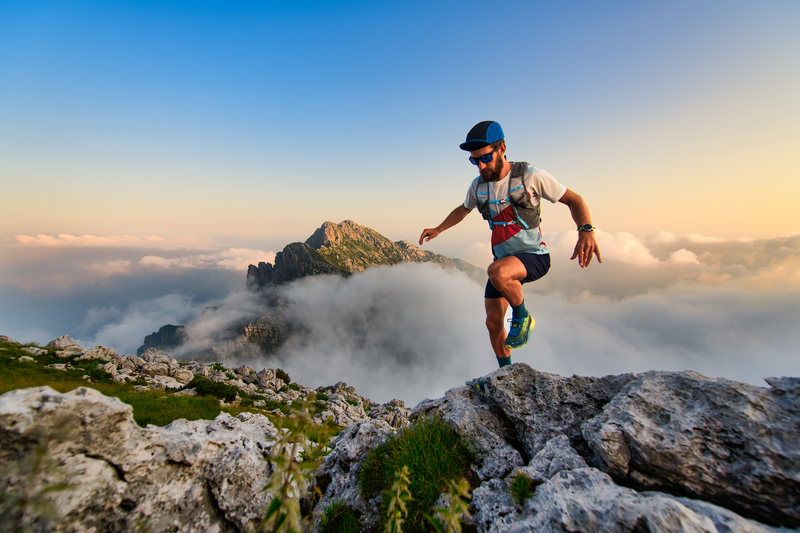
94% of researchers rate our articles as excellent or good
Learn more about the work of our research integrity team to safeguard the quality of each article we publish.
Find out more
ORIGINAL RESEARCH article
Front. Nutr. , 14 October 2022
Sec. Nutritional Epidemiology
Volume 9 - 2022 | https://doi.org/10.3389/fnut.2022.1006018
This article is part of the Research Topic Ultra-Processed Foods and Human and Planetary Health View all 12 articles
Objective: To investigate the association between ultra-processed food consumption at 23–25 years of age and measurements of body composition–fat mass, fat mass distribution and lean mass at 37–39 years of age in Brazilian adults.
Methods: 1978/1979 birth cohort study conducted with healthy adults from Ribeirão Preto, São Paulo, Brazil. A total of 1,021 individuals participated in the fat mass analysis (measured by air displacement plethysmography) and 815 in the lean mass analysis and fat mass distribution (assessed by dual energy X-ray absorptiometry). Food consumption was evaluated by a food frequency questionnaire. Food items were grouped according to the level of processing as per the NOVA classification. Ultra-processed food consumption was expressed as a percentage of total daily intake (g/day). Linear regression models were used to estimate the effect of ultra-processed food consumption (g/day) on body mass index, body fat percentage, fat mass index, android fat, gynoid fat, android-gynoid fat ratio, lean mass percentage, lean mass index and appendicular lean mass index. Marginal plots were produced to visualize interactions.
Results: The mean daily ultra-processed food consumption in grams was 35.8% (813.3 g). There was an association between ultra-processed food consumption and increase in body mass index, body fat percentage, fat mass index, android fat and gynoid fat and decrease in lean mass percentage, only in women.
Conclusion: A high ultra-processed food consumption is associated with a long-term increase in fat mass and a decrease in lean mass in adult women.
The body composition assessment is important to describe and monitor nutritional status with accuracy, in addition to several physiological processes and pathological conditions, making it possible to identify individuals at risk and plan appropriate therapeutic interventions (1, 2). Excess fat mass (FM) is related to higher morbidity and mortality, and the metabolic risk related to fat accumulation is strongly dependent on its distribution (3). The monitoring of lean mass (LM) allows the identification of cachexia, sarcopenia and sarcopenic obesity, situations that are related to increased functional decline and high risk of disease and mortality (2).
Several factors affect body composition, and diet and level of physical activity are some of the most important aspects to be considered (4, 5). Low-carbohydrate diets, for example, may be effective in decreasing FM in obese individuals (6), whereas high-protein diets may have a beneficial effect on weight loss, increasing FM loss and preserving fat-free mass (FFM) (7). However, the diet effect on body composition varies according to age, nutritional quality of this diet, nutritional status, sex of individuals, level of physical activity, hormonal load, genetics, and lifestyle, among other factors (8–10).
The nutritional quality of diets has been widely evaluated using the NOVA food classification system, which groups food according to the nature, purpose and extent of industrial processing rather than in terms of nutrients and food types (11–13). Food items are grouped into 4 groups: Group 1 - Raw or minimally processed foods; Group 2 - Processed culinary ingredients; Group 3 - Processed foods, and Group 4 - Ultra-processed foods (UPF) (11–13), the last consisting of industrial formulations made from numerous food-derived ingredients, with little or no whole food, often added with additives that modify their sensory attributes. UPF has, on average, higher energy density, more free sugar, more total and saturated fats and lower concentrations of fiber, proteins, vitamins, and minerals (11–13). Thus, diets with high UPF participation have their nutritional quality impaired (14–18).
UPF is associated with increased overweight, abdominal obesity, hypertension, coronary and cerebrovascular diseases, dyslipidemia, metabolic syndrome, gastrointestinal disorders, diabetes mellitus, depression, asthma, frailty in the older people, total and breast cancer, and increased overall mortality (14–18). In Brazil, according to the 2017-2018 Household Budget Survey (POF 2017–2018), the mean UPF contribution to the total dietary energy intake (TDEI) of the diet was 19.7% (19).
Although an association between UPF consumption and excess weight was demonstrated (20–24), few studies estimated its long-term effect on body composition. Costa et al. (25) found that a 100 g increase in the UPF contribution to the daily intake of children at age six was associated with a gain of 0.14 kg/m2 in the fat mass index (FMI) at age 11. In adolescents, a 1% increase in the UPF contribution percentage to total dietary energy intake was associated with a 0.04 kg decrease in muscle mass (MM) and a 0.01 kg/m2 decrease in lean mass index (LMI) (26). A cohort study that evaluated associations between UPF consumption and adiposity trajectories from childhood to early adulthood (from seven to 24 years of age) showed an annual increase of 0.03 kg/m2 in FMI in the fifth quintile of UPF consumption when compared to the first (27).
To date, to our knowledge, only one longitudinal study evaluated the association between high UPF consumption and changes in body composition (assessed by great validity methods) in adults and older people. Individuals aged 55–75 years with metabolic syndrome, overweight and obesity were followed for 12 months and it was found that a 10% daily increase in UPF consumption was associated with an accumulation of 0.09 g in visceral fat, 0.05 in android-gynoid fat ratio (AGR), and 0.09% in body fat percentage (BFP) (28). No studies were found that evaluated the UPF effect on the LM of adults.
In this sense, the aim of this study was to investigate, prospectively, the association between UPF consumption at 23–25 years of age and the composition and distribution of body fat and lean mass at 37–39 years in adults followed by the 1978/1979 Ribeirão Preto birth cohort study (São Paulo, Brazil).
This is a prospective cohort study with data from a matrix research entitled “Estudo epidemiológico-social da saúde perinatal em Ribeirão Preto, SP” (29). Data from three of the five phases of the cohort study were used: baseline (birth) (1978/1979); the fourth phase, at 23–25 years of age (2002/2004), and the fifth phase, when the participants were between 37 and 39 years old (2016/2017). At birth (baseline), 6,827 children born in hospitals to mothers residing in Ribeirão Preto were evaluated. In the fourth phase, 2,063 individuals were evaluated, characterizing 30.2% of the original cohort. In the fifth phase, data were collected from 1,775 participants. Further details of each cohort stage, its objectives, methods, and eligible individuals were previously published (29, 30).
For this study, the assessment encompassed only those born in a single birth, who participated in the fourth and fifth phases of the cohort, whose food consumption data were collected at 23–25 years and body composition data were evaluated at 37–39 years, totaling 1,050 individuals for the analysis of fat mass (Body Mass Index–BMI, BFP, FMI) and 839 for the analysis of lean mass (percentage of muscle mass–LMP, LMI and appendicular lean mass index -ALMI) and distribution of body fat (android fat–AF, gynoid fat–GF and AGR). Individuals at the extremes of caloric intake (above or below three standard deviations of the mean total caloric intake, n = 08) and at the extremes of body composition (below the 1st percentile and above the 99th percentile) (21 for adiposity and 17 for lean mass and distribution of body fat) were excluded, totaling 1,021 individuals for analysis of adiposity and 815 for analysis of lean mass and distribution of body fat. In Supplementary Figure S1, a detailed flowchart of the cohort phases is shown.
Food consumption was assessed using a semi-quantitative Food Frequency Questionnaire (FFQ) adapted for use in programs for the prevention of Chronic Noncommunicable Diseases (NCDs) aimed at adults, including the age group assessed in this study (31). The questionnaire was applied by nutritionists, with the aid of a photo album to help estimate the portions consumed. The FFQ contained 75 food items, referring to food consumption in the last 12 months, with options for the number of times (0–9) and the frequency of consumption (daily, weekly, or monthly), and the reference average portion size, for the individual to estimate whether the portion usually consumed was small (smaller than that presented), medium (equal to that presented), or large (greater than that presented) (32). The food portions were classified according to the percentage distribution of weights equivalent to the household measures referred to in the 24-h dietary recall (24 hDR), previously applied as part of the FFQ elaboration. The average portion presented in each food item represented the 50th percentile and the small and large portions, respectively, the 25th and 75th percentiles. The methodology of the validation has been described in detail by Molina et al. (32).
To obtain the consumption of each food item in grams (g) or milliliters (ml), the referred frequencies were converted into daily frequency and multiplied by the serving size. The nutrient and energy intake of each food item was calculated using food composition tables (33–35). In the case of alcoholic beverages, kilocalories from alcohol were also considered. The sum of calories from all food items reported in the FFQ made up the total calories consumed. The consumption of macronutrients, fiber and alcohol was adjusted to 1,000 kcal/gram.
Food items were grouped according to NOVA classification into raw or minimally processed, processed, and ultra-processed foods. Culinary ingredients were grouped together with the group of raw/minimally processed foods, in a group called “food preparations” (36). When food items from different groups were grouped in the FFQ, it was decided to divide the share of these food items into more than one group, by means of an estimate, using as a parameter the consumption observed in the state of São Paulo according to the Brazilian Household Budget Survey (2002–2003) (37), the closest to the data collection period of our presentation. Finally, the percentage of participation of calories and grams from UPF consumed by participants in the daily total of grams and calories, respectively, was calculated.
Body weight (kg) and height (cm) were evaluated in the fifth phase. Trained researchers performed these measurements using a high-precision scale coupled to an Air Displacement Plethysmography (ADP) device (COSMED BodPod® Gold Standard, Concord, USA) with 100 gram graduation. Height was measured using portable AlturaExata® stadiometers with 0.1 cm accuracy (Belo Horizonte, Brazil). Participants were assessed in an upright position, with the head oriented according to the Frankfort horizontal plane.
BMI was calculated by dividing weight in kilograms by height in square meters (kg/m2). Body adiposity was measured by LMI and BFP. This was evaluated by the ADP and converted into kg of fat mass, considering the individual's weight. The FMI was obtained by dividing the fat mass (kg) by the height in square meters (38).
To assess LM and body fat distribution, dual-energy R-ray absorptiometry (DXA) was used. The GE Healthcare® Lunar Prodigy Densitometer (Chicago, United States) was used in a full-body scan with the participant immobile in the supine position on the table, with legs together and arms along the body. The body weight estimated by DXA was different from the weight obtained with the digital scale. Thus, it was necessary to adjust the MM by calculating the LMP estimated by DXA. The adjusted MM (kg) was then obtained by multiplying the LMP by the weight recorded on the digital scale (26). Appendicular lean mass (ALM) (kg) was calculated as the sum of the MM of the arms and legs. The MMI (kg/m2) was calculated as the ratio between the adjusted LM (kg) and the height in square meters, while the ALMI (kg/m2) was obtained as the ratio between the ALM (kg) and height in square meters (39).
Body fat distribution was assessed by measuring AF (kg) and GF (kg). The AF region comprised the space between the ribs and the pelvis, and the GF comprised the region relative to the hip and thighs. The lateral limits established were the lines of the arms when in a normal position for the full-body scan. Central fat distribution was measured through the ratio between AF and GF (AGR) (28).
At 23–25 years of age, data were obtained by trained personnel using structured questionnaires, and the following variables were evaluated: sex (male/female); age categorized in years (23 years/24 years/25 years); race/ethnic group (white/black/brown/yellow or indigenous); family income in minimum wages (< 5 minimum wages/5–9.9 minimum wages/or ≥10 minimum wages); marital status (with a partner/without a partner); current smoking (yes/no); television (TV) and reading time (< 3 h/ ≥3 h per day) (40), and anabolic steroid use (yes/no). The level of physical activity was assessed according to the International Physical Activity Questionnaire–IPAQ (high, moderate, and low) (41).
In order to avoid possible bias due to loss to follow-up (74.0%) that occurred during the cohort period, weighting was performed by the inverse of the selection probability (participation in the follow-up). For that, the following baseline variables were used: type of birth (cesarean section/vaginal); sex (male/female); birth weight (< 2500 g / ≥2,500 g); parity (1 birth/ 2–4 births/ ≥5 births); prematurity (preterm birth < 37 weeks/ term birth ≥37 weeks); maternal age (< 20 years/20–34 years/> 34 years); maternal schooling (≤ 4 years of schooling/ 5–8 years of schooling/ 9–11 years of schooling/ ≥12 years of schooling); maternal smoking during pregnancy (yes/no); maternal marital status (with a partner/without a partner), number of prenatal consultations (0 consultations/1–5 consultations/ ≥6 consultations); maternal occupation (non-manual/skilled manual/unskilled manual); length at birth (< 50 cm/ ≥50 cm), and family income in quartiles.
This study had as an exposure variable the proportion of consumption in grams of UPF at 23–25 years of age, obtained by the percentage of total daily intake (daily intake of UPF (g)/total daily intake of foods and beverages(g)*100). Outcomes were body adiposity (measured by BMI, BFP and LMI), distribution of body fat (GA, GG and AGR) and lean mass (MMP, LMI and ALMI), assessed at 37–39 years. All variables were treated continuously.
In order to establish the minimum set of adjustment variables, reduce confounder bias, collision bias and avoid the inclusion of unnecessary variables in multivariate analysis, a theoretical model was developed based on the construction of a Directed Acyclic Graph (DAG), through the online DAGitty (version 3.0) software (42), according to Supplementary Figure S2. The variables to compose the minimum adjustment set for confounders identified in the DAG by the backdoor criterion (42) were: age, skin color, family income, sex, marital status, level of physical activity, smoking, screen time, anabolic steroid use, and alcohol consumption. As in the FFQ, alcohol consumption was already taken into account within the groups, and it was decided not to use it as an adjustment variable. In addition, as analyzes were performed by % of UPF grams, the TDEI variable was included in the adjustment. All adjustment variables were considered at 23–25 years of age.
The variables used to analyze loss to follow-up were evaluated using the Chi-square test and, by means of logistic regression, a weight was generated for each participant, obtained by the inverse of the selection probability.
Statistical analyzes were performed using Stata® (version 14.0) software (Stata Corporation, College Station, Texas, USA). Qualitative variables were described in absolute and relative frequencies and quantitative variables in means and standard deviations. To assess the normality of distribution of variables, asymmetry and kurtosis coefficients were used.
Sociodemographic and lifestyle variables were compared using Pearson's chi-square or Fisher's exact test. Means of total calorie consumption, food groups, macronutrients, fiber, alcohol, and body composition were compared between sexes using Student's t-test. The significance level was set at 0.05 and a 95% confidence interval was adopted.
Linear regression models were fitted to verify the association between UPF consumption and body composition measurements. Unadjusted and adjusted analyzes were performed for confounder variables identified in the DAG from the backdoor criterion. Analyzes were performed with linear UPF consumption variables and, later, quadratic terms were added to investigate non-linear effects. The presence of possible interactions (UPF consumption and sex) was also tested. For interactions, a 0.10 significance level was considered. Based on the adjusted regression models, in the presence of interaction, conditional effects were plotted for each body composition outcome according to UPF consumption, for each sex (43). These conditional effects are statistics calculated from predictions of a previously fitted model on fixed values of some covariates and mean or otherwise integrating over the remaining covariates (44).
All analyzes were also performed to verify the association between the UPF consumption caloric percentage (in % kcal) and body composition, whose results are shown in Supplementary Tables S2–S4 and Supplementary Figures S3–S8. For these analyses, adjustment was performed for the variables selected in the DAG, with the exception of alcohol consumption. Furthermore, the TDEI variable was included in the adjustment.
At 23–25 years of age, 50.8% were male, white (61.8%), without a partner (68.6%), non-smokers (82.9%), and with reading and TV time ≥3 h (66.5%). The most prevalent family income was < 5 minimum wages (34.4%). Almost half (49.2%) of the participants had a low level of physical activity, followed by moderate (29.9%) and high (20.5%) levels. Only 2.2% used anabolic steroids (Table 1).
Table 1. Sociodemographic characteristics and lifestyle habits of adults aged 23–25 years participating in the 1978/1979 Ribeirão Preto birth cohort study (São Paulo, Brazil, 2002–2004).
The average daily food consumption was 2,165.5g (±744.1 g), with 35.8% (±15.3%) coming from UPF, 7.2% (±5.7%) from processed foods, and 57.0 % (±15.2%) from food preparations. The mean UPF consumption (% grams) was 36.3% (±14.7%) among women and 35.3% (±15.8%) among men (Table 2).
Table 2. Food group consumption according to the NOVA classification and macronutrients and alcohol consumption by 23–25-year old participants (2002/2004) of the 1978/1979 Ribeirão Preto birth cohort study (São Paulo, Brazil).
There was a high consumption of sugar-sweetened beverages (SSB) (24.65% of the total food items/beverage consumed per day) by the sample studied. After these, the most consumed UPF was snacks, dairy products and candies. The most consumed food items/beverages in each group and the percentage contribution to the total dietary intake (in % of grams and in % of caloric contribution) are listed in Supplementary Tables S1, S2, respectively.
At 37–39 years of age, women had higher total body adiposity (BFP, FMI), distributed in the gynoid region, while men had higher BMI, and greater LM (LMP, LMI, ALMI) and AF (Table 3).
Table 3. Mean of body composition measurements at 37–39 years (2016/2017) according to sex of participants of the 1978/1979 Ribeirão Preto birth cohort study (São Paulo, Brazil).
In the analysis in % grams, a longitudinal association was observed between UPF consumption and FMI (crude analysis: β = 0.02; 95%CI 0.00, 0.04; p = 0.017, and adjusted β = 0.02; 95%CI 0.00, 0.04; p = 0.022); and between UFP consumption and BFP (crude analysis: β = 0.05; 95%CI 0.00, 0.09; p = 0.019, and adjusted β = 0.04; 95%CI 0.00, 0.08; p = 0.012) (Table 4).
Table 4. Crude and adjusted linear regression analysis between ultra-processed foods consumption (% grams) at 23–25 years (2002/2004) and body composition measurements at 37–39 years (2016/2017) in participants of the 1978/1979 Ribeirão Preto birth cohort study (São Paulo, Brazil).
There was interaction between sex and UPF consumption for the BMI (p = 0.058), FMI (p = 0.021), BFP (p = 0.010), AF (p = 0.005), GF (p = 0.017) and LMP (p = 0.002) variables (Table 4). Therefore, the results of the linear regressions of the association between UPF consumption and these variables were calculated conditional effects for each sex (Table 5). In the crude analysis, there was an association between UPF consumption and increased BMI (β = 0.04; 95%CI 0.01, 0.07; p = 0.024), FMI (β = 0.04; 95%CI 0.01,0.06; p = 0.003), BFP (β = 0.08; IC95% 0.03, 0.13; p = 0.001), AF (β = 0.01; IC95% 0.00, 0.01; p = 0.007), GF (β = 0.01; IC95% 0.00, 0.02; p = 0.021), and decrease in LMP (β = −0.06; 95%CI −0.11, −0.02; p = 0.004), only in women. In the adjusted analysis, a 10% increase in the percentage of UPF consumption (in grams) was associated with a longitudinal increase of 0.4 kg/m2 in BMI (β = 0.04; 95%CI 0.01, 0.07; p = 0.023), 0, 4 kg/m2 of LMI (β = 0.04; 95%CI 0.01,0.06; p = 0.002), 0.8% in BFP (β = 0.08; IC95% 0.04, 0.13; p = < 0.001), 0.1 kg of AF (β = 0.01; 95%CI 0.00,0.01; p = 0.003), 0.1 kg of GF (β = 0.01; 95%CI 0.00, 0.02; p = 0.011), and a 0.7% decrease in LMP (β = −0.07; 95%CI −0.11, −0.03; p = 0.001), only in women (Table 5 and Supplementary Figures S3–S8).
Table 5. Conditional effects of ultra-processed foods consumption (% grams) according to sex at 23–25 years (2002/2004) and body composition measurements at 37–39 years (2016/2017) in participants of the 1978/1979 Ribeirão Preto birth cohort study (São Paulo, Brazil).
In analyzes in % of caloric contribution, the longitudinal association was observed only in the crude analyzes for the FMI (p = 0.001), BFP (p < 0.001), GF (p = 0.031), AGR (p = 0.021), LMP (p = 0.003), LMI (p = 0.003) and ALMI (p = 0.001) variables. After adjustments, significance was maintained only for the BFP variable (p = 0.042) (Supplementary Table S3). There was interaction between sex and UPF consumption for the BMI (p = 0.038), FMI (p = 0.018), BFP (p = 0.017), AF (p = 0.071), GF (p = 0.038) and LMP (p = 0.004) variables (Supplementary Table S3). In the analysis of these conditional effects by sex, the crude analysis showed an association between UPF consumption and increased BMI (β = 0.05; 95%CI 0.00, 0.09; p = 0.032), FMI (β = 0.04; 95%CI 0.01, 0.08).; p = 0.008), BFP (β = 0.09; 95%CI 0.02, 0.16; p = 0.007), and decrease in LMP (β = −0.07; 95%CI −0.13, −0.004; p = 0.038), only in women. In the adjusted analysis, the 10% increase in the UPF consumption percentage was associated with a longitudinal increase of 0.5 kg/m2 in BMI (β = 0.05; 95%CI 0.01, 0.09; p = 0.016), 0.5 kg/m2 in FMI (β = 0.05; 95%CI 0.02, 0.08; p = 0.003), 1.1% in BFP (β = 0.11; 95%CI 0.04, 0.17; p = 0.002), and 0.8% decrease in LMP (β = −0.08; 95%CI −0.14, −0.02; p = 0.006), only in women (Supplementary Tables S3, S4 and Supplementary Figures S3–S8).
Quadratic terms of UPF consumption (in %grams and in % caloric contribution) were tested for all body composition variables, but there was no statistical significance.
This study investigated the association between the UPF contribution percentage and the diet and body composition assessed by high validity methods in adults of a cohort study. The greatest UPF contribution to the diet was associated with an increase in fat and a decrease in lean mass years later, only among women. These findings demonstrate that a higher UPF consumption has a negative impact on the body composition of women in the long term, contributing to increasing the prevalence of overweight, which is also a risk factor for the emergence of other NCDs.
As limitations, we point out the use of the FFQ, a method that is subject to memory and measurement bias due to not counting the consumption of food items that are not listed, tending to overestimate food intake. In addition, the FFQ used was not originally constructed aimed at identifying food consumption according to the degree of processing, given that data collection took place in a period before the emergence of the NOVA classification, and some different food groups were placed in the same question. To minimize this limitation, adjustments were made, considering the estimates of food consumption for the state of São Paulo at the time of data collection (37). We reiterate, however, that the instrument used was adapted for use in NCD prevention programs aimed at adults, and was validated (32), reflecting the food consumption of the time, and although there are some limitations, the FFQ is a widely used method in epidemiological studies due to its low cost, practicality, ability to assess the usual diet without changing the pattern of food consumption (45), as long as the methodological aspects for its elaboration are carefully planned, ensuring data reliability and accuracy (45).
Another limitation was the use of the screen time variable, considering that the database only had information about TV time and reading in aggregate form. We emphasize that at the time of data collection (2002/2004), the use of screens such as computers, smartphones and video games, among others, was not yet so widespread in Brazil, therefore not appearing in the large population surveys carried out in the country until then (40). Finally, another limitation of this study involved the losses to follow-up. Inverse probability weighting was used to minimize the effects of these losses on analysis.
As strengths, we highlight the fact that longitudinal studies that evaluated the body composition of adults with high validity methods are still scarce and, to date, there are no studies that have demonstrated associations between UPF consumption and the body composition of adults, especially the influence of UPF consumption on body lean mass.
The UPF contribution percentage in grams in the diet of adults in Ribeirão Preto was 35.8% (equivalent to 38.1% of TDEI). This represents practically double the Brazilian average (19.7% of TDEI), according to the 2017–2018 Household Budget Survey (POF) (19), which can be explained by the fact that the municipality is at more advanced levels of nutritional transition compared to other regions of the country, characterized by high caloric intake, high consumption of foods with low nutritional quality, and a sedentary lifestyle. Furthermore, young individuals, in the age group of the population of this study, tend to consume high levels of UPF in Brazil (46). A high UPF consumption in the diet of adults has been evidenced by several studies, indicating a high risk of developing NCDs due to the unbalanced nutritional composition of these products (14–22).
A highlight is the high ultra-processed SSB (soft drinks and industrialized juices) consumption, corresponding, in grams, to practically of the total of food items/beverages consumed/day (24.7%), which is equivalent to 10.24% in caloric contribution percentage (Supplementary Tables S1, S2). High SSB consumption is associated with potential hormonal dysregulation, insulin resistance, dyslipidemia, increased adiposity and obesity, and is also related to obesogenic behaviors, such as sedentary lifestyle and high screen time (47, 48), lifestyle habits that have been common in the population of this study. In addition, a higher SSB consumption (but not the general UPF group) was associated with higher urinary concentrations of bisphenol A (BPA), an endocrine disruptor that is associated with increased prevalence of risk factors for abdominal obesity, diabetes and hypertension (48, 49).
The pattern of body composition found in this research, where women had higher levels of total adiposity and GF, and men had higher means of BMI, AF, AGR and lean mass was also found in other ethnic groups (38). This pattern can be explained due to biological differences (50, 51), represents a higher risk of sarcopenic obesity for women (51) and a higher risk of cardiovascular diseases and metabolic syndrome (52) for men considering that body fat distribution has a greater impact on cardio-metabolic risk than excess total adiposity (51).
Despite this pattern of body distribution, our results showed a deleterious effect of UPF only on the body composition of women, corroborating other evidence that associates UPF with excess body weight and consequent higher adiposity (20–22, 53–57), especially in women. In another Brazilian study, the authors found a positive association between UPF consumption and overweight and obesity in women, but not in men (21). In Switzerland, UPF was associated with excess body weight only in women (54). In South Korea, UPF was also associated with obesity only among women (55). In the United States, Juul et al. (20) observed significant positive associations between UPF consumption and overweight or obesity in both sexes, but with a more pronounced association among women, a result also found in the United Kingdom (20, 22).
Scientific evidence suggests that UPF promotes changes in body composition through a range of mechanisms, such as the unbalanced nutritional composition of foods and beverages, which favors increased energy and nutrient consumption and ingredients of low nutritional quality, such as refined sugars (11–18); the presence of food additives and contaminants produced during processing, which alter the profile and composition of the intestinal microbiota (56), and changes in the food matrix induced by food processing that seem to influence the kinetics of nutrient absorption and alterations in gut-brain satiety signaling (48, 53), among other mechanisms not yet clarified that interrelate, promoting inflammation, oxidative stress and consequent weight gain (48). The effects of these mechanisms appear to affect the sexes differently, with more impact on women. UPF is associated with a higher glycemic response, and women appear to be more sensitive to the UPF hyperglycemic effect than men (48). Besides, changes in the intestinal microbiota caused by UPF seem to affect men and women differently (56).
As for the distribution of body fat, a higher UPF consumption favored the increase in AF and GF, but not in AGR, also only among women. Despite the non-association with AGR, which is an indicator of abdominal obesity, UPF is found to be more harmful to women with regard to the association with an increase in AF, which is associated with a greater risk of cardiovascular outcomes and metabolic syndrome (28, 52). We emphasize that this association for AF may not have been found in the analysis in the UPF caloric contribution percentage due to the fact that the analysis in grams takes into account foods and beverages widely consumed by the population, which have a high content of additives and low caloric contribution, such as SSB, especially diet and light soft drinks.
An association between UPF consumption and a decrease in LMP was demonstrated only in women. Although no association was found for LMI and ALMI, which are indices relativized by height are commonly associated with the diagnosis of sarcopenia, especially the latter, these results are worrying given the importance of LM in maintaining the population's health and quality of life. LM provides useful information about an individual's health and nutrition, playing an important role in maintaining bone density, preserving strength, reducing the risk of injuries and falls, and improving metabolism and general health. Reduction in LM with age or due to sarcopenia is associated with decreased function and quality of life and the frailty development (57, 58). Thus, although the reduction in LM was not detected by the indices generally used in the diagnosis of sarcopenia, the decrease in LMP already points to a deleterious effect of UPF on LM, not having been detected by LMI and ALMI because this population is still found at the peak of the LM. Furthermore, this pattern of body distribution characterized by high adiposity and decreased LM in women points to the development of sarcopenic obesity, which makes them more prone to the development of diabetes mellitus, abdominal obesity, and an increased risk of death (59).
Our results indicate that the high UPF contribution percentage to the diet of Brazilian adults is associated with a longitudinal increase in FM and a decrease in LM only among women, favoring a deleterious composition pattern for females.
The original contributions presented in the study are included in the article/Supplementary material, further inquiries can be directed to the corresponding author/s.
The studies involving human participants were reviewed and approved by Research Ethics Committee (CEP) of the University Hospital of FMRP-USP School of Medicine. The patients/participants provided their written informed consent to participate in this study.
LR performed material preparation and data analysis and wrote the first draft of the manuscript. All authors commented on the other versions, contributed to the study conception and design, read, and approved the final manuscript.
This research was funded by the Coordination for the Improvement of Higher Education Personnel-Brazil (CAPES), the National Council for Scientific and Technological Development -CNPq (grant number 400943/2013-1), the Research Support Foundation of the State of São Paulo -FAPESP (grant number 00/09508-7) and the Teaching, Research, and Assistance Support Foundation (FAEPA).
We would like to thank CAPES, CNPq, FAPESP, FAEPA, and the Graduate Program in Collective Health (UFMA).
The authors declare that the research was conducted in the absence of any commercial or financial relationships that could be construed as a potential conflict of interest.
All claims expressed in this article are solely those of the authors and do not necessarily represent those of their affiliated organizations, or those of the publisher, the editors and the reviewers. Any product that may be evaluated in this article, or claim that may be made by its manufacturer, is not guaranteed or endorsed by the publisher.
The Supplementary Material for this article can be found online at: https://www.frontiersin.org/articles/10.3389/fnut.2022.1006018/full#supplementary-material
ADP, Air displacement plethysmography; AG, Android fat; AGR, Android-gynoid fat ratio; ALMI, Appendicular lean mass index; BPA, Bisphenol A; BFP, Body fat percentage; BMI, Body mass index; NCDs, Chronic noncommunicable diseases; DXA, Dual-energy R-ray absorptiometry; FM, Fat mass; FMI, Fat mass index; FFM, Fat-free mass; FFQ, Food frequency questionnaire; GF, Gynoid fat; LM, Lean mass; LMI, Lean mass index; LMP, Lean mass percentage; MM, Muscle mass; POF, Household Budget Survey; SSB, Sugar-sweetened beverages; TDEI, Total dietary energy intake; UPF, Ultra-processed food.
1. Mazzoccoli G. Body composition: where and when. Eur J Radiol. (2016) 85:1456–60. doi: 10.1016/j.ejrad.2015.10.020
2. Kuriyan R. Body composition techniques. Indian J Med Res. (2018) 148:648–58. doi: 10.4103/ijmr.IJMR_1777_18
3. Borga M, West J, Bell JD, Harvey NC, Romu T, Heymsfield SB, et al. Advanced body composition assessment: from body mass index to body composition profiling. J Investig Med. (2018) 66:1–9. doi: 10.1136/jim-2018-000722
4. Westerterp KR. Exercise, energy balance and body composition. Eur J Clin Nutr. (2018) 72:1246–50. doi: 10.1038/s41430-018-0180-4
5. Xu F, Greene GW, Earp JE, Adami A, Delmonico MJ, Lofgren IE, et al. Relationships of physical activity and diet quality with body composition and fat distribution in US adults. Obesity (Silver Spring). (2020) 28:2431–40. doi: 10.1002/oby.23018
6. Hashimoto Y, Fukuda T, Oyabu C, Tanaka M, Asano M, Yamazaki M, et al. Impact of low-carbohydrate diet on body composition: meta-analysis of randomized controlled studies. Obes Rev. (2016) 17:499–509. doi: 10.1111/obr.12405
7. Wycherley TP, Moran LJ, Clifton PM, Noakes M, Brinkworth GD. Effects of energy-restricted high-protein, low-fat compared with standard-protein, low-fat diets: a meta-analysis of randomized controlled trials. Am J Clin Nutr. (2012) 96:1281–98. doi: 10.3945/ajcn.112.044321
8. Kim JE, O'Connor LE, Sands LP, Slebodnik MB, Campbell WW. Effects of dietary protein intake on body composition changes after weight loss in older adults: a systematic review and meta-analysis. Nutr Rev. (2016) 74:210–24. doi: 10.1093/nutrit/nuv065
9. Aragon AA, Schoenfeld BJ, Wildman R, Kleiner S, VanDusseldorp T, Taylor L, et al. International society of sports nutrition position stand: diets and body composition. J Int Soc Sports Nutr. (2017) 14:14–6. doi: 10.1186/s12970-017-0174-y
10. Han CS, Dingemanse NJ. You are what you eat: diet shapes body composition, personality and behavioural stability. BMC Evol Biol. (2017) 17:8. doi: 10.1186/s12862-016-0852-4
11. Monteiro CA, Levy RB, Claro RM, Castro IR, Cannon G, A. new classification of foods based on the extent and purpose of their processing. Cad Saude Publica. (2010) 26:2039–49. doi: 10.1590/S0102-311X2010001100005
12. Monteiro CA, Levy RB, Claro RM, de Castro IR, Cannon G. Increasing consumption of ultra-processed foods and likely impact on human health: evidence from Brazil. Public Health Nutr. (2011) 14:5–13. doi: 10.1017/S1368980010003241
13. Monteiro CA, Cannon G, Levy RB, Moubarac JC, Louzada ML, Rauber F, et al. Ultra-processed foods: what they are and how to identify them. Public Health Nutr. (2019) 22:936–41. doi: 10.1017/S1368980018003762
14. Lane MM, Davis JA, Beattie S, Gómez-Donoso C, Loughman A, O'Neil A, et al. Ultraprocessed food and chronic noncommunicable diseases: a systematic review and meta-analysis of 43 observational studies. Obes Rev. (2021) 22:e13146. doi: 10.1111/obr.13146
15. Monteiro CA, Cannon G, Lawrence M, Louzada MLC, Machado PP. Ultra-processed Foods, Diet Quality, Health Using the NOVA Classification System. Rome: FAO. (2019). p. 48. Available online at: https://www.fao.org/3/ca5644en/ca5644en.pdf (accessed July 21, 2021).
16. Pagliai G, Dinu M, Madarena MP, Bonaccio M, Iacoviello L, Sofi F. Consumption of ultra-processed foods and health status: a systematic review and meta-analysis. Br J Nutr. (2021) 125:308–18. doi: 10.1017/S0007114520002688
17. Chen X, Zhang, Z, Yang H, Qiu P, Wang H, et al. Consumption of ultra-processed foods and health outcomes: a systematic review of epidemiological studies. Nutr J. (2020) 19:86. doi: 10.1186/s12937-020-00604-1
18. Elizabeth L, Machado P, Zinöcker M, Baker P, Lawrence M. Ultra-processed foods and health outcomes: a narrative review. Nutrients. (2020) 12:1955. doi: 10.3390/nu12071955
19. Instituto Brasileiro de Geografia e Estatística,. Pesquisa de orçamentos familiares 2017-2018: análise do consumo alimentar pessoal no Brasil. (2020). Available online at: https://biblioteca.ibge.gov.br/visualizacao/livros/liv101742.pdf (accessed March 15, 2021).
20. Juul F, Martinez-Steele E, Parekh N, Monteiro CA, Chang VW. Ultra-processed food consumption and excess weight among US adults. Br J Nutr. (2018) 120:90–100. doi: 10.1017/S0007114518001046
21. Louzada MLC, Martins AP, Canella DS, Baraldi LG, Levy RB, Claro RM, et al. Ultra-processed foods and the nutritional dietary profile in Brazil. Rev Saude Publica. (2015) 49:38. doi: 10.1590/S0034-8910.2015049006132
22. Rauber F, Chang K, Vamos EP, Louzada MLC, Monteiro CA, Millett C, et al. Ultra-processed food consumption and risk of obesity: a prospective cohort study of UK Biobank. Eur J Nutr. (2021) 60:2169–80. doi: 10.1007/s00394-020-02367-1
23. Askari M, Heshmati J, Shahinfar H, Tripathi N, Daneshzad E. Ultra-processed food and the risk of overweight and obesity: a systematic review and meta-analysis of observational studies. Int J Obes. (2020) 44:2080–91. doi: 10.1038/s41366-020-00650-z
24. Canhada SL, Luft VC, Giatti L, Duncan BB, Chor D, Fonseca MJMD, et al. Ultra-processed foods, incident overweight and obesity, and longitudinal changes in weight and waist circumference: the Brazilian Longitudinal Study of Adult Health (ELSA-Brasil). Public Health Nutr. (2020) 23:1076–86. doi: 10.1017/S1368980019002854
25. Costa CS, Rauber F, Leffa PS, Sangalli CN, Campagnolo P, Vitolo MR. Ultra-processed food consumption and its effects on anthropometric and glucose profile: a longitudinal study during childhood. Nutr Metab Cardiovasc Dis. (2019) 29:177–84. doi: 10.1016/j.numecd.2018.11.003
26. Viola PCAF, Carvalho CA, Bragança MLBM, França AKTDC, Alves MTSSBE, da Silva AAM. High consumption of ultra-processed foods is associated with lower muscle mass in Brazilian adolescents in the RPS birth cohort. Nutrition. (2020) 79-80:110983. doi: 10.1016/j.nut.2020.110983
27. Chang K, Khandpur N, Neri D, Touvier M, Huybrechts I, Millett C, et al. Association between childhood consumption of ultraprocessed food and adiposity trajectories in the avon longitudinal study of parents and children birth cohort. JAMA Pediatr. (2021) 175:e211573. doi: 10.1001/jamapediatrics.2021.1573
28. Konieczna J, Morey M, Abete I, Bes-Rastrollo M, Ruiz-Canela M, Vioque J, et al. Contribution of ultra-processed foods in visceral fat deposition and other adiposity indicators: Prospective analysis nested in the PREDIMED-Plus trial. Clin Nutr. (2021) 40:4290–300. doi: 10.1016/j.clnu.2021.01.019
29. Barbieri MA, Ferraro AA, Simões VMF, Goldani MZ, Cardoso VC, Silva AAM, et al. Cohort Profile: The 1978-79 Ribeirao Preto (Brazil) birth cohort study. Int J Epidemiol. (2022) 51:27–28g. doi: 10.1093/ije/dyab190
30. Barbieri MA, Bettiol H, Silva AAM, Cardoso VC, Simões VM, Gutierrez MR, et al. Health in early adulthood: the contribution of the 1978/79 Ribeirão Preto birth cohort. Braz J Med Biol Res. (2006) 39:1041–55. doi: 10.1590/S0100-879X2006000800007
31. Ribeiro AB, Cardoso MA. Construção de um questionário de freqüência alimentar como subsídio para programas de prevenção de doenças crônicas não transmissíveis. Rev Nutr. (2002) 15:239–45. doi: 10.1590/S1415-52732002000200012
32. Molina MC, Bettiol H, Barbieri MA, Silva AA, Conceição SI, Dos-Santos JE. Food consumption by young adults living in Ribeirão Preto, SP, 2002/2004. Braz J Med Biol. (2007) 40:1257–66. doi: 10.1590/S0100-879X2006005000168
33. Núcleo de Estudos e Pesquisas em Alimentação da Universidade Estadual de Campinas,. Tabela Brasileira de Composição de Alimentos – TACO. (2011). Available online at: https://www.nepa.unicamp.br/taco/contar/taco_4_edicao_ampliada_e_revisada.pdf?arquivo=1 (accessed March 10, 2021).
34. Instituto Brasileiro de Geografia e Estatística - Pesquisa de Orçamentos Familiares 2008-2009,. Tabelas de Composição Nutricional dos Alimentos Consumidos no Brasil. (2010). Available online at: https://biblioteca.ibge.gov.br/visualizacao/livros/liv50002.pdf (accessed March 12,2021).
35. United United States Department of Agriculture Agricultural Research Service –, USDA,. USDA National Nutrient Database for Standard Reference, Release 24. (2011). Available online at: http://www.ars.usda.gov/ba/bhnrc/ndl (accessed March 10, 2021).
36. Louzada MLC, Canella DS, Jaime PC, Monteiro CA. Alimentação e saúde: a fundamentação científica do guia alimentar para a população brasileira. São Paulo: Faculdade de Saúde Pública da Universidade de São Paulo (2019). p. 133. Available online at: http://doi.org/10.11606/9788588848344 (accessed July 21, 2021).
37. Instituto Brasileiro de Geografia e Estatística,. Pesquisa de orçamentos familiares 2002/2003: análise da disponibilidade domiciliar de alimentos e do estado nutricional no Brasil. (2004). Available online at: https://biblioteca.ibge.gov.br/visualizacao/livros/liv4472.pdf (accessed January 15, 2021).
38. Kelly TL, Wilson KE, Heymsfield SB. Dual energy X-Ray absorptiometry body composition reference values from NHANES. PLoS ONE. (2009) 4:e7038. doi: 10.1371/journal.pone.0007038
39. Baumgartner RN, Koehler KM, Gallagher D, Romero L, Heymsfield SB, Ross RR, et al. Epidemiology of sarcopenia among the elderly in New Mexico. Am J Epidemiol. (1998) 147:755–63. doi: 10.1093/oxfordjournals.aje.a009520
40. Brasil. Ministério da Saúde. Vigitel Brasil 2012: vigilância de fatores de risco e proteção para doenças crônicas por inquérito telefônico. (2013). Available online at: https://bvsms.saude.gov.br/bvs/publicacoes/vigitel_brasil_2012.pdf (accessed July 21, 2021).
41. Matsudo S, Araújo T, Matsudo V, Andrade D, Andrade E, Oliveira LC, et al. Questionário Internacional de Atividade Física (IPAQ): estudo de validade e reprodutibilidade no Brasil. Rev Bras Ativ Fis. (2012) 6:5–18. Available online at: https://rbafs.org.br/RBAFS/article/view/931 (accessed July 21, 2021).
42. Silva AAM. Introdução à Inferência Causal em Epidemiologia: uma abordagem gráfica e contrafatual. Rio de Janeiro: Editora Fiocruz (2021). p. 402.
43. Kline RB. Principles and Practice of Structural Equation Modeling. New York: The Guilford Press (2015). p. 534.
44. StataCorp. Stata: Release 17. (2021). Available online at: https://www.stata.com/manuals/u.pdf (accessed February 22, 2021).
45. Cade J, Thompson R, Burley V, Warm D. Development, validation and utilisation of food-frequency questionnaires - a review. Public Health Nutr. (2002) 5:567–87. doi: 10.1079/PHN2001318
46. Costa CDS, Sattamini IF, Steele EM, Louzada MLDC, Claro RM, Monteiro CA. Consumption of ultra-processed foods and its association with sociodemographic factors in the adult population of the 27 Brazilian state capitals (2019). Rev Saude Publica. (2021) 55:47. doi: 10.11606/s1518-8787.2021055002833
47. Silva DCG, Segheto W, Amaral FCS, Reis NDA, Veloso GSS, Pessoa MC, et al. Consumo de bebidas açucaradas e fatores associados em adultos. Cienc Saude colet. (2019) 24:899–906. doi: 10.1590/1413-81232018243.05432017
48. Juul F, Vaidean G, Parekh N. Ultra-processed foods and cardiovascular diseases: potential mechanisms of action. Adv Nutr. (2021) 12:1673–80. doi: 10.1093/advances/nmab049
49. Buckley JP, Kim H, Wong E, Rebholz CM. Ultra-processed food consumption and exposure to phthalates and bisphenols in the US National Health and Nutrition Examination Survey, 2013-2014. Environ Int. (2019) 131:105057. doi: 10.1016/j.envint.2019.105057
50. Imboden MT, Swartz AM, Finch HW, Harber MP, Kaminsky LA. Reference standards for lean mass measurements using GE dual energy x-ray absorptiometry in Caucasian adults. PLoS ONE. (2017) 12:e0176161. doi: 10.1371/journal.pone.0176161
51. Bredella MA. Sex differences in body composition. Adv Exp Med Biol. (2017) 1043:9–27. doi: 10.1007/978-3-319-70178-3_2
52. Wiklund P, Toss F, Weinehall L, Hallmans G, Franks PW, Nordström A, et al. Abdominal and gynoid fat mass are associated with cardiovascular risk factors in men and women. J Clin Endocrinol Metab. (2008) 93:4360–66. doi: 10.1210/jc.2008-0804
53. Hall KD, Ayuketah A, Brychta R, Cai H, Cassimatis T, Chen KY, et al. Ultra-processed diets cause excess calorie intake and weight gain: an inpatient randomized controlled trial of ad libitum food intake. Cell Metab. (2019) 30:67–77.e3. doi: 10.1016/j.cmet.2019.05.020
54. Pestoni G, Habib L, Reber E, Rohrmann S, Staub K, Stanga Z, et al. Ultraprocessed food consumption is strongly and dose-dependently associated with excess body weight in swiss women. Obesity. (2021) 29:601–9. doi: 10.1002/oby.23091
55. Sung H, Park JM, Oh SU, Ha K, Joung H. Consumption of ultra-processed foods increases the likelihood of having obesity in Korean women. Nutrients. (2021) 13:698. doi: 10.3390/nu13020698
56. Cuevas-Sierra A, Milagro FI, Aranaz P, Martínez JA, Riezu-Boj JI. Gut microbiota differences according to ultra-processed food consumption in a spanish population. Nutrients. (2021) 13:2710. doi: 10.3390/nu13082710
57. Fielding RA, Vellas B, Evans WJ, Bhasin S, Morley JE, Newman AB, et al. Sarcopenia: an undiagnosed condition in older adults. Current consensus definition: prevalence, etiology, and consequences. J Am Med Dir. (2011) 12:249–56. doi: 10.1016/j.jamda.2011.01.003
58. Rizzoli R, Reginster JY, Arnal JF, Bautmans I, Beaudart C, Bischoff-Ferrari H, et al. Quality of life in sarcopenia and frailty. Calcif Tissue Int. (2013) 93:101–20. doi: 10.1007/s00223-013-9758-y
Keywords: ultra-processed foods (UPFs), food consumption, diet, body composition, body fat
Citation: Rudakoff LCS, Magalhães EIDS, Viola PCAF, de Oliveira BR, Coelho CCNDS, Bragança MLBM, Arruda SPM, Cardoso VC, Bettiol H, Barbieri MA, Levy RB and Silva AAMD (2022) Ultra-processed food consumption is associated with increase in fat mass and decrease in lean mass in Brazilian women: A cohort study. Front. Nutr. 9:1006018. doi: 10.3389/fnut.2022.1006018
Received: 28 July 2022; Accepted: 12 September 2022;
Published: 14 October 2022.
Edited by:
Raquel Mendonça, Universidade Federal de Ouro Preto, BrazilReviewed by:
Jordana Herzog Siqueira, Federal University of Espirito Santo, BrazilCopyright © 2022 Rudakoff, Magalhães, Viola, de Oliveira, da Silva Coelho, Bragança, Arruda, Cardoso, Bettiol, Barbieri, Levy and da Silva. This is an open-access article distributed under the terms of the Creative Commons Attribution License (CC BY). The use, distribution or reproduction in other forums is permitted, provided the original author(s) and the copyright owner(s) are credited and that the original publication in this journal is cited, in accordance with accepted academic practice. No use, distribution or reproduction is permitted which does not comply with these terms.
*Correspondence: Lívia Carolina Sobrinho Rudakoff, bGl2aWEucnVkYWtvZmZAaWZtYS5lZHUuYnI=
Disclaimer: All claims expressed in this article are solely those of the authors and do not necessarily represent those of their affiliated organizations, or those of the publisher, the editors and the reviewers. Any product that may be evaluated in this article or claim that may be made by its manufacturer is not guaranteed or endorsed by the publisher.
Research integrity at Frontiers
Learn more about the work of our research integrity team to safeguard the quality of each article we publish.