- 1Department of Gastric and Colorectal Surgery, General Surgery Center, The First Hospital of Jilin University, Changchun, Jilin, China
- 2Department of Clinical Epidemiology, The First Hospital of Jilin University, Changchun, Jilin, China
Background: Gastro-oesophageal reflux disease (GORD) is a common gastrointestinal dysfunction that significantly affects the quality of daily life, and health interventions are challenging to prevent the risk of GORD. In this study, we used Mendelian randomization framework to genetically determine the causal associations between multifaceted modifiable factors and the risk of GORD.
Materials and methods: Sixty-six exposures with available instrumental variables (IVs) across 6 modifiable pathways were included in the univariable MR analysis (UVMR). Summary-level genome-wide association studies (GWAS) datasets for GORD were retrieved from the Neale Lab (GORDNeale, Ncases = 29975, Ncontrols = 390556) and FinnGen (GORDFinn, Ncases = 13141, Ncontrols = 89695). Using the METAL software, meta-analysis for single nucleotide polymorphisms (SNPs) from GORDNeale and GORDFinn was conducted with an inverse variance weighted (IVW) fixed-effect model. Moreover, we leveraged partition around medoids (PAM) clustering algorithm to cluster genetic correlation subtypes, whose hub exposures were conditioned for multivariable MR (MVMR) analyses. P-values were adjusted with Bonferroni multiple comparisons.
Results: Significant causal associations were identified between 26 exposures (15 risk exposures and 11 protective exposures) and the risk of GORD. Among them, 13 risk exposures [lifetime smoking, cigarette consumption, insomnia, short sleep, leisure sedentary behavior (TV watching), body mass index (BMI), body fat percentage, whole body fat mass, visceral adipose tissue, waist circumference, hip circumference, major depressive disorder, and anxious feeling], and 10 protective exposures (leisure sedentary behavior (computer use), sitting height, hand grip strength (left and right), birth weight, life satisfaction, positive affect, income, educational attainment, and intelligence) showed novel significant causal associations with the risk of GORD. Moreover, 13 exposures still demonstrated independent associations with the risk of GORD following MVMR analyses conditioned for hub exposures (educational attainment, smoking initiation and BMI). In addition, 12 exposures showed suggestive causal associations with the risk of GORD.
Conclusion: This study systematically elucidated the modifiable factors causally associated with the risk of GORD from multifaceted perspectives, which provided implications for prevention and treatment of GORD.
Introduction
As a common digestive system disease, GORD clinically presents with intraesophageal symptoms, such as acid regurgitation, heartburn and chest pain, and extraesophageal symptoms, including bronchial asthma, chronic cough, and hoarseness (1). Long-term abnormal reflux also increases the risk of pathological oesophageal stricture or even oesophageal adenocarcinoma (2). Currently, the highest prevalence of GORD is observed in North and Central America, followed by Europe (3). GORD symptoms can be influenced by multiple determinants, such as excessive acid exposure caused by anatomical or physiological defects of the oesophagogastric junction, the frequencies of reflux attacks and the acidity of reflux fluid (4). Moreover, poor health status and lifestyle, such as obesity (5, 6), smoking (6, 7), and sleep disorders (8, 9), are common precipitating factors that unilaterally or mutually affect the risk of GORD. However, there is insufficient evidence to determine the causal relationship between each factor and the risk of GORD due to limited studies, potential confounding factors and reverse causalities. In this case, it is of great significance to clarify the risk factors and protective factors related to the causations of GORD in all aspects for the prevention, clinical diagnosis and the treatment of GORD.
MR mainly relies on IVs to detect and quantify causality between exposure and outcome (10). This design overcome the effects of the potential residual confounders and the reverse causalities (11). With the development of the genome-wide association studies (GWAS) based on large sample sizes (12), MR analysis offers a more accurate and representative assessment of the causal associations between exposures and outcomes by primarily using genetic variants. Firstly, according to the Mendel’s second law, the inheritance of a trait is independent of others with randomness. Therefore, the genetic variants of the offspring will not be disturbed by environmental confounding factors (13). Secondly, the distributions of the genetic variants precede the acquired exposures and outcomes including various diseases, so that the order of the three factors is in line with the causal timing and is not affected by reverse causality (14). Thirdly, genetic variants associated with specific exposures will index lifetime differences and thus produce causal estimates that are unsusceptible to the attenuation by errors (regression dilution bias) (15). To date, central obesity indicators (BMI, waist circumference, hip circumference) and daily habits (smoking and alcohol consumption) have been examined for their causal effects on the risk of GORD using MR analysis (16). However, there have been no studies evaluating factors that are causally associated with the risk of GORD as comprehensively as possible.
In this study, we screened a total of 66 exposures across 6 modifiable pathways (daily habits, health status, nutritional and biochemical biomarkers, nutritional and developmental status, emotional factors, socioeconomic factors) for the purpose of identifying the factors that causally associated with the risk of GORD using MR analysis in the discovery and replication phases. Moreover, a meta-analysis based on the above two-phase determined the factors that were causally associated with the risk of GORD to the greatest extent, and also enhanced the statistical efficacy of MR results in the discovery and replication phases.
Materials and methods
Identification of exposures for Mendelian randomization analysis
We retrieved factors from PubMed that were associated with the risk of GORD with the query strategy “((Gastroesophageal Reflux[Mesh] OR GERD[Title/Abstract] OR GORD[Title/Abstract] OR Gastric Acid Reflux [Title/Abstract] OR Gastric Acid Reflux[Title/Abstract] OR Gastro Esophageal Reflux[Title/Abstract] OR Gastro oesophageal Reflux[Title/Abstract] OR Gastroesophageal Reflux[Title/Abstract] OR Gastro-oesophageal Reflux[Title/Abstract] OR Reflux, Gastroesophageal[Title/Abstract] OR Esophageal Reflux [Title/Abstract])) AND ((relative[Title/Abstract] AND risk[Title/Abstract]) OR (relative risk[Text Word]) OR (risks[Text Word])).” Reviews, meta-analyses and population-based studies were included, and we identified 58 modifiable factors (Supplementary Table 1) related to the risk of GORD. We next determined the numerous exposures used for MR analyses according to the definitions of the modifiable factors. (1) Factor “smoking behaviors” corresponded to the exposures: smoking initiation, lifetime smoking, cigarette consumption, age of initiation of smoking, and smoking cessation. (2) Factor “sleep disorders” corresponded to the exposures: insomnia, short sleep, long sleep, daytime sleepiness and daytime napping. (3) Factor “physical activity levels” corresponded to the exposures: leisure sedentary behavior (TV watching), leisure sedentary behavior (computer use), moderate to vigorous physical activity levels, 10+ min vigorous activity, strenuous sports or other exercises. (4) Factor “obesity” corresponded to the exposures: BMI, body fat percentage, whole body fat mass, visceral adipose tissue, waist circumference, hip circumference, and waist-to-hip ratio. (6) Factor “height” was categorized into exposures: standing height and sitting height. (7) Factor “muscle mass” corresponded to the exposures: whole body fat-free mass, hand grip strength (left and right). (8) Factor “positive subjective well-beings” corresponded to the exposures: life satisfaction and positive affect. Sex-specific and disease-specific phenotypes, such as “sex-hormone-related factors” and “common medications,” were excluded. Finally, the 66 exposures (Table 1; Supplementary Table 2) across 6 modifiable pathways were identified to explore causal associations with the risk of GORD.
Data sources of gastro-oesophageal reflux disease
Neale Lab1 performed GWAS across more than 7,000 phenotypes from 6 ethnic groups (European, East Asian, African, American, Central South Asian, and Middle Eastern) with the UK Biobank (UKB) approval. Similarly, FinnGen2 is a large public consortium for genetic and health data from Finnish participants. The summary-level GWAS datasets in the Neale Lab and FinnGen consortiums are free and publicly available.
For the outcome cohort, the ‘‘K21 Gastro-oesophageal reflux disease’’ GWAS dataset obtained from the Neale Lab (GORDNeale)3 was used for the discovery phase. GORDNeale GWAS dataset, which contained 29975 cases and 390556 controls and was adjusted for covariates (age, sex, age*sex, age^2, age^2*sex and the first 10 principal components), was analyzed with Scalable and Accurate Implementation of Generalized mixed model (SAIGE) (17), and implemented in the hail batch.4 In the replication phase, the “finngen_R6_K11_REFLUX” GWAS dataset (13141 cases and 189695 controls) obtained from FinnGen (GORDFinn) was analyzed using SAIGE (17)5 and adjusted for covariates including age, sex, 10 principal components, and genotyping batch. Moreover, to effectively complement and identify the causalities between the 66 exposures and the risk of GORD, we employed a sample-size-weighted approach with METAL (18) to perform the IVW fixed-effect meta-analysis (GORDmeta) for the SNPs of summary-level GORDNeale and GORDFinnGen datasets.
Selection of instrumental variables
In this study, all IV data derived from European-descent GWAS datasets followed the MR principles. Namely, IVs should be closely related to exposures; IVs cannot affect outcomes directly but rather through exposures, and no associations were found between IVs and known or unknown confounders. Moreover, genome-wide statistically significant SNPs (P < 5*10–8) without linkage disequilibrium (R2 > 0.001, clumping Kb < 10000) were used as independent IVs. Meanwhile, the effect values of exposures and outcomes were harmonized to the same effect allele to ensure accurate MR analysis (19).
Estimation of heritability and genetic correlation
The linkage disequilibrium score regression (LDSC) is an effective method for estimating heritability (h2snp) and genetic correlations (rg) between different phenotypes based on summary-level GWAS datasets (20). According to the European population-characterized LD score profiles of the 1000GenomesProjects6 (21), we calculated the heritability using LDSC for 66 exposures in 6 modifiable pathways, and identified 2,145 (66 exposures*32.5) genetic correlations between 66 exposures (including self-diagnosis), as well as genetic correlations between 66 exposures and GORDNeale and GORDFinn. P ≤ 0.05 was considered as the threshold of statistical significance for genetic correlations. P ≤ 2.261E-05 (0.05/2145, Bonferroni multiple comparisons) was considered as the threshold of genetic correlation with significantly statistical significance.
Univariable Mendelian randomization analysis
The process of UVMR is shown in Figure 1. In this study, the two-sample UVMR was performed using the “TwoSampleMR” package of R software. The IVW method was principally employed to explore the causality between each exposure and the risk of GORD, with the weighted median method and MR-Egger method for enhancing the findings. Under the premise of ensuring that all IVs are valid, the IVW method uses the reciprocal of the variance of each IV as a weight to calculate the causal estimate of a single IV, and the causal estimate corresponding to each IV can be summed into a weighted estimate as a whole (22, 23). The weighted median estimate is the median of the distribution of all IV estimates sorted by weight, and the weight of each IV causal estimate depends on the accuracy of the estimation. In cases where at least half of SNPs are valid IVs, the weighted median provides a consistent estimate of the final effect (24). The MR-Egger method does not force the regression line to pass through the origin, allowing the included IVs to have directional pleiotropy. When the intercept of MR-Egger analysis is significantly different from 0, it indicates that there is directional gene pleiotropy; when the intercept of the regression is 0, or the intercept is not statistically significant (P > 0.05), the slope of MR-Egger represents the causal estimate of exposure on the outcome (25). Therefore, the MR-Egger method can be utilized to examine and correct the horizontal pleiotropy. MR-PRESSO with the “MRPRESSO package” of R software was applied to detect outliers (26). In this study, when outliers were detected, they would be eliminated, and MR analysis would be reconducted until no outliers remained. Causal associations with P ≤ 7.576E-04 (0.05/66) were deemed as significant, and causal associations with 7.576E-04 < P ≤ 0.05 were defined as suggestive causal associations.
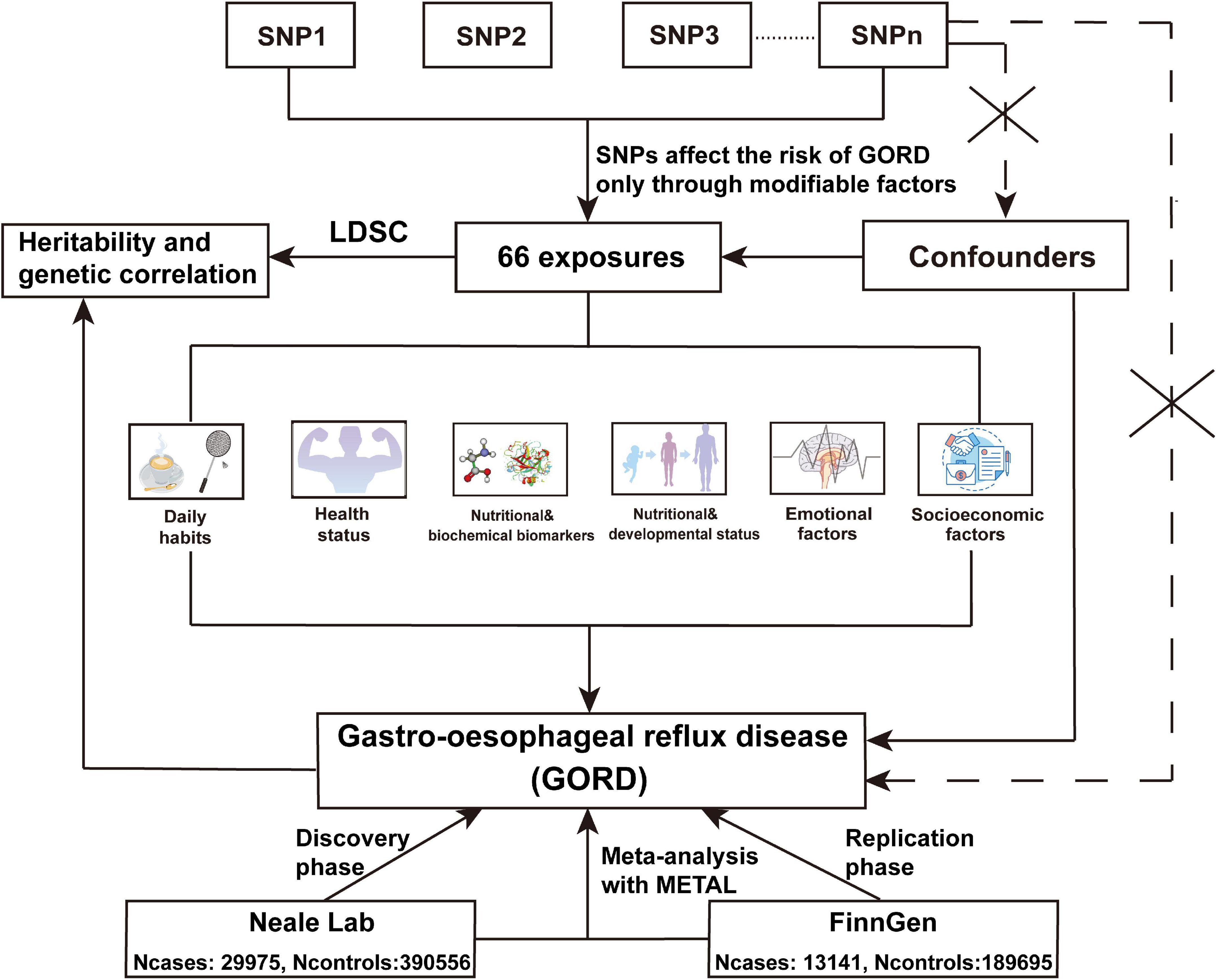
Figure 1. The flow chart of the UVMR analyses. In UVMR analysis, we assessed the causal associations between 66 exposures across 6 modifiable pathways and the risk of GORD from discovery phase, replication phase, and IVW fixed-effect meta-analysis using METAL for SNPs from GORDNeale and GORDFinn. The selection of IVs (SNPs) abided by 3 assumptions of MR analysis. (1) IVs should be closely related to exposure; (2) IVs cannot affect outcome directly, but only through exposure; (3) IVs should not be associated with the known or unknown confounders.
Genetic correlation clustering and multivariable Mendelian randomization analysis
We performed the PAM clustering algorithm to cluster genetic correlation subgroups for exposures that were identified to be significantly causally associated with the risk of GORD (P ≤ 7.576E-04). A vital advantage of the PAM clustering algorithm included the ability to robustly cluster different data types within limited samples, minimizing the influences of data noise and isolated points on the clustering results. Inter-exposure similarity was measured using Euclidean distance, 80% of the exposures were taken at one time, 1,000 replicate samplings were conducted, and the slope of the CDF curve and area under the CDF curve determined the most appropriate number of clusters to be placed. Exposures that were genetically correlated with all other exposures with statistical significance (P ≤ 0.05) in each cluster were defined as the hub exposures. MVMR principle hypothesizes that genetic-level links existed in individual exposures, and that SNPs are strongly associated with at least one of these exposures (27). Thus, as shown in Figure 2, to further clarify whether significant causal exposures were directly causally associated with the risk of GORD rather than being mediated by hub exposures, we chose significant causal exposures that had statistically significant genetic correlations with hub exposures for MVMR, which also included SNPs that were identified as statistically significant from the genome-wide level with at least one specific exposure (P < 5*10–8) to be IVs and GORDmeta GWAS dataset as the source of outcome GWAS dataset. MVMR-IVW method (27) and MVMR-MR Egger method (28) were employed for MVMR analyses. Horizontal pleiotropy was examined by the MR-Egger intercept test.
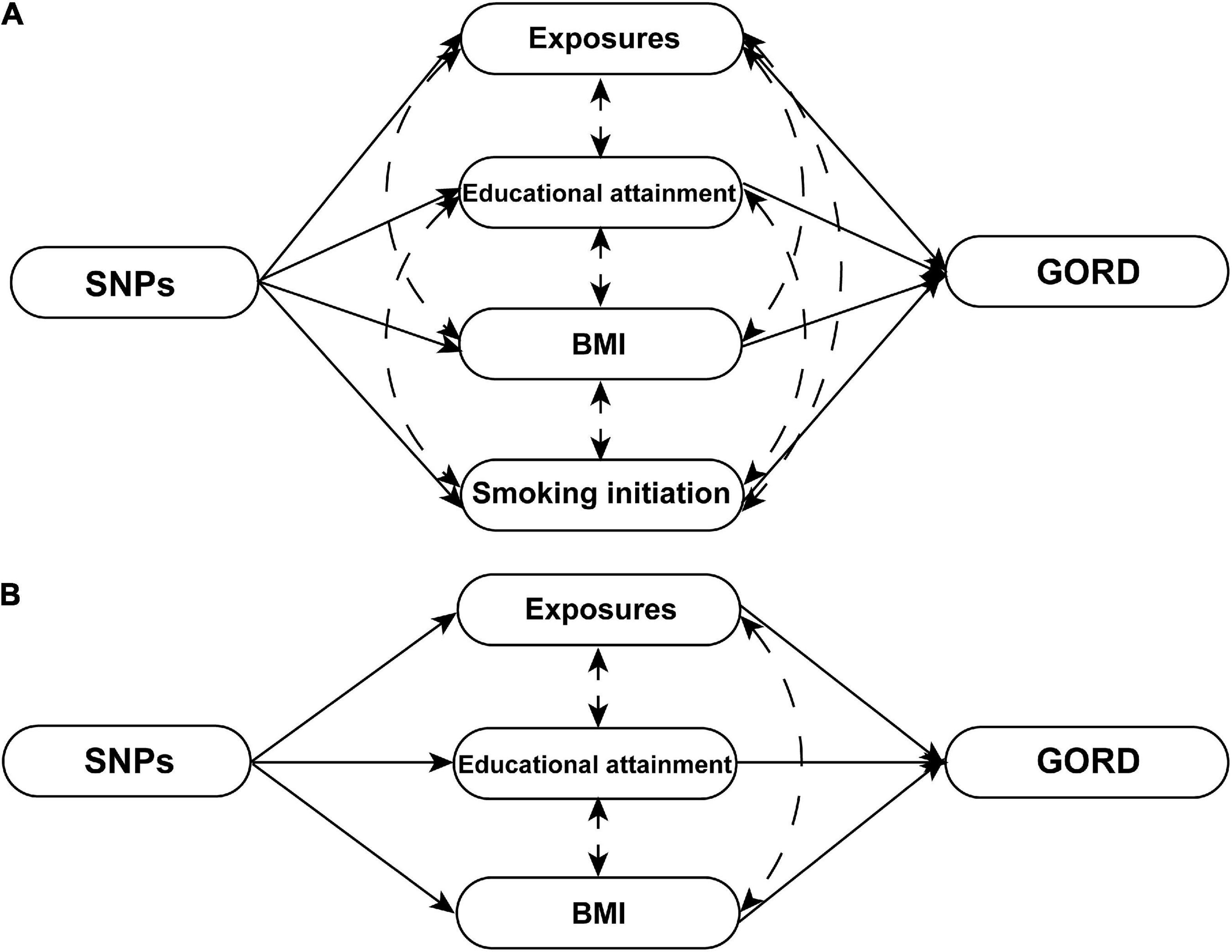
Figure 2. The schematics of MVMR analyses. (A) The schematic of MVMR analyses with adjustment of educational attainment, smoking initiation, and BMI for 11 significant causal exposures [cigarette consumption, insomnia, short sleep, leisure sedentary behavior (TV watching), waist-to-hip ratio, height (standing and sitting), major depressive disorder, anxious feeling, life satisfaction, and positive affect]. (B) The schematic of MVMR analyses with adjustment of educational attainment and BMI for four significant causal exposures [leisure sedentary behavior (computer use), hand grip strength (left and right), and birth weight]. Exposures that respectively strongly genetically correlated with the BMI (body fat percentage, whole body fat mass, visceral adipose tissue, and waist circumference), smoking initiation (lifetime smoking), and educational attainment (income and intelligence) were excluded from MVMR analyses.
Statistical analysis
Weak IV bias was evaluated using the F statistic [F statistic = (Beta/SE)2]. The general assumption is that there are no weak instrumental variable biases when the SNPs F statistics are greater than 10 (29), and in this study, the SNPs F statistics less than or equal to 10 were removed from MR analysis. The Cochran’s Q test P-value and I2 were employed to evaluate and quantify the heterogeneity of SNPs. In this study, the multiplicative random effects model was adopted for MR analysis with Cochran’s Q test P ≤ 0.05, and the fixed-effect model was utilized to conduct MR analysis with Cochran’s Q test P > 0.05 (30). When the number of SNPs involved in MR analysis was less than or equal to 3, the fixed-effect model was applied for MR analysis. The mRnd online tool was used to calculate statistical power of MR analysis7 (31). In this study, all data analysis and visualization were completed using R software version 4.1.2.
Results
Overall heritability and genetic correlation of the 66 exposures
We estimated heritability and genetic correlation using LDSC for 66 exposures, GORDNeale and GORDFinn (Figures 3A–F; Supplementary Table 3). Total observed scale heritability ranged from the lowest waist-to-hip ratio (h2snp = 0.011, SE = 4.000E-03) to highest schizophrenia (h2snp = 0.655, SE = 0.024). GORDNeale (h2snp = 0.018, SE = 1.500E-03) and GORDFinn (h2snp = 0.015, SE = 2.500E-03) possessed largely consistent heritability. The exposure-exposure, exposure-GORDNeale, exposure-GORDFinn, and GORDNeale-GORDFinn genetic correlations were shown in Figure 3G and Table 2. Particularly, GORDNeale and GORDFinn shared a high degree of genetic correlation (rg = 0.929, P = 3.389E-21), and 39 of 48 exposures that showed a statistically significant genetic correlation with GORDNeale also had a similar pattern of genetic correlation with GORDFinn.
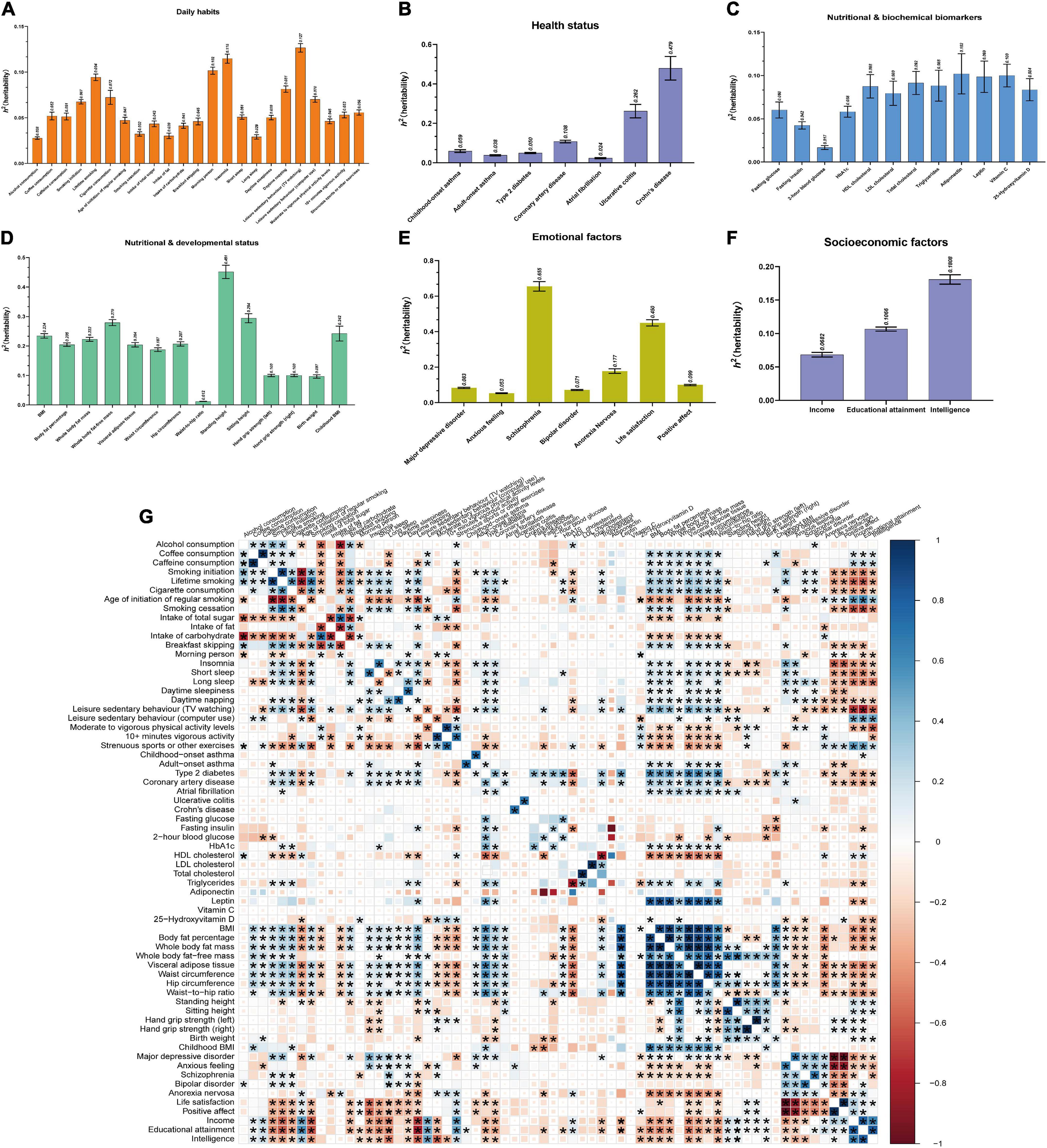
Figure 3. Estimations of heritability and genetic correlations for 66 exposures using LDSC. (A–F) Estimations of heritability for 66 exposures across 6 modifiable pathways (daily habits, health status, nutritional and biochemical biomarkers, nutritional and developmental status, emotional factors, and socioeconomic factors). Mean with SEM was represented with error bar. (G) Genetic correlations between 66 exposures. Totally, there were 2,145 (66 exposures*32.5) genetic correlations among 66 exposures. The sizes and colors of squares respectively represented the significance level and genetic correlation directions. If P ≤ 0.05 (the threshold of genetic correlation with statistical significance), the squares would be full-sized. P ≤ 2.331E-05 (0.05/2145, Bonferroni multiple comparisons) was considered as the threshold of genetic correlation with significantly statistical significance between two exposures, whose squares would be showed with asterisk.
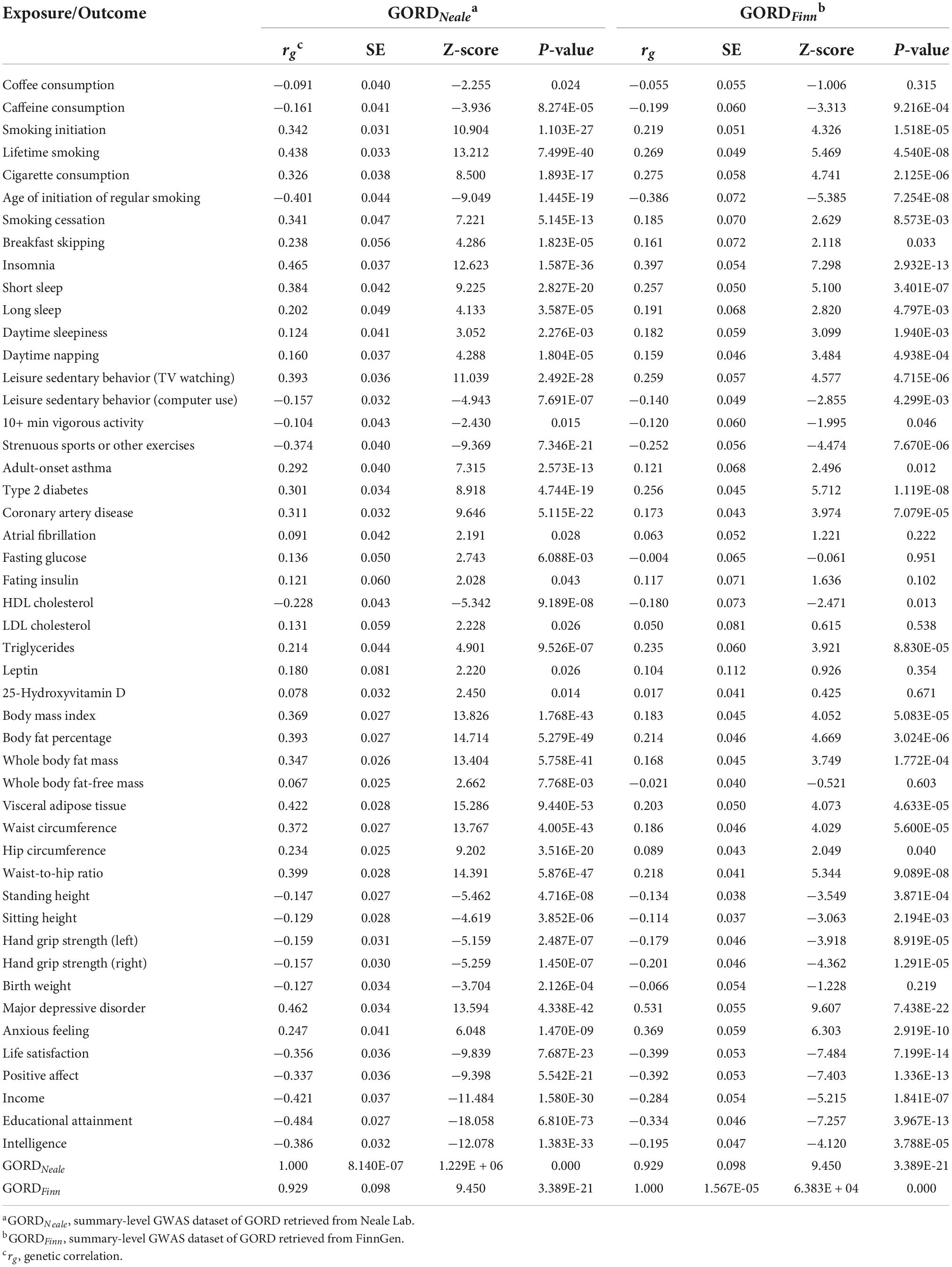
Table 2. Statistically significant genetic correlation between 48 exposures and GORDNeale as well as GORDFinn.
Univariable Mendelian randomization analysis in the discovery phase and replication phase
In the discovery and replication phases, all causal associations were examined by the MR-PRESSO method, and outliers were eliminated. In the discovery phase, based on the IVW method, a total of 34 exposures (Figure 4; Supplementary Table 4) were causally associated with the risk of GORD with statistical significance (P ≤ 0.05). Among them, the following 20 exposures were causally associated with an increased risk of GORD: smoking initiation, lifetime smoking, cigarette consumption, smoking cessation, insomnia, short sleep, daytime sleepiness, daytime napping, leisure sedentary behavior (TV watching), type 2 diabetes, coronary artery disease, BMI, body fat percentage, whole body fat mass, visceral adipose tissue, waist circumference, hip circumference, waist-to-hip ratio, major depressive disorder, and anxious feeling. The other 14 exposures showed causal associations with a decreased risk of GORD: leisure sedentary behavior (computer use), 10+ min vigorous activity, strenuous sports or other exercises, height (standing and sitting), hand grip strength (left and right), birth weight, life satisfaction, positive affect, income, educational attainment, and intelligence. Moreover, heterogeneities were observed in the SNPs corresponding to the following exposures that causally were associated with the risk of GORD (P ≤ 0.05): smoking initiation, lifetime smoking, insomnia, short sleep, daytime sleepiness, daytime napping, leisure sedentary behavior (TV watching), BMI, body fat percentage, whole body fat mass, visceral adipose tissue, waist circumference, hip circumference, waist-to-hip ratio, height (standing and sitting), hand grip strength (left and right), birth weight, anxious feeling, life satisfaction, positive affect, income, educational attainment, and intelligence (Supplementary Figures 1–5). Furthermore, no horizontal pleiotropy was detected in the causal association between the exposures and the risk of GORD.
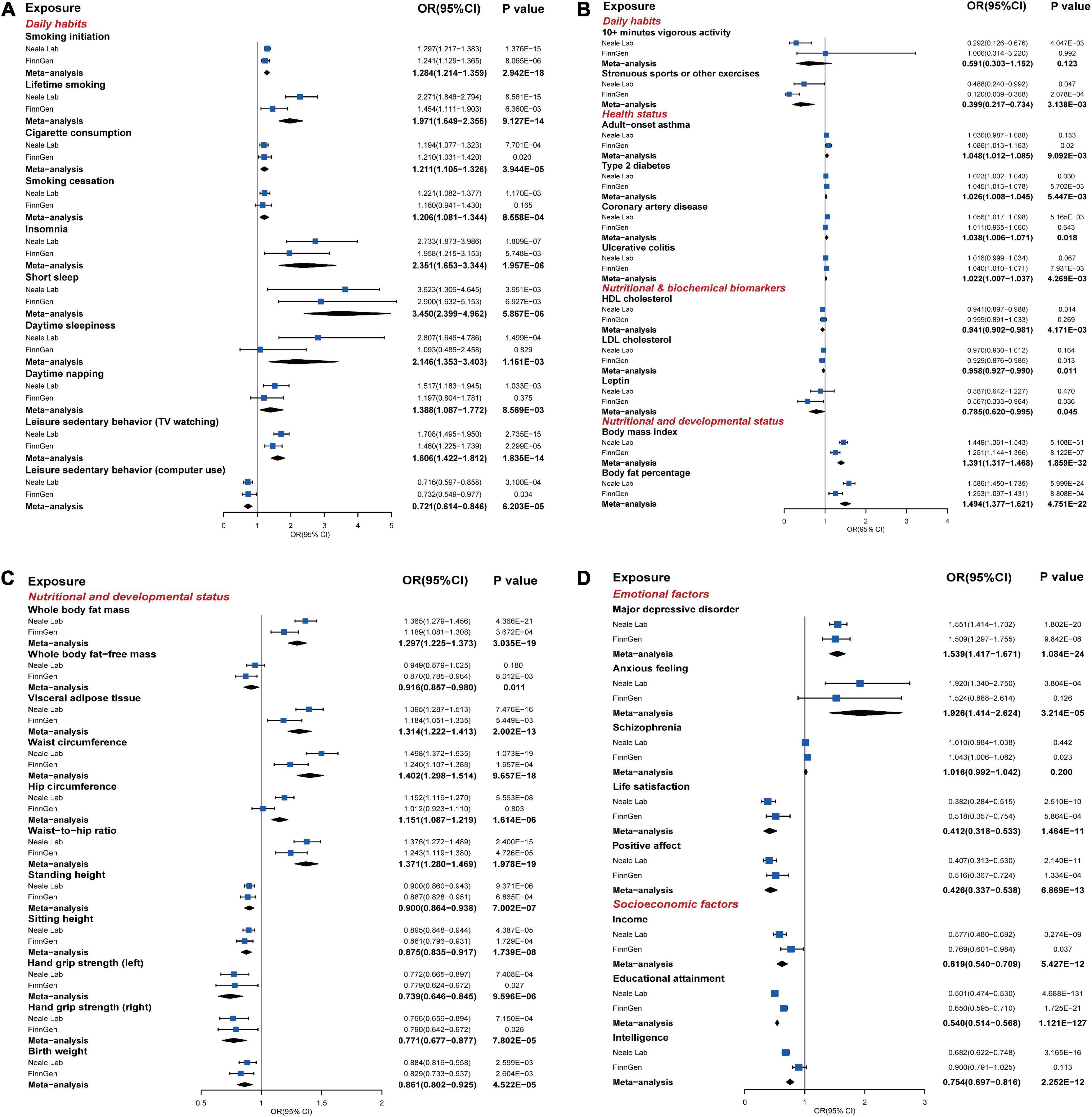
Figure 4. The statistically significant results of MR analysis in the discovery phase or replication phase and meta-analysis (P ≤ 0.05). (A–D) The forest plots graphically illustrated the aggregated results for 40 exposures that have statistically significant causal associations with the risk of GORD based on the IVW method in the discovery phase, replication phase, or meta-analysis, where “Neale Lab,” “FinnGen,” and “Meta-analysis,” respectively, denoted the MR results in the discovery phase, replication phase, and meta-analysis. The causal effects were represented as odds ratios (ORs) with IVW method (blue box). The MR results of the discovery phase, replication phase, and meta-analysis with IVW method, weighted median method, MR-Egger method, and MR-PRESSO method were summarized in Supplementary Tables 4–6 in detail.
In the replication phase (Figure 4; Supplementary Table 5), a total of 29 exposures showed causal associations with the risk of GORD (P ≤ 0.05), among which, the causal associations between the following 25 exposures were consistent with the results in the discovery phase: smoking initiation, lifetime smoking, cigarette consumption, insomnia, short sleep, leisure sedentary behaviors (TV watching and computer use), strenuous sports or other exercises, type 2 diabetes, BMI, body fat percentage, whole body fat mass, visceral adipose tissue, waist circumference, waist-to-hip ratio, height (standing and sitting), hand grip strength (left and right), birth weight, major depressive disorder, life satisfaction, positive affect, income, and educational attainment. Meanwhile, exposures “child-onset asthma,” “ulcerative colitis,” “whole body fat-free mass,” and “schizophrenia,” which had not previously shown causal associations with the risk of GORD in the discovery phase, also showed causal associations with the risk of GORD in the replication phase. SNPs in the educational attainment, BMI, smoking initiation, sitting height, waist circumference, whole body fat mass, standing height, body fat percentage, birth weight, type 2 diabetes, visceral adipose tissue and short sleep suggested heterogeneities (Supplementary Figures 1–5). Although horizontal pleiotropy (P = 0.029, MR-Egger intercept test) was detected in the causal association between the smoking initiation and the risk of GORD, the result from MR-Egger method was still robust (P = 0.022, MR-Egger method).
Meta-analysis for the discovery phase and replication phase
We performed UVMR for the 66 exposures and GORDmeta, which contained 43116 GORD cases and 580251 controls from the GORDNeale and GORDFinn, to complement and consolidate the causal associations between exposures and the risk of GORD. In total, as shown in Figure 4 and Supplementary Tables 6, 26 exposures were finally identified to be significantly causally associated with the risk of GORD (P ≤ 7.463E-04), which included 15 risk exposures: smoking initiation (OR = 1.284, P = 5.867E-06), lifetime smoking (OR = 1.971, P = 9.127E-14), cigarette consumption (OR = 1.211, P = 3.944E-05), insomnia (OR = 2.351, P = 1.957E-06), short sleep (OR = 3.450, P = 5.867E-06), leisure sedentary behavior (TV watching) (OR = 1.606, P = 1.835E-14), BMI (OR = 1.391, P = 1.859E-32), body fat percentage (OR = 1.494, P = 4.751E-22), whole body fat mass (OR = 1.297, P = 3.035E-19), visceral adipose tissue (OR = 1.314, P = 2.002E-13), waist circumference (OR = 1.402, P = 9.657E-18), hip circumference (OR = 1.151, P = 1.614E-06), waist-to-hip ratio (OR = 1.371, P = 1.978E-19), major depressive disorder (OR = 1.539, P = 1.084E-24), and anxious feeling (OR = 1.926, P = 3.214E-05); 11 protective exposures: leisure sedentary behavior (computer use) (OR = 0.721, P = 1.599E-04, FDR = 1.124E-04), height (standing and sitting) (OR = 0.900, P = 7.002E-07 and OR = 0.875, P = 1.739E-08), hand grip strength (left and right) (OR = 0.739, P = 9.596E-06 and OR = 0.771, P = 7.802E-05), birth weight (OR = 0.861, P = 4.522E-05), life satisfaction (OR = 0.412, P = 1.464E-11), positive affect (OR = 0.426, P = 6.869E-13), income (OR = 0.619, P = 5.427E-12), educational attainment (OR = 0.540, P = 1.121E-127), and intelligence (OR = 0.754, P = 2.252E-12). Meanwhile, smoking cessation, daytime sleepiness, daytime napping, adult-onset asthma, type 2 diabetes, coronary artery disease, ulcerative colitis suggestively causally increased risk of GORD, and strenuous sports or other exercises, HDL cholesterol, LDL cholesterol, leptin, and whole body fat-free mass suggestively causally decreased risk of GORD.
Causalities independent of hub exposures
According to the results of the Bonferroni multiple comparisons from UVMR analyses, 26 causal exposures were identified to have significant causal effects on the risk of GORD. K = 3 (cluster A, cluster B, and cluster C) (Figures 5A–D) was used as the standard of the PAM algorithm to cluster 351 genetic correlations between 26 significant causal exposures (Figure 5E). Among them, educational attainment, smoking initiation and BMI in clusters A, B, and C were genetically correlated with all the other exposures individually, and were defined as hub exposures. MVMR analysis suggested that educational attainment (MVMR-IVW: OR = 0.579, P = 1.426E-20; MVMR-Egger: OR = 0.579, P = 1.235E-18), smoking initiation (MVMR-IVW: OR = 1.114, P = 0.036; MVMR-Egger: OR = 1.114, P = 0.037), and BMI (MVMR-IVW: OR = 1.214, P = 8.715E-09; MVMR-Egger: OR = 1.214, P = 1.641E-08) all showed independent causal effects on the risk of GORD. As the results of statistically significant genetic correlation of the other exposures with educational attainment, smoking initiation and BMI, we evaluated the independent causal effects of cigarette consumption, insomnia, short sleep, leisure sedentary behavior (TV watching), waist-to-hip ratio, height (standing and sitting), major depressive disorder, anxious feeling, life satisfaction and positive affect with adjustment of educational attainment (32), smoking initiation (33), and BMI (34), and independent causal effects of leisure sedentary behavior (computer use), hand grip strength (left and right) and birth weight with adjustment of educational attainment (32) and BMI (34). Exposures that respectively strongly genetically correlated with the BMI [body fat percentage (rg = 0.902, P = 0.000), whole body fat mass (rg = 0.912, P = 0.000), visceral adipose tissue (rg = 0.932, P = 0.000), waist circumference (rg = 0.900, P = 0.000), and hip circumference (rg = 0.849, P = 0.000)], smoking initiation [lifetime smoking (rg = 0.921, P = 0.000)], and educational attainment [income (rg = 0.804, P = 0.000) and intelligence (rg = 0.807, P = 0.000)] were excluded from MVMR analyses. After MVMR analyses (Figure 5F; Supplementary Table 7), it was found that insomnia (MVMR-IVW: OR = 2.286, P = 1.176E-07; MVMR-Egger: OR = 1.747, P = 3.283E-04), short sleep (MVMR-IVW: OR = 2.788, P = 2.134E-05; MVMR-Egger: OR = 2.795, P = 9.198E-05), waist-to-hip ratio (MVMR-IVW: OR = 1.195, P = 1.837E-04; MVMR-Egger: OR = 1.196, P = 2.075E-04), height (standing and sitting) (standing: MVMR-IVW: OR = 0.944, P = 0.013; MVMR-Egger: OR = 0.944, P = 0.013; sitting: MVMR-IVW: OR = 0.928, P = 5.544E-03; MVMR-Egger: OR = 0.928, P = 5.735E-03), hand grip strength (left and right) (left: MVMR-IVW: OR = 0.838, P = 0.031; MVMR-Egger: OR = 0.839, P = 0.035; right: MVMR-IVW: OR = 0.820, P = 0.027; MVMR-Egger: OR = 0.820, P = 0.827), birth weight (MVMR-IVW: OR = 0.811, P = 6.940E-03; MVMR-Egger: OR = 0.811, P = 7.215E-03), major depressive disorder (MVMR-IVW: OR = 1.361, P = 7.761E-11; MVMR-Egger: OR = 1.362, P = 1.919E-10), anxious feeling (MVMR-IVW: OR = 1.485, P = 3.753E-04; MVMR-Egger: OR = 1.485, P = 3.993E-04), life satisfaction (MVMR-IVW: OR = 0.462, P = 4.673E-07; MVMR-Egger: OR = 0.461, P = 7.215E-07), and positive affect (MVMR-IVW: OR = 0.519, P = 1.597E-05; MVMR-Egger: OR = 0.518, P = 2.017E-05) retained their causal effects on the risk of GORD, similar to the UVMR analyses. Moreover, cigarette consumption and leisure sedentary behaviors (TV watching and computer use) did not exhibit independent causal associations with the risk of GORD following MVMR analyses.
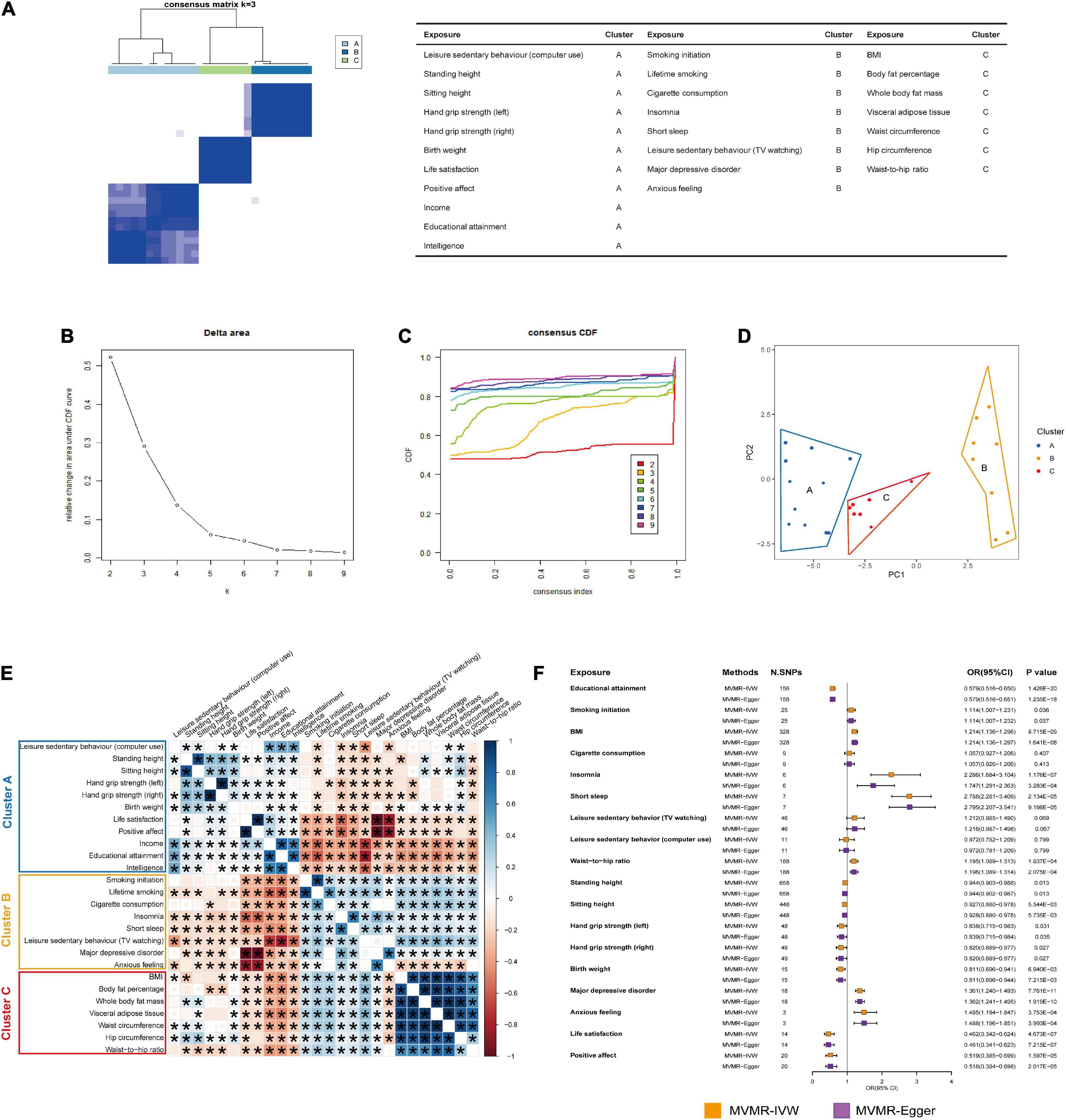
Figure 5. PAM clustering and MVMR analyses. (A) The PAM clustering matrix heatmap when K = 3. (B) The change of slope of CDF curve in PAM clustering for 26 exposures. (C) The change of area under CDF curve in PAM clustering for 26 exposures. (D) Principal component analysis (PCA) for 26 exposures in cluster A, cluster B, and cluster C. (E) Genetic correlations between 26 exposures. The size and color of squares respectively represented the significance level and genetic correlation directions. Genetic correlations with P ≤ 0.05 would be showed with asterisks. (F) The forest plot showed the results of the causal associations between 15 exposures and the risk of GORD after MVMR analyses with adjustment of educational attainment, smoking initiation, or BMI. The causal effects were represented as ORs with MVMR-IVW method (orange box) and MVMR-Egger method (purple box). The MVMR results estimated with MVMR-IVW method and MVMR-Egger method were summarized in Supplementary Table 7 in detail.
Discussion
Previous MR studies examined the causal associations between smoking initiation, asthma, type 2 diabetes, BMI, waist circumference, waist-to-hip ratio, standing height, and the risk of GORD primarily among European-ancestry individuals in the UKB database (16, 35–37), whose MR findings were also replicated in this study with larger-scale GORD GWAS datasets from Neale Lab and FinnGen consortium. In this study, we systematically examined causal associations between 66 exposures across 6 modifiable pathways and the risk of GORD in order to comprehensively elucidate the causal modifiable factors that were associated with the risk of GORD. Totally, 26 significant causal associations and 12 suggestive causal associations were observed. Meanwhile, after MVMR analyses, 15 significant causal exposures among smoking behaviors, sleep disorders, obesity, muscle mass, height (standing and sitting), negative emotions, positive subjective well-beings, and socioeconomic factors retained independent causal associations with the risk of GORD.
Novel significant risk causal associations
Obesity, especially central obesity, contributes the risk of GORD by increasing the frequency of the transient lower oesophageal sphincter relaxation (TLESR) (38), intra-abdominal pressure (39) and the possibility of oesophageal acid exposure (40). We further extended MR analyses to include body fat percentage, whole body fat mass, visceral adipose tissue, waist circumference, hip circumference, and waist-to-hip ratio to systematically demonstrate causal associations between central obesity and the risk of GORD. A higher waist-to-hip ratio is observed to be a better indicator of the occurrence of GORD than BMI (41), and is also associated with the development of GORD (42). In this study, it was further confirmed that the waist-to-hip ratio, whether it was adjusted for BMI or not, presented a strong causal association with the risk of GORD. At present, there are few epidemiological investigations on the association between height and the pathogenesis of GORD. We speculated that taller people have less abdominal pressure and were therefore less likely to develop GORD. The BMI-adjusted and BMI-unadjusted MVMR analyses also verified the independent reverse causalities of the height (standing and the sitting) with the risk of GORD.
Several large cohort studies and meta-analyses have demonstrated evidence linking smoking to GORD (3, 43, 44). Currently, it is believed that smoking causes GORD by prolonging the acid gap time, decreasing the secretion of saliva and neutralizing bases (45) and loosening the lower oesophageal sphincter (LES) (46). In this study, the causalities between smoking behaviors and the risk of GORD were explored systematically from smoking initiation, lifetime smoking, cigarette consumption, and smoking cessation, and the independent positive causal associations between smoking initiation and the risk of GORD were also demonstrated.
Poor sleep quality appeared to be closely related to the risk of GORD. Mody et al. recorded 68.3% of 11685 GORD respondents with sleep difficulties, 49.1% experiencing difficulty falling sleep, and 58.3% struggling to stay asleep (47). This study provided genetic evidence that insomnia and short sleep contributed to the risk of GORD. At present, the associations between insomnia, short sleep and BMI as well as smoking initiation have been demonstrated (48, 49). We performed MVMR analyses with adjustment of educational attainment, BMI and smoking initiation for insomnia and short sleep and confirmed the independence of the causal association between insomnia, short sleep, and the risk of GORD.
As of now, epidemiological studies are lacking regarding the direct impact of sedentary behavior on the risk of GORD. In a UK prospective cohort study, sedentary occupations had a higher risk of Barrett’s oesophagitis comparing with standing occupations (50). In this study, a significant positive causality was found between prolonged TV watching time and the risk of GORD; in contrast, prolonged computer use time had a significant negative causality with the risk of GORD. Furthermore, we also attempted to assess whether sedentary behavior had an independent causal effect on the risk of GORD to rule out the potential causal effects of the remaining factors, such as BMI, smoking behaviors, and educational attainment (51), on the risk of GORD. Following MVMR analyses for TV watching and computer use, we detected that the strong causal effects were attenuated in comparison with the original, which exactly evidenced that sedentary behaviors including TV watching and computer use did not have causal effects on the risk of GORD independently.
Gastro-oesophageal reflux disease is common in individuals with anxious feeling and depression. A recent large cross-sectional study based on 19,099 participants provided evidence that anxious feeling and depression were associated with an increased risk of GORD (52). Corticotropin-releasing hormone (CRH) is a key mediating factor for emotional stress. Broers et al. illustrated that CRH increased oesophageal sensitivity along with increased oesophageal contractility and decreased relaxation of LES, thus causing reflux symptoms (53). In the investigation of GORD pathogenesis, anxious feeling and depression might also be implicated in a similar pattern. GORD may be exacerbated by anxious feeling and depression by reducing the visceral sensitivity index (VSI), enhancing reflux hypersensitivity, and functional heartburn (54). Our study further highlighted the independent causalities of anxious feeling and depression on the risk of GORD.
Novel significant protective causal associations
Hand grip strength are customarily considered as surrogates for skeletal muscle mass, which is typically influenced by malnutrition and aging (55, 56). In this study, we found that hand grip strength (left and right) had significant negative causal associations with the risk of GORD regardless of BMI and educational attainment. The relationship between muscle mass and the risk of GORD was preliminarily investigated in a context of previous cohort study. A cohort study based on 574 patients with sarcopenia indicated that sarcopenia was positively associated with a high risk of GORD (OR = 1.170, 95% CI = 1.016–1.346) (57). Although the underlying mechanisms of the relationship between muscle mass and the risk of GORD are still obscure, this study suggested that there was a deterministic reverse causal association between muscle mass and the risk of GORD at the genetic level, which might serve as a catalyst for further mechanistic studies. Currently, adults with low birth weight are closely bound up with the risk of GORD (58). Current researches support the hypothesis that GORD is a chronic disease that will last throughout the whole life. It usually begins in infancy, but does not progress in childhood, while becomes clinically significant in adulthood (59, 60). In this study, we confirmed an independently inverse causal association between birth weight based on a GWAS dataset from a long-term population study and the risk of GORD.
It has been demonstrated that specific negative emotions have causal effects on the increased risk of GORD; however, there have been rare studies to investigate the correlations between positive emotions and the risk of GORD. An enlightening finding revealed that effective psychological intervention therapy has been shown to significantly improve GORD symptoms, such as globus sensation and non-cardiac chest pain (61), or, to put it another way, there was a theoretical possibility that protective effects of positive emotions on GORD could be achieved. Our study replenished these protective causalities by confirming that the positive subjective feelings (such as positive affect and life satisfaction) were significantly causally associated with a decreased risk of GORD. It could also be interpreted that positive subjective feelings contributed to protection against the risk of GORD.
Socioeconomic factors are also strongly associated with the risk of GORD in many aspects as well. According to a global meta-analysis containing 102 studies (3), the low-income groups had a higher prevalence of GORD compared with the middle-income groups (OR: 1.58, 95% CI: 1.20–2.08) or the high-income groups (OR: 1.68, 95% CI: 1.38–2.05); those with lower educational levels had a higher prevalence of GORD than the individuals at medium educational levels (OR: 1.47, 95% CI: 1.25–1.73) and the individuals with higher educational levels (OR: 1.78, 95% CI: 1.39–2.28). In this study, reverse causal associations between income, educational attainment as well as intelligence and the risk of GORD were also established. Typically, a higher educational level correlates with healthier lifestyles, better economic status, healthier cognition and more consummate medical care (62, 63). Socioeconomic factors, including income, educational attainment and intelligence, all played protective roles in the risk of GORD, while focusing on a single social factor and discrediting it as confounding was also unreasonable for the risk of GORD. Perhaps the causal associations of the socioeconomic factors on the risk of GORD can also be regarded as the consequence of a wide-ranging interaction.
Strengths and limitations
We reviewed and summarized the modifiable factors and previous MR results that were associated with the risk of GORD to expand the scope of exposures included in the MR analysis to the largest extent, ensuring comprehensiveness of MR analysis. This study was also the first to rigorously and systematically examine genetic liabilities of modifiable factors for the risk of GORD, mitigating the impacts of potential confounders and reverse causalities as much as possible. Moreover, MR results in the discovery phase and replication phase were complementary, and meta-analysis for the above two phases enhanced the causal associations between exposures and the risk of GORD. MVMR analyses adjusted for hub exposures (educational attainment, smoking initiation, or BMI) estimated from PAM clustering algorithm also revealed novel insights into the independent causal effects of specific exposures on the risk of GORD.
Some limitations were also taken into consideration in this study. Firstly, despite the fact that we systematically searched and summarized the factors related to the risk of GORD and SNPs recruited from exposures, the limitations of review scope design, as well as the lack of SNPs for some exposures might result in omissions or deviations when assessing causal associations. Secondly, it is generally accepted in MR analysis that there are two mechanisms for pleiotropy: one is that a genetic variant directly affects multiple phenotypes, which is defined as type I pleiotropy (horizontal pleiotropy), and the second is that a genetic variant affects one exposure, while simultaneously also affecting other phenotypes through this expose, which is defined as type II pleiotropy (vertical pleiotropy) (64). As a general rule, type II pleiotropy (vertical pleiotropy) has no biased effects on causality. Therefore, we supplemented the MR-Egger method and MR-PRESSO methods in the MR analysis in order to minimize the bias with the detection of type I pleiotropy (horizontal pleiotropy) as much as possible. Thirdly, given that this study was mainly based on the European population, extrapolating the causal associations in this study to other ethnic populations may result in deviations due to genetic heterogeneities among different ethnic populations.
Conclusion
In summary, we performed MR analysis in this study to identify the factors causally associated with the risk of GORD from 66 exposures across 6 modifiable pathways, which also provided references on the public preventive and therapeutic strategies for GORD from multiple perspectives, such as weight loss, proper high-level physical activities, high-quality sleep, non-smoking, and positive mental state.
Data availability statement
The original contributions presented in this study are included in the article/Supplementary material, further inquiries can be directed to the corresponding author.
Ethics statement
Consents for GWAS datasets were all acquired from public database.
Author contributions
YS and JJ designed this study. YS, XC, and KS drafted the manuscript. YS, XC, DC, YC, KS, ZJ, and JJ conducted data collection and analysis. YW and JJ provided medical ethical reference. All authors contributed to the article and approved the submitted version.
Funding
This work was supported by the National Natural Science Foundation of China, Grant/Award numbers: 81874279 and 82002932, Jilin Province Department of Finance, Grant/Award number: JLSWSRCZX2020-010, Health Commission of Jilin Province, Grant/Award number: 2021JC002, and Scientific and Technological Development Program of Jilin Province, Grant/Award number: 20200201326JC.
Conflict of interest
The authors declare that the research was conducted in the absence of any commercial or financial relationships that could be construed as a potential conflict of interest.
Publisher’s note
All claims expressed in this article are solely those of the authors and do not necessarily represent those of their affiliated organizations, or those of the publisher, the editors and the reviewers. Any product that may be evaluated in this article, or claim that may be made by its manufacturer, is not guaranteed or endorsed by the publisher.
Supplementary material
The Supplementary Material for this article can be found online at: https://www.frontiersin.org/articles/10.3389/fnut.2022.1009122/full#supplementary-material
Footnotes
- ^ http://www.nealelab.is/uk-biobank
- ^ https://r6.finngen.fi/
- ^ https://pan.ukbb.broadinstitute.org/phenotypes
- ^ https://hail.is/docs/batch/index.html
- ^ https://github.com/weizhouUMICH/SAIGE/tree/finngen_r6_jk
- ^ https://alkesgroup.broadinstitute.org/LDSCORE
- ^ https://shiny.cnsgenomics.com/mRnd/
References
1. Ali T, Miner PB. New developments in gastroesophageal reflux disease diagnosis and therapy. Curr Opin Gastroenterol. (2008) 24:502–8. doi: 10.1097/MOG.0b013e3283025c6d
2. El-Serag HB, Sweet S, Winchester CC, Dent J. Update on the epidemiology of gastro-oesophageal reflux disease: a systematic review. Gut. (2014) 63:871–80. doi: 10.1136/gutjnl-2012-304269
3. Eusebi LH, Ratnakumaran R, Yuan Y, Solaymani-Dodaran M, Bazzoli F, Ford AC. Global prevalence of, and risk factors for, gastro-oesophageal reflux symptoms: a meta-analysis. Gut. (2018) 67:430–40. doi: 10.1136/gutjnl-2016-313589
4. Gyawali CP, Kahrilas PJ, Savarino E, Zerbib F, Mion F, Smout AJPM, et al. Modern diagnosis of GERD: the Lyon consensus. Gut. (2018) 67:1351–62. doi: 10.1136/gutjnl-2017-314722
5. Richter JE, Rubenstein JH. Presentation and epidemiology of gastroesophageal reflux disease. Gastroenterology. (2018) 154:267–76. doi: 10.1053/j.gastro.2017.07.045
6. Ness-Jensen E, Hveem K, El-Serag H, Lagergren J. Lifestyle intervention in gastroesophageal reflux disease. Clin Gastroenterol Hepatol. (2016) 14:175–82.e3. doi: 10.1016/j.cgh.2015.04.176
7. Ness-Jensen E, Lagergren J. Tobacco smoking, alcohol consumption and gastro-oesophageal reflux disease. Best Pract Res Clin Gastroenterol. (2017) 31:501–8. doi: 10.1016/j.bpg.2017.09.004
8. Lim KG, Morgenthaler TI, Katzka DA. Sleep and nocturnal gastroesophageal reflux: an update. Chest. (2018) 154:963–71. doi: 10.1016/j.chest.2018.05.030
9. Oh JH. Gastroesophageal reflux disease: recent advances and its association with sleep. Ann N Y Acad Sci. (2016) 1380:195–203. doi: 10.1111/nyas.13143
10. Lawlor DA, Harbord RM, Sterne JAC, Timpson N, Davey Smith G. Mendelian randomization: using genes as instruments for making causal inferences in epidemiology. Stat Med. (2008) 27:1133–63.
11. Burgess S, Timpson NJ, Ebrahim S, Davey Smith G. Mendelian randomization: where are we now and where are we going? Int J Epidemiol. (2015) 44:379–88. doi: 10.1093/ije/dyv108
12. Wang MH, Cordell HJ, Van Steen K. Statistical methods for genome-wide association studies. Semin Cancer Biol. (2019) 55:53–60. doi: 10.1016/j.semcancer.2018.04.008
13. Smith GD, Lawlor DA, Harbord R, Timpson N, Day I, Ebrahim S. Clustered environments and randomized genes: a fundamental distinction between conventional and genetic epidemiology. PLoS Med. (2007) 4:e352. doi: 10.1371/journal.pmed.0040352
14. Sekula P, Del Greco MF, Pattaro C, Köttgen A. Mendelian randomization as an approach to assess causality using observational data. J Am Soc Nephrol. (2016) 27:3253–65.
15. Smith GD, Ebrahim S. Mendelian randomization: prospects, potentials, and limitations. Int J Epidemiol. (2004) 33:30–42.
16. Yuan S, Larsson SC. Adiposity, diabetes, lifestyle factors and risk of gastroesophageal reflux disease: a Mendelian randomization study. Eur J Epidemiol. (2022) 37:747–54. doi: 10.1007/s10654-022-00842-z
17. Zhou W, Nielsen JB, Fritsche LG, Dey R, Gabrielsen ME, Wolford BN, et al. Efficiently controlling for case-control imbalance and sample relatedness in large-scale genetic association studies. Nat Genet. (2018) 50:1335–41. doi: 10.1038/s41588-018-0184-y
18. Willer CJ, Li Y, Abecasis GR. METAL: fast and efficient meta-analysis of genomewide association scans. Bioinformatics. (2010) 26:2190–1. doi: 10.1093/bioinformatics/btq340
19. Hartwig FP, Davies NM, Hemani G, Davey Smith G. Two-sample Mendelian randomization: avoiding the downsides of a powerful, widely applicable but potentially fallible technique. Int J Epidemiol. (2016) 45:1717–26. doi: 10.1093/ije/dyx028
20. Bulik-Sullivan BK, Loh P-R, Finucane HK, Ripke S, Yang J, Patterson N, et al. LD Score regression distinguishes confounding from polygenicity in genome-wide association studies. Nat Genet. (2015) 47:291–5. doi: 10.1038/ng.3211
21. Zheng J, Erzurumluoglu AM, Elsworth BL, Kemp JP, Howe L, Haycock PC, et al. LD Hub: a centralized database and web interface to perform LD score regression that maximizes the potential of summary level GWAS data for SNP heritability and genetic correlation analysis. Bioinformatics. (2017) 33:272–9. doi: 10.1093/bioinformatics/btw613
22. Pierce BL, Burgess S. Efficient design for Mendelian randomization studies: subsample and 2-sample instrumental variable estimators. Am J Epidemiol. (2013) 178:1177–84. doi: 10.1093/aje/kwt084
23. Burgess S, Butterworth A, Thompson SG. Mendelian randomization analysis with multiple genetic variants using summarized data. Genet Epidemiol. (2013) 37:658–65. doi: 10.1002/gepi.21758
24. Bowden J, Davey Smith G, Haycock PC, Burgess S. Consistent estimation in Mendelian randomization with some invalid instruments using a weighted median estimator. Genet Epidemiol. (2016) 40:304–14. doi: 10.1002/gepi.21965
25. Bowden J, Davey Smith G, Burgess S. Mendelian randomization with invalid instruments: effect estimation and bias detection through Egger regression. Int J Epidemiol. (2015) 44:512–25. doi: 10.1093/ije/dyv080
26. Verbanck M, Chen C-Y, Neale B, Do R. Detection of widespread horizontal pleiotropy in causal relationships inferred from Mendelian randomization between complex traits and diseases. Nat Genet. (2018) 50:693–8. doi: 10.1038/s41588-018-0099-7
27. Burgess S, Thompson SG. Multivariable Mendelian randomization: the use of pleiotropic genetic variants to estimate causal effects. Am J Epidemiol. (2015) 181:251–60. doi: 10.1093/aje/kwu283
28. Rees JMB, Wood AM, Burgess S. Extending the MR-Egger method for multivariable Mendelian randomization to correct for both measured and unmeasured pleiotropy. Stat Med. (2017) 36:4705–18. doi: 10.1002/sim.7492
29. Bowden J, Del Greco MF, Minelli C, Davey Smith G, Sheehan NA, Thompson JR. Assessing the suitability of summary data for two-sample Mendelian randomization analyses using MR-Egger regression: the role of the I2 statistic. Int J Epidemiol. (2016) 45:1961–74. doi: 10.1093/ije/dyw220
30. Hemani G, Bowden J, Davey Smith G. Evaluating the potential role of pleiotropy in Mendelian randomization studies. Hum Mol Genet. (2018) 27:R195–208. doi: 10.1093/hmg/ddy163
31. Brion M-JA, Shakhbazov K, Visscher PM. Calculating statistical power in Mendelian randomization studies. Int J Epidemiol. (2013) 42:1497–501. doi: 10.1093/ije/dyt179
32. Lee JJ, Wedow R, Okbay A, Kong E, Maghzian O, Zacher M, et al. Gene discovery and polygenic prediction from a genome-wide association study of educational attainment in 1.1 million individuals. Nat Genet. (2018) 50:1112–21. doi: 10.1038/s41588-018-0147-3
33. Liu M, Jiang Y, Wedow R, Li Y, Brazel DM, Chen F, et al. Association studies of up to 1.2 million individuals yield new insights into the genetic etiology of tobacco and alcohol use. Nat Genet. (2019) 51:237–44. doi: 10.1038/s41588-018-0307-5
34. Elsworth B, Lyon M, Alexander T, Liu Y, Matthews P, Hallett J, et al. The MRC IEU OpenGWAS data infrastructure. bioRxiv [Preprint]. (2020). doi: 10.1101/2020.08.10.244293
35. Lai FY, Nath M, Hamby SE, Thompson JR, Nelson CP, Samani NJ. Adult height and risk of 50 diseases: a combined epidemiological and genetic analysis. BMC Med. (2018) 16:187. doi: 10.1186/s12916-018-1175-7
36. Freuer D, Linseisen J, Meisinger C. Asthma and the risk of gastrointestinal disorders: a Mendelian randomization study. BMC Med. (2022) 20:82. doi: 10.1186/s12916-022-02283-7
37. Green HD, Beaumont RN, Wood AR, Hamilton B, Jones SE, Goodhand JR, et al. Genetic evidence that higher central adiposity causes gastro-oesophageal reflux disease: a Mendelian randomization study. Int J Epidemiol. (2020) 49:1270–81. doi: 10.1093/ije/dyaa082
38. Wu JC, Mui L-M, Cheung CM, Chan Y, Sung JJ. Obesity is associated with increased transient lower esophageal sphincter relaxation. Gastroenterology. (2007) 132:883–9.
39. Varela JE, Hinojosa M, Nguyen N. Correlations between intra-abdominal pressure and obesity-related co-morbidities. Surg Obes Relat Dis. (2009) 5:524–8. doi: 10.1016/j.soard.2009.04.003
40. El-Serag HB, Ergun GA, Pandolfino J, Fitzgerald S, Tran T, Kramer JR. Obesity increases oesophageal acid exposure. Gut. (2007) 56:749–55.
41. Ringhofer C, Lenglinger J, Riegler M, Kristo I, Kainz A, Schoppmann SF. Waist to hip ratio is a better predictor of esophageal acid exposure than body mass index. Neurogastroenterol Motil. (2017) 29:e13033. doi: 10.1111/nmo.13033
42. Edelstein ZR, Bronner MP, Rosen SN, Vaughan TL. Risk factors for Barrett’s esophagus among patients with gastroesophageal reflux disease: a community clinic-based case-control study. Am J Gastroenterol. (2009) 104:834–42. doi: 10.1038/ajg.2009.137
43. Hallan A, Bomme M, Hveem K, Møller-Hansen J, Ness-Jensen E. Risk factors on the development of new-onset gastroesophageal reflux symptoms. A population-based prospective cohort study: the HUNT study. Am J Gastroenterol. (2015) 110:393–400; quiz 401. doi: 10.1038/ajg.2015.18
44. Cheng Y, Kou F, Liu J, Dai Y, Li X, Li J. Systematic assessment of environmental factors for gastroesophageal reflux disease: an umbrella review of systematic reviews and meta-analyses. Dig Liver Dis. (2021) 53:566–73. doi: 10.1016/j.dld.2020.11.022
45. Kahrilas PJ, Gupta RR. The effect of cigarette smoking on salivation and esophageal acid clearance. J Lab Clin Med. (1989) 114:431–8.
46. Kahrilas PJ, Gupta RR. Mechanisms of acid reflux associated with cigarette smoking. Gut. (1990) 31:4–10.
47. Mody R, Bolge SC, Kannan H, Fass R. Effects of gastroesophageal reflux disease on sleep and outcomes. Clin Gastroenterol Hepatol. (2009) 7:953–9. doi: 10.1016/j.cgh.2009.04.005
48. Dashti HS, Ordovás JM. Genetics of sleep and insights into its relationship with obesity. Annu Rev Nutr. (2021) 41:223–52. doi: 10.1146/annurev-nutr-082018-124258
49. Boakye D, Wyse CA, Morales-Celis CA, Biello SM, Bailey MES, Dare S, et al. Tobacco exposure and sleep disturbance in 498 208 UK Biobank participants. J Public Health (Oxf). (2018) 40:517–26. doi: 10.1093/pubmed/fdx102
50. Lam S, Alexandre L, Luben R, Hart AR. The association between physical activity and the risk of symptomatic Barrett’s oesophagus: a UK prospective cohort study. Eur J Gastroenterol Hepatol. (2018) 30:71–5. doi: 10.1097/MEG.0000000000000998
51. van de Vegte YJ, Said MA, Rienstra M, van der Harst P, Verweij N. Genome-wide association studies and Mendelian randomization analyses for leisure sedentary behaviours. Nat Commun. (2020) 11:1770. doi: 10.1038/s41467-020-15553-w
52. Choi JM, Yang JI, Kang SJ, Han YM, Lee J, Lee C, et al. Association between anxiety and depression and gastroesophageal reflux disease: results from a large cross-sectional study. J Neurogastroenterol Motil. (2018) 24:593–602. doi: 10.5056/jnm18069
53. Broers C, Melchior C, Van Oudenhove L, Vanuytsel T, Van Houtte B, Scheerens C, et al. The effect of intravenous corticotropin-releasing hormone administration on esophageal sensitivity and motility in health. Am J Physiol Gastrointest Liver Physiol. (2017) 312:G526–34. doi: 10.1152/ajpgi.00437.2016
54. Losa M, Manz SM, Schindler V, Savarino E, Pohl D. Increased visceral sensitivity, elevated anxiety, and depression levels in patients with functional esophageal disorders and non-erosive reflux disease. Neurogastroenterol Motil. (2021) 33:e14177. doi: 10.1111/nmo.14177
55. Abe T, Loenneke JP, Thiebaud RS. Fat-free adipose tissue mass: impact on peak oxygen uptake (VO) in adolescents with and without obesity. Sports Med. (2019) 49:9–15. doi: 10.1007/s40279-018-1020-3
56. Norman K, Stobäus N, Gonzalez MC, Schulzke J-D, Pirlich M. Hand grip strength: outcome predictor and marker of nutritional status. Clin Nutr. (2011) 30:135–42. doi: 10.1016/j.clnu.2010.09.010
57. Kim YM, Kim J-H, Baik SJ, Jung DH, Park JJ, Youn YH, et al. Association between skeletal muscle attenuation and gastroesophageal reflux disease: a health check-up cohort study. Sci Rep. (2019) 9:20102. doi: 10.1038/s41598-019-56702-6
58. Kaijser M, Akre O, Cnattingius S, Ekbom A. Preterm birth, low birth weight, and risk for esophageal adenocarcinoma. Gastroenterology. (2005) 128:607–9.
59. El-Serag HB, Gilger M, Carter J, Genta RM, Rabeneck L. Childhood GERD is a risk factor for GERD in adolescents and young adults. Am J Gastroenterol. (2004) 99:806–12. doi: 10.1111/j.1572-0241.2004.30098.x
60. Waring JP, Feiler MJ, Hunter JG, Smith CD, Gold BD. Childhood gastroesophageal reflux symptoms in adult patients. J Pediatr Gastroenterol Nutr. (2002) 35:334–8.
61. Riehl ME, Kinsinger S, Kahrilas PJ, Pandolfino JE, Keefer L. Role of a health psychologist in the management of functional esophageal complaints. Dis Esophagus. (2015) 28:428–36. doi: 10.1111/dote.12219
62. Lawrence EM. Why do college graduates behave more healthfully than those who are less educated? J Health Soc Behav. (2017) 58:291–306. doi: 10.1177/0022146517715671
63. Stringhini S, Sabia S, Shipley M, Brunner E, Nabi H, Kivimaki M, et al. Association of socioeconomic position with health behaviors and mortality. JAMA. (2010) 303:1159–66. doi: 10.1001/jama.2010.297
64. Davey Smith G, Hemani G. Mendelian randomization: genetic anchors for causal inference in epidemiological studies. Hum Mol Genet. (2014) 23:R89–98. doi: 10.1093/hmg/ddu328
65. Zhong VW, Kuang A, Danning RD, Kraft P, van Dam RM, Chasman DI, et al. A genome-wide association study of bitter and sweet beverage consumption. Hum Mol Genet. (2019) 28:2449–57. doi: 10.1093/hmg/ddz061
66. Said MA, van de Vegte YJ, Verweij N, van der Harst P. Associations of observational and genetically determined caffeine intake with coronary artery disease and diabetes mellitus. J Am Heart Assoc. (2020) 9:e016808. doi: 10.1161/JAHA.120.016808
67. Wootton RE, Richmond RC, Stuijfzand BG, Lawn RB, Sallis HM, Taylor GMJ, et al. Evidence for causal effects of lifetime smoking on risk for depression and schizophrenia: a Mendelian randomisation study. Psychol Med. (2020) 50:2435–43. doi: 10.1017/S0033291719002678
68. Meddens SFW, de Vlaming R, Bowers P, Burik CAP, Linnér RK, Lee C, et al. Genomic analysis of diet composition finds novel loci and associations with health and lifestyle. Mol Psychiatry. (2021) 26:2056–69. doi: 10.1038/s41380-020-0697-5
69. Dashti HS, Merino J, Lane JM, Song Y, Smith CE, Tanaka T, et al. Genome-wide association study of breakfast skipping links clock regulation with food timing. Am J Clin Nutr. (2019) 110:473–84. doi: 10.1093/ajcn/nqz076
70. Jones SE, Lane JM, Wood AR, van Hees VT, Tyrrell J, Beaumont RN, et al. Genome-wide association analyses of chronotype in 697,828 individuals provides insights into circadian rhythms. Nat Commun. (2019) 10:343. doi: 10.1038/s41467-018-08259-7
71. Lane JM, Jones SE, Dashti HS, Wood AR, Aragam KG, van Hees VT, et al. Biological and clinical insights from genetics of insomnia symptoms. Nat Genet. (2019) 51:387–93. doi: 10.1038/s41588-019-0361-7
72. Dashti HS, Jones SE, Wood AR, Lane JM, van Hees VT, Wang H, et al. Genome-wide association study identifies genetic loci for self-reported habitual sleep duration supported by accelerometer-derived estimates. Nat Commun. (2019) 10:1100. doi: 10.1038/s41467-019-08917-4
73. Wang H, Lane JM, Jones SE, Dashti HS, Ollila HM, Wood AR, et al. Genome-wide association analysis of self-reported daytime sleepiness identifies 42 loci that suggest biological subtypes. Nat Commun. (2019) 10:3503. doi: 10.1038/s41467-019-11456-7
74. Dashti HS, Daghlas I, Lane JM, Huang Y, Udler MS, Wang H, et al. Genetic determinants of daytime napping and effects on cardiometabolic health. Nat Commun. (2021) 12:900. doi: 10.1038/s41467-020-20585-3
75. Klimentidis YC, Raichlen DA, Bea J, Garcia DO, Wineinger NE, Mandarino LJ, et al. Genome-wide association study of habitual physical activity in over 377,000 UK Biobank participants identifies multiple variants including CADM2 and APOE. Int J Obes (Lond). (2018) 42:1161–76. doi: 10.1038/s41366-018-0120-3
76. Ferreira MAR, Mathur R, Vonk JM, Szwajda A, Brumpton B, Granell R, et al. Genetic Architectures of childhood- and adult-onset asthma are partly distinct. Am J Hum Genet. (2019) 104:665–84. doi: 10.1016/j.ajhg.2019.02.022
77. Mahajan A, Taliun D, Thurner M, Robertson NR, Torres JM, Rayner NW, et al. Fine-mapping type 2 diabetes loci to single-variant resolution using high-density imputation and islet-specific epigenome maps. Nat Genet. (2018) 50:1505–13. doi: 10.1038/s41588-018-0241-6
78. van der Harst P, Verweij N. Identification of 64 novel genetic loci provides an expanded view on the genetic architecture of coronary artery disease. Circ Res. (2018) 122:433–43. doi: 10.1161/CIRCRESAHA.117.312086
79. Nielsen JB, Thorolfsdottir RB, Fritsche LG, Zhou W, Skov MW, Graham SE, et al. Biobank-driven genomic discovery yields new insight into atrial fibrillation biology. Nat Genet. (2018) 50:1234–9. doi: 10.1038/s41588-018-0171-3
80. Liu JZ, van Sommeren S, Huang H, Ng SC, Alberts R, Takahashi A, et al. Association analyses identify 38 susceptibility loci for inflammatory bowel disease and highlight shared genetic risk across populations. Nat Genet. (2015) 47:979–86. doi: 10.1038/ng.3359
81. Chen J, Spracklen CN, Marenne G, Varshney A, Corbin LJ, Luan J, et al. The trans-ancestral genomic architecture of glycemic traits. Nat Genet. (2021) 53:840–60. doi: 10.1038/s41588-021-00852-9
82. Willer CJ, Schmidt EM, Sengupta S, Peloso GM, Gustafsson S, Kanoni S, et al. Discovery and refinement of loci associated with lipid levels. Nat Genet. (2013) 45:1274–83. doi: 10.1038/ng.2797
83. Dastani Z, Hivert M-F, Timpson N, Perry JRB, Yuan X, Scott RA, et al. Novel loci for adiponectin levels and their influence on type 2 diabetes and metabolic traits: a multi-ethnic meta-analysis of 45,891 individuals. PLoS Genet. (2012) 8:e1002607. doi: 10.1371/journal.pgen.1002607
84. Kilpeläinen TO, Carli JFM, Skowronski AA, Sun Q, Kriebel J, Feitosa MF, et al. Genome-wide meta-analysis uncovers novel loci influencing circulating leptin levels. Nat Commun. (2016) 7:10494. doi: 10.1038/ncomms10494
85. Zheng J-S, Luan J, Sofianopoulou E, Imamura F, Stewart ID, Day FR, et al. Plasma vitamin C and type 2 diabetes: genome-wide association study and Mendelian randomization analysis in European populations. Diabetes Care. (2021) 44:98–106. doi: 10.2337/dc20-1328
86. Revez JA, Lin T, Qiao Z, Xue A, Holtz Y, Zhu Z, et al. Genome-wide association study identifies 143 loci associated with 25 hydroxyvitamin D concentration. Nat Commun. (2020) 11:1647. doi: 10.1038/s41467-020-15421-7
87. Karlsson T, Rask-Andersen M, Pan G, Höglund J, Wadelius C, Ek WE, et al. Contribution of genetics to visceral adiposity and its relation to cardiovascular and metabolic disease. Nat Med. (2019) 25:1390–5. doi: 10.1038/s41591-019-0563-7
88. Pulit SL, Stoneman C, Morris AP, Wood AR, Glastonbury CA, Tyrrell J, et al. Meta-analysis of genome-wide association studies for body fat distribution in 694 649 individuals of European ancestry. Hum Mol Genet. (2019) 28:166–74. doi: 10.1093/hmg/ddy327
89. Warrington NM, Beaumont RN, Horikoshi M, Day FR, Helgeland Ø, Laurin C, et al. Maternal and fetal genetic effects on birth weight and their relevance to cardio-metabolic risk factors. Nat Genet. (2019) 51:804–14. doi: 10.1038/s41588-019-0403-1
90. Felix JF, Bradfield JP, Monnereau C, van der Valk RJP, Stergiakouli E, Chesi A, et al. Genome-wide association analysis identifies three new susceptibility loci for childhood body mass index. Hum Mol Genet. (2016) 25:389–403. doi: 10.1093/hmg/ddv472
91. Howard DM, Adams MJ, Clarke T-K, Hafferty JD, Gibson J, Shirali M, et al. Genome-wide meta-analysis of depression identifies 102 independent variants and highlights the importance of the prefrontal brain regions. Nat Neurosci. (2019) 22:343–52. doi: 10.1038/s41593-018-0326-7
92. Nagel M, Watanabe K, Stringer S, Posthuma D, van der Sluis S. Item-level analyses reveal genetic heterogeneity in neuroticism. Nat Commun. (2018) 9:905. doi: 10.1038/s41467-018-03242-8
93. Trubetskoy V, Pardiñas AF, Qi T, Panagiotaropoulou G, Awasthi S, Bigdeli TB, et al. Mapping genomic loci implicates genes and synaptic biology in schizophrenia. Nature. (2022) 604:502–8. doi: 10.1038/s41586-022-04434-5
94. Mullins N, Forstner AJ, O’Connell KS, Coombes B, Coleman JRI, Qiao Z, et al. Genome-wide association study of more than 40,000 bipolar disorder cases provides new insights into the underlying biology. Nat Genet. (2021) 53:817–29. doi: 10.1038/s41588-021-00857-4
95. Watson HJ, Yilmaz Z, Thornton LM, Hübel C, Coleman JRI, Gaspar HA, et al. Genome-wide association study identifies eight risk loci and implicates metabo-psychiatric origins for anorexia nervosa. Nat Genet. (2019) 51:1207–14. doi: 10.1038/s41588-019-0439-2
96. Baselmans BML, Jansen R, Ip HF, van Dongen J, Abdellaoui A, van de Weijer MP, et al. Multivariate genome-wide analyses of the well-being spectrum. Nat Genet. (2019) 51:445–51. doi: 10.1038/s41588-018-0320-8
Keywords: Mendelian randomization, gastro-oesophageal reflux disease, modifiable factors, meta-analysis, discovery phase, replication phase
Citation: Sun Y, Cao X, Cao D, Cui Y, Su K, Jia Z, Wu Y and Jiang J (2022) Genetic estimation of correlations and causalities between multifaceted modifiable factors and gastro-oesophageal reflux disease. Front. Nutr. 9:1009122. doi: 10.3389/fnut.2022.1009122
Received: 04 August 2022; Accepted: 17 October 2022;
Published: 01 November 2022.
Edited by:
Roberta Zupo, National Institute of Gastroenterology S. de Bellis Research Hospital (IRCCS), ItalyReviewed by:
Habtamu Abera Goshu, Bio and Emerging Technology Institute (BETin), EthiopiaMassimiliano Cocca, INSERM U1052 Centre de Recherche en Cancérologie de Lyon, France
Copyright © 2022 Sun, Cao, Cao, Cui, Su, Jia, Wu and Jiang. This is an open-access article distributed under the terms of the Creative Commons Attribution License (CC BY). The use, distribution or reproduction in other forums is permitted, provided the original author(s) and the copyright owner(s) are credited and that the original publication in this journal is cited, in accordance with accepted academic practice. No use, distribution or reproduction is permitted which does not comply with these terms.
*Correspondence: Jing Jiang, jiangjing19702000@jlu.edu.cn