- 1Department of Clinical Epidemiology & Clinical Trial Unit (CTU), Children's Hospital of Fudan University, National Children's Medical Center, Shanghai, China
- 2International Peace Maternity and Child Health Hospital of China Welfare Institute, Shanghai, China
Background and Aim: Serum bilirubin levels are recently shown to be a novel protector of gestational diabetes mellitus (GDM), yet whether they could be affected by carbohydrate quality is unclear. We aimed to examine the associations between dietary carbohydrate parameters and serum bilirubin levels during early pregnancy, with further exploration on a potential mediating role of serum bilirubin levels on carbohydrate parameters-GDM pathways.
Methods: 260 healthy but overweight or obese gravidae (BMI ≥24 kg/m2) derived from a historical cohort in two hospitals in China were included. The associations between carbohydrate parameters (total carbohydrate intake, glycemic index GI, fiber intake, glycemic load GL) and serum bilirubin levels (total bilirubin, TB and direct bilirubin, DB) and GDM were evaluated by multivariable regression analysis. Generalized structural equation modeling was then applied to perform adjusted mediation analysis.
Results: Increased serum bilirubin levels (mmol/L) and decreased GDM occurrence were observed following dietary carbohydrate intake (%E) and GL (g/1,000 kcal) in highest tertile compared to the lowest tertile [carbohydrate: TB: β = 0.926 (95%CI: 0.069, 1.782), DB: β = 0.554 (95%CI: 0.192, 0.916);GL:TB: β = 1.170 (95%CI: 0.339, 2.001); DB: β = 0.369 (95%CI: 0.016, 0.700); carbohydrate: adjusted OR = 0.43 (95%CI:0.19–0.99); GL: adjusted OR = 0.36 (95%CI:0.16, 0.84)]. The mediating effect of carbohydrate intake and GL on GDM through bilirubin levels was evaluated as modest (carbohydrate: 6.2% for TB, 1.3% for DB; GL: 8.7% for TB, 2.3% for DB). No association was observed regarding GI and fiber.
Conclusions: Mildly elevated serum bilirubin levels appeared to be in response to higher energies consumed from carbohydrate during early pregnancy in healthy overweight or obese gravidae. However, the mediating effect of bilirubin levels on carbohydrate-GDM pathways is not evident. Larger investigation is further needed for solid evidence.
Introduction
Parallel to the rise in obesity and maternal age, the prevalence of gestational diabetes mellitus (GDM) is increasing worldwide, affecting up to 18% pregnancies globally and approximately 10% in China during last decade (1–3). Since early pregnancy, the alterations in maternal metabolism facilitate peripheral insulin resistance in gravidae, especially those with high pre-pregnancy BMI, and thereby at a higher risk of GDM (4). Therefore, identifying early indicators in GDM pathogenesis should be beneficial in disease prevention in this at-risk population.
Previous experimental evidence supported a protective role of heme catabolic pathways in pathogenesis of diabetes mellitus (5). Bilirubin, as the end product of heme catabolism in the systemic circulation, were recently found to have salutary effects on prevention of diabetes mellitus (6) through anti-oxidative cytoprotective properties, antioxidant actions, and anti-inflammatory effects (5). Mildly elevated serum bilirubin has been demonstrated to inhibit lipoprotein oxidation and up-regulation of several pro-inflammatory biomarkers such as endothelial adhesion molecules and c-reactive protein (5, 7–9). A prospective cohort study of nearly 3,000 gravidae reported that women with higher serum direct bilirubin levels during mid- pregnancy have lower GDM risk (10).
According to several reviews, chronic inflammation and oxidation underlies the pathogenesis of insulin resistance, which could be altered by dietary macronutrients, and dietary carbohydrate quality is one of them (11–13). Yet, as a novel protector of GDM, whether serum bilirubin levels in response to carbohydrate parameters is unknown. Since carbohydrate is the cornerstone in GDM diets, it is possible that bilirubin predisposes GDM through similar mechanisms, which could be modified by different carbohydrate quality. However, this has not been explored.
This study was whereby conducted, with the aim to examine if bilirubin could be affected by carbohydrate quality, and further explore if bilirubin is a potential mediator in the carbohydrate quality-GDM pathway. The findings from this study will help provide a novel mechanistic insight into the pathogenesis of GDM influenced by carbohydrate parameters and bilirubin. In addition, the results will be helpful in identifying early indicator of GDM, especially for dietary modification in overweight and obese gravidae, who are greatly predisposed to GDM.
Materials and Methods
The Cohort
This study was a secondary data analysis using a historical cohort (ClinicalTrials.gov registry: NCT01628835). Details of the previous study design, recruitment, methods and responses could be found elsewhere (14). Briefly, overweight or obese gravidae [BMI ≥24 kg/m2 according to national consensus of BMI cut-off for Chinese adults (15)] aged 20–45 years old were recruited at first antenatal visit ≤ 14 gestational weeks in two hospitals [Kunshan Maternity and Child Care Center (Kunshan city, Jiangsu province, China) and the International Peace Maternity and Child Health Hospital of China Welfare Institute (Shanghai, China)] from June 2012 to October 2015. After excluding those with multiple pregnancy, artificial impregnation, special diets (e.g., vegans) and diagnosed chronic diseases (a history of hypertension, diabetes, heart diseases, mental disorders), 400 gravidae were eligible for follow-up till delivery. In the previous research, participants were randomly allocated to receive either standard (n = 200) or individualized dietary counseling (n = 200) throughout the pregnancy. The researchers found two counseling approaches received similar effectiveness on maternal and neonatal outcomes (including GDM, macronutrient intakes, and insulin or glucose levels). All participants provided written informed consent before baseline data collection.
Dietary Assessment
Dietary intake was assessed by 24-h dietary recall. Trained clinical dieticians asked participants to report their food intake in one recent weekday before the visit, which best reflected their regular dietary habit. Portion-size food molds were used to assess the serving sizes. Because carbohydrate quality was the principal consideration when matching a particular food with one in food lists, nutrient intakes were calculated primarily based on Chinese nutrient composition tables with published GI values of over 200 food items (16, 17). If the food was not available in the Chinese food lists, the food assignment was further updated to incorporate newly published GI values from international standards (18–20). Daily intakes of total carbohydrate (g) and fiber (g) were whereby calculated. Dietary GI was averaged during daily meals. The value of carbohydrate intake (g) of each consumed food was then multiplied by the respective GI to obtain the daily glycemic load (GL) (18).
The carbohydrate parameters (total carbohydrate intake, fiber intake, dietary GI, GL) were converted to relative intakes adjusted by total energy intake using residual nutrient density models (21). Total carbohydrate intake was computed as percentage of energy (%E) by using the following equation: Total carbohydrate intake(%E) = (total carbohydrate intake (g) × 4) ÷ total energy intake (kcal) × 100%. Fiber intake and GL were computed as grams for every 1,000 kcal. Validation of relative intakes among dietary parameters was assessed by intercorrelations (see Supplementary Table 1). We also grouped participants into tertiles by intakes of total carbohydrate, fiber, and GL. Dietary GI was grouped into three levels (low GI: ≤ 55, medium GI: 55–70, high GI: ≥70) according to international consensus (22).
Non-Dietary Assessment
At first antenatal visit, demographic characteristics and gestational lifestyle factors were collected through self-reported questionnaire in all participants. Anthropometric measurements and blood samples were collected at the same visit. BMI was calculated as weight (kg)/height (m)2. The gestational week was estimated from self-reported last menstrual period and corrected by the first routine ultrasound examinations. GDM was defined based on the routine 75 g oral glucose tolerance test (OGTT) screening mostly at 24 gestation weeks (few deferred but not later than 28 gestational weeks) according to international standards (23, 24). Baseline laboratory data was directly extracted from the hospital information system, which included serum bilirubin, fasting plasma glucose, serum insulin, lipid profiles, glycosylated hemoglobin (HbA1c), and blood pressure. The homeostasis model assessment of insulin resistance (HOMA-IR) was calculated as follows: [fasting plasma glucose (mmol/L)] × (fasting insulin [mIU/L])/22.5 (25). Gestational weight gain was calculated as body weight (kg) difference between baseline and antenatal visit at 24 gestational weeks.
Participants Included in This Secondary Study
In this secondary study, we reused the pre-existing data of the same cohort. The baseline dietary data and OGTT results were available in all 400 subjects. We further excluded those without bilirubin measurements (n = 130), implausible dietary intake in regards to total energy intake (<500 or >3,500 kcal/day) and prescribed medication use since 3 months before pregnancy. As a result, a total of 260 participants were included.
Statistical Analysis
Characteristics of study participants were expressed as the mean (standard deviation) for normally distributed variables, median (inter-quartile range) for skewed variables and frequency with percentage for categorical variables. Group comparisons were performed by Bonferroni multiple-comparison test, Kruskal-Wallis test and χ2-tests, respectively.
We firstly examined two paths (path a: carbohydrate parameter-bilirubin; path c: carbohydrate parameter-GDM, see Figure 1) separately by multivariable regression analysis, which were in line with the first two conditions in Baron & Kenny's four-step sequential verification models (26, 27). Carbohydrate parameters were treated as continuous or in groups. Bilirubin were serum total bilirubin (TB) and direct bilirubin (DB) levels (mmol/L).
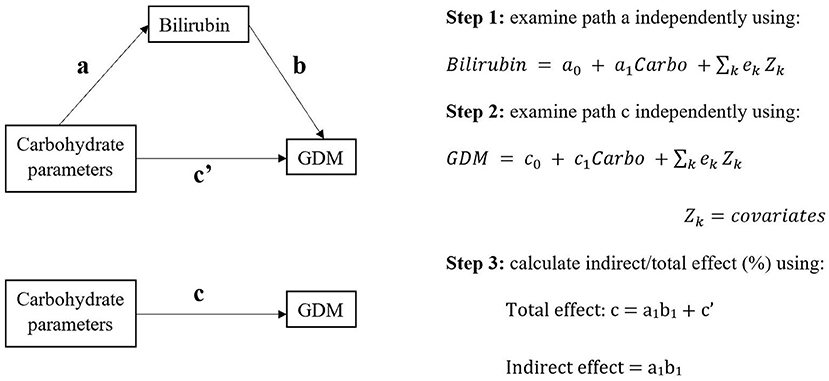
Figure 1. The study design of potential causal pathways among carbohydrate parameters, bilirubin, and gestational diabetes mellitus (GDM).
Covariates were selected as a priori based on previous literatures (2, 5, 28–31) and the authors' background knowledge, which included gestational week, maternal age (years), total energy intake (kcal), pregnancy alcohol drinking (yes, no), baseline BMI (kg/m2), baseline fasting plasma glucose (mmol/L), and triglycerides (mmol/L). Cohort-specific covariates (hospital and dietary counseling) were also included. Total carbohydrate intake, fiber intake, and dietary GI were adjusted from the other two in order to minimize interference among carbohydrate parameters. Pregnancy smoking, maternal education, and household income were not included in the analysis due to highly missing values (>50%). Physical activity was not available for analysis because of qualitative design.
A potential association was defined as 95% confidence interval of estimates beyond null after adjusting for the abovementioned covariates. If the two paths were consistent for the same carbohydrate parameter, generalized structural equation modeling (GSEM) would be then applied to determine total and indirect effect of carbohydrate parameter on GDM through bilirubin. The GSEM model constructed three paths (exposure, mediator, and outcome) simultaneously with adjustment of same covariates as before. GSEM calculated total and indirect effect based on Baron and Kenny's mediation model [(26); Figure 1]. The proportion of mediating effect was calculated as indirect effect/total effect × 100%.
To examine the robustness of the observed associations, we also compared baseline metabolic status of mothers (see Supplementary Table 2) and further adjusted for other well-known risk factors of GDM (2, 28, 29, 31) in sensitivity analysis. This included parity (0, 1, ≥2), folic acid and vitamin supplement since 3 months pre-pregnancy till baseline (folic acid + vitamin, folic acid only, vitamin only, no, or unknown), family history of diabetes or hypertension (yes, no), gestational weight gain (kg), and gestational hypertension (yes, no).
The data analysis was performed with STATA (Version 15.1, StataCorp L.P., and College Station, TX). The significance level for Bonferroni, Kruskal-Wallis, χ2-tests and GSEM was set at two-sided p < 0.05.
Results
Characteristics of Study Population
Characteristics of study population are summarized in Table 1. At baseline, the participants reported average intakes of 1,485 (±471) kcal total energy, with 54.2 (±12.7) %E total carbohydrate and 88.4 (±26.2) g/1,000 kcal GL. Fiber intake was 6.2 g/1,000 kcal (25th:4.7, 75th:8.4). Daily GI was 66.1 (25th:60.6, 75th:70.3). Baseline serum TB was 9.4 (±2.9) mmol/L. Baseline serum DB was 3.1 (±1.2) mmol/L. 57 (21.9%) participants developed GDM.
When carbohydrate parameters were grouped in Table 2, most maternal characteristics except gestational hypertension were generally balanced across different levels of carbohydrate parameters. Lower bilirubin levels were observed in those having lower GI foods compared to high GI foods. We also found that both serum bilirubin levels increased as carbohydrate intake (%E) increased by tertiles.
Carbohydrate Parameters and Serum Bilirubin Levels
Serum bilirubin levels were positively associated with total carbohydrate intake (%E) and GL (g/1,000 kcal) (Table 3) at baseline after adjusting for covariates. TB increased 0.030 (95%CI: 0.002, 0.058) mmol/L for every 1%E increase in total carbohydrate while 0.014 (95%CI: 0.002, 0.026) mmol/L elevation for DB correspondingly. The magnitudes of coefficients were amplified when total carbohydrate intake was grouped into tertiles. There were 0.865 mmol/L (95%CI: 0.009, 1.721) and 0.926 mmol/L (95%CI: 0.069, 1.782) increase in TB for tertile 2 and 3, respectively, compared to the lowest tertile. Similarly, DB increased 0.303 (95%CI: −0.058, 0.665) mmol/L in tertile 2 and 0.554 (95%CI: 0.192, 0.916) mmol/L in tertile 3 compared to the lowest tertile. Dietary GL showed similar trends. Bilirubin levels rose as GL increased by 1 g/1,000 kcal [TB: 0.021 (95%CI: 0.008, 0.034); DB: 0.006 (95%CI: 0.001, 0.010)] as well as in the highest GL tertile [TB: 1.170 (95%CI: 0.339, 2.001), DB: 0.369 (95%CI: 0.016, 0.700)] compared to the lowest tertile. Conversely, we did not find the association between dietary GI or fiber with bilirubin levels, based on estimates with wide 95%CI in either direction (Table 3).
The sensitivity analysis of further adjustment on risk factors of GDM, showed attenuated but consistent associations (see Supplementary Table 3).
Carbohydrate Parameters and GDM
As shown in Figure 2, total carbohydrate intake (%E) and GL (g/1,000 kcal) at baseline was inversely associated with GDM onset. The associations became stronger in the highest tertile compared to the lowest tertile [carbohydrate: adjusted OR: 0.43 (95%CI: 0.19, 0.99); GL: adjusted OR: 0.38 (95%CI: 0.17, 0.86)]. We found no association between fiber intake or GI on later GDM occurrence, either in continuous scale or in categories, given a wide 95%CI containing null value (Figure 2).
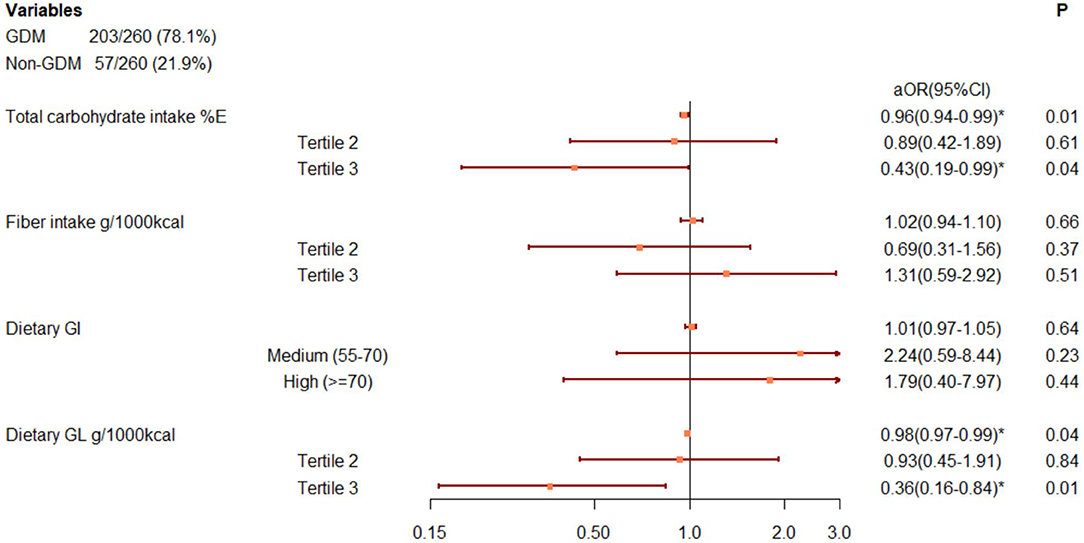
Figure 2. Associations between carbohydrate parameters and GDM onset, after adjusting for pregnancy alcohol drinking, baseline BMI, gestational week, maternal age, total energy intake, dietary counseling, hospital, baseline fasting glucose, and triglyceride levels. Adjustment within carbohydrate parameters: carbohydrate: fiber and GI, fiber: carbohydrate and GI; GI: carbohydrate and fiber. *Adjusted odds ratio with 95%CI beyond null. %E, percentage of total energy, d, day, GI, glycemic index, GL, glycemic load, GDM, gestational diabetes mellitus.
The sensitivity analysis of further adjustment on risk factors of GDM showed attenuated but consistent results (see Supplementary Table 4).
Mediation Analysis
The results of mediation analysis for carbohydrate/GL-TB/DB-GDM pathways were presented in Tables 4, 5. The potential effect of carbohydrate or GL, either in total or indirectly through bilirubin levels, were inversely associated with GDM onset since all estimates were skewed to negative values. Yet, whereas the carbohydrate and GL had significant total effects on GDM, the mediating effects of TB and DB were not significant. The proportions of indirect effects showed that around 6.2% of the effect of carbohydrate intake (%E) on GDM was mediated by TB level, while 1.3% coming from DB level. Nearly 8.7% of the effect of GL (g/1,000 kcal) on GDM was indirectly through TB level and 2.3% through DB level. When carbohydrate and GL was measured by tertiles, the proportion of indirect effect increased for carbohydrate, as 8.3% through TB and 2.0% through DB in the pathways. GL tertiles showed similar proportion of indirect effect as GL in g/1,000 kcal (Table 5).
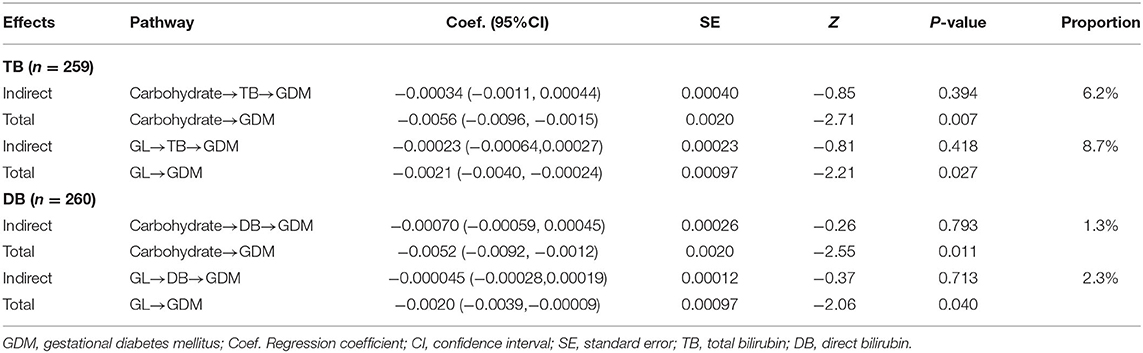
Table 4. Effect of carbohydrate intake and GL on GDM in total and indirectly through bilirubin levels.
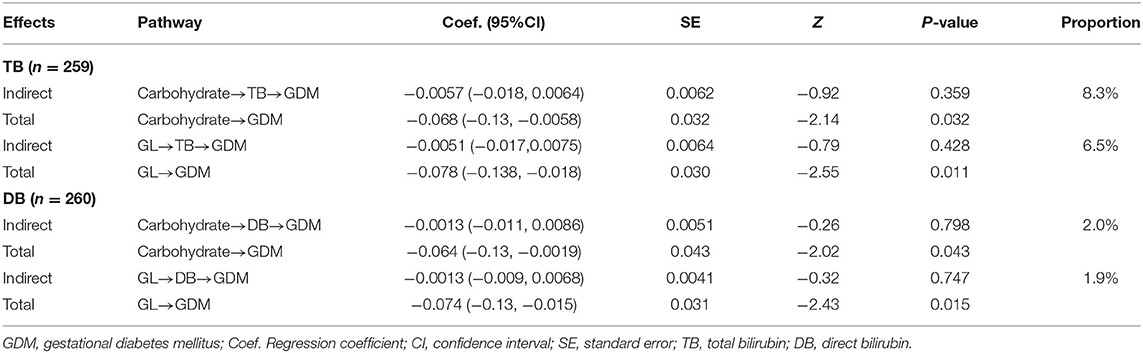
Table 5. Effect of carbohydrate and GL tertiles on GDM in total and indirectly though bilirubin levels.
Discussion
Overall, this study reused a pre-existing data of overweight and obese gravidae, with initial exploration on associations of serum bilirubin levels with carbohydrate quality, and indirect effect of carbohydrate intake (mediated through bilirubin) on GDM. Participants consuming higher total carbohydrate intake (%E) and dietary GL (g/1,000 kcal) in early pregnancy appeared to have higher serum bilirubin levels and were less likely to suffer from GDM. Meanwhile, bilirubin levels were hypothesized as a potential mediator in the association between carbohydrate/GL and GDM. However, the mediation analysis suggested the indirect effect through bilirubin was minimal compared to direct effect.
Carbohydrate-rich diets have been expected to increase diabetes risk by directly facilitating post-prandial glucose concentrations (32). However, mounting evidence supports carbohydrate restriction at the cost of increasing calories from fat intake as less optimal according to recent reviews (33, 34). Although limiting carbohydrate helps control glycemia, substituting fat for carbohydrate in obese women with pre-pregnancy insulin resistance may promote intrauterine overnutrition (33–35). Furthermore, diets high in fat may promote insulin resistance partially through elevation of free fatty acid (FFAs), which concomitantly impaired insulin signaling (36). These underlying mechanisms may explain the direct effect of carbohydrate intake (%E) on GDM. In our data, we observed a high intercorrelation between carbohydrate intake (%E) and fat intake (%E) (r = −0.93, Supplementary Table 1). While we were not sure about the reason for the highly negative correlation, the observed associations might be explained by replacement of fat intake, which were in line with previous results from observational studies and clinical trials, that high-carbohydrate with low-fat diets led to decreased risk of GDM and lower fasting glucose in GDM patients (28, 31, 37–40).
Bilirubin has been recognized as a product of heme catabolism with potent antioxidant and anti-inflammatory properties (5). Given a potential link between different carbohydrate parameters with alteration on inflammatory markers and oxidative indicators (41–43), we believe bilirubin can be modified by different carbohydrate quality. In this study, we are the first that found both serum total and direct bilirubin levels increased mildly following higher energies from total carbohydrate intake in overweight and obese gravidae. Although limited by the absence of previous evidence, we consider the directions of the associations as reasonable since replacement of fat (%E) with carbohydrate (%E) has shown potential antioxidative and anti-inflammatory effects (44–47). Despite this, whether bilirubin was directly induced by carbohydrate intake or regulated through other biomarkers or key products in heme catabolism still needs further exploration.
However, mediation analysis by GSEM indicated only marginal non-significant effect of bilirubin levels involving in the carbohydrate-GDM association. This is probably because the total effect of carbohydrate on GDM was already mild. As a consequence, it precluded obvious mediating effect to be detected from statistical models, and further masked if different mediators existed and produced opposite effects. As bilirubin was a potentially negative mediator, its effect could be partially neutralized by other mediators that increased GDM, particularly in longitudinal studies (48). Given that the mediated relationship is an intrinsically causal relationship (26), this observational design only provided conservative estimations that should be interpreted with caution. If the observed mediation was true, the proportions of indirect effect suggested around 8% in the association was contributed by TB level and <3% was responsible for DB level. Because DB was a subset of TB (49), it might explain the difference in magnitude and indicate that the mediating effect was through overall bilirubin metabolism rather than bilirubin subtypes. Further exploration with a wide coverage of biomarkers involved in bilirubin homeostasis or heme catabolism might help provide a bigger picture.
In our study, dietary GL showed similar results as carbohydrate. The observed association might be explained by the intercorrelation that the dietary GL in this study was affected mainly by carbohydrate intake rather than GI (r= 0.86 with carbohydrate and 0.61 with GI, Supplementary Table 1). This led to limited implication for GL in terms of its comprehensive effect accounting for both carbohydrate quantity and quality. Even so, we still observed higher proportions of indirect effect of bilirubin levels for GL compared to carbohydrate intake. This gap was narrowed regarding tertiles of carbohydrate and GL. These suggested that bilirubin response on GL-GDM and carbohydrate-GDM pathways was seemingly different. This hypothesis should be investigated in a future study.
In addition, we failed to detect an association regarding either GI or fiber. The discrepancies were possibly due to the high skewness in GI and fiber intake in our study, which impeded comparison between extreme levels and underestimated the associations. Besides, as low-GI foods often have high fiber content (34), the adjustment within carbohydrate parameters (i.e. carbohydrate intake, GI, fiber) in the models might blunt independent effects of GI and fiber on health outcomes.
Strength and Limitation
This was the first study that found bilirubin could be affected by dietary factors. However, the mediating role of bilirubin levels on carbohydrate/GL-GDM associations contained uncertainty. The causal inference of the relationship remains controversial and needs to be confirmed by further dietary therapy on bilirubin levels and GDM prevention. Therefore, this secondary data analysis has little clinical implication on dietary recommendation. Instead, it sheds a new light into the underlying etiology of GDM, as well as the value of increasing serum bilirubin as an early protector of GDM following dietary modification, which called for future attention. The positive association between bilirubin and total carbohydrate intake (%E), may be explained by potential anti-inflammatory and anti-oxidative capacities, and regarding this, we still need more clinical trials to prove this benefit in at-risk population.
Some limitations of our study merit discussion. It is known that we were doing a secondary data analysis using cohort from a RCT program. Dietary counseling approaches, though controlled in the models, did affect the GDM outcome to some extent. As the data collection was not designed for this study aim, the data had limited availability that was impossible to be retrieved after years. Although we were able to adjust for a wide variety of lifestyle maternal factors, residual confounding was possible since some covariates were eliminated from analysis. Other factors affecting GDM such as vitamin D deficiency, FFAs, central obesity and polycystic ovary syndrome were not available in this study. Secondly, our study only included overweight and obese gravidae, so the results might not be generalized to normal-weight gravidae. It is noteworthy that insulin resistance and elevated triglyceride can be present in normal-weight people, especially when visceral adiposity exists, and this could not be fully explained by BMI (50). Hence, whether our findings are supportive to this population still needs investigation, preferably covering repeated insulin tolerance tests, visceral adiposity and lipid profile into analysis. Thirdly, the dietary intakes contained some error. Within-individual diet variability was not considered due to inability to repeat measurements on 24-h dietary recall. Besides, GI values were unavailable in some food items and insufficient calculation was possible. Future high-quality dietary data is needed to address this main flaw, as well as take more dietary factors (e.g., macronutrient subtypes, micronutrients, different vitamin supplement and biochemical indicators of dietary intake) into consideration. Self-reported information was also subject to underreporting and interviewer bias. While carbohydrate parameters seemed exchangeable across most maternal characteristics and results were robust in sensitivity analysis, the causal inference of examined associations was in uncertainty based on the observational nature of the study. Finally, due to limited statistical power in this small study, larger-scale, and well-designed investigations are warranted to draw firm conclusions.
Conclusions
In summary, results from our study suggested mildly elevated serum bilirubin levels appeared to be in response to higher energies consumed from total carbohydrate intake during early pregnancy in overweight and obese gravidae. However, the mediating effect of bilirubin levels seemed modest, compared to direct effect of carbohydrate on GDM. Further large and well-designed investigations might help increase certainty, and give clues to the pathogenesis of GDM. The value of increasing serum bilirubin as an early protector of GDM following dietary modification, is also worthy of future attention.
Data Availability Statement
The original contributions presented in the study are included in the article/Supplementary Materials, further inquiries can be directed to the corresponding author/s.
Ethics Statement
The studies involving human participants were reviewed and approved by ethical review and approval were waived for this study, due to prior ethical clearance from the Ethics Committee of Children's Hospital of Fudan University (Approval No. 071-2012). The patients/participants provided their written informed consent to participate in this study.
Author Contributions
WH and WY contributed to conception and design of the study. LW took charge of data collection and curation. WH performed the statistical analysis and wrote the original draft of the manuscript. WH, YZ, and XC performed software and visualization. WY provided supervision on the whole research procedure. All authors contributed to manuscript revision, read, and approved the submitted version.
Conflict of Interest
The authors declare that the research was conducted in the absence of any commercial or financial relationships that could be construed as a potential conflict of interest.
Publisher's Note
All claims expressed in this article are solely those of the authors and do not necessarily represent those of their affiliated organizations, or those of the publisher, the editors and the reviewers. Any product that may be evaluated in this article, or claim that may be made by its manufacturer, is not guaranteed or endorsed by the publisher.
Acknowledgments
The authors want to give great thanks to all the staffs, nurses, and technicians who are from the Kunshan Maternity and Child Care Center and the International Peace Maternity and Child Health Hospital of China Welfare Institute out of the author list for data collection. We are also grateful to all participants who are the origin of the data.
Supplementary Material
The Supplementary Material for this article can be found online at: https://www.frontiersin.org/articles/10.3389/fnut.2021.701422/full#supplementary-material
References
1. Leng J, Shao P, Zhang C, Tian H, Zhang F, Zhang S, et al. Prevalence of gestational diabetes mellitus and its risk factors in Chinese pregnant women: a prospective population-based study in Tianjin, China. PLoS ONE. (2015) 10:e0121029. doi: 10.1371/journal.pone.0121029
2. Chiefari E, Arcidiacono B, Foti D, Brunetti A. Gestational diabetes mellitus: an updated overview. J Endocrinol Invest. (2017) 40:899–909. doi: 10.1007/s40618-016-0607-5
3. Metzger BE, Gabbe SG, Persson B, Buchanan TA, Catalano PA, Damm P, et al. International association of diabetes and pregnancy study groups recommendations on the diagnosis and classification of hyperglycemia in pregnancy. Diabetes Care. (2010) 33:676–82. doi: 10.2337/dc09-1848
4. Di Cianni G, Miccoli R, Volpe L, Lencioni C, Del Prato S. Intermediate metabolism in normal pregnancy and in gestational diabetes. Diabetes Metab Res Rev. (2003) 19:259–70. doi: 10.1002/dmrr.390
5. Vítek L. The role of bilirubin in diabetes, metabolic syndrome, and cardiovascular diseases. Front Pharmacol. (2012) 3:55. doi: 10.3389/fphar.2012.00055
6. Kunutsor SK, Frysz M, Verweij N, Kieneker LM, Bakker SJL, Dullaart RPF. Circulating total bilirubin and risk of non-alcoholic fatty liver disease in the PREVEND study: observational findings and a Mendelian randomization study. Eur J Epidemiol. (2019) 35:123–37. doi: 10.1007/s10654-019-00589-0
7. Dullaart RP, de Vries R, Lefrandt JD. Increased large VLDL and small LDL particles are related to lower bilirubin in type 2 diabetes mellitus. Clin Biochem. (2014) 47:170–5. doi: 10.1016/j.clinbiochem.2014.08.008
8. Luján MG, Igino R, Donald OJ, Fleur B, Alessia B, Sukowati C, et al. Bilirubin inhibits the TNFalpha-related induction of three endothelial adhesion molecules. Biochem Biophys Res Commun. (2009) 386:338–44. doi: 10.1016/j.bbrc.2009.06.029
9. Roy S, Banerjee U, Dasgupta A. Protective role of bilirubin against increase in hsCRP in different stages of hypothyroidism. Indian J Clin Biochem. (2016) 31:43–9. doi: 10.1007/s12291-015-0495-z
10. Liu C, Zhong C, Zhou X, Chen R, Wu J, Wang W, et al. Higher direct bilirubin levels during mid-pregnancy are associated with lower risk of gestational diabetes mellitus. Endocrine. (2017) 55:165–72. doi: 10.1007/s12020-016-1103-6
11. Hotamisligil GS. Inflammation and metabolic disorders. Nature. (2006) 444:860–7. doi: 10.1038/nature05485
12. Buyken AE, Goletzke J, Joslowski G, Felbick A, Cheng G, Herder C, et al. Association between carbohydrate quality and inflammatory markers: systematic review of observational and interventional studies. Am J Clin Nutr. (2014) 99:813–33. doi: 10.3945/ajcn.113.074252
13. Calder PC, Ahluwalia N, Brouns F, Buetler T, Clement K, Cunningham K, et al. Dietary factors and low-grade inflammation in relation to overweight and obesity. Br J Nutr. (2011) 106(Suppl. 3):S5–78. doi: 10.1017/S0007114511005460
14. Zhang Y, Wang L, Yang W, Niu D, Li C, Wang L, et al. Effectiveness of low glycemic index diet consultations through a diet glycemic assessment app tool on maternal and neonatal insulin resistance: a randomized controlled trial. JMIR Mhealth Uhealth. (2019) 7:e12081. doi: 10.2196/12081
15. Bei-Fan Z Cooperative Meta-Analysis Group of the Working Group on Obesity in China. Predictive values of body mass index and waist circumference for risk factors of certain related diseases in Chinese adults: study on optimal cut-off points of body mass index and waist circumference in Chinese adults. Asia Pacif J Clin Nutr. (2002) 11:S685–93. doi: 10.1046/j.1440-6047.11.s8.9.x
16. Chen XF, Li YP, Yu JJ. Chinese DRIs in perspective of global disease burden: challenges, evidence and thinking. Chin J Evidence Based Med. (2013) 13:1162–71. doi: 10.7507/1672-2531.20130199
17. Yang Y, Safety IoNaF, Wang G, Pan X. Chinese Food Composition Table 2002. Peking: Peking University Medical Press (2002).
18. Neuhouser ML, Tinker LF, Cynthia T, Bette C, Van HL, Linda S, et al. Development of a glycemic index database for food frequency questionnaires used in epidemiologic studies. J Nutr. (2006) 136:1604. doi: 10.1093/jn/136.6.1604
19. Foster-Powell K, Miller JB. International table of glycemic index and glycemic load values. Diabetes Care. (2008) 31:2281–3. doi: 10.2337/dc08-1239
20. Cook PA, Coles-Rutishauser I, Seelig M. Comparable Data on Food and Nutrient Intake and Physical Measurements from the 1983, 1985 and 1995 National Nutrition Surveys. Canberra: History. (2001).
21. Willett WC, Howe GR, Kushi LH. Adjustment for total energy intake in epidemiologic studies. Am J Clin Nutr. (1997) 65(Suppl. 4):1220–8S; discussion: 1229–31S. doi: 10.1093/ajcn/65.4.1220S
22. Augustin LSA, Kendall CWC, Jenkins DJA, Willett WC, Astrup A, Barclay AW, et al. Glycemic index, glycemic load and glycemic response: an international scientific consensus summit from the international carbohydrate quality consortium (ICQC). Nutr Metab Cardiovasc Dis. (2015) 25:795–815. doi: 10.1016/j.numecd.2015.05.005
23. Coustan DR, Lowe LP, Metzger BE, Dyer AR, International Association of D, Pregnancy Study G. The Hyperglycemia and Adverse Pregnancy Outcome (HAPO) study: paving the way for new diagnostic criteria for gestational diabetes mellitus. Am J Obstet Gynecol. (2010) 202:654 e1–6. doi: 10.1016/j.ajog.2010.04.006
24. Freathy RM, Hayes MG, Urbanek M, Lowe LP, Lee H, Ackerman C, et al. Hyperglycemia and Adverse Pregnancy Outcome (HAPO) study: common genetic variants in GCK and TCF7L2 are associated with fasting and postchallenge glucose levels in pregnancy and with the new consensus definition of gestational diabetes mellitus from the International Association of Diabetes and Pregnancy Study Groups. Diabetes. (2010) 59:2682–9. doi: 10.2337/db10-0177
25. Matthews DR, Hosker JP, Rudenski AS, Naylor BA, Treacher DF, Turner RC. Homeostasis model assessment: insulin resistance and beta-cell function from fasting plasma glucose and insulin concentrations in man. Diabetologia. (1985) 28:412–9. doi: 10.1007/bf00280883
26. Pardo A, Román M. Reflections on the Baron and Kenny model of statistical mediation. Anales De Psicologia. (2013) 29:614–23. doi: 10.6018/analesps.29.2.139241
27. Baron RM, Kenny DA. The moderator-mediator variable distinction in social psychological research: conceptual, strategic, and statistical considerations. J Pers Soc Psychol. (1999) 51:1173.
28. Zhang C, Ning Y. Effect of dietary and lifestyle factors on the risk of gestational diabetes: review of epidemiologic evidence. Am J Clin Nutr. (2011) 94(Suppl. 6):9–9S doi: 10.3945/ajcn.110.001032
29. Zhang C, Liu S, Solomon CG, Hu FB. Dietary fiber intake, dietary glycemic load, and the risk for gestational diabetes mellitus. Diabetes Care. (2006) 29:2223–30. doi: 10.2337/dc06-0266
30. Sluijs I, Beulens JW, van der Schouw YT, van der AD, Buckland G, Kuijsten A, et al. Dietary glycemic index, glycemic load, and digestible carbohydrate intake are not associated with risk of type 2 diabetes in eight European countries. J Nutr. (2013) 143:93–9. doi: 10.3945/jn.112.165605
31. Looman M, Schoenaker D, Soedamah-Muthu SS, Geelen A, Feskens EJM, Mishra GD. Pre-pregnancy dietary carbohydrate quantity and quality, and risk of developing gestational diabetes: the Australian Longitudinal Study on Women's Health. Br J Nutr. (2018) 120:435–44. doi: 10.1017/S0007114518001277
32. Sheard NF, Clark NG, Brand-Miller JC, Franz MJ, Pi-Sunyer FX, Mayer-Davis E, et al. Dietary carbohydrate (amount and type) in the prevention and management of diabetes a statement by the American diabetes association. Diabetes Care. (2004) 27:2266–71. doi: 10.2337/diacare.27.9.2266
33. Hernandez TL. Carbohydrate content in the GDM diet: two views: view 1: nutrition therapy in gestational diabetes: the case for complex carbohydrates. Diabetes Spectr. (2016) 29:82–8. doi: 10.2337/diaspect.29.2.82
34. Kapur K, Kapur A, Hod M. Nutrition management of gestational diabetes mellitus. Ann Nutr Metab. (2021) 76(Suppl. 3):17–29. doi: 10.1159/000509900
35. Farabi SS, Hernandez TL. Low-carbohydrate diets for gestational diabetes. Nutrients. (2019) 11:1737. doi: 10.3390/nu11081737
36. Sivan E, Chen X, Homko CJ, Ree Ce EA, Boden G. Longitudinal study of carbohydrate metabolism in healthy obese pregnant women. Diabetes Care. (1997) 20:1470–5. doi: 10.2337/diacare.20.9.1470
37. Ley SH, Hanley AJ, Retnakaran R, Sermer M, Zinman B, O'Connor DL. Dietary fat and carbohydrate intake during early pregnancy and risk of gestational diabetes. Paediatr Perinat Epidemiol. (2011) 22:47–59. doi: 10.1111/j.1365-3016.2007.00899.x
38. Saldana TM, Maria S, Adair LS. Effect of macronutrient intake on the development of glucose intolerance during pregnancy. Am J Clin Nutr. (2004) 79:479–86. doi: 10.1093/ajcn/79.3.479
39. Hernandez TL, Van Pelt RE, Anderson MA, Daniels LJ, West NA, Donahoo WT, et al. A higher-complex carbohydrate diet in gestational diabetes mellitus achieves glucose targets and lowers postprandial lipids: a randomized crossover study. Diabetes Care. (2014) 37:1254–62. doi: 10.2337/dc13-2411
40. Wei B, Katherine B, Tobias DK, Olsen SF, Jorge C, Allan V, et al. Prepregnancy low-carbohydrate dietary pattern and risk of gestational diabetes mellitus: a prospective cohort study. Am J Clin Nutr. (2014) 99:1378. doi: 10.3945/ajcn.113.082966
41. Goletzke J, Buyken AE, Joslowski G, Bolzenius K, Remer T, Carstensen M, et al. Increased intake of carbohydrates from sources with a higher glycemic index and lower consumption of whole grains during puberty are prospectively associated with higher IL-6 concentrations in younger adulthood among healthy individuals. J Nutr. (2014) 144:1586–93. doi: 10.3945/jn.114.193391
42. Franco-Sena AB, De Oliveira LC, De Jesus Pereira Pinto T, Rodrigues Farias D, Dos Santos Vaz J, Kac G. Factors associated with prospective leptin concentrations throughout pregnancy in pregestational normal weight, overweight and obese women. Clin Endocrinol. (2015) 82:127–35. doi: 10.1111/cen.12487
43. Gregersen S, Samocha-Bonet D, Heilbronn LK, Campbell LV. Inflammatory and oxidative stress responses to high-carbohydrate and high-fat meals in healthy humans. J Nutr Metab. (2012) 2012:238056. doi: 10.1155/2012/238056
44. Kasim-Karakas SE, Alex T, Uma S, Ishwaral J. Responses of inflammatory markers to a low-fat, high-carbohydrate diet: effects of energy intake. Am J Clin Nutr. (2006) 83:774–9. doi: 10.1093/ajcn/83.4.774
45. Kamari Y, Grossman E, Oron-Herman M, Peleg E, Shabtay Z, Shamiss A, et al. Metabolic stress with a high carbohydrate diet increases adiponectin levels. Hormone Metab Res. (2007) 39:384–8. doi: 10.1055/s-2007-976534
46. Sparti A, Milon H, Di Vetta V, Schneiter P, Tappy L, Jéquier E, et al. Effect of diets high or low in unavailable and slowly digestible carbohydrates on the pattern of 24-h substrate oxidation and feelings of hunger in humans. Am J Clin Nutr. (2000) 72:1461–8. doi: 10.1093/ajcn/72.6.1461
47. Kamuren ZT, Sanders R, Iii J. Low-carbohydrate diet and oxidative stress in diabetic and nondiabetic rats. J Biochem Mol Toxicol. (2006) 20:259–69. doi: 10.1002/jbt.20142
48. David P, MacKinnon, Jennifer L, KrullChondra, et al. Equivalence of the mediation, confounding and suppression effect. Prevent Sci. (2000) 1:173–81. doi: 10.1023/A:1026595011371
49. Stocker R, Yamamoto Y, Mcdonagh A, Glazer A, Ames B. Bilirubin is an antioxidant of possible physiological importance. Science. (1987) 235:1043–6. doi: 10.1126/science.3029864
Keywords: carbohydrate, bilirubin, gestational diabetes mellitus, glycemic load, pregnant women
Citation: He W, Wang L, Zhang Y, Jiang Y, Chen X, Wang Y, Dou Y, Chen H and Yan W (2021) Higher Serum Bilirubin Levels in Response to Higher Carbohydrate Intake During Early Pregnancy and Lower Gestational Diabetes Mellitus Occurrence in Overweight and Obese Gravidae. Front. Nutr. 8:701422. doi: 10.3389/fnut.2021.701422
Received: 28 April 2021; Accepted: 11 August 2021;
Published: 30 August 2021.
Edited by:
Ioannis Zabetakis, University of Limerick, IrelandReviewed by:
Silvia Vannuccini, University of Florence, ItalyOtilia Perichart-Perera, Instituto Nacional de Perinatología (INPER), Mexico
Copyright © 2021 He, Wang, Zhang, Jiang, Chen, Wang, Dou, Chen and Yan. This is an open-access article distributed under the terms of the Creative Commons Attribution License (CC BY). The use, distribution or reproduction in other forums is permitted, provided the original author(s) and the copyright owner(s) are credited and that the original publication in this journal is cited, in accordance with accepted academic practice. No use, distribution or reproduction is permitted which does not comply with these terms.
*Correspondence: Weili Yan, eWFud2xAZnVkYW4uZWR1LmNu