- 1Department of Clinical Epidemiology, Shengjing Hospital of China Medical University, Shenyang, China
- 2Clinical Research Center, Shengjing Hospital of China Medical University, Shenyang, China
Background and Aims: The associations between dietary carbohydrate and diverse health outcomes remain controversial and confusing. To summarize the existing evidence of the association between dietary carbohydrate intake and diverse health outcomes and to evaluate the credibility of these sources of evidence. We performed this umbrella review of evidence from meta-analyses of observational studies.
Methods: PubMed, Embase, Web of Science databases, and manual screening of references up to July 2020 were searched. Systematic reviews with meta-analyses of observational studies in humans investigating the association between dietary carbohydrate intake and multiple health outcomes were identified. We assessed the evidence levels by using summary effect sizes, 95% prediction intervals, between-study heterogeneity, evidence of small-study effects, and evidence of excess significance bias for each meta-analysis.
Results: We included 43 meta-analyses of observational research studies with 23 health outcomes, including cancer (n = 26), mortality (n = 4), metabolic diseases (n = 4), digestive system outcomes (n = 3), and other outcomes [coronary heart disease (n = 2), stroke (n = 1), Parkinson's disease (n = 1), and bone fracture (n = 2)]. This umbrella review summarized 281 individual studies with 13,164,365 participants. Highly suggestive evidence of an association between dietary carbohydrate intake and metabolic syndrome was observed with adjusted summary odds ratio of 1.25 [95% confidence interval (CI) 1.15–1.37]. The suggestive evidences were observed in associations of carbohydrate consumption with esophageal adenocarcinoma (0.57, 95% CI = 0.42–0.78) and all-cause mortality (adjusted summary hazard ratio 1.19, 95% CI = 1.09–1.30).
Conclusions: Despite the fact that numerous systematic reviews and meta-analyses have explored the relationship between carbohydrate intake and diverse health outcomes, there is no convincing evidence of a clear role of carbohydrate intake. However, there is highly suggestive evidence suggested carbohydrate intake is associated with high risk of metabolic syndrome, suggestive evidence found its association with increased risk of all-cause mortality and decreased risk of esophageal adenocarcinoma.
Systematic Review Registration: CRD42020197424.
Introduction
Dietary carbohydrates constitute a specific group of substances with a range of chemical, physical, and physiological properties, which serve as the main and preferable source of body energy (1, 2). Carbohydrates are classified into available carbohydrates and resistant carbohydrates based on their digestion and absorption by the digestive system (3). Available carbohydrates can be absorbed in the small intestine and provide energy to important tissues, such as the brain, red blood cells, and the developing fetus (3, 4). Resistant carbohydrate, such as prebiotics and resistant starch, may influence the activity of intestinal microbiota and other physiological function (1, 3).
Historically, in terms of energy supplied to glucose-dependent tissues, such as brain, consumption of increased amounts of carbohydrate may have provided a substantial evolutionary advantage (4). The importance of dietary carbohydrate intake for disease prevention has been stressed by health organizations and programs such as the American Cancer Society and the National Cholesterol Education Program (NCEP) (5). The intake of carbohydrate recommended by the NCEP is 50–60% of the total calories (6). However, dietary carbohydrate intake has been an anathema in the public view due to different reasons including worries about obesity and adverse effects of carbohydrates on the level of high-density lipoprotein and triglycerides (5). In fact, these seemingly contradictory concepts are due to the different effects of various types of carbohydrates on health. A previous umbrella review found that there is convincing evidence of an inverse association between whole grains (contain fiber, vitamins, minerals, and phytochemicals with antioxidant properties) consumption and risk of type 2 diabetes and colorectal cancer (7). Nevertheless, refined carbohydrates, such as white rice and noodles, might reflect poor food quality and confer a chronically high glycemic load that can lead to negative metabolic consequences and other adverse health outcomes (8). In the last decade, numerous epidemiologic studies have shown that dietary carbohydrate intake is potentially linked to increasing risk of many health outcomes including total mortality (8), endometrial cancer (9), colorectal cancer (10), coronary heart disease (11), type 2 diabetes (12), metabolic syndrome (13), cortical and nuclear cataract (14), which are of great important to public health and societal cost. However, the findings from these studies are, to some extent, controversial and confusing as substantial heterogeneity and inherent study biases (such as residual confounding and selective reporting of positive results). For example, the association between dietary carbohydrate intake and mortality has not been unified (8, 15, 16).
To the best of our knowledge, there has been no attempt to comprehensively summaries the studies addressing the association between dietary carbohydrates intake and multiple health outcomes. Previous efforts to systematically appraise the evidence on dietary carbohydrate have been focused on single disease endpoints. Hence, we performed the first umbrella review of published meta-analyses and systematic reviews. The aim of the present study is to investigate the breadth and strength of the existing evidence by systematically assessing the quality of the studies in order to identify potential biases and highlight those with the strongest evidence of medical evidence.
Methods
Umbrella Review Methods
Umbrella review is a method that synthesizes a large number of existing systematic reviews and/or meta-analyses on risk factors, rather than performing these systematic reviews from the beginning. We conducted an umbrella review, i.e., a comprehensive and systematic search, to organize, and evaluate the existing evidence of dietary carbohydrate intake and diverse health outcomes from multiple systematic reviews and meta-analyses (17). The protocol of the study was registered on PROSPERO (CRD42020197424). This study was performed following the Meta-analyses Of Observational Studies in Epidemiology (MOOSE) guidelines (Supplementary Table 1) (18).
Literature Search
Two independent investigators (Y-SL and J-LL) comprehensively searched the published literature using the PubMed, Embase, and Web of Science databases from inception to July 10, 2020 for systematic reviews or meta-analyses of observational studies that evaluated the evidence regarding the effects of dietary carbohydrate on health. The key words used in the search strategy were “carbohydrate” or “carbohydrates,” and “meta” or “meta-analysis” or “systematic review.” The detailed search strategy is shown in Supplementary Table 2. No language restrictions were considered for the selection of eligible studies for this review. Furthermore, we conducted a manual search of the reference lists of the retrieved articles. A third investigator (Q-JW) arbitrated any differences that could not be resolved by consensus. Only data from published papers were included, and the study authors were not contacted.
Study Selection and Exclusion Criteria
Articles were eligible if they were meta-analyses and had been conducted systematically. We included only meta-analyses or systematic reviews of observational studies (cohort studies, case-control studies, and cross-sectional studies) in humans. Meta-analyses were included when they summarized any combination of relative risks, odds ratios, relative rates, or hazard ratios from studies investigating the association between dietary carbohydrate and any health-related outcome (e.g., cardiovascular disease, cancer, death, obesity or overweight, diabetes, and metabolic diseases).
No randomized controlled trials related to our research question were found. We excluded studies of genetic polymorphisms of carbohydrate metabolism. Systematic reviews without a quantitative synthesis, meta-analyses based on individual data without a systematic review, or systematic reviews of ecological studies with no individual data were excluded. Meta-analyses or systematic reviews that did not present study specific data [risk estimates, 95% confidence intervals (CIs), or the number of events, controls, or total sample size] were also excluded.
Separate meta-analyses for different eligible outcomes within individual articles were assessed separately. When more than one meta-analysis presented overlapping datasets on the same outcome, only the meta-analysis with the largest dataset was retained for the main analysis. All the selection and exclusion procedures were carried out by two independent investigators (Y-SL and Q-JW).
Data Abstraction
Data extraction was performed independently by two investigators (Y-SL and Y-TJ) using a custom-made data extraction form. Disagreements were re-evaluated by a third investigator (Q-JW). When a meta-analysis or systematic review reported both summarized results and results divided according to subgroups, the summarized results were preferred, since they were derived from a larger sample. The following key study characteristics were abstracted from each systematic review and meta-analysis: (1) first author, (2) publication year, (3) journal name, (4) study design, (5) number of studies included, (6) total number of cases and participants, (7) outcome(s) of interest, and (8) type of effect metric.
For primary studies from each systematic review and meta-analysis included, the following key study characteristics were abstracted: (1) first author, (2) publication year, (3) study design, (4) study population, (5) outcome(s) of interest investigated, (6) unit of exposure comparison, (7) methods of ascertainment of dietary carbohydrate intake (e.g., food-frequency questionnaire and 24-h recall), (8) type of comparison (high vs. low analysis or dose-response analysis), (9) total number of cases and/or controls, (10) maximally adjusted risk estimates and 95% CIs, and (11) the effect of dose-response relation. If a risk factor was examined in more than one comparison, we extracted the data from the comparison with the largest number of component studies.
Assessment of the Methodological Quality of the Included Studies
The methodological quality of the meta-analyses included was assessed using the validated AMSTAR (A Measurement Tool to Assess Systematic Reviews) instrument. AMSTAR measures 11 items that allow a comprehensive evaluation of systematic reviews (19). AMSTAR has been shown to be a reliable and valid tool for the quality assessment of systematic reviews and meta-analyses of both interventional and observational research (20, 21), which includes search quality ratings, analysis, and transparency of a meta-analysis. The answers include “yes,” “no,” “cannot answer,” and “not applicable” for every question. For each “yes” response, participants score one point, and the maximum score is 11. The methodological quality was categorized into high quality, moderate quality, and low quality based on an overall score of at least 8 points, 4–7 points, and 3 points or less, respectively (20, 22).
Statistical Analyses
For each exposure and outcome pair, we calculated the summary effect and the 95% CI using fixed and random effects methods, respectively, to test the stability and reliability of associations between dietary carbohydrate and health outcomes. Furthermore, 95% prediction intervals (95% PIs) for the summary random effects estimates were used to show true effects for 95% of the summarized studies or similar (exchangeable) studies that might be conducted in the future (23, 24). For the largest study of each meta-analysis, we estimated the standard error (SE) of the effect size and examined whether the SE was <0.10. In a study with a SE of <0.10, the difference between the effect estimates and the upper or lower 95% CI was <0.20 (i.e., this uncertainty is lower than what is considered a small effect size) (25).
We used the I2 statistic as an estimate of proportion of variance reflecting true differences in effect size. Values exceeding 50% or 75% were considered to represent large or very large heterogeneity, respectively (26). We assessed evidence for small study effects using the regression asymmetry test proposed by Egger and colleagues (27). A P-value of ≤ 0.10 in the regression asymmetry test with a more conservative effect in the largest study was considered evidence for small-study effects bias (28).
We applied the excess significance test to investigate whether the observed number of studies (O) with nominally significant results (“positive” studies, P < 0.05) was different from the expected number of significant results (E). The detailed description of this method is described in a previous study (28). Briefly, the expected number of studies with significant results is calculated in each meta-analysis via the sum of the statistical power estimates for each component study (29). Since the true effect size for any meta-analysis is not known, we estimated the power of each component study using the effect size of the largest study (smallest SE) in a meta-analysis (29). The statistical power of each study was calculated using an algorithm from a non-central t distribution (30). Excess significance for single meta-analyses was defined at P < 0.10. The comparison of observed vs. expected values was performed separately for each meta-analysis, and was also extended to groups, including many meta-analyses, after summing the observed and expected values from each meta-analysis. As described elsewhere, the number of expected “positive” (i.e., significant data sets) studies can be compared with the observed number of significant studies through a chi-square-based test (29). The larger the difference between observed and expected values, the higher the excess of significance bias.
All analyses were performed using STATA software, version 15 (StataCorp, College Station, TX).
Credibility Assessment
We categorized the strength of the evidence of dietary carbohydrate intake for outcomes into convincing, highly suggestive, suggestive, weak evidence, or non-significant associations according to the following criteria (31).
(1) The evidence was defined as convincing when the P-value of the random-effects model was smaller than 10−6, the meta-analysis included more than 1,000 cases or more than 20,000 participants for continuous outcomes, if the largest component study in the meta-analysis reported a significant result (P < 0.05), if the 95% PIs excluded the null hypothesis, if the I2 statistic for heterogeneity was <50%, if there was no evidence of small study effects (P > 0.10), and if excess significance bias (P > 0.10) was indicated.
(2) The evidence was defined as highly suggestive if the P-value for the random-effects model was <10−6, if the meta-analysis included more than 1,000 cases or more than 20,000 participants for continuous outcomes, and if the largest component study reported a significant result.
(3) The evidence was defined as suggestive if the P-value for random-effects was < 10−3, or if there were more than 1,000 cases or more than 20,000 participants for continuous outcomes.
(4) The evidence was defined as weak if the P-value for significant associations was <0.05.
(5) We used the ‘non-significant associations' classification if all association tests yielded a P-value > 0.05.
Results
Of the 12,532 articles (excluding duplicates) initially identified in three databases, 12,345 articles were excluded after title and abstract review. Finally, 24 eligible articles were identified (8–13, 15, 32–48). The search yielded 43 meta-analyses after full-text screening (Figure 1). After data abstraction, 30 of the 43 meta-analyses were selected for the main analysis for systematically assessing the quality of existing evidence. The characteristics of the 24 articles are shown in Supplementary Table 3. The list of excluded studies and the exclusion grounds during the process of full-text review are shown in Supplementary Table 4.
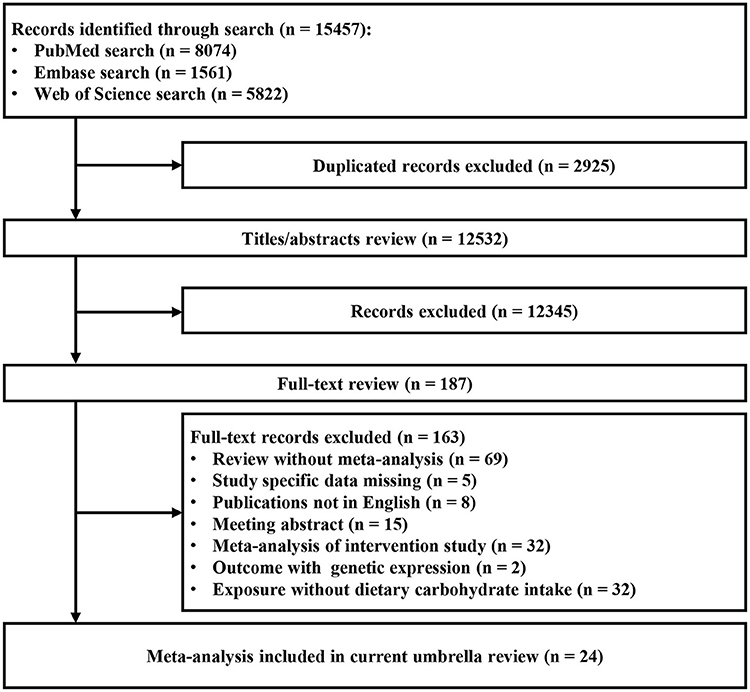
Figure 1. Flowchart of selection of studies for inclusion in umbrella review on dietary carbohydrate intake and health outcomes.
Characteristic of the Meta-Analysis
In all 43 meta-analyses identified via full-text screening, more than one published meta-analysis was examined for 23 outcomes, including cancer [breast cancer (n = 1 meta-analysis), colorectal cancer (n = 4), digestive system cancers (n = 2), endometrial cancer (n = 3), esophageal adenocarcinoma (n = 2), esophageal cancer (n = 3), esophageal squamous cell carcinoma (n = 2), gastric cancer (n = 2), liver cancer (n = 1), pancreatic cancer (n = 3), prostate cancer (n =3)], mortality [all-cause mortality (n = 2), specific-cause mortality (n = 2)], metabolic diseases [type 2 diabetes (n = 3), metabolic syndrome (n = 2)], digestive system outcomes [ulcerative colitis (n = 1), Crohn's disease (n = 1), inflammatory bowel diseases (n = 1)], other outcomes [coronary heart disease (n = 2), stroke (n = 1), Parkinson's disease (n = 1), and bone fracture (n = 2)]. The percentage of outcomes per category is shown in Supplementary Figure 1. The characteristics, quantitative synthesis, and credibility assessment of evidence of all eligible meta-analyses are shown in Supplementary Tables 5, 6, respectively.
After retaining the largest dataset for the main analysis, there were 30 meta-analyses summarizing 281 individual studies. The median number of primary studies in each meta-analysis was eight (range: 4–22), the median number of cases was 3,600 (range: 388–69,164), and the median number of participants was 309,923 (range: 1,344–2,666,588). The number of cases was >1,000 in 27 meta-analyses (90.0%), three meta-analyses included <1,000 cases (outcomes: Crohn's disease, ulcerative colitis, and liver cancer).
Summary Effect Size
Of the 30 meta-analyses, summary random effects estimates were significant (P ≤ 0.05) in 9 (30.0%), whereas summary fixed effects were significant in 11 meta-analyses (36.7%). At a stricter threshold of P < 0.001, 3 (10.0%) and 6 (20.0%) meta-analyses produced significant summary results using the random and fixed effects models, respectively. At P < 10−6, 1 (3.3%) and 4 (13.3%) meta-analyses were significant by the random and fixed effects models, respectively (Table 1). Moreover, the largest study showed statistically significant results in 4 (13.3%) meta-analyses (Table 2). The effects of the largest studies were more conservative than the summary effects of the meta-analysis in 13 of the 30 (43.3%) meta-analyses.
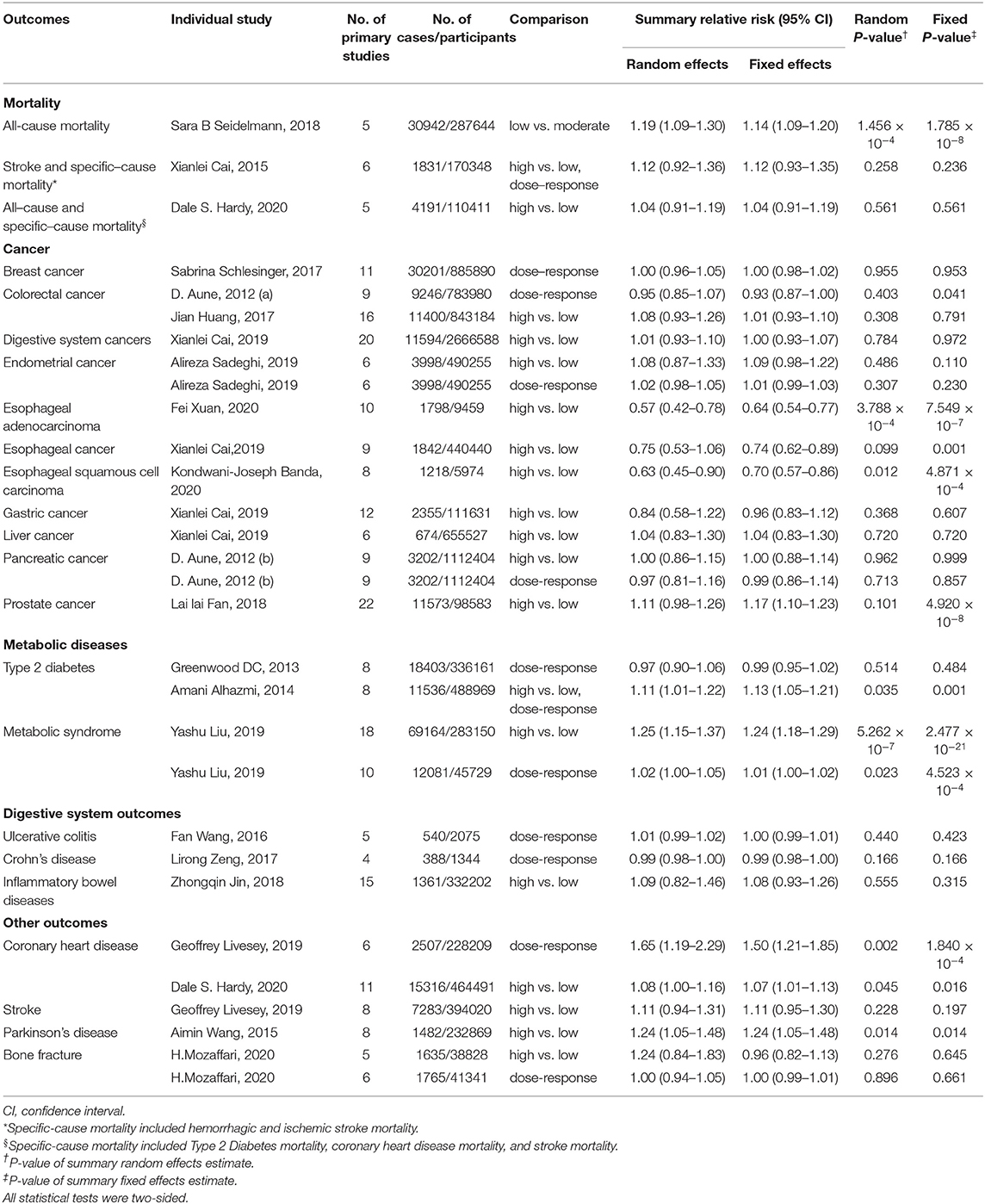
Table 1. The characteristics and quantitative synthesis of the eligible meta-analyses reporting dietary carbohydrate intake relation to multiple outcomes.
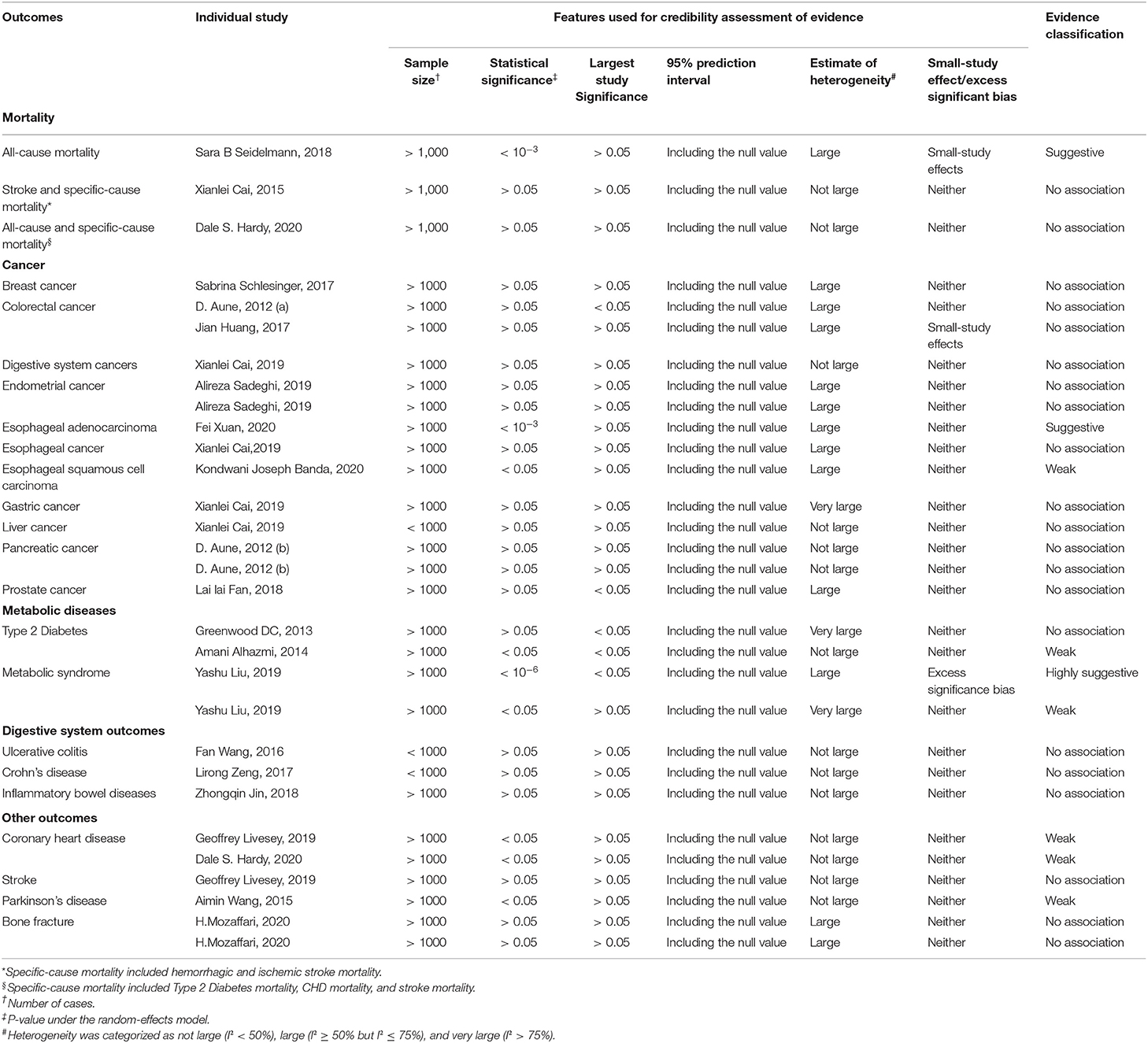
Table 2. Credibility assessment of evidence for the meta-analyses reporting association of dietary carbohydrate intake relation to multiple outcomes.
Heterogeneity Between Studies
There was large heterogeneity in 13 (43.3%) meta-analyses and very large heterogeneity in three (10.0%) meta-analyses. The meta-analyses with very large heterogeneity included outcomes such as type 2 diabetes, gastric cancer, and metabolic syndrome. In addition, uncertainty of the summary effects was further assessed by calculating 95% PIs. The 95% PIs of all meta-analyses included the null value (Table 2).
Small Study Effects and Excess Significance
According to the Egger's test results, two (6.7%) meta-analyses (colorectal cancer and all-cause mortality) showed small study effects, and a more conservative effect was found in the largest studies. For the remaining 28 (93.3%) meta-analyses, no evidence was found for small-study effects and comparison between the summary random effects estimate and the point estimate of the largest study. Excess significance was present at P < 0.1 and O > E. As a result, only one (3.3%) meta-analysis tested positive in the excess significance test (Table 2).
Strength of Evidence
Based on the findings, the meta-analyses with nominally significant summary results were categorized into convincing, highly suggestive, suggestive, or weak evidence (Table 3). No meta-analysis presented convincing evidence, one (3.3%) presented highly suggestive evidence of the association between an increase in dietary carbohydrate intake and a higher risk of metabolic syndrome, and two (6.7%) presented suggestive evidence for three outcomes. In these studies, dietary carbohydrate intake was found to be associated with decreased risk of esophageal adenocarcinoma, and increased risk of all-cause mortality. Six (20.0%) meta-analyses with five outcomes presented weak evidence. The evidence reported for a negative effect of dietary carbohydrate intake on four different outcomes (coronary heart disease, type 2 diabetes, metabolic syndrome, and Parkinson's disease) was considered weak, as was the positive association between dietary carbohydrate intake and esophageal squamous cell carcinoma (Table 3).
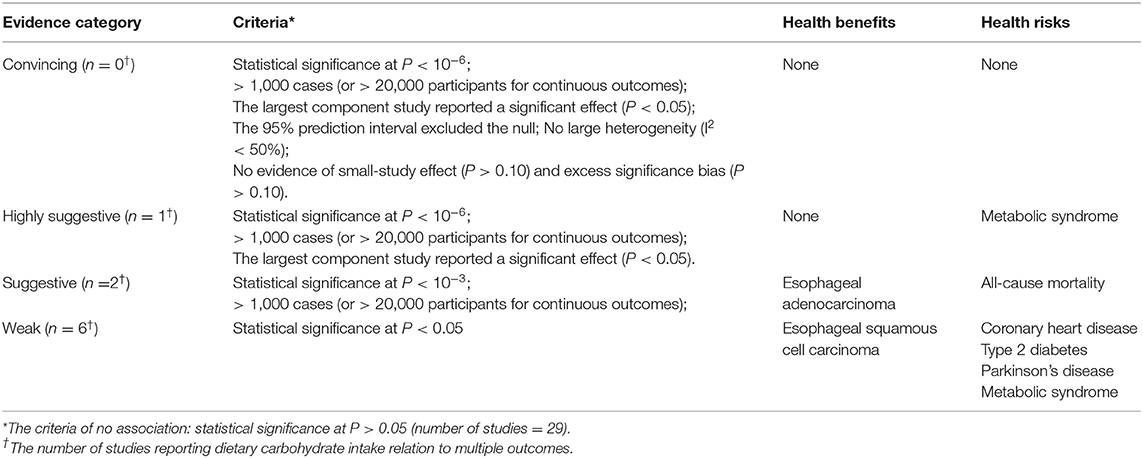
Table 3. Summary of evidence grading for studies reporting dietary carbohydrate intake relation to multiple outcomes.
Methodological Quality
Overall scores of AMSTAR for each eligible article are shown in Supplementary Figure 2, and single items are summarized in Supplementary Table 7. The AMSTAR score ranged from 5 to 10. Eight (33.3%) studies were considered to be of high quality and 16 (66.7%) of moderate quality. No study fell in the low or critically low quality category. In general, the main flaws in these eligible articles were that the study design was not established before the conduct of the review, the gray literature was not accounted for in the literature search, and the list of excluded studies was not provided.
Discussion
Principal Findings
Our umbrella review provides an overview and appraisal of meta-analyses on the link between dietary carbohydrate intake and multiple health outcomes. Overall, our review examined the association of dietary carbohydrate intake with 23 outcomes, including cancer, mortality, metabolic diseases, digestive system diseases, and other outcomes. There was no association supported by convincing evidence with strong epidemiological credibility. There is evidence highly suggestive of the correlation between dietary carbohydrate intake and increased risk of metabolic syndrome. We observed suggestive evidence for the associations of carbohydrate consumption with decreased risk of esophageal adenocarcinoma and increased risk of all-cause mortality.
There is weak evidence of the dose-response correlation between dietary carbohydrate intake and increased risk of metabolic syndrome and coronary heart disease. A meta-analysis including 284,638 participants indicated that carbohydrate intake and prevalence of metabolic syndrome, with a 2.6% increase in the risk of metabolic syndrome per 5% energy intake from carbohydrates. In addition, a meta-analysis of prospective cohort studies suggested that per 98 g/d carbohydrate increment was associated with 65% increased risk of coronary heart disease.
Comparison With Other Studies and Possible Explanations
Evidence from 3 cohort and 15 case-control studies supported a risk effect of carbohydrate consumption for metabolic syndrome. So far, there are no reference values of carbohydrate intake for the prevention and treatment of metabolic syndrome. The European Food Information Council indicated that the European Food Safety Authority has provided a reference range of carbohydrate daily intake between 45 and 60% combined with reduced fat and saturated fat intake to improve metabolic risk factors for chronic disease (49). Moreover, the highly suggestive evidence was consistent with a meta-analysis of randomized controlled trials. This meta-analysis estimated the long-term (6-month or longer) effect of low-carbohydrate diets on metabolic risk factors, and indicated an association of low-carbohydrate diets with loss of weight and improvement in metabolic risk factors (50). In addition, a previous study suggested that metabolic regulation of insulin gene expression enables cells to maintain adequate stores of intracellular insulin to sustain the secretory demand. Glucose is the major physiological regulator of insulin gene expression (51) and this may partly explain the association between dietary carbohydrate intake and level of blood glucose, which is a component of metabolic syndrome. Notably, our results showed that a higher intake of carbohydrate was weakly associated with a higher risk of type 2 diabetes. Moreover, the dose-response analyses revealed a significant association between carbohydrate consumption and risk of type 2 diabetes. Similarly, low-quality evidence of an association between total carbohydrates intake and type 2 Diabetes was found in another umbrella review (22). In addition, we found evidence of excess significance bias for the association between carbohydrate intake and metabolic syndrome, which resulted in a relative excess of reported statistically significant results as compared to what would be expected in a body of evidence (29).
We found suggestive evidence of an association between higher dietary carbohydrate intake and lower risk of esophageal adenocarcinoma. The key mediators associating dietary and lifestyle factors with carcinogenesis have been reported to be hyperinsulinemia, insulin-like growth factor, and insulin resistance (52, 53). Elevated insulin levels could inhibit the development and progression of many types of cancer by suppressing insulin-like growth factor-binding protein or by increasing the production of insulin receptors (54–56). Hence, dietary carbohydrate intake could decrease the risk of oesophageal adenocarcinoma by raising the levels of insulin. Moreover, a higher intake of carbohydrate could reflect a higher consumption of plant-based foods (especially fruits and vegetables), which has been confirmed to have an inverse relationship with oesophageal cancer (57). However, there was no credible evidence of an association between carbohydrate intake and other cancer types.
Suggestive evidence was also found for the association between carbohydrate consumption and risk of all-cause mortality. This finding was consistent with that from a large perspective cohort study in 135,335 individuals from 18 countries, with a median follow-up of 7.4 years (58). Notably, another meta-analysis pooled the effect size of different geographic regions to investigate the association between carbohydrate consumption and mortality. This meta-analysis suggested an insignificant association between the intake of carbohydrates and mortality in Europe and Asia (15). Another prospective cohort study using data from the US National Health and Nutrition Examination Survey from 1999 to 2014 from 37,233 Adult, also indicated differences in the association of low-carbohydrate with mortality among non-Hispanic whites and other populations in the US (59). Moreover, a study showed that a long- standing refined grain-based diet might have contributed to trigger the evolution of biological adaptations to mitigate the side effects of diets (60). Further evidence from a genome-wide meta-analysis of observational studies revealed an association between common genetic variants and macronutrient intake (61). Thus, it is reasonable to hypothesize that genetic factors may influence the association between dietary carbohydrate intake and mortality. In future studies, the genetic variability in carbohydrate metabolism should be considered. Meanwhile, more and higher quality research will aid the development of reference values for carbohydrate intake for different ethnic groups and disease-state populations.
Strength and Limitations
This study is the first umbrella review to provide a systematic and comprehensive summary of the published literature to explore the role of carbohydrates in human health-related outcomes. This review may also help investigators to judge the relative importance of carbohydrates for various health outcomes. Moreover, we evaluated the methodological quality using AMSTAR, which offered good evidence of validity and reliability. Beyond assessing the quality of studies, we explored the extent of bias and heterogeneity among the included meta-analyses. Furthermore, we identified the low quality evidence of the association between dietary carbohydrate intake and multiple outcomes to provide directions for future research.
The findings of our work should also be interpreted in light of its limitations. Although we used systematic methods that included a robust search strategy, in many meta-analyses the authors have stated their assumption but included insufficient information to allow us to judge the suitability of the pooling. Thus, these meta-analyses with insufficient information were excluded from our review. Moreover, the umbrella review is a method of synthesizing existing evidence, which depends on the selection of the estimates from each primary study and its representation in the meta-analysis. Hence, the included individual studies with poor quality outcomes need to be interpreted with caution. Nevertheless, none of the meta-analyses included in our umbrella review had low or critically low methodological quality. Thus, bias due to quality of the studies had little effect on the results. Furthermore, this umbrella review had an observational study design, and hence bias from reverse causality and recall bias cannot be avoided. In addition, although the most important confounders were adjusted for in most of the primary studies, residual confounding cannot be completely ruled out.
Significantly, the differences of amount of comparison in each primary study may cause inaccurate evaluations of summary effects. These inevitable differences should attribute to the meta-analyses which pooled the risk estimates from the highest vs. lowest category of exposure. For example, one meta-analysis included two studies which both compared the highest quartile with the lowest quartile intake of dietary carbohydrate. However, the highest and lowest quartiles of these two studies were completely different (303 vs. 242 g/day and 162 vs. 114 g/day) (Supplementary Table 3) (33). Dose-response studies are less susceptible to such bias, but only ten Dose-response Meta-analysis Were included in the present umbrella review. Therefore, this result should be interpreted with caution. For this reason, future primary studies with dose-response analysis are needed to interpret the summary estimates more reasonably in evidence-based medicine.
Conclusions
Our study found highly suggestive evidence of the association between dietary carbohydrate intake and increased risk of metabolic syndrome, and suggestive evidence of the association of carbohydrate consumption with increased risk of mortality and decreased risk of esophageal adenocarcinoma. Notably, the evidence of the associations between carbohydrate intake and other health outcomes were weak or even non-existent. Thus, the enthusiasm of low carbohydrate diet and the anathema of low carbohydrate food should be reconsidered. Furthermore, the large heterogeneity of dietary assessment methods and the inadequacies related to the study design suggest the need for recommendations for guiding future interventions to be sufficiently powered to detect clinical outcomes. Future randomized controlled trials in large sample sizes are needed to confirm these observational findings and to study the effects of different carbohydrate subtypes.
Data Availability Statement
The original contributions presented in the study are included in the article/Supplementary Material, further inquiries can be directed to the corresponding author/s.
Author Contributions
Q-JW and Y-HZ contributed to the study design. Y-SL and J-LL conducted the literature search. Y-SL, Q-JW, and Y-TJ extracted the data and conducted the analyses. Y-SL, HS, YX, and QC wrote the first draft of the manuscript and edited the manuscript. All authors contributed to the article and approved the submitted version.
Funding
This work was supported by the National Key R&D Program of China (No. 2017YFC0907402 to Y-HZ), the Natural Science Foundation of China (No. 82073647 to Q-JW), LiaoNing Revitalization Talents Program (No. XLYC1802095 to Y-HZ and No. XLYC1907102 to Q-JW), Shenyang high level innovative talents support program (No. RC190484 to Q-JW), and 345 Talent Project of Shengjing Hospital of China Medical University (No. M0268 to Q-JW).
Conflict of Interest
The authors declare that the research was conducted in the absence of any commercial or financial relationships that could be construed as a potential conflict of interest.
Acknowledgments
We really appreciate Min Xie, Huan Zhao, Jia-Jia Bai, Jia-Yu Zhang, Ming-Zhu Xu, Jie Luan, Fang-Hua Liu, and Han-Yuan Wang for the preparation of data.
Supplementary Material
The Supplementary Material for this article can be found online at: https://www.frontiersin.org/articles/10.3389/fnut.2021.670411/full#supplementary-material
Abbreviations
NCEP, National Cholesterol Education Program; AMSTAR, A Measurement Tool to Assess Systematic Reviews; SE, standard error; CIs, confidence intervals; PIs, prediction intervals.
References
1. Cummings JH, Stephen AM. Carbohydrate terminology and classification. Eur J Clin Nutr. (2007) 61(Suppl 1):S5–18. doi: 10.1038/sj.ejcn.1602936
2. Churuangsuk C, Lean MEJ, Combet E. Low and reduced carbohydrate diets: challenges and opportunities for type 2 diabetes management and prevention. Proc Nutr Soc. (2020) 5:1–16. doi: 10.1017/S0029665120000105
3. Englyst KN, Liu S, Englyst HN. Nutritional characterization and measurement of dietary carbohydrates. Eur J Clin Nutr. (2007) 61(Suppl. 1):S19–39. doi: 10.1038/sj.ejcn.1602937
4. Hardy K, Brand-Miller J, Brown KD, Thomas MG, Copeland L. The importance of dietary carbohydrate in human evolution. Q Rev Biol. (2015) 90:251–68. doi: 10.1086/682587
5. Connor WE, Duell PB, Connor SL. Benefits and hazards of dietary carbohydrate. Curr Atheroscler Rep. (2005) 7:428–34. doi: 10.1007/s11883-005-0059-4
6. Expert Panel on Detection E, Adults ToHBCi. Executive summary of the third report of the national cholesterol education program (NCEP) expert panel on detection, evaluation, and treatment of high blood cholesterol in adults (Adult Treatment Panel III). JAMA. (2001) 285:2486–97. doi: 10.1001/jama.285.19.2486
7. Tieri M, Ghelfi F, Vitale M, Vetrani C, Marventano S, Lafranconi A, et al. Whole grain consumption and human health: an umbrella review of observational studies. Int J Food Sci Nutr. (2020) 71:668–77. doi: 10.1080/09637486.2020.1715354
8. Seidelmann SB, Claggett B, Cheng S, Henglin M, Shah A, Steffen LM, et al. Dietary carbohydrate intake and mortality: a prospective cohort study and meta-analysis. Lancet Public Health. (2018) 3:E419–28. doi: 10.1016/S2468-2667(18)30135-X
9. Sadeghi A, Sadeghian M, Nasiri M, Rahmani J, Khodadost M, Pirouzi A, et al. Carbohydrate quantity and quality affect the risk of endometrial cancer: a systematic review and dose-response meta-analysis. Clin Nutr. (2019) 39:1681–91. doi: 10.1016/j.clnu.2019.08.001
10. Huang J, Pan G, Jiang H, Li W, Dong J, Zhang H, et al. A meta-analysis between dietary carbohydrate intake and colorectal cancer risk: evidence from 17 observational studies. Bio Rep. (2017) 37:BSR20160553. doi: 10.1042/BSR20160553
11. Livesey G, Livesey H. Coronary heart disease and dietary carbohydrate, glycemic index, and glycemic load: dose-response meta-analyses of prospective cohort studies. Mayo Clin Proc Innov Qual Outcomes. (2019) 3:52–69. doi: 10.1016/j.mayocpiqo.2018.12.007
12. Alhazmi A, Stojanovski E, McEvoy M, Garg ML. Macronutrient intakes and development of type 2 diabetes: a systematic review and meta-analysis of cohort studies. J Am Coll Nutr. (2012) 31:243–58. doi: 10.1080/07315724.2012.10720425
13. Liu Y-S, Wu Q-J, Xia Y, Zhang J-Y, Jiang Y-T, Chang Q, et al. Carbohydrate intake and risk of metabolic syndrome: a dose-response meta-analysis of observational studies. Nutr Meta Cardiov Dis. (2019) 29:1288–98. doi: 10.1016/j.numecd.2019.09.003
14. Wu H, Zhang H, Li P, Gao T, Lin J, Yang J, et al. Association between dietary carbohydrate intake and dietary glycemic index and risk of age-related cataract: a meta-analysis. Invest Ophthalmol Vis Sci. (2014) 55:3660–8. doi: 10.1167/iovs.13-13695
15. Hardy DS, Garvin JT, Xu H. Carbohydrate quality, glycemic index, glycemic load and cardiometabolic risks in the US, Europe and Asia: a dose–response meta-analysis. Nutr Meta Cardiov Dis. (2020) 30:853–71. doi: 10.1016/j.numecd.2019.12.050
16. Senior AM, Nakagawa S, Raubenheimer D, Simpson SJ. Global associations between macronutrient supply and age-specific mortality. Proc Natl Acad Sci USA. (2020) 117:30824–35. doi: 10.1073/pnas.2015058117
17. Ioannidis JP. Integration of evidence from multiple meta-analyses: a primer on umbrella reviews, treatment networks and multiple treatments meta-analyses. CMAJ. (2009) 181:488–93. doi: 10.1503/cmaj.081086
18. Stroup DF, Berlin JA, Morton SC, Olkin I, Williamson GD, Rennie D, et al. Meta-analysis of observational studies in epidemiology: a proposal for reporting. Meta-analysis of observational studies in epidemiology (MOOSE) group. JAMA. (2000) 283:2008–12. doi: 10.1001/jama.283.15.2008
19. Shea BJ, Grimshaw JM, Wells GA, Boers M, Andersson N, Hamel C, et al. Development of AMSTAR: a measurement tool to assess the methodological quality of systematic reviews. BMC Med Res Methodol. (2007) 7:10. doi: 10.1186/1471-2288-7-10
20. Shea BJ, Hamel C, Wells GA, Bouter LM, Kristjansson E, Grimshaw J, et al. AMSTAR is a reliable and valid measurement tool to assess the methodological quality of systematic reviews. J Clin Epidemiol. (2009) 62:1013–20. doi: 10.1016/j.jclinepi.2008.10.009
21. Shea BJ, Bouter LM, Peterson J, Boers M, Andersson N, Ortiz Z, et al. External validation of a measurement tool to assess systematic reviews (AMSTAR). PLoS ONE. (2007) 2:e1350. doi: 10.1371/journal.pone.0001350
22. Neuenschwander M, Ballon A, Weber KS, Norat T, Aune D, Schwingshackl L, et al. Role of diet in type 2 diabetes incidence: umbrella review of meta-analyses of prospective observational studies. BMJ. (2019) 366:l2368. doi: 10.1136/bmj.l2368
23. Higgins JP, Thompson SG, Spiegelhalter DJ. A re-evaluation of random-effects meta-analysis. J R Stat Soc Ser A Stat Soc. (2009) 172:137–59. doi: 10.1111/j.1467-985X.2008.00552.x
24. IntHout J, Ioannidis JP, Rovers MM, Goeman JJ. Plea for routinely presenting prediction intervals in meta-analysis. BMJ Open. (2016) 6:e010247. doi: 10.1136/bmjopen-2015-010247
25. Belbasis L, Bellou V, Evangelou E, Ioannidis JP, Tzoulaki I. Environmental risk factors and multiple sclerosis: an umbrella review of systematic reviews and meta-analyses. Lancet Neurol. (2015) 14:263–73. doi: 10.1016/S1474-4422(14)70267-4
26. Ioannidis JP, Patsopoulos NA, Evangelou E. Uncertainty in heterogeneity estimates in meta-analyses. BMJ. (2007) 335:914–6. doi: 10.1136/bmj.39343.408449.80
27. Egger M, Davey Smith G, Schneider M, Minder C. Bias in meta-analysis detected by a simple, graphical test. BMJ. (1997) 315:629–34. doi: 10.1136/bmj.315.7109.629
28. Chinn S. A simple method for converting an odds ratio to effect size for use in meta-analysis. Stat Med. (2000) 19:3127–31. doi: 10.1002/1097-0258(20001130)19:22&<3127::AID-SIM784&>3.0.CO;2-M
29. Ioannidis JP, Trikalinos TA. An exploratory test for an excess of significant findings. Clin Trials. (2007) 4:245–53. doi: 10.1177/1740774507079441
30. Lubin JH, Gail MH. On power and sample size for studying features of the relative odds of disease. American J Epidemiol. (1990) 131:552–66. doi: 10.1093/oxfordjournals.aje.a115530
31. Kalliala I, Markozannes G, Gunter MJ, Paraskevaidis E, Gabra H, Mitra A, et al. Obesity and gynaecological and obstetric conditions: umbrella review of the literature. BMJ. (2017) 359:j4511. doi: 10.1136/bmj.j4511
32. Cai X, Li X, Tang M, Liang C, Xu Y, Zhang M, et al. Dietary carbohydrate intake, glycaemic index, glycaemic load and digestive system cancers: an updated dose-response meta-analysis. Br J Nutr. (2019) 121:1081–96. doi: 10.1017/S0007114519000424
33. Aune D, Chan DSM, Lau R, Vieira R, Greenwood DC, Kampman E, et al. Carbohydrates, glycemic index, glycemic load, and colorectal cancer risk: a systematic review and meta-analysis of cohort studies. Can Causes Contr. (2012) 23:521–35. doi: 10.1007/s10552-012-9918-9
34. Aune D, Chan DSM, Vieira AR, Rosenblatt DAN, Vieira R, Greenwood DC, et al. Dietary fructose, carbohydrates, glycemic indices and pancreatic cancer risk: a systematic review and meta-analysis of cohort studies. Ann Oncol. (2012) 23:2536–46. doi: 10.1093/annonc/mds076
35. Banda KJ, Chiu HY, Hu SH, Yeh HC, Lin KC, Huang HC. Associations of dietary carbohydrate and salt consumption with esophageal cancer risk: a systematic review and meta-analysis of observational studies. Nutr Rev. (2020) 78:688–98. doi: 10.1093/nutrit/nuz097
36. Chen YH, Nan CJ, Fan LL, Su HX, Gu XJ. Carbohydrate intake and the risk of prostate cancer. Clin Chim Acta. (2018) 484:60–71. doi: 10.1016/j.cca.2018.05.032
37. Schlesinger S, Chan DSM, Vingeliene S, Vieira AR, Abar L, Polemiti E, et al. Carbohydrates, glycemic index, glycemic load, and breast cancer risk: a systematic review and dose-response meta-analysis of prospective studies. Nutr Rev. (2017) 75:420–41. doi: 10.1093/nutrit/nux010
38. Wang R-j, Tang J-e, Chen Y, Gao J-g. Dietary fiber, whole grains, carbohydrate, glycemic index, and glycemic load in relation to risk of prostate cancer. Oncotargets Therapy. (2015) 8:2415–26. doi: 10.2147/OTT.S88528
39. Xuan F, Li W, Guo X, Liu C. Dietary carbohydrate intake and the risk of esophageal cancer: a meta-analysis. Bio Rep. (2020) 40:BSR20192576. doi: 10.1042/BSR20192576
40. Ye Y, Wu Y, Xu J, Ding K, Shan X, Xia D. Association between dietary carbohydrate intake, glycemic index and glycemic load, and risk of gastric cancer. Eur J Nutr. (2017) 56:1169–77. doi: 10.1007/s00394-016-1166-4
41. Zhai L, Cheng S, Zhang D. Dietary carbohydrate and prostate cancer risk: a meta-analysis. Nutr Can Int J. (2015) 67:594–602. doi: 10.1080/01635581.2015.1019639
42. Cai X, Wang C, Wang S, Cao G, Jin C, Yu J, et al. Carbohydrate intake, glycemic index, glycemic load, and stroke: a meta-analysis of prospective cohort studies. Asia Pacific J Public Health. (2015) 27:486–96. doi: 10.1177/1010539514566742
43. Greenwood DC, Threapleton DE, Evans CEL, Cleghorn CL, Nykjaer C, Woodhead C, et al. Glycemic index, glycemic load, carbohydrates, and type 2 diabetes systematic review and dose-response meta-analysis of prospective studies. Diab Care. (2013) 36:4166–71. doi: 10.2337/dc13-0325
44. Jin Z-Q, Lu H-G, Wu Q-B, Ge H-X, Zhou T-T, Zhang X-Q, et al. A meta-analysis of dietary carbohydrate intake and inflammatory bowel disease risk: evidence from 15 epidemiology studies. Rev Espanola De Enfer Diges. (2019) 111:5–9. doi: 10.17235/reed.2018.5490/2018
45. Wang F, Feng J, Gao Q, Ma M, Lin X, Liu J, et al. Carbohydrate and protein intake and risk of ulcerative colitis: systematic review and dose-response meta-analysis of epidemiological studies. Clin Nutr. (2017) 36:1259–65. doi: 10.1016/j.clnu.2016.10.009
46. Zeng L, Hu S, Chen P, Wei W, Tan Y. Macronutrient intake and risk of crohn's disease: systematic review and dose-response meta-analysis of epidemiological studies. Nutrients. (2017) 9:500. doi: 10.3390/nu9050500
47. Mozaffari H, Daneshzad E, Azadbakht L. Dietary carbohydrate intake and risk of bone fracture: a systematic review and meta-analysis of observational studies. Public Health. (2020) 181:102–9. doi: 10.1016/j.puhe.2019.12.001
48. Wang A, Lin Y, Wu Y, Zhang D. Macronutrients intake and risk of Parkinson's disease: a meta-analysis. Geriatr Gerontol Int. (2015) 15:606–16. doi: 10.1111/ggi.12321
49. Council TEFI. New Nutrition Guidelines for Europe, Halfway There. (2011). Available online at: https://www.eufic.org/en/healthy-living/article/new-nutrition-guidelines-for-europe-halfway-there (accessed September 3, 2020).
50. Hu T, Mills KT, Yao L, Demanelis K, Eloustaz M, Yancy WS, et al. Effects of low-carbohydrate diets versus low-fat diets on metabolic risk factors: a meta-analysis of randomized controlled clinical trials. Am J Epidemiol. (2012) 176:S44–54. doi: 10.1093/aje/kws264
51. Poitout V, Hagman D, Stein R, Artner I, Robertson RP, Harmon JS. Regulation of the insulin gene by glucose and fatty acids. J Nutr. (2006) 136:873–6. doi: 10.1093/jn/136.4.873
52. Renehan AG, Zwahlen M, Minder C, O'Dwyer ST, Shalet SM, Egger M. Insulin-like growth factor (IGF)-I, IGF binding protein-3, and cancer risk: systematic review and meta-regression analysis. Lancet. (2004) 363:1346–53. doi: 10.1016/S0140-6736(04)16044-3
53. Montesi L, Mazzotti A, Moscatiello S, Forlani G, Marchesini G. Insulin resistance: mechanism and implications for carcinogenesis and hepatocellular carcinoma in NASH. Hepatol Int. (2013) 7(Suppl. 2):814–22. doi: 10.1007/s12072-013-9451-2
54. Gong Y, Ma Y, Sinyuk M, Loganathan S, Thompson RC, Sarkaria JN, et al. Insulin-mediated signaling promotes proliferation and survival of glioblastoma through Akt activation. Neuro Oncol. (2016) 18:48–57. doi: 10.1093/neuonc/nov096
55. Pollak M. Insulin and insulin-like growth factor signalling in neoplasia. Nat Rev Cancer. (2008) 8:915–28. doi: 10.1038/nrc2536
56. Gao J, Chang YS, Jallal B, Viner J. Targeting the insulin-like growth factor axis for the development of novel therapeutics in oncology. Cancer Res. (2012) 72:3–12. doi: 10.1158/0008-5472.CAN-11-0550
57. Li B, Jiang G, Zhang G, Xue Q, Zhang H, Wang C, et al. Intake of vegetables and fruit and risk of esophageal adenocarcinoma: a meta-analysis of observational studies. Eur J Nutr. (2014) 53:1511–21. doi: 10.1007/s00394-014-0656-5
58. Dehghan M, Mente A, Zhang X, Swaminathan S, Li W, Mohan V, et al. Associations of fats and carbohydrate intake with cardiovascular disease and mortality in 18 countries from five continents (PURE): a prospective cohort study. Lancet. (2017) 390:2050–62. doi: 10.1016/S0140-6736(17)32252-3
59. Shan Z, Guo Y, Hu FB, Liu L, Qi Q. Association of low-carbohydrate and low-fat diets with mortality among US adults. JAMA Intern Med. (2020) 180:513–23. doi: 10.1001/jamainternmed.2019.6980
60. Landini A, Yu S, Gnecchi-Ruscone GA, Abondio P, Sazzini M. Genomic adaptations to cereal-based diets contribute to mitigate metabolic risk in some human populations of east asian ancestry evolutionary applications. Evol Appl. (2020) 14:297–313. doi: 10.1111/eva.13090
Keywords: carbohydrate, dietary, health outcomes, meta-analysis, umbrella review
Citation: Liu Y-S, Wu Q-J, Lv J-L, Jiang Y-T, Sun H, Xia Y, Chang Q and Zhao Y-H (2021) Dietary Carbohydrate and Diverse Health Outcomes: Umbrella Review of 30 Systematic Reviews and Meta-Analyses of 281 Observational Studies. Front. Nutr. 8:670411. doi: 10.3389/fnut.2021.670411
Received: 21 February 2021; Accepted: 24 March 2021;
Published: 29 April 2021.
Edited by:
Giuseppe Grosso, NNEdPro Global Centre for Nutrition and Health, United KingdomReviewed by:
Justyna Godos, University of Catania, ItalyÁngela Hernández-Ruiz, Fundación Iberoamericana de Nutrición (FINUT), Spain
Copyright © 2021 Liu, Wu, Lv, Jiang, Sun, Xia, Chang and Zhao. This is an open-access article distributed under the terms of the Creative Commons Attribution License (CC BY). The use, distribution or reproduction in other forums is permitted, provided the original author(s) and the copyright owner(s) are credited and that the original publication in this journal is cited, in accordance with accepted academic practice. No use, distribution or reproduction is permitted which does not comply with these terms.
*Correspondence: Yu-Hong Zhao, zhaoyuhong@sj-hospital.org
†These authors have contributed equally to this work