- 1Aquaphotomics Research Department, Graduate School of Agricultural Science, Kobe University, Kobe, Japan
- 2Nichiei Intec Co., Ltd., Tokyo, Japan
The objective of this study was to use aquaphotomics and near-infrared (NIR) spectroscopy to follow the changes in strawberries during cold storage in the refrigerator with an electric field generator (supercooling fridge, SCF) and without it (control fridge, CF). The NIR spectra of strawberries stored in these refrigerators were collected over the course of 15 days using a portable mini spectrometer and their weight was measured daily. The spectral data in the region of the first overtone of water (1,300–1,600 nm) were analyzed using aquaphotomics multivariate analysis. The results showed a decrease in weight loss of strawberries, but the loss of weight was significantly lower in SCF, compared to the CF. The reduction of weight loss due to exposure to an electric field was comparable to the use of coatings. The aquaphotomics analysis showed that the NIR spectra adequately captured changes in the fruit over the storage period, and that it is possible to predict how long the fruit spent in storage, regardless of the storage type. During aquaphotomics analysis, 19 water absorbance bands were found to be consistently repeating and to have importance for the description of changes in strawberries during cold storage. These bands defined the water spectral pattern (WASP), multidimensional biomarker that was used for the description of the state and dynamics of water in strawberries during time spent in storage. Comparison of WASPs of strawberries in CF and SCF showed that exposure to an electric field leads to a delay in ripening by around 3 days. This was evidenced by the increased amount of structural, strongly bound water and vapor-like trapped water in the strawberries stored in SCF. This particular state of water in strawberries stored in SCF was related to the hardening of the strawberry skin and prevention of moisture loss, in agreement with the results of significantly decreased weight loss.
Introduction
Strawberry is a well-known fruit consumed in both fresh and processed forms, not only because of its unique taste and fragrance but also its beneficial health properties. Strawberries contain numerous nutrients, vitamins, and minerals and plenty of compounds with biological effects, such as anthocyanins, flavonoids, and phenolic acids, which are shown to have anti-oxidative, anti-inflammatory, antihypertensive, anticancer, and anti-neurodegenerative properties (1–3).
Strawberry is a non-climacteric fruit, one of the most perishable ones, with a short post-harvest life, prone to rapid spoilage, mechanical injury, softening, and infections by several pathogens (2, 4), which necessitates special care during transport and storage to maintain a stable supply throughout the year. To slow down the metabolism and reduce deterioration, immediately after harvesting and prior to transport or storage, strawberries are cooled to 0°C (5, 6), followed by continuous storage at low-temperatures (0–4°C) (7) to control fruit respiration and extend the shelf-life which is usually around 5 days (8). In addition to the prevalent techniques of controlling decay, which are based on rapid cooling after harvest and storage at low temperature, other techniques are investigated to maintain quality, such as heat treatment, smart packaging materials, edible coatings, modified storage atmosphere, irradiation, and others (5, 8–19). All the physical and chemical preservation techniques are aimed to control the available oxygen and moisture transfer, thereby decreasing the respiration and transpiration trend and reducing weight loss, shrinkage, and microbial activity (13, 20, 21).
Packaging and coating benefits are, however, dependent on consumers’ behavior, and several studies showed that a large percentage of consumers (at least 50%, up to 89% in some cases) remove the coating or open the package, not recognizing that it has a function of preserving the food quality (22–24). In addition, as several studies showed, most of the current preservation strategies are money and time-consuming, while fruit still experiences changes in color or flavor (14, 25–27). The application of electromagnetic fields in cooling systems is one of the novel strategies explored with the aim to develop better storage conditions for agricultural products. Research studies have shown that electromagnetic treatment helps the biological systems activate their defensive reactions, leading to an increase in the ability to repair the physical damages and benefit preservation (28–32).
Most fruits, including strawberries, have high water content per weight. In strawberries, about 90% of their weight is water. Water is a strong absorber of light - it absorbs over the entire electromagnetic spectrum, but in the near-infrared (NIR) spectral region 700–2,500 nm, the absorbance of water, especially compared to infrared light, is much smaller, making spectroscopic characterization possible for samples with high water content (33). Near-infrared (NIR) spectroscopy (NIRS) is a widely used non-destructive method that allows the acquisition of data without damaging the samples.
The primary features of the NIR spectrum of water, or any sample with high water content, are two prominent bands, called the first overtone of water and combination band, with several smaller bands due to higher overtones and combinations (34). Owing to the employment of many chemometrics and data analysis techniques, it is now well-recognized that these broad features are composed of many specific water absorbance bands that can be assigned to specific water molecular species (conformations). This new lens to the bio-system investigation was first recognized by a young scientific discipline, aquaphotomics, established by Prof. Dr. Roumiana Tsenkova in 2005 (35). The NIR aquaphotomics takes advantage of non-destructive NIRS measurements and ground-breaking knowledge of water–light interaction for the development of the non-destructive, integrative analysis of intact biological systems (34). This concept opens a novel, dynamic, non-invasive way of biosystems monitoring.
This research aimed to utilize aquaphotomic NIRS for monitoring during cold storage and better understanding of the mechanisms for maintaining freshness, choosing the strawberry fruit as a fruit-model system. The combination of NIRS and aquaphotomics has been already used to monitor aqueous and biological systems non-invasively for various purposes, such as evaluation of fresh and processed fruits and vegetables quality (36–42), milk quality (43, 44), viral infections in plants, bacterial cultures (45, 46) and fermentation (47–49), and physiological monitoring and diagnostics in higher organisms (50–55). The second aim of the investigation was to compare the effects of different cooling technologies on the preservation of strawberries. The applications of aquaphotomics in the monitoring during storage and estimation of freshness and shelf-life have only recently been explored (41, 56). This study employed two different cooling technologies. The first cooling system was based on conventional refrigeration technology, while the second one, employed exactly the same system but with the addition of an electric field generation device. The effects of different cooling systems were explored using non-destructive measurements by near-infrared spectroscopy (NIRS) and evaluated using an innovative aquaphotomics approach, which provides information about the molecular structure of water in the strawberries and connects it with their system functionality. The aquaphotomics analysis was focused on the spectra of strawberries collected in the NIR range 1,300–1,600 nm, the first overtone of water symmetric and asymmetric stretching vibrations. To this date, this range of spectra is considered the best for the understanding of the water molecular structure in various bio-aqueous systems in relation to their observed functionality, which is well-documented in many literature sources (35, 57–60).
To the authors’ knowledge, only one research study used a similar approach to monitor changes during the storage of fresh produce (41), but this study went even further with the added novelty of exploring the differences in stored fruit depending on the employed cooling technology. While there are scientific studies concerned with the exploration of how novel cooling technologies affect food during storage or extend shelf life, the studies which explain the underlying mechanisms behind the shelf-life extension are still scarce. The application of aquaphotomics and in-depth investigation of water molecular structure in fruit during storage and how it changes with the time spent in storage, described in minute details, as this work will show, is a pioneering one and might set the direction of future research in this area. Interpretation of the found differences within the framework of known functionality of different water molecular species could serve as a basis for improved refrigeration, maintaining freshness, and prolonged shelf life. This information will provide novel possibilities for the development of non-destructive food monitoring technologies and a better understanding of postharvest food preservation mechanisms.
Materials and methods
Samples and storage conditions
Strawberries (Fragaria L.) of the ‘New Harumi’ cultivar were purchased in the local supermarket in Hyogo prefecture in Japan. After the transport to laboratory, the fruits were screened for uniformity, defects, and damage, and without washing or any other treatment, randomly distributed on the paper plates, eight strawberries per plate. Each individual fruit was assigned with the number label on the paper to provide identification of each biological replicate. The samples were then stored in different cooling system environments, 24 strawberries (three plates) per each storage condition – in the commercially available refrigerator (Model no. YRC-080RM2, Nichiei Intec Co., Ltd, referred to as a “Control Fridge: CF”), and also commercially available refrigerator, which employs electric field (Super Cooling Plant, Nichiei Intec Co., Ltd., referred to as a “Super Cooling Fridge: SCF” (61). The sinusoidal waveform in the frequency range of 48–62 Hz is used to form an electric field; the field is static with a strength of 3,000 V.
The nominal working temperature of both refrigerators was set to the same value of T = 0°C with a relative humidity of 90%. The samples were stored without any cover, packaging, or coating, in order to examine only the effects of different cooling systems, simulating the typical scenario of how the majority of consumers would behave.
Weight loss monitoring
The weight of each strawberry sample was measured immediately before the spectral acquisition for each fruit each day during the period of monitoring. Weight loss rate was calculated from the weight difference compared to the first measurement, when the storage period started, for each individual strawberry, according to the following equation:
Where Wf represents the weight of a particular sample before storage (fresh) and Ws represents the weight of the sample on the respective day of storage.
To explore the dynamic changes in weight loss, the daily weight loss was also calculated. Daily weight loss represents the weight difference between a certain day of storage compared to the weight measured on the previous day, which is plotted as a function of time and shows the dynamic weight change in the strawberries.
Near infrared spectral acquisition
Spectral acquisition was performed using a portable, hand-held MicroNIR instrument (Viavi Solutions, Santa Rosa, CA, USA) in the spectral range of 908–1,670 nm, with approximately 7 nm resolution step.
The spectra were collected from 2 positions, front and back (Figure 1), per sample, with three consecutive measurements, for 14 days of the storage duration. The measurements were performed at 3.00 PM every day. The spectra collected on the first day of experiment, prior to putting the strawberries in the cold storage, are labeled as “day 0;” therefore, these are the spectra of fresh strawberries. The spectra collected afterward are labeled according to the number of days spent in cold storage, such as “day 1” and “day 2” finishing with “day 14”. In total, 2,160 spectra (15 days × 24 strawberries × 2 positions × 3 consecutive measurements) were collected from each storage condition group.
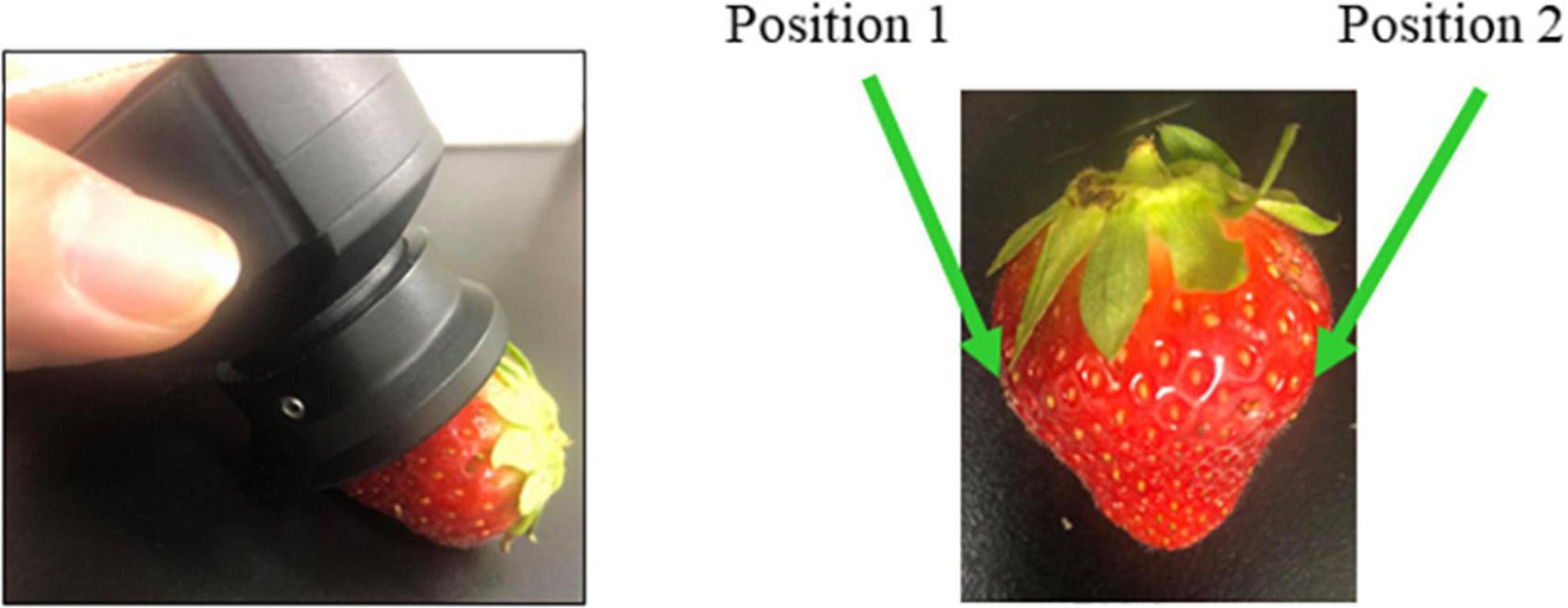
Figure 1. Acquisition of strawberry spectra using hand-held MicroNIR spectrometer was performed on two measurement positions.
Data analysis
Statistical analysis of weight loss
A paired samples t-test assuming equal variance at 95% confidence level was performed to confirm the significant difference in the %weight loss rate and daily weight loss between the two different cooling storage systems during the first 7 days of the storage. The statistical analysis was performed using Microsoft Excel (Microsoft, Redmond, WA, USA).
Multivariate analysis
Multivariate analysis of the water spectra was performed using Pirouette software v4.5 (Infometrix Inc. USA). Standard normal variate (SNV) transformation (62) was applied as a pre-processing treatment to eliminate the baseline differences among the spectra before calculating the difference spectra. In all other instances, the analysis was performed using raw spectra to introduce all available information to the model.
The difference spectra were calculated by subtracting the averaged spectrum of SNV-transformed spectra acquired on the first day of the storage [Sday (1)], from each of the averaged SNV-transformed spectra on subsequent days [Sday(n)] to see the spectral changes during the storage, as given by the following equation:
Soft independent modeling of class analogies (SIMCA) analysis, a supervised classification method (63), was performed with the objective of discrimination between different days of storage [class variable – duration of storage (days)]. This algorithm employs principal components analysis of spectra for the construction of mathematical models for each sample group (class: storage day) with confidence limits. Interclass distances are calculated using between-class residuals, and variable importance is determined by comparing the average residual variance of each class to all classes and the residual variance of all classes to themselves. Variable importance, known as discriminating power, was used to find variables (wavelengths) with the highest contribution to discrimination between classes. The analysis was performed using mean-centered spectra, and the SIMCA models were developed separately for each cooling system.
Partial least squares regression (PLSR) analysis (64) was performed to explore spectral changes as the function of the storage duration (number of storage days). The models were developed separately for each different cooling system, and the water spectral data were mean-centered prior to regression modeling using the number of days of storage as a dependent variable. The analysis was applied twice to investigate changes during 4 and during 14 days of storage, according to the changing trends shown in the difference spectra. The validation was performed using stepwise exclusion of six spectra in each iteration. The accuracy of the PLSR models was evaluated by determining the coefficient of determination of calibration (Rc2), standard error of calibration (SEC), coefficient of determination of cross validation (Rcv2), and standard error of cross-validation (SECV). The optimum number of latent variables (LV) was determined based on the lowest SEC and lowest SECV, for calibration and cross-validation, respectively. The maximum number of LVs (factors) included in all models was limited to 15 in order to include as much as possible information but based on visual inspection of regression vectors for any appearance of “jaggedness” to prevent overfitting (65).
Aquagrams
Aquagrams (66, 67) were used to visualize the water spectral pattern in the strawberry flesh and how it changes along with time and depending whether the strawberries were stored in controlled or supercooling fridge. The representative water absorbance bands that were used as radial axes on aquagram, were selected from all the analysis results, following the procedure described elsewhere (67, 68) to ensure their importance and consistency. There are several ways to create aquagrams, depending on the purpose of the investigation (67). In this study, the aquagrams were calculated according to the procedure for calculating classical aquagram (67). In brief, the SNV – transformed and normalized absorbance values were averaged for each day, for SCF and CF conditions separately, and the values calculated for the first day of storage were subtracted. This calculated difference in absorbance was plotted at the found representative water absorbance bands to illustrate the changes in strawberries each day and depending on the storage conditions. The aquagram display was created in two different ways, first to allow the comparison of the storage conditions in general, and second, to provide better insight into the differences between the strawberries stored in different conditions on day-to-day basis.
Results and discussion
Weight loss of the strawberries during storage
Although the values were slightly different between individual strawberries, due to the natural variety of the biological replicates, the weight of the strawberries during storage, on average, linearly decreased with the progress of storage time irrespective of the applied cooling system. The weight was monitored for 14 days; however, here, only the results of weight change after 7 days will be presented, to allow comparison with most of the available literature sources.
There was a significant difference in the weight loss rate of strawberries stored in the control CF (mean M = 7.3%, standard deviation SD = 0.009737) and supercooling fridge SCF (mean M = 5.9%, standard deviation SD = 0.009964) [t(df) = 3.3342, p = 0.000849; Figure 2A] after 7 days of storage. The weight loss during this period was shown to be fluctuating on a day-by-day basis (Figure 2B) but mainly followed the linear trend as the function of storage days, in agreement with many previous studies (5, 10, 69). Despite the similar pattern in weight loss from day to day, the weight loss of strawberries in SCF was each day smaller in comparison with CF.
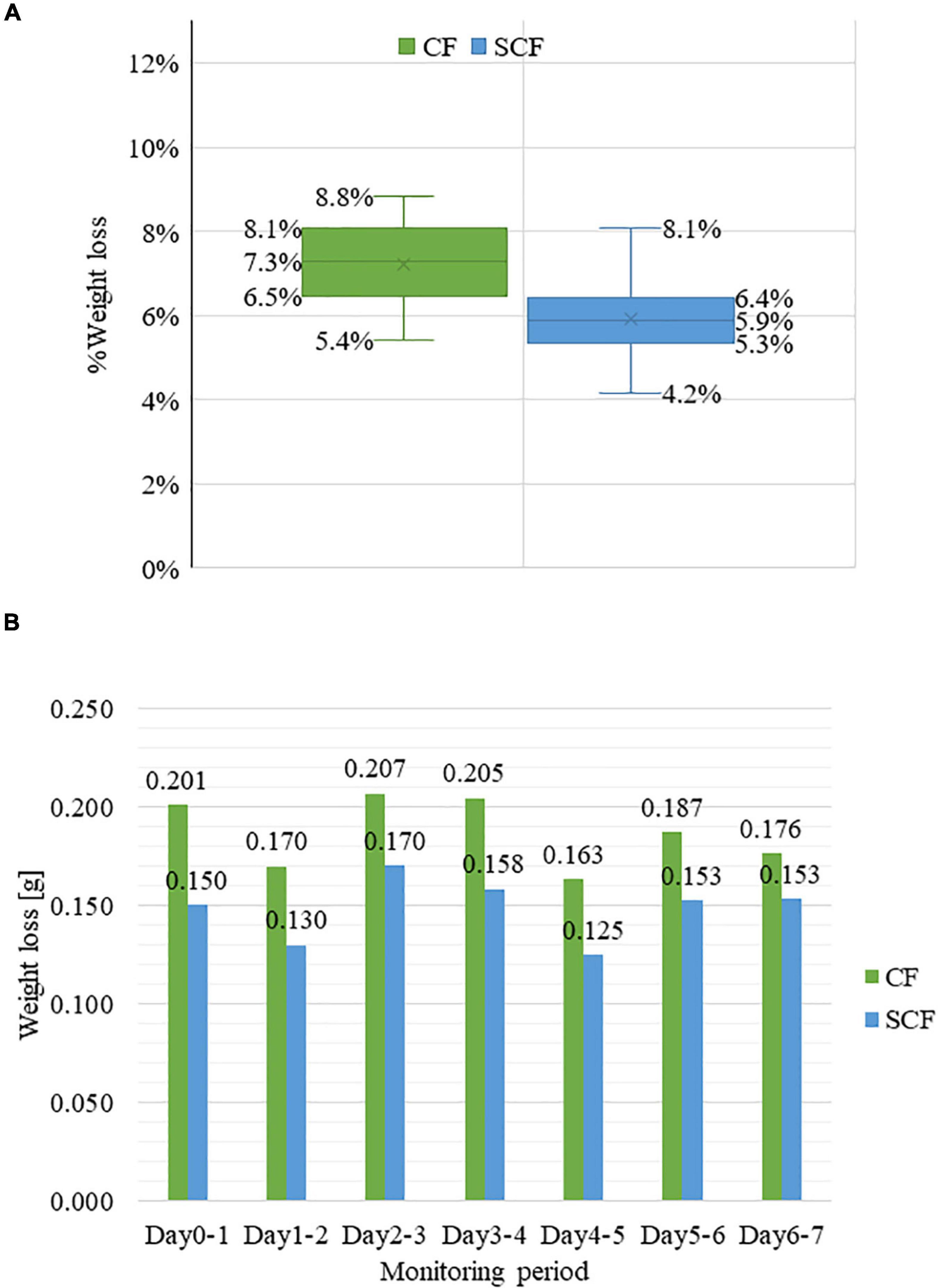
Figure 2. Weight loss during the storage. (A) % weight loss of strawberry samples in control fridge (CF) and supercooling fridge (SCF) after 7 days of storage (B) the daily weight change of the strawberry samples in CF and SCF conditions within 7 days.
The loss of weight in strawberries is associated with the respiration rate and evaporation of water through the fruit surface; the rapid loss of water is the major factor associated with the perishability of fruits, leading to shrinkage and deterioration (70–72). The observed conservation of weight in strawberries in SCF indicates that the exposure to the electric field might be delaying water loss, probably through prevention of the water evaporation. The weight loss of around 6% after 7 days in cold storage is comparable to the influence of coatings which form semi-permeable layer on fruit surface and act as protective barrier to reduce transpiration (7, 73, 74). For example, using chitosan coatings with different molecular weight was reported to limit the weight loss in strawberries to 5.28–5.66% after 14 days of storage at 4°C with the relative humidity of 85% (69). Gelatin coating resulted in 5.26% weight loss after 12 days of storage at 4°C and the relative humidity of 95% (10).
Super cooling fridge showed more potential to limit the water loss in the strawberry samples with a significant difference. Since water loss in strawberry is actually related to respiration and transpiration, this finding suggests that that presence of electric field in SCF influences these major cellular processes (13, 75). Controlling relative humidity in storage in combination with using electric field exposure seems to be promising direction to further limit the water loss which will be investigated in the next phase of this research.
Difference spectra and the changes in the water matrix of fruit flesh during storage
The raw absorbance spectra of strawberries collected over the course of 15 days is presented in Figure 3A for strawberries in CF and Figure 3B for SCF conditions, respectively. Prior to spectral subtraction, the spectra from both groups were corrected for baseline differences using SNV transformation.
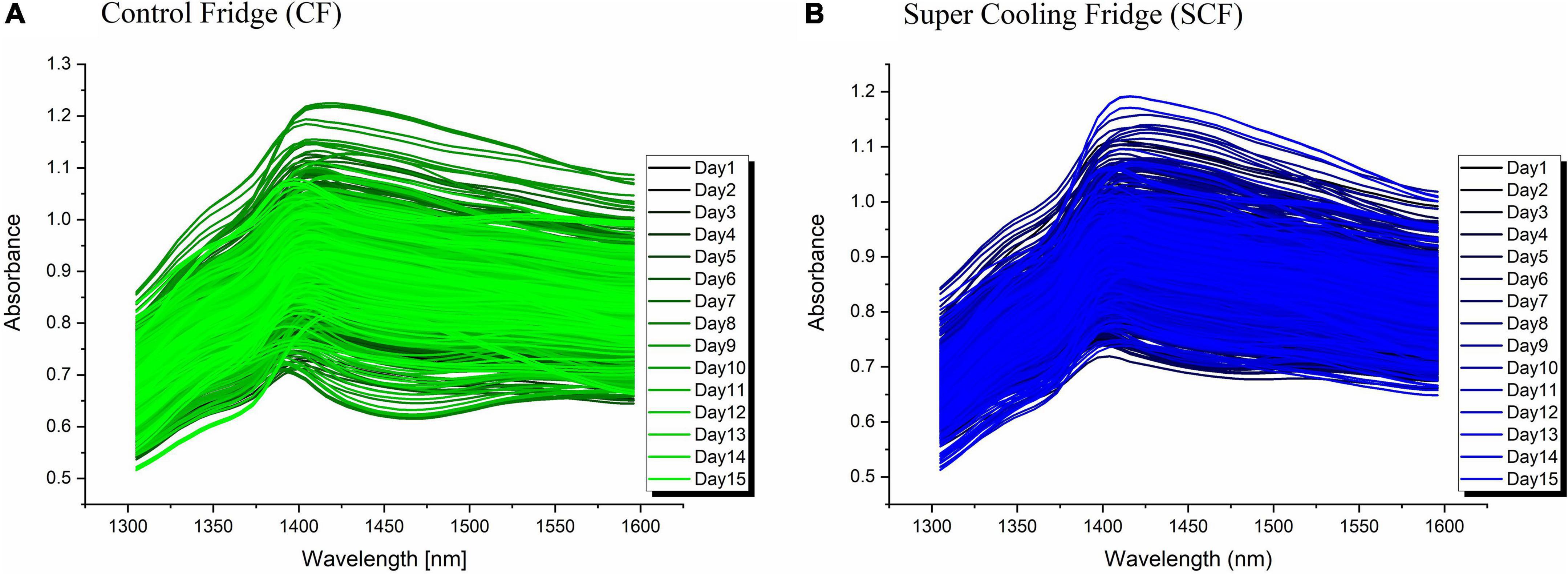
Figure 3. Raw absorbance spectra of strawberries in (A) control fridge (CF); (B) Super Cooling Fridge (SCF).
The difference between spectra measured when the samples were just divided into two different groups and assigned to different storage conditions (day 0), and the spectra measured after the samples spent 1 day of storage (day 1) in CF and SCF, respectively, are shown in Figure 4A. The spectra for each group were averaged, and the difference spectra calculated by subtracting average spectrum at day 0 from average spectrum at day 1. The difference spectra reveal decreased absorbance with the minimum in 1,350–1,450 nm regions after 1 day of cold storage. The entire region corresponds to absorption of weakly hydrogen-bonded water, which encompasses proton hydrates, water hydration shells, and water vapor (56) among other water species, indicating the loss of water from fruit through transpiration, in agreement with what was observed in weight loss measurements.
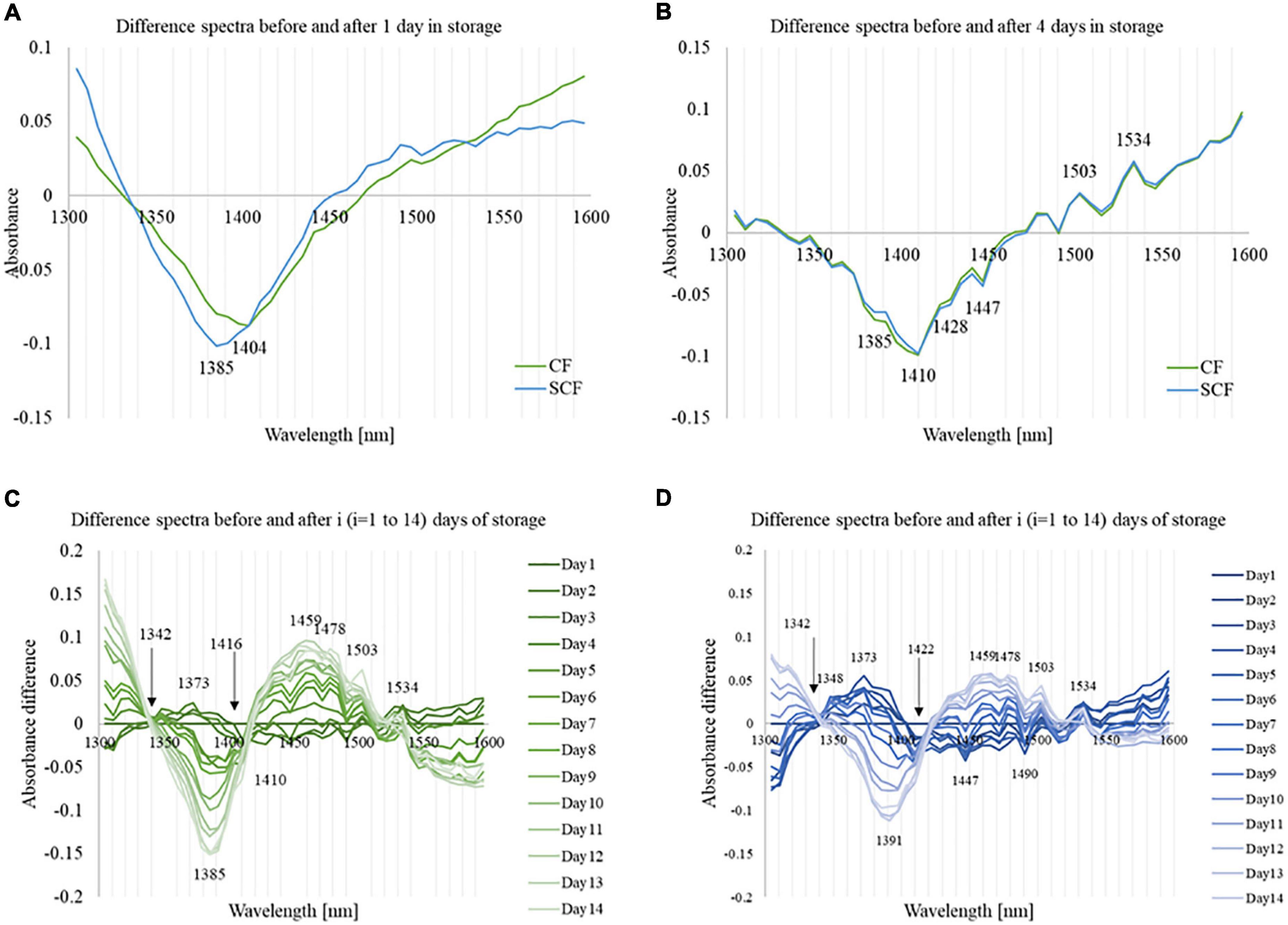
Figure 4. Difference spectra of the strawberry [Day(i) – Day(1)] at different times during storage. (A) The difference spectra from day 0 to day 1 showing the impact of low-temperature storage and different cooling systems. (B) The difference spectra from day 0 to day 4 showing initial changes during storage and differences between the cooling systems. (C) The difference spectra of the strawberry [Day(i) – Day(1)] during the storage in control fridge (CF). (D) The difference spectra of the strawberry [Day(i) – Day(1)] during the storage in supercooling fridge (SCF).
The change for strawberries in SCF showed the minimum absorbance peak located at 1,385 nm (water hydration shell) (66). In comparison, the change in absorbance was less intense for strawberries stored in CF in the region 1,350–1,400 nm, but slightly more in 1,400–1,450 nm, with the minimum located at 1,404 nm (free water) (66). These results revealed different nature of changes in the water molecular structure of strawberry flesh due to the cooling in CF and SCF.
The differences in effects of CF and SCF started to decrease after the strawberries spent 4 days in storage, as can be seen from the difference spectra between the 4th and 1st day of storage of strawberries in the CF and SCF fridge given in Figure 4B. Interestingly, the subtracted spectra in both groups had very similar spectral patterns based mostly on the same water absorbance bands. It is important to note here that the subtracted spectra were not spectra collected prior to cold storage (day 0); therefore, the difference spectra reflect only the changes in strawberries due to the time spent in cold storage.
The value of absorbance in the region 1,350–1,450 nm stayed on the negative side, showing that the continuous loss of weakly hydrogen-bonded water is a common feature for both CF and SCF. The subtle difference between the storage conditions could be seen in the absorbance region around 1,380–1,410 nm, where absorbance bands of water hydration shells (1,380–1,388 nm), trapped water (1,396–1,403 nm) and free water molecules (1,404–1,418 nm) are located (66, 76). The decrease in absorbance of these water species, as well as the weight loss, is more pronounced for strawberries stored in CF.
The daily difference spectra during the storage of strawberries in CF and SCF when compared to day 1 are presented in Figures 4C,D, respectively. The difference spectra were calculated compared to day 1, to investigate how the spectra are change each day during cold storage and if there is a difference between the cooling systems. In the first 3 days, the spectra revealed increasing trend of the absorbance in 1,340–1,400 nm region, with the highest peak at 1,373 nm (proton hydrates, one of the absorbance bands of water vapor) (44, 56, 59). The absorbance in that part reached the maximum value on the 4th day of the storage, and then declined. CF and SCF provided similar results, while the maximum of the absorbance change at 1,373 nm is larger in the SCF group. The increased absorbance in this region suggests increase in the amount of gaseous phase of water within the fruit, which is characterized by highly mobile water species that can be exchanged with the environment. These water species are highly related to the water activity (56), too.
After 5th day, the absorbance in 1,340–1,420 nm of the CF group drop rapidly, while the change in SCF condition occurred gradually. The decreased absorbance at 1,340–1,420 nm had main negative peak located at 1,385 nm for CF, and at 1,391 nm for SCF conditions, respectively, which suggests controlled dehydration for the SCF group. The absorbance in the region 1,434–1,540 nm showed only minor changes until the 4th day of storage, then increased, with the major positive peaks located at 1,459, 1,478, 1,503, and 1,534 nm, and the main negative peaks at 1,447 and 1,490 nm. The absorbance at wavelengths above 1,534 nm was intensively decreased in the case of CF after 5 days of storage, while for SCF, the decrease was very gradual over the storage period and leveled in the last days of monitoring. Taking this into consideration, it seems that beginning from day 5, the changes in spectra of strawberries become very pronounced which is consistent with the reports that the shelf life of strawberries is around 5 days in cold storage (8).
It is interesting to point out that at two locations in the spectra there was a minimum change in absorbance throughout the storage, for both cold storage types (indicated by arrows in Figures 4C,D). The first one is located at around 1,342 nm, while the second one was located at 1,416 nm in the case of the CF, while at 1,422 nm in the case of SCF.
All peaks that were found in the analysis so far are located within the first overtone of water symmetric and asymmetric stretching vibrations, and with the exception of 1,534 nm, all are within the ranges of, already discovered in other systems, Water Matrix Coordinates (WAMACS), absorbance bands of particular water molecular conformations (66). More detailed assignment of these bands and interpretation from the aspect of water molecular structure will be provided later, in the 3.5 Choosing WAMACS and 3.6 “Aquagrams” section.
Soft independent modeling of class analogies analysis of the storage time classification
Soft independent modeling of class analogies analysis was performed with the objective of classifying spectra of strawberries according to the day of spectral acquisition, therefore, developing a model for prediction of how many days the strawberries were stored according to their spectra. The analysis was repeated three times, to explore the possibility of prediction for different lengths of storage time and for exploration of the most influential variables in SIMCA models. These variables, as given by discriminative power vector, can provide insight into the changes in water matrix of the fruit during storage as a function of the storage time and also as a function of different cooling system technology.
For all three SIMCA analyses, performed for the periods of 4, 8, and all 14 days, the results showed good accuracy of classification between the storage days and satisfying interclass distance.
In the case of SIMCA models developed based on the spectra from all 14 days, the classification accuracy was exceptionally high for both CF (97.87% accuracy) and SCF (99.26% accuracy) storage conditions, showing excellent possibilities of using NIR spectra for prediction of the freshness of strawberries, i.e., of predicting how many days the fruit spent in cold storage.
The values of interclass distance between storage days was larger than 1, which can be considered good, according to the reports that less than 0.8 would be a small difference (49). The discriminating powers of SIMCA models provided in Figure 5 allow comparison of influential variables over time (since models were built for 4, 7, and 14 days) and depending on employed cooling technology - CF (Figure 5A) and SCF (Figure 5B), respectively. Discriminating power shows which absorbance bands contributed most to the successful discrimination between storage days.
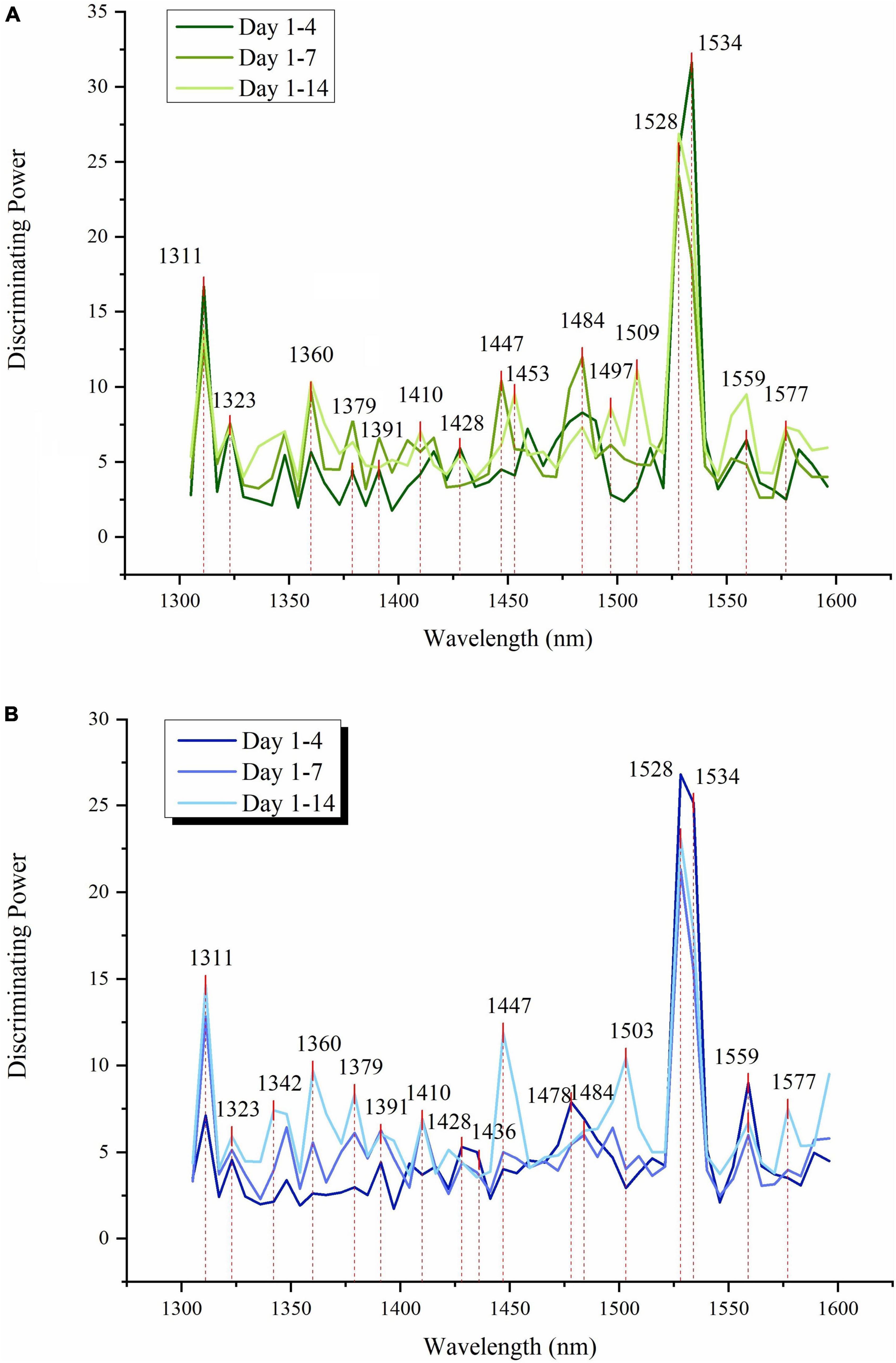
Figure 5. Soft independent modeling of class analogies (SIMCA) discriminating powers of absorbance bands show the importance of individual variables in NIR spectra for discrimination between the storage days of strawberries during 4, 8, and 15 days of storage (A) SIMCA results for CF group (B) SIMCA results for SCF group.
The absorbance band with the highest discriminating power, representing an essential feature for all classification models, was found located at 1,528–1,534 nm for both CF and SCF conditions. In the classification model using 14 days of data, the discriminating powers of SIMCA models for CF and SCF show the common peaks at 1,311 and 1,360 nm, while 1,447 nm was more prominent in the SCF discriminating powers. SIMCA analysis results for different time periods (4, 7, and 14 days) revealed that the absorbance bands at 1,311 nm and 1,528–1,540 nm are always the variables with the highest discriminating power, describing where in spectra are the largest differences with respect to the day of the storage.
The discriminating power at 1,311 nm shows an increase along with time for SCF models but decreases for CF SIMCA models. The band located at 1,447 nm showed higher discriminating power during the first 7 days of storage for strawberries in CF, but it had highest power for 14 days of storage data when it comes to the SCF group. For CF data, the discriminating power of 1,534 nm surpassed 1,528 nm in the model using 4 days of data, but for the longer period, the discriminating power of 1,528 became higher than 1,534 nm, while in SCF 1,528 nm always had higher discriminating power than 1,534 nm regardless of the length of time.
The interesting difference between the discriminating powers between CF and SCF group is the pair of absorbance bands located at 1,360 and 1,379 nm. In the SCF group, peaks showed steady increase in discriminating power, while in the CF group, the band 1,379 nm showed an increase for 7 days, but then it dropped for 14 days model. There is also a difference in the absorbance bands featured as influential in models for CF in SCF in the area 1,484–1,510 nm. In the case of CF group, there are three different peaks located at 1,484, 1,497, and 1,509 nm, but for SCF group, there is just one broad band located at 1,503 nm.
The interesting aspect of this analysis is that despite different storage conditions, the same absorbance bands were shown as the most important for the description of how strawberries change during cold storage, and yet the analysis also captured the specifics of water molecular reorganization in fruit over time, as a function of different cooling technology.
Partial least squares regression analysis of water spectral changes during storage
According to the difference spectra results, which showed different trends of the spectral changes between the first 4 days and later storage period, the PLS regression analysis was first performed using spectral data collected during the first 4 days, and second, using all 14 days spectra [including the spectra collected before placing the strawberries in the storage (day 0)]. The dependent variable in both cases was time, expressed as “days of storage”. The PLSR models were then calculated for CF and SCF data separately, and their performances were compared (Figure 6).
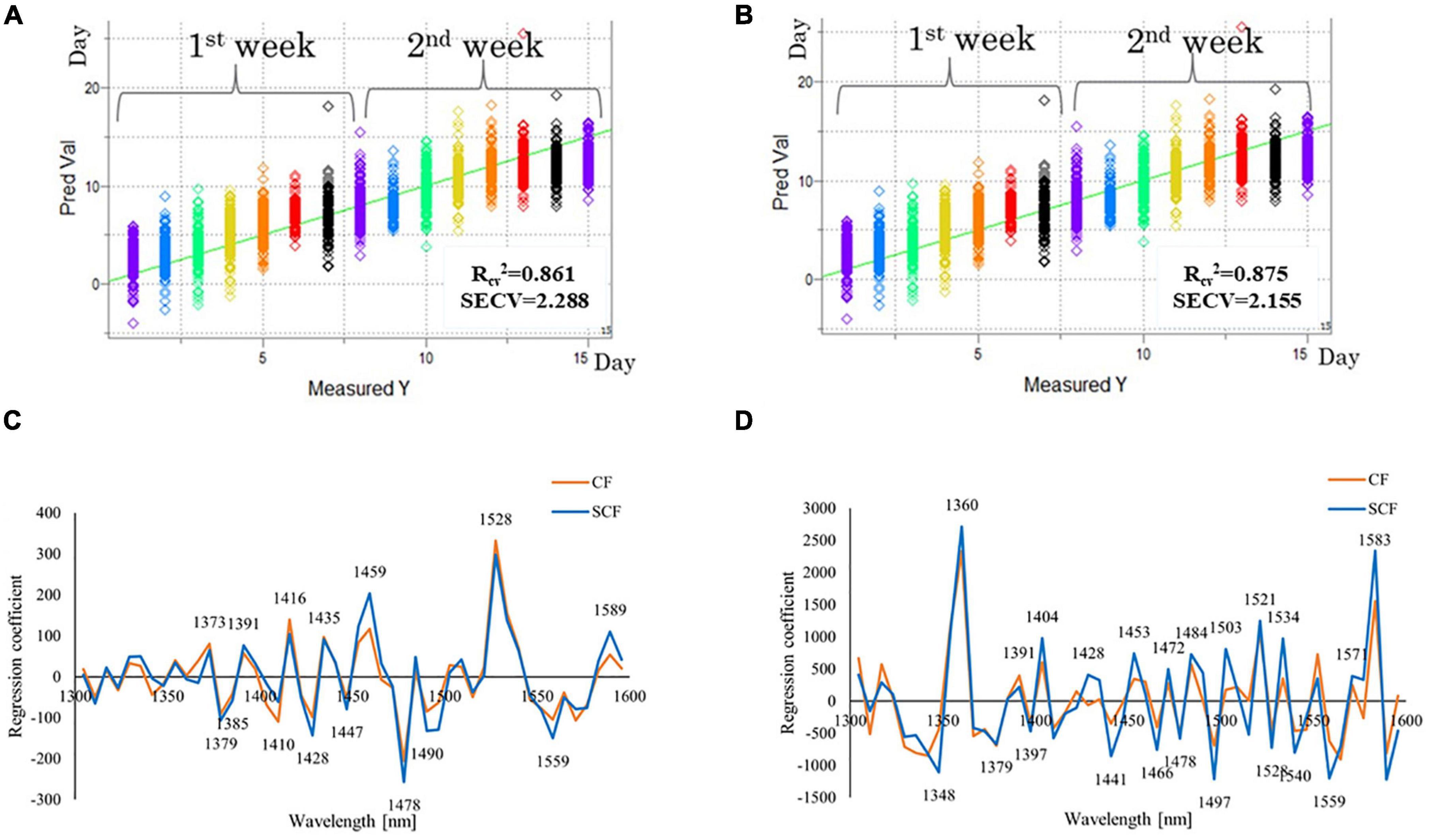
Figure 6. Results of partial least squares (PLS) regression modeling using time as a dependent variable (days of storage). (A) Y-fit plot, showing agreement between actual number of storage days and predicted number of storage days using PLS time regression model for spectra of strawberries in the control fridge (CF) condition (B) Y-fit plot, showing agreement between actual number of storage days and predicted number of storage days using PLS time regression model for spectra of strawberries in the supercooling fridge (SCF) condition (C) regression vectors of PLSR models built using spectral data of strawberry stored in CF and SCF conditions - comparison between regression vectors of models for the first 4 days of storage; (D) regression vectors of PLSR models built using spectral data of strawberry stored in CF and SCF conditions - comparison between regression vectors of models for the 15 days of storage.
The predictions of the days of storage were successful, regardless of storage conditions with slightly less accuracy for the CF (coefficient of determination Rcv2 = 0.861, standard error of cross-validation SECV = 2.288) compared to SCF (coefficient of determination Rcv2 = 0.875, standard error of cross-validation SECV = 2.155 days). The PLSR results showed that the actual day of storage can be predicted with around 2 days error. The Y-fit plots that show agreement between actual and predicted day of storage show a very good linear relationship for both CF (Figure 6A) and SCF (Figure 6B) for all 14 days. The cause of error can be attributed to the differences between the individual samples which can be expected since the models were developed using raw spectral data. The adequate spectral pretreatment aimed at removal of influence of physical differences between the samples (such as surface curvature of the fruit) can probably lead to decreased error.
The results revealed that in the same time span both models for CF and SCF provided similar regression vectors as presented in Figure 6C for 4 days and Figure 6D for 14 days PLSR models. The time regression models for 14 days of storage fit linear pattern, describing the spectral change over the time with very high coefficient of determination (R2 > 0.85; Figures 6A,B). This is the second analysis that confirms the ability of the NIR spectra to capture the information related to the passage of time in cold storage, and the potential for the estimation of freshness of strawberries.
The regression vectors shown in Figure 6C (for 4 days models) and Figure 6D (for 14 days models) can provide information which variables, i.e., absorbance bands were the most informative for modeling of storage time. As can be seen from these figures, in the first 4 days of the storage (Figure 6C), 1,528 nm appeared as a dominant peak with the highest, positive regression coefficient for both CF and SCF groups. In the model of 14 days of data (Figure 6D), 1,360 and 1,583 nm became much more prominent. There is also significant difference in the overall magnitude of regression vectors coefficients for 4 days models and 14 days models, which are almost eight times larger in the second case. There is also a difference between these models when it comes to the sign of regression vector coefficients – the peaks located at 1,373, 1,391–1,398, 1,416, and 1,528 nm were positive at first, during the 4 days period, but then become negative for the longer storage data. In comparison, the regression coefficient of 1,435 nm stayed positive in all sets, but with less weight.
The differences in regression vectors from the aspect of cooling systems are visible for the 4 days models as well as in 14 days models. The differences appear at 1,350–1,373, 1,391–1,400 nm, especially at 1,459, 1,490–1,500, and 1,559–1,583 nm for the 4 days model (Figure 6C). For the 14 days models, the differences in regression vectors appear at 1,330–1,348, 1,391, especially at 1,428 nm which shows almost no correlation with time for the CF model, and 1,441, 1,453, 1,466, 1,484, and 1,497 nm, again, very large difference at 1,503 and 1,550 nm, and finally again large difference at around 1,580 nm, where even the sign of the regression coefficients for CF and SCF is the opposite.
Taken together, this exploratory analysis of influential variables in PLS regression models show that the spectra of strawberries capture events on a molecular level in the fruit as it is stored, which seems to be common irrespective of the storage conditions. However, they also show distinct differences in effects of cooling systems, at specific absorbance bands which were already observed during previous analyses.
Choosing water matrix coordinates for aquagram display of water spectral patterns of strawberries
The analysis of spectral data of stored strawberries, despite differences in storage conditions, showed repeating and consistent absorbance bands at which the absorbance changed as a function of storage time. When all the bands from different analyses were put together (Supplementary Table 1), the importance of certain absorbance bands for description of changes in strawberries during storage, based on the number of their appearances as important variables in the analyses became evident.
Out of 28 absorbance bands found important for the description of changes during cold storage, 24 belong to well-known WAMACs, that is, small wavelength ranges 6–20 nm in length that corresponds to the absorbance of specific water molecular conformations (57, 58, 66) (Table 1). Additionally, four absorbance bands featured prominently in the analysis – 1,503, 1,528, 1,534, and 1,559 nm. These bands are not yet recognized as WAMACs. All four bands are located within the part of the NIR spectra where it is known that strongly hydrogen-bonded water absorbs. These four bands carried much weight in the analysis indicating the importance of their distinction and we tentatively labeled them as new WAMACs Ci, Cj, Ck, and Cl that may be of interest to monitor freshness of the agricultural products in general (Table 1).
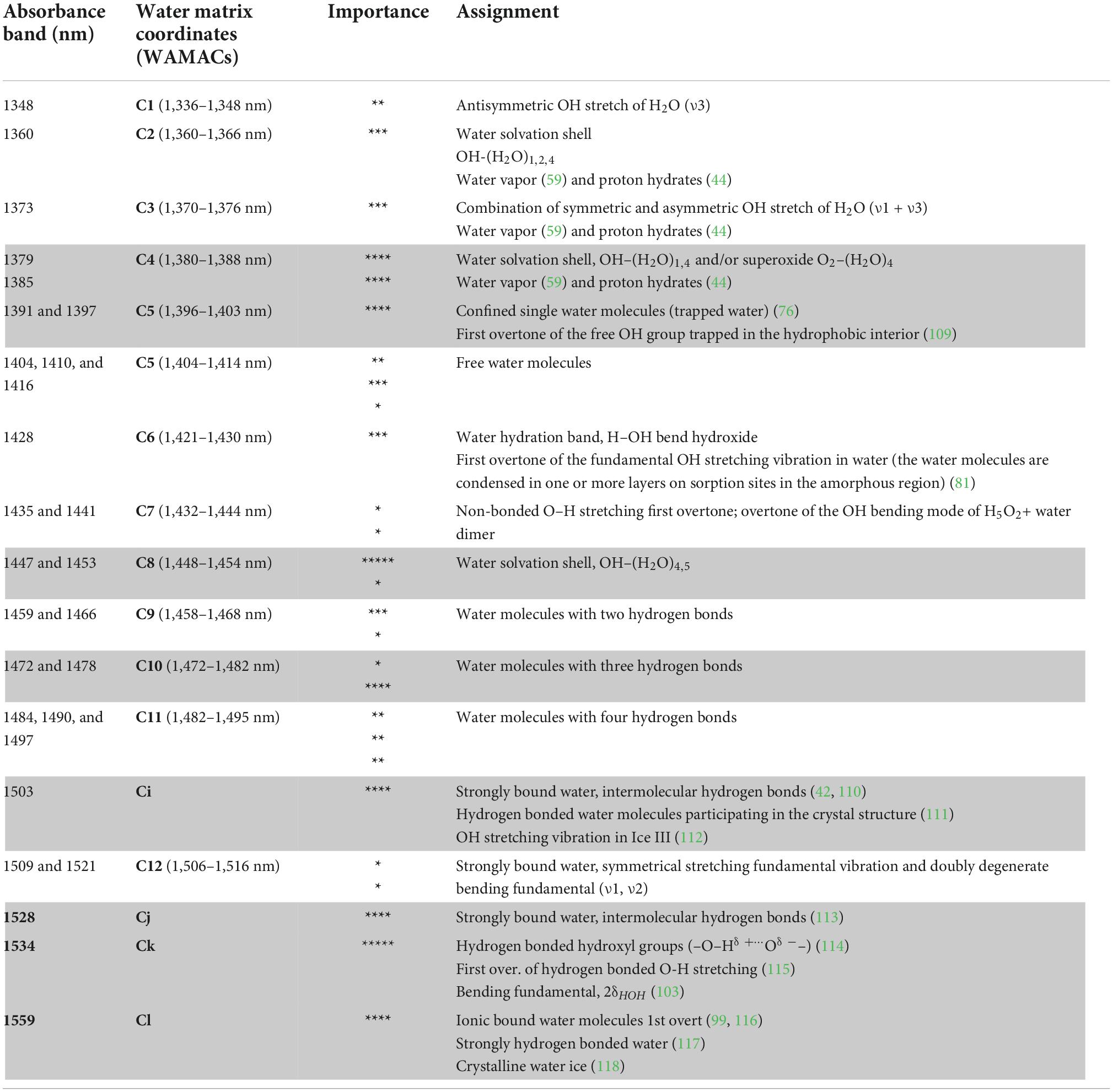
Table 1. Water matrix coordinates (WAMACs) that carry information about the changes in strawberry during cold storage [Number of stars “*” in the column Importance represents the number of occurrences of the absorbance band as an influential variable during the whole analysis, as summarized in detail in Supplementary Table 1. Unless otherwise indicated in the table, the source of all information are references (58, 66)].
Judging by the importance of the WAMACs, it can be observed that C4, C5, C8, and C10 and newly defined Ci, Cj, Ck, and Cl (rows highlighted in gray in Table 1) are not only important for the description of the changes during storage but also reflect the differences in strawberries as a function of different cooling storage treatment.
Together, these WAMACs could be thought of multidimensional biomarker that can be used to describe the process of change in strawberries during storage.
Aquagrams – Water spectral patterns of strawberries during cold storage
Based on the previously found WAMACs, 19 representative water absorbance bands were selected to visualize the collective spectral change during storage of strawberries. These absorbance bands define radial axes on aquagrams on which values of difference in normalized absorbance between the respective day and the first day of storage were plotted, as presented in Figure 7, for cold storage in the control fridge (Figure 7A) and supercooling fridge (Figure 7B). For simplicity and practical reasons, the aquagrams are displayed for the 7 days, because of the usual shelf-life of strawberries of 5 days in cold storage.
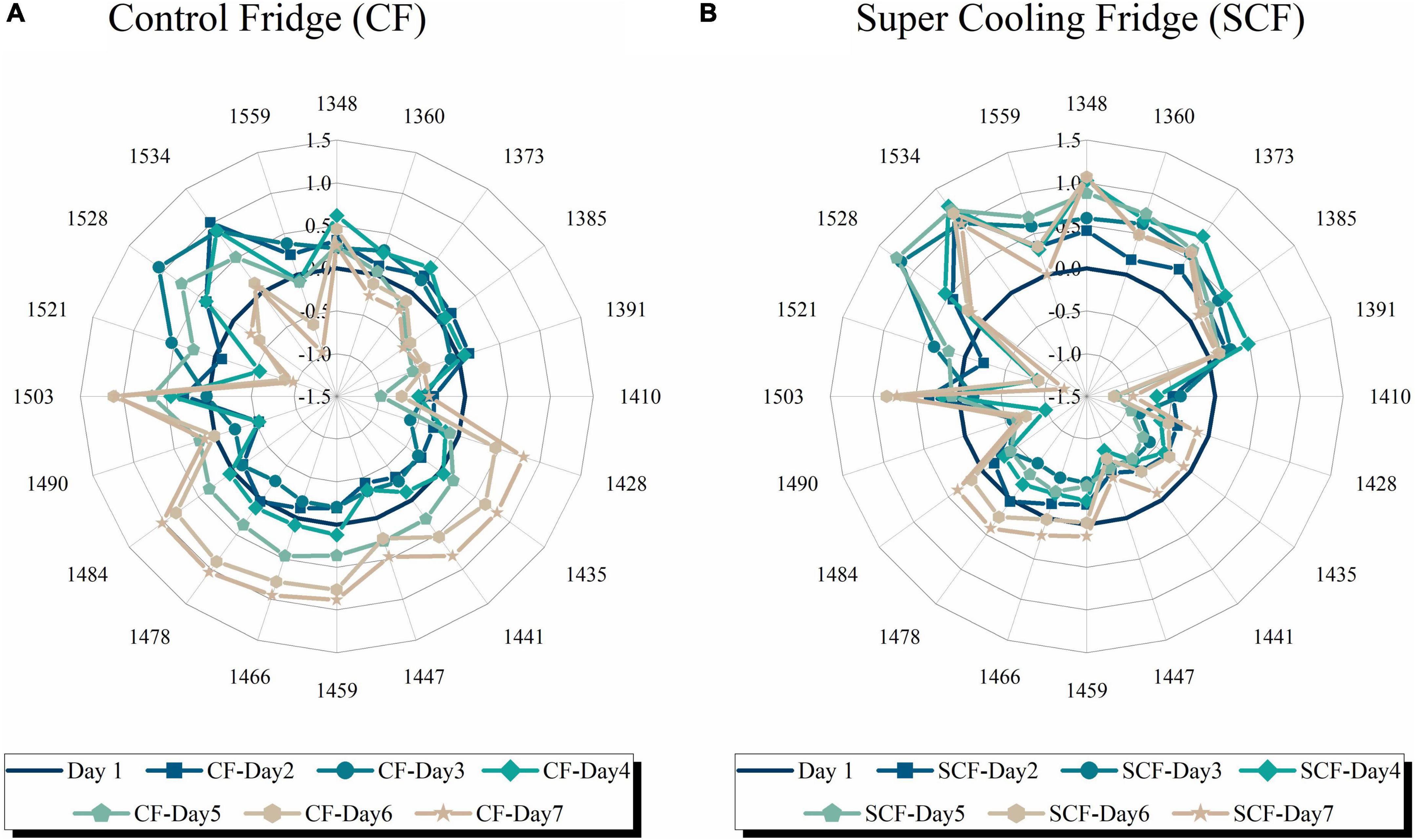
Figure 7. Aquagrams of strawberries showing changes in water molecular matrix of the fruit flesh during 7 days of storage in: (A) Control fridge (CF) and (B) supercooling fridge.
The comparison of how water spectral pattern of strawberries change during cold storage provided stark differences depending on whether the strawberries were kept in control or supercooling fridge. First, in the area of highly active water species, which are vapor-like (57) absorbing in the region 1,348–1,385 nm, it can be observed that when strawberries are in the CF the absorbance decreases with time, while in the SCF the absorbance is increased and stays relatively stable during this 1 week. In the CF, there is particularly decrease at 1,373 and 1,385 nm on days 5–7. All these vapor-like, weakly bonded water species (water molecules in proton and ion hydration shells, water species involved with hydration of soluble charged compounds) are with high energy and very mobile (56). The observed difference suggests increased content of soluble compounds in strawberries stored in SCF, active respiration, and exchange of water in the gaseous phase with the environment and, since the bands 1,360, 1,373, and 1,385 nm are the signature bands of moisture and water activity (56), it also implies the existence of water available to participate in necessary biochemical reactions.
The differences are also present at the bands of free (1,410 nm) and quazi-free water (1,391 nm). For the case of CF, after the initial period of no changes at 1,391 nm, absorbance decreases after fifth day in storage. On the contrary, the absorbance at this band is increased for the case of SCF and declines gradually. This absorbance band is related to trapped water, either in between hydration shells of ions or in the hydrophobic interior, which implies that there is the ongoing production of some compounds in strawberries during this time, which results in their hydration and subsequently confined interior in which single water molecules get confined. This would be in agreement with increased absorbance at hydration shells bands (1,360 and 1,385 nm) signaling the increase in soluble compounds observed previously. Taken together, this implies that upon cold storage in SCF, the strawberries show more signs of active metabolism and production of soluble compounds. It is interesting to point out that the close absorbance band located at 1,398 nm, which is also attributed to trapped water, was found to be highly correlated with vitamin C content and shrinkage of fruit during hot air drying (77).
The absorbance of free water molecules (1,410 nm) is decreased immediately upon storage in both fridges; however, there is difference in the magnitude of this decrease. The decrease is much stronger, very sharp and regular in the case of SCF, while it is not regular and shows fluctuations in CF. This is most probably heavily related to the observed weight loss of strawberries and can be indicated loss of juiciness (56). It was previously observed that decrease in free water molecules is an important defense reaction in response to desiccation stress and that decrease of free water molecules might be the survival strategy in plants in the stress conditions (78). Since a decrease of free molecules is the common reaction in strawberry for both cold storage conditions, this might indeed be the case; however, the gradual and regular decrease in the case of SCF indicates organized control of water loss [also a part of survival strategy as found before (78)].
The largest differences in aquagrams could be observed in the area from 1,428 to 1,490 nm. In the SCF, the absorbance of strawberries seemed to be decreased immediately upon storage and kept that way, and only on days 6 and 7, there is increase at the bands from 1,459 to 1,484 nm. However, such decrease of absorbance was not observed for the CF, and in contrast, the absorbance shows huge increase at all these bands starting from day 4. The band at 1,428 nm, ascribed to hydration water, belongs to the WAMACS C6. In numerous studies, this band was attributed to amorphous regions in cellulose (79–84); however, in the case of strawberry, this water is associated with other polysaccharides. It seems that absorbance increase at this band is associated with drying and increased density (80, 85). There are several research studies that report bands from this particular WAMACS region, as important for the changes during processing and storage. For example, band 1,420 nm was found to be the optimal wavelength for vitamin C content prediction in apple slices during drying (77) and also had high correlation with internal quality of fruit (86, 87). Absorbance at 1,420 nm was in another study reported to be closely related to weight loss during the storage of mushrooms (88), while in another study, it was found that the absorbance at this band particularly increases as a function of storage time [similar to the change of soluble solids content (SSC)] (87). It is worth noting that, in difference spectra, there was actually a minimal change in absorbance at 1,416 nm in the case of CF and 1,422 nm in the case of SCF. While it is not clear what exactly is the difference between the bands 1,416, 1,422, and 1,428 nm, seeing that they are usually attributed to the same water molecular conformation, it is worth noting that this WAMACS region is repeatedly showing the importance of the changes during storage and internal quality of vegetable and fruits. The stark difference in behavior of absorbance at the band 1,428 nm, depending on whether strawberries were stored in CF and SCF seems to further indicate the importance of this band for preservation in cold storage, most probably because it is related to the state of turgor and cytoplasm in the cells, its shrinkage and pulling away from the cell walls. That may explain why the bands from this WAMACS region were so often found related to internal quality, damage, and vitamin C production, seeing that production of vitamin C is the part of the protection against damage. The behavior of absorbance at this band in the case of CF seems to be in agreement with the typical changes during storage; however, the opposite behavior in the case of SCF seems to indicate the presence of electric field slows down this process.
The absorbance bands from 1,435 to 1,490 nm can be related to the water molecular species interacting with biomolecules (1,435 nm), water species participating in hydration (1,459 nm) and water molecules bonding to each other, i.e., creating clusters with 1–4 hydrogen bonds. In the research about the texture of apples, it was found that water spectral pattern characterized by the low absorbance in the region 1,344–1,382 nm and high at 1,410–1,492 nm corresponds to the firm, juicy, and crispy sensory profile (36). The difference in these two particular absorbance regions, in the case of strawberries, might indicate that the presence of electric field actually slows down the process of post-harvest ripening of strawberries in the cold storage.
Next, common for both refrigeration conditions, there is a sharp decrease in absorbance at 1,492 nm, water absorbance band of water molecules with four hydrogen bonds, one of the two bands, the other one being 1,410 nm, free water molecules, related to the influence of temperature (89). For pure water, if the temperature increases the absorbance at the band 1,492 nm will decrease, while at 1,410 nm increase, because the temperature causes breaking of the hydrogen bonds in tetrahedral structure creating more free molecules. It is, therefore, interesting that in strawberry after the fruit was placed in the cold storage there was a decrease in absorbance at this particular band. This might indicate that there is an innate mechanism in the fruit which fights the creation of this type of hydrogen-bonded water. However, it seems that with the progress of time, this mechanism fails in the case of the CF, where increase in absorbance can be observed after day 4, which suggests creation of seeds of ice crystals that may become the source of damage. From this aspect, the presence of electric field in the SCF seems to act protectively.
The behavior of absorbance at band 1,503 nm is common for both storage conditions; there is a sharp, strong increase in absorbance immediately upon the storage. There are many reports about this particular band in characterization of minerals and attributed to vibrational mode of hydrous defects in the crystal structure (90–96). In analogy to these reports, the absorbance at this band can be considered as indicator of shallow, superficial, surface damage, and small openings in the structure of materials that enhance exposure to the environment. If this is correct interpretation that would mean some surface damage on the strawberry skin appeared in both storage conditions, leaving the fruit vulnerable. The region beyond 1,503 nm, encompassing bands from 1,521 to 1,559 nm includes absorbance bands of water species that are tightly bound to the structural elements of the fruit tissue, including crystalline and polymer-bound water (97–100) and represent the water that cannot be lost to processes such as drying or squeezing (101); this water is lost only if the structural elements associated with it are lost. The difference between the water species absorbing at particular bands is in the level of complexity of the structure they are engaged in, and in the strength of the bonds, which is increased with the increase in wavelength. Having this in mind, it seems that in both cold storage conditions, the strawberries suffered some level of tissue damage, seeing a sharp decrease at 1,521 and 1,559 nm bands. The band at 1,534 nm was often attributed to strongly bound water (102, 103) related to water–polymers interaction [such as cellulose (104)] that appears during initial phases of drying (105), while the band at 1,559 nm is typically assigned to bond vibration of O–H stretch in crystalline cellulose (104, 106). Here, it is important to note that while other reports are attributing these bands to cellulose, they are in fact water absorbance bands, and in the case of strawberries, this strongly bound water is bound to numerous polysaccharides, typical building block of cell walls. With the progress of time in storage, the decrease in absorbance can be observed at all those bands in the case of CF, but not in the case of SCF.
The second most striking difference between the spectral patterns of strawberries in CF and SCF seems to be exactly the decrease in absorbance at the bands of strongly bound water. This seems to be connected with the increase in absorbance of the so-called bulk water, all those small clusters of hydrogen-bonded water species observed earlier in the region 1,435–1,484 nm, which happens in the case of the CF. While the strongly bound water, as the term itself clearly indicates, is strongly bound to structures in the fruit, the bulk water is water not associated to fruit, it is the “water hydrating other water molecules”. The decrease in strongly bound water can be connected to softening of the fruit during ripening and increased bulk water with swelling and expansion of vacuolar volume (107). Since these changes are not present in the SCF, it suggests that ripening of strawberries is delayed by the presence of electric field.
To examine better this possibility and see better the transformation of water spectral pattern over time, a series of aquagrams was created showing comparison of water spectral patterns of strawberries in CF and SCF, 1 day at a time (Figures 8A–F). From this series of plots, the first interesting observation is that the patterns after 1 day of storage (Figure 8A) are nearly identical, testifying to the equality of the samples at the beginning of the experimental period, while the last plot (Figure 8F) shows remarkably well how different storage conditions led to the completely different water spectral patterns, i.e., how spectral pattern as a multidimensional marker accurately depicted the difference in the final state of the strawberries caused by different storage conditions. The first changes can be observed on day 3 of storage (Figure 8B) where higher absorbance can be observed at strongly bound water bands (1,528–1,559 nm) and at vapor-like and trapped water (1,348–1,391 nm) in the case of strawberries in SCF. This increase is continued over subsequent days (Figures 8C–F), while the spectral pattern of strawberries in CF is primarily characterized by decrease of absorbance at strongly bound water bands, and large increase in absorbance of bulk water. But the patterns become more and more similar, for example, the pattern of strawberries in SCF fridge on day 7 (Figure 8F) looks very much like the pattern of strawberries in CF fridge on day 4 (Figure 8C). This strongly suggests the fruit is going through the similar process, but with some time delay in the case of SCF fridge. To test this assumption, one more aquagram is created (Figure 9), where the water spectral patterns of strawberries on days 6 and 7 in SCF are compared to the spectral pattern of strawberries in CF on day 4. The spectral patterns show strikingly similar shape, indicating that the fruit indeed goes through a similar process, which is in SCF delayed for around 3 days. The possible mechanism of how this happens might be explained by the influence of electric field on the surface hardening of the strawberries, which limit the water diffusion and thus evaporation, as research findings reported to be the case in electric field treated apples post-harvest (108). This would be very consistent with the observed increased absorbance of strongly bound water and conserved vapor-like water within strawberries in SCF.
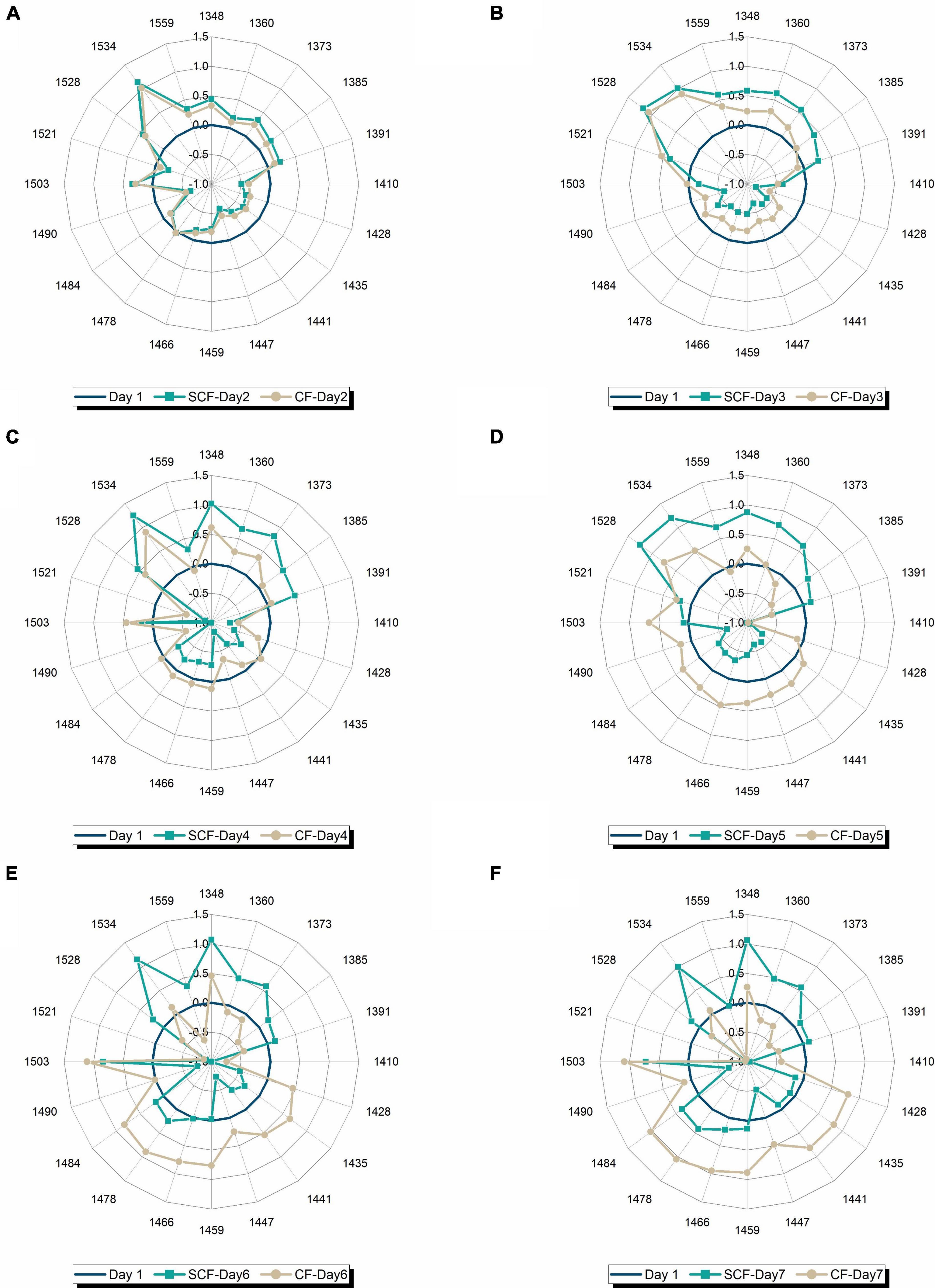
Figure 8. Comparison of water spectral patterns of strawberries stored in control (CF) and supercooling fridge (SCF) on day-to-day basis: (A) After 1 day of storage, (B) after 2 days of storage, (C) after 3 days of storage, (D) after 4 days of storage, (E) after 5 days of storage, and (F) after 1 week of cold storage.
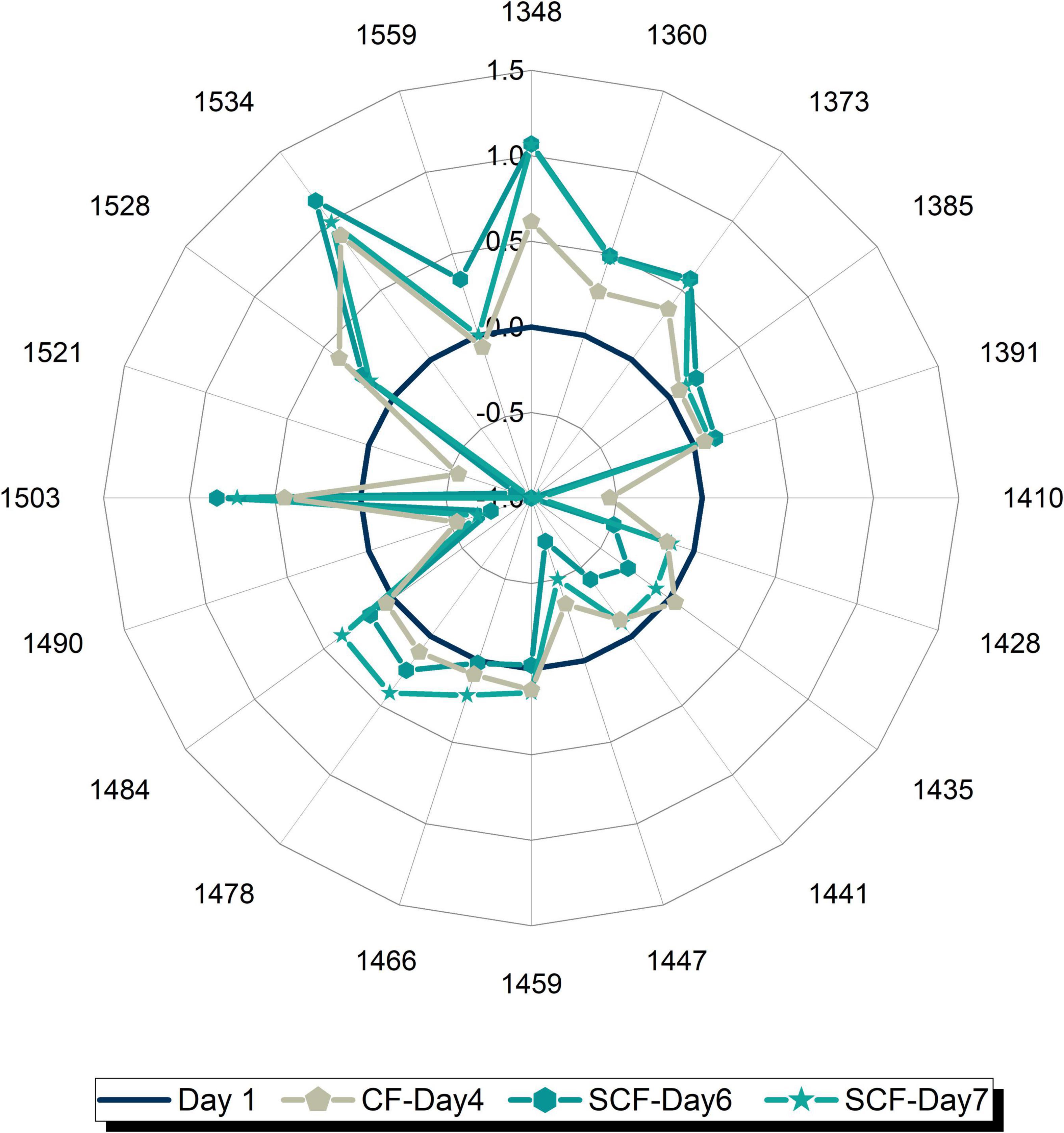
Figure 9. Comparison of water spectral patterns of strawberries in the control fridge (CF) on day 4 and strawberries in supercooling fridge (SCF) on days 6 and 7 shows that the presence of electric field delays the ripening in cold storage.
The important finding in this study also is that the water spectral pattern as a multidimensional biomarker captures perfectly the process of ripening and shows that it can be described only by using water absorbance bands. The consistently found importance of these particular bands was confirmed through several analyses and they can be considered as WAMACS or water matrix coordinates that define the space of variables describing the changes of postharvest ripening that can probably be used for other biological systems as well. Numerous research works have already found these bands related to certain functionalities in other bio-aqueous systems, and this information is summarized in Table 2.
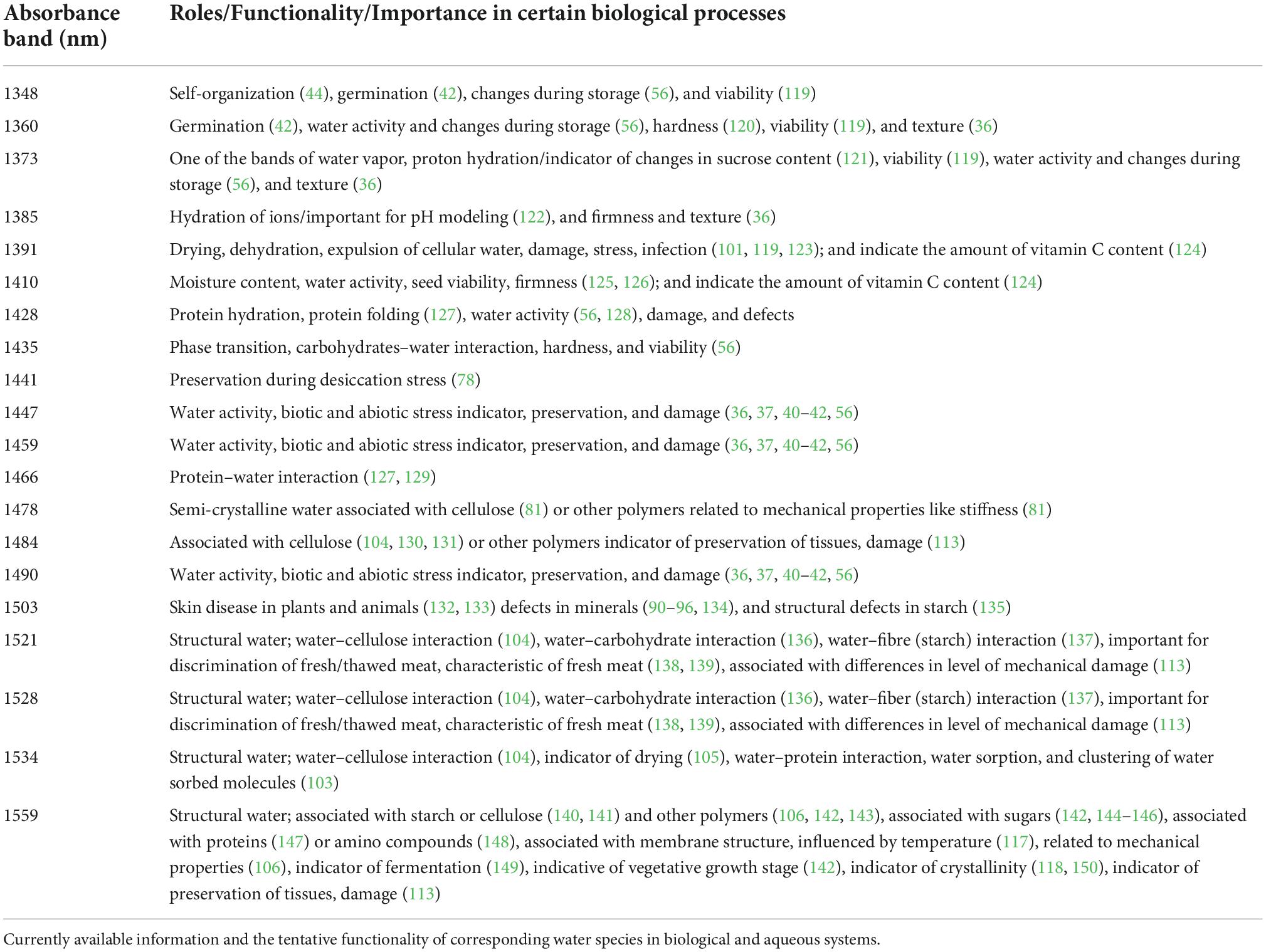
Table 2. Water matrix coordinates (WAMACS) that can be used to describe the changes during post-harvest ripening of strawberries during cold storage.
Conclusion
This study aimed at investigating the spectral changes during the storage of strawberry in order to confirm the potential of aquaphotomics NIRS for monitoring during cold storage. Further, the same technology was applied to assess the influence of the storage environments with a particular objective to find and explain the differences in applied cooling systems [without (CF) and with the electric field exposure (SCF)] on the stored fruit. The water matrix transformation during the post-harvest degradation of strawberry as well as the influence of electric field could be observed using the NIR spectra of strawberries in the 1,300–1,600 nm region.
Over the course of several analyses, the same water absorbance bands were found to be important for the description of the changes in fruit during storage. The main outcomes of the analyses were that it was possible to detect spectral changes over storage and predict the time spent in storage. The consistently repeating water absorbance bands were used to define water spectral patterns as biomarkers of the state of the strawberries in cold storage and allowed comparison of strawberries stored in different conditions. This comparison showed that the presence of electric field in supercooling fridge slows down the ripening processing, delaying it for about 3 days. The possible mechanism behind can be explained by the influence of electric field on skin hardening, which then acts as a natural coating and slows down evaporation. This agreed with the lower water loss percentage recorded for strawberries in the supercooling fridge.
Using this technique of spectral monitoring, the ripening process can be observed in a non-destructive way. This provides the potential of aquaphotomics using NIR for the post-harvest monitoring, and quality evaluation of strawberry and other agricultural products at different storage conditions.
Data availability statement
The raw data supporting the conclusions of this article will be made available by the authors, without undue reservation.
Author contributions
TF, TK, and RT: conceptualization and resources. RT, SA, and JM: methodology and investigation. SA: software. SA, TF, and RT: validation. SA and JM: formal analysis, writing—original draft preparation, and visualization. SA and RT: data curation. JM: writing—review and editing. RT: supervision and project administration. RT and TK: funding acquisition. All authors read and agreed to the published version of the manuscript.
Acknowledgments
SA gratefully acknowledges the financial support provided by the government scholarships from the Office of the Civil Service Commission (OCSC), Thailand. We gratefully acknowledge the help of Nichiei Intec Co., Ltd., Japan for providing the refrigerators used for cold storage.
Conflict of interest
The cooling systems examined in this study are produced by the Nichiei Intec Co., Ltd., a company where TF and TK were employed. All opinions presented in this manuscript belong to the authors alone, and not to any institution to which they are or were affiliated.
The remaining authors declare that the research was conducted in the absence of any commercial or financial relationships that could be construed as a potential conflict of interest.
Publisher’s note
All claims expressed in this article are solely those of the authors and do not necessarily represent those of their affiliated organizations, or those of the publisher, the editors and the reviewers. Any product that may be evaluated in this article, or claim that may be made by its manufacturer, is not guaranteed or endorsed by the publisher.
Supplementary material
The Supplementary Material for this article can be found online at: https://www.frontiersin.org/articles/10.3389/fnut.2022.1058173/full#supplementary-material
References
1. Seeram N, Adams L, Zhang Y, Lee R, Sand D, Scheuller H, et al. Blackberry, black raspberry, blueberry, cranberry, red raspberry, and strawberry extracts inhibit growth and stimulate apoptosis of human cancer cells in vitro. J Agric Food Chem. (2006) 54:9329–39. doi: 10.1021/jf061750g
2. Yang F, Li H, Li F, Xin Z, Zhao L, Zheng Y, et al. Effect of nano-packing on preservation quality of fresh strawberry (fragaria ananassa duch. cv fengxiang) during storage at 4 °c. J Food Sci. (2010) 75:C236–40. doi: 10.1111/j.1750-3841.2010.01520.x
3. Basu A, Nguyen A, Betts N, Lyons T. Strawberry as a functional food: an evidence-based review. Crit Rev Food Sci Nutr. (2014) 54:790–806. doi: 10.1080/10408398.2011.608174
4. Vu K, Hollingsworth R, Leroux E, Salmieri S, Lacroix M. Development of edible bioactive coating based on modified chitosan for increasing the shelf life of strawberries. Food Res Int. (2011) 44:198–203. doi: 10.1016/j.foodres.2010.10.037
5. Hernández-Muñoz P, Almenar E, Ocio M, Gavara R. Effect of calcium dips and chitosan coatings on postharvest life of strawberries (Fragaria x ananassa). Postharvest Biol Technol. (2006) 39:247–53. doi: 10.1016/j.postharvbio.2005.11.006
6. Crisosto C, Garner D, Doyle J, Day K. Relationship between fruit respiration, bruising susceptibility, and temperature in sweet cherries. HortScience. (1993) 28:132–5. doi: 10.21273/HORTSCI.28.2.132
7. Tahir H, Xiaobo Z, Jiyong S, Mahunu G, Zhai X, Mariod A. Quality and postharvest-shelf life of cold-stored strawberry fruit as affected by gum arabic (Acacia senegal) edible coating. J Food Biochem. (2018) 42:e12527. doi: 10.1111/jfbc.12527
8. Han C, Zhao Y, Leonard S, Traber M. Edible coatings to improve storability and enhance nutritional value of fresh and frozen strawberries (Fragaria x ananassa) and raspberries (Rubus ideaus). Postharvest Biol Technol. (2004) 33:67–78. doi: 10.1016/j.postharvbio.2004.01.008
9. BaoGang W, WenSheng L, JunJun Y, Lei S, XiaoYuan F, YuRu H, et al. Effect of pre-cooling on qualities of strawberry during logistics. J Fruit Sci. (2013) 30:878–83.
10. Barrazueta-Rojas S, Falconí J, Ojeda M, Oleas-López J, Mendoza-Zurita G. Pysicochemical properties and application of edible coatings in strawberry Fragaria × ananassa) preservation. Rev Fac Nac Agron Medellin. (2018) 71:8631–41. doi: 10.15446/rfnam.v71n3.73548
11. Pan J, Vicente A, Martínez G, Chaves A, Civello P. Combined use of UV-C irradiation and heat treatment to improve postharvest life of strawberry fruit. J Sci Food Agric. (2004) 84:1831–8. doi: 10.1002/jsfa.1894
12. Petriccione M, Mastrobuoni F, Pasquariello M, Zampella L, Nobis E, Capriolo G, et al. Effect of chitosan coating on the postharvest quality and antioxidant enzyme system response of strawberry fruit during cold storage. Foods. (2015) 4:501–23. doi: 10.3390/foods4040501
13. Barikloo H, Ahmadi E. Effect of nanocomposite-based packaging and chitosan coating on the physical, chemical, and mechanical traits of strawberry during storage. J Food Meas Charact. (2018) 12:1795–817. doi: 10.1007/s11694-018-9795-3
14. Wang Y, Li R, Lu R, Xu J, Hu K, Liu Y. Preparation of Chitosan/Corn Starch/Cinnamaldehyde Films for Strawberry Preservation. Foods. (2019) 8:423. doi: 10.3390/foods8090423
15. Lal Basediya A, Samuel D, Beera V. Evaporative cooling system for storage of fruits and vegetables - A review. J Food Sci Technol. (2013) 50:429–42. doi: 10.1007/s13197-011-0311-6
16. Pérez A, Olías R, Olías J, Sanz C. Strawberry quality as a function of the “high pressure fast cooling” design. Food Chem. (1998) 62:161–8. doi: 10.1016/S0308-8146(97)00211-2
17. Nunes M, Brecht J, Sargent S, Morais A. Effects of delays to cooling and wrapping on strawberry quality (cv. Sweet Charlie). Food Control. (1995) 6:323–8. doi: 10.1016/0956-7135(95)00024-0
18. Ouattara B, Sabato S, Lacroix M. Use of gamma-irradiation technology in combination with edible coating to produce shelf-stable foods. Radiat Phys Chem. (2002) 63:305–10. doi: 10.1016/S0969-806X(01)00517-5
19. Harker F, Elgar H, Watkins C, Jackson P, Hallett I. Physical and mechanical changes in strawberry fruit after high carbon dioxide treatments. Postharvest Biol Technol. (2000) 19:139–46. doi: 10.1016/S0925-5214(00)00090-9
20. Lazaridou A, Biliaderis C. Thermophysical properties of chitosan, chitosan-starch and chitosan-pullulan films near the glass transition. Carbohydr Polym. (2002) 48:179–90. doi: 10.1016/S0144-8617(01)00261-2
21. Jayakumar R, Nwe N, Tokura S, Tamura H. Sulfated chitin and chitosan as novel biomaterials. Int J Biol Macromol. (2007) 40:175–81. doi: 10.1016/j.ijbiomac.2006.06.021
22. Plumb A, Downing P, Parry A. Consumer attitudes to food waste and food packaging. Barbury,: Wrap (2013). p. 75.
23. Céline M, Valérie G, Karine G, Sandrine C, Nathalie G, Stéphane G, et al. Consumer behaviour in the prediction of postharvest losses reduction for fresh strawberries packed in modified atmosphere packaging. Postharvest Biol Technol. (2020) 163:111119. doi: 10.1016/j.postharvbio.2020.111119
24. Yokokawa N, Kikuchi-Uehara E, Sugiyama H, Hirao M. Framework for analyzing the effects of packaging on food loss reduction by considering consumer behavior. J Clean Prod. (2018) 174:26–34. doi: 10.1016/j.jclepro.2017.10.242
25. Holcroft D, Kader A. Carbon dioxide–induced changes in color and anthocyanin synthesis of stored strawberry fruit. HortScience. (1999) 34:1244–8.
26. Pelayo C, Ebeler S, Kader A. Postharvest life and flavor quality of three strawberry cultivars kept at 5°C in air or air+20 kPa CO2. Postharvest Biol Technol. (2003) 27:171–83. doi: 10.1016/S0925-5214(02)00059-5
27. Klopotek Y, Otto K, Böhm V. Processing strawberries to different products alters contents of vitamin C, total phenolics, total anthocyanins, and antioxidant capacity. J Agric Food Chem. (2005) 53:5640–6. doi: 10.1021/jf047947v
28. Ivanovich K, Evgenyevich S, Vasilyevich G, Nikolaevna D, Igorevich V. Features of usage of electromagnetic field of extremely low frequency for the storage of agricultural products. J Electromagn Anal Appl. (2013) 05:236–41. doi: 10.4236/jemaa.2013.55038
29. Aslanova M, Dydykin A, Fedulova LV, Derevitskaya O. An effect of the electromagnetic treatment on oxidative stability and microbiological safety of meat semi-prepared products. Theory Pract meat Process. (2017) 2:39–48. doi: 10.21323/2414-438X-2017-2-3-39-48
30. Ahmed J, Ramaswamy H. Applications of magnetic field in food preservation. In: Rahman M editor. Handbook of food preservation. Boca Raton: CRC Press (2020). p. 873–84. doi: 10.1201/9780429091483-55
31. Guo L, Azam S, Guo Y, Liu D, Ma H. Germicidal efficacy of the pulsed magnetic field against pathogens and spoilage microorganisms in food processing: an overview. Food Control. (2022) 136:108496. doi: 10.1016/J.FOODCONT.2021.108496
32. Kang T, You Y, Jun S. Supercooling preservation technology in food and biological samples: a review focused on electric and magnetic field applications. Food Sci Biotechnol. (2020) 29:303–21. doi: 10.1007/S10068-020-00750-6/FIGURES/6
33. Workman J. Handbook of organic compounds: NIR, IR, Raman, and UV spectra featuring polymers and surfaces. San Diego, CA: Academic Press (2001). p. 503.
34. Tsenkova R. Introduction aquaphotomics: dynamic spectroscopy of aqueous and biological systems describes peculiarities of water. J Near Infrared Spectrosc. (2009) 17:303–14.
35. Tsenkova R. Aquaphotomics: dynamic spectroscopy of aqueous and biological systems describes peculiarities of water. J Near Infrared Spectrosc. (2009) 17:303–13.
36. Vanoli M, Lovati F, Grassi M, Buccheri M, Zanella A, Cattaneo MP, et al. Water spectral pattern as a marker for studying apple sensory texture. Adv Hortic Sci. (2018) 32:343–51. doi: 10.13128/ahs-22380
37. Cattaneo T, Cutini M, Cammerata A, Stellari A, Marinoni L, Bisaglia C, et al. Near infrared spectroscopic and aquaphotomic evaluation of the efficiency of solar dehydration processes in pineapple slices. J Near Infrared Spectrosc. (2021) 29:352–8.
38. Kaur H. Investigating aquaphotomics for fruit quality assessment. Hamilton: The University of Waikato (2020). p. 227.
39. Kaur H, Künnemeyer R, McGlone A. Investigating aquaphotomics for temperature-independent prediction of soluble solids content of pure apple juice. J Near Infrared Spectrosc. (2020) 28:103–12. doi: 10.1177/0967033519898891
40. Cattaneo T, Vanoli M, Grassi M, Rizzolo A, Barzaghi S. The aquaphotomics approach as a tool for studying the influence of food coating materials on cheese and winter melon samples. J Near Infrared Spectrosc. (2016) 24:381–90. doi: 10.1255/jnirs.1238
41. Marinoni L, Buccheri M, Bianchi G, Cattaneo T. Aquaphotomic, E-nose and electrolyte leakage to monitor quality changes during the storage of ready-to-eat rocket. Molecules. (2022) 27:2252. doi: 10.3390/molecules27072252
42. Nugraha D, Zaukuu J, Bósquez J, Bodor Z, Vitalis F, Kovacs Z. Near-infrared spectroscopy and aquaphotomics for monitoring mung bean (Vigna radiata) sprout growth and validation of ascorbic acid content. Sensors. (2021) 21:611. doi: 10.3390/S21020611
43. Tsenkova R, Muncan J. Aquaphotomics for bio-diagnostics in dairy - applications of near-infrared spectroscopy. Singapore: Springer (2021).
44. Muncan J, Kovacs Z, Pollner B, Ikuta K, Ohtani Y, Terada F, et al. Near infrared aquaphotomics study on common dietary fatty acids in cow’s liquid, thawed milk. Food Control. (2020) 122:107805. doi: 10.1016/j.foodcont.2020.107805
45. Slavchev A, Kovacs Z, Koshiba H, Bazar G, Pollner B, Krastanov A, et al. Monitoring of water spectral patterns of lactobacilli as a tool for rapid selection of probiotic candidates. J Near Infrared Spectrosc. (2017) 25:423–31.
46. Slavchev A, Kovacs Z, Koshiba H, Nagai A, Bázár G, Krastanov A, et al. Monitoring of water spectral pattern reveals differences in probiotics growth when used for rapid bacteria selection. PLoS One. (2015) 10:e0130698. doi: 10.1371/journal.pone.0130698
47. Muncan J, Tei K, Tsenkova R. Real-time monitoring of yogurt fermentation process by aquaphotomics near-infrared spectroscopy. Sensors. (2021) 21:177. doi: 10.3390/s21010177
48. Morita H, Hasunuma T, Vassileva M, Kondo A, Tsenkova R. A new screening method for recombinant Saccharomyces cerevisiae strains based on their xylose fermentation ability measured by near infrared spectroscopy. Anal Methods. (2014) 6:6628–34. doi: 10.1039/c4ay00785a
49. Morita H, Hasunuma T, Vassileva M, Tsenkova R, Kondo A. Near infrared spectroscopy as high-throughput technology for screening of xylose-fermenting recombinant Saccharomyces cerevisiae strains. Anal Chem. (2011) 83:4023–9. doi: 10.1021/ac103128p
50. Kinoshita K, Miyazaki M, Morita H, Vassileva M, Tang C, Li D, et al. Spectral pattern of urinary water as a biomarker of estrus in the giant panda. Sci Rep. (2012) 2:856. doi: 10.1038/srep00856
51. Kinoshita K, Morita H, Miyazaki M, Hama N, Kanemitsu H, Kawakami H, et al. Near infrared spectroscopy of urine proves useful for estimating ovulation in giant panda (Ailuropoda melanoleuca). Anal Methods. (2010) 2:1671–5. doi: 10.1039/c0ay00333f
52. Santos-Rivera M, Woolums A, Thoresen M, Meyer F, Vance C. Bovine respiratory syncytial virus (BRSV) infection detected in exhaled breath condensate of dairy calves by near-infrared aquaphotomics. Molecules. (2022) 27:549. doi: 10.3390/MOLECULES27020549
53. Santos-Rivera M, Woolums A, Thoresen M, Blair E, Jefferson V, Meyer F, et al. Profiling Mannheimia haemolytica infection in dairy calves using near infrared spectroscopy (NIRS) and multivariate analysis (MVA). Sci Rep. (2021) 11:1392. doi: 10.1038/s41598-021-81032-x
54. Cui X, Yu X, Cai W, Shao X. Water as a probe for serum–based diagnosis by temperature– dependent near–infrared spectroscopy. Talanta. (2019) 204:359–66. doi: 10.1016/j.talanta.2019.06.026
55. Li Y, Guo L, Li L, Yang C, Guang P, Huang F, et al. Early diagnosis of type 2 diabetes based on near-infrared spectroscopy combined with machine learning and aquaphotomics. Front Chem. (2020) 8:580489. doi: 10.3389/fchem.2020.580489
56. Malegori C, Muncan J, Mustorgi E, Tsenkova R, Oliveri P. Analysing the water spectral pattern by near-infrared spectroscopy and chemometrics as a dynamic multidimensional biomarker in preservation: rice germ storage monitoring. Spectrochim Acta A Mol Biomol Spectrosc. (2022) 265:120396. doi: 10.1016/J.SAA.2021.120396
57. van de Kraats E, Munćan J, Tsenkova R. Aquaphotomics – origin, concept, applications and future perspectives. Substantia. (2019) 3:13–28. doi: 10.13128/substantia-702
58. Muncan J, Tsenkova R. Aquaphotomics—from innovative knowledge to integrative platform in science and technology. Molecules. (2019) 24:2742. doi: 10.3390/molecules24152742
59. Tsenkova R, Kovacs Z, Kubota Y. Aquaphotomics: near infrared spectroscopy and water states in biological systems. In: DiSalvo E editor. Membrane hydration. Cham: Springer (2015). p. 189–211. doi: 10.1007/978-3-319-19060-0_8
60. Muncan J, Aouadi B, Tsenkova R. New perspectives in plant and plant-based food quality determination: aquaphotomics. In: Goyal M, Kovacs Z, Nath A, Suleria H editors. Plant-based bioactives, functional foods, beverages and medicines: processing, analysis and health benefit. New Jersey, NJ: Apple Academic Press (2023).
61. Nichiei Intec Co. Ltd. 日栄インテックの鮮度保持システム - Nichi intec preservation system. (2018). Available online at: https://www.nichieiintec.jp/scs/ (accessed September 13, 2022).
62. Dhanoa M, Barnes R, Lister S. Standard normal variate transformation and de-trending of near-infrared diffuse reflectance spectra. Appl Spectrosc. (1989) 43:772–7.
63. Wold S, Sjostrom M. SIMCA: a method for analyzing chemical data in terms of similarity and analogy. Washington, DC: American Chemical Society (1977). p. 243–82. doi: 10.1021/bk-1977-0052.ch012
64. Martens H, Martens M. Multivariate analysis of quality?: an introduction. New York, NY: Wiley (2001). p. 445.
65. Gowen A, Downey G, Esquerre C, O’Donnell C. Preventing over-fitting in PLS calibration models of near-infrared (NIR) spectroscopy data using regression coefficients. J Chemom. (2011) 25:375–81. doi: 10.1002/cem.1349
66. Tsenkova R. Aquaphotomics: water in the biological and aqueous world scrutinised with invisible light. Spectrosc Eur. (2010) 22:6–10.
67. Tsenkova R, Munćan J, Pollner B, Kovacs Z. Essentials of aquaphotomics and its chemometrics approaches. Front Chem. (2018) 6:363. doi: 10.3389/fchem.2018.00363
68. Kovacs Z, Muncan J, Veleva P, Oshima M, Shigeoka S, Tsenkova R. Aquaphotomics for monitoring of groundwater using short-wavelength near-infrared spectroscopy. Spectrochim Acta Part A Mol Biomol Spectrosc. (2022) 279:121378. doi: 10.1016/J.SAA.2022.121378
69. Jiang Y, Yu L, Hu Y, Zhu Z, Zhuang C, Zhao Y, et al. The preservation performance of chitosan coating with different molecular weight on strawberry using electrostatic spraying technique. Int J Biol Macromol. (2020) 151:278–85. doi: 10.1016/j.ijbiomac.2020.02.169
70. Aharoni Y, Barkai-Golan R. Pre-harvest fungicide sprays and polyvinyl wraps to control Botrytis rot and prolong the post- harvest storage life of strawberries. J Hortic Sci. (1987) 62:177–81. doi: 10.1080/14620316.1987.11515767
71. Dhital R, Mora N, Watson D, Kohli P, Choudhary R. Efficacy of limonene nano coatings on post-harvest shelf life of strawberries. LWT. (2018) 97:124–34. doi: 10.1016/J.LWT.2018.06.038
72. Shehata S, Abdeldaym E, Ali M, Mohamed R, Bob R, Abdelgawad K. Effect of some citrus essential oils on post-harvest shelf life and physicochemical quality of strawberries during cold storage. Agron. (2020) 10:1466. doi: 10.3390/AGRONOMY10101466
73. Bahmani R, Razavi F, Mortazavi S, Gohari G, Juárez-Maldonado A. Evaluation of proline-coated chitosan nanoparticles on decay control and quality preservation of strawberry fruit (cv. Camarosa) during cold storage. Horticulture. (2022) 8:648. doi: 10.3390/HORTICULTURAE8070648
74. Hernández-Carrillo J, Orta-Zavalza E, González-Rodríguez S, Montoya-Torres C, Sepúlveda-Ahumada D, Ortiz-Rivera Y. Evaluation of the effectivity of reuterin in pectin edible coatings to extend the shelf-life of strawberries during cold storage. Food Packag Shelf Life. (2021) 30:100760. doi: 10.1016/J.FPSL.2021.100760
75. Tanada-Palmu P, Grosso C. Effect of edible wheat gluten-based films and coatings on refrigerated strawberry (Fragaria ananassa) quality. Postharvest Biol Technol. (2005) 36:199–208. doi: 10.1016/j.postharvbio.2004.12.003
76. Kojić D, Tsenkova R, Tomobe K, Yasuoka K, Yasui M. Water confined in the local field of ions. ChemPhysChem. (2014) 15:4077–86. doi: 10.1002/cphc.201402381
77. Arefi A, Sturm B, von Gersdorff G, Nasirahmadi A, Hensel O. Vis-NIR hyperspectral imaging along with Gaussian process regression to monitor quality attributes of apple slices during drying. LWT. (2021) 152:112297.
78. Kuroki S, Tsenkova R, Moyankova D, Muncan J, Morita H, Atanassova S, et al. Water molecular structure underpins extreme desiccation tolerance of the resurrection plant Haberlea rhodopensis. Sci Rep. (2019) 9:3049. doi: 10.1038/s41598-019-39443-4
79. Fujimoto T, Kobori H, Tsuchikawa S. Prediction of wood density independently of moisture conditions using near infrared spectroscopy. J Near Infrared Spectrosc. (2012) 20:353–9. doi: 10.1255/jnirs.994
80. Tsuchikawa S, Hirashima Y, Sasaki Y, Ando K. Near-infrared spectroscopic study of the physical and mechanical properties of wood with meso- and micro-scale anatomical observation. Appl Spectrosc. (2005) 59:86–93. doi: 10.1366/0003702052940413
81. Fujimoto T, Yamamoto H, Tsuchikawa S. Estimation of wood stiffness and strength properties of hybrid larch by near-infrared spectroscopy. Appl Spectrosc. (2007) 61:882–8. doi: 10.1366/000370207781540150
82. Rambo M, Ferreira M. Determination of cellulose crystallinity of banana residues using near infrared spectroscopy and multivariate analysis. J Braz Chem Soc. (2015) 26:1491–9. doi: 10.5935/0103-5053.20150118
83. Tsuchikawa S, Murata A, Kohara M, Mitsui K. Spectroscopic monitoring of biomass modification by light-irradiation and heat treatment. J Near Infrared Spectrosc. (2003) 11:401–5. doi: 10.1255/jnirs.391
84. Wu Y, Tsuchikawa S, Hayashi K. Application of near infrared spectroscopy to assessments of colour change in plantation-grown Eucalyptus grandis wood subjected to heat and steaming treatments. J Near Infrared Spectrosc. (2005) 13:371–6. doi: 10.1255/jnirs.568
85. Williams P. Influence of water on prediction of composition and quality factors: the aquaphotomics of low moisture agricultural materials. J Near Infrared Spectrosc. (2009) 17:315–28. doi: 10.1255/jnirs.862
86. Gao M, Guo W, Huang X, Du R, Zhu X. Effect of pressing and impacting bruises on optical properties of kiwifruit flesh. Postharvest Biol Technol. (2021) 172:111385. doi: 10.1016/J.POSTHARVBIO.2020.111385
87. Cheng J, Guo W, Du R, Zhou Y. Optical properties of different kiwifruit cultivars (Actinidia deliciosa and Actinidia chinensis) and their correlation with internal quality. Infrared Phys Technol. (2022) 123:104113. doi: 10.1016/J.INFRARED.2022.104113
88. Esquerre C, Gowen A, Tsenkova R, O’Donnell C, Downey G. Identification of water matrix coefficients in mushrooms (Agaricus bisporus) using robust ensemble of monte carlo uninformative variable eliminarion. Near infrared spectroscopy: proceedings of the 14th international conference. Bangkok: IM Publications Open (2009). p. 163–4.
89. Segtnan V, Šašić Š, Isaksson T, Ozaki Y, Šašić S, Isaksson T, et al. Studies on the structure of water using two-dimensional near-infrared correlation spectroscopy and principal component analysis. Anal Chem. (2001) 73:3153–61. doi: 10.1021/ac010102n
90. Frost R, Scholz R, Lõpez A. Raman and infrared spectroscopic characterization of the arsenate-bearing mineral tangdanite– and in comparison with the discredited mineral clinotyrolite. J Raman Spectrosc. (2015) 46:920–6. doi: 10.1002/JRS.4691
91. Rémazeilles C, Refait P. Fe(II) hydroxycarbonate Fe2(OH)2CO3 (chukanovite) as iron corrosion product: synthesis and study by fourier transform infrared spectroscopy. Polyhedron. (2009) 28:749–56. doi: 10.1016/J.POLY.2008.12.034
92. Litasov K, Ohtani E. Systematic study of hydrogen incorporation into fe-bearing wadsleyite and water storage capacity of the transition zone. AIP Conf Proc. (2008) 987:113. doi: 10.1063/1.2896954
93. Walker A, Demouchy S, Wright K. Computer modelling of the energies and vibrational properties of hydroxyl groups in α- and β-Mg2SiO4. Eur J Mineral. (2006) 18:529–43. doi: 10.1127/0935-1221/2006/0018-0529
94. Shi G, Stavola M, Pearton S, Thieme M, Lavrov EV, Weber J. Hydrogen local modes and shallow donors in ZnO. Phys Rev B Condens Matter Mater Phys. (2005) 72:195211. doi: 10.1103/PHYSREVB.72.195211/FIGURES/6/MEDIUM
95. Shi G, Saboktakin M, Stavola M, Pearton S. “Hidden hydrogen” in as-grown ZnO. Appl Phys Lett. (2004) 85:5601. doi: 10.1063/1.1832736
96. Jacobsen S, Demouchy S, Frost D, Ballaran T, Kung J. A systematic study of OH in hydrous wadsleyite from polarized FTIR spectroscopy and single-crystal X-ray diffraction: oxygen sites for hydrogen storage in Earth’s interior. Am Mineral. (2005) 90:61–70. doi: 10.2138/AM.2005.1624
97. Seyer J, Luner P, Kemper M. Application of diffuse reflectance near-infrared spectroscopy for determination of crystallinity. J Pharm Sci. (2000) 89:1305–16. doi: 10.1002/1520-6017(200010)89:103.0.CO;2-Q
98. Sagawa N, Shikata T. Hydration behavior of poly(ethylene oxide)s in aqueous solution as studied by near-infrared spectroscopic techniques. J Phys Chem B. (2013) 117:10883–8. doi: 10.1021/jp405794t
99. Awatani T, Midorikawa H, Kojima N, Ye J, Marcott C. Morphology of water transport channels and hydrophobic clusters in Nafion from high spatial resolution AFM-IR spectroscopy and imaging. Electrochem Commun. (2013) 30:5–8. doi: 10.1016/j.elecom.2013.01.021
100. Bázár G, Kovacs Z, Tanaka M, Furukawa A, Nagai A, Osawa M, et al. Water revealed as molecular mirror when measuring low concentrations of sugar with near infrared light. Anal Chim Acta. (2015) 896:52–62. doi: 10.1016/J.ACA.2015.09.014
102. Dopfer O, Roth D, Maier J. Microsolvation of the water cation in neon: infrared spectra and potential energy surface of the H2O+-Ne open-shell ionic complex. J Chem Phys. (2001) 114:7081–93. doi: 10.1063/1.1359770
103. Musto P, Galizia M, Pannico M, Scherillo G, Mensitieri G. Time-resolved Fourier transform infrared spectroscopy, gravimetry, and thermodynamic modeling for a molecular level description of water sorption in poly(ε-caprolactone). J Phys Chem B. (2014) 118:7414–29. doi: 10.1021/JP502270H/SUPPL_FILE/JP502270H_SI_001.PDF
104. Schwanninger M, Rodrigues J, Fackler KA. Review of band assignments in near infrared spectra of wood and wood components. J Near Infrared Spectrosc. (2011) 19:287–308. doi: 10.1255/jnirs.955
105. Kakuda H, Okada T, Hasegawa T. Temperature-induced molecular structural changes of linear poly(ethylene imine) in water studied by mid-infrared and near-infrared spectroscopies. J Phys Chem B. (2009) 113:13910–6. doi: 10.1021/JP9048204
106. Via B, Jiang W. Nonlinear multivariate modeling of strand mechanical properties with near-infrared spectroscopy. For Chron. (2013) 89:621–30. doi: 10.5558/TFC2013-113
107. Knee M, Sargent J, Osborne D. Cell wall metabolism in developing strawberry fruits. J Exp Bot. (1977) 28:377–93.
108. Atungulu G, Nishiyama Y, Koide S. Electrode configuration and polarity effects on physicochemical properties of electric field treated apples post harvest. Biosyst Eng. (2004) 87:313–23. doi: 10.1016/J.BIOSYSTEMSENG.2003.11.011
109. Zhang L, Noda I, Czarnik-Matusewicz B, Wu Y. Multivariate estimation between mid and near-infrared spectra of hexafluoroisopropanol-water mixtures. Anal Sci. (2007) 23:901–5. doi: 10.2116/analsci.23.901
110. Solcaniova E, Kovac S. Hydrogen bonding in phenols. IV. Intramolecular OH. n Hydrogen bonds of some alkyl derivatives. Chem Zvesti. (1969) 691:687–91.
111. Frost R, Dickfos M, Čejka J. Raman spectroscopic study of the uranyl carbonate mineral zellerite. J Raman Spectrosc. (2008) 39:582–6. doi: 10.1002/JRS.1879
112. Bertie J, Whalley E. Infrared spectra of ices II, III, and V in the range 4000 to 350 cm -1. J Chem Phys. (1964) 40:1646–59. doi: 10.1063/1.1725374
113. Osborne B. Near infrared spectroscopic studies of starch and water in some processed cereal foods. J Near Infrared Spectrosc. (1996) 4:195–200. doi: 10.1255/jnirs.90
114. Som T, Karmakar B. Structure and properties of low-phonon antimony glasses and nano glass-ceramics in K2O–B2O3–Sb2O3 system. J Non Cryst Solids. (2010) 356:987–99. doi: 10.1016/J.JNONCRYSOL.2010.01.026
115. Cai C, Tao Y, Wang B, Wen M, Yang H, Cheng Y. Investigating the adsorption process of isoamyl alcohol vapor onto silica gel with near-infrared process analytical technology. Spectrosc Lett. (2014) 48:190–7. doi: 10.1080/00387010.2013.872668
116. Frost R, Erickson K, Čejka J, Reddy BJ. A Raman spectroscopic study of the uranyl sulphate mineral johannite. Spectrochim Acta A Mol Biomol Spectrosc. (2005) 61:2702–7. doi: 10.1016/J.SAA.2004.10.013
117. Wenz J. Influence of steroids on hydrogen bonds in membranes assessed by near infrared spectroscopy. Biochim Biophys Acta Biomembr. (2021) 1863:183553. doi: 10.1016/j.bbamem.2021.183553
118. Mastrapa R, Moore M, Hudson R, Ferrante R, Brown R, Mastrapa R, et al. Proton irradiation of crystalline water ice: timescales for amorphization in the kuiper belt. DPS. (2005) 37:56.10.
119. Kandpal L, Lohumi S, Kim M, Kang J, Cho B. Near-infrared hyperspectral imaging system coupled with multivariate methods to predict viability and vigor in muskmelon seeds. Sensors Actuators B Chem. (2016) 229:534–44. doi: 10.1016/j.snb.2016.02.015
120. Hong B, Rubenthaler G, Allan R. Wheat pentosans. II. Estimating kernel hardness and pentosans in water extracts by near-infrared reflectance. Cereal Chem. (1989) 66:374–7.
121. Omar A, Atan H, Matjafri M. Peak response identification through near-infrared spectroscopy analysis on aqueous sucrose, glucose, and fructose solution. Spectrosc Lett. (2012) 45:190–201. doi: 10.1080/00387010.2011.604065
122. Omar A, Matjafri M. Near infrared spectral linearisation in quantifying soluble solids content of intact carambola. Sensors. (2013) 13:4876–83. doi: 10.3390/s130404876
123. Gowen A, Tsenkova R, Esquerre C, Downey G, O’Donnell C. Use of near infrared hyperspectral imaging to identify water matrix co-ordinates in mushrooms (Agaricus bisporus) subjected to mechanical vibration. J Near Infrared Spectrosc. (2009) 17:363–71. doi: 10.1255/jnirs.860
124. Sun X, Dong X, Cai L, Hao Y, Ouyang A, Liu Y. Visible-NIR spectroscopy and least square support vector machines regression for determination of vitamin c of mandarin fruit. Sens Lett. (2012) 10:506–10. doi: 10.1166/sl.2012.1891
125. Lu R. Multispectral imaging for predicting firmness and soluble solids content of apple fruit. Postharvest Biol Technol. (2004) 31:147–57. doi: 10.1016/j.postharvbio.2003.08.006
126. Lu R. Prediction of apple fruit firmness by near-infrared multispectral scattering. J Texture Stud. (2004) 35:263–76. doi: 10.1111/j.1745-4603.2004.tb00837.x
127. Chatani E, Tsuchisaka Y, Masuda Y, Tsenkova R. Water molecular system dynamics associated with amyloidogenic nucleation as revealed by real time near infrared spectroscopy and aquaphotomics. PLoS One. (2014) 9:e101997. doi: 10.1371/journal.pone.0101997
128. Heiman A, Licht S. Fundamental baseline variations in aqueous near-infrared analysis. Anal Chim Acta. (1999) 394:135–47. doi: 10.1016/S0003-2670(99)00312-8
129. Tsenkova R, Iordanova I, Toyoda K, Brown D. Prion protein fate governed by metal binding. Biochem Biophys Res Commun. (2004) 325:1005–12. doi: 10.1016/j.bbrc.2004.10.135
130. Pu Y, Ragauskas A, Lucia L, Naithani V, Jameel H. Near-infrared spectroscopy and chemometric analysis for determining oxygen delignification yield. J Wood Chem Technol. (2008) 28:122–36. doi: 10.1080/02773810802125008
131. Awais M, Altgen M, Mäkelä M, Altgen D, Rautkari L. Hyperspectral near-infrared image assessment of surface-acetylated solid wood. ACS Appl Bio Mater. (2020) 3:5223–32. doi: 10.1021/ACSABM.0C00626/ASSET/IMAGES/LARGE/MT0C00626_0005.JPEG
132. Freitas E, Brito A, de Oliveira S, de Oliveira E. Early diagnosis of cassava frog skin disease in powdered tissue samples using near-infrared spectroscopy. Eur J Plant Pathol. (2020) 156:547–58. doi: 10.1007/S10658-019-01904-X/FIGURES/4
133. Dacal-Nieto A, Formella A, Carrión P, Vazquez-Fernandez E, Fernández-Delgado M. Common scab detection on potatoes using an infrared hyperspectral imaging system. In: Maino G, Foresti G editors. Image analysis and processing – ICIAP 2011. Lecture notes in computer science. Berlin: Springer (2011). p. 303–12. doi: 10.1007/978-3-642-24088-1_32
134. Herklotz F, Chaplygin I, Lavrov EV, Neiman A, Reeves R, Allen M. Bistability of a hydrogen defect with a vibrational mode at 3326cm-1 in ZnO. Phys Rev B. (2019) 99:115203. doi: 10.1103/PHYSREVB.99.115203/FIGURES/7/MEDIUM
135. Wang W, Jin X, Zhu Y, Zhu C, Yang J, Wang H, et al. Effect of vapor-phase glutaraldehyde crosslinking on electrospun starch fibers. Carbohydr Polym. (2016) 140:356–61. doi: 10.1016/J.CARBPOL.2015.12.061
136. Yang X, Guang P, Xu G, Zhu S, Chen Z, Huang F. Manuka honey adulteration detection based on near-infrared spectroscopy combined with aquaphotomics. LWT. (2020) 132:109837. doi: 10.1016/j.lwt.2020.109837
137. Badaró A, Morimitsu F, Ferreira A, Clerici M, Fernandes Barbin D. Identification of fiber added to semolina by near infrared (NIR) spectral techniques. Food Chem. (2019) 289:195–203. doi: 10.1016/J.FOODCHEM.2019.03.057
138. Sannia M, Serva L, Balzan S, Segato S, Novelli E, Fasolato L. Application of near-infrared spectroscopy for frozen-thawed characterization of cuttlefish (Sepia officinalis). J Food Sci Technol. (2019) 56:4437–47. doi: 10.1007/s13197-019-03957-6
139. Andueza D, Picard F, Hocquette J, Listrat A. Prediction of the intramuscular connective tissue components of fresh and freeze-dried samples by near infrared spectroscopy. Meat Sci. (2021) 179:108537. doi: 10.1016/J.MEATSCI.2021.108537
140. Fengel D. Influence of water on the OH valency range in deconvoluted FTIR spectra of cellulose. Holzforschung. (1993) 47:103–8. doi: 10.1515/HFSG.1993.47.2.103/MACHINEREADABLECITATION/RIS
141. Paladini G, Venuti V, Crupi V, Majolino D, Fiorati A, Punta C. 2D correlation spectroscopy (2DCoS) analysis of temperature-dependent FTIR-ATR spectra in branched polyethyleneimine/TEMPO-oxidized cellulose nano-fiber xerogels. Polymers. (2021) 13:528. doi: 10.3390/POLYM13040528
142. Hassanzadeh A, Murphy S, Pethybridge S, van Aardt J. Growth stage classification and harvest scheduling of snap bean using hyperspectral sensing: a greenhouse study. Remote Sens. (2020) 12:3809. doi: 10.3390/RS12223809
143. Devasahayam S, Bandyopadhyay S, Hill D. Study of victorian brown coal dewatering by super absorbent polymers using attenuated total reflection fourier transform infrared spectroscopy. Miner Process Extr Metall Rev. (2016) 37:220–6. doi: 10.1080/08827508.2016.1168417
144. Liu J, Liu R, Xu K. Accuracy of noninvasive glucose sensing based on near-infrared spectroscopy. Appl Spectrosc. (2015) 69:1313–8. doi: 10.1366/14-07728
145. Saiga N, Hamada C, Ikeda J. Near infrared spectroscopy assessment of the glucose solution processed by ultrasonic cavitation. Ultrasonics. (2006) 44:e101–4. doi: 10.1016/J.ULTRAS.2006.06.029
146. Wiercigroch E, Szafraniec E, Czamara K, Pacia M, Majzner K, Kochan K, et al. Raman and infrared spectroscopy of carbohydrates: a review. Spectrochim Acta A Mol Biomol Spectrosc. (2017) 185:317–35. doi: 10.1016/J.SAA.2017.05.045
147. Li H, Lv X, Wang J, Li J, Yang H, Qin Y. Quantitative determination of soybean meal content in compound feeds: comparison of near-infrared spectroscopy and real-time PCR. Anal Bioanal Chem. (2007) 389:2313–22. doi: 10.1007/S00216-007-1624-1/FIGURES/6
148. Unger R, Braun U, Fankhänel J, Daum B, Arash B, Rolfes R. Molecular modelling of epoxy resin crosslinking experimentally validated by near-infrared spectroscopy. Comput Mater Sci. (2019) 161:223–35. doi: 10.1016/J.COMMATSCI.2019.01.054
149. Chen S, Wang C, Tsai C, Yang I, Luo S, Chuang Y. Fermentation quality evaluation of tea by estimating total catechins and theanine using near-infrared spectroscopy. Vib Spectrosc. (2021) 115:103278. doi: 10.1016/J.VIBSPEC.2021.103278
Keywords: strawberry, cold storage, aquaphotomics, near infrared spectroscopy, water, water molecular structure, electric field (EF), monitoring
Citation: Muncan J, Anantawittayanon S, Furuta T, Kaneko T and Tsenkova R (2022) Aquaphotomics monitoring of strawberry fruit during cold storage – A comparison of two cooling systems. Front. Nutr. 9:1058173. doi: 10.3389/fnut.2022.1058173
Received: 30 September 2022; Accepted: 21 November 2022;
Published: 09 December 2022.
Edited by:
John-Lewis Zinia Zaukuu, Kwame Nkrumah University of Science and Technology, GhanaReviewed by:
Alessandra Biancolillo, University of L’Aquila, ItalyLei Nie, Shandong University, China
Copyright © 2022 Muncan, Anantawittayanon, Furuta, Kaneko and Tsenkova. This is an open-access article distributed under the terms of the Creative Commons Attribution License (CC BY). The use, distribution or reproduction in other forums is permitted, provided the original author(s) and the copyright owner(s) are credited and that the original publication in this journal is cited, in accordance with accepted academic practice. No use, distribution or reproduction is permitted which does not comply with these terms.
*Correspondence: Roumiana Tsenkova, cnRzZW5Aa29iZS11LmFjLmpw
†These authors have contributed equally to this work and share first authorship