- MAS Microbiota Group, School of Biomedical and Health Sciences, Universidad Europea de Madrid, Madrid, Spain
A high intake of dietary saturated fatty acids (SFAs) is related to an increased risk of obesity, inflammation and cancer-related diseases, and this risk is attenuated only when SFAs are replaced by unsaturated fats and unrefined carbohydrates. The gut microbiota has recently emerged as a new environmental factor in the pathophysiology of these disorders, and is also one of the factors most influenced by diet. We sought to determine whether the gut microbiota of healthy individuals whose intake of SFAs exceeds World Health Organization (WHO) recommendations exhibits features similar to those reported in people with obesity, inflammation, cancer or metabolic disease. Healthy non-obese subjects were divided into two groups based on their SFAs intake. Body composition and gut microbiota composition were analyzed, and associations between bacterial taxa, diet and body fat composition were determined globally and separately by sex. Metagenome functional pathways were predicted by PICRUSt analysis. Subjects whose SFAs intake exceeded WHO recommendations also had a dietary pattern of low fiber intake. This high saturated fat/low fiber diet was associated with a greater sequence abundance of the Anaerotruncus genus, a butyrate producer associated with obesity. Analysis of data of high SFAs intake by sex showed that females presented with a greater abundance of Campylobacter, Blautia, Flavonifractor and Erysipelatoclostridium, whereas males showed higher levels of Anaerotruncus, Eisenbergiella, a genus from the order Clostridiales (FamilyXIIIUCG_001) and two genera from the Lachnospiraceae family. PICRUSt analysis confirmed these data, showing a correlation with a decrease in the abundance of sequences encoding for transporters of some metals such as iron, which is needed to maintain a healthy metabolism. Thus, the microbiota of healthy people on a high SFAs diet contain bacterial taxa (Anaerotruncus, Lachnospiraceae Flavonifractor, Campylobacter, Erysipelotrichacea and Eisenbergiella) that could be related to the development of some diseases, especially obesity and other pro-inflammatory diseases in women. In summary, the present study identifies bacterial taxa that could be considered as early predictors for the onset of different diseases in healthy subjects. Also, sex differences in gut microbiota suggest that women and men differentially benefit from following a specific diet.
Introduction
An unbalanced or unhealthy diet is associated with the onset of several diseases. For example, a diet rich in red meat has been linked to colorectal cancer, and there is strong evidence for the involvement of some dietary fats in weight increase and cardiovascular disease (CVD) (1). The increased intake of saturated fatty acids (SFAs) is associated with higher levels of low-density lipoprotein (LDL) in serum (2) and elevated oxidative stress, which can play a significant role in cancer progression and colonic inflammation (3). There is also evidence to suggest that decreasing the intake of SFAs and replacing them with simple carbohydrates does not reduce CVD incidence, and only when SFAs are replaced by unsaturated fats (mainly polyunsaturated) is a decrease in CVD incidence observed (4). The World Health Organization (WHO) recommends that 30% or less of the total energy intake in adults should come from dietary lipids, with SFAs comprising <10% and trans-fats <1% (5, 6). However, the last few decades have seen a significant increase in the consumption of processed and ultra-processed food products, which are rich in fats, particularly SFAs. Indeed, a recent cross-sectional study performed in a nationally representative Spanish population found that total fat intake exceeded 38% of the total energy intake, with SFAs accounting for over 11.5% (7). Moreover, a high-fat diet (HFD) in humans is associated with a reduced consumption of carbohydrates, especially those that constitute the dietary fiber, and the average fiber intake rarely reaches the daily recommendation of >25 g/d (8).
Accumulating data suggest that the relationship between our diet and our health is mediated, at least in part, by the gut microbiota, which has recently emerged as an influential factor in many diseases. Imbalances in the gut microbiota (termed dysbiosis) are associated with colon cancer, metabolic syndrome, diabetes, and cardiovascular events, among others (9). Among the known factors that can shape the gut microbiota, dietary components are one of the most important (10), and several studies have shown that HFD modifies the gut microbiota of both animals and humans. For example, HFD-fed mice show increases in the abundance of Firmicutes, Proteobacteria and Actinobacteria, and concomitant reductions in health-promoting bacteria such as those of the Bacteroidetes phylum, and Bifidobacterium and Akkermansia genera (11–15). Additionally, HFD diminishes microbial richness and increases the abundance of Gram-negative bacteria, which can enhance intestinal permeability (16). In turn, this can lead to the translocation of lipopolysaccharide (LPS) to the systemic circulation (17), triggering low-grade inflammation (18, 19). HFD consumption in humans results in loss of bacterial diversity (20), with a higher abundance of Bilophila, Alistipes, Blautia and several genera of the Gammaproteobacteria class, and a lower abundance of Bacteroides, Clostridium, and Roseburia spp. (21, 22). Importantly, not only is the quantity of fat important, but also the type. Animal studies indicate that whereas SFAs have a detrimental effect on gut microbiota, weight gain, intestinal permeability and proinflammatory status, monounsaturated and polyunsaturated fats can have beneficial effects on the host microbial ecosystem, body weight loss and anti-inflammatory response (23). To date, however, very few studies have explored the relationship between SFAs intake and gut microbiota. In light of the above evidence, the present study was designed to compare the microbiota features of subjects whose SFAs intake exceeded WHO recommendations with those who consumed recommended amounts of SFAs. We studied the microbiota composition of healthy non-obese men and women to explore features that could precede the development of some chronic disorders in a healthy population. We analyzed all data by sex, as the parameters of body composition, dietary requirements predisposition, incidence of diseases and associated microbiota dysbiosis differs between men and women (24).
Materials and Methods
Study Design and Subjects
This was an observational cross-sectional study of healthy individuals. Volunteers were recruited using posters, social networks, and magazines. The study was carried out at Universidad Europea de Madrid (Madrid, Spain), and was limited to men and women aged 18–45 years and with a body mass index (BMI) of 18.5–25 kg/m2. Exclusion criteria were the following: any kind of pathology (during the study or in the 6 months prior to it), previous gastrointestinal surgery, antibiotics intake or modification of diet during 3 months prior to the study, intake of any drug (with the exception of contraceptive pills) 1 month before the study, smoking, use of prebiotics or probiotics, vegetarian or vegan diets, nutritional or ergogenic complements, pregnancy, or lactation. All subjects were Caucasian. A total of 109 volunteers were recruited and gave written and informed consent prior to participation. According to WHO recommendations on SFAs intake, which should be <10% of total energy intake, volunteers were divided into two groups based on the results of a validated food frequency questionnaire (FFQ): low SFA intake (LSFA), when intake was <9% of total energy, and high SFA intake (HSFA) when is more than 11% of total energy (6). Volunteers with intermediate (9–11%) intake of SFAs were not considered for the study (Supplementary Figure 1). The Ethics Committee for Clinical Research of the Hospital Ramón y Cajal (Madrid, Spain) approved the study (protocol #CEIC 338-17).
Anthropometry and Body Composition
Height and weight were measured with a stadiometer (Asimed T2, Barcelona, Spain) and a balance scale (Año Sayol SL, Barcelona, Spain), respectively; and body mass index (BMI) was calculated as weight (kg) divided by height (m2). Body composition was evaluated on the day of sample collection by dual-energy X ray absorptiometry (DEXA) (Hologic DEXA scan, Hologic Inc, Barcelona, Spain). The following body composition measurements were collected: estimated visceral adipose tissue (VAT), body fat percentage (BFP), body fat mass (BFM), total lean mass and fat and lean mass distribution in the trunk and extremities. The following indices were calculated using the obtained values: adiposity index (AI) = total fat/height2; lean mass index (LMI) = total lean mass/height2; and appendicular lean mass index (AppLMI) = lean mass in arms + legs/height2.
Stool Collection and Bacterial DNA Extraction
Participants were provided with an Fe-Col® Fecal Sample Collection Kit (Alpha Laboratories Ltd., Eastleigh, Hampshire, UK) and an icebox and a cooler to maintain the samples at −20°C until they reached the laboratory where they were aliquoted and conserved at −80°C. Bacterial DNA was extracted using the E.Z.N.A.® Stool DNA Kit (Omega-Biotek, Norcross, GA) and a bead-beating homogenizer (Bullet Blender Storm, Next Advance, Averill Park, NY). Concentration and purity of DNA were measured on a Nanodrop 1000 spectrophotometer (ThermoFisher Scientific, Waltham, MA).
Food Frequency Questionnaire
Dietary pattern assessment was carried out using a validated FFQ with 93 food items (25). The FFQ was given to participants to complete on the day they donated the fecal samples. Data from the FFQ were analyzed using Dietsource software 3.0 (Novartis, Barcelona, Spain) to obtain the total amount of carbohydrates, protein, fats and fiber ingested, and the total energy ingested.
Sequencing and Bioinformatics
The hypervariable regions V3 and V4 of the bacterial 16S rRNA gene were amplified using the primer pair 5′-TCGTCGGCAGCGTCAGATGTGTATAAGAGACAG-3′ and 5′ GTCTCGTGGGCTCGGAGATGTGTATAAGAGACAG-3′ (26). The 459-bp amplicon was visualized in an ethidium bromide-stained 0.8% agarose gel, and bands were excised and cleaned using the MinElute Gel Extraction Kit (Qiagen, Hilden, Germany). DNA amplicons were sequenced on a MiSeq Illumina platform (Illumina, San Diego, CA). Sequence outputs were analyzed using the Quantitative Insights into Microbial Ecology (QIIME2) program v18.2, April 2018 (27). The 16S paired reads were imported in QIIME2 and processed with the DADA2 plugin (28), adjusting the maximum expected error threshold to 1.0 (29) and filtering chimera with the consensus method. Taxonomy classification was assigned with the q2-feature-classifier plugin (30) using the BLAST+ method (31) with the following settings: reference sequences SILVA (32, 33) database release 132 clustered at 97% sequence similarity; reference taxonomy label SILVA taxonomy 7 levels; maximum number of hits to keep for each query, 1; reject match if percent identity to query is lower than 0.9. Diversity analyses were conducted using the q2-diversity plugin in QIIME2. Beta-diversity was evaluated by calculating Bray-Curtis, Jaccard, unweighted and weighted Unifrac distance metrics (34). To study alpha diversity, observed operational taxonomic units (OTUs), evenness, and Shannon and Faith's phylogenetic diversity indices were calculated.
Kyoto Encyclopedia of Genes and Genomes (KEGG) ortholog abundance predictions were obtained with PICRUSt2 (35) software (Phylogenetic Investigation of Communities by Reconstruction of Unobserved States) using the default “max parsimony” method for hidden-state prediction and a NSTI (Nearest Sequenced Taxon Index) value of 0.1.
Statistical Analysis
Statistical analysis was carried out using QIIME2 v18.2, SPSS software 22.0 (SPSS, Chicago, IL) and the R statistical package 3.5.2. Variable normal distribution was assessed using the Shapiro-Wilk test. When normal distribution was not assumed, non-parametric tests were performed. Intergroup comparisons of variables were performed with a t-test or the Kruskal-Wallis test. Linear discriminant analysis coupled with effect size (LEfSe v1.0) was performed to identify bacterial taxa differentially represented between groups at species or higher taxonomy levels (36). Significance was set initially at p < 0.05 and when correlation analyses were performed, data were corrected with the Benjamini-Hochberg false discovery rate (FDR); FDR-adjusted p-values or q-values < 0.3 were considered significant. Linear regression analysis was performed for those microbial taxa that were significantly different between high and low SFAs consumption. The stepwise backward elimination method was used with the covariates that were significantly different between groups, considering diet variables (carbohydrates, protein, fats, SFAs, mono- and polyunsaturated fatty acids [MUFAs and PUFAs, respectively], ratio SFAs/unsaturated fat and fiber). Multiple regression models were built when multiple variables were predictive.
Results
Subjects and Dietary Habits
The study design flow-chart is shown in Supplementary Figure 1. Of the 109 volunteers recruited, 71 met the inclusion criteria and included 37 men and 34 women (S1 aged between 22 and 43 years. Among the men, 17 followed a diet low in SFAs (LSFA-M, SFA (%) = 7.49 ± 1.0) and 20 followed a diet high in SFAs (HSFA-M, SFA (%) = 12.62 ± 1.30). Among the women, 18 followed a diet low in SFAs (LSFA-W, SFA (%) = 7.12 ± 1.31) and 16 a diet high in SFAs (HSFA-W, SFA (%) = 12.25 ± 1.01) (Table 1).
Data on macronutrients and food intake extracted from the FFQ are shown in Table 1 and Supplementary Table 1, respectively. No significant differences between the groups were found for energy or protein intake. Dietary reports of the LSFA groups (both men and women) revealed a balanced diet for carbohydrates and fats, in accordance with recommendations for 50–60% carbohydrates and 30–35% fat, although protein intake was above the recommended dose (10–15% proteins). Dietary reports of the HSFA groups revealed that total fat intake was above the recommended dose and carbohydrates intake was below (Table 1). Fiber intake was significantly lower in the HSFA group than in the LSFA group (p < 0.01) and was below WHO recommendations (25 g/d). Likewise, the consumption of vegetables, fruits, and nuts was significantly lower in the HSFA group than in the LSFA group, whereas the consumption of potatoes was significantly higher (Supplementary Table 1). Correlation analyses of dietary variables showed a positive association between intake of saturated fats and intake of total fat (R = 0.866, q < 0.001), MUFAs (R = 0.792, q < 0.001), PUFAs (R = 0.755, q < 0.001), foods containing saturated fats (dairy products, R = 0.370, q = 0.004; chocolate, R = 0.379, q = 0.02; and red meat, R = 0.493, q < 0.001), and also potatoes (R = 0.359, q = 0.004), potato chips (R = 0.571, q = 0.007), and white bread (R = 0.359, q = 0.001). Saturated fat intake was negatively associated with the intake of fiber (R = −0.503, q < 0.001), fruits (R = −0.489, q < 0.001), vegetables (R = −0.313, q = 0.008), and nuts (R = −0.279, q = 0.03).
Dietary habits were also analyzed by sex. Total fat consumption was significantly higher (double) in the HSFA-M (male) group than in the LSFA-M group (p < 0.01). Likewise, the intake of MUFAs was significantly higher in the HSFA-M group, whereas no differences were found for PUFAs intake (Table 1). Analysis of food consumption showed a significantly higher intake of potatoes, red meat and processed meat in the HSFA-M group than in the LSFA-M group (Supplementary Table 1), whereas the opposite was seen for fruit intake (Supplementary Table 1). For women, significant differences were found in the energy provided by diet (p = 0.01), which was higher in the LSFA-W group than in the HSFA-W group. The LSFA-W group also had a higher intake of carbohydrates (p < 0.001) and fiber (p < 0.001). Differences were also found in relation to the percentages of the energy provided by total fat and the percentages of the different types of fat, with the consumption of all being significantly greater in women following an HSFA diet (Table 1). In contrast to the results for men, no differences were found in the consumption of red and processed meat in the women SFA groups, but the intake of fruits and vegetables and fish were significantly lower in the HSFA-W group than in the LFSA-W group (p = 0.03) (Supplementary Table 1).
As body composition differs between in men and women, body composition data were stratified for sex. While no significant differences in body mass were found between groups, BMI was significantly different between the HSFA-M and LSFA-M groups (Supplementary Table 2). Parameters related to body fat composition, BFP, VAT and AI, were all significantly higher in the HSFA-M group, whereas no differences were found in relation to lean mass. No significant differences were found in the body composition parameters of women (Supplementary Table 2).
Fecal Microbiota
Analysis of the sequencing data showed that average number of reads per sample was 26,890. Rarefaction curves based on observed species, Shannon, and phylogenetic distance measures were virtually saturated, indicating sufficient sequencing depth (data not shown). There were no significant differences in alpha-diversity parameters between the LSFA and HSFA groups: observed OTUs (p = 0.872), Shannon (p = 0.279), evenness (p = 0.052) or Faith's phylogenetic diversity (p = 0.774). There was, however, a trend toward significance for evenness, which would indicate probable differences in taxa abundance distribution between the groups. Also, no significant differences were observed for beta-diversity (Bray-Curtis p = 0.139; Jaccard p = 0.137; unweighted p = 0.215 and weighted Unifrac p = 0.741, distance metrics). When data were analyzed by sex, no significant differences between groups (HSFA-M, LSFA-M, HSFA-W and LSFA-W) were found for alpha- or beta-diversity parameters. Likewise, no significant differences were found when the ratio Firmicutes/Bacteroidetes (F/B ratio) of the HSFA and LSFA groups were compared (p = 0.639), or when data were analyzed by sex (HSFA-M F/B ratio = 0.60 ± 0.33, LSFA-M F/B ratio = 0.79 ± 0.33 p = 0.120; HSFA-W F/B ratio = 1.27 ± 0.63, LSFA-W F/B ratio = 1.32 ± 0.77 p = 0.866). Significant associations were found for the entire population when the F/B ratio was considered: a positive association was found between this ratio and the total percentage of body fat (R = 0.389, p = 0.02), and a negative association was found for body composition lean mass (R = −0.429, p = 0.001).
LEfSe analysis of the entire population studied (considering both men and women) at the genus level showed an increase in the abundance of sequences belonging to Blautia, Lactobacillus, Flavonifractor, Anaerotruncus genera and an uncultured genus of the Ruminoccococeae family in the HSFA group, whereas the microbiota of the LSFA group was enriched for a genus from the Ruminococcaceae family (Ruminococcaceae UCG014), Saccharimonadales, Patescibacteria and an uncultured genus of the Saccharimonadaceae family (Figure 1).
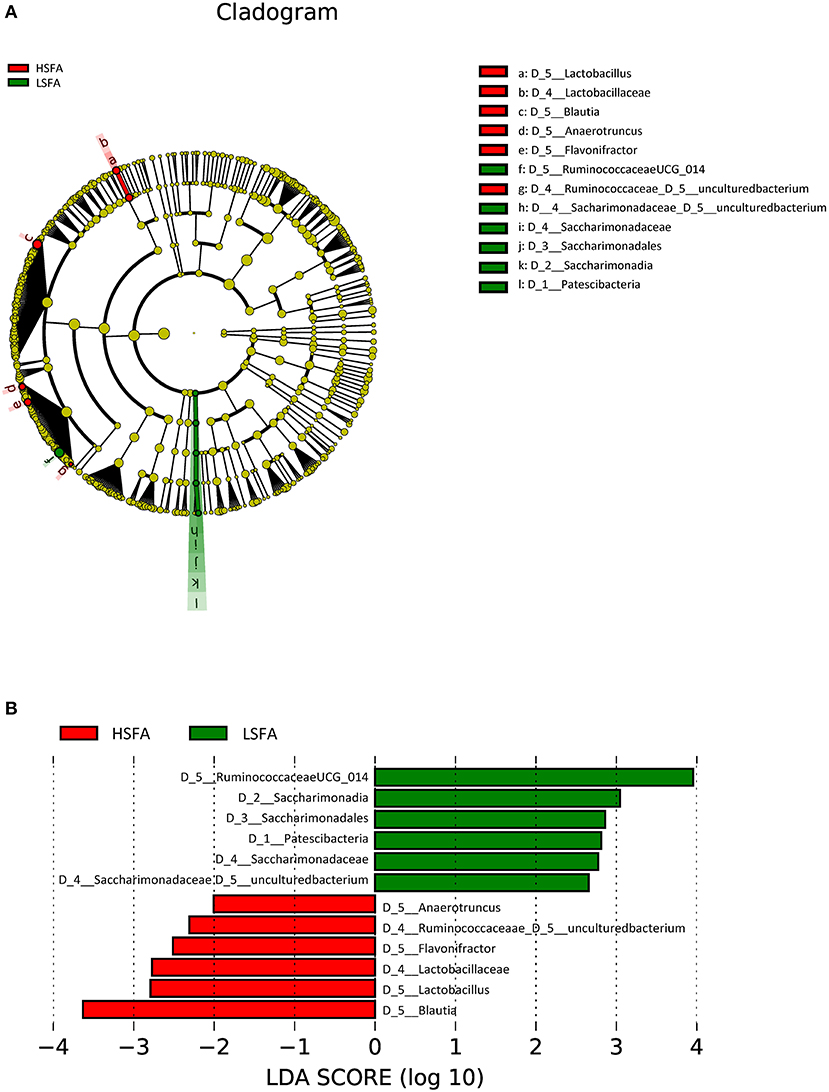
Figure 1. Cladogram (A) and histogram (B) representation of differentially abundant genera between LSFA and HSFA groups. The central point represents the root of the tree (Bacteria), and each ring represents the next lower taxonomic level (phylum through genera). The diameter of each circle represents the relative abundance of the taxon; Histogram of LDA score, only the taxa with a significant LDA threshold value above 2 are shown.
Analysis by sex revealed that the predominant taxa for the HSFA-M group included an uncultured genus from the Lachnospiraceae family, the genera Anaerotruncus, Eisenbergiella, a genus from the order Clostridiales (FamilyXIIIUCG_001), and the GCA_900066575 genus from the Lachnospiraceae family, whereas for the LSFA-M group the preponderant taxa at the genus level were a genus from the Ruminococcaceae family (Ruminococcaceae UCG_014), an uncultured genus of the Flavobacteriaceae family, and Dialister, Anaerostipes, Acetitomaculum, and Veillonella genera (Figure 2A). The highest LDA score for the HSFA-M group was an unidentified genus of the Lachnospiraceae family, whereas the highest score in the LSFA-M group was for an unknown genus of the Ruminococcaceae family (Figure 2B).
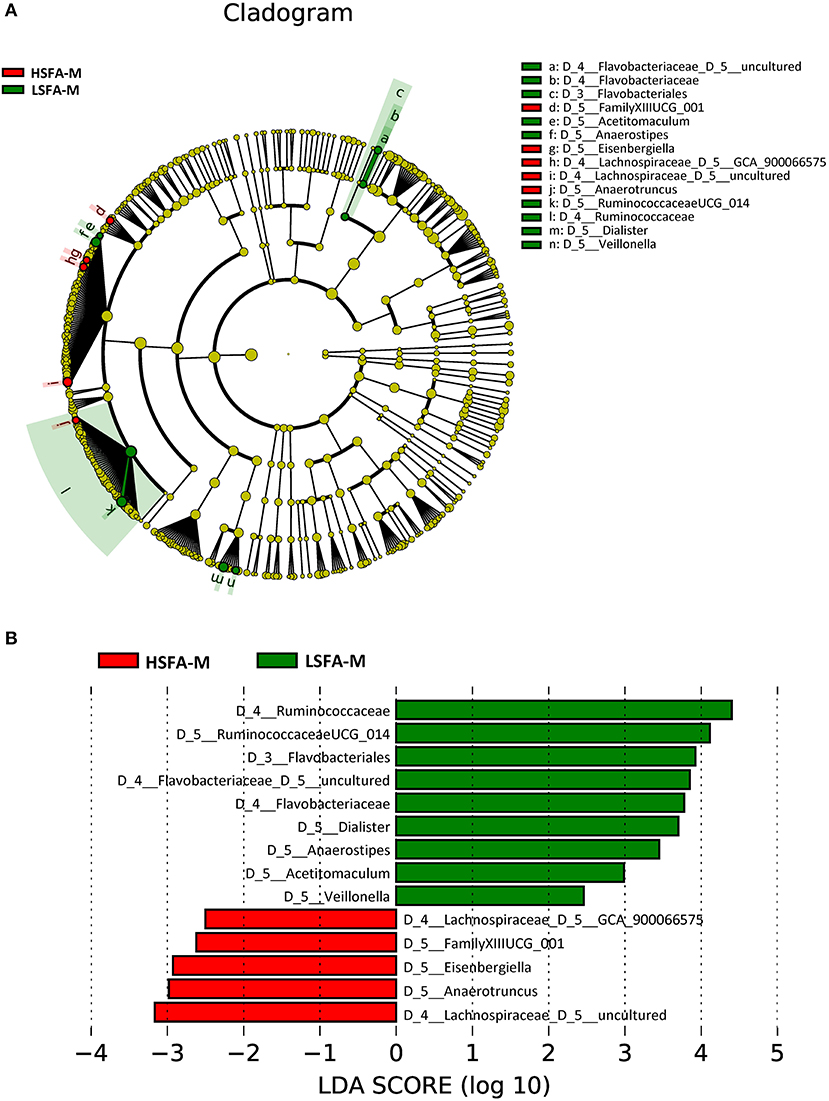
Figure 2. Cladogram (A) and histogram (B) representation of differentially abundant genera in men, between LSFA-M and HSFA-M groups.
Analysis of the microbiota from the HSFA-W group revealed an enrichment of the phylum Epsilobacteraeota, specifically the Campylobacter genus. In addition, there was a higher representation of Blautia, Erysipelatoclostridium, Flavonifractor, a genus from the Ruminococcaceae family (Ruminiclostridium 5), Porphyromonas, and Faecalitalea in the HSFA-W group (Figure 3). By contrast, the LSFA-W group showed a greater abundance of Christensenellaceae (R_7) and Ruminococcaceae (UCG_003) genera, and an uncultured rumen bacterium of the vadinBE97 family (Figure 3). The highest LDA scores were for Blautia in the HSFA-W group, and for an uncultured genus of the Christensenellaceae family in the LSFA-W group (Figure 3).
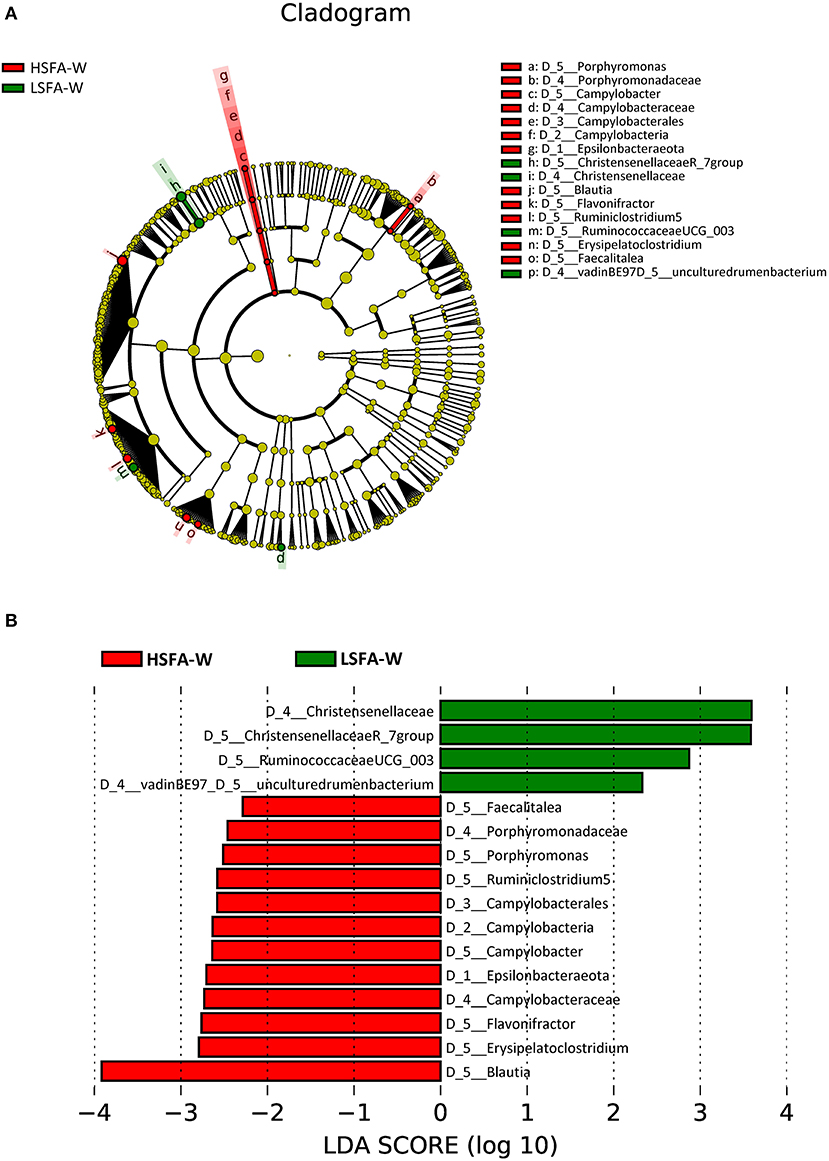
Figure 3. Cladogram (A) and histogram (B) representation of differentially abundant genera in women between LSFA-W and HSFA-W groups.
Correlation of Gut Microbial Composition and Diet
Non-parametric bivariate correlation analysis was performed to study the association between diet and gut microbiota. Spearman's rank heatmap of gut microbiota and diet (Figure 4) showed significant correlations for six genera in men, 10 genera in women, and five genera when men and women were studied altogether, according to SFAs consumption. For the entire population studied, Anaerotruncus, Flavonifractor and Blautia correlated negatively with carbohydrates and positively with total fat and SFAs, with the opposite found for Ruminococcaceae (UCG.014) (Figure 4A). For men, SFAs consumption correlated negatively with Anaerostipes, Veillonella, Ruminococcaceae (UCG.014) and positively with Anaerotruncus (Figure 4B). Moreover, positive correlations were found in women between fat (PUFAs, MUFAs, SFAs and total fat) and the genera Faecalitalea, Erisipelatoclostridium, Blautia, Flavonifractor, a genus from the Ruminococcaceae family (Ruminiclostridium 5) and Campylobacter, whereas negative correlations were found between fat and Christensenellaceae (R_7 group), Ruminococcaceae (UCG-00), and an uncultured genus of vadinBE97 (Figure 4C).
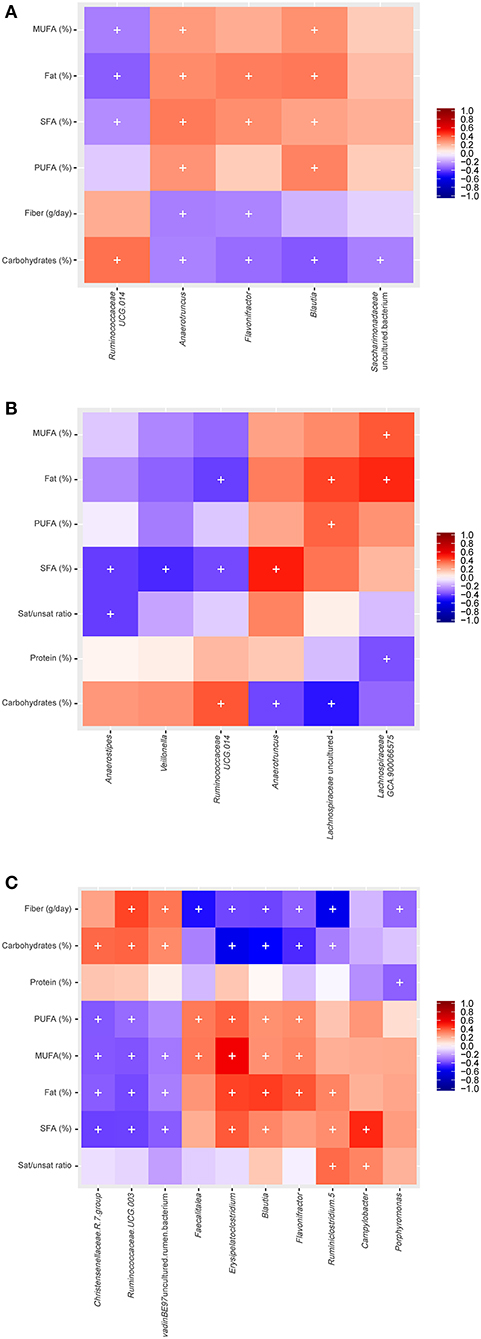
Figure 4. Spearman correlation between gut microbiota and diet for the studied groups: (A) Both men and women (B) men (C) women (+: p ≤ 0.05; p.adj < 0.3, BH correction).
Multiple Regression Analysis
Multiple regression analysis was performed to evaluate the influence of variables (diet and body composition) on differences found in microbial taxa (Tables 2–4). The presence of Anaerotruncus was positively explained by the amount of SFAs in diet, the abundance of Blautia was partly due to consumption of PUFAs, and the presence of Flavonifractor by total fats. Carbohydrates intake partially explained, either positively or negatively, the presence of Ruminococcaceae (UCG014), Saccharimonadaceae genus, Flavonifractor, and Blautia in the HSFA and LSFA groups (Table 2). For men, Anaerotruncus was positively and partially explained by SFAs, whereas Dialister and Anaerostipes were negatively explained by SFAs, and Flavobacteriaceae by red meat. The intake of carbohydrates was positively related to the presence of Ruminococcaceae (UCG014) and Dialister, the latter also being linked to the intake of fiber and fruits. The related Ruminococcaceae bacterial taxa enriched in the HSFA-M group were explained by MUFAs intake. Adiposity body parameters partially predicted the presence of Veillonella and Eisenbergiella and Lachnospiraceae (GCA-900066575) (Table 3). For women, total energy intake and fiber had a positive impact on Ruminococcaceae (UCG-003) genus and the vadinBE97.5 family, whereas carbohydrates had a negative relation with Flavonifractor, Blautia and Erysipelatoclostridium. SFAs explained, in part, the presence of Campylobacter, whereas the differences in Blautia, Flavonifractor and Erysipelatoclostridium were explained by the amount of total fats and the intake of MUFAs and/or PUFAs (Table 4).
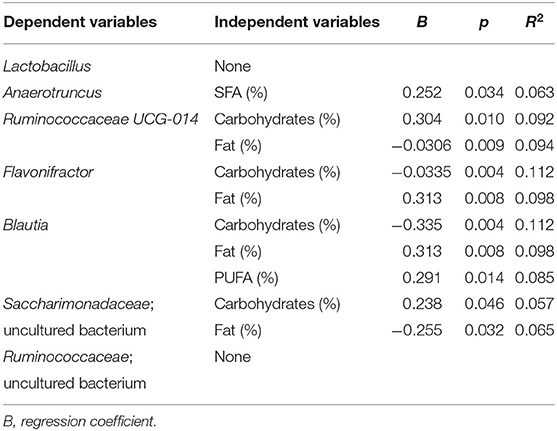
Table 2. Multivariate linear regression analysis of the bacterial taxa significantly different between HSFA and LSFA groups as dependent variants, and diet and body composition parameters as independent variants.
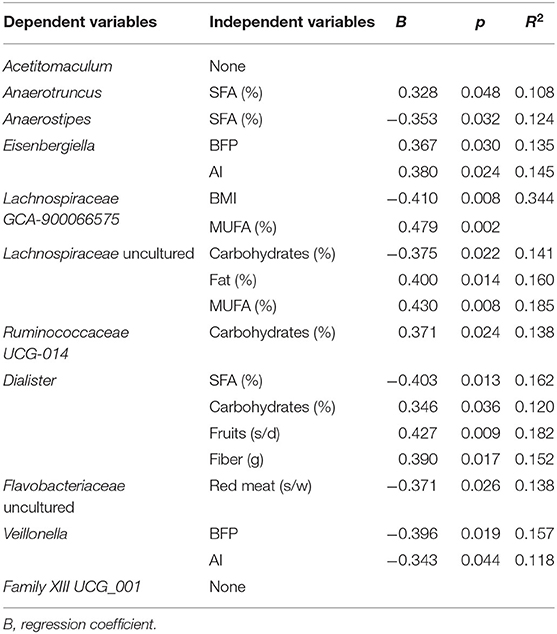
Table 3. Multivariate linear regression analysis of the bacterial taxa significantly different between HSFA-M and LSFA-M groups as dependent variants, and diet and body composition parameters as independent variants.
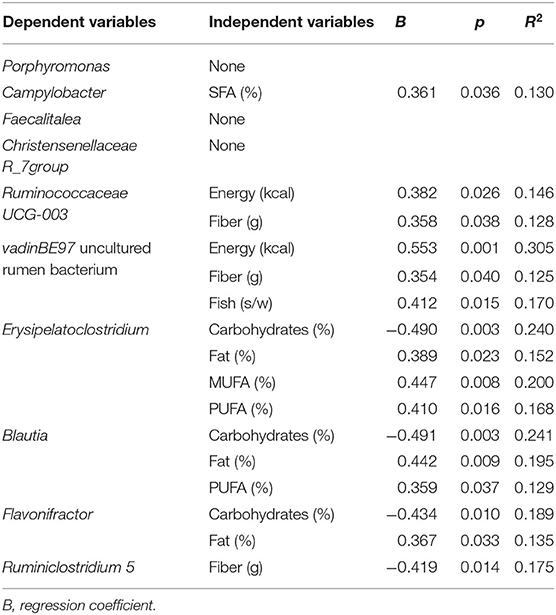
Table 4. Multivariate linear regression analysis of the bacterial taxa significantly different between HSFA-W and LSFA-W groups as dependent variants, and diet and body composition parameters as independent variants.
Predicted Functional Metagenome
PICRUSt analysis was used to infer the functional capabilities of the microbial communities by predicting functional genes that are associated with different taxa. LEfSe analysis identified several pathways that were enriched in high and low SFAs consumption groups. Considering the differences observed when analyzing microbiota composition, the PICRUSt and Lefse analysis were performed with the population stratified by sex.
Only the outer membrane receptor for ferrienterochelin and colicins (K16089) was enriched in HSFA-W (Supplementary Figure 2A). For men, there were differences in four pathways (response regulator HydG, L-fucose permease, other glycan degradation involving alpha-L-fucosidase and antimicrobial resistance with a multidrug efflux system) in the HSFA-M group, and only one in the LSFA-M group (ABC-2 type transport system ATP-binding protein) (Supplementary Figure 2B).
Discussion
Unhealthy eating habits can lead to the development of numerous chronic illnesses such as metabolic syndrome, CVD, and diabetes. There is growing evidence that some of these disorders are associated with specific features of the gut microbiota, which differ from those of healthy individuals (37). However, it is not known with any precision when the microbiota begins to present characteristics that differ from those found in healthy subjects. We aimed to determine whether the microbiota of a healthy population, in the absence of obesity or any type of diagnosed disease, and following a diet rich in HSFA, has a higher proportion of bacterial taxa reported to be related to the onset of pathological processes. While the population was initially divided into low- and high-SFA intake according to dietary SFAs content, an intrinsic negative association between SFAs and fiber intake was observed. Therefore, our results show the effect of a diet rich in SFAs and low in fiber.
We analyzed the data by sex because of the known differences between males and females for predisposition and incidence of diseases, and development of microbiota dysbiosis (24). Our results indicate that the gut microbiota of both healthy men and women following an HSFA/low-fiber diet has a greater presence of bacteria that could be related to some chronic diseases, including Anaerotruncus, Eisenbergiella, Lachnospiraceae, Campylobacter, Flavonifractor, and Erysipelatoclostridium. High fat intake is considered an energy-dense diet and is usually associated with a low intake of carbohydrates, including fiber, and a moderate intake of proteins, which overall disrupts the dietary pattern and leads to an imbalanced diet (38). Obesity, diabetes and atherosclerosis have been previously associated with diets rich in fats but low in fiber (39). Dietary fiber contributes beneficially to gastrointestinal health and a “good” gut microbiota, whereas a deficiency in plant fibers, including legumes, grains, fruits and vegetables, is related to numerous gastrointestinal disorders. Although diet is known to shape the gut microbiota, there are relatively few human studies that address the effect of macronutrient content. It was initially thought that the interaction of dietary fats with intestinal microbiota was negligible, since dietary fat is absorbed in the proximal portion of the intestine and does not reach the colon. It is now known, however, that it does indeed reach the colon, where it can serve as a food substrate for some bacteria and also exert antimicrobial effects (23). Most studies focusing on the effect of HFD on gut microbiota have been performed in animal models (23), where it has been observed that HSFA diets induce microbial changes associated with obesity, including loss of bacterial diversity and inflammatory response (40).
We observed that when participants were studied together (both men and women), those with a high intake of SFAs (HSFA) showed an increased abundance of Anaerotruncus, Eisenbergiella, Lachnospiraceae, Campylobacter, Flavonifractor, and Erysipelatoclostridium, which are known to correlate with weight gain (41) and have also been reported in various diseases, such as obesity (42), colorectal cancer (43, 44), lupus erythematosus (45), allergies (40), and some intestinal diseases (46). Conversely, participants on an LSFA diet showed an increase in bacterial taxa linked to beneficial effects on health (47, 48).
Changes in the abundance of Anaerotruncus, Anaerostipes, and Ruminococcaceae (UCG-014) in men (HSAF-M) and Campylobacter in women (HSFA-W) were partially explained by high or low SFA intake. Recent studies have reported that HFD increases intestinal permeability and LPS translocation into the circulation, triggering systemic inflammation, oxidative stress and changes in gut microbiota composition (49, 50). Supporting our findings, the abundance of the genus Anaerotruncus, a butyrate producer, has been reported to increase in mice under HFD feeding (51, 52), and also in obese mice (53, 54) and humans (55). A study of older community-dwelling men reported a positive association between Anaerotruncus and a Western-type diet (56). Interestingly, this genus has been associated with metabolic disease, and was found to be more abundant in women than in men (24). In contrast to this latter study, we found that Anaerotruncus abundance is linked with SFA consumption in the entire population (both men and women) and also only when men were considered. In a study on intestinal barrier dysfunction, Gao et al. found that Anaerotruncus was related to intestinal permeability indices, LPS, and tight junction proteins in rats on HFD (50), suggesting its involvement in loss of barrier function and LPS production. Campylobacter, a genus that correlates with SFA intake in women in our study, comprises 20 species with the majority related to intestinal, systemic and periodontal infections (46). It has been reported that mice fed a diet rich in SFAs have an increased susceptibility to Campylobacter infection (57).
We found negative correlations between SFAs intake and abundance of Anaerostipes and Ruminococcaceae (UCG-014) in men. Anaerostipes abundance was inversely correlated with SFAs in the HSFA-M group. Similar to Anaerotruncus, Anaerostipes is a butyrate-producing genus that is associated with the gut microbiota of healthy individuals (58, 59). Anaerostipes has been reported to decrease in abundance in HFD-induced metabolic syndrome (60), and to increase after low-fat vegetarian diets (61) and fiber-rich diets (62) and also when the BMI decreases (63), in agreement with the data obtained in the present study. Ruminococcaceae have been shown to negatively correlate with metabolic disease in humans (64), and we observed that the genus Ruminococcaceae (UCG-014) negatively correlated with SFAs consumption. Zhao et al. reported similar results in rats, where this taxon significantly decreased in abundance after HFD feeding (65).
Men following an HSFA diet with a low fiber intake showed unfavorable anthropometric patterns, which could increase the risk of obesity and other related disorders. Also, Campylobacter, Flavonifractor, and Erysipelotrichaceae, all prevalent in the HSFA-W group, are associated with inflammation, indicating that high-fat intake increases the levels of inflammation, especially in women, which could be a reason for the high risk of obesity and colon cancer (43, 45, 46, 66). It is important to highlight that these bacterial taxa associated with different diseases have been found in healthy participants who consume high levels of SFAs. The bacterial taxa found in this population could be used as early predictors for the onset of some diseases and inflammatory disorders in the healthy population.
Focusing on the relevant functional categories highlighted by the PiCRUSt analysis, we found that the HSFA-W group showed a positive association with the presence of colicins, which is in accordance with a study reporting an enrichment of ferric enterobactin and colicins in women with high fat intake (67). Enterobactin presumably acts on transferrin to decrease iron assimilation. It is not yet known whether the high levels of dietary fat influence iron absorption, but it seems that women with obesity might have decreased iron assimilation (68). In men, SFAs consumption seems positively associated with HydG, a response regulator of hydrogenase 3 activity and also related to iron and other metal cofactors such as zinc (69). Iron is an essential nutrient for many bacteria, and iron supplementation has been reported to affect the composition of the gut microbiota (38). Iron deficiency in humans is the most common form of malnutrition, causing iron-deficiency anemia, and is likely due to poor iron absorption in the gut (68).
HSFA in men was associated with the presence of L-fucose permease and alpha-L-fucosidase, which increase the risk of Campylobacter jejuni infections (70). The HSFA-M group was also associated with a multidrug efflux system (71). Fucose is a product of the degradation of intestinal mucin. Prolonged high-fat diets induce low-grade chronic intestinal inflammation in mice, and diets high in SFAs are a risk factor for the development of human inflammatory bowel disease, resulting in mucin degradation and activation of fucose metabolism pathways. This likely increases the prevalence of Campylobacter spp. (70). LSFA in men seems to be associated with a better performance of the ABC-2 type transporters, which could favor the elimination of xenobiotics.
Overall, our study shows that the microbiota of healthy subjects following a diet rich in SFAs diet contains bacterial taxa that could be related to the development of certain diseases, especially obesity and other pro-inflammatory diseases in women, and correlates with the modification of anthropometrical patterns in men. Differences in gut microbiota according to sex suggest that women and men could be differentially influenced by HSFA diets. Moreover, consumption of SFAs was found to be associated with a low fiber intake; with their combined effect likely synergistic for promoting an unhealthy microbiota, which could influence the development of inflammatory and obesity-related diseases. The establishment of personalized diet interventions for preventing diseases will require a deeper understanding of how sex influences the response to diet and the development of chronic diseases, and how the gut microbiota can be a mediator between the two factors. Our study identifies bacterial taxa that could be considered as early predictors of disease onset in a healthy population.
Data Availability Statement
The datasets presented in this study can be found in online repositories. The names of the repository/repositories and accession number(s) can be found below:
NCBI BioProject; Accession No. PRJNA647292.
Ethics Statement
The studies involving human participants were reviewed and approved by Ethics Committee for Clinical Research of the Hospital Ramón y Cajal (Madrid, Spain) (CEIC 338-17). The patients/participants provided their written informed consent to participate in this study.
Author Contributions
ML and MM performed the experiments. CB and MB analyzed the data. MB, CS, SM-L, ML, and RG-S wrote the paper. All authors contributed to the article and approved the submitted version.
Funding
This research was supported by the Ramón y Cajal program (grant 2012_11910), AGL2016-77288-R project of the Ministry of Economy and Competitiveness, Spain, and Universidad Europea de Madrid grant 2018/UEM4.
Conflict of Interest
The authors declare that the research was conducted in the absence of any commercial or financial relationships that could be construed as a potential conflict of interest.
Acknowledgments
The authors acknowledged all the study participants.
Supplementary Material
The Supplementary Material for this article can be found online at: https://www.frontiersin.org/articles/10.3389/fnut.2020.583608/full#supplementary-material
References
1. Schwingshackl L, Schwedhelm C, Hoffmann G, Knüppel S, Laure Preterre A, Iqbal K, et al. Food groups and risk of colorectal cancer. Int J Cancer. (2018) 142:1748–58. doi: 10.1002/ijc.31198
2. Chiu S, Williams PT, Krauss RM. Effects of a very high saturated fat diet on LDL particles in adults with atherogenic dyslipidemia: a randomized controlled trial. PLoS ONE. (2017) 12:e0170664. doi: 10.1371/journal.pone.0170664
3. Bojková B, Winklewski PJ, Wszedybyl-Winklewska M. Dietary fat and cancer—which is good, which is bad, and the body of evidence. Int J Mol Sci. (2020) 21:1–56. doi: 10.3390/ijms21114114
4. Kris-Etherton PM, Petersen K, Van Horn L. Convincing evidence supports reducing saturated fat to decrease cardiovascular disease risk. BMJ Nutr Prev Heal. (2018) 1:23–6. doi: 10.1136/bmjnph-2018-000009
6. FAO/WHO. Food and Agriculture Organization of the United Nations. World Health Organization. Evaluation of certain contaminants in food. WHO Tech Rep Ser. (2010) 959:55–64. Available online at: https://apps.who.int/iris/handle/10665/44514
7. Ruiz E, Ávila JM, Valero T, Pozo S Del, Rodriguez P, Aranceta-Bartrina J, et al. Energy intake, profile, and dietary sources in the spanish population: findings of the ANIBES study. Nutrients. (2015) 7:4739–62. doi: 10.3390/nu7064739
8. Conlon MA, Bird AR. The impact of diet and lifestyle on gut microbiota and human health. Nutrients. (2015) 7:17–44. doi: 10.3390/nu7010017
9. Sekirov I, Russell SL, Caetano M Antunes L, Finlay BB. Gut microbiota in health and disease. Physiol Rev. (2010) 90:859–904. doi: 10.1152/physrev.00045.2009
10. Falony G, Joossens M, Vieira-Silva S, Wang J, Darzi Y, Faust K, et al. Population-level analysis of gut microbiome variation. Science. (2016) 352:560–4. doi: 10.1126/science.aad3503
11. Hildebrandt MA, Hoffmann C, Sherrill-Mix SA, Keilbaugh SA, Hamady M, Chen YY, et al. High-fat diet determines the composition of the murine gut microbiome independently of obesity. Gastroenterology. (2009) 137:1716–24. doi: 10.1053/j.gastro.2009.08.042
12. Daniel H, Gholami AM, Berry D, Desmarchelier C, Hahne H, Loh G, et al. High-fat diet alters gut microbiota physiology in mice. ISME J. (2014) 8:295–308. doi: 10.1038/ismej.2013.155
13. Turnbaugh PJ, Bäckhed F, Fulton L, Gordon JI. Diet-induced obesity is linked to marked but reversible alterations in the mouse distal gut microbiome. Cell Host Microbe. (2008) 3:213–23. doi: 10.1016/j.chom.2008.02.015
14. Cani PD, Delzenne NM. The gut microbiome as therapeutic target. Pharmacol Ther. (2011) 130:202–12. doi: 10.1016/j.pharmthera.2011.01.012
15. Schneeberger M, Everard A, Gómez-Valadés AG, Matamoros S, Ramírez S, Delzenne NM, et al. Akkermansia muciniphila inversely correlates with the onset of inflammation, altered adipose tissue metabolism and metabolic disorders during obesity in mice. Sci Rep. (2015) 5:1–14. doi: 10.1038/srep16643
16. Ji Y, Sakata Y, Tso P. Nutrient-induced inflammation in the intestine. Curr Opin Clin Nutr Metab Care. (2011) 14:315–21. doi: 10.1097/MCO.0b013e3283476e74
17. Ghoshal S, Witta J, Zhong J, de Villiers W, Eckhardt E. Chylomicrons promote intestinal absorption of lipopolysaccharides. J Lipid Res. (2009) 50:90–7. doi: 10.1194/jlr.M800156-JLR200
18. Zhang C, Zhang M, Wang S, Han R, Cao Y, Hua W, et al. Interactions between gut microbiota, host genetics and diet relevant to development of metabolic syndromes in mice. ISME J. (2010) 4:232–41. doi: 10.1038/ismej.2009.112
19. Devkota S, Wang Y, Musch MW, Leone V, Fehlner-Peach H, Nadimpalli A, et al. Dietary-fat-induced taurocholic acid promotes pathobiont expansion and colitis in Il10-/- mice. Nature. (2012) 487:104–8. doi: 10.1038/nature11225
20. Roýtiö H, Mokkala K, Vahlberg T, Laitinen K. Dietary intake of fat and fibre according to reference values relates to higher gut microbiota richness in overweight pregnant women. Br J Nutr. (2017) 118:343–52. doi: 10.1017/S0007114517002100
21. Agans R, Gordon A, Kramer DL, Perez-Burillo S, Rufián-Henares JA, Paliy O. Dietary fatty acids sustain the growth of the human gut microbiota. Appl Environ Microbiol. (2018) 84:e01525-18. doi: 10.1128/AEM.01525-18
22. David LA, Maurice CF, Carmody RN, Gootenberg DB, Button JE, Wolfe BE, et al. Diet rapidly and reproducibly alters the human gut microbiome. Nature. (2014) 505:559–63. doi: 10.1038/nature12820
23. Cândido FG, Valente FX, Grześkowiak ŁM, Moreira APB, Rocha DMUP, Alfenas R de CG. Impact of dietary fat on gut microbiota and low-grade systemic inflammation: mechanisms and clinical implications on obesity. Int J Food Sci Nutr. (2018) 69:125–43. doi: 10.1080/09637486.2017.1343286
24. Santos-Marcos JA, Perez-Jimenez F, Camargo A. The role of diet and intestinal microbiota in the development of metabolic syndrome. J Nutr Biochem. (2019) 70:1–27. doi: 10.1016/j.jnutbio.2019.03.017
25. Vioque J, Gonzalez L. Validity of a food frequency questionnaire (preliminary results). Eur J Cancer Prev. (1991) 1:19–20. doi: 10.1097/00008469-199110001-00029
26. Klindworth A, Pruesse E, Schweer T, Peplies J, Quast C, Horn M, et al. Evaluation of general 16S ribosomal RNA gene PCR primers for classical and next-generation sequencing-based diversity studies. Nucleic Acids Res. (2013) 41:e1. doi: 10.1093/nar/gks808
27. Bolyen E, Rideout JR, Dillon MR, Bokulich NA, Abnet CC, Al-ghalith GA, et al. QIIME 2: reproducible, interactive, scalable, and extensible microbiome data science. PeerJ Prepr. (2018) 6:e27295. doi: 10.7287/peerj.preprints.27295
28. Callahan BJ, McMurdie PJ, Rosen MJ, Han AW, Johnson AJA, Holmes SP. DADA2: high-resolution sample inference from Illumina amplicon data. Nat Methods. (2016) 13:581–3. doi: 10.1038/nmeth.3869
29. Edgar RC, Flyvbjerg H. Error filtering, pair assembly and error correction for next-generation sequencing reads. Bioinformatics. (2015) 31:3476–82. doi: 10.1093/bioinformatics/btv401
30. Bokulich NA, Kaehler BD, Rideout JR, Dillon M, Bolyen E, Knight R, et al. Optimizing taxonomic classification of marker-gene amplicon sequences with QIIME 2's q2-feature-classifier plugin. Microbiome. (2018) 6:90. doi: 10.1186/s40168-018-0470-z
31. Camacho C, Coulouris G, Avagyan V, Ma N, Papadopoulos J, Bealer K, Madden TL. BLAST+: architecture and applications. BMC Bioinformatics. (2009) 10:421. doi: 10.1186/1471-2105-10-421
32. Quast C, Pruesse E, Yilmaz P, Gerken J, Schweer T, Yarza P, et al. The SILVA ribosomal RNA gene database project: improved data processing and web-based tools. Nucleic Acids Res. (2013) 41:D590-6. doi: 10.1093/nar/gks1219
33. Yilmaz P, Parfrey LW, Yarza P, Gerken J, Pruesse E, Quast C, et al. The SILVA and "All-species Living Tree Project (LTP)" taxonomic frameworks. Nucleic Acids Res. (2014) 42:D643-8. doi: 10.1093/nar/gkt1209
34. Lozupone C, Knight R. UniFrac: a new phylogenetic method for comparing microbial communities. Appl Environ Microbiol. (2005) 71:8228–35. doi: 10.1128/AEM.71.12.8228-8235.2005
35. Langille MGI, Zaneveld J, Caporaso JG, McDonald D, Knights D, Reyes JA, et al. Predictive functional profiling of microbial communities using 16S rRNA marker gene sequences. Nat Biotechnol. (2013) 31:814–21. doi: 10.1038/nbt.2676
36. Segata N, Izard J, Waldron L, Gevers D, Miropolsky L, Garrett WS, et al. Metagenomic biomarker discovery and explanation. Genome Biol. (2011) 12:R60. doi: 10.1186/gb-2011-12-6-r60
37. Tilg H, Moschen AR. Microbiota and diabetes: an evolving relationship. Gut. (2014) 63:1513–21. doi: 10.1136/gutjnl-2014-306928
38. Schneider BC, Dumith SC, Orlandi SP, Assunção MCF. Dieta e gordura corporal na adolescência e início da vida adulta: Uma revisão sistemática de estudos longitudinais. Cienc e Saude Coletiva. (2017) 22:1539–52. doi: 10.1590/1413-81232017225.13972015
39. Moreira APB, Texeira TFS, Ferreira AB, Do Carmo Gouveia Peluzio M, De Cássia Gonçalves Alfenas R. Influence of a high-fat diet on gut microbiota, intestinal permeability and metabolic endotoxaemia. Br J Nutr. (2012) 108:801–9. doi: 10.1017/S0007114512001213
40. Hussain M, Bonilla-Rosso G, Kwong Chung CKC, Bäriswyl L, Rodriguez MP, Kim BS, et al. High dietary fat intake induces a microbiota signature that promotes food allergy. J Allergy Clin Immunol. (2019) 144:157–70. doi: 10.1016/j.jaci.2019.01.043
41. Togo AH, Khelaifia S, Bittar F, Maraninchi M, Raoult D, Million M. “Eisenbergiella massiliensis”, a new species isolated from human stool collected after bariatric surgery. New Microbes New Infect. (2016) 13:15–6. doi: 10.1016/j.nmni.2016.05.015
42. Méndez-Salazar EO, Ortiz-López MG, Granados-Silvestre M de los Á, Palacios-González B, Menjivar M. Altered gut microbiota and compositional changes in Firmicutes and Proteobacteria in mexican undernourished and obese children. Front Microbiol. (2018) 9:2494. doi: 10.3389/fmicb.2018.02693
43. Ai D, Pan H, Han R, Li X, Liu G, Xia LC. Using decision tree aggregation with random forest model to identify gut microbes associated with colorectal cancer. Genes. (2019) 10:112. doi: 10.3390/genes10020112
44. Munch Roager H, Vogt JK, Kristensen M, Hansen LBS, Ibrügger S, Maerkedahl RB, et al. Whole grain-rich diet reduces body weight and systemic low-grade inflammation without inducing major changes of the gut microbiome: a randomised cross-over trial. Gut. (2019) 68:83–93. doi: 10.1136/gutjnl-2017-314786
45. He Z, Shao T, Li H, Xie Z, Wen C. Alterations of the gut microbiome in Chinese patients with systemic lupus erythematosus. Gut Pathog. (2016) 8:1–7. doi: 10.1186/s13099-016-0146-9
46. Lee S, Lee J, Ha J, Choi Y, Kim S, Lee H, et al. Clinical relevance of infections with zoonotic and human oral species of Campylobacter. J Microbiol. (2016) 54:459–67. doi: 10.1007/s12275-016-6254-x
47. Scheiman J, Luber JM, Chavkin TA, MacDonald T, Tung A, Pham LD, et al. Meta-omics analysis of elite athletes identifies a performance-enhancing microbe that functions via lactate metabolism. Nat Med. (2019) 25:1104–9. doi: 10.1038/s41591-019-0485-4
48. Hosseini E, Grootaert C, Verstraete W, Van de Wiele T. Propionate as a health-promoting microbial metabolite in the human gut. Nutr Rev. (2011) 69:245–58. doi: 10.1111/j.1753-4887.2011.00388.x
49. Kim SJ, Kim SE, Kim AR, Kang S, Park MY, Sung MK. Dietary fat intake and age modulate the composition of the gut microbiota and colonic inflammation in C57BL/6J mice. BMC Microbiol. (2019) 19:193. doi: 10.1186/s12866-019-1557-9
50. Gao Z, Wu H, Zhang K, Hossen I, Wang J, Wang C, et al. Protective effects of grape seed procyanidin extract on intestinal barrier dysfunction induced by a long-term high-fat diet. J Funct Foods. (2020) 64:103663. doi: 10.1016/j.jff.2019.103663
51. Liu Y, Li T, Alim A, Ren D, Zhao Y, Yang X. Regulatory effects of stachyose on colonic and hepatic inflammation, gut microbiota dysbiosis, and peripheral CD4+ T cell distribution abnormality in high-fat diet-fed mice. J Agric Food Chem. (2019) 67:11665–74. doi: 10.1021/acs.jafc.9b04731
52. Kong C, Gao R, Yan X, Huang L, Qin H. Probiotics improve gut microbiota dysbiosis in obese mice fed a high-fat or high-sucrose diet. Nutrition. (2019) 60:175–84. doi: 10.1016/j.nut.2018.10.002
53. Shin NR, Lee JC, Lee HY, Kim MS, Whon TW, Lee MS, et al. An increase in the Akkermansia spp. population induced by metformin treatment improves glucose homeostasis in diet-induced obese mice. Gut. (2014) 63:727–35. doi: 10.1136/gutjnl-2012-303839
54. Xu P, Wang J, Hong F, Wang S, Jin X, Xue T, et al. Melatonin prevents obesity through modulation of gut microbiota in mice. J Pineal Res. (2017) 62:e12399. doi: 10.1111/jpi.12399
55. Togo AH, Valero R, Delerce J, Raoult D, Million M. “Anaerotruncus massiliensis,” a new species identified from human stool from an obese patient after bariatric surgery. New Microbes New Infect. (2016) 14:56–7. doi: 10.1016/j.nmni.2016.07.015
56. Shikany JM, Demmer RT, Johnson AJ, Fino NF, Meyer K, Ensrud KE, et al. Association of dietary patterns with the gut microbiota in older, community-dwelling men. Am J Clin Nutr. (2019) 110:1003–14. doi: 10.1093/ajcn/nqz174
57. Bereswill S, Plickert R, Fischer A, Kühl AA, Loddenkemper C, Batra A, et al. What you eat is what you get: novel Campylobacter models in the quadrangle relationship between nutrition, obesity, microbiota and susceptibility to infection. Eur J Microbiol Immunol. (2011) 1:237–48. doi: 10.1556/EuJMI.1.2011.3.8
58. Sekelja M, Berget I, Næs T, Rudi K. Unveiling an abundant core microbiota in the human adult colon by a phylogroup-independent searching approach. ISME J. (2011) 5:519–31. doi: 10.1038/ismej.2010.129
59. Zhang J, Guo Z, Xue Z, Sun Z, Zhang M, Wang L, et al. A phylo-functional core of gut microbiota in healthy young Chinese cohorts across lifestyles, geography and ethnicities. ISME J. (2015) 9:1979–90. doi: 10.1038/ismej.2015.11
60. Wang J, Lang T, Shen J, Dai J, Tian L, Wang X. Core gut bacteria analysis of healthy mice. Front Microbiol. (2019) 10:887. doi: 10.3389/fmicb.2019.00887
61. Pagliai G, Russo E, Niccolai E, Dinu M, Di Pilato V, Magrini A, et al. Influence of a 3-month low-calorie Mediterranean diet compared to the vegetarian diet on human gut microbiota and SCFA: the CARDIVEG Study. Eur J Nutr. (2019) 59:2011–24. doi: 10.1017/S0029665120001251
62. Aoe S, Nakamura F, Fujiwara S. Effect of wheat bran on fecal butyrate-producing bacteria and wheat bran combined with barley on bacteroides abundance in japanese healthy adults. Nutrients. (2018) 10:1980. doi: 10.3390/nu10121980
63. Haro C, Rangel-Zúñiga OA, Alcalá-Díaz JF, Gómez-Delgado F, Pérez-Martínez P, Delgado-Lista J, et al. Intestinal microbiota is influenced by gender and body mass index. PLoS ONE. (2016) 11:e0154090. doi: 10.1371/journal.pone.0154090
64. He Y, Wu W, Wu S, Zheng H-M, Li P, Sheng H-F, et al. Linking gut microbiota, metabolic syndrome and economic status based on a population-level analysis. Microbiome. (2018) 6:172. doi: 10.1186/s40168-018-0557-6
65. Zhao L, Zhang Q, Ma W, Tian F, Shen H, Zhou M. A combination of quercetin and resveratrol reduces obesity in high-fat diet-fed rats by modulation of gut microbiota. Food Funct. (2017) 8:4644–56. doi: 10.1039/C7FO01383C
66. Munch H, Vogt JK, Kristensen M, Benedicte L, Hansen S, Ibrügger S, et al. Gut microbiota Whole grain-rich diet reduces body weight and systemic low-grade inflammation without inducing major changes of the gut microbiome: a randomised cross-over trial. Gut. (2017) 68:1–11. doi: 10.1136/gutjnl-2017-314786
67. Agus A, Denizot J, Thévenot J, Martinez-Medina M, Massier S, Sauvanet P, et al. Western diet induces a shift in microbiota composition enhancing susceptibility to adherent-invasive E. coli infection and intestinal inflammation. Sci Rep. (2016) 6:19032. doi: 10.1038/srep19032
68. Aigner E, Feldman A, Datz C. Obesity as an emerging risk factor for iron deficiency. Nutrients. (2014) 6:3587–600. doi: 10.3390/nu6093587
69. Bortolus M, Costantini P, Doni D, Carbonera D. Overview of the maturation machinery of the h-cluster of [FeFe]-hydrogenases with a focus on HYdF. Int J Mol Sci. (2018) 19:3118. doi: 10.3390/ijms19103118
70. Stahl M, Friis LM, Nothaft H, Liu X, Li J, Szymanski CM, Stintzi A. L-fucose utilization provides Campylobacter jejuni with a competitive advantage. Proc Natl Acad Sci USA. (2011) 108:7194–9. doi: 10.1073/pnas.1014125108
Keywords: microbiota, saturated-fatty acids, fiber, obesity, inflammation
Citation: Bailén M, Bressa C, Martínez-López S, González-Soltero R, Montalvo Lominchar MG, San Juan C and Larrosa M (2020) Microbiota Features Associated With a High-Fat/Low-Fiber Diet in Healthy Adults. Front. Nutr. 7:583608. doi: 10.3389/fnut.2020.583608
Received: 15 July 2020; Accepted: 23 November 2020;
Published: 18 December 2020.
Edited by:
Angel Gil-Izquierdo, Spanish National Research Council, SpainReviewed by:
Baltasar Mayo, Consejo Superior de Investigaciones Científicas (CSIC), SpainRen-You Gan, Chinese Academy of Agricultural Sciences, China
Copyright © 2020 Bailén, Bressa, Martínez-López, González-Soltero, Montalvo Lominchar, San Juan and Larrosa. This is an open-access article distributed under the terms of the Creative Commons Attribution License (CC BY). The use, distribution or reproduction in other forums is permitted, provided the original author(s) and the copyright owner(s) are credited and that the original publication in this journal is cited, in accordance with accepted academic practice. No use, distribution or reproduction is permitted which does not comply with these terms.
*Correspondence: María Bailén, bWFyaWFiYWlsZW5AZ21haWwuY29t