- 1Department of Neurosurgery, The Second Affiliated Hospital of Kunming Medical University, Kunming, China
- 2Center for Life Sciences, School of Life Sciences, Yunnan University, Kunming, China
Introduction: Diffuse high-grade gliomas are the most common malignant adult neuroepithelial tumors in humans and a leading cause of cancer-related death worldwide. The advancement of high throughput transcriptome sequencing technology enables rapid and comprehensive acquisition of transcriptome data from target cells or tissues. This technology aids researchers in understanding and identifying critical therapeutic targets for the prognosis and treatment of diffuse high-grade glioma.
Methods: Spatial transcriptomics was conducted on two cases of isocitrate dehydrogenase (IDH) wild-type diffuse high-grade glioma (Glio-IDH-wt) and two cases of IDH-mutant diffuse high-grade glioma (Glio-IDH-mut). Gene set enrichment analysis and clustering analysis were employed to pinpoint differentially expressed genes (DEGs) involved in the progression of diffuse high-grade gliomas. The spatial distribution of DEGs in the spatially defined regions of human glioma tissues was overlaid in the t-distributed stochastic neighbor embedding (t-SNE) plots.
Results: We identified a total of 10,693 DEGs, with 5,677 upregulated and 5,016 downregulated, in spatially defined regions of diffuse high-grade gliomas. Specifically, SPP1, IGFBP2, CALD1, and TMSB4X exhibited high expression in carcinoma regions of both Glio-IDH-wt and Glio-IDH-mut, and 3 upregulated DEGs (SMOC1, APOE, and HIPK2) and 4 upregulated DEGs (PPP1CB, UBA52, S100A6, and CTSB) were only identified in tumor regions of Glio-IDH-wt and Glio-IDH-mut, respectively. Moreover, Kyoto Encyclopedia of Genes and Genomes (KEGG) and gene ontology (GO) enrichment analyses revealed that upregulated DEGs were closely related to PI3K/Akt signaling pathway, virus infection, and cytokine-cytokine receptor interaction. Importantly, the expression of these DEGs was validated using GEPIA databases. Furthermore, the study identified spatial expression patterns of key regulatory genes, including those involved in protein post-translational modification and RNA binding protein-encoding genes, with spatially defined regions of diffuse high-grade glioma.
Discussion: Spatial transcriptome analysis is one of the breakthroughs in the field of medical biotechnology as this can map the analytes such as RNA information in their physical location in tissue sections. Our findings illuminate previously unexplored spatial expression profiles of key biomarkers in diffuse high-grade glioma, offering novel insight for the development of therapeutic strategies in glioma.
1 Introduction
Diffuse high-grade gliomas are among the most aggressive primary brain tumors, characterized by invasive growth, rapid progress, incomplete resection challenges, frequent recurrence, and poor prognosis (Jo et al., 2023). Intratumoral heterogeneity poses the greatest obstacle in treating these tumors (Comba et al., 2021), influencing clinical presentation, treatment efficacy, sensitivity variations, and drug resistance. While multimodal treatment strategies, including surgical resection, radiotherapy, chemotherapy, targeted drug therapy, and supportive care (Luo et al., 2023), have improved patient prognosis, the median survival time remains a bleak 14.6 months (Zou et al., 2013). Therefore, identifying effective therapeutic targets is crucial to enhance patient outcomes.
Recent advancements in sequencing technologies have revolutionized tumor research (Nakagawa and Fujita, 2018). Biomarker studies, evolving from single-omics to multi-omics integration (encompassing genomics, transcriptomics, epigenetics, microbiome, metabolomics, proteomics, and radiomics), have enhanced diagnosis and prognostic capabilities across various cancers (Qi et al., 2020). Multi-omics approaches have revealed the immune response mechanisms in gliomas (Cheng et al., 2021; Johnson et al., 2022), uncovering novel biomarkers for treatment response prediction and prognosis. High-throughput sequencing of gene expression profiles aids personalized treatment strategies (Goodwin et al., 2016), yes lack spatial cellular information crucial for understanding cell differentiation and interactions during tumorigenesis (Ståhl et al., 2016).
Spatial transcriptome sequencing, an emerging technique, integrates gene expression with cellular spatial information, offering new insight into tumor heterogeneity, microenvironment, and immunity of tumors (Du et al., 2023; Wang X. et al., 2023). For example, Berglund et al. (2018) highlighted NR4A1 as highly expressed in the reactive stroma of prostate cancer sections. Wang F. et al. (2023), demonstrated the enrichment of MCAM+ fibroblasts in liver metastatic tumors using scRNA-seq combined with spatial transcriptomics, revealing their role in promoting CD8_CXCL13 cell generation via the Notch pathway, potentially accelerating liver metastatic colorectal cancer development. Spatial transcriptome sequencing also annotates tissue components, unveiling previously unexplored heterogeneity landscapes. Moreover, recent work integrating scRNA-seq with spatial transcriptomics across 16 glioblastomas confirmed chemotactic attraction of cancer-associated fibroblasts (CAFs) toward glioblastoma stem cells (GSCs), wherein CAFs enriched GSCs via upregulation of osteopontin and hepatocyte growth factor (Jain et al., 2023). Similarly, another study showed that spatial transcriptomics revealed niche-specific enrichment and vulnerabilities of radial glial stem-like cells in malignant gliomas (Ren et al., 2023). Zhao et al. (2023) reported that combining scRNA-seq and spatial transcriptome identified BARD1 as a potential therapeutic target for glioblastoma patients. Of note, spatial transcriptomics can not only present an overall view of the immune microenvironment in glioma, but also be used to explore the dynamic development and spatial specificity of immune cells in the microenvironment (Buehler et al., 2023; Sun et al., 2023). Therefore, spatial transcriptome technology may help us to capture genome-wide readouts across biological tissue space and identify therapeutic biomarkers that may have prognostic significance in glioma.
In this study, we utilize spatial transcriptome sequencing to delineate comprehensive spatial characteristics of diffuse high-grade glioma. Specifically, we identify potential therapeutic targets from spatially defined regions of IDH wild-type and mutant high-grade glioma, validating these targets through public databases. Additionally, our analysis uncovers key regulatory genes in ubiquitination, RNA binding protein, and kinase influencing the spatial biology of high-grade glioma. These findings provide potential targets for prognosis and precision therapy in glioma management.
2 Materials and methods
2.1 Sample information
Two formalin-fixed paraffin-embedded (FFPE) tissues of patients with IDH-wild-type diffuse high-grade glioma (Glio-IDH-wt) and two FFPE tissues of patients with IDH-mutant diffuse high-grade glioma (Glio-IDH-mut) were collected for further spatial transcriptome RNA sequencing. This study involving human FFPE samples was reviewed and approved by The Second Affiliated Hospital of Kunming Medical University (No: APPROVED-PJ-SCIENTIFIC-2024-71).
2.2 Spatial sequencing library preparation (FFPE-V2)
Formalin-fixed, paraffin-embedded (FFPE) samples passing the RNA quality control (DV200 > 30%) were used for spatial transcriptomic construction and sequencing. 5-micron thick sections were mounted onto a Visium Gene Expression slide (10X Genomics), baked at 42°C for 3 h, and dried in a desiccator at room temperature overnight. For deparaffinization, the slide was incubated at 60°C for 2 h, immersed in xylene, and rehydrated in an ethanol gradient. Hematoxylin–eosin staining (H&E) staining was then performed using Mayer’s hematoxylin (Millipore Sigma), bluing reagent (Dako, Agilent), and alcoholic eosin (Millipore Sigma). Stained slides were scanned under a microscope, followed by decrosslinking using 0.1 N HCl and TE Buffer (pH 9.0) to release RNA that was sequestered by formalin. The stained slide was incubated with a Human whole transcriptome probe panel and then transferred to Cytassist (10X Genomics).
A human whole transcriptome probe panel (10X) that consisted of three pairs of specific probes (5′ containing Small RNA Read 2S and 3′ containing poly-A) for mostly genes was hybridized to RNA. Probe pairs were then ligated to seal the junctions between them and to form the single-stranded ligation products. The samples were treated with RNase and permeabilized to release the ligation products. A poly-A portion of the products was then captured by the poly (dT) regions of the capture probes percolated on the Visium slide that also includes an Illumina Read 1, spatial. Barcode, and unique molecular identifier (UMI). Probes were extended to produce spatially barcoded ligated probe products and released from the slide for indexing via Sample Index PCR and final library construction and sequencing. Visium Spatial Gene Expression libraries consisted of Illuminapaired-end sequences flanked with P5/P7. The 16-bp Spatial Barcode and 12-bp UMI were encoded in Read 1, while Read 2S was used to sequence the ligated probe insert.
2.3 Sequencing data processing
After sequencing, raw fastq files, and bright field H&E images are processed by Space Ranger (version 2.1.0) to align to genome GRCh38 (downloaded from 10× Genomics, version 2020-April). Expression matrices and spatial coordinate files generated by Space Ranger are used for the next analysis.
2.4 Normalization, integration, and clustering of spatial transcriptomic data
We used Seurat (Hao et al., 2021) (version 4.3.0) to perform normalization, integration, and clustering. Expression matrices are read into the R environment, and a Seurat object is created for each sample. The expression count matrices are normalized using the Seurat function SCTransform with the parameter variable.features.n = 9,000, while leaving other parameters as default. Subsequently, we used the Seurat function FindClusters to cluster each sample and annotate them with H&E images. These four Seurat objects are integrated using the Seurat functions SelectIntegrationFeatures, PrepSCTIntegration, FindIntegrationAnchors, and IntegrateData.
For the function SelectIntegrationFeatures, we use the customized parameter nfeatures = 9,000. In FindIntegrationAnchors, we set customized parameters normalization. Method = “SCT,” reduction = “rpca,” k.anchor = 4, and dims = 1:30. Similarly, in IntegrateData, we set customized parameters normalization. Method = “SCT” and dims = 1:50. Other parameters are set as default. Finally, the function RunTSNE is used to perform dimension reduction.
2.5 Cell type identification by RCTD
We utilized RCTD (Cable et al., 2022) for cell type identification. Single-cell data are obtained from the Broad Institute with accession code SCP503 (Richards et al., 2021). Raw count data are input into RCTD and run with the parameter doublet_mode set to “doublet.”
2.6 Differentially expressed genes and enrichment analysis
To analyze the expression of DEGs between the tumor area and normal tissue adjacent to the tumor area (NAT) of Glio-IDH-wt and Glio-IDH-mut, we use the Seurat function FindMarkers to perform the Wilcox rank sum test between Glio-IDH-wt tumor cell densely populated area and Glio-IDH-mut tumor cell densely populated area against NAT. Genes with avg_log2FC > 1, p_val_adj < 0.05 and genes with avg_log2FC < −1, p_val_adj < 0.05 are used to perform Gene Ontology (GO) and Kyoto Encyclopedia of Genes and Genomes (KEGG) enrichment analysis with R package clusterProfiler (Wu et al., 2021) (version 4.8.3) with function enrichGO and enrichKEGG. For Venn diagram showed in Figure 1B, we choose differentially expressed genes with p_val_adj < 0.05, avg_log2FC > 1 and pct.1 > pct.2. In our dataset, genes with significantly higher expression in tumor cell densely populated area compared to NAT were selected, as well as the increased level of genes in tumor tissues compared with normal tissues in the online database (GEPIA) (Tang et al., 2019). Meanwhile, the correlation between DEGs and overall survival were analyzed using GEPIA database. For differential expression analysis between Glio-IDH-wt and Glio-IDH-mut tumor area, we first remove spots from Normal tissue adjacent to the tumor area, blood vessel rich area, and junction area. Then Seurat function FindMarkers with parameter min.pct > 0.3 is used for analysis. Genes with avg_log2FC > 0.5, p_val_adj < 0.05, pct.1 > 0.3 and pct.2 > 0.3 are used for GO enrichment analysis. Gene Expression Profiling Interactive Analysis 2 (GEPIA2) data are downloaded and illustrated with the Python package GEPIA (version 0.3).
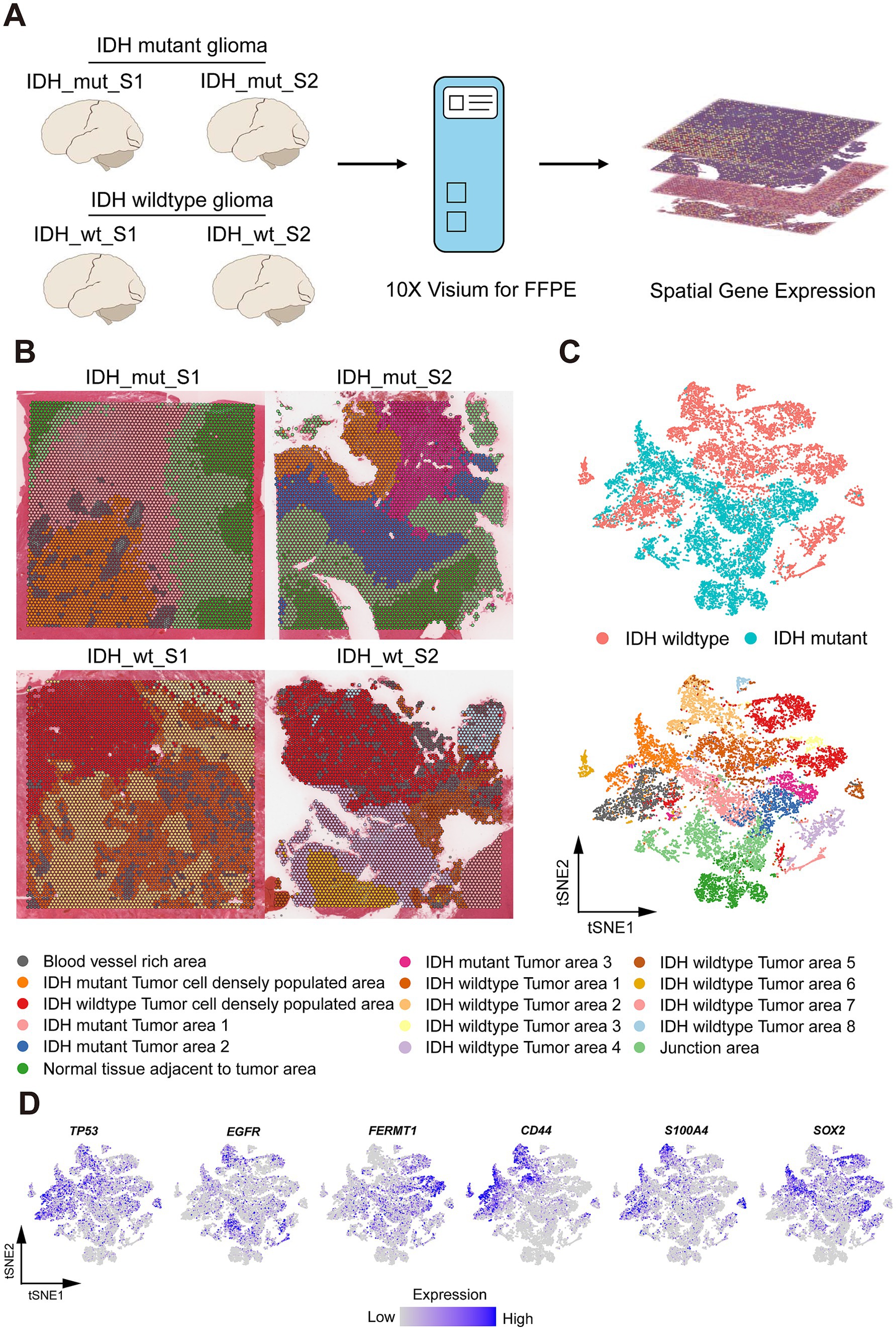
Figure 1. Spatial Profile of Glio-IDH-wt and Glio-IDH-mut. (A) Workflow of glioblastoma sample collection, spatial transcriptomics experiment, and data analysis; (B) Spatial cluster identities of 4 glioblastoma samples, colored by spatial region (bottom); (C) tSNE plot of 4 glioblastoma samples, colored by spatial region (bottom) and Glio-IDH-wt and Glio-IDH-mut, respectively. Each point indicates one spot of spatial transcriptomics; (D) Expression pattern of glioblastoma marker genes (TP53, EGFR, and FERMT1) and glioblastoma stem cell (GSC) markers (CD44, S100A4, and SOX2).
2.7 Module score analysis
For the gene module score, we use the Seurat function AddModuleScore to calculate. The ubiquitin gene list is obtained from iUUCD (Zhou et al., 2018).1 The kinase gene list is downloaded from KLIFS (Kanev et al., 2021).2 RNA binding protein gene lists are downloaded from previous studies (Wang et al., 2020). All gene lists used in this study are uploaded as Supplementary material.
3 Results
3.1 Spatial distribution profile of Glio-IDH-wt and Glio-IDH-mut
The spatial distribution profile of Glio-IDH-wt (n = 2) and Glio-IDH-mut (n = 2) was analyzed using a structured workflow (Figure 2A). Each section’s spatial clustering was determined using hematoxylin and eosin staining and cell markers, with four samples categorized into 4 distinct clusters through t-distributed stochastic neighbor embedding (tSNE) plots (Figures 2B,C). These clusters included blood vessel-rich area, tumor cell densely populated area, normal tissue adjacent to tumor area, and junction area. Additionally, biomarkers associated with glioma progression (TP53, EGFR, and FERMT1) and glioma stem cells (CD44, S100A4, and SOX2) were examined across different spatial regions of both Glio-IDH-wt and Glio-IDH-mut (Figure 2D). Specifically, TP53, CD44, and S100A4 were mainly enriched in the tumor area and blood vessel-rich area of Glio-IDH-wt, and tumor cell densely populated area of Glio-IDH-mut, EGFR was mainly enriched in tumor area of Glio-IDH-wt and junction area of Glio-IDH-mut, FERMT1 was mainly enriched in tumor cell densely populated area of Glio-IDH-wt and tumor area of Glio-IDH-mut, SOX2 was mainly enriched in tumor cell densely populated area of Glio-IDH-wt and tumor cell densely populated area and tumor area of Glio-IDH-mut, while these genes were not detected in normal tissue adjacent to tumor area of Glio-IDH-mut. In summary, the spatially defined region of four diffuse high-grade glioma samples is reasonable and reliable.
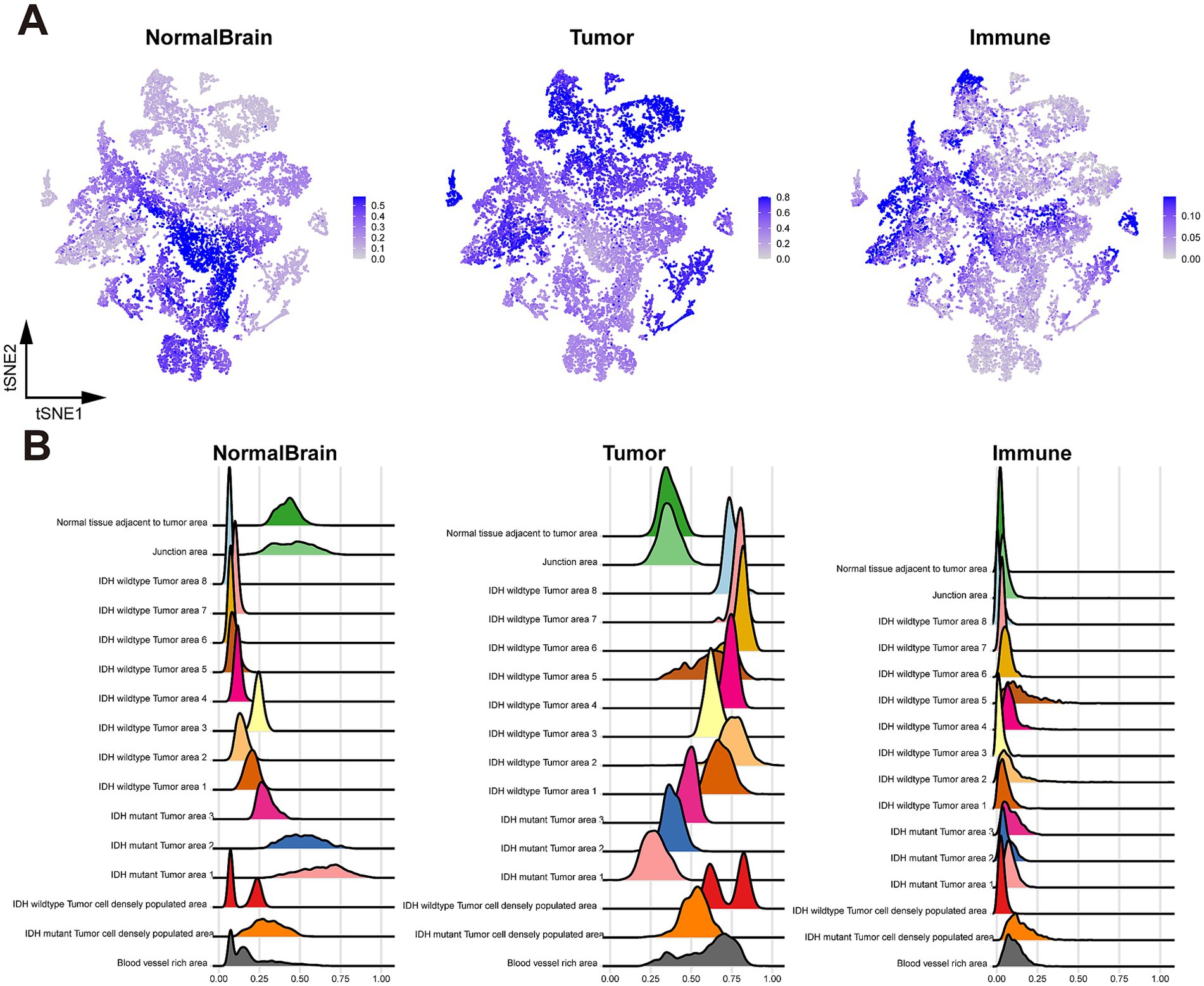
Figure 2. Cell type identification with RCTD. (A) tSNE plot colored by cell type weights of normal brain cells (left), tumor cells (middle), and immune cells (right); (B) Ridge plot of cell type weights of normal brain cells (left), tumor cells (middle), and immune cells (right), grouped by spatial region.
3.2 Cell type analysis of spatially defined regions
To enhance the characterization of tissue heterogeneity in both Glio-IDH-wt and Glio-IDH-mut, we performed a sub-clustering analysis of cell type across various spatial regions. As depicted in Figure 3, normal brain cells were primarily found in the normal tissue adjacent to the tumor area and junction area of Glio-IDH-mut, glioma cells were distributed in the tumor cell densely populated area, blood vessel-rich area, and tumor area of both Glio-IDH-wt and Glio-IDH-mut, as well as the percentage of immune cells in the tumor cell densely populated area and tumor area of Glio-IDH-mut were higher than those in the Glio-IDH-wt.
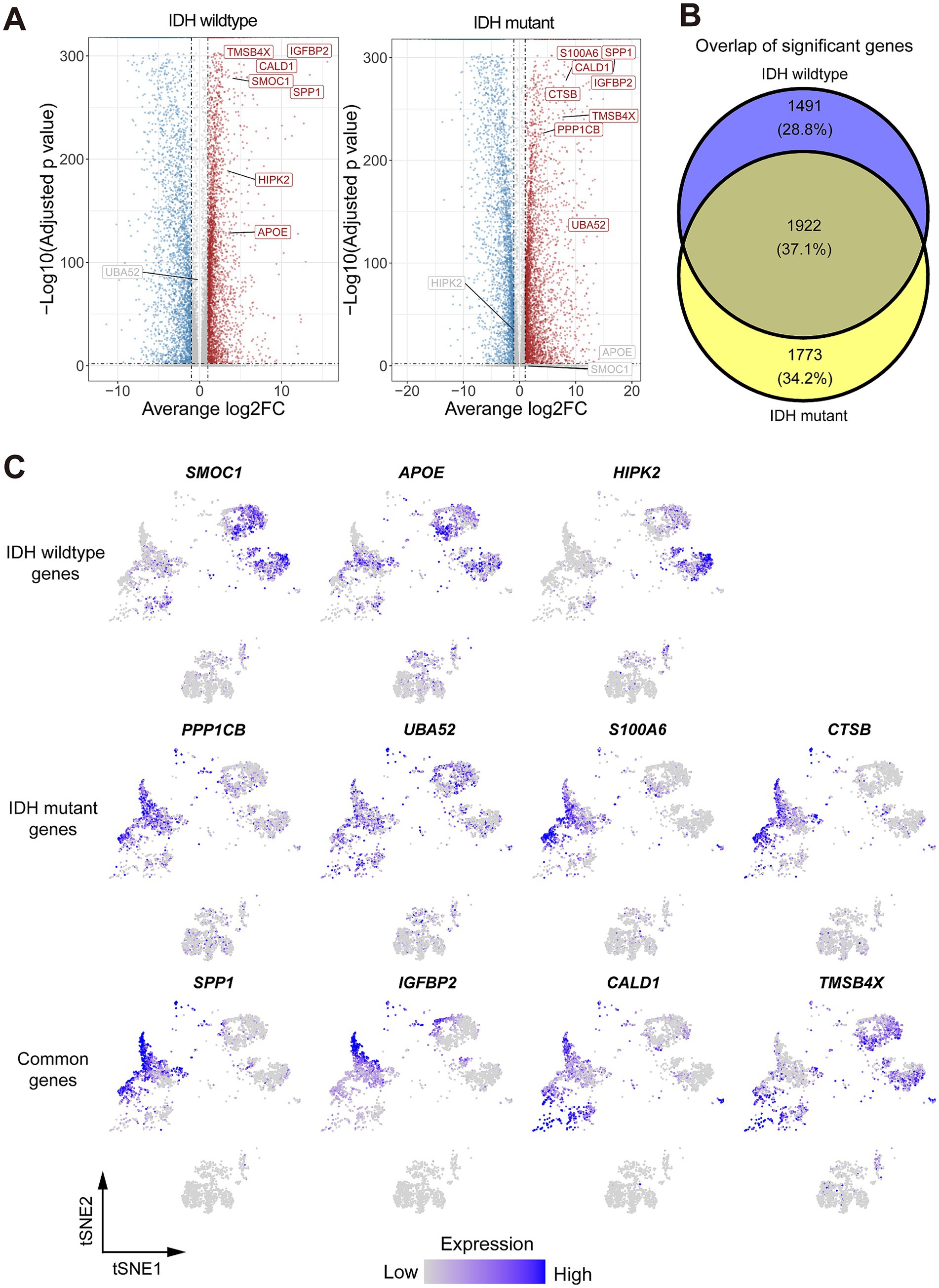
Figure 3. Identification of differentially expressed genes (DEGs) and their related pathways in both Glio-IDH-wt and Glio-IDH-mut. (A) Volcano plot showing DEGs between tumor area and normal tissue adjacent to the tumor area. Genes with average log2(Fold change) > 1 and adjusted p-value < 0.01 have higher expression levels in the tumor area and are colored with red in the volcano plot. Genes with average log2(Fold change) < −1 and adjusted p-value < 0.01 have higher expression levels in normal tissue adjacent to the tumor area and colored with blue in the volcano plot; (B) A Venn diagram of upregulated DEGs in tumor tissues of both Glio-IDH-wt and Glio-IDH-mut; (C) tSNE plot of upregulated DEGs in spatially defined region of both Glio-IDH-wt and Glio-IDH-mut.
3.3 Differentially expressed gene analysis of Glio-IDH-wt and Glio-IDH-mut
To address the lack of effective therapeutic targets for diffuse high-grade glioma, we identified the expression profiles of DEGs in Glio-IDH-wt and Glio-IDH-mut using the Seurat function FindMarkers and clustering package. Our analysis results showed that 6,759 DEGs (3,648 upregulated and 3,111 downregulated) in the cancerous tissues of Glio-IDH-wt (Supplementary Table S1). Conversely, a total of 7,435 DEGs (4,113 upregulated and 3,322 downregulated) were identified in the cancerous tissues of Glio-IDH-mut compared to adjacent normal tissues (Figure 1A; Supplementary Table S2). Venn diagrams identified a total of 1,922 upregulated DEGs in spatially defined tumor regions of both Glio-IDH-wt and Glio-IDH-mut (Figure 1B). Meanwhile, a total of 1,491 DEGs were only enhanced in the umor regions of Glio-IDH-wt and 1,773 upregulated DEGs in tumor regions of Glio-IDH-mut. Additionally, tSNE plots showed that the upregulated DEGs were mainly distributed in the spatially defined tumor cell densely populated area of both Glio-IDH-wt and Glio-IDH-mut (Figure 1C). Moreover, the top 3 upregulated DEGs (SMOC1, APOE, and HIPK2) of Glio-IDH-wt and the top 4 upregulated DEGs of Glio-IDH-mut (PPP1CB, UBA52, S100A6, and CTSB) and both Glio-IDH-wt and Glio-IDH-mut (SPP1, IGFBP2, CALD1, and TMSB4X) in glioblastoma multiforme (GBM) and brain lower grade glioma (LGG) were evaluated using the GEPIA database (Supplementary Figure S1), and these upregulated DEGs showed significant correlations with overall survival (Supplementary Figure S2).
3.4 Biological pathway enrichment analysis of upregulated DEGs in Glio-IDH-wt and Glio-IDH-mut
Based on the above results, the main functional pathways and biological mechanisms by which DEGs play a critical role in the onset and progression of diffuse high-grade gliomas are the next major thing we urgently need to understand, so we performed KEGG analysis and GO analysis on this basis. KEGG enrichment analysis revealed that the upregulated DEGs in tumor areas of both Glio-IDH-wt and Glio-IDH-mut were mainly enriched in eight pathways, including PI3K/Akt signaling pathway, human papillomavirus infection, cytokine-cytokine receptor interaction, cytoskeleton in muscle cells, focal adhesion, human cytomegalovirus infection, regulation of actin cytoskeleton, and human T-cell leukemia virus 1 infection (Figure 4A). Meanwhile, the upregulated DEGs in tumor areas of Glio-IDH-mut were enriched in five pathways, including MAPK signaling pathway, tight junction, NOD-like receptor signaling pathway, protein processing in endoplasmic reticulum, and JAK–STAT signaling pathway, while five pathways were enriched in tumor areas of Glio-IDH-wt such as relaxin signaling pathway, staphylococcus aureus infection, endocrine resistance, melanoma, and cholesterol metabolism (Figure 4B). Additionally, GO enrichment analysis (biological process) revealed that these upregulated DEGs in both Glio-IDH-wt and Glio-IDH-mut were mainly enriched in pathways such as positive regulation of cell adhesion, mononuclear cell differentiation, positive regulation of cytokine production, regulation of cell–cell adhesion, leukocyte cell–cell adhesion, cytokine-mediated signaling pathway, and epithelial cell proliferation (Figure 4C). For Glio-IDH-mut, the upregulated DEGs were enriched in biological pathways such as muscle system process, cell growth, response to oxidative stress, positive regulation of protein localization, and negative regulation of phosphorus metabolic process (Figure 4D). For Glio-IDH-wt, the upregulated DEGs were enriched in biological pathways including axonogenesis, cellular process involved in reproduction in multicellular organism, alcohol metabolic process, steroid metabolic process, organic acid biosynthetic process, and developmental maturation (Figure 4D).
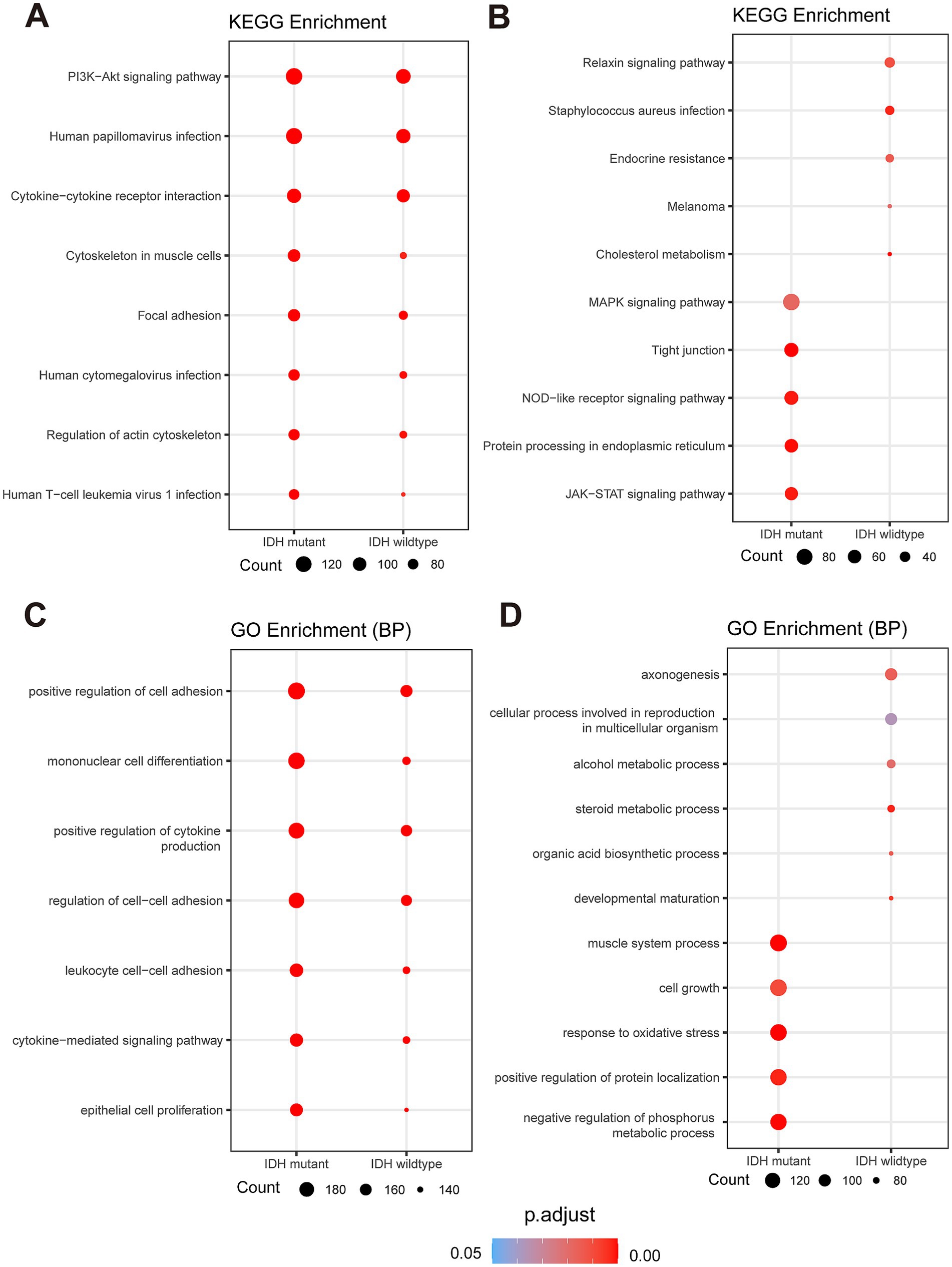
Figure 4. Spatial and functional characterization of DEGs in Glio-IDH-wt and Glio-IDH-mut. Dot plot of enriched KEGG (A,B) and GO (C,D) terms for upregulated DEGs in tumor area of Glio-IDH-wt and Glio-IDH-mut. Genes with avg_log2FC > 1, p_val_adj < 0.05 and genes with avg_log2FC < −1, p_val_adj < 0.05 are used to perform GO and KEGG enrichment analysis.
3.5 Functional role of ubiquitination, RNA binding protein, and kinase in the progression of Glio-IDH-wt and Glio-IDH-mut
Protein post-translational modification, such as ubiquitination and phosphorylation, constitute essential molecular mechanisms influencing the malignant behaviors of cancer cells and are crucial in various tumor developments (Pan and Chen, 2022). Meanwhile, the advancement of proteomics and epigenetics has identified numerous RNA-binding proteins, offering potential therapeutic targets for glioma (Liu et al., 2024). As expected, GO enrichment analysis illustrated the upregulated DEGs in tumor area of Glio-IDH-wt were mainly enriched in cell growth, response to oxidative stress, activation of immune response, response to decreased oxygen levels, DNA-binding transcription factor binding, intrinsic apoptotic signaling pathway, immune response-regulating signaling, ubiquitin-like protein ligase binding, and negative regulation of phosphorylation compared with the Glio-IDH-mut group (Figure 5A; Supplementary Table S3). Combining the current research hotspots and our research interests, we decided to take the ubiquitination, RNA binding protein, and kinase as the emphasis of subsequent research. Correspondingly, tSNE plots illustrated heightened expression of key regulatory genes in ubiquitination and RNA binding proteins within spatially defined tumor regions of both Glio-IDH-wt and Glio-IDH-mut (Figures 5B,C) Conversely, the expression of key regulatory genes in kinase was enhanced in the normal tissue adjacent to the glioma tumor. Moreover, the gene lists associated with ubiquitination, RNA binding protein, and kinase were shown in Supplementary Table S4. These findings suggested that aberrant protein post-translational modification and dysregulation of RNA-binding proteins play an important role in the progression of diffuse high-grade gliomas.
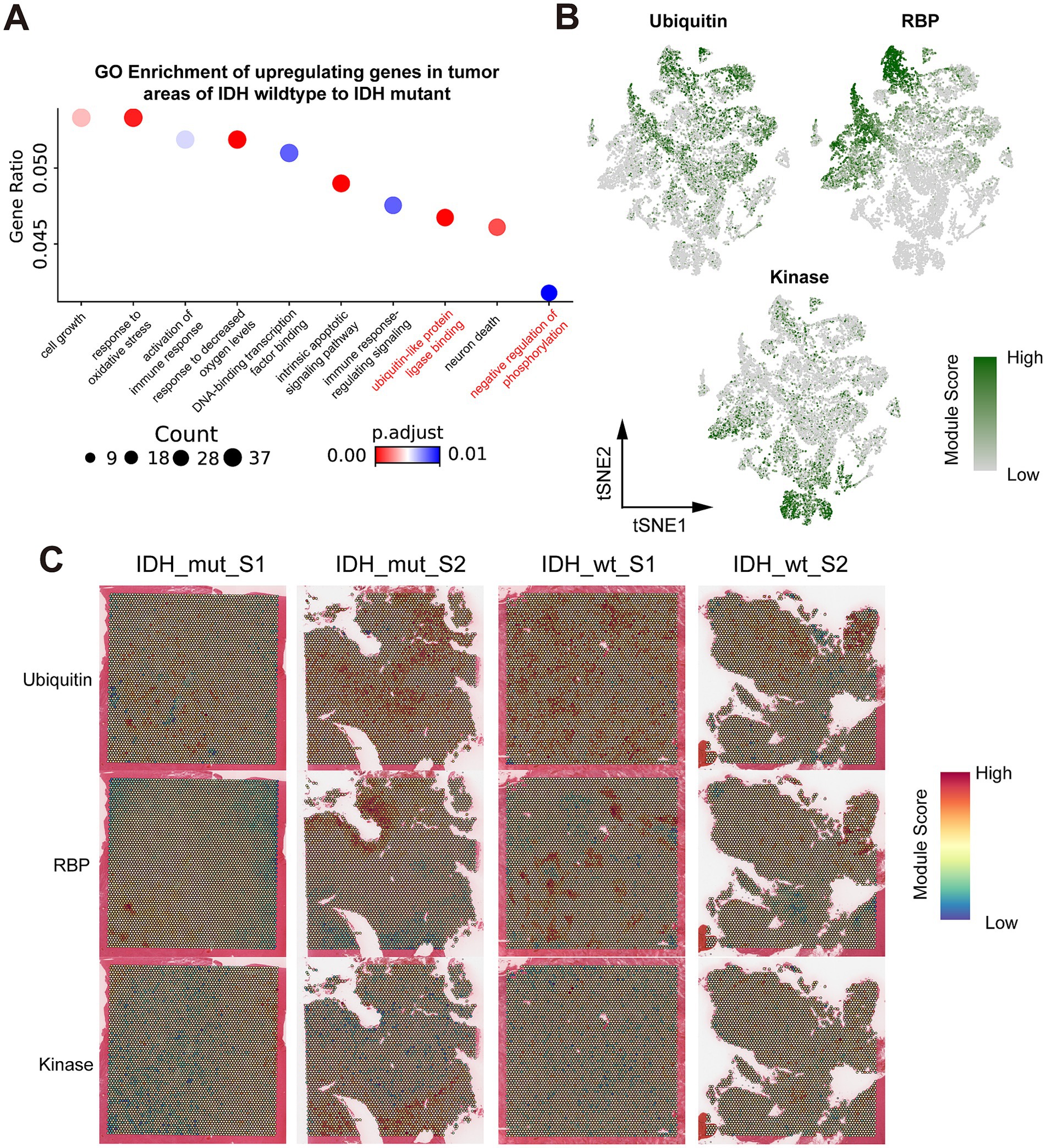
Figure 5. Spatial transcriptomics resolves the key regulatory genes in ubiquitination, RNA binding protein, and kinase in Glio-IDH-wt and Glio-IDH-mut. (A) GO enrichment of genes which upregulating in IDH wildtype tumor areas, compared to IDH mutant tumor areas; (B) tSNE plot colored by the gene module ubiquitin, RBP, and kinase, grouped by spatial region; (C) Spatial map of gene module scores for ubiquitin, RBP, and kinases.
4 Discussion
High-throughput sequencing of cancer patient gene expression can significantly enhance personalized and precise treatment strategies for clinicians (Morganti et al., 2019). Most current studies primarily focus on identifying overall gene expression differences between cancerous tissues and adjacent non-tumor tissues, lacking spatial information on complex tissue structures that could elucidate spatial specificity in gene expression (Marx, 2021). The landscape of tumor research has been transformed by advancements in sequencing techniques, yet conventional methods fail to integrate gene expression data with spatial cellular information. In contrast, spatial transcriptome sequencing, an emerging sequencing technique distinct from RNA-sequencing (RNA-seq) and single-cell RNA-seq (scRNA-seq), provides spatial context to gene expression data, offering insights into cellular positional relationship with tissue sections (Burgess, 2019; Wagner et al., 2019). In this study, spatial transcriptomic technology was employed to explore spatial heterogeneity in diffuse high-grade gliomas, characterizing their spatial features and comparing key DEGs between Glio-IDH-mut and Glio-IDH-wt, as well as within the tumors.
Our study identified 5,677 upregulated and 5,016 downregulated DEGs between tumor and non-tumor tissues of diffuse high-grade gliomas, highlighting notable upregulated genes such as SMOC1, APOE, HIPK2, PPP1CB, UBA52, S100A6, CTSB, SPP1, IGFBP2, CALD1, and TMSB4X. Previous studies have validated that these upregulated DEGs, except for SMOC1 and UBA52, were associated with poor prognosis in malignant tumors and presented limited treatment options (Zhang et al., 2014; Abdulla et al., 2017; An et al., 2021; Hu et al., 2021; Liu et al., 2021; Lin et al., 2022; Conte et al., 2023; Zhang Z. et al., 2024; Zhang X. et al., 2024). In gliomas, SMOC1 (Wang J. et al., 2021), S100A6 (Hong et al., 2023), CTSB (Ma et al., 2022), SPP1 (Chen et al., 2019), and IGFBP2 (Moore et al., 2009) serve as a potential therapeutic target in glioma. Similarly, APOE-mediated lipid transport may represent a new therapeutic target in brain tumors (Nicoll et al., 2003). Sardina et al. (2023) summarized that HIPK2 may serve as a therapeutic target and diagnostic or prognostic marker in neurological disorders. PPP1CB has been shown to serve as an oncogene in high-grade glioma by activating the Ras-ERK, JAK3-STAT3, and PI3K-Akt pathways (Chiarle et al., 2008; Aghajan et al., 2016). UBA52, one of the genes associated with ubiquitin, encodes a fusion protein consisting of ubiquitin at the N-terminus and ribosomal protein L40 at the C-terminus (Dubois et al., 2020; Cockram et al., 2021), which has been reported to contribute to the development and progression of various tumors, including colorectal cancer (Zhou et al., 2019) and non-small cell lung cancer (Wang et al., 2019). Other studies have demonstrated that S100A6 (Qi et al., 2023), CTSB (Zhang et al., 2020), and CALD1 (Ma et al., 2024) have been proven to promote cancer cell malignant phenotypes, such as proliferation, migration, invasion, and epithelial-mesenchymal transition. Numerous studies have proved that SPP1-positive macrophages act as metastasis accelerators that promote tumor progression (Liu et al., 2023; Du et al., 2024). A recent study showed that cancer-associated fibroblast-associated gene IGFBP2 facilitated glioma progression by activation of M2 macrophage polarization (Zhang X. et al., 2024). However, the functional role of SMOC1, APOE, HIPK2, UBA52, S100A6, CTSB, SPP1, IGFBP2, CALD1, and TMSB4X in the onset and progression of diffuse high-grade glioma remains unclear.
Exiting studies have linked aberrant activation of the PI3K/Akt pathway (Barzegar Behrooz et al., 2022), human papillomavirus infection (Limam et al., 2020), cytokine-cytokine receptor interaction (Wang J. J. et al., 2021), and human T-cell leukemia virus 1 infection (Jin et al., 2006) with glioma development and progression. In our analysis, we observed similar results, where upregulated DEGs in tumor regions of both Glio-IDH-wt and Glio-IDH-mut exhibited enrichment in pathways such as PI3K/Akt, human papillomavirus infection, cytokine-cytokine receptor interaction, human T-cell leukemia virus 1 infection, cytoskeleton in muscle cells, focal adhesion, human cytomegalovirus infection, regulation of actin cytoskeleton, and human T-cell leukemia virus 1 infection. Meanwhile, the upregulated DEGs of Glio-IDH-mut were mainly enriched in “MAPK signaling pathway,” “tight junction,” “NOD-like receptor signaling pathway,” “protein processing in endoplasmic reticulum,” and “JAK–STAT signaling pathway.” Previous studies have proved that activation of the MAPK pathway (Pan et al., 2023), endoplasmic reticulum stress (Markouli et al., 2020), NOD-like receptor pathway (Chen et al., 2024), and JAK–STAT pathway (Zhang et al., 2019) accelerated glioma progression. Our results also showed that the enrichment analysis results of upregulated DEGs of Glio-IDH-wt were mainly enriched in the relaxin signaling pathway, staphylococcus aureus infection, endocrine resistance, melanoma, and cholesterol metabolism. Several studies have proved that the relaxin pathway may serve as a potential therapeutic approach to control malignant tumors (Neschadim et al., 2015; Klonisch et al., 2017). Cuervo et al. (2010) found that staphylococcus aureus infection may increase mortality in cancer patients. Wang T. et al. (2023), reported that cholesterol transport served as a novel potential target pathway for IDH-mut glioma. Moreover, GO analysis revealed that the upregulated DEGs of both Glio-IDH-wt and Glio-IDH-mut were related to positive regulation of cell adhesion, mononuclear cell differentiation, positive regulation of cytokine production, regulation of cell–cell adhesion, epithelial cell proliferation, and so on, which is consistent with the former studies (Wu et al., 2019). Furthermore, GO analysis found that axonogenesis, cellular process involved in reproduction in multicellular organism, alcohol metabolic process, steroid metabolic process, organic acid biosynthetic process, and developmental maturation were enriched in Glio-IDH-wt, whereas Glio-IDH-mut showed enrichments of muscle system process, cell growth, response to oxidative stress, positive regulation of protein localization, and negative regulation of phosphorus metabolic process. The above results indicated that these DEGs play a crucial role in the development and progression of diffuse high-grade gliomas.
Post-translational modification of proteins is a crucial regulatory mechanism that alters their physical and chemical properties, conformation, and binding ability of proteins, thus affecting their activity, stability, and function (Su et al., 2017). Research on protein post-translational modification in malignant tumors, such as glioma, currently emphasizes on phosphorylation, ubiquitination, glycosylation, and acetylation. These modifications regulate protein function, modulate signaling pathways, and impact downstream gene expression, affecting cancer cell proliferation, invasion, apoptosis, drug resistance, and chemotherapy sensitivity (Han et al., 2018; Cao and Yan, 2020). Moreover, protein post-translational modification is a significant focus in epigenetic research, and recent studies highlight its relevance to glioma pathogenesis, offering diagnostic and therapeutic targets (Pienkowski et al., 2023). Our study reveals that key regulatory genes involved in ubiquitination and RNA binding protein were highly expressed in spatially defined tumor regions of both Glio-IDH-wt and Glio-IDH-mut, while key regulatory genes in kinase were predominantly enhanced in adjacent normal tissue. We identified the top 6 key regulatory genes in ubiquitination (TRIM54, PPARG, KLHL35, DNAI1, WDR78, and CORO1A), RNA binding protein (ENO2, LGls3, HSP90AA1, HSPA1B, and HK2), and kinase (KALRN, LRRK1, MET, PDK1, PET, and TRIB3). Notably, these genes, such as PPARG, HK2, and PDK1, play critical roles in the regulation of aerobic glycolysis in cancer cells, which is associated with the IDH phenotype in GBM cells (Strickland and Stoll, 2017; Dong et al., 2023; Venneti et al., 2023).
In conclusion, our systematic investigation of spatially distributed DEGs in diffuse high-grade gliomas identified 10 key genes and their associated signaling pathways. These findings are supported by existing literature and databases linking these differential genes to malignant tumor progression. This study contributes to the development of personalized treatment strategies for diffuse high-grade gliomas. However, the functional role and underlying mechanism of SMOC1, HIPK2, UBA52, S100A6, PPP1CB, CALD1, and TMSB4X in the occurrence and development of diffuse high-grade gliomas was still unknown. This is also the main point of our future research.
Data availability statement
All data generated in this work are uploaded to the National Genomics Data Center (https://ngdc.cncb.ac.cn) with the BioProject accession number PRJCA027587. All code used in this work is uploaded to GitHub (https://github.com/fuyu-sama/gbm).
Ethics statement
The studies involving humans were approved by The Second Affiliated Hospital of Kunming Medical University. The studies were conducted in accordance with the local legislation and institutional requirements. The participants provided their written informed consent to participate in this study.
Author contributions
YY: Conceptualization, Funding acquisition, Investigation, Methodology, Supervision, Writing – original draft, Resources, Writing – review & editing. YH: Data curation, Formal analysis, Software, Writing – original draft, Methodology, Writing – review & editing. KaZ: Data curation, Investigation, Methodology, Writing – original draft, Validation. MH: Data curation, Investigation, Resources, Writing – original draft. WL: Data curation, Software, Validation, Writing – original draft. KuZ: Investigation, Methodology, Writing – original draft. NZ: Conceptualization, Project administration, Supervision, Writing – review & editing, Funding acquisition.
Funding
The author(s) declare that financial support was received for the research, authorship, and/or publication of this article. This study was supported by the Project of the National Natural Science Foundation of China (No. 82172998 and 81960459), the Project of Yunnan Province Science and Technology Department (No. 202201AY070001-098 and 202301AY070001-123), and the Project of Yunnan Province Medical and health talents (No. YNWR-MY-2018-033).
Conflict of interest
The authors declare that the research was conducted in the absence of any commercial or financial relationships that could be construed as a potential conflict of interest.
Publisher’s note
All claims expressed in this article are solely those of the authors and do not necessarily represent those of their affiliated organizations, or those of the publisher, the editors and the reviewers. Any product that may be evaluated in this article, or claim that may be made by its manufacturer, is not guaranteed or endorsed by the publisher.
Supplementary material
The Supplementary material for this article can be found online at: https://www.frontiersin.org/articles/10.3389/fnmol.2024.1466302/full#supplementary-material
SUPPLEMENTARY FIGURE S1 | Expression of SMOC1, APOE, HIPK2, PPP1CB, UBA52, S100A6, CTSB, SPP1, IGFBP2, CALD1, and TMSB4X in glioblastoma multiforme (GBM) and brain lower grade glioma (LGG) was examined by GEPIA database.
SUPPLEMENTARY FIGURE S2 | Correlation of the expression of SMOC1, APOE, HIPK2, PPP1CB, UBA52, S100A6, CTSB, SPP1, IGFBP2, CALD1, and TMSB4X with overall survival of patient with glioblastoma multiforme (GBM) and brain lower grade glioma (LGG) was examined by GEPIA database.
SUPPLEMENTARY TABLE S1 | DEGs were identified in the cancerous tissues of Glio-IDH-wt compared to adjacent normal tissues.
SUPPLEMENTARY TABLE S2 | DEGs were identified in the cancerous tissues of Glio-IDH-mut compared to adjacent normal tissues.
SUPPLEMENTARY TABLE S3 | Gene list associated with upregulated DEGs in tumor area of Glio-IDH-wt compared with Glio-IDH-mut.
SUPPLEMENTARY TABLE S4 | Gene list associated with ubiquitination, RNA binding protein, and kinase.
Footnotes
References
Abdulla, M. H., Valli-Mohammed, M. A., Al-Khayal, K., Al Shkieh, A., Zubaidi, A., Ahmad, R., et al. (2017). Cathepsin B expression in colorectal cancer in a Middle East population: potential value as a tumor biomarker for late disease stages. Oncol. Rep. 37, 3175–3180. doi: 10.3892/or.2017.5576
Aghajan, Y., Levy, M. L., Malicki, D. M., and Crawford, J. R. (2016). Novel PPP1CB-ALK fusion protein in a high-grade glioma of infancy. BMJ Case Rep. 2016:bcr2016217189. doi: 10.1136/bcr-2016-217189
An, H. W., Kim, S. Y., Kwon, J. W., Seok, S. H., Woo, S. H., Kim, D. Y., et al. (2021). In vivo CRISPR-Cas9 knockout screening using quantitative PCR identifies thymosin beta-4 X-linked that promotes diffuse-type gastric cancer metastasis. Mol. Carcinog. 60, 597–606. doi: 10.1002/mc.23326
Barzegar Behrooz, A., Talaie, Z., Jusheghani, F., Łos, M. J., Klonisch, T., and Ghavami, S. (2022). Wnt and PI3K/Akt/mTOR survival pathways as therapeutic targets in glioblastoma. Int. J. Mol. Sci. 23:1353. doi: 10.3390/ijms23031353
Berglund, E., Maaskola, J., Schultz, N., Friedrich, S., Marklund, M., Bergenstråhle, J., et al. (2018). Spatial maps of prostate cancer transcriptomes reveal an unexplored landscape of heterogeneity. Nat. Commun. 9:2419. doi: 10.1038/s41467-018-04724-5
Buehler, M., Yi, X., Ge, W., Blattmann, P., Rushing, E., Reifenberger, G., et al. (2023). Quantitative proteomic landscapes of primary and recurrent glioblastoma reveal a protumorigeneic role for FBXO2-dependent glioma-microenvironment interactions. Neuro-Oncology 25, 290–302. doi: 10.1093/neuonc/noac169
Burgess, D. J. (2019). Spatial transcriptomics coming of age. Nat. Rev. Genet. 20:317. doi: 10.1038/s41576-019-0129-z
Cable, D. M., Murray, E., Zou, L. S., Goeva, A., Macosko, E. Z., Chen, F., et al. (2022). Robust decomposition of cell type mixtures in spatial transcriptomics. Nat. Biotechnol. 40, 517–526. doi: 10.1038/s41587-021-00830-w
Cao, J., and Yan, Q. (2020). Cancer epigenetics, tumor immunity, and immunotherapy. Trends Cancer 6, 580–592. doi: 10.1016/j.trecan.2020.02.003
Chen, J., Hou, C., Zheng, Z., Lin, H., Lv, G., and Zhou, D. (2019). Identification of secreted phosphoprotein 1 (SPP1) as a prognostic factor in lower-grade gliomas. World Neurosurg. 130, e775–e785. doi: 10.1016/j.wneu.2019.06.219
Chen, J., Liang, R. S., Zhuang, B. B., Chen, H. D., Liu, S., Zhang, G. L., et al. (2024). Cordycepin inhibits glioma growth by downregulating PD-L1 expression via the NOD-like receptor/NFKB1/STAT1 axis. Chem. Biol. Interact. 400:111178. doi: 10.1016/j.cbi.2024.111178
Cheng, Q., Duan, W., He, S., Li, C., Cao, H., Liu, K., et al. (2021). Multi-omics data integration analysis of an immune-related gene signature in LGG patients with epilepsy. Front. Cell Dev. Biol. 9:686909. doi: 10.3389/fcell.2021.686909
Chiarle, R., Voena, C., Ambrogio, C., Piva, R., and Inghirami, G. (2008). The anaplastic lymphoma kinase in the pathogenesis of cancer. Nat. Rev. Cancer 8, 11–23. doi: 10.1038/nrc2291
Cockram, P. E., Kist, M., Prakash, S., Chen, S. H., Wertz, I. E., and Vucic, D. (2021). Ubiquitination in the regulation of inflammatory cell death and cancer. Cell Death Differ. 28, 591–605. doi: 10.1038/s41418-020-00708-5
Comba, A., Faisal, S. M., Varela, M. L., Hollon, T., Al-Holou, W. N., Umemura, Y., et al. (2021). Uncovering spatiotemporal heterogeneity of high-grade gliomas: from disease biology to therapeutic implications. Front. Oncol. 11:703764. doi: 10.3389/fonc.2021.703764
Conte, A., Valente, V., Paladino, S., and Pierantoni, G. M. (2023). HIPK2 in cancer biology and therapy: recent findings and future perspectives. Cell. Signal. 101:110491. doi: 10.1016/j.cellsig.2022.110491
Cuervo, S. I., Cortés, J. A., Sánchez, R., Rodríguez, J. Y., Silva, E., Tibavizco, D., et al. (2010). Risk factors for mortality caused by Staphylococcus aureus bacteremia in cancer patients. Enferm. Infecc. Microbiol. Clin. 28, 349–354. doi: 10.1016/j.eimc.2009.06.015
Dong, W., Liu, Y., Wang, P., Ruan, X., Liu, L., Xue, Y., et al. (2023). U3 snoRNA-mediated degradation of ZBTB7A regulates aerobic glycolysis in isocitrate dehydrogenase 1 wild-type glioblastoma cells. CNS Neurosci. Ther. 29, 2811–2825. doi: 10.1111/cns.14218
Du, J., An, Z. J., Huang, Z. F., Yang, Y. C., Zhang, M. H., Fu, X. H., et al. (2023). Novel insights from spatial transcriptome analysis in solid tumors. Int. J. Biol. Sci. 19, 4778–4792. doi: 10.7150/ijbs.83098
Du, Y., Lin, Y., Gan, L., Wang, S., Chen, S., Li, C., et al. (2024). Potential crosstalk between SPP1 + TAMs and CD8 + exhausted T cells promotes an immunosuppressive environment in gastric metastatic cancer. J. Transl. Med. 22:158. doi: 10.1186/s12967-023-04688-1
Dubois, M. L., Meller, A., Samandi, S., Brunelle, M., Frion, J., Brunet, M. A., et al. (2020). UBB pseudogene 4 encodes functional ubiquitin variants. Nat. Commun. 11:1306. doi: 10.1038/s41467-020-15090-6
Goodwin, S., McPherson, J. D., and McCombie, W. R. (2016). Coming of age: ten years of next-generation sequencing technologies. Nat. Rev. Genet. 17, 333–351. doi: 10.1038/nrg.2016.49
Han, Z. J., Feng, Y. H., Gu, B. H., Li, Y. M., and Chen, H. (2018). The post-translational modification, SUMOylation, and cancer (review). Int. J. Oncol. 52, 1081–1094. doi: 10.3892/ijo.2018.4280
Hao, Y., Hao, S., Andersen-Nissen, E., Mauck, W. M. 3rd, Zheng, S., Butler, A., et al. (2021). Integrated analysis of multimodal single-cell data. Cell 184, 3573–3587.e29. doi: 10.1016/j.cell.2021.04.048
Hong, B., Zhang, H., Xiao, Y., Shen, L., and Qian, Y. (2023). S100A6 is a potential diagnostic and prognostic biomarker for human glioma. Oncol. Lett. 26:458. doi: 10.3892/ol.2023.14045
Hu, L., Xu, H., Wang, X., Wu, B., Chen, F., Chen, W., et al. (2021). The expression and clinical prognostic value of protein phosphatase 1 catalytic subunit beta in pancreatic cancer. Bioengineered 12, 2763–2778. doi: 10.1080/21655979.2021.1934243
Jain, S., Rick, J. W., Joshi, R. S., Beniwal, A., Spatz, J., Gill, S., et al. (2023). Single-cell RNA sequencing and spatial transcriptomics reveal cancer-associated fibroblasts in glioblastoma with protumoral effects. J. Clin. Invest. 133:e147087. doi: 10.1172/jci147087
Jin, Q., Agrawal, L., Vanhorn-Ali, Z., and Alkhatib, G. (2006). GLUT-1-independent infection of the glioblastoma/astroglioma U87 cells by the human T cell leukemia virus type 1. Virology 353, 99–110. doi: 10.1016/j.virol.2006.05.003
Jo, J., Diaz, M., Horbinski, C., Mackman, N., Bagley, S., Broekman, M., et al. (2023). Epidemiology, biology, and management of venous thromboembolism in gliomas: An interdisciplinary review. Neuro-Oncology 25, 1381–1394. doi: 10.1093/neuonc/noad059
Johnson, A. L., Laterra, J., and Lopez-Bertoni, H. (2022). Exploring glioblastoma stem cell heterogeneity: immune microenvironment modulation and therapeutic opportunities. Front. Oncol. 12:995498. doi: 10.3389/fonc.2022.995498
Kanev, G. K., de Graaf, C., Westerman, B. A., de Esch, I. J. P., and Kooistra, A. J. (2021). KLIFS: an overhaul after the first 5 years of supporting kinase research. Nucleic Acids Res. 49, D562–d569. doi: 10.1093/nar/gkaa895
Klonisch, T., Glogowska, A., Thanasupawat, T., Burg, M., Krcek, J., Pitz, M., et al. (2017). Structural commonality of C1q TNF-related proteins and their potential to activate relaxin/insulin-like family peptide receptor 1 signalling pathways in cancer cells. Br. J. Pharmacol. 174, 1025–1033. doi: 10.1111/bph.13559
Limam, S., Missaoui, N., Hmissa, S., Yacoubi, M. T., Krifa, H., Mokni, M., et al. (2020). Investigation of human cytomegalovirus and human papillomavirus in glioma. Cancer Investig. 38, 394–405. doi: 10.1080/07357907.2020.1793352
Lin, X., Zhang, J., Zhao, R. H., Zhang, W. J., Wu, J. F., and Xue, G. (2022). APOE is a prognostic biomarker and correlates with immune infiltrates in papillary thyroid carcinoma. J. Cancer 13, 1652–1663. doi: 10.7150/jca.63545
Liu, X., Qin, J., Nie, J., Gao, R., Hu, S., Sun, H., et al. (2023). ANGPTL2+cancer-associated fibroblasts and SPP1+macrophages are metastasis accelerators of colorectal cancer. Front. Immunol. 14:1185208. doi: 10.3389/fimmu.2023.1185208
Liu, X., Wu, L., Wang, L., and Li, Y. (2024). Identification and classification of glioma subtypes based on RNA-binding proteins. Comput. Biol. Med. 174:108404. doi: 10.1016/j.compbiomed.2024.108404
Liu, Y., Xie, S., Zhu, K., Guan, X., Guo, L., and Lu, R. (2021). CALD1 is a prognostic biomarker and correlated with immune infiltrates in gastric cancers. Heliyon 7:e07257. doi: 10.1016/j.heliyon.2021.e07257
Luo, J., Pan, M., Mo, K., Mao, Y., and Zou, D. (2023). Emerging role of artificial intelligence in diagnosis, classification and clinical management of glioma. Semin. Cancer Biol. 91, 110–123. doi: 10.1016/j.semcancer.2023.03.006
Ma, K., Chen, X., Liu, W., Chen, S., Yang, C., and Yang, J. (2022). CTSB is a negative prognostic biomarker and therapeutic target associated with immune cells infiltration and immunosuppression in gliomas. Sci. Rep. 12:4295. doi: 10.1038/s41598-022-08346-2
Ma, W. Q., Miao, M. C., Ding, P. A., Tan, B. B., Liu, W. B., Guo, S., et al. (2024). CALD1 facilitates epithelial-mesenchymal transition progression in gastric cancer cells by modulating the PI3K-Akt pathway. World J. Gastrointest. Oncol. 16, 1029–1045. doi: 10.4251/wjgo.v16.i3.1029
Markouli, M., Strepkos, D., Papavassiliou, A. G., and Piperi, C. (2020). Targeting of endoplasmic reticulum (ER) stress in gliomas. Pharmacol. Res. 157:104823. doi: 10.1016/j.phrs.2020.104823
Marx, V. (2021). Method of the year: spatially resolved transcriptomics. Nat. Methods 18, 9–14. doi: 10.1038/s41592-020-01033-y
Moore, L. M., Holmes, K. M., Smith, S. M., Wu, Y., Tchougounova, E., Uhrbom, L., et al. (2009). IGFBP2 is a candidate biomarker for Ink4a-Arf status and a therapeutic target for high-grade gliomas. Proc. Natl. Acad. Sci. USA 106, 16675–16679. doi: 10.1073/pnas.0900807106
Morganti, S., Tarantino, P., Ferraro, E., D'Amico, P., Duso, B. A., and Curigliano, G. (2019). Next generation sequencing (NGS): a revolutionary technology in pharmacogenomics and personalized medicine in cancer. Adv. Exp. Med. Biol. 1168, 9–30. doi: 10.1007/978-3-030-24100-1_2
Nakagawa, H., and Fujita, M. (2018). Whole genome sequencing analysis for cancer genomics and precision medicine. Cancer Sci. 109, 513–522. doi: 10.1111/cas.13505
Neschadim, A., Summerlee, A. J., and Silvertown, J. D. (2015). Targeting the relaxin hormonal pathway in prostate cancer. Int. J. Cancer 137, 2287–2295. doi: 10.1002/ijc.29079
Nicoll, J. A., Zunarelli, E., Rampling, R., Murray, L. S., Papanastassiou, V., and Stewart, J. (2003). Involvement of apolipoprotein E in glioblastoma: immunohistochemistry and clinical outcome. Neuroreport 14, 1923–1926. doi: 10.1097/00001756-200310270-00008
Pan, S., and Chen, R. (2022). Pathological implication of protein post-translational modifications in cancer. Mol. Asp. Med. 86:101097. doi: 10.1016/j.mam.2022.101097
Pan, Z., Chen, C., Huang, X., Xiong, Y., Kang, X., Zhou, J., et al. (2023). Migration-inducing gene-7 promotes glioma cell proliferation and invasiveness via activating the MAPK signaling pathway. Neoplasma 70, 534–544. doi: 10.4149/neo_2023_230307N121
Pienkowski, T., Kowalczyk, T., Cysewski, D., Kretowski, A., and Ciborowski, M. (2023). Glioma and post-translational modifications: a complex relationship. Biochim. Biophys. Acta Rev. Cancer 1878:189009. doi: 10.1016/j.bbcan.2023.189009
Qi, X., Lin, Y., Chen, J., and Shen, B. (2020). Decoding competing endogenous RNA networks for cancer biomarker discovery. Brief. Bioinform. 21, 441–457. doi: 10.1093/bib/bbz006
Qi, M., Yi, X., Yue, B., Huang, M., Zhou, S., and Xiong, J. (2023). S100A6 inhibits MDM2 to suppress breast cancer growth and enhance sensitivity to chemotherapy. Breast Cancer Res. 25:55. doi: 10.1186/s13058-023-01657-w
Ren, Y. M., Huang, Z. Y., Zhou, L. L., Xiao, P., Song, J. W., He, P., et al. (2023). Spatial transcriptomics reveals niche-specific enrichment and vulnerabilities of radial glial stem-like cells in malignant gliomas. Nat. Commun. 14:1028. doi: 10.1038/s41467-023-36707-6
Richards, L. M., Whitley, O. K. N., MacLeod, G., Cavalli, F. M. G., Coutinho, F. J., Jaramillo, J. E., et al. (2021). Gradient of developmental and injury response transcriptional states defines functional vulnerabilities underpinning glioblastoma heterogeneity. Nat. Cancer 2, 157–173. doi: 10.1038/s43018-020-00154-9
Sardina, F., Conte, A., Paladino, S., Pierantoni, G. M., and Rinaldo, C. (2023). HIPK2 in the physiology of nervous system and its implications in neurological disorders. Biochim. Biophys. Acta, Mol. Cell Res. 1870:119465. doi: 10.1016/j.bbamcr.2023.119465
Ståhl, P. L., Salmén, F., Vickovic, S., Lundmark, A., Navarro, J. F., Magnusson, J., et al. (2016). Visualization and analysis of gene expression in tissue sections by spatial transcriptomics. Science 353, 78–82. doi: 10.1126/science.aaf2403
Strickland, M., and Stoll, E. A. (2017). Metabolic reprogramming in glioma. Front. Cell Dev. Biol. 5:43. doi: 10.3389/fcell.2017.00043
Su, M. G., Weng, J. T., Hsu, J. B., Huang, K. Y., Chi, Y. H., and Lee, T. Y. (2017). Investigation and identification of functional post-translational modification sites associated with drug binding and protein-protein interactions. BMC Syst. Biol. 11:132. doi: 10.1186/s12918-017-0506-1
Sun, L., Kienzler, J. C., Reynoso, J. G., Lee, A., Shiuan, E., Li, S., et al. (2023). Immune checkpoint blockade induces distinct alterations in the microenvironments of primary and metastatic brain tumors. J. Clin. Invest. 133:e169314. doi: 10.1172/JCI169314
Tang, Z., Kang, B., Li, C., Chen, T., and Zhang, Z. (2019). GEPIA2: an enhanced web server for large-scale expression profiling and interactive analysis. Nucleic Acids Res. 47, W556–W560. doi: 10.1093/nar/gkz430
Venneti, S., Kawakibi, A. R., Ji, S., Waszak, S. M., Sweha, S. R., Mota, M., et al. (2023). Clinical efficacy of ONC201 in H3K27M-mutant diffuse midline gliomas is driven by disruption of integrated metabolic and epigenetic pathways. Cancer Discov. 13, 2370–2393. doi: 10.1158/2159-8290.Cd-23-0131
Wagner, J., Rapsomaniki, M. A., Chevrier, S., Anzeneder, T., Langwieder, C., Dykgers, A., et al. (2019). A single-cell atlas of the tumor and immune ecosystem of human breast cancer. Cell 177, 1330–1345.e18. doi: 10.1016/j.cell.2019.03.005
Wang, X., Almet, A. A., and Nie, Q. (2023). The promising application of cell-cell interaction analysis in cancer from single-cell and spatial transcriptomics. Semin. Cancer Biol. 95, 42–51. doi: 10.1016/j.semcancer.2023.07.001
Wang, F., Chen, X., Yu, X., and Lin, Q. (2019). Degradation of CCNB1 mediated by APC11 through UBA52 ubiquitination promotes cell cycle progression and proliferation of non-small cell lung cancer cells. Am. J. Transl. Res. 11, 7166–7185
Wang, F., Long, J., Li, L., Wu, Z. X., Da, T. T., Wang, X. Q., et al. (2023). Single-cell and spatial transcriptome analysis reveals the cellular heterogeneity of liver metastatic colorectal cancer. Sci. Adv. 9:eadf5464. doi: 10.1126/sciadv.adf5464
Wang, Z., Tang, W., Yuan, J., Qiang, B., Han, W., and Peng, X. (2020). Integrated analysis of RNA-binding proteins in glioma. Cancers (Basel) 12:892. doi: 10.3390/cancers12040892
Wang, J. J., Wang, H., Zhu, B. L., Wang, X., Qian, Y. H., Xie, L., et al. (2021). Development of a prognostic model of glioma based on immune-related genes. Oncol. Lett. 21:116. doi: 10.3892/ol.2020.12377
Wang, J., Xia, S., Zhao, J., Gong, C., Xi, Q., and Sun, W. (2021). Prognostic potential of secreted modular calcium-binding protein 1 in low-grade glioma. Front. Mol. Biosci. 8:666623. doi: 10.3389/fmolb.2021.666623
Wang, T., Zhou, Y., Fan, Y., Duan, H., Guo, X., Chang, J., et al. (2023). PERK-mediated cholesterol excretion from IDH mutant glioma determines anti-tumoral polarization of microglia. Adv. Sci. (Weinh) 10:e2205949. doi: 10.1002/advs.202205949
Wu, T., Hu, E., Xu, S., Chen, M., Guo, P., Dai, Z., et al. (2021). clusterProfiler 4.0: a universal enrichment tool for interpreting omics data. Innovation (Camb.) 2:100141. doi: 10.1016/j.xinn.2021.100141
Wu, F., Zhao, Z., Chai, R. C., Liu, Y. Q., Li, G. Z., Jiang, H. Y., et al. (2019). Prognostic power of a lipid metabolism gene panel for diffuse gliomas. J. Cell. Mol. Med. 23, 7741–7748. doi: 10.1111/jcmm.14647
Zhang, B., Hong, C. Q., Luo, Y. H., Wei, L. F., Luo, Y., Peng, Y. H., et al. (2022). Prognostic value of IGFBP2 in various cancers: a systematic review and meta-analysis. Cancer Med. 11, 3035–3047. doi: 10.1002/cam4.4680
Zhang, Z., Liu, B., Lin, Z., Mei, L., Chen, R., and Li, Z. (2024). SPP1 could be an immunological and prognostic biomarker: from pan-cancer comprehensive analysis to osteosarcoma validation. FASEB J. 38:e23783. doi: 10.1096/fj.202400622RR
Zhang, X., Sun, X., Guo, C., Li, J., and Liang, G. (2024). Cancer-associated fibroblast-associated gene IGFBP2 promotes glioma progression through induction of M2 macrophage polarization. Am. J. Physiol. Cell Physiol. 326, C252–C268. doi: 10.1152/ajpcell.00234.2023
Zhang, Y., Xia, Q., and Lin, J. (2019). Runx1 promotes the development of glioma cells by regulating JAK-STAT signalling pathway. Arch. Med. Sci. 18, 761–776. doi: 10.5114/aoms.2019.87268
Zhang, J., Zhang, K., Jiang, X., and Zhang, J. (2014). S100A6 as a potential serum prognostic biomarker and therapeutic target in gastric cancer. Dig. Dis. Sci. 59, 2136–2144. doi: 10.1007/s10620-014-3137-z
Zhang, J., Zhou, B., Jin, S., Huang, Z., Ma, B., Shao, Q., et al. (2020). Mechanism of action of Panax notoginoside against lung cancer in mice based on response to CTSB gene. BMC Complement. Med. Ther. 20:367. doi: 10.1186/s12906-020-03159-0
Zhao, S. Y., Wang, Q., Ni, K. X., Zhang, P. P., Liu, Y., Xie, J. H., et al. (2023). Combining single-cell sequencing and spatial transcriptome sequencing to identify exosome-related features of glioblastoma and constructing a prognostic model to identify BARD1 as a potential therapeutic target for GBM patients. Front. Immunol. 14:1263329. doi: 10.3389/fimmu.2023.1263329
Zhou, Q., Hou, Z., Zuo, S., Zhou, X., Feng, Y., Sun, Y., et al. (2019). LUCAT1 promotes colorectal cancer tumorigenesis by targeting the ribosomal protein L40-MDM2-p53 pathway through binding with UBA52. Cancer Sci. 110, 1194–1207. doi: 10.1111/cas.13951
Zhou, J., Xu, Y., Lin, S., Guo, Y., Deng, W., Zhang, Y., et al. (2018). iUUCD 2.0: an update with rich annotations for ubiquitin and ubiquitin-like conjugations. Nucleic Acids Res. 46, D447–D453. doi: 10.1093/nar/gkx1041
Keywords: glioma, isocitrate dehydrogenase, spatial transcriptomics, therapeutic target, key regulatory genes
Citation: Yang Y, Hong Y, Zhao K, Huang M, Li W, Zhang K and Zhao N (2024) Spatial transcriptomics analysis identifies therapeutic targets in diffuse high-grade gliomas. Front. Mol. Neurosci. 17:1466302. doi: 10.3389/fnmol.2024.1466302
Edited by:
Peng Jin, Emory University, United StatesReviewed by:
Shuquan Rao, Chinese Academy of Medical Sciences and Peking Union Medical College, ChinaYulin Jin, Emory University, United States
Copyright © 2024 Yang, Hong, Zhao, Huang, Li, Zhang and Zhao. This is an open-access article distributed under the terms of the Creative Commons Attribution License (CC BY). The use, distribution or reproduction in other forums is permitted, provided the original author(s) and the copyright owner(s) are credited and that the original publication in this journal is cited, in accordance with accepted academic practice. No use, distribution or reproduction is permitted which does not comply with these terms.
*Correspondence: Ninghui Zhao, zhaoninghui@hotmail.com
†These authors have contributed equally to this work