- 1Department of Cardiology, Guangdong Provincial Key Laboratory of Coronary Heart Disease Prevention, Guangdong Cardiovascular Institute, Guangdong Provincial People's Hospital, Guangdong Academy of Medical Sciences, Guangzhou, China
- 2Department of Dermatology, Shenzhen People's Hospital, The Second Clinical Medical College, Jinan University, The First Affiliated Hospital, Southern University of Science and Technology, Shenzhen, China
- 3Guangdong Provincial People's Hospital, School of Medicine, South China University of Technology, Guangzhou, China
- 4Department of Cardiology, The First People's Hospital of Foshan, Foshan, China
- 5Guangdong Provincial People's Hospital, The Second School of Clinical Medicine, Southern Medical University, Guangzhou, China
- 6Department of Endocrinology, Longyan First Hospital Affiliated With Fujian Medical University, Longyan, China
Background: Previous studies reported that patients with coronary artery disease (CAD) and well-controlled baseline LDL-C (<1.8 mmol/L) still had higher long-term all-cause mortality. However, no study has been conducted to explore the independent risk factors for long-term mortality. In addition, there also was no study evaluating the population attributable risk (PAR) of independent risk factors in combination with their prevalence and relative risk. Therefore, we aimed to identify the independent risk factors and estimate their PAR in patients with CAD and well-controlled baseline LDL-C (<1.8 mmol/L).
Methods: We analyzed 4,863 consecutive CAD patients with well-controlled baseline LDL-C admitted to Guangdong Provincial People's Hospital in China from January 2007 to December 2018. Independent risk factors for long-term all-cause death were evaluated through stepwise approach and multivariable Cox regression analysis. PAR of independent risk factors was calculated with their hazard ratio and prevalence among our cohort.
Results: The overall mortality was 16.00% (n = 778) over a median follow-up period of 5.93 years. Independent risk factors for all-cause death included malnutrition, age ≥75 years, congestive heart failure (CHF), chronic kidney disease (CKD) and atrial fibrillation. Among these risk factors of interest, the hazard ratio (HR) of severe malnutrition was the highest (HR 2.82, 95% CI: 1.86–4.26), and the PAR of mild malnutrition was the highest (19.49%, 95% CI: 0.65–36.01%).
Conclusion: Malnutrition, age ≥75 years, CHF, CKD and atrial fibrillation were independent predictors for long-term all-cause mortality in CAD patients with well-controlled LDL-C levels. Considering prevalence of these risk factors, more attention should be paid to the occurrence of mild malnutrition for these patients.
Clinical Trial Registration: ClinicalTrials.gov, identifier: NCT04407936.
Introduction
It has been established that serum low-density lipoprotein cholesterol (LDL-C) is a key risk factor for coronary artery disease (CAD) (1–3). Guidelines also recommended LDL-C as a target for the primary and secondary prevention of CAD (1). However, LDL-C levels are not always elevated in patients with CAD (4, 5). Previous study showed CAD patients with well-controlled baseline LDL-C (<1.8 mmol/L) had higher long-term all-cause mortality than those with poorly controlled baseline LDL-C levels (≥1.8 mmol/L) (6). Currently, there is not enough information on the management of this population improving prognosis for clinicians. It is necessary to identify the independent predictors of all-cause death.
In clinical practice, prevalence of risk factors should also be taken into account. The population attributable risk (PAR) combines the relative risk of a risk factor with its prevalence in a given population to determine the proportion of outcomes associated with (or attributable to) that factor (7). It can evaluate the prevalence and risk degree of risk factors comprehensively. Therefore, this study aims to determine the independent predictors of mortality for CAD patients with baseline well-controlled LDL-C levels and estimate their PAR among a large CAD cohort.
Method
Study Design and Participants
The retrospective observational study was conducted in Guangdong Provincial People's Hospital, China (Clinicaltrials.gov NCT04407936) from January 2007 to December 2018. During this period, there were 88,938 patients underwent coronary angiography (CAG) and 59,667 patients were diagnosed as CAD. We excluded patients <18 years old (n = 19), prior myocardial infarction (n = 3,922), prior undergoing PCI (n = 4,996), prior undergoing coronary artery bypass grafting (n = 328), cancer (n = 659), lacking LDL-C examination (n = 1,782), missing follow-up information of mortality (n = 6,662) and LDL-C level ≥1.8 mmol/L(n = 36,366). A total of 4,863 patients were eventually included in the study (Figure 1). The study protocol was approved by the Ethics Committee of Guangdong Provincial People's Hospital (No. GDREC2019555H[R1]) and was conducted in accordance with the Declaration of Helsinki.
Procedures
The clinical information for each patient in cohort was extracted from the electronic Clinical Management System of the Guangdong Provincial People's Hospital. Baseline data were collected on demographic characteristics, coexisting conditions, laboratory tests, nutritional status and medication at discharge. In all patients, blood samples except lipid profiles were collected at admission or before coronary angiography (CAG) and percutaneous coronary intervention (PCI). The lipid profiles were measured by fasting blood samples which were collected between 06:00 and 08:00 in the morning. For patients admitted between 0:00 and 06:00, blood samples were collected between 06:00 and 08:00 in the morning of the same day. For the remaining patients, blood samples were collected between 06:00 and 08:00 on the next day after admission. CAG/PCI was performed in accordance with the standard clinical practice guidelines (8–10). Senior cardiologists were responsible for the data quality control and periodical data verification. Follow-up data were monitored and recorded by trained nurses through outpatient interviews and telephone follow-up. The follow-up information of patients lost to follow-up was retrieved from Guangdong Public Security System.
Clinical Outcome and Definition
The primary endpoint was long-term all-cause mortality. CAD was confirmed by CAG and discriminated according to the 10th Revision Codes of the International Classification of Diseases. Comorbidities included acute myocardial infarction (AMI), diabetes mellitus, hypertension, chronic kidney disease (CKD), atrial fibrillation, chronic obstructive pulmonary disease (COPD), congestive heart failure (CHF) and anemia. CHF was defined as New York Heart Association (NYHA) class >2 or Killip class >1 (11). CKD was recognized as estimated glomerular filtration rate (eGFR) ≤ 60 mL/min/1.73 m2 and the eGFR was determined by the Modification of Diet in Renal Disease (MDRD) formula (12–14). Anemia was defined as hematocrit <39% for men and hematocrit <36% for women, according to the World Health Organization criteria (15). Nutritional status was assessed by the Controlling Nutritional Status (CONUT) score, a screening tool for the nutritional status of hospitalized patients (16). It was calculated by serum albumin, cholesterol, and total lymphocyte count. Different scores represent different nutritional status (0–1 is normal; 2–4 is mild malnutrition; 5–8 is moderate malnutrition; 9–12 is severe malnutrition).
Statistical Analysis
Patients were divided into 2 groups: those who died or survived during the follow-up period. Data are reported as mean (SD), median (interquartile range [IQR]), or numbers and percentages. Differences of continuous variables with normal or non-normal distributions between two groups were evaluated using independent sample student t-test or Wilcoxon rank sum test. Categorical variables were tested using Pearson chi-square test.
Risk factors which were significantly different in the two groups and with missing value <15% were included in the univariable Cox regression analysis to preliminarily determine the association with long-term all-cause mortality. When interaction or collinearity existed between variables, the modifiable or categorical one was preferred to facilitate clinical application. Correlations between LDL-C and nutritional status and its components were assessed by the Spearman correlation test. Then variables associated with all-cause mortality with p < 0.05 in the univariate analysis were enrolled in a Cox regression analysis with backward stepwise selection to determine the independent predictors of all-cause mortality in CAD patients with well-controlled LDL-C levels. Multivariable Cox regression model including all the remaining risk factors was then fitted. The results were reported as the adjusted hazard ratio (HR) and associated 95% confidence intervals (CI). Furthermore, to comprehensively consider the prevalence and HR of risk factors, the study calculated the population attributable risk (PAR). PAR was calculated using the equation PAR = P (HR-1)/ [1 + P (HR-1)], where P is the prevalence of eventually determined risk factor in our cohort. The standard error of PAR was calculated using the delta method (17). All data analysis was performed by R software, version 3.6.3 (R Foundation for Statistical Computing). The results of all analyses with p < 0.05 were considered statistically significant.
Result
Baseline Characteristic
Continuing with previous studies (6), this study included 4,863 CAD patients with well-controlled baseline LDL-C level (<1.8 mmol/L). Among all recruited patients, the long-term all-cause mortality rate was 16.00% (n = 778) during a median follow-up of 5.93 (IQR 4.08–8.40) years. Table 1 detailed the patient's clinical characteristics. Compared with long-term survivors, those who died during the follow-up period were more likely to be older and had higher prevalence of AMI, CHF, CKD, atrial fibrillation and anemia. In addition, the rates of moderate malnutrition and severe malnutrition were also higher.
Correlations Between LDL-C and Nutritional Status and Its Components
Figure 2 showed all correlations between LDL-C and nutritional status evaluated by CONUT score and components of CONUT score. LDL-C did not show a significant correlation with nutritional status (r = −0.08, p < 0.001). As for the correlations between LDL-C and components of CONUT score, there was no strong correlation between LDL-C and total cholesterol (r = 0.30, p < 0.001). Additionally, LDL-C was not correlated with lymphocyte count and albumin (p = 0.65 and 0.14).
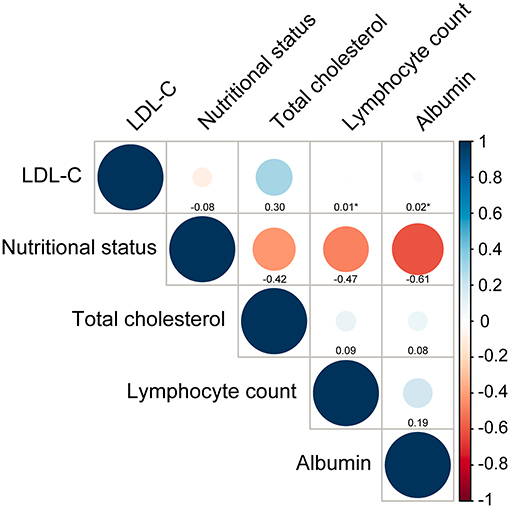
Figure 2. Correlations between LDL-C and nutritional status and its components. Nutritional status is assessed by Controlling Nutritional Status (CONUT) score. Total cholesterol, lymphocyte count and albumin are components of CONUT score. *p-value for spearman correlation >0.05.
Risk Factors for All-Cause Mortality
The multivariate Cox regression analysis with backward stepwise identified that age ≥75 years (HR 1.61, 95% CI: 1.37–1.91), CHF (HR 1.84, 95% CI: 1.48–2.28), CKD (HR 1.71, 95% CI: 1.46–2.01), atrial fibrillation (HR 1.93, 95% CI: 1.34–2.79), mild malnutrition (HR 1.37, 95% CI: 1.01–1.86), moderate malnutrition (HR 1.79, 95% CI: 1.30–2.47) and severe malnutrition (HR 2.82, 95% CI: 1.86–4.26) were independently associated with long-term all-cause mortality (Table 2). The results of Cox model with additional adjustment for AMI were showed in Supplementary Table 1, which were similar as the results in above multivariate model.
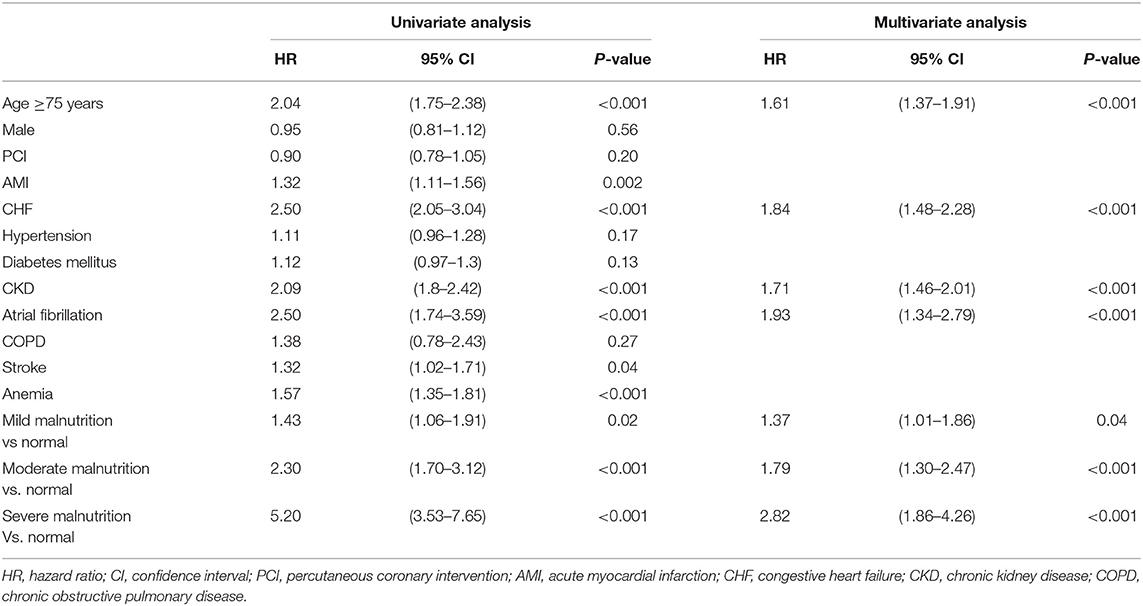
Table 2. Univariate regression analysis and multivariate analysis with backward stepwise for the association between all-cause death and clinical findings during follow-up period.
PAR of Risk Factors for All-Cause Mortality
Among these risk factors of interest of all-cause death, the prevalence was highest for mild malnutrition (65.43%), followed by CKD (24.84%), moderate malnutrition (22.17%), age ≥75 years (19.10%), CHF (8.42%), atrial fibrillation (2.73%), and the lowest was 2.70% for severe malnutrition. The PAR was highest for mild malnutrition (19.49, 95% CI: 0.65–36.01%), followed by CKD (14.99, 95% CI: 10.25–20.06%), moderate malnutrition (14.90, 95% CI: 6.24–24.58%), age ≥75 years (10.44, 95% CI: 6.60–14.81%), CHF (6.61, 95% CI: 3.88–9.73%), severe malnutrition (4.68, 95% CI: 2.27-8.09%) and atrial fibrillation (2.48, 95% CI: 0.92–4.66%) (Figure 3). The PAR based on Cox model with additional adjustment for AMI were showed in Supplementary Table 1, which were similar as the above results.
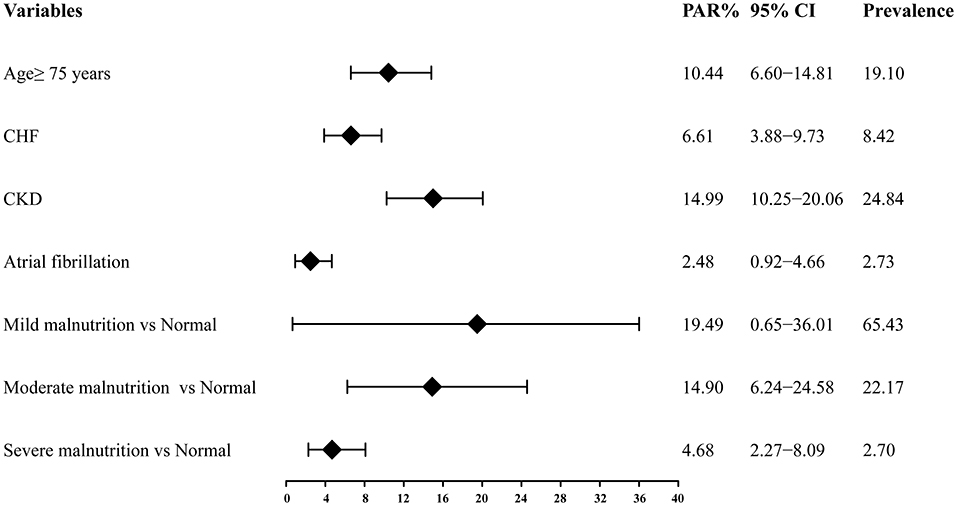
Figure 3. Population attributable risk of the independent risk factors of long-term all-cause mortality. PAR, population attributable risk; CI, confidence interval; CHF, congestive heart failure; CKD, chronic kidney disease.
Discussion
To our acknowledge, it is the first study to explore the independent risk factors of long-term all-cause mortality in CAD patients with well-controlled LDL-C levels. Furthermore, this study estimated the proportion of all-cause mortality attributed to four risk factors by calculating PAR. The relative risk was highest for severe malnutrition. Considering the prevalence of identified risk factors, the highest PAR was found for mild malnutrition, followed by CKD, moderate malnutrition, age ≥75 years, CHF, severe malnutrition and atrial fibrillation.
Among these identified risk factors, malnutrition showed a significant association with poor prognosis. Previous studies reported many nutrition assessment tools, categorized as subjective nutritional screening assessment tools and objective nutrition screening tools (18). Subjective nutritional screening assessment tools such as Subjective Global Assessment (SGA) (19), Mini Nutritional Assessment (MNA) (20), Nutritional Risk Index (NRI) (21), etc. require anthropometric measurements, indication of disease severity, clinical assessment, or an extensive questionnaire addressing several aspects of nutritional intake. Although these tools can evaluate the nutrition status comprehensively, they are complex and time-consuming, which may not be convenient in clinical practice. Objective nutrition screening tools evaluate nutritional status by height, weight and/ or blood parameters which are readily available from routine clinical laboratory examination. These tools including CONUT score (16), Prognostic Nutritional Index (PNI) (22), Geriatric Nutritional Risk Index (GNRI) (23), etc. are convenient in clinical practice. Previous studies demonstrated that the CONUT score has been demonstrated as a suitable tool for evaluating the nutritional status among CAD patients (24). In our study, we useed the CONUT score as the screening tool to assess patients' nutritional status. Compared with normal nutritional status, each type of malnutrition increased the risk of all-cause mortality. Risks increased by 37%, 79% and 182% in mild, moderate and severe malnutrition. Severe malnutrition had the highest risk degree among all of the determined independent predictors. A retrospective observational study conducted by (24) also drew the same conclusion (24). Their study enrolled 5,062 patients with acute coronary syndrome and found that all three levels of malnutrition increased the risk of all-cause mortality after adjusting for confounding factors. Mild, moderate and severe malnutrition increases the risk of all-cause death by 36, 102, and 265%, respectively. In another observational cohort study, included 1,987 patients with stable CAD who underwent elective PCI (25). This study divided patients into two groups according to their CONUT score. One group was nutritionally normal and the other was malnourished which included mild, moderate and severe malnutrition. Findings of this study demonstrated that malnutrition increased the risk of a composite outcome of all-cause death and non-fatal MI by 64%. Although the relative risk of severe malnutrition is the highest, it only occurs in 2.70% of enrolled patients. After considering the prevalence of identified risk factors in the current study, our results indicated that mild malnutrition was associated with 19.49% of the occurrence of long-term all-cause mortality, which was the highest. Mild malnutrition is also the most common type of malnutrition. In the above two studies, mild malnutrition accounted for the largest proportion of malnutrition. Our results show the same phenomenon. Among all the patients, malnutrition accounted for 90.30%, and in the malnutrition, mild malnutrition accounted for 72.46%. Previous studies reported that concentration of LDL-C and total cholesterol decreased during the acute phase of AMI. acute phase of AMI. A prospective observational study involving 1,275 AMI patients, illustrated that total cholesterol and LDL-C decreased 7% and 10% after admission compared to baseline (26). Similar results were also be found in other three studies (27–29). This may lead to lower total cholesterol level in AMI patients, leading to worse nutritional status. However, our study found no effect on results after extra adjustment for AMI.
Nutritional status assessed by the CONUT score which consists of three laboratory markers: albumin, cholesterol and lymphocyte count, also reflects protein and lipid metabolism as well as immune function measured from blood tests (30). Lower albumin levels reflect increased production of catabolic cytokines, muscle catabolism, and appetite suppression, which are associated chronic inflammatory diseases (31). Cholesterol homeostasis occurs as part of innate immune response and its disruption may augment inflammatory responses (32). There are also evidences that cholesterol concentration is associated with the regulation of immune cell function which can improve antitumour activity and activate immune signaling (33–35). Lymphocyte counts reflect the host immunity and have been studied with respect to their association with nutritional status (36). A low lymphocyte percentage was shown to be independently and significantly associated with worse prognosis (37). Failure to recognize or to anticipate the development of malnutrition can increase morbidity and mortality.
Our study also showed that CKD was an independent risk factor of long-term prognosis, which was consistent with previous studies (38, 39). The results indicated that CKD had the second highest PAR for all-cause mortality and was associated with 14.99% of the death cases. Indeed, CKD is one of the major causes of long-term mortality among patients with CAD. Even after adjustment for traditional risk factors of CAD, including age, dyslipidemia, diabetes, and hypertension, the risk of long-term mortality linearly increases with deteriorating kidney function (40, 41). Previous researches have illustrated that as glomerular filtration rate (GFR) declines below 60 to 75 ml/min/1.73 m2, the risk of developing CAD increases linearly (12, 41), especially in those patients with CKD stages G3a to G4 (15-60 ml/min/1.73 m2), which have about double and triple the risk of cardiovascular death, respectively, compared with those in the non-CKD setting. With the decline of GFR, the prevalence of clinical symptoms of CAD increases, along with the prevalence of multivessel coronary artery disease, severe coronary calcification, longer atherosclerotic lesions with greater luminal encroachment, microangiopathy, left ventricular hypertrophy (LVH), and higher plaque burden. Cardiovascular malformation in patients with CKD is associated with identified (e.g., diabetes, dyslipidemia, and hypertension), potential CKD-related risk factors of CAD (e.g., CKD-mineral and bone disorder, anemia, inflammation, and oxidative stress) and the dialysis-associated factors (type, frequency, and pattern of continuous renal replacement therapy). With the continuous deterioration of renal function, the calcification of vessels also increases and is associated with mortality in advanced kidney disease (AKD); both severe calcification of the subintima and media of large vessels are associated with adverse long-term prognosis. Various studies suggest that there are significant opportunities to improve treatment of identified risk factors, and KDIGO guidelines for lipid management in CKD provide specific treatment recommendations (42). Nevertheless, there are several reasons why treatment of established risk factors of CVD is lacking, including weak evidence of effectiveness or extrapolation of evidence from the non-CKD setting. Continued work is required to better understand the interaction between CAD, CKD, and prognosis.
Based on the findings of the current study, CAD patients with well-controlled baseline LDL-C levels still have higher all-cause mortality. According to determined risk factors and their prevalence, malnutrition contributes greatly to the poor prognosis in this population. Among different degrees of malnutrition, mild malnutrition is the most common and is often overlooked by clinicians. However, mild malnutrition contributes the greatest to poor prognosis, which requires clinician's attention and timely intervention. Furthermore, mild malnutrition may be a target in the long-term management of such patients, and new prospective study involving prognosis management in these patients should be conducted to evaluate the appropriate management strategy.
Limitation
There were some limitations to this analysis. First, this study was a retrospective single-center study, so the prevalence of the risk factors may not be representative enough. However, Guangdong Provincial People's Hospital is the largest cardiology center in South China, and PAR can only be calculated based on observational data. Second, data of included patients were limited, without information about body weight, BMI, waist circumference or adiposity, which might help to assess nutritional status comprehensively. However, we chose the CONUT score based on three laboratory indicators as a nutritional status assessment tool. This may also objectively evaluate the nutritional status of the patient. Third, LDL-C level and total concentration decrease at the acute phase of AMI. Thus, LDL-C value and total cholesterol value before admission or later after admission may be more suitable for AMI patients. However, our study lacks such data. Finally, since this was an observational study, we can only suggest that modifying these risk factors may lead to a lower risk of long-term all-cause mortality rather than prove it. The value our results add is in providing these cost-effective targets for further intervention trials.
Conclusion
Malnutrition, age ≥75 years, CHF, CKD and atrial fibrillation were independent predictors for long-term all-cause mortality in CAD patients with well-controlled LDL-C levels. Severe malnutrition had the highest HR. Considering the prevalence of these risk factors, mild malnutrition contributed the greatest to the long-term all-cause mortality. More attention should be paid to mild malnutrition among patients with CAD and well-controlled LDL-C level (<1.8 mmol/L).
Data Availability Statement
The datasets analyzed during the current study will be available from the corresponding author on a reasonable request when the study is finished. Requests to access the datasets should be directed to the corresponding author, Yong Liu, liuyong@gdph.org.cn.
Ethics Statement
The studies involving human participants were reviewed and approved by Research Ethics Committee of Guangdong Provincial People's Hospital, Guangdong Academy of Medical Sciences (No. GDREC2019555H[R1]). Written informed consent for participation was not required for this study in accordance with the national legislation and the institutional requirements.
Author Contributions
YL and SC had full access to data and took responsibility for the data analyses integrity and accuracy. YL and BW: concept and design. YoY: data management. BW, ZG, FL, HL, ZM, MY, YaY, QL, HH, and WW: drafting of the manuscript. JC, YZ, and SD: critical revision. NT and YL: final approval to publish. All authors contributed to acquisition, analyses and interpretation of data, and read and approved the final manuscript.
Funding
This study was supported by the National Key Research and Development Program of China (Grant no. 2016YFC1301202), the Beijing Lisheng Cardiovascular Health Foundation (LHJJ20141751), Guangdong Provincial Science and Technology Plan Project (2017B030314041) and Guangdong Provincial People's Hospital Dengfeng Project Fund (DFJH201919 and DFJH2020026).
Conflict of Interest
The authors declare that the research was conducted in the absence of any commercial or financial relationships that could be construed as a potential conflict of interest.
Publisher's Note
All claims expressed in this article are solely those of the authors and do not necessarily represent those of their affiliated organizations, or those of the publisher, the editors and the reviewers. Any product that may be evaluated in this article, or claim that may be made by its manufacturer, is not guaranteed or endorsed by the publisher.
Acknowledgments
We acknowledge Jingru Deng and Liangguang Meng in Guangdong Provincial Key Laboratory of Coronary Heart Disease Prevention for help in data extraction and verification.
Supplementary Material
The Supplementary Material for this article can be found online at: https://www.frontiersin.org/articles/10.3389/fnut.2021.725537/full#supplementary-material
Abbreviations
LDL-C, low-density lipoprotein cholesterol; CAD, coronary artery disease; CAG, coronary angiography; PCI, percutaneous coronary intervention; AMI, acute myocardial infarction; CHF, congestive heart failure; CKD, chronic kidney disease; COPD, chronic obstructive pulmonary disease; HR, hazard ratio; CI, confidence interval.
References
1. Mach F, Baigent C, Catapano AL, Koskinas KC, Casula M, Badimon L, et al. 2019 ESC/EAS guidelines for the management of dyslipidaemias: lipid modification to reduce cardiovascular risk. Eur Heart J. (2020) 411:111–88. doi: 10.15829/1560-4071-2020-3826
2. Baigent C, Blackwell L, Emberson J, Holland Le, Reith C, Bhala N, et al. Efficacy and safety of more intensive lowering of LDL cholesterol: a meta-analysis of data from 170,000 participants in 26 randomised trials. Lancet. (2010) 376:1670–81. doi: 10.1016/s0140-6736(10)61350-5
3. Giugliano RP, Desai NR, Kohli P, Rogers WJ, Somaratne R, Huang F, et al. Efficacy, safety, and tolerability of a monoclonal antibody to proprotein convertase subtilisin/kexin type 9 in combination with a statin in patients with hypercholesterolaemia (laplace-timi 57): a randomised, placebo-controlled, dose-ranging, phase 2 study. Lancet. (2012) 380:2007–17. doi: 10.1016/s0140-6736(12)61770-x
4. Ai M, Otokozawa S, Asztalos BF, Ito Y, Nakajima K, White CC, et al. Small dense ldl cholesterol and coronary heart disease: results from the framingham offspring study. Clin Chem. (2010) 56:967–76. doi: 10.1373/clinchem.2009.137489
5. Lim TS, Yun JS, Cha SA, Song KH, Yoo KD, Ahn YB, et al. Elevated lipoprotein(a) levels predict cardiovascular disease in type 2 diabetes mellitus: a 10-year prospective cohort study. Korean J Intern Med. (2016) 31:1110–9. doi: 10.3904/kjim.2016.030
6. Wang B, Liu J, Chen S, Ying M, Chen G, Liu L, et al. Malnutrition affects cholesterol paradox in coronary artery disease: a 41,229 chinese cohort study. Lipids Health Dis. (2021) 20:36. doi: 10.21203/rs.3.rs-266119/v1
7. Buchbinder SP, Glidden DV, Liu AY, Mcmahan V, Guanira JV, Mayer KH, et al. HIV pre-exposure prophylaxis in men who have sex with men and transgender women: a secondary analysis of a phase 3 randomised controlled efficacy trial. Lancet Infect Dis. (2014) 14:468–75. doi: 10.1016/s1473-3099(14)70025-8
8. Kushner FG, Hand M, Smith SC, King SB, Anderson JL, Antman EM, et al. 2009 focused updates: ACC/AHA guidelines for the management of patients with ST-elevation myocardial infarction (updating the 2004 guideline and 2007 focused update) and ACC/AHA/SCAI guidelines on percutaneous coronary intervention (updating the 2005 guideline and 2007 focused update) a report of the american college of cardiology foundation/American Heart Association task force on practice guidelines. J Am Coll Cardiol. (2009) 5423:2205–41. doi: 10.1002/ccd.22351
9. Jneid H, Anderson JL, Wright RS, Adams CD, Bridges CR, Casey DE, et al. 2012 ACCF/AHA focused update of the guideline for the management of patients with unstable angina/non-ST-elevation myocardial infarction (updating the 2007 guideline and replacing the 2011 focused update): a report of the American college of cardiology foundation/American Heart Association task force on practice guidelines. J AM COLL CARDIOL. (2012) 607:645–81. doi: 10.1016/j.jacc.2012.06.004
10. Levine GN, Bates ER, Blankenship JC, Bailey SR, Bittl JA, Cercek B, et al. 2015 ACC/AHA/SCAI focused update on primary percutaneous coronary intervention for patients with ST-elevation myocardial infarction: an update of the 2011 ACCF/AHA/SCAI guideline for percutaneous coronary intervention and the 2013 ACCF/AHA guideline for the management of ST-elevation myocardial infarction. J Am Coll Cardiol. (2016) 67:1235–50. doi: 10.1016/j.jacc.2015.10.005
11. Mehran R, Aymong ED, Nikolsky E, Lasic Z, Iakovou I, Fahy M, et al. A simple risk score for prediction of contrast-induced nephropathy after percutaneous coronary intervention: development and initial validation. J Am Coll Cardiol. (2004) 447:1393–9. doi: 10.1016/s0735-1097(04)01445-7
12. Manjunath G, Tighiouart H, Ibrahim H, Macleod B, Salem DN, Griffith JL, et al. Level of kidney function as a risk factor for atherosclerotic cardiovascular outcomes in the community. J Am Coll Cardiol. (2003) 41:47–55. doi: 10.1016/s0735-1097(02)02663-3
13. National Kidney Foundation. K/DOQI clinical practice guidelines for chronic kidney disease: evaluation, classification, and stratification. Am J Kidney Dis. (2002) 39:S1–266.
14. Levey AS, Bosch JP, Lewis JB, Greene T, Rogers N, Roth D. A more accurate method to estimate glomerular filtration rate from serum creatinine: a new prediction equation. modification of diet in renal disease study group. Ann intern med. (1999) 130:461–70. doi: 10.7326/0003-4819-130-6-199903160-00002
15. Nutritional anaemias. report of a WHO scientific group. world health organ tech rep ser. (1968) 405:5–37.
16. Ignacio De. Ulíbarri J, González-Madroño A, De Villar NG, González P, González B, et al. CONUT: a tool for controlling nutritional status first validation in a hospital population. Nutr Hosp. (2005) 20:38–45.
17. Willey JZ, Moon YP, Kahn E, Rodriguez CJ, Rundek T, Cheung K, et al. Population attributable risks of hypertension and diabetes for cardiovascular disease and stroke in the Northern Manhattan study. J Am Heart Assoc. (2014) 3:e001106. doi: 10.1161/jaha.114.001106
18. Van Bokhorst-De Van Der Schueren Ma, Guaitoli PR, Jansma EP, De Vet HCW. Nutrition screening tools: does one size fit all? a systematic review of screening tools for the hospital setting. Clin Nutr. (2014) 33:39–58. doi: 10.1016/j.clnu.2013.04.008
19. Baker JP, Detsky AS, Wesson DE, Wolman SL, Stewart S, Whitewell J, et al. Nutritional assessment: a comparison of clinical judgement and objective measurements. N Engl J Med. (1982) 306:969–72. doi: 10.1056/nejm198204223061606
20. Guigoz Y, Vellas B, Garry PJ. Assessing the nutritional status of the elderly: the mini nutritional assessment as part of the geriatric evaluation. Nutr Rev. (1996) 54:S59–65. doi: 10.1111/j.1753-4887.1996.tb03793.x
21. Wolinsky FD, Coe RM, Mcintosh WA, Kubena KS, Prendergast JM, Chavez MN, et al. Progress in the development of a nutritional risk index. J Nutr. (1990) 120:1549–53. doi: 10.1093/jn/120.suppl_11.1549
22. Buzby GP, Mullen JL, Matthews DC, Hobbs CL, Rosato EF. Prognostic nutritional index in gastrointestinal surgery. Am J Surg. (1980) 1391:160–7. doi: 10.1016/0002-9610(80)90246-9
23. Bouillanne O, Morineau G, Dupont C, Coulombel I, Vincent JP, Nicolis I, et al. Geriatric nutritional risk index: a new index for evaluating at-risk elderly medical patients. Am J Clin Nutr. (2005) 824:777–83. doi: 10.1093/ajcn/82.4.777
24. Roubín SR, Assi EA, Fernandez MC, Pardal CB, Castro AL, Parada JA, et al. Prevalence and prognostic significance of malnutrition in patients with acute coronary syndrome. J Am Coll Cardiol. (2020) 767:828–40. doi: 10.1016/j.jacc.2020.06.058
25. Wada H, Dohi T, Miyauchi K, Doi S, Konishi H, Naito R, et al. Prognostic impact of nutritional status assessed by the controlling nutritional status score in patients with stable coronary artery disease undergoing percutaneous coronary intervention. Clin Res Cardiol. (2017) 106:875–83. doi: 10.1007/s00392-017-1132-z
26. Fresco C, Maggioni AP, Signorini S, Merlini PA, Mocarelli P, Fabbri G, et al. Variations in lipoprotein levels after myocardial infarction and unstable angina: the LATIN trial. Ital Heart J. (2002) 3:587–92.
27. Brugada R, Wenger NK, Jacobson TA, Clark WS, Cotsonis G, Iglesias A. Changes in plasma cholesterol levels after hospitalization for acute coronary events. Cardiology. (1996) 87:194–9. doi: 10.1159/000177086
28. Pitt B, Loscalzo J, Ycas J, Raichlen JS. Lipid levels after acute coronary syndromes. J Am Coll Cardiol. (2008) 51:1440–5. doi: 10.1016/j.jacc.2007.11.075
29. Shrivastava AK, Singh HV, Raizada A, Singh SK. Serial measurement of lipid profile and inflammatory markers in patients with acute myocardial infarction. Excli J. (2015) 14:517–26. doi: 10.17179/excli2014-671
30. Chen SC, Yang YL, Wu CH, Huang SS, Chan WL, Lin SJ, et al. Association between preoperative nutritional status and clinical outcomes of patients with coronary artery disease undergoing percutaneous coronary intervention. Nutrients. (2020) 12:1295. doi: 10.3390/nu12051295
31. Merker M, Felder M, Gueissaz L, Bolliger R, Tribolet P, Kägi-Braun N, et al. Association of baseline inflammation with effectiveness of nutritional support among patients with disease-related malnutrition: a secondary analysis of a randomized clinical trial. JAMA Netw Open. (2020) 3:e200663. doi: 10.1001/jamanetworkopen.2020.0663
32. Tall AR, Yvan-Charvet L. Cholesterol, inflammation and innate immunity. Nat Rev Immunol. (2015) 15:104–16. doi: 10.1038/nri3793
33. Míguez MJ, Rosenberg R, Burbano X, Malow R. Cholesterol as a mediator of alcohol-induced risks for respiratory disease hospitalizations among people living with HIV. J AIDS Clin Res. (2011) Suppl 1:001. doi: 10.4172/2155-6113.S1-001
34. Yang W, Bai Y, Xiong Y, Zhang J, Chen S, Zheng X, et al. Potentiating the antitumour response of cd8(+) T cells by modulating cholesterol metabolism. Nature. (2016) 531:651–5. doi: 10.1038/nature17412
35. Yasumasu T, Takahara K, Sadayasu T, Date H, Isozumi K, Kouzuma R, et al. Effect of plasma lipoproteins on natural killer cell activity in the elderly population. J Gerontol A Biol Sci Med Sci. (2003) 58:561–5. doi: 10.1093/gerona/58.6.m561
36. Leandro-Merhi VA, Bráz VN, Aquino JL. Is total lymphocyte count related to nutritional markers in hospitalized older adults? Arq Gastroenterol. (2017) 54:79–82. doi: 10.1590/s0004-2803.2017v54n1-16
37. Bian C, Wu Y, Shi Y, Xu G, Wang J, Xiang M, et al. Predictive value of the relative lymphocyte count in coronary heart disease. Heart Vessels. (2010) 25:469–73. doi: 10.1007/s00380-010-0010-7
38. Gansevoort RT, Correa-Rotter R, Hemmelgarn BR, Jafar TH, Heerspink HJ, Mann JF, et al. Chronic kidney disease and cardiovascular risk: epidemiology, mechanisms, and prevention. Lancet. (2013) 382:339–52. doi: 10.1016/s0140-6736(13)60595-4
39. Sarnak MJ, Amann K, Bangalore S, Cavalcante JL, Charytan DM, Craig JC, et al. Chronic kidney disease and coronary artery disease: JACC state-of-the-art review. J Am Coll Cardiol. (2019) 74:1823–38. doi: 10.1016/j.jacc.2019.08.1017
40. Go AS, Chertow GM, Fan D, Mcculloch CE, Hsu CY. Chronic kidney disease and the risks of death, cardiovascular events, and hospitalization. N Engl J Med. (2004) 351:1296–305. doi: 10.1056/nejmoa041031
41. Matsushita K, Van Der Velde M, Astor BC, Woodward M, Levey AS, De Jong PE, et al. Association of estimated glomerular filtration rate and albuminuria with all-cause and cardiovascular mortality in general population cohorts: a collaborative meta-analysis. Lancet. (2010) 375:2073–81. doi: 10.1016/s0140-6736(10)60674-5
Keywords: mild nutrition, low-density lipoprotein cholesterol, coronary artery disease, long-term all-cause mortality, risk factor, population attributable risk
Citation: Wang B, Guo Z, Liu J, Li H, Mai Z, Lin F, Ying M, Yu Y, Chen S, Li Q, Huang H, Wei W, Yang Y, Dong S, Zhou Y, Chen J, Tan N and Liu Y (2021) Mild Malnutrition Contributes the Greatest to the Poor Prognosis in Coronary Artery Disease With Well-Controlled Low-Density Lipoprotein Cholesterol Levels: A 4,863 Chinese Cohort Study. Front. Nutr. 8:725537. doi: 10.3389/fnut.2021.725537
Received: 15 June 2021; Accepted: 06 September 2021;
Published: 29 September 2021.
Edited by:
NADA ROTOVNIK KOZJEK, Institute of Oncology Ljubljana, SloveniaReviewed by:
Leah Gramlich, University of Alberta, CanadaIchiro Sakuma, Hokko Memorial Hospital, Japan
Copyright © 2021 Wang, Guo, Liu, Li, Mai, Lin, Ying, Yu, Chen, Li, Huang, Wei, Yang, Dong, Zhou, Chen, Tan and Liu. This is an open-access article distributed under the terms of the Creative Commons Attribution License (CC BY). The use, distribution or reproduction in other forums is permitted, provided the original author(s) and the copyright owner(s) are credited and that the original publication in this journal is cited, in accordance with accepted academic practice. No use, distribution or reproduction is permitted which does not comply with these terms.
*Correspondence: Yong Liu, liuyong@gdph.org.cn; Ning Tan, tanning100@126.com
†These authors have contributed equally to this work