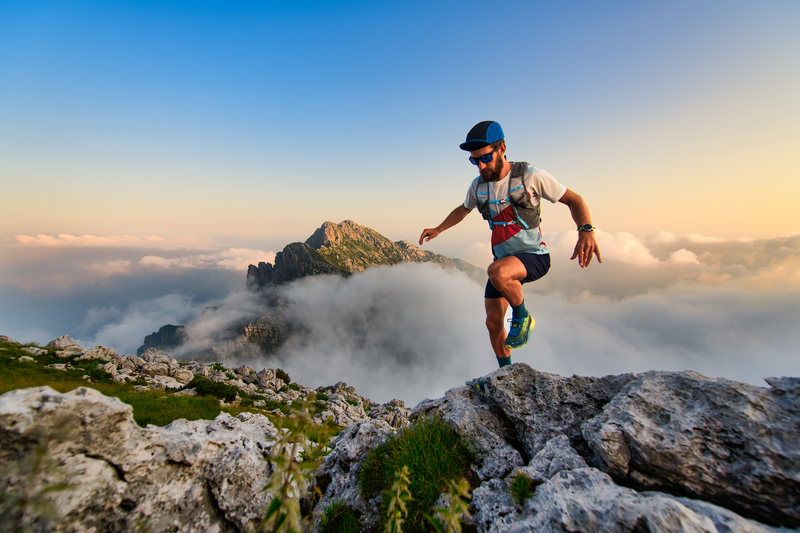
94% of researchers rate our articles as excellent or good
Learn more about the work of our research integrity team to safeguard the quality of each article we publish.
Find out more
ORIGINAL RESEARCH article
Front. Nutr. , 02 December 2022
Sec. Nutritional Immunology
Volume 9 - 2022 | https://doi.org/10.3389/fnut.2022.1060095
This article is part of the Research Topic Nutritional Modulation of Inflammation and Insulin Resistance View all 8 articles
Coronavirus disease 2019 (COVID-19) and diabetes mellitus (DM) are two major diseases threatening human health. The susceptibility of DM patients to COVID-19 and their worse outcomes have forced us to explore efficient routes to combat COVID-19/DM. As the most active form of Vitamin D, 1,25-dihydroxyvitamin D (1,25(OH)2D) has been shown a beneficial effect in the treatment of COVID-19/DM. However, the anti-COVID-19/DM mechanisms of 1,25(OH)2D remain unclear. In this study, an approach combining network pharmacology and molecular docking was performed to reveal the potential hub target genes and underlying mechanisms of 1,25(OH)2D in the treatment of COVID-19/DM. The hub targets and interaction pathways related to 1,25(OH)2D were identified by integrating the key 1,25(OH)2D-target-signaling pathway-COVID-19/DM networks. Fifteen hub targets of 1,25(OH)2D against COVID-19DM were determined, including EGFR, PIK3R1, PIK3CA, STAT3, MAPK1, ESR1, HSP90AA1, LCK, MTOR, IGF1, AR, NFKB1, PIK3CB, PTPN1, and MAPK14. An enrichment analysis of the hub targets further revealed that the effect of 1,25(OH)2D against COVID-19/DM involved multiple biological processes, cellular components, molecular functions and biological signaling pathways. Molecular docking disclosed that 1,25(OH)2D docked nicely with the hub target proteins, including EGFR, PIK3R1, and PIK3CA. These findings suggested that the potential mechanisms of 1,25(OH)2D against COVID-19/DM may be related to multiple biological targets and biological signaling pathways.
The coronavirus disease 2019 (COVID-19) is caused by the severe acute respiratory syndrome coronavirus type 2 (SARS-CoV-2), which brings an unprecedented threat to human health all over the world. As of September 24, 2022, the SARS-CoV-2 had infected approximately 612 million people and had caused at least 6,513 thousand deaths around the world, and both counts are increasing daily. Currently, there are few vaccines, including ChAdOx1 nCoV-19, mRNA-1273, BNT162b2 and CoronaVac, that effectively prevent hundreds of thousands of deaths from SARS-CoV-2. Nevertheless, it was reported that the vaccination program was associated with new vaccine-related adverse reactions, such as hypersensitivity myocarditis (1, 2), Bell's palsy (3), thrombosis and immune thrombocytopenia (4). SARS-CoV-2 attacks cells in the upper and lower airways and then promptly replicates in the infected cells, accompanied by the induction of large amounts of proinflammatory cytokines and chemokines, leading to the so-called cytokine tornado (5). Hence, respiratory symptoms, such as alveolar edema, acute respiratory distress syndrome and vascular leakage, may generally occur in COVID-19 patients. Diabetes mellitus (DM) is a widespread metabolic and endocrine disease, which is likewise another major threat to human health (6). In 2021, the number of DM patients aged 20–79 is estimated to be 536.6 million, and more than 6.7 million died from DM-related causes worldwide (7). Epidemiological investigation demonstrates that DM ranks second among the comorbidities of COVID-19 and that patients with DM are markedly predisposed to SARS-CoV-2 infection (8, 9). In addition, DM is also one of the risk factors most associated with worse outcomes in COVID-19 patients (10, 11). Consequently, there is a crying need to explore effective treatments for COVID-19 patients, particularly for DM patients infected with SARS-CoV-2, which may contribute to improving patient prognosis and survival.
Vitamin D (VD), a fat-soluble vitamin, has been found to be associated with many diseases and VD supplementation has become one of the most common nutritional treatments for these diseases around the world. VD is originally world-renowned for regulating calcium and phosphorus metabolism and promoting bone tissue growth. Recent evidence has revealed that the benefits of VD exceed its effects on the skeletal system, reporting on the endocrine and immune systems (5, 12). Currently, studies have shown that VD has a beneficial effect in altering the progression and severity of DM (13, 14) and COVID-19 patients (15–17) and preventing DM patients from being contaminated with SARS-CoV-2 (18, 19). VD deficiency has been implicated in the pathogenesis of obesity and diabetes, affecting insulin secretion (20, 21) and exacerbating inflammatory states (22). Besides, VD may modulate various pathways of the immune system to fight against COVID-19, including inhibiting entry and replication of SARS-CoV-2, increasing the concentrations of anti-inflammatory cytokines, decreasing the level of proinflammatory cytokines, accelerating the production of natural antimicrobial peptides, and activating defense cells that destroy SARS-CoV-2 (23). Furthermore, VD deficiency is also a risk factor contributing to people infected with SARS-CoV-2 (24). VD is inherently inactive and must be further metabolized into an active form for biological effects by the enzymes vitamin D-25-hydroxylase in the liver and 25-hydroxyvitamin D-1-hydroxylase in the kidney (25). Serves as the most active form of VD, 1,25-dihydroxyvitamin D (1,25(OH)2D) (Figure 1) exerts its biological activities by binding with the target receptor in the target tissue and then leads to up- or down-regulation of the related genes (26). Studies have shown that patients who take 1,25(OH)2D supplementation have an improvement in oxygenation (27), and lower risks of SARS-CoV2 infection, COVID-19 severity and mortality (28). In addition, one study showed that 1,25(OH)2D exhibits significant potent activity against SARS-CoV-2 in human nasal epithelial cells and human hepatoma cells in vitro (29). It was also reported that lower 1,25 (OH)2D levels was significantly associated with DM prevalence in US middle-aged Caucasian men and women (30). Moreover, studies also shown that 1,25(OH)2D supplementation decreased the increase in glycemia, and increased insulin secretion (31, 32), and attenuated diabetic cardiomyopathy (33). Furthermore, there are reports showing that 1,25(OH)2D exerts its anti-diabetic effect by ameliorating inflammation (34) and exerts its anti-microbial effect in a variety of bacteria, viruses and pathogens by regulating the innate and adaptive immune response (5). However, the role of 1,25(OH)2D against COVID-19/DM is still unknown. Therefore, the potential targets and mechanisms of 1,25(OH)2D against COVID-19/DM are needed to be further studied.
Network pharmacology, serves as a drug development tool, plays a crucial role in predicting potential targets and revealing underlying mechanisms in clinical disease therapy (35). In this paper, we aimed at exploring the therapeutic action of 1,25(OH)2D against COVID-19/DM and revealing its pharmacological pathways by combining network pharmacological analysis with molecular docking technology.
The flow-process diagram of the study was shown in Figure 2. The genes related to 1,25(OH)2D were gained from accessible online public databases including the Drugbank database (36) and the Swiss Target Prediction database (37). In addition, we also obtained the genes related to 1,25(OH)2D from the PharmMapper database (38), the SuperPred database (39) and the TargetNet database (40) with a score ≥ 0.6. The protein names were converted to their gene names by utilizing the Uniprot database (41). We accessed the above databases on August 14, 2022.
Further, the genes of DM and COVID-19 were identified by searching on the publicly accessible online databases on August 15, 2022. With the key words including “severe acute respiratory syndrome coronavirus 2,” “SARS-CoV-2,” “coronavirus COVID-19,” “coronavirus Disease 2019,” “COVID-19,” “diabetes mellitus” and “diabetes,” the genes related to COVID-19 and DM were gained from the GeneCards database (https://www.genecards.org/) (42) with a score ≥ 10. Moreover, we also searched the Drugbank database (36), the Online Mendelian Inheritance in Man (OMIM) database (43), the National Center for Biotechnology Information (NCBI) database and the Therapeutic Target database (TTD) (44) for DM and COVID-19 related genes. All the genes related to 1,25(OH)2D, COVID-19 and DM were obtained and imported into a free online website (http://bioinformatics.psb.ugent.be/webtools/Venn/) to predict the potential targets of 1,25(OH)2D against COVID-19/DM were confirmed. Eventually, a group of mutual genes was acquired.
The mutual genes were uploaded to the STRING database to construct protein–protein interaction (PPI) network, and then a tab separated values (TSV) file was obtained with a confidence value of interaction score ≥ 0.9. Further, the TSV file was imported into the Cytoscape software (Version 3.7.1) to visualize the PPI network of the identified genes. Furthermore, the Cytoscape software was also executed to select the hub targets of the identified genes by utilizing the “cytoHubba” function.
The hub targets were imported into the DAVID database (45) for enrichment analysis involving Gene Ontology and Kyoto Encyclopedia of Genes and Genomes pathways enrichment analysis. Ultimately, the GO and KEGG enrichment data related to hub targets were imported into an online tool for visualized analysis including histogram, bubble chart and circle diagram.
The hub targets data in the GO and KEGG pathways of 1,25(OH)2D against COVID-19/DM were uploaded to the Cytoscape software to establish a multiple interaction network diagram of drug–target–GO and KEGG pathway–disease.
Furthermore, the binding affinities of the hub target proteins to 1,25(OH)2D were confirmed by using molecular docking imitation in silico. The 3-dimensional structure file of 1,25(OH)2D was gained from the PubChem database (https://pubchem.ncbi.nlm.nih.gov/), which was further converted to mol2 file by applying the Openbabel software (vision 2.4.1). The mol2 file was imported into the AutoDock Vina program to add hydrogen, set torsion tree and then convert to pdbqt file. In addition, we obtained the 3D structures of the hub target protein from the Protein Data Bank (PDB) database (https://www.rcsb.org/). With AutoDock Vina software, each protein was processed with a few steps, including hydrogen addition and water deletion, and then it was saved as pdbqt files. Grid boxes in the AutoDock Vina software were performed to set the active docking center to include all residues surrounding the original ligand. Finally, the setting of the docking parameters was evaluated by calculating the root mean square deviation (RMSD) of the docked ligand molecule from the original ligand molecule by Pymol software. An RMSD≤4 Å was commonly served as the threshold for matching the docking ligand configuration and the original ligand configuration. Moreover, the docking results were imported into the LigPlot+ software (version 2.2.5) to analyze the hydrophobic interactions between ligands and target proteins.
After excluding duplicates, a total of 312 1,25(OH)2D related genes were obtained. Furthermore, a total of 2,585 COVID-19 related target genes and 2,749 DM related target genes were identified after eliminating duplicates in each cluster (Supplementary Table S1). Eventually, we identified 75 overlapped genes in these three clusters, which were gained by processing with the Venn diagram. Simultaneously, the overlapped genes were further used to construct their interactional network (Figure 3).
Figure 3. Venn diagram of 1,25-dihydroxyvitamin D effects against COVID-19 and diabetes mellitus (DM) (A). Mutual target genes of 1,25-dihydroxyvitamin D and COVID-19/DM for constructing a protein–protein interaction network (B).
In order to construct PPI network and determine the topological parameters of 1,25(OH)2D against COVID-19/DM, the mapped cross-proteins were inputted to the Cytoscape software. Further, the score of maximal clique centrality (MCC) method was applied to determine the hub targets. Finally, the top fifteen targets with a score > 20 were considered as the hub targets, including EGFR, PIK3R1, PIK3CA, STAT3, MAPK1, ESR1, HSP90AA1, LCK, MTOR, IGF1, AR, NFKB1, PIK3CB, PTPN1, and MAPK14 (Figure 4; Supplementary Table S2).
Figure 4. Protein–protein interaction networks and hub target genes. Hub target genes revealed in inner track. The darker the color, the higher the score.
Firstly, the enrichment analysis revealed that the biological processes (BPs) of hub targets were predominantly implicated in signal transduction, positive regulation of gene expression, positive regulation of lamellipodium assembly, positive regulation of protein kinase B signaling, positive regulation of protein import into nucleus, phosphatidylinositol 3-kinase signaling, positive regulation of transcription, DNA-templated, positive regulation of transcription from RNA polymerase II promoter, platelet activation, positive regulation of smooth muscle cell proliferation, transcription from RNA polymerase II promoter, positive regulation of cell migration, negative regulation of gene expression, negative regulation of gene expression, response to muscle stretch, phosphorylation, regulation of multicellular organism growth, positive regulation of glucose import, negative regulation of apoptotic process, and phosphatidylinositol-mediated signaling. In addition, the hub targets related to cellular components (CCs) were principally involved in macromolecular complex, cytosol, cytosol, phosphatidylinositol 3-kinase complex, class IA, phosphatidylinositol 3-kinase complex, plasma membrane, nucleus, nucleoplasm, secretory granule lumen, ficolin-1-rich granule lumen, membrane, perinuclear region of cytoplasm, glutamatergic synapse, chromatin, extracellular region, and mitochondrion. Moreover, the hub targets associated with molecular functions (MFs) were primarily engaged in protein phosphatase binding, enzyme binding, RNA polymerase II transcription factor activity, ligand-activated sequence-specific DNA binding, nitric-oxide synthase regulator activity, kinase activity, protein kinase binding, insulin receptor substrate binding, ATPase binding, ATP binding, identical protein binding, insulin receptor binding, transcription factor binding, transcription coactivator binding, phosphotyrosine binding, DNA binding, protein serine/threonine kinase activity, protein binding, phosphatidylinositol 3-kinase activity, chromatin binding, and transcriptional activator activity, RNA polymerase II transcription regulatory region sequence-specific binding (Figures 5A–C; Supplementary Tables S3–5). Finally, a total of 120 KEGG pathways related to hub targets were identified as well (p < 0.05), including PD-L1 expression and PD-1 checkpoint pathway in cancer, prostate cancer, chemical carcinogenesis-receptor activation, endocrine resistance, HIF-1 signaling pathway, proteoglycans in cancer, prolactin signaling pathway, pancreatic cancer, EGFR tyrosine kinase inhibitor resistance, pathways in cancer, growth hormone synthesis, secretion and action, acute myeloid leukemia, FoxO signaling pathway, human cytomegalovirus infection, glioma, breast cancer, AGE-RAGE signaling pathway in diabetic complications, progesterone-mediated oocyte maturation, T cell receptor signaling pathway, and Kaposi sarcoma-associated herpesvirus infection (Figures 6A–C; Supplementary Table S6).
Figure 5. Gene ontology enrichment analysis findings in 1,25-dihydroxyvitamin D against COVID-19/DM. Bar charts of biological processes, cellular components, and molecular functions (A), bubble chart of biological processes, cellular components, and molecular functions (B), circle chart of hub targets and biological processes (C).
Figure 6. Kyoto Encyclopedia of genes and genomes enrichment analysis findings in 1,25-dihydroxyvitamin D against COVID-19/DM. Bar chart of KEGG pathways (A), bubble chart of KEGG pathways (B), circle chart of hub targets and KEGG pathways (C).
A multiple interaction network diagram of drug–target–GO and KEGG pathway–disease was constructed by using the Cytoscape software, revealing that the role of 1,25(OH)2D against COVID-19/DM was involved in multiple BPs, CCs, MFs, and KEGG pathways (Figure 7).
The highest scoring hub targets, including EGFR (PBD ID: 5HG8), PIK3R1 (PBD ID: 4JPS) and PIK3CA (PBD ID: 6PYS), were selected for docking validation. The parameters in the grid box of EGFR were set as 12.724, −3.444, and −30.049 for the center-x-y-z and 15, 15, and 15 for the size-x-y-z. The original ligand 634 docked with the 5HG8 protein by combining with the amino acid residues GLN-791 (2.0 Å) and MET-793 (2.0 Å) (Figure 8A). The RMSD and free bonding energy of the 634 were 0.221 Å and −8.5 kcal/mol, respectively. The hydrogen bond formation between 1,25(OH)2D and the 5HG8 protein involved the amino acid residue MET-793 (3.0 Å) (Figure 8B) with −7.1 kcal/mol free bonding energy. In addition, the parameters in the grid box of PIK3R1 were set as −1.166, −8.926, and 16.981 for center-x-y-z and 15, 15, and 17.25 for size-x-y-z. The original ligand 1LT docked with the 4JPS protein by bonding with the amino acid residues SER-854 (1.9 Å), VAL-851 (2.1 Å, 2.5 Å) and GLN-859 (1.9 Å, 2.9 Å) (Figure 8C). The RMSD and free bonding energy of the 1LT were 0.095 Å and −10.3 kcal/mol, respectively. The hydrogen bond formation between 1,25(OH)2D and the 4JPS protein included the amino acid residues ARG-770 (3.2 Å) and LYS-802 (3.0 Å) (Figure 8D) with −7.7 kcal/mol free bonding energy. Furthermore, the parameters in the grid box of PIK3CA are set as −19.077, 11.460, and 28.185 for center-x-y-z and 15, 15, and 15 for size-x-y-z. The original ligand P5J docked with the 6YPS protein by docking with the amino acid residues LYS-802 (2.9 Å) and GLU-849 (2.9 Å) (Figure 8E). The RMSD and free bonding energy of the P5J were 0.006 Å and −11.3 kcal/mol, respectively. The hydrogen bond formation between 1,25(OH)2D and the 6YPS protein involved the amino acid residue VAL-851 (3.1 Å) (Figure 8F) with −8.1 kcal/mol free bonding energy.
Figure 8. Molecular docking details of the original ligands and 1,25-dihydroxyvitamin D to the target protein. 634-5HG8 (A), 1,25-dihydroxyvitamin D-5HG8 (B), 1LT-4JPS (C), 1,25-dihydroxyvitamin D-4JPS (D), P5J-6PYS (E), 1,25-dihydroxyvitamin D-6PYS (F).
Furthermore, we performed an analysis of the hydrophobic interactions between the ligands and the target proteins by using the LigPlot+ software. The hydrophobic amino acids of the docking domain of the 5GH8 protein, which engage in the hydrophobic interactions with the ligand 634, are Cys775, Leu844, Phe856, Val726, Cys797, Arg841, Ala743, Met 790, Gly796, Leu718, and Gly 719 (Figure 9A), and with 1,25(OH)2D are Arg841, Leu718, Phe856, Gly719, Val726, Leu844, Leu792, Leu799, Asp800, Cys797, Ser720, and Gly796 (Figure 9B). Similarly, the ligand 1LT was found to interact with the docking domain of the 4JPS protein through nine hydrophobic amino acids, including Val850, Trp780, Try836, Met 922, Phe930, Ile932, His855, Ile848, and Ile800 (Figure 9C), and 1,25(OH)2D was found to interact more effectively with the docking domain of the 4JPS protein through thirteen hydrophobic amino acids, including Val851, Gln859, Ile800, Tyr836, Ile848, Ser774, Ile932, Met772, Pro778, Ser854, Trp780, Val850, and Met922 (Figure 9D). Equivalently, the hydrophobic interactions between the ligand P5J and the docking domain of the 6PYS protein are through fifteen hydrophobic amino acids, including Val851, Val850, Glu849, Ile932, Ile848, Tyr836, Asp933, Ser774, Trp780, Met922, Gln859, Ile800, Met772, Thr856, and Pro778 (Figure 9E). The hydrophobic interactions between 1,25(OH)2D and the docking domain of the 6PYS protein are through fourteen hydrophobic amino acids, including Thr856, Glu849, Ser774, Val850, Gln859, Ile932, Trp780, Met772, Asp933, Tyr836, Met922, Ile800, Phe930, and Ile848 (Figure 9F). From the above results, it is clear that the original ligands and the docked ligands share many hydrophobic amino acids with the target proteins.
Figure 9. The hydrophobic interactions of original ligands and 1,25-dihydroxyvitamin D with the target protein. Hydrophobic interactions are indicated by red opposite arcs. Hydrogen bonds are shown as green dashed lines. 634-5HG8 (A), 1,25-dihydroxyvitamin D-5HG8 (B), 1LT-4JPS (C), 1,25-dihydroxyvitamin D-4JPS (D), P5J-6PYS (E), 1,25-dihydroxyvitamin D-6PYS (F).
Since the first outbreak of COVID-19 in China, a lot of attention has been focused on people with DM due to the worse outcomes among those infected (46). The large amount of proinflammatory cytokines and chemokines formed after SARS-CoV-2 infection may contribute to increasing the risk of poor prognosis in DM patients (5, 46). In this paper, the combination of bioinformatics analysis, network pharmacology and molecular docking was performed to determine the potential targets, biological function and signaling pathways of 1,25(OH)2D in the treatment of COVID-19/DM. Firstly, fifteen hub targets of 1,25(OH)2D against COVID-19/DM included EGFR, PIK3R1, PIK3CA, STAT3, MAPK1, ESR1, HSP90AA1, LCK, MTOR, IGF1, AR, NFKB1, PIK3CB, PTPN1, and MAPK14 were identified by applying network pharmacology that is a frequently-used approach to pharmaceutical research for multiple diseases included COVID-19 and DM. Previous studies indicated that most of these hub targets may be vital to clarify the therapeutic mechanism of COVID-19 and DM diseases.
EGFR, serves as a member of the epidermal growth factor receptor (HER) family, has physiological functions in regulating epithelial tissue development and homeostasis (47). Numerous cells in the lung infected with SARS-CoV-2 cause EGFR overexpression, which contributes to worsening the pulmonary disease and triggering fibrosis (48). In addition, deletion of EGFR in podocytes also leads to delaying the development of diabetic nephropathy (49). EGFR activation also leads to STAT3 phosphorylation, which can induce inflammatory responses and imbalanced anti-virus adaptive immune responses, inhibit anti-virus interferon responses, and promote M2 macrophage polarization, pulmonary fibrosis, and thrombosis (50). Besides, activation of STAT3 not only contributes to involvement in the development of diabetic insulin resistance in DM (51) but also participates in the progress of diabetes-associated cardiovascular disease, such as myocardial interstitial fibrosis and myocardial infarction (52, 53). The Class I phosphoinositide 3-kinases (PI3Ks) included PIK3R1, PIK3CA, and PIK3CB, are a group of heterodimeric lipid kinases that regulate vital cellular processes involving survival, growth, proliferation, and metabolism (54). Previous studies show that PI3Ks play a crucial role in regulating the development of DM (55–57).
Our results also revealed that the mitogen-activated protein kinase family members, such as MAPK1 and MAPK14, were potential targets of 1,25(OH)2D against COVID-19/DM. These two targets can be activated by provoking phosphorylation during changes in the internal environment, including oxidative stress, osmotic viral infection, inflammatory factors and pressure changes (58). ESR1 is a crucial sex factor that provides a protective umbrella to COVID-19 patients by suppressing the immune and inflammatory responses induced by SARS-CoV-2 infection (59) and protects DM patients by improving glycemic homeostasis (60). In addition, recent studies reveal that inhibition of HSP90AA1 activity contributes to reducing SARS-CoV- 2 viral replication and tumor necrosis factor (TNF) mRNA levels (61), and enhancing glucose-stimulated insulin secretion and expressions of genes tightly associated with β-cell function (62). LCK, serves as a cytoplasmic tyrosine kinase, is expressed in natural killer cells and T cells and plays an irreplaceable role in activating T cells. Hence, selective inhibition of LCK expression can lead to immunosuppression (63). MTOR is a key protein in the mTOR pathway that appears to play a crucial role in regulating the immune dysregulation processes underlying COVID-19 in diabetic patients (64). In addition, continuous hyperactivation of MTOR in diabetic myocardium has been demonstrated, which makes MTOR become a potential target in the prevention of diabetes-related cardiovascular disease (53). Current evidence also shows that MTOR activation plays a crucial role in the pathogenesis of insulin resistance in type 2 diabetes mellitus (T2DM) (65, 66). Furthermore, recent studies show that higher IGF1 level is associated with lower incidence of T2DM and lower risk of COVID-19 mortality (64, 67).
In the next section of this paper, the enrichment analysis of hub targets revealed their importance in diverse biological processes. In this paper, we focused on the functions and signaling pathways involved in the pathogenesis of COVID-19/DM. The signaling pathways, including HIF-1 signaling pathway, FoxO signaling pathway, T cell receptor signaling pathway, PI3K-Akt signaling pathway and so on (Figure 4; Supplementary Table S6), were found to be targeted by 1,25(OH)2D. HIF-1 acts as a regulator of oxygen homeostasis and has been reported to enhance immunity by regulating the tasks of neutrophils, lymphocytes, dendritic cells and macrophages, which are disrupted in COVID-19 infections, contributing to hypoxic conditions (68). Research has also demonstrated that inhibition or activation of the HIF-1 signaling pathway was associated with β-cell dysfunction, insulin resistance and glucose intolerance (69). The FoxO signaling pathway plays a critical role in multifarious physiological and cellular processes, including cell proliferation, regulation of programmed cell death, cell cycle regulation, and regulation of glycogenolysis and gluconeogenesis. Fang et al. found that FoxO signaling pathway was related to diabetic complications and COVID-19 by analyzing the differentially expressed genes in those patients (70). In addition, the complex molecular mechanism mediated by T cell receptor signaling pathway can cause the activation of T cells, thereby affecting the immune response of the body (71). It was reported that T cell receptor signaling pathway might represent the main pathological mechanism of gestational diabetes mellitus (72). Furthermore, the PI3K-Akt signaling pathway has been confirmed to be participated in a variety of cellular processes including synthesis, glucose transport and breakdown in DM (73), and also to be involved in the pathogenesis of pulmonary fibrosis and immune response process of the host cell to resist viral invasion (74).
Finally, the interaction of 1,25(OH)2D and its targets was identified by conducting molecular docking. Molecular docking is a frequently used tool for predicting possible interactions between two molecules and is a potent approach for structure-based drug discovery (75). In this paper, we applied molecular docking to analyze the binding affinity of 1,25(OH)2D to EGFR, PIK3R1, and PIK3CA. Our results revealed that 1,25(OH)2D has a strong binding affinity with these three targets by forming of hydrogen bonds and hydrophobic interactions, indicating the drug-protein interaction and the anti-COVID-19/DM activity of 1,25(OH)2D. Nonetheless, all of the findings in this paper were predicted only by integrated bioinformatics analysis and in silico approaches. However, the current network information technology is not comprehensive enough, and the real-time update and accuracy of these databases need to be improved. Thus, all of the findings obtained in this paper should be further validated from a pharmacodynamic perspective, and preclinical studies are needed to account for the multiple biological targets and biological signaling pathways of 1,25(OH)2D in the treatment of COVID-19/DM.
In summary, our results identified the possible hub targets and underlying pharmacological mechanisms of 1,25(OH)2D in the treatment of COVID-19/DM by applying network pharmacology and molecular docking. The hub targets including EGFR, PIK3R1, PIK3CA, STAT3, MAPK1, etc., and the biological signaling pathways including HIF-1 signaling pathway, FoxO signaling pathway, T cell receptor signaling pathway, PI3K-Akt signaling pathway, etc. involved in these targets are considered to play a critical role in 1,25(OH)2D against COVID-19/DM. Therefore, the effect of 1,25(OH)2D in the treatment of COVID-19/DM may be achieved by targeting multiple biological targets and biological signaling pathways. However, preclinical validation is required to confirm these findings in further studies.
The original contributions presented in the study are included in the article/Supplementary material, further inquiries can be directed to the corresponding authors.
FZ, ZY, and CW conceived and designed the study and drafted the manuscript. FZ, YX, and CT performed the data analysis and drew charts and graphs. FZ and CT performed the bioinformatics and statistical analyses. All authors contributed to this paper and approved the published version of the manuscript.
This study was funded by the National Natural Science Foundation of China (Grant No. 81960705).
The authors declare that the research was conducted in the absence of any commercial or financial relationships that could be construed as a potential conflict of interest.
All claims expressed in this article are solely those of the authors and do not necessarily represent those of their affiliated organizations, or those of the publisher, the editors and the reviewers. Any product that may be evaluated in this article, or claim that may be made by its manufacturer, is not guaranteed or endorsed by the publisher.
The Supplementary Material for this article can be found online at: https://www.frontiersin.org/articles/10.3389/fnut.2022.1060095/full#supplementary-material
1. Käsk L, Larsen TH, Schjøtt J, Saeed S. The possibility of hypersensitivity myocarditis following COVID-19 vaccines: implications for contrast echocardiography. Cardiology. (2022) 147:417–8. doi: 10.1159/000525349
2. Kounis NG, Mplani V, Koniari I, Velissaris D. Hypersensitivity myocarditis and COVID-19 vaccines. Kardiol Pol. (2022) 80:109–10. doi: 10.33963/KP.a2021.0166
3. Wan EYF, Chui CSL, Lai FTT, Chan EWY, Li X, Yan VKC, et al. Bell's palsy following vaccination with mRNA (BNT162B2) and inactivated (Coronavac) SARS-CoV-2 vaccines: a case series and nested case-control study. Lancet Infect Dis. (2022) 22:64–72. doi: 10.1016/S1473-3099(21)00451-5
4. National Institute for Health and Care Excellence: Guidelines. COVID-19 Rapid Guideline: Vaccine-Induced Immune Thrombocytopenia and Thrombosis (Vitt). London: National Institute for Health and Care Excellence (NICE) Copyright © NICE 2021. (2022).
5. Bilezikian JP, Bikle D, Hewison M, Lazaretti-Castro M, Formenti AM, Gupta A, et al. Mechanisms in endocrinology: vitamin D and COVID-19. Eur J Endocrinol. (2020) 183:R133–r47. doi: 10.1530/EJE-20-0665
6. Zimmet PZ, Magliano DJ, Herman WH, Shaw JE. Diabetes: a 21st century challenge. Lancet Diabetes Endocrinol. (2014) 2:56–64. doi: 10.1016/S2213-8587(13)70112-8
7. Idf Diabetes Atlas. Brussels: International Diabetes Federation © International Diabetes Federation (2021).
8. Muniyappa R, Gubbi S. COVID-19 pandemic, coronaviruses, and diabetes mellitus. Am J Physiol Endocrinol Metab. (2020) 318:E736–e41. doi: 10.1152/ajpendo.00124.2020
9. Li X, Xu S, Yu M, Wang K, Tao Y, Zhou Y, et al. Risk factors for severity and mortality in adult COVID-19 inpatients in Wuhan. J Allergy Clin Immunol. (2020) 146:110–8. doi: 10.1016/j.jaci.2020.04.006
10. Zhou F, Yu T, Du R, Fan G, Liu Y, Liu Z, et al. Clinical course and risk factors for mortality of adult inpatients with COVID-19 in Wuhan, China: a retrospective cohort study. Lancet. (2020) 395:1054–62. doi: 10.1016/S0140-6736(20)30566-3
11. Singh AK, Khunti K. COVID-19 and diabetes. Annu Rev Med. (2022) 73:129–47. doi: 10.1146/annurev-med-042220-011857
12. Park JE, Pichiah PBT, Cha YS. Vitamin D and metabolic diseases: growing roles of vitamin D. J Obesity Metab Syndr. (2018) 27:223–32. doi: 10.7570/jomes.2018.27.4.223
13. Lemieux P, Weisnagel SJ, Caron AZ, Julien AS, Morisset AS, Carreau AM, et al. Effects of 6-month vitamin D supplementation on insulin sensitivity and secretion: a randomised, placebo-controlled trial. Eur J Endocrinol. (2019) 181:287–99. doi: 10.1530/EJE-19-0156
14. Zhang Y, Tan H, Tang J, Li J, Chong W, Hai Y, et al. Effects of vitamin D supplementation on prevention of type 2 diabetes in patients with prediabetes: a systematic review and meta-analysis. Diabetes Care. (2020) 43:1650–8. doi: 10.2337/dc19-1708
15. Annweiler C, Beaudenon M, Gautier J, Gonsard J, Boucher S, Chapelet G, et al. High-dose versus standard-dose vitamin D supplementation in older adults with COVID-19 (Covit-Trial): a multicenter, open-label, randomized controlled superiority trial. PLoS Med. (2022) 19:e1003999. doi: 10.1371/journal.pmed.1003999
16. Bae JH, Choe HJ, Holick MF, Lim S. Association of vitamin D status with COVID-19 and its severity: vitamin D and COVID-19: a narrative review. Rev Endocr Metab Disord. (2022) 23:579–99. doi: 10.1007/s11154-021-09705-6
17. Kaya MO, Pamukçu E, Yakar B. The role of vitamin D deficiency on COVID-19: a systematic review and meta-analysis of observational studies. Epidemiol Health. (2021) 43:e2021074. doi: 10.4178/epih.e2021074
18. Ahmed AS, Alotaibi WS, Aldubayan MA, Alhowail AH, Al-Najjar AH, Chigurupati S, et al. Factors affecting the incidence, progression, and severity of COVID-19 in type 1 diabetes mellitus. Biomed Res Int. (2021) 2021:1676914. doi: 10.1155/2021/1676914
19. Singh SK, Jain R, Singh S. Vitamin D deficiency in patients with diabetes and COVID-19 infection. Diabetes Metab Syndr. (2020) 14:1033–5. doi: 10.1016/j.dsx.2020.06.071
20. Leung PS. The potential protective action of vitamin D in hepatic insulin resistance and pancreatic islet dysfunction in type 2 diabetes mellitus. Nutrients. (2016) 8:147. doi: 10.3390/nu8030147
21. Pramono A, Jocken JWE, Blaak EE. Vitamin D deficiency in the aetiology of obesity-related insulin resistance. Diabetes Metab Res Rev. (2019) 35:e3146. doi: 10.1002/dmrr.3146
22. Vondra K, Hampl R. Vitamin D and new insights into pathophysiology of type 2 diabetes. Horm Mol Biol Clin Investig. (2021) 42:203–8. doi: 10.1515/hmbci-2020-0055
23. Kumar R, Rathi H, Haq A, Wimalawansa SJ, Sharma A. Putative roles of vitamin D in modulating immune response and immunopathology associated with COVID-19. Virus Res. (2021) 292:198235. doi: 10.1016/j.virusres.2020.198235
24. Mitchell F. Vitamin-D and COVID-19: do deficient risk a poorer outcome? Lancet Diabetes Endocrinol. (2020) 8:570. doi: 10.1016/S2213-8587(20)30183-2
25. Charoenngam N, Holick MF. Immunologic effects of vitamin D on human health and disease. Nutrients. (2020) 12:2097. doi: 10.3390/nu12072097
26. Haussler MR, Haussler CA, Jurutka PW, Thompson PD, Hsieh JC, Remus LS, et al. The vitamin D hormone and its nuclear receptor: molecular actions and disease states. J Endocrinol. (1997) 154:S57–73.
27. Elamir YM, Amir H, Lim S, Rana YP, Lopez CG, Feliciano NV, et al. A randomized pilot study using calcitriol in hospitalized COVID-19 patients. Bone. (2022) 154:116175. doi: 10.1016/j.bone.2021.116175
28. Oristrell J, Oliva JC, Subirana I, Casado E, Domínguez D, Toloba A, et al. Association of calcitriol supplementation with reduced COVID-19 mortality in patients with chronic kidney disease: a population-based study. Biomedicines. (2021) 9:509. doi: 10.3390/biomedicines9050509
29. Mok CK, Ng YL, Ahidjo BA, Hua Lee RC, Choy Loe MW, Liu J, et al. Calcitriol, the Active Form of Vitamin D, Is a Promising Candidate for COVID-19 Prophylaxis (2020). Available online at: https://www.researchgate.net/publication/342388949
30. Brock KE, Huang WY, Fraser DR, Ke L, Tseng M, Mason RS, et al. Diabetes prevalence is associated with serum 25-hydroxyvitamin D and 1,25-dihydroxyvitamin D in us middle-aged Caucasian men and women: a cross-sectional analysis within the prostate, lung, colorectal and ovarian cancer screening trial. Br J Nutr. (2011) 106:339–44. doi: 10.1017/S0007114511001590
31. Eftekhari MH, Akbarzadeh M, Dabbaghmanesh MH, Hasanzadeh J. Impact of treatment with oral calcitriol on glucose indices in type 2 diabetes mellitus patients. Asia Pac J Clin Nutr. (2011) 20:521–6. doi: 10.6133/apjcn.2011.20.4.04
32. Kjalarsdottir L, Tersey SA, Vishwanath M, Chuang JC, Posner BA, Mirmira RG, et al. 1,25-dihydroxyvitamin D enhances glucose-stimulated insulin secretion in mouse and human islets: a role for transcriptional regulation of voltage-gated calcium channels by the vitamin D receptor. J Steroid Biochem Mol Biol. (2019) 185:17–26. doi: 10.1016/j.jsbmb.2018.07.004
33. Lee TW, Kao YH, Lee TI, Chang CJ, Lien GS, Chen YJ. Calcitriol modulates receptor for advanced glycation end products (rage) in diabetic hearts. Int J Cardiol. (2014) 173:236–41. doi: 10.1016/j.ijcard.2014.02.041
34. Tian LQ, Yu YT, Jin MD, Duan HL, Huang G, Zhang ML. Early 1,25-dihydroxyvitamin D supplementation effectively lowers the incidence of type 2 diabetes mellitus via ameliorating inflammation in Kk-a(Y) Mice. J Nutr Sci Vitaminol. (2021) 67:84–90. doi: 10.3177/jnsv.67.84
35. Li R, Li Y, Liang X, Yang L, Su M, Lai KP. Network pharmacology and bioinformatics analyses identify intersection genes of niacin and COVID-19 as potential therapeutic targets. Brief Bioinform. (2021) 22:1279–90. doi: 10.1093/bib/bbaa300
36. Wishart DS, Feunang YD, Guo AC, Lo EJ, Marcu A, Grant JR, et al. Drugbank 5.0: a major update to the drugbank database for 2018. Nucleic Acids Res. (2018) 46:D1074–82. doi: 10.1093/nar/gkx1037
37. Daina A, Michielin O, Zoete V. Swisstargetprediction: updated data and new features for efficient prediction of protein targets of small molecules. Nucleic Acids Res. (2019) 47:W357–64. doi: 10.1093/nar/gkz382
38. Wang X, Shen Y, Wang S, Li S, Zhang W, Liu X, et al. Pharmmapper 2017 update: a web server for potential drug target identification with a comprehensive target pharmacophore database. Nucleic Acids Res. (2017) 45:W356–60. doi: 10.1093/nar/gkx374
39. Nickel J, Gohlke BO, Erehman J, Banerjee P, Rong WW, Goede A, et al. Superpred: update on drug classification and target prediction. Nucleic Acids Res. (2014) 42:W26–31. doi: 10.1093/nar/gku477
40. Yao ZJ, Dong J, Che YJ, Zhu MF, Wen M, Wang NN, et al. Targetnet: a web service for predicting potential drug-target interaction profiling via multi-target sar models. J Comput Aided Mol Des. (2016) 30:413–24. doi: 10.1007/s10822-016-9915-2
41. Uniprot: the universal protein knowledgebase in 2021. Nucleic Acids Res. (2021) 49:D480–9. doi: 10.1093/nar/gkaa1100
42. Stelzer G, Rosen N, Plaschkes I, Zimmerman S, Twik M, Fishilevich S, et al. The genecards suite: from gene data mining to disease genome sequence analyses. Curr Protocols Bioinform. (2016) 54:1.30.1–1.30.3. doi: 10.1002/cpbi.5
43. Amberger JS, Bocchini CA, Schiettecatte F, Scott AF, Hamosh A. Omim.Org: online mendelian inheritance in man (Omim®), an online catalog of human genes and genetic disorders. Nucleic Acids Res. (2015) 43:D789–98. doi: 10.1093/nar/gku1205
44. Zhou Y, Zhang Y, Lian X, Li F, Wang C, Zhu F, et al. Therapeutic target database update 2022: facilitating drug discovery with enriched comparative data of targeted agents. Nucleic Acids Res. (2022) 50:D1398–d407. doi: 10.1093/nar/gkab953
45. Sherman BT, Hao M, Qiu J, Jiao X, Baseler MW, Lane HC, et al. David: a web server for functional enrichment analysis and functional annotation of gene lists (2021 update). Nucleic Acids Res. (2022) 50:W216–21. doi: 10.1093/nar/gkac194
46. Apicella M, Campopiano MC, Mantuano M, Mazoni L, Coppelli A, Del Prato S. COVID-19 in people with diabetes: understanding the reasons for worse outcomes. Lancet Diabetes Endocrinol. (2020) 8:782–92. doi: 10.1016/S2213-8587(20)30238-2
47. Mendelsohn J, Baselga J. Epidermal growth factor receptor targeting in cancer. Semin Oncol. (2006) 33:369–85. doi: 10.1053/j.seminoncol.2006.04.003
48. Londres HD, Armada JJ, Martínez AH, Abdo Cuza AA, Sánchez YH, Rodríguez AG, et al. Blocking EGFR with nimotuzumab: a novel strategy for COVID-19 treatment. Immunotherapy. (2022) 14:521–30. doi: 10.2217/imt-2022-0027
49. Li Y, Pan Y, Cao S, Sasaki K, Wang Y, Niu A, et al. Podocyte EGFR inhibits autophagy through upregulation of rubicon in type 2 diabetic nephropathy. Diabetes. (2021) 70:562–76. doi: 10.2337/db20-0660
50. Jafarzadeh A, Nemati M, Jafarzadeh S. Contribution of STAT3 to the pathogenesis of COVID-19. Microb Pathog. (2021) 154:104836. doi: 10.1016/j.micpath.2021.104836
51. Xu L, Li Y, Yin L, Qi Y, Sun H, Sun P, et al. miR-125a-5p ameliorates hepatic glycolipid metabolism disorder in type 2 diabetes mellitus through targeting of STAT3. Theranostics. (2018) 8:5593–609. doi: 10.7150/thno.27425
52. Wang L, Li J, Li D. Losartan reduces myocardial interstitial fibrosis in diabetic cardiomyopathy rats by inhibiting JAK/STAT signaling pathway. Int J Clin Exp Pathol. (2015) 8:466–73.
53. Samidurai A, Roh SK, Prakash M, Durrant D, Salloum FN, Kukreja RC, et al. STAT3-miR-17/20 signalling axis plays a critical role in attenuating myocardial infarction following rapamycin treatment in diabetic mice. Cardiovasc Res. (2020) 116:2103–15. doi: 10.1093/cvr/cvz315
54. Rathinaswamy MK, Burke JE. Class I phosphoinositide 3-kinase (Pi3k) regulatory subunits and their roles in signaling and disease. Adv Biol Regul. (2020) 75:100657. doi: 10.1016/j.jbior.2019.100657
55. Cai W, Zhang J, Yang J, Fan Z, Liu X, Gao W, et al. MicroRNA-24 attenuates vascular remodeling in diabetic rats through PI3K/Akt signaling pathway. Nutr Metab Cardiovasc Dis. (2019) 29:621–32. doi: 10.1016/j.numecd.2019.03.002
56. Yang X, Li X, Lin Q, Xu Q. Up-regulation of microRNA-203 inhibits myocardial fibrosis and oxidative stress in mice with diabetic cardiomyopathy through the inhibition of PI3K/Akt signaling pathway via PIK3CA. Gene. (2019) 715:143995. doi: 10.1016/j.gene.2019.143995
57. Clément K, Le Stunff C, Meirhaeghe A, Dechartres A, Ferrieres J, Basdevant A, et al. In obese and non-obese adults, the cis-regulatory rs361072 promoter variant of PIK3CB is associated with insulin resistance not with type 2 diabetes. Mol Genet Metab. (2009) 96:129–32. doi: 10.1016/j.ymgme.2008.11.160
58. Li J, Zhang K, Bao J, Yang J, Wu C. potential mechanism of action of Jing Fang Bai Du San in the treatment of COVID-19 using docking and network pharmacology. Int J Med Sci. (2022) 19:213–24. doi: 10.7150/ijms.67116
59. Li F, Boon ACM, Michelson AP, Foraker RE, Zhan M, Payne PRO. Estrogen hormone is an essential sex factor inhibiting inflammation and immune response in COVID-19. Sci Rep. (2022) 12:9462. doi: 10.1038/s41598-022-13585-4
60. Gregorio KCR, Laurindo CP, Machado UF. Estrogen and glycemic homeostasis: the fundamental role of nuclear estrogen receptors ESR1/ESR2 in glucose transporter GLUT4 regulation. Cells. (2021) 10:99. doi: 10.3390/cells10010099
61. Wyler E, Mösbauer K, Franke V, Diag A, Gottula LT, Arsiè R, et al. Transcriptomic profiling of SARS-CoV-2 infected human cell lines identifies HSP90 as target for COVID-19 therapy. iScience. (2021) 24:102151. doi: 10.1016/j.isci.2021.102151
62. Yang X, Zhang Y, Xu W, Deng R, Liu Y, Li F, et al. Potential role of HSP90 in rat islet function under the condition of high glucose. Acta Diabetol. (2016) 53:621–8. doi: 10.1007/s00592-016-0852-2
63. Vico-Barranco I, Arbulo-Echevarria MM, Serrano-García I, Pérez-Linaza A, Miranda-Sayago JM, Miazek A, et al. A novel, LAT/Lck double deficient T cell subline J.Cam1.7 for combined analysis of early TCR signaling. Cells. (2021) 10:343. doi: 10.3390/cells10020343
64. Pinchera B, Scotto R, Buonomo AR, Zappulo E, Stagnaro F, Gallicchio A, et al. Diabetes and COVID-19: the potential role of mTOR. Diabetes Res Clin Pract. (2022) 186:109813. doi: 10.1016/j.diabres.2022.109813
65. Laplante M, Sabatini DM. mTOR signaling in growth control and disease. Cell. (2012) 149:274–93. doi: 10.1016/j.cell.2012.03.017
66. Howell JJ, Manning BD. mTOR couples cellular nutrient sensing to organismal metabolic homeostasis. Trends Endocrinol Metab. (2011) 22:94–102. doi: 10.1016/j.tem.2010.12.003
67. Fan X, Yin C, Wang J, Yang M, Ma H, Jin G, et al. Pre-diagnostic circulating concentrations of insulin-like growth factor-1 and risk of COVID-19 mortality: results from UK Biobank. Eur J Epidemiol. (2021) 36:311–8. doi: 10.1007/s10654-020-00709-1
68. Khanal P, Duyu T, Patil BM, Dey YN, Pasha I, Wanjari M, et al. Network pharmacology of AYUSH recommended immune-boosting medicinal plants against COVID-19. J Ayurveda Integr Med. (2022) 13:100374. doi: 10.1016/j.jaim.2020.11.004
69. Gonzalez FJ, Xie C, Jiang C. The role of hypoxia-inducible factors in metabolic diseases. Nat Rev Endocrinol. (2018) 15:21–32. doi: 10.1038/s41574-018-0096-z
70. Fang L, Tang T, Hu M. Identification of differentially expressed genes in COVID-19 and integrated bioinformatics analysis of signaling pathways. Genet Res. (2021) 2021:2728757. doi: 10.1155/2021/2728757
71. Alcover A, Alarcón B, Di Bartolo V. Cell biology of T cell receptor expression and regulation. Annu Rev Immunol. (2018) 36:103–25. doi: 10.1146/annurev-immunol-042617-053429
72. Chen YM, Zhu Q, Cai J, Zhao ZJ, Yao BB, Zhou LM, et al. Upregulation of T cell receptor signaling pathway components in gestational diabetes mellitus patients: joint analysis of mRNA and circRNA expression profiles. Front Endocrinol. (2021) 12:774608. doi: 10.3389/fendo.2021.774608
73. Mahmoudi A, Atkin SL, Nikiforov NG, Sahebkar A. Therapeutic role of curcumin in diabetes: an analysis based on bioinformatic findings. Nutrients. (2022) 14:3244. doi: 10.3390/nu14153244
74. Yang F, Chen R, Li WY, Zhu HY, Chen XX, Hou ZF, et al. D-limonene is a potential monoterpene to inhibit PI3K/Akt/IKK-?/NF-κB P65 signaling pathway in coronavirus disease 2019 pulmonary fibrosis. Front Med. (2021) 8:591830. doi: 10.3389/fmed.2021.591830
Keywords: COVID-19, diabetes mellitus, bioinformatics, network pharmacology, molecular docking, 1,25-dihydroxyvitamin D
Citation: Zeng F, Xu Y, Tang C, Yan Z and Wei C (2022) Integrated bioinformatics and in silico approaches reveal the biological targets and molecular mechanisms of 1,25-dihydroxyvitamin D against COVID-19 and diabetes mellitus. Front. Nutr. 9:1060095. doi: 10.3389/fnut.2022.1060095
Received: 02 October 2022; Accepted: 17 November 2022;
Published: 02 December 2022.
Edited by:
Carol Gois Leandro, Federal University of Pernambuco, BrazilReviewed by:
Pamela Senesi, University of Milan, ItalyCopyright © 2022 Zeng, Xu, Tang, Yan and Wei. This is an open-access article distributed under the terms of the Creative Commons Attribution License (CC BY). The use, distribution or reproduction in other forums is permitted, provided the original author(s) and the copyright owner(s) are credited and that the original publication in this journal is cited, in accordance with accepted academic practice. No use, distribution or reproduction is permitted which does not comply with these terms.
*Correspondence: Zhigang Yan, bHpnNzg5OEAxNjMuY29t; Chaohe Wei, OTA3NjgzMTExQHFxLmNvbQ==
†These authors have contributed equally to this work
Disclaimer: All claims expressed in this article are solely those of the authors and do not necessarily represent those of their affiliated organizations, or those of the publisher, the editors and the reviewers. Any product that may be evaluated in this article or claim that may be made by its manufacturer is not guaranteed or endorsed by the publisher.
Research integrity at Frontiers
Learn more about the work of our research integrity team to safeguard the quality of each article we publish.