obesity among children and adolescents: a meta-analysis. Eur Rev Med Pharmacol Sci. (2015) 19:614–23.
39. Liu C, Mou S, Cai Y. FTO gene variant and risk of overweight and obesity among children and adolescents: a systematic review and meta-analysis. PLoS ONE. (2013) 8:e82133. doi: 10.1371/journal.pone.0082133
40. Xi B, Takeuchi F, Meirhaeghe A, Kato N, Chambers JC, Morris AP, et al. Associations of genetic variants in/near body mass index-associated genes with type 2 diabetes: a systematic meta-analysis. Clin Endocrinol. (2014) 81:702–10. doi: 10.1111/cen.12428
41. Willer CJ, Speliotes EK, Loos RJF Li S, Lindgren CM, Heid IM, Berndt SI, et al. Six new loci associated with body mass index highlight a neuronal influence on body weight regulation. Nat Genet. (2009) 41:25–34. doi: 10.1038/ng.287
42. Lee H-J, Jang HB, Kim H-J, Ahn Y, Hong K-W, Cho SB, et al. The dietary monounsaturated to saturated fatty acid ratio modulates the genetic effects of GCKR on serum lipid levels in children. Clin Chim Acta. (2015) 450:155–61. doi: 10.1016/j.cca.2015.08.012
43. Horvatovich K, Bokor S, Polgar N, Kisfali P, Hadarits F, Jaromi L, et al. Functional glucokinase regulator gene variants have inverse effects on triglyceride and glucose levels, and decrease the risk of obesity in children. Diabetes Metab. (2011) 37:432–9. doi: 10.1016/j.diabet.2011.02.003
44. Santoro N, Zhang CK, Zhao H, Pakstis AJ, Kim G, Kursawe R, et al. Variant in the glucokinase regulatory protein (GCKR) gene is associated with fatty liver in obese children and adolescents. Hepatology. (2012) 55:781–9. doi: 10.1002/hep.24806
45. Alfred T, Ben-Shlomo Y, Cooper R, Hardy R, Deary IJ, Elliott J, et al. Associations between a polymorphism in the pleiotropic GCKR and Age-related phenotypes: the HALCyon programme. PLoS ONE. (2013) 8:e70045. doi: 10.1371/journal.pone.0070045
46. Wang Y, Cai L, Wu Y, Wilson RF, Weston C, Fawole O, et al. What childhood obesity prevention programmes work? A systematic review and meta-analysis. Obes Rev. (2015) 16:547–65. doi: 10.1111/obr.12277
47. Chang H-W, Lin F-H, Li P-F, Huang C-L, Chu N-F, Su S-C, et al. Association between a glucokinase regulator genetic variant and metabolic syndrome in taiwanese adolescents. Genet Test Mol Biomark. (2016) 20:137–42. doi: 10.1089/gtmb.2015.0241
48. Zain SM, Mohamed Z, Mohamed R. Common variant in the glucokinase regulatory gene rs780094 and risk of nonalcoholic fatty liver disease: a meta-analysis. J Gastroenterol Hepatol. (2015) 30:21–7. doi: 10.1111/jgh.12714
49. Fesinmeyer MD, Meigs JB, North KE, Schumacher FR, BuŽková P, Franceschini N, et al. Genetic variants associated with fasting glucose and insulin concentrations in an ethnically diverse population: results from the Population Architecture using Genomics and Epidemiology (PAGE) study. BMC Med Genet. (2013) 14:98. doi: 10.1186/1471-2350-14-98
50. Li H, Xu R, Peng X, Wang Y, Wang T. Association of glucokinase regulatory protein polymorphism with type 2 diabetes and fasting plasma glucose: a meta-analysis. Mol Biol Rep. (2013) 40:3935–42. doi: 10.1007/s11033-012-2470-6
51. Xi B, Cheng H, Shen Y, Chandak GR, Zhao X, Hou D, et al. Study of 11 BMI-associated loci identified in GWAS for associations with central obesity in the Chinese children. PLoS ONE. (2013) 8:e56472. doi: 10.1371/journal.pone.0056472
52. Beyerlein A, von Kries R, Ness AR, Ong KK. Genetic markers of obesity risk: stronger associations with body composition in overweight compared to normal-weight children. PLoS ONE. (2011) 6:e19057. doi: 10.1371/journal.pone.0019057
53. Li S, Zhao JH, Luan J, Ekelund U, Luben RN, Khaw K-T, et al. Physical activity attenuates the genetic predisposition to obesity in 20,000 men and women from EPIC-Norfolk prospective population study. PLoS Med. (2010) 7:e1000332. doi: 10.1371/journal.pmed.1000332
54. Queiroz EM, Cândido APC, Castro IM, Bastos AQA, Machado-Coelho GLL, Freitas RN. IGF2, LEPR, POMC, PPARG, and PPARGC1 gene variants are associated with obesity-related risk phenotypes in Brazilian children and adolescents. Braz J Med Biol Res. (2015) 48:595–602. doi: 10.1590/1414-431x20154155
55. Zandoná MR, Rodrigues RO, Albiero G, Campagnolo PDB, Vitolo MR, Almeida S, et al. Polymorphisms in LEPR, PPARG and APM1 genes: associations with energy intake and metabolic traits in young children. Arq Bras Endocrinol Metabol. (2013) 57:603–11. doi: 10.1590/S0004-27302013000800004
56. Yang MM, Wang J, Fan JJ, Ng TK, Sun DJ, Guo X, et al. Variations in the obesity gene “LEPR” contribute to risk of type 2 diabetes mellitus: evidence from a meta-analysis. J Diabetes Res. (2016) 2016:5412084. doi: 10.1155/2016/5412084
57. Mahmoudi T, Farahani H, Nobakht H, Dabiri R, Zali MR. Genetic variations inLeptin andLeptin receptor and susceptibility to colorectal cancer and obesity. Iran J Cancer Prev. (2016) 9:e7013. doi: 10.17795/ijcp-7013
58. Domínguez-Reyes T, Astudillo-López CC, Salgado-Goytia L, Muñoz-Valle JF, Salgado-Bernabé AB, Guzmán-Guzmán IP, et al. Interaction of dietary fat intake with APOA2, APOA5 and LEPR polymorphisms and its relationship with obesity and dyslipidemia in young subjects. Lipids Health Dis. (2015) 14:106. doi: 10.1186/s12944-015-0112-4
59. Askari G, Heidari-Beni M, Mansourian M, Esmaeil-Motlagh M, Kelishadi R. Interaction of lipoprotein lipase polymorphisms with body mass index and birth weight to modulate lipid profiles in children and adolescents: the CASPIAN-III Study. São Paulo Med J. (2016) 134:121–9. doi: 10.1590/1516-3180.2015.00792608
60. Emamian M, Avan A, Pasdar A, Mirhafez SR, Sadeghzadeh M, Moghadam MS, et al. The lipoprotein lipase S447X and cholesteryl ester transfer protein rs5882 polymorphisms and their relationship with lipid profile in human serum of obese individuals. Gene. (2015) 558:195–9. doi: 10.1016/j.gene.2014.12.070
61. White MJ, Eren F, Agirbaşli D, Chen J, Hu T, Moore JH, et al. Systems genetics approach to dyslipidemia in children and adolescents. OMICS. (2015) 19:248–59. doi: 10.1089/omi.2014.0140
62. Legry V, Bokor S, Beghin L, Galfo M, Gonzalez-Gross M, Molnar D, et al. Associations between common genetic polymorphisms in the liver X receptor alpha and its target genes with the serum HDL-cholesterol concentration in adolescents of the HELENA Study. Atherosclerosis. (2011) 216:166–9. doi: 10.1016/j.atherosclerosis.2011.01.031
63. Sagoo GS, Tatt I, Salanti G, Butterworth AS, Sarwar N, van Maarle M, et al. Seven lipoprotein lipase gene polymorphisms, lipid fractions, and coronary disease: a HuGE association review and meta-analysis. Am J Epidemiol. (2008) 168:1233–46. doi: 10.1093/aje/kwn235
64. Xi B, Takeuchi F, Chandak GR, Kato N, Pan HW, AGEN-T2D Consortium, et al. Common polymorphism near the MC4R gene is associated with type 2 diabetes: data from a meta-analysis of 123,373 individuals. Diabetologia. (2012) 55:2660–6. doi: 10.1007/s00125-012-2655-5
65. den Hoed M, Ekelund U, Brage S, Grontved A, Zhao JH, Sharp SJ, et al. Genetic susceptibility to obesity and related traits in childhood and adolescence: influence of loci identified by genome-wide association studies. Diabetes. (2010) 59:2980–8. doi: 10.2337/db10-0370
66. Xi B, Chandak GR, Shen Y, Wang Q, Zhou D. Association between common polymorphism near the MC4R gene and obesity risk: a systematic review and meta-analysis. PLoS ONE. (2012) 7:e45731. doi: 10.1371/journal.pone.0045731
67. Loos RJF, Lindgren CM Li S, Wheeler E, Zhao JH, Prokopenko I, Inouye M, et al. Common variants near MC4R are associated with fat mass, weight and risk of obesity. Nat Genet. (2008) 40:768–75. doi: 10.1038/ng.140
68. Qi L, Kraft P, Hunter DJ, Hu FB. The common obesity variant near MC4R gene is associated with higher intakes of total energy and dietary fat, weight change and diabetes risk in women. Hum Mol Genet. (2008) 17:3502–8. doi: 10.1093/hmg/ddn242
69. Lauria F, Siani A, Picó C, Ahrens W, Bammann K, De Henauw S, et al. A Common variant and the transcript levels of MC4R gene are associated with adiposity in children: the IDEFICS Study. J Clin Endocrinol Metab. (2016) 101:4229–36. doi: 10.1210/jc.2016-1992
70. Hester JM, Wing MR Li J, Palmer ND, Xu J, Hicks PJ, Roh BH, et al. Implication of European-derived adiposity loci in African Americans. Int J Obes. (2012) 36:465–73. doi: 10.1038/ijo.2011.131
71. Wheeler E, Huang N, Bochukova EG, Keogh JM, Lindsay S, Garg S, et al. Genome-wide SNP and CNV analysis identifies common and low-frequency variants associated with severe early-onset obesity. Nat Genet. (2013) 45:513–7. doi: 10.1038/ng.2607
72. Cheung CYY, Tso AWK, Cheung BMY, Xu A, Ong KL, Fong CHY, et al. Obesity susceptibility genetic variants identified from recent genome-wide association studies: implications in a chinese population. J Clin Endocrinol Metab. (2010) 95:1395–403. doi: 10.1210/jc.2009-1465
73. Zain SM, Mohamed Z, Jalaludin MY, Fauzi F, Hamidi A, Zaharan NL. Comprehensive evaluation of the neuropeptide-Y gene variants in the risk of obesity: a case-control study and meta-analysis. Pharmacogenet Genomics. (2015) 25:501–10. doi: 10.1097/FPC.0000000000000164
74. Olza J, Gil-Campos M, Leis R, Rupérez AI, Tojo R, Cañete R, et al. Influence of variants in the NPY gene on obesity and metabolic syndrome features in Spanish children. Peptides. (2013) 45:22–7. doi: 10.1016/j.peptides.2013.04.007
75. Hohmann S, Buchmann AF, Witt SH, Rietschel M, Jennen-Steinmetz C, Schmidt MH, et al. Increasing association between a neuropeptide Y promoter polymorphism and body mass index during the course of development. Pediatr Obes. (2012) 7:453–60. doi: 10.1111/j.2047-6310.2012.00069.x
76. Muñoz-Yáñez C, Pérez-Morales R, Moreno-Macías H, Calleros-Rincón E, Ballesteros G, González RA, et al. Polymorphisms FTO rs9939609, PPARG rs1801282 and ADIPOQ rs4632532 and rs182052 but not lifestyle are associated with obesity related-traits in Mexican children. Genet Mol Biol. (2016) 39:547–53. doi: 10.1590/1678-4685-gmb-2015-0267
77. Stryjecki C, Peralta-Romero J, Alyass A, Karam-Araujo R, Suarez F, Gomez-Zamudio J, et al. Association between PPAR-γ2 Pro12Ala genotype and insulin resistance is modified by circulating lipids in Mexican children. Sci Rep. (2016) 6:24472. doi: 10.1038/srep24472
78. Dedoussis GV, Vidra N, Butler J, Papoutsakis C, Yannakoulia M, Hirschhorn JN, et al. Peroxisome proliferator-activated receptor-gamma (PPARgamma) Pro12Ala polymorphism and risk for pediatric obesity. Clin Chem Lab Med. (2009) 47:1047–50. doi: 10.1515/CCLM.2009.242
79. Li Y, Zhu J, Ding JQ. Association of the PPARγ2 Pro12Ala polymorphism with increased risk of cardiovascular diseases. Genet Mol Res. (2015) 14:18662–74. doi: 10.4238/2015.December.28.15
80. Gouda HN, Sagoo GS, Harding A-H, Yates J, Sandhu MS, Higgins JPT. The association between the peroxisome proliferator-activated receptor-gamma2 (PPARG2) Pro12Ala gene variant and type 2 diabetes mellitus: a HuGE review and meta-analysis. Am J Epidemiol. (2010) 171:645–55. doi: 10.1093/aje/kwp450
81. Galbete C, Toledo E, Martínez-González MA, Martínez JA, Guillén-Grima F, Marti A. Pro12Ala variant of the PPARG2 gene increases body mass index: an updated meta-analysis encompassing 49,092 subjects. Obesity. (2013) 21:1486–95. doi: 10.1002/oby.20150
82. Bordoni L, Marchegiani F, Piangerelli M, Napolioni V, Gabbianelli R. Obesity-related genetic polymorphisms and adiposity indices in a young Italian population. IUBMB Life. (2017) 69:98–105. doi: 10.1002/iub.1596
83. Hotta K, Nakamura M, Nakamura T, Matsuo T, Nakata Y, Kamohara S, et al. Association between obesity and polymorphisms in SEC16B, TMEM18, GNPDA2, BDNF, FAIM2 and MC4R in a Japanese population. J Hum Genet. (2009) 54:727–31. doi: 10.1038/jhg.2009.106
84. Xi B, Shen Y, Reilly KH, Zhao X, Cheng H, Hou D, et al. Sex-dependent associations of genetic variants identified by GWAS with indices of adiposity and obesity risk in a Chinese children population. Clin Endocrinol. (2013) 79:523–8. doi: 10.1111/cen.12091
85. Cropano C, Santoro N, Groop L, Dalla Man C, Cobelli C, Galderisi A, et al. The rs7903146 variant in theTCF7L2Gene increases the risk of prediabetes/type 2 diabetes in obese adolescents by impairing β-cell function and hepatic insulin sensitivity. Diabetes Care. (2017) 40:1082–9. doi: 10.2337/dc17-0290
86. Locke AE, Kahali B, Berndt SI, Justice AE, Pers TH, Day FR, et al. Genetic studies of body mass index yield new insights for obesity biology. Nature. (2015) 518:197–206. doi: 10.1038/nature14177
87. Reinehr T, Friedel S, Mueller TD, Toschke AM, Hebebrand J, Hinney A. Evidence for an influence of TCF7L2 polymorphism rs7903146 on insulin resistance and sensitivity indices in overweight children and adolescents during a lifestyle intervention. Int J Obes. (2008) 32:1521–4. doi: 10.1038/ijo.2008.146
88. Körner A, Berndt J, Stumvoll M, Kiess W, Kovacs P. TCF7L2 gene polymorphisms confer an increased risk for early impairment of glucose metabolism and increased height in obese children. J Clin Endocrinol Metab. (2007) 92:1956–60. doi: 10.1210/jc.2006-2514
89. Verma SS, Lucas AM, Lavage DR, Leader JB, Metpally R, Krishnamurthy S, et al. Identifying genetic associations with variability in metabolic health and blood count laboratory values: diving into the quantitative traits by leveraging longitudinal data from an EHR. Pac Symp Biocomput. (2017) 22:533–44. doi: 10.1142/9789813207813_0049
90. Povel CM, Boer JMA, Reiling E, Feskens EJM. Genetic variants and the metabolic syndrome: a systematic review. Obes Rev. (2011) 12:952–67. doi: 10.1111/j.1467-789X.2011.00907.x
91. Guan Y, Yan LH, Liu XY, Zhu XY, Wang SZ, Chen LM. Correlation of the TCF7L2 (rs7903146) polymorphism with an enhanced risk of type 2 diabetes mellitus: a meta-analysis. Genet Mol Res. (2016) 15:gmr.15037969. doi: 10.4238/gmr.15037969
92. Wang S, Song K, Srivastava R, Fathzadeh M, Li N, Mani A. The protective effect of transcription factor 7-like 2 risk allele rs7903146 against elevated fasting plasma triglyceride in type 2 diabetes: a meta-analysis. J Diabetes Res. (2015) 2015:468627. doi: 10.1155/2015/468627
93. Chang S, Wang Z, Wu L, Lu X, Shangguan S, Xin Y, et al. Association between TCF7L2 polymorphisms and gestational diabetes mellitus: a meta-analysis. J Diabetes Investig. (2017) 8:560–70. doi: 10.1111/jdi.12612
94. Speliotes EK, Willer CJ, Berndt SI, Monda KL, Thorleifsson G, Jackson AU, et al. Association analyses of 249,796 individuals reveal 18 new loci associated with body mass index. Nat Genet. (2010) 42:937–48. doi: 10.1038/ng.686
95. Graff M, Scott RA, Justice AE, Young KL, Feitosa MF, Barata L, et al. Correction: Genome-wide physical activity interactions in adiposity - A meta-analysis of 200,452 adults. PLoS Genet. (2017) 13:e1006972. doi: 10.1371/journal.pgen.1006972
96. Prats-Puig A, Grau-Cabrera P, Riera-Pérez E, Cortés-Marina R, Fortea E, Soriano-Rodríguez P, et al. Variations in the obesity genes FTO, TMEM18 and NRXN3 influence the vulnerability of children to weight gain induced by short sleep duration. Int J Obes. (2013) 37:182–7. doi: 10.1038/ijo.2012.27
97. Rask-Andersen M, Jacobsson JA, Moschonis G, Chavan RA, Sikder MAN, Allzén E, et al. Association of TMEM18 variants with BMI and waist circumference in children and correlation of mRNA expression in the PFC with body weight in rats. Eur J Hum Genet. (2012) 20:192–7. doi: 10.1038/ejhg.2011.176
98. Takeuchi F, Yamamoto K, Katsuya T, Nabika T, Sugiyama T, Fujioka A, et al. Association of genetic variants for susceptibility to obesity with type 2 diabetes in Japanese individuals. Diabetologia. (2011) 54:1350–9. doi: 10.1007/s00125-011-2086-8
99. Lee H, Ash GI, Angelopoulos TJ, Gordon PM, Moyna NM, Visich PS, et al. Obesity-related genetic variants and their associations with physical activity. Sports Med Open. (2015) 1:34. doi: 10.1186/s40798-015-0036-6
100. Almén MS, Jacobsson JA, Shaik JHA, Olszewski PK, Cedernaes J, Alsiö J, et al. The obesity gene, TMEM18, is of ancient origin, found in majority of neuronal cells in all major brain regions and associated with obesity in severely obese children. BMC Med Genet. (2010) 11:58. doi: 10.1186/1471-2350-11-58
101. Abadi A, Peralta-Romero J, Suarez F, Gomez-Zamudio J, Burguete-Garcia AI, Cruz M, et al. Assessing the effects of 35 European-derived BMI-associated SNPs in Mexican children. Obesity. (2016) 24:1989–95. doi: 10.1002/oby.21590
102. Thorleifsson G, Walters GB, Gudbjartsson DF, Steinthorsdottir V, Sulem P, Helgadottir A, et al. Genome-wide association yields new sequence variants at seven loci that associate with measures of obesity. Nat Genet. (2009) 41:18–24. doi: 10.1038/ng.274
103. Ochoa MC, Santos JL, Azcona C, Moreno-Aliaga MJ, Martínez-González MA, Martínez JA, et al. Association between obesity and insulin resistance with UCP2-UCP3 gene variants in Spanish children and adolescents. Mol Genet Metab. (2007) 92:351–8. doi: 10.1016/j.ymgme.2007.07.011
104. Zhang M, Wang M, Zhao Z-T. Uncoupling protein 2 gene polymorphisms in association with overweight and obesity susceptibility: a meta-analysis. Meta Gene. (2014) 2:143–59. doi: 10.1016/j.mgene.2013.10.009
105. Brondani LA, Assmann TS, de Souza BM, Bouças AP, Canani LH, Crispim D. Meta-analysis reveals the association of common variants in the uncoupling protein (UCP) 1-3 genes with body mass index variability. PLoS ONE. (2014) 9:e96411. doi: 10.1371/journal.pone.0096411
106. Dhamrait SS, Williams AG, Day SH, Skipworth J, Payne JR, World M, et al. Variation in the uncoupling protein 2 and 3 genes and human performance. J Appl Physiol. (2012) 112:1122–7. doi: 10.1152/japplphysiol.00766.2011
107. de Luis DA, Aller R, Izaola O, Romero E. Effect of−55CT Polymorphism of UCP3 on insulin resistance and cardiovascular risk factors after a high protein/low carbohydrate versus a standard hypocaloric diet. Ann Nutr Metab. (2016) 68:157–63. doi: 10.1159/000444150
108. Gable DR, Stephens JW, Cooper JA, Miller GJ, Humphries SE. Variation in the UCP2-UCP3 gene cluster predicts the development of type 2 diabetes in healthy middle-aged men. Diabetes. (2006) 55:1504–11. doi: 10.2337/db05-1645
109. Otabe S, Clement K, Dina C, Pelloux V, Guy-Grand B, Froguel P, et al. genetic variation in the 5' flanking region of the UCP3 gene is associated with body mass index in humans in interaction with physical activity. Diabetologia. (2000) 43:245–9. doi: 10.1007/s001250050037
110. Salopuro T, Pulkkinen L, Lindström J, Kolehmainen M, Tolppanen A-M, Eriksson JG, et al. Variation in the UCP2 and UCP3 genes associates with abdominal obesity and serum lipids: the Finnish Diabetes Prevention Study. BMC Med Genet. (2009) 10:94. doi: 10.1186/1471-2350-10-94
111. HGNC Database of Human Gene Names. HUGO Gene Nomenclature Committee. Available online at: https://www.genenames.org/ (accessed February 26, 2018).
112. Ortíz H, Cuadrado J, León K, Esteban M, Galán I, Bravo J, et al. Diseño del estudio ELOIN y prevalencia de sobrepeso y obesidad en la población infantil de 4 años de la Comunidad de Madrid. Madrid: Informe de la Dirección General de Atención Primaria. (2014). Available online at: https://www.comunidad.madrid/sites/default/files/doc/sanidad/epid/diseno_eloin.pdf
113. European Food Safety Authority. General principles for the collection of national food consumption data in the view of a pan-European dietary survey. EFSA J. (2009) 7:51. doi: 10.2903/j.efsa.2009.1435
114. Ortega R, López-Sobaler A, Andrés P, Requejo A, Aparicio A, Molinero L. DIAL Software for Assessing Diets and Food Calculations (for Windows, version 3.3.6.0). Madrid: Departamento de Nutrición y Ciencia de los Alimentos (UCM) y Alceingeniería, S.A. (2013).
115. Serra-Majem L, Ribas L, Ngo J, Ortega RM, García A, Pérez-Rodrigo C, et al. Food, youth and the Mediterranean diet in Spain. Development of KIDMED, Mediterranean Diet Quality Index in children and adolescents. Public Health Nutr. (2004) 7:931–5. doi: 10.1079/PHN2004556
116. Ortega R, Requejo A, López-Sobaler A. “Modelos de cuestionario de actividad.,” Nutriguía. Manual de nutrición clínica en atención primaria. Madrid: Complutense (2006). 468 p.
117. WHO. Physical Status: The Use and Interpretation of Anthropometry. Available online at: http://www.who.int/childgrowth/publications/physical_status/en/ (accessed December 22, 2019).
118. Lupton JR, Brooks J, Butte NF, Caballero B, Flatt JP, Fried SK. Dietary Reference Intakes for Energy, Carbohydrate, Fiber, Fat, Fatty Acids, Cholesterol, Protein, and Amino Acids. Washington, DC: National Academy Press (2002).
119. OMROM Healthcare. Available online at: https://www.omron-healthcare.es/on/demandware.static/-/Sites-master-catalog/default/dw50f02349/pdfs/ES/IM-HBF-511B-E-ES-10-08-2017.pdf (accessed December 22, 2019).
120. Fernández C, Lorenzo H, Vrotsou K, Aresti U, Rica I, Sánchez E. Estudio de Crecimiento de Bilbao. Curvas y tablas de crecimiento. Estudio Transversal. Bilbao: Fundación Faustino Orbegozo (2011).
121. Stewart A, Marfell-Jones M. International Society for Advancement of Kinanthropometry. International Standards for Anthropometric Assessment. Lower Hutt: International Society for the Advancement of Kinanthropometry (2011).
122. Frisancho AR. New norms of upper limb fat and muscle areas for assessment of nutritional status. Am J Clin Nutr. (1981) 34:2540–5. doi: 10.1093/ajcn/34.11.2540
123. Cole TJ, Bellizzi MC, Flegal KM, Dietz WH. Establishing a standard definition for child overweight and obesity worldwide: international survey. BMJ. (2000) 320:1240–3. doi: 10.1136/bmj.320.7244.1240
124. WHO. Growth Reference Data for 5-19 Years. Available online at: http://www.who.int/growthref/en/ (accessed December 22, 2019).
125. Mataix Verdú J, López Jurado M. “Valoración del estado nutricional,” Nutrición y Alimentación Humana. Barcelona: Ergón (2015). p. 751–800.
126. López-Sobaler A, Quintas Herrero M. “Estudio antopométrico” NutriguíaR Manual de Nutrición Clínica. Madrid: Médica Panamericana (2015). p. 153–63.
127. Panjikkaran ST, Kumari K. Augmenting BMI and waist-height ratio for establishing more efficient obesity percentiles among school-going children. Indian J Commun Med. (2009) 34:135–9. doi: 10.4103/0970-0218.51233
128. Ashwell M. Obesity risk: importance of the waist-to-height ratio. Nurs Stand. (2009) 23:49–54; quiz 55. doi: 10.7748/ns2009.06.23.41.49.c7050
129. de la Cerda F, Herrero C. Hipertensión Arterial en Niños y Adolescentes. Asociación Española de Pediatría y Asociación Española de Nefrología Pediátrica. Protoc Diagn Pediatr. (2014) 1:171–89. https://www.aeped.es/sites/default/files/documentos/12_hta.pdf
130. Bahreynian M, Qorbani M, Khaniabadi BM, Motlagh ME, Safari O, Asayesh H, et al. Association between obesity and parental weight status in children and adolescents. J Clin Res Pediatr Endocrinol. (2017) 9:111–7. doi: 10.4274/jcrpe.3790
131. Bel-Serrat S, Heinen MM, Mehegan J, O'Brien S, Eldin N, Murrin CM, et al. School sociodemographic characteristics and obesity in schoolchildren: does the obesity definition matter? BMC Public Health. (2018) 18:337. doi: 10.1186/s12889-018-5246-7
132. Wadolowska L, Ulewicz N, Sobas K, Wuenstel JW, Slowinska MA, Niedzwiedzka E, et al. Dairy-related dietary patterns, dietary calcium, body weight and composition: a study of obesity in polish mothers and daughters, the MODAF project. Nutrients. (2018) 10:90. doi: 10.3390/nu10010090
133. Koca T, Akcam M, Serdaroglu F, Dereci S. Breakfast habits, dairy product consumption, physical activity, and their associations with body mass index in children aged 6–18. Eur J Pediatr. (2017) 176:1251–7. doi: 10.1007/s00431-017-2976-y
134. Estudio de la situación nutricional de la población infantil en la ciudad de Madrid - Ayuntamiento de Madrid. Available online at: https://www.madrid.es/portales/munimadrid/es/Inicio/Servicios-sociales-y-salud/Salud/Publicaciones/Estudio-de-la-situacion-nutricional-de-la-poblacion-infantil-en-la-ciudad-de-Madrid/?vgnextfmt=default&vgnextoid=72cde4ceef1ee510VgnVCM1000001d4a900aRCRD&vgnextchannel=e6898fb9458fe410VgnVCM1000000b205a0aRCRD (accessed January 26, 2021).
135. Mǎrginean CO, Mǎrginean C, Melit LE. New insights regarding genetic aspects of childhood obesity: a minireview. Front Pediatr. (2018) 6:271. doi: 10.3389/fped.2018.00271
136. Gilvary C, Madhukar N, Elkhader J, Elemento O. The missing pieces of artificial intelligence in medicine. Trends Pharmacol Sci. (2019) 40:555–64. doi: 10.1016/j.tips.2019.06.001
137. Dugan TM, Mukhopadhyay S, Carroll A, Downs S. Machine learning techniques for prediction of early childhood obesity. Appl Clin Inform. (2015) 6:506–20. doi: 10.4338/ACI-2015-03-RA-0036
138. Nishtar S, Gluckman P, Armstrong T. Ending childhood obesity: a time for action. Lancet. (2016) 387:825–7. doi: 10.1016/S0140-6736(16)00140-9
139. Visiedo A, de Baranda PS, Crone D, Aznar S, Perez-Llamas F. Programs to prevent obesity in school children 5 to 10 years old: a review. Nutr Hosp. (2016) 33:814–24. doi: 10.20960/nh.375
140. Wolfenden L, Nathan NK, Sutherland R, Yoong SL, Hodder RK, Wyse RJ, et al. Strategies for enhancing the implementation of school-based policies or practices targeting risk factors for chronic disease. Cochr Database Syst Rev. (2017) 11:CD011677. doi: 10.1002/14651858.CD011677.pub2
141. Belsky DW, Moffitt TE, Sugden K, Williams B, Houts R, McCarthy J, et al. Development and evaluation of a genetic risk score for obesity. Biodemogr Soc Biol. (2013) 59:85–100. doi: 10.1080/19485565.2013.774628
142. Goldstein BA, Yang L, Salfati E, Assimes TL. Contemporary considerations for constructing a genetic risk score: an empirical approach. Genet Epidemiol. (2015) 39:439–45. doi: 10.1002/gepi.21912
Keywords: pediatric obesity, early intervention, single nucleotide polymorphisms, machine learning, nutrition
Citation: Marcos-Pasero H, Aguilar-Aguilar E, de la Iglesia R, Espinosa-Salinas I, Molina S, Colmenarejo G, Martínez JA, Ramírez de Molina A, Reglero G and Loria-Kohen V (2022) “GENYAL” Study to Childhood Obesity Prevention: Methodology and Preliminary Results. Front. Nutr. 9:777384. doi: 10.3389/fnut.2022.777384
Received: 15 September 2021; Accepted: 04 February 2022;
Published: 08 March 2022.
Edited by:
Jorge Moreno-Fernandez, University of Granada, SpainReviewed by:
Masahide Hamaguchi, Kyoto Prefectural University of Medicine, JapanMargarita Torres-Tamayo, Instituto Nacional de Cardiologia Ignacio Chavez, Mexico
Francisco Jesús Llorente Cantarero, University of Cordoba, Spain
Copyright © 2022 Marcos-Pasero, Aguilar-Aguilar, de la Iglesia, Espinosa-Salinas, Molina, Colmenarejo, Martínez, Ramírez de Molina, Reglero and Loria-Kohen. This is an open-access article distributed under the terms of the Creative Commons Attribution License (CC BY). The use, distribution or reproduction in other forums is permitted, provided the original author(s) and the copyright owner(s) are credited and that the original publication in this journal is cited, in accordance with accepted academic practice. No use, distribution or reproduction is permitted which does not comply with these terms.
*Correspondence: Viviana Loria-Kohen, dmxvcmlhQHVjbS5lcw==