- 1Medical Support Center, No. 940 Hospital of the Joint Logistics Support Force of the Chinese People’s Liberation Army, Lanzhou, China
- 2Gansu University of Traditional Chinese Medicine, Lanzhou, China
Epilepsy is a chronic neurological disorder that poses significant challenges to patients and their families. Effective detection and prediction of epilepsy can facilitate patient recovery, reduce family burden, and streamline healthcare processes. Therefore, it is essential to propose a deep learning method for efficient detection and prediction of epileptic electroencephalography (EEG) signals. This paper reviews several key aspects of epileptic EEG signal processing, focusing on epilepsy detection and prediction. It covers publicly available epileptic EEG datasets, preprocessing techniques, feature extraction methods, and deep learning-based networks used in these tasks. The literature is categorized based on patient independence, distinguishing between patient-independent and non-patient-independent studies. Additionally, the evaluation methods are classified into general classification indicators and specific epilepsy prediction criteria, with findings organized according to the prediction cycles reported in various studies. The review reveals several important insights. Despite the availability of public datasets, they often lack diversity in epilepsy types and are collected under controlled conditions that may not reflect real-world scenarios. As a result, signal preprocessing methods tend to be limited and may not fully represent practical conditions. Feature extraction and network designs frequently emphasize fusion mechanisms, with recent advances in Convolutional Neural Networks (CNNs) and Recurrent Neural Networks (RNNs) showing promising results, suggesting that new network models warrant further exploration. Studies using patient-independent data generally produce better results than those relying on non-patient-independent data. Metrics based on general classification methods typically perform better than those using specific epilepsy prediction criteria, though future research should focus on the latter for more accurate evaluation. Epilepsy prediction cycles are typically kept under 1 h, with most studies concentrating on intervals of 30 min or less.
1 Introduction
Epilepsy is a chronic neurological disorder affecting over 50 million people worldwide, according to the World Health Organization. It can impact individuals of all ages and is characterized by recurrent seizures, which result from sudden, abnormal discharges of neurons in the brain (Pelkonen et al., 2020). These seizures can vary widely in severity and presentation, ranging from brief lapses in awareness to full-body convulsions. The underlying causes of epilepsy are diverse, including genetic, infectious, structural, immune, metabolic, and sometimes unknown factors (Scheffer et al., 2017). The primary treatments for epilepsy include medication and surgery, but these interventions are not always effective (Perucca, 2021). Approximately 30% of patients continue to experience recurrent seizures despite treatment (Liu et al., 2021). These seizures can lead to a range of symptoms, including loss of consciousness, muscle twitching, and difficulty breathing (World Health Organization, 2024). In severe cases, seizures can result in falls, injury, and even drowning, posing significant risks to life. Additionally, the social implications of epilepsy, such as the potential for discrimination and the stigma associated with the condition, can exacerbate psychological distress. Studies have shown that individuals with epilepsy, especially children and adolescents, are more likely to suffer from anxiety and depression due to the chronic and unpredictable nature of their condition (Puka et al., 2017). EEG, which records the brain’s electrical activity, is a crucial tool in diagnosing and managing epilepsy. However, analyzing EEG data is time-consuming and requires the expert of trained neurologists. The accuracy of diagnosis heavily depends on the neurologist’s experience and skill. This traditional approach has its limitations: particularly given the complexity and volume of EEG data that must be reviewed (Chandani and Kumar, 2018). In recent years, the development of deep learning techniques has offered new possibilities for improving epilepsy detection and prediction. These advanced algorithms can process and analyze large volumes of EEG data more efficiently than traditional methods, assisting neurologists in diagnosing epilepsy and predicting seizures more accurately. By providing timely warnings, these technologies can help patients take preventive measures, reducing the physical and psychological impact of seizures. This paper provides a comprehensive review of the current research on epileptic EEG signal detection and prediction using deep learning methods. EEG has the advantages of simplicity, safety, high temporal resolution, and high utilization, making it a key tool in diagnosing epilepsy (Wei et al., 2021; Ein Shoka et al., 2023). Given this prevalence, this paper concentrates on reviewing literature specifically related to EEG signals in the context of epilepsy. We will explore four key areas: the nature of epileptic EEG data, preprocessing techniques, feature extraction methods, and deep learning-based detection and prediction algorithms. This review also addresses gaps in existing literature, including issues related to data partitioning, model evaluation methods, and prediction timeframes, offering a more detailed perspective on the state of the field.
2 Epileptic EEG signals
2.1 Partitioning of epileptic EEG signal states
EEG signals of an epileptic patient can be categorized into four states: Post-ictal state, Inter-ictal state: Pre-ictal state and Ictal state. The pre-ictal state is the state minutes before the actual occurrence of the seizure. The ictal state is the state actual occurrence of the seizure. The post-ictal state is the state after the seizure has passed. The inter-ictal state is the state between post-ictal state and preictal state. Signals during ictal and inter-ictal periods are often used as data for detecting the occurrence of epilepsy. Signals during pre-ictal and inter-ictal periods are often used as data to predict whether epilepsy will occur or not (Aslam et al., 2022). Figure 1 illustrates the four states of epileptic EEG signals.
2.2 Data presentation
The 10–20 International Standard Electrode Placement System, established by the International Federation of Clinical Neurophysiology, is widely recognized as the standard method for electrode placement in EEG data acquisition (Maillard and Ramantani, 2017). This system is employed by most publicly available epileptic datasets, ensuring consistency and reliability in data collection. In this section, we will discuss commonly used datasets in epilepsy research. Most of these datasets are freely accessible, with the exception of the Bonn dataset, which requires a purchase. These datasets typically organize epileptic EEG data on a patient-by-patient basis, with each patient’s data stored in separate folders. This organization leads to two primary methods of data segmentation for epilepsy detection and prediction: patient-independent and non-patient-independent methods.
Patient-independent methods refer to data from a particular patient as training, validation, and testing data for the model. This data partitioning approach can be further divided into two types: segment-based approach and event-based approach. Segment-based approach means that the data of a particular patient is divided into training, validation, and testing sets. The event-based approach means: dividing the data of a patient into k groups according to the number of seizures, using the k-1 group of data as the training and validation set, and the kth group of data as the test set. This approach generally yields more accurate evaluation results compared to non-patient-independent methods. However, it requires a substantial amount of patient-specific data, which must be recorded over different seizure periods to effectively train the model for detecting or predicting seizures in that particular patient. Figure 2A shows the segment-based data partitioning method and Figure 2B shows the event-based data partitioning method.
On the other hand, non-patient-independent methods refer to the use of all patients’ data as training, validation, and testing data for the model. This data partitioning can be further divided into two types: the all-patient approach and the cross-patient approach. The difference is as follows: the all-patient approach divides data from all patients into training, validation, and testing sets. The cross-patient approach refers to dividing the data of n-1 patients as the training set and validation set, and the remaining patient data as the test set. Figure 3A shows the all-patient data division method and Figure 3B shows the cross-patient data division method.
3 Techniques for pre-processing EEG signals in epilepsy
During EEG acquisition, artifacts such as electrocardiogram (ECG) signals, electromyogram (EMG) signals, and thermal noise can contaminate the data. Effective preprocessing of these artifacts is essential for improving the accuracy of epilepsy detection and prediction models. Several techniques are widely employed in the preprocessing of epileptic EEG signals, including thermal noise reduction, artifact removal, and data enhancement methods.
3.1 Thermal noise processing of EEG signals
Electromagnetic interference in the ambient environment and thermal noise inherent in the device can severely damage the low-amplitude EEG signal. The raw EEG signal is highly non-stationary and dynamic, and the scalp EEG itself is small in amplitude, so it is easily affected by high-frequency interferences as well as 50 Hz or 60 Hz signals (Lakehal and Ferdi, 2024; Wang et al., 2024). A 50 Hz or 60 Hz notch filter is commonly used to remove the industrial frequency noise (Raghu et al., 2020). High-frequency noise is filtered out using a high pass filter, low pass filter, band pass filter, etc (Liu et al., 2022).
3.2 Removal of artifacts in EEG signals
To address thermal noise and artifacts in EEG data, various signal processing methods have been developed. Techniques such as Wavelet Transform (WT), Empirical Mode Decomposition (EMD), and Blind Source Separation (BSS) are commonly employed to identify and remove these artifacts.
WT denoising works by using a basis function to compute the wavelet coefficient that represent the degree of similarity between the basis function and the original signal. Noise is removed by setting a threshold for these coefficients; those below the threshold are discarded, and the remaining coefficients are used to reconstruct the denoised signal. For example, Zhou et al. (2021) proposed a dynamic thresholding method based on Discrete Wavelet Transform (DWT) for artifact removal, which has proven effective in enhancing EEG signal quality. Similarly, Yedurkar and Metkar (2020) applied DWT with adaptive filtering to remove low-frequency physiological artifacts while retaining more useful signal components.
However, the basis function in wavelet denoising is set manually and lacks adaptability. This limitation can be addressed by EMD, which decomposes any complex signal into multiple Intrinsic Mode Functions (IMFs) at different frequencies. A denoised signal can be obtained by setting a threshold to discard inappropriate IMFs and reconstructing the remaining ones. Parija et al. (2020) processed EEG signals by selecting the first four high-frequency IMFs from EMD, although the selection criteria lack a strong theoretical basis. Moctezuma and Molinas (2020) further refined EMD by filtering out noisy IMFs using Minkowski distance, while Karabiber Cura et al. (2020) applied energy, correlation, and other statistical measures to screen suitable IMFs for both EMD and Ensemble Empirical Mode Decomposition (EEMD). Hassan et al. (2020) obtained clean EEG signals using Complete Ensemble Empirical Mode Decomposition with Adaptive Noise (CEEMDAN) and NIG parameters. Bari and Fattah (2020) concluded that extracting the first IMF components is the most desirable by comparing the amplitudes and frequencies of IMFs obtained after CEEMDAN decomposition. Variational Modal Decomposition (VMD) offers an alternative approach, solving the problem of mode mixing in EMD and reducing computational complexity, as demonstrated by Liu et al. (2022), who removed noise by correlating VMD functions with the original signal. Peng et al. (2021) proposed the Elastic Variational Mode Decomposition (EVMD) algorithm, which is able to capture the center frequency variations of EEG signals in various frequency band segments compared to VMD, thus improving the experimental performance.
However, EMD has limitations, including mode mixing, noise introduction, and high computational complexity. These drawbacks can be addressed by BSS. BSS operates on the principle that a signal can be represented as a linear combination of different source signals. By decomposing the signal into multiple source components, discarding the noisy ones, and reconstructing the remaining components, a denoised signal can be obtained. Classical BSS techniques include Canonical Correlation Analysis (CCA), Principal Component Analysis (PCA), and Independent Component Analysis (ICA). While CCA and PCA are effective for analyzing linear signals, ICA is better suited for nonlinear signals, making it a more commonly used method for artifact removal in EEG data. Islam et al. (2020) introduced an advanced ICA variant, Infomax ICA, which showed improved reliability in separating components based on mutual information. Becker et al. (2015) enhanced ICA with a penalized semialgebraic deflation algorithm, reducing computational complexity while maintaining performance. Beyond conventional approaches, several innovative methods have been developed for denoising epileptic EEG signals. Sardouie et al. (2014) introduced two semi-blind source separation techniques based on time-frequency analysis: Time-Frequency Generalized Eigenvalue Decomposition (TF-GEVD) and Time-Frequency Denoised Source Separation (TF-DSS). These methods were shown to outperform traditional techniques. Qiu et al. (2018) proposed the Denoising Sparse Autoencoder (DSAE), an advanced deep neural network that combines the strengths of sparse and denoising autoencoders. By enforcing sparsity in the hidden layers, this method efficiently represents EEG signals, particularly when dealing with non-smooth, noisy data. The DSAE’s ability to learn robust representations of the underlying EEG signals makes it a valuable tool for improving the accuracy of seizure detection. Lopes et al. (2021) explored a deep learning approach by developing a Deep Convolutional Neural Network (DCNN) model for artifact removal in EEG data. This model is particularly effective in handling nonlinear signal sources and removing various artifacts, including those caused by eye blinks, muscle activity, and channel motion. Despite its complexity, the DCNN model offers significant improvements in denoising performance.
However, BSS requires a reference waveform to effectively remove noise by linking it with multiple source signal waveforms. Recognizing that each denoising method has its strengths and limitations, researchers have also explored hybrid approaches to improve the purity of EEG signals. Du et al. (2024) employed a combination of CEEMDAN and Continuous Wavelet Transform (CWT) for joint denoising of epileptic EEG signals. This approach further improves signal quality by integrating the benefits of both CEEMDAN and CWT, offering a more comprehensive noise reduction strategy. Please refer to Table 2 for details.
3.3 Data enhancement
In addition to artifact removal, data enhancement techniques are crucial for improving the quality of EEG signals, especially when dealing with small or imbalanced datasets. Data augmentation methods, such as flipping, windowing, and adding noise, are commonly used to artificially expand datasets (Wan et al., 2023). Palanisamy and Rengaraj (2024) employed these techniques by increasing the amplitude and applying random transformations to EEG signals, thereby enhancing the robustness of deep learning models.
Generative Adversarial Networks (GANs) represent a more sophisticated approach to data augmentation. Initially proposed by Goodfellow et al. (2014), GANs generate synthetic data that can balance training datasets, as demonstrated by Gao et al. (2022) with their GAN-based model for seizure detection. Temporal Generative Adversarial Networks (TGAN) and Wasserstein Generative Adversarial Networks (WGANs) have also been employed to address temporal dependencies and gradient vanishing issues in EEG data, respectively. Nasiri and Clifford (2021) propose a Generative Transferable Adversarial Network (GTAN) that generates transferable adversarial features to address the performance degradation of traditional algorithms due to inter-individual variation in EEG data. A comparison of each method is shown in Table 3.
4 Feature extraction techniques for epileptic EEG signals
Feature extraction can streamline and organize underlying patterns, making them more manageable. Although neural networks are capable of automatically identifying useful features from raw data, well-engineered features can simplify problem-solving. This approach often requires less data and fewer resources to achieve effective results (Francois, 2022). Feature extraction in EEG signal analysis involves time-domain features, frequency-domain features, time-frequency domain features, and nonlinear dynamics features. Time-domain features provide a general overview of the signal’s distribution but do not capture frequency-related information. Conversely, frequency-domain features convert signals from the time domain to the frequency domain, enabling the extraction of frequency-specific information. Time-frequency domain methods offer a more nuanced approach by representing spectral signals over time, combining both temporal and spectral analyses (Pham, 2021; Qin et al., 2024). Nonlinear dynamics, on the other hand, are used to quantify the chaotic nature of EEG signals, offering insights into their complexity (Yan et al., 2022).
Sun and Chen (2022) utilized entropy-based features, such as Sample Entropy (SampEn), Permutation Entropy (PermEn), and Fuzzy Entropy (FuzzyEn), both individually and in combination, to form three-dimensional feature vectors. Their findings indicated that combining SampEn, PermEn, and FuzzyEn produced the highest accuracy and recall rates. However, this approach did not incorporate time or frequency domain information. Fei et al. (2017) addressed this limitation by employing the Fractional Fourier Transform (FrFT), the adaptive maximal Lyapunov exponent, and its energy, which captured both the chaotic and frequency domain characteristics of epileptic EEG signals. Wang et al. (2019) Extracting features of the signal using Teager energy entropy, curve length and Teager-Huang transform results in better results than ordinary energy entropy. Zhang et al. (2024) took a more comprehensive approach by extracting features using DWT, Power Spectral Density (PSD), standard deviation, band energy, and FuzzyEn. While this method effectively gathered diverse feature information, it also introduced redundancy and increased computational complexity. To mitigate these issues, Zhang et al. (2018) generated 2,794 features for each sample using multiple feature extraction methods. These features were evaluated by a series of three consecutive selection algorithms, i.e., VarA, iRFE, and BackFS, to screen important features. Table 4 presents a summary of various feature extraction methods employed in the analysis of epileptic EEG signals.
5 Algorithms for detection and prediction of epileptic EEG signals
After preprocessing and feature extraction on the data, the processed signal is then fed into the network model for deep feature extraction and classification.
5.1 Assessment of indicators
In evaluating deep learning models for epileptic EEG signal detection and prediction, specific assessment metrics are employed to measure performance effectively.
For seizure detection, the evaluation is typically straightforward, relying on standard classification metrics such as Accuracy, Sensitivity, Specificity, Precision, F1 Score, Receiver Operating Characteristic (ROC) curve, Area Under the ROC Curve (AUC), and False Positive Rate (FPR). These metrics provide a clear measure of how well the model distinguishes between seizure and non-seizure events.
In contrast, seizure prediction models are designed to differentiate between inter-ictal (periods between seizures) and pre-ictal (periods just before a seizure) states, which requires a more nuanced approach. While prediction models often use the same metrics as detection models, a specialized set of criteria—known as the Epilepsy Prediction Evaluation Criteria—was introduced by Maiwald et al. (2004). This method includes several key metrics: Maximum False Positive Rate (FPRmax): The highest allowable prediction error rate within a given time interval. Seizure Onset Period (SOP): The timeframe during which a seizure is expected to occur following a prediction. Seizure Prediction Horizon (SPH): The time interval between the prediction and the SOP. Figure 4 provides a schematic representation of SPH and SOP.
The process for applying these criteria begins with setting initial values for FPRmax, SPH, and SOP. The model’s parameters are then adjusted to ensure that the false prediction rate for each patient remains within the set FPRmax. The sensitivity for each patient is calculated, and the average sensitivity across all patients is determined. This process is repeated iteratively to establish reasonable ranges for FPRmax, SPH, and SOP, as shown in Figure 5. This methodology builds on earlier work by Osorio et al. (1998), who emphasized the importance of considering both FPR and sensitivity in prediction models. Notably, there is a trade-off between the FPR and sensitivity.
5.2 Convolutional neural networks
CNNs, first introduced in 1980, can learn both feature extraction and classification layers within the network. This dual learning enhances the network’s generalization ability, making CNNs particularly effective for processing complex signals (Hassan et al., 2024). Given the non-linear and intricate nature of EEG signals, CNNs are well-suited for their analysis (Hassan et al., 2024). CNNs are structured with several key components: an input layer, convolutional layers, pooling layers, fully connected layers, and an output layer. The convolutional layers, equipped with convolutional kernels, are crucial for extracting features from the input data. Pooling layers further compress the feature space, which reduces computational complexity. The fully connected layers consolidate these features and serve as classifiers. This architecture allows CNNs to be both efficient in computation and effective in training due to the localized and globally shared connections between neurons in the convolutional layers. Figure 6 shows the Diagram of CNN.
Several studies have explored CNNs for epilepsy detection. Acharya et al. (2018) introduced a 1-Dimensional Convolutional Neural Network (1D-CNN) that directly classifies EEG signals without prior feature extraction. Their approach achieved an average accuracy of 88.7%, with a sensitivity of 95% and specificity of 90%. In contrast, Wang et al. (2021) used a stacked 1D-CNN on two public datasets, also skipping pre-processing and feature extraction. Their results demonstrated high accuracy and specificity (over 99%), but the absence of a test set leaves their findings open to debate. Avcu et al. (2019) utilized a 2-Dimensional Convolutional Neural Network (2D-CNN) with only two channels of data, outperforming models that used 18 channels. Das et al. (2024) compared 1D-CNN and 2D-CNN by reshaping data into both formats, finding that the 2D-CNN offered superior classification performance. Pan et al. (2022) proposed a lightweight 2D-CNN that processed both raw data and data transformed by various techniques, such as Discrete Fourier Transform (DFT), Short-Time Fourier Transform (STFT), DWT. While this hybrid method improved detection performance with limited data, the lack of a test set data raised concerns about model reliability. Xu et al. (2024) introduced a 3-Dimensional Convolutional Neural Network (3D-CNN) aimed at reducing the latency of seizure detection through probabilistic prediction, achieving detection delays at least 50% shorter than previously reported.
Table 5 lists various epilepsy detection models based on CNNs, detailing their accuracy (Acc), sensitivity (Sen), and specificity (Spe). The table also indicates the data partitioning methods used, with #1 indicating a segment-based data partitioning approach, #2 indicating an event-based data partitioning approach, *1 indicating an all-patient-based data partitioning approach. If the symbols (#1,#2,*1) are not indicated, it means that the method of partitioning the dataset is not applicable or not specified in the article.
CNNs prediction based on metrics of common classification methods: Shafiezadeh et al. (2024) introduced a calibration pipeline using a 2D-CNN model. By incorporating a small amount of data from test set patients into the training set, their approach fine-tunes the model to predict epileptic events from new patients. This method improved accuracy, sensitivity, and specificity by over 15% on the CHB-MIT dataset and by more than 19% on a non-public dataset. But the computational complexity is too high. Zhang et al. (2021) Proposed Lightweight-2D-CNN reduces computational complexity.
Table 6 outlines various CNNs prediction models and their performance metrics, including accuracy (Acc), sensitivity (Sen), specificity (Spe), and pre-epileptic time (PT) in minutes. It also denotes data partitioning methods: #2 indicating an event-based data partitioning approach, *1 indicating an all-patient-based data partitioning approach, and *2 indicating a cross-patient data partitioning approach. * indicates that the article only describes how the data are divided for non-independent patients and does not mention a specific way of partitioning the data. If the symbols (#2,*1,*2,*) are not indicated, it means that the method of partitioning the dataset is not applicable or not specified in the article.
CNNs prediction model based on evaluation criteria for epilepsy prediction: Truong et al. (2018) proposed a 2D-CNN model tested on both scalp and intracranial EEG data. Their model achieved a sensitivity greater than 89% across both datasets, though the Freiburg dataset exhibited a higher FPR. Ra and Li (2023) utilized Simultaneous Extractive Transform (SET) and Singular Value Decomposition (SET-SVD) to enhance time-frequency resolution, achieving a sensitivity over 99.7% with a 1D-CNN model. Ozcan and Erturk (2019) explored spatiotemporal correlations in EEG signals using a 3D-CNN, which showed an 85.7% sensitivity and an FPR of 0.096/h on the CHB-MIT dataset. To improve interpretability: Prathaban and Balasubramanian (2021) developed a dynamic learning framework with a 3D-CNN model optimized by the Fletcher Reeves algorithm. Their phase-transform-based method demonstrated precise real-time seizure prediction using scalp EEG data.
Table 7 presents CNN prediction models based on evaluation criteria such as sensitivity (Sen), FPR, SOP, and SPH, all measured in minutes. It also denotes data partitioning methods: # indicates that the article only describes how the data are divided for independent patients and does not mention a specific way of partitioning the data. If the # is not indicated, it means that the method of partitioning the dataset is not applicable or not specified in the article.
5.3 Recurrent neural networks
RNNs are designed to process time series data and consist of an input, hidden, and output layer. Unlike CNNs, RNNs allow neurons to receive information not only from other neurons but also from themselves, creating a network with loops. This loop structure enables RNNs to maintain a memory of previous inputs through hidden units, making them particularly suitable for processing EEG data. Figure 7 illustrates the structure of an RNN, where ht represents the hidden state at time t, and the delay mechanism records the most recent hidden state. However, RNNs often struggle with the issue of vanishing gradients, which is mitigated by LSTM and GRU.
LSTM networks address this issue by using input and forget gates to manage memory. As shown in Figure 8A, LSTM cells use the external state ht − 1 at time t − 1 and the input xt at time tt to calculate the forget gate ft, input gate it, output gate ot, and candidate state ĉt. These elements are then combined with the memory unit ct − 1 to update the memory unit ct at time tt. Finally, the external state ht at time tt is derived from the memory unit ct and the output gate ot. While the complementary relationship between the forget and input gates enhances the LSTM’s memory management, it also introduces some redundancy.
GRU offers a simpler alternative to LSTM by consolidating the input and forget functions into a single gate. Figure 8B shows the structure of a GRU cell, where the reset gate rt controls the influence of the previous state ht − 1 on the candidate state ĥt. The update gate zt regulates the balance between retaining information from the historical state and incorporating new information from the candidate state. xt represents the input at time tt, and ht is the resulting state.
For epilepsy detection using RNNs, Hu et al. (2020) proposed a Bi-directional Long Short-Term Memory Network (Bi-LSTM) that classifies EEG data using 11 time-domain features extracted through Local Mean Decomposition (LMD). Their method achieved an average sensitivity of 93.61% and specificity of 91.85%. Zhang et al. (2022) also employed a Bi-directional Gated Recurrent Unit (Bi-GRU) for epilepsy detection. They utilized DWT and wavelet energy features, achieving an average sensitivity of 93% and specificity of 98.49%. Tuncer and Bolat (2022) used only instantaneous frequency and spectral entropy features with a Bi-LSTM network, obtaining an average classification accuracy of 97.78%, surpassing Hu’s results but using the Bonn dataset.
In terms of epilepsy prediction, Singh and Malhotra (2022) used a Two-Layer Long Short-Term Memory Network (2 L-LSTM), incorporating Fast Fourier Transform (FFT), spectral power, and mean spectral amplitude for feature extraction. Tsiouris et al. (2018) employed a range of features, including statistical moments, time-domain features, and various transforms (FFT, SD, DWT), within a 2 L-LSTM framework. Their approach demonstrated sensitivity and specificity greater than 99%, outperforming Kuldeep’s results.
5.4 Generating adversarial networks
GANs were proposed by Goodfellow et al. (2020). GANs consist of two components: a generator and a discriminator. The generator creates synthetic data, which is then evaluated by the discriminator to determine its authenticity. Feedback from the discriminator helps both components improve their performance. The structure of GANs is shown in Figure 9. Some of the epileptic EEG datasets have less amount of data, e.g., New Delhi dataset, Bonn dataset, this situation leads to poor learning ability of the network and risk of overfitting. GANs are capable of generating simulated data that is very similar to the real data compared to the other data enhancement methods, which improves the performance of the model. GANs have recently been explored for epilepsy classification tasks.
Truong et al. (2019) were among the first to apply GANs to seizure prediction. They used a Deep Convolutional Generative Adversarial Network (DCGAN) to learn features from Time-Frequency domain signals processed with STFT. Despite their innovative approach, DCGAN is known for issues with gradient vanishing and instability (Fathallah et al., 2023).
5.5 Transfer learning
Transfer learning can be traced back to the late 20th century and the early 21st century (Burrello et al., 2019; Pan and Yang, 2009). Transfer learning is the use of knowledge gained from one task to improve performance on a related task. First, a basic network is trained on the source dataset and task, and then the learned features (network weights) are applied to a new network trained on a different but related dataset and task. This approach helps to address the problem of EEG data scarcity, and compared to other network models, transfer learning can reduce the resources and time required to train deep learning models (Yang et al., 2024; Weiss et al., 2016; Butt et al., 2024)
In the context of epilepsy detection and prediction, Liang et al. (2020) utilized a combination of the Visual Geometry Group network (VGG) and LSTM for analyzing EEG signals. Their method demonstrated strong results through cross-validation, though sensitivity for some patients was as low as 60%. Gao et al. (2020) employed three deep convolutional neural networks—Inception-ResNet-v2 (Inception Residual Network Version 2), Inception-v3 Network (Inception-v3), and Residual Network 152 (ResNet152)—to classify EEG data into various categories: interictal, reictal I, reictal II, and postictal. They set preictal I and II durations to 30 min and 10 min, respectively, to assess the importance of event duration. Takahashi et al. (2020) proposed an Autoencoder-assisted VGG Network (AE-VGG-16) for seizure detection, which significantly reduced FPR. Toraman (2020) compared three pre-trained CNN models: VGG16, Residual Network (ResNet), and Densely Connected Network (DenseNet)—using spectrogram images to differentiate between pre-seizure and inter-seizure states. The study found that the ResNet model provided the best performance.
5.6 Fusion model
Fusion modeling combines different network types to leverage their strengths and mitigate their weaknesses. This approach enhances model performance by integrating various methodologies. Transformers and Residual blocks are commonly used in fusion models. The Transformer was first proposed in 2014 by Bahdanau (2014). A Transformer Network includes an input layer, encoder, decoder, and output layer, with a multi-head attention mechanism added between the encoder and decoder (Wang et al., 2024; Zhang et al., 2024a; Cho et al., 2014). This mechanism effectively manages the performance of the model as EEG sequence length increases and improves processing efficiency for long sequences, thus reducing time complexity (De Santana Correia and Colombini, 2022; Rukhsar and Tiwari, 2023). Figure 10A shows the Transformer structure. The ResNet was introduced in 2015 by He et al. (2016). The residual block helps to retain the data from the previous layers and solve problems such as overfitting of the EEG signal on other networks and gradient vanishing. Figure 10B shows the residual block structure.
In the field of epilepsy detection, Sunaryono et al. (2022) introduced a hybrid model combining a 1D-CNN with majority voting and Deep Neural Networks (DNNs). This method achieved 100% accuracy in detecting epilepsy from EEG signals, though its effectiveness may vary with datasets other than the University of Bonn dataset. Awais et al. (2024) proposed a Graph Convolutional Neural Network-Long Short-Term Memory Network (GCN-LSTM), which takes into account the spatial aspects of EEG signals. Huang et al. (2024) developed a Temporal Convolutional Neural Network with a Self-Attention (TCN-SA) layer to extract crucial features using self-attention. Zhu et al. (2024) introduced a Squeeze-and-Excitation Temporal Convolutional Network with Bidirectional Gated Recurrent Units (SE-TCN-Bi-GRU) that automatically selects important EEG channels, though it involves multiple parameters. Zhou et al. (2024) proposed a Lightweight Multi-Attention EEG Network (LMA-EEGNet) that reduces parameter count and network complexity while maintaining effective feature extraction.
Table 8 summarizes various detection algorithms based on hybrid models, detailing their accuracy (Acc), sensitivity (Sen), and specificity (Spe). Data partitioning methods are indicated, with #1 indicating a segment-based data partitioning approach, #2 indicating an event-based data partitioning approach, *2 indicating a cross-patient data partitioning approach. # indicates that the article only describes how the data are divided for independent patients and does not mention a specific way of partitioning the data; * indicates that the article only describes how the data are divided for non-independent patients and does not mention a specific way of partitioning the data. If the symbols (#1,#2,*2,#,*) are not indicated, it means that the method of partitioning the dataset is not applicable or not specified in the article.
Fusion Model Predictions Based on Common Classification Indicators: Aslam et al. (2022) employed a Convolutional Neural Network combined with Long Short-Term Memory (CNN-LSTM) to classify EEG signals and predict seizures. This approach yielded effective results in classifying temporal sequences. To enhance the handling of long-term temporal dependencies, Indurani and Vandana (2023) utilized a Time-Attention Convolutional Neural Network with a Recurrent Neural Network (TA-CNN-LSTM). Meanwhile, Ma et al. (2023) introduced a Convolutional Bi-directional Long Short-Term Memory Network model based on Multi-Channel Feature Fusion (MCFF-CNN-Bi-LSTM). This model, which integrates an attention mechanism and channel fusion, effectively manages long-term temporal signals while reducing computational complexity.
Table 9 summarizes various hybrid models evaluated using predictive and categorical assessment methods. Accuracy (Acc), sensitivity (Sen), and specificity (Spe) are the primary metrics. Data partitioning methods are indicated, with #1 indicating a segment-based data partitioning approach, #2 indicating an event-based data partitioning approach, and *2 indicating a cross-patient data partitioning approach. # indicates that the article only describes how the data are divided for independent patients and does not mention a specific way of partitioning the data; * indicates that the article only describes how the data are divided for non-independent patients and does not mention a specific way of partitioning the data. If the symbols (#1,#2,*2,#,*) are not indicated, it means that the method of partitioning the dataset is not applicable or not specified in the article.
Fusion Model Predictions Based on Epilepsy-Specific Evaluation Criteria: Wei et al. (2019) used a CNN-LSTM model to predict epileptic seizures, focusing on its effectiveness in handling EEG signals. Yan et al. (2022) proposed a three-transformer tower model to address the spatial and temporal characteristics of EEG signals. However, this model may suffer from parameter redundancy and insufficient representation of spatial structures. Liu et al. (2024) introduced a Pseudo 3D-CNN combined with Directed Convolutional Long Short-Term Memory (P3D BiConvLstm3D), which employs Max-Relevance and Min-Redundancy (mRMR) and a 3D channel attention mechanism to enhance feature selection and reduce redundancy.
Table 10 provides a summary of hybrid models based on predictive and epilepsy-specific evaluation criteria. Sensitivity (Sen), FPR, SOP, SPH are key metrics. Data partitioning methods are indicated, with #1 indicating a segment-based data partitioning approach, #2 indicating an event-based data partitioning approach. # indicates that the article only describes how the data are divided for independent patients and does not mention a specific way of partitioning the data.
Ngiam et al. (2011) pioneered the use of deep learning techniques to integrate and learn from multiple types of data. Multimodal learning in deep learning extends beyond EEG signals, incorporating various data types to enhance model performance in real-time epilepsy detection. By integrating diverse data sources, these models achieve more accurate and comprehensive results.
Ahmedt-Aristizabal et al. (2018) utilized a CNN-LSTM model to combine features from facial, body, and hand movements, effectively distinguishing between Mesial Temporal Lobe Epilepsy (MTLE) and Extratemporal Lobe Epilepsy (ETLE). This approach highlights the effectiveness of incorporating multiple types of input data in improving diagnostic accuracy. Sirpal et al. (2019) integrated Functional Near-Infrared Spectroscopy (fNIRS) with EEG in a multimodal EEG-fNIRS-LSTM-RNN model, achieving up to an 8% improvement in classification accuracy. This model demonstrates the benefits of combining different data modalities for enhanced seizure detection. Hosseini et al. (2020) proposed a model combining Long Short-Term Memory-Support Vector Machine (LSTM-SVM) with edge computing, using both resting state-functional Magnetic Resonance Imaging (rs-fMRI) and EEG data. This approach allows for autonomous localization of epileptic foci and improved prediction capabilities. Martini et al. (2021) employed Stereo Electroencephalogram (SEEG) and video electroencephalogram data in a self-supervised LSTM-based network. This model’s use of self-supervised dynamic thresholding enhanced robustness and efficiency, significantly improving sensitivity in real-time epilepsy prediction. Aluvalu et al. (2024) introduced a Multimodal Convolutional Neural Network (MMFCNN) utilizing a cross-attention mechanism. By filtering data through a cognitive framework and analyzing patient movements, gestures, and facial expressions, the MMFCNN achieved an impressive accuracy rate of 99.2%.
6 Discussion
Among the publicly available datasets for epilepsy detection and prediction, the CHB-MIT dataset is the most widely used. This preference is due to several advantages of the CHB-MIT dataset: Large Volume of Data: CHB-MIT provides a substantial amount of data, which supports effective model training. Flexible Data Structure: The dataset is organized by patient, with each patient’s data stored in separate folders. It includes continuous recordings of both seizure and non-seizure phases, making it suitable for various analytical needs. Researchers can utilize the data for both detection and prediction tasks, with the flexibility to employ both patient-independent and non-patient-independent data partitions. Additionally, it allows for customization of seizure periods, inter-ictal, and pre-ictal phases, enabling more precise model analysis.
Despite its benefits, the CHB-MIT dataset, like other publicly available datasets, has notable limitations. The scope of epilepsy datasets is limited, and the data are collected under controlled conditions that may not capture the full complexity of real-life epilepsy occurrences. For instance, epilepsy can manifest during diverse activities such as walking, eating, or exercising, where EEG signals can be highly variable. Current public datasets may not fully address these real-world scenarios, leaving gaps in the comprehensiveness of available data.
Most research in the field employs patient-independent data partitioning, with only a few studies using non-patient-independent methods. While non-patient-independent partitioning is less common, it is an essential approach for certain analyses. Additionally, some studies do not clearly specify their data segmentation methods, which can lead to confusion among readers. This paper argues that explicitly stating the data segmentation approach is crucial for enhancing the clarity and understanding of the literature.
EEG preprocessing and data enhancement methods vary widely in the literature. Many researchers employ basic filtering techniques for EEG signals. The artifact removal methods such as WT, EMD, and BSS can improve the data quality, but they require the use of reasonable thresholds. Some researchers, on the other hand, have achieved satisfactory classification results by using raw data directly. This practice may be dictated by the nature of overt epileptic EEG signals, which are usually collected under controlled conditions where subjects try to remain as motionless as possible, making the partial noise very faint. However, real-world conditions differ, as epileptic patients may not stay stationary. Data augmentation using GANs involves complex training processes with high computational demands. A more accessible approach is window overlapping, which segments data to enhance its volume effectively. Combining multiple publicly available EEG datasets is also common, as it not only increases the volume of data but also demonstrates the model’s generalization capability and reliability.
Given the strengths and limitations of various feature extraction methods, feature fusion techniques have become popular. Feature fusion combines EEG features of different dimensions to provide a more comprehensive view of the data. However, an excessive number of features can lead to information redundancy. To address this issue, several feature selection methods have been proposed, including the Fisher score, Group Search Algorithm, Crow Search Algorithm, and Optimal Feature Selection Algorithm (Kapoor et al., 2023; Subasi et al., 2019). Traditional manual feature extraction methods often suffer from low generalization and suboptimal performance. To overcome these limitations, researchers have increasingly integrated manual feature extraction with deep learning techniques (Cao et al., 2019; Yuan et al., 2018). This combined approach leverages the strengths of both methods, enhancing model performance and accuracy in epileptic seizure detection and prediction.
Currently, evaluation criteria for epilepsy detection tasks are relatively standardized, focusing primarily on classification metrics. For epilepsy prediction tasks, evaluation methods are categorized into two main types: those based on classification metrics and those specific to epilepsy prediction. Despite their widespread use, these criteria have notable shortcomings: particularly in the definition and application of the FPR. Most studies define FPR as the number of false positives recorded within an hour of EEG monitoring. However, there is variation in this definition across the literature. For instance, one approach uses confusion matrices to determine FPR, while another defines it as the number of false positives during the event interval (Wei et al., 2018; Khan et al., 2017). This latter method can be problematic because, with varying intervals between episodes, longer intervals result in a lower FPR, while shorter intervals lead to a higher FPR. This inconsistency makes it challenging to compare results across different studies and datasets.
Deep learning algorithms for epilepsy detection and prediction have seen a wide range of approaches. For our study, we reviewed more than 2,000 research papers on machine learning and deep learning techniques for detecting and predicting epileptic events using EEG signals. These papers are from ScienceDirect and Springer databases and span from 2020 to 2024. We conducted a keyword visualization analysis of the trends in these papers using VOSviewer software. The results of the analysis show that deep learning, as a subset of machine learning: lays an important role in processing EEG signals for epilepsy detection and prediction, as shown in Figure 11. CNNs are the most commonly used models in this field. In contrast, RNNs, GANs, and transfer learning models are less frequently employed. GANs, initially used primarily for data augmentation, were first applied to epilepsy prediction in 2019, highlighting their potential for further development in this area (Truong et al., 2019). Multimodal deep learning methods offer the advantage of learning features from various perspectives, enabling real-time detection and prediction. However, these methods require substantial data and advanced hardware, which can be limiting. Spiking Neural Networks (SNNs), which model biological neuron dynamics and offer high capacity with low energy consumption, are less commonly used due to their training difficulties (Akopyan et al., 2015; Sengupta et al., 2019). Zhang et al. (2024b) have addressed this issue by introducing a biologically inspired impulse recurrent neural network that performs well in cross-patient epilepsy detection, potentially expanding the practical applications of SNNs. In response to the high memory consumption and redundancy issues associated with traditional CNNs, Liu et al. (2024) proposed a Cosine Convolutional Neural Network (CosCNN) that reduces memory cost by nearly 75% with minimal loss in accuracy. Another innovation, the Capsule Network introduced by Sabour et al. (2017), overcomes the limitation of CNNs in capturing feature relationships and has been successfully applied to epilepsy detection and prediction (Li et al., 2022; Toraman, 2021).
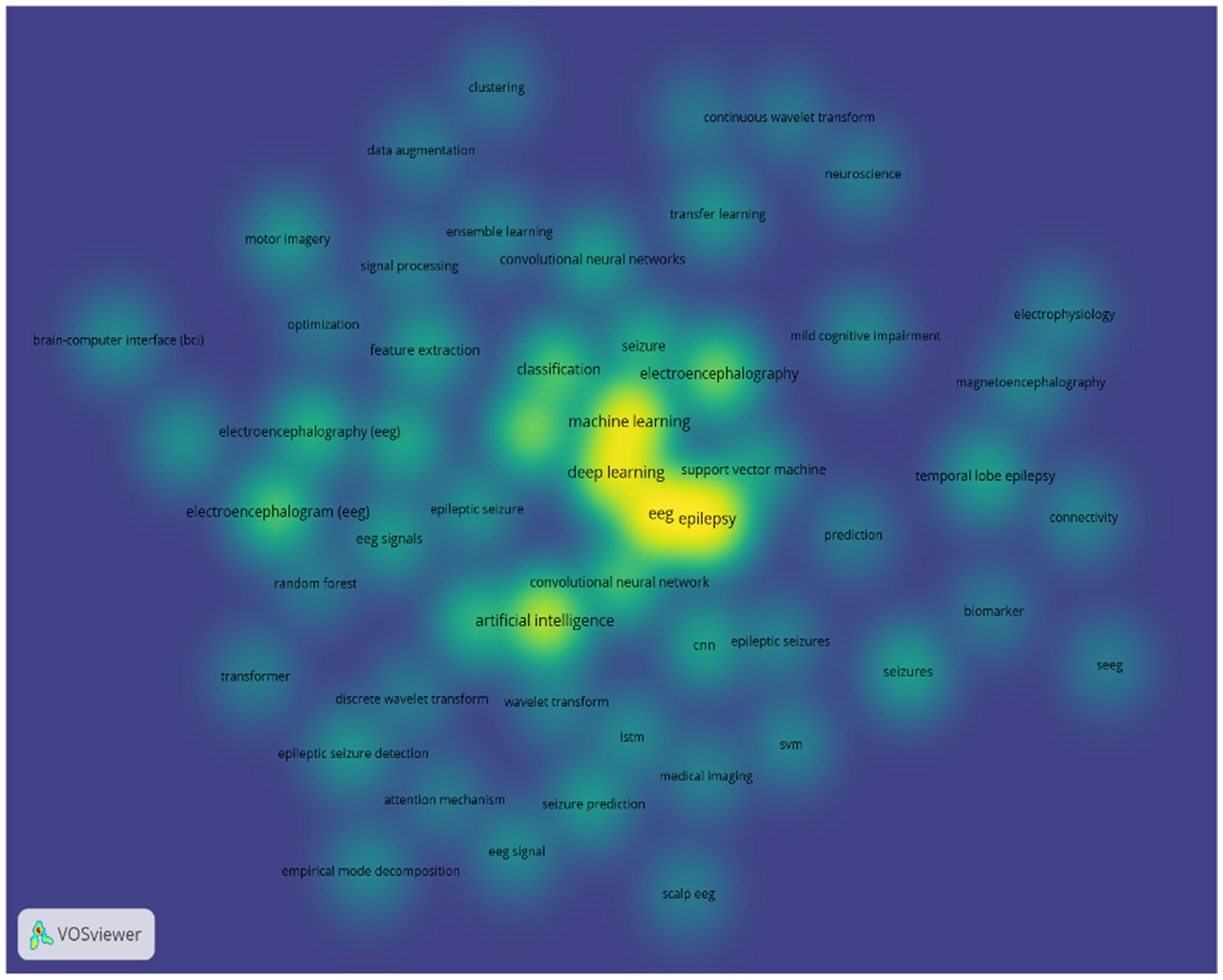
Figure 11. Analysis of machine learning and deep learning methods for EEG-based epilepsy detection and prediction.
Fusion models, which combine various algorithms, generally yield better results. Attention mechanisms are frequently employed in these models due to their ability to handle long time series data efficiently, making them well-suited for epileptic EEG signals. Combining CNNs with RNNs is also common, leveraging CNNs for feature extraction and RNNs for time series processing to capture both deep features and temporal aspects of EEG data. With the increasing availability of publicly accessible datasets, researchers are now combining multiple datasets to enhance model generalization and recognition. However, it is crucial to differentiate between validation and test sets: validation data can be used for model training, while test data should remain unseen during training to ensure accurate evaluation.
Overall, algorithms for epilepsy detection tend to outperform those for epilepsy prediction. This discrepancy may be attributed to dataset characteristics, such as those found in the CHB-MIT and Freiburg datasets. The fixed seizure periods in detection tasks create a more distinct contrast between ictal and interictal states, making classification easier compared to the subtler differences between pre-ictal and interictal periods.
Evaluation criteria for epilepsy prediction often involve stricter requirements aligned with clinical judgments. Typical pre-seizure windows are defined as less than 1 h, with specific periods such as 1–15 min for SPH and 30 min for SOP. These criteria reflect the challenges of aligning predictive algorithms with practical clinical needs.
7 Conclusion
Epilepsy is a chronic neurological disorder that significantly affects both the physical and mental health of patients, potentially leading to conditions such as anxiety and depression (Watanabe et al., 2024). For healthcare providers, manually analyzing a large volume of epileptic EEG signals is extremely challenging, with detection accuracy heavily reliant on individual expertise. As technology advances, employing deep learning techniques for epilepsy.
Significant progress has been made in improving algorithms for preprocessing, feature extraction, and neural networks. Nevertheless, several limitations remain. First, there is a scarcity of diverse, publicly available datasets for different types of epilepsy, with most data collected under specific conditions that may not reflect real-world scenarios. Second, data preprocessing methods are often limited and lack variety. Third, there is inconsistency in the evaluation of FPR. Fourth, no standard evaluation criteria exist for epilepsy prediction models. Fifth, the ability to distinguish between interictal and preictal periods needs further refinement. Sixth, many studies rely on empirical definitions for seizure prediction and evaluation, with inconsistent settings for SPH and SOP. Seventh, high computational and time complexity of current models hampers real-time detection capabilities.
To address these issues, future research should focus on: (1) expanding the dataset to include more diverse epilepsy cases; (2) integrating methods like temporal and feature thresholding to enhance interpretability and segmentation between interictal and preictal periods; (3) utilizing advanced seizure prediction features to optimize parameters such as SPH, SOP, and FPRmax; (4) refining algorithmic models to minimize memory and computational demands, thus facilitating real-time detection; and (5) exploring models from other research areas to enhance the effectiveness of epilepsy detection and prediction systems.
Author contributions
XizZ: Writing – original draft, Writing – review & editing. XiaZ: Writing – review & editing. QH: Writing – review & editing. FC: Writing – review & editing.
Funding
The author(s) declare that financial support was received for the research, authorship, and/or publication of this article. This study was supported by the Natural Science Foundation of Gansu Province [Grant no. 22JR5RA002].
Acknowledgments
I want to thank my tutor for his patient guidance, gratitude for my tutor and classmates for their advice and encouragement.
Conflict of interest
The authors declare that the research was conducted in the absence of any commercial or financial relationships that could be construed as a potential conflict of interest.
Publisher’s note
All claims expressed in this article are solely those of the authors and do not necessarily represent those of their affiliated organizations, or those of the publisher, the editors and the reviewers. Any product that may be evaluated in this article, or claim that may be made by its manufacturer, is not guaranteed or endorsed by the publisher.
References
Acharya, U. R., Oh, S. L., Hagiwara, Y., Tan, J. H., and Adeli, H. (2018). Deep convolutional neural network for the automated detection and diagnosis of seizure using EEG signals. Comput. Biol. Med. 100, 270–278. doi: 10.1016/j.compbiomed.2017.09.017
Ahmedt-Aristizabal, D., Fookes, C., Denman, S., Nguyen, K., Fernando, T., Sridharan, S., et al. (2018). A hierarchical multimodal system for motion analysis in patients with epilepsy. Epilepsy Behav. 87, 46–58. doi: 10.1016/j.yebeh.2018.07.028
Akopyan, F., Sawada, J., Cassidy, A., Alvarez-Icaza, R., Arthur, J., Merolla, P., et al. (2015). Truenorth: design and tool flow of a 65 mw 1 million neuron programmable neurosynaptic chip. IEEE Trans. Comput. Aided Des. Integr. Circuits Syst. 34, 1537–1557. doi: 10.1109/TCAD.2015.2474396
Aluvalu, R., Aravinda, K., Maheswari, V. U., Kumar, K. J., Rao, B. V., and Prasad, K. M. (2024). Designing a cognitive smart healthcare framework for seizure prediction using multimodal convolutional neural network. Cogn. Neurodyn. 1, 1–13. doi: 10.1007/s11571-023-10049-x
Andrzejak, R. G., Lehnertz, K., Mormann, F., Rieke, C., David, P., and Elger, C. E. (2001). Indications of nonlinear deterministic and finite-dimensional structures in time series of brain electrical activity: dependence on recording region and brain state. Phys. Rev. E 64:061907. doi: 10.1103/PhysRevE.64.061907
Aslam, M. H., Usman, S. M., Khalid, S., Anwar, A., Alroobaea, R., Hussain, S., et al. (2022). Classification of EEG signals for prediction of epileptic seizures. Appl. Sci. 12:7251. doi: 10.3390/app12147251
Avcu, M. T., Zhang, Z., and Shih Chan, D. W. (2019). Seizure detection using least EEG channels by deep convolutional neural network. arxiv 2019:3229. doi: 10.1109/ICASS2019.8683229
Awais, M., Belhaouari, S. B., and Kassoul, K. (2024). Graphical insight: revolutionizing seizure detection with EEG representation. Biomedicines 12:1283. doi: 10.3390/biomedicines12061283
Bahdanau, D. (2014). Neural machine translation by jointly learning to align and translate. arxiv :0473. doi: 10.48550/arXiv.1409.0473
Bari, M. F., and Fattah, S. A. (2020). Epileptic seizure detection in EEG signals using normalized IMFs in CEEMDAN domain and quadratic discriminant classifier. Biomed. Signal Process. Control 58:101833. doi: 10.1016/j.bspc.2019.101833
Bbrinkm Sbaldassano Will Cukierski . (2014). Data from: UPenn and Mayo Clinic's seizure detection challenge. Kaggle. Available at: https://kaggle.com/competitions/seizure-detection.
Becker, H., Albera, L., Comon, P., Kachenoura, A., and Merlet, I. (2015). A penalized semialgebraic deflation ICA algorithm for the efficient extraction of interictal epileptic signals. IEEE J. Biomed. Health Inform. 21, 94–104. doi: 10.1109/JBHI.2015.2504126
Brinkmann, B. H., Wagenaar, J., Abbot, D., Adkins, P., Bosshard, S. C., Chen, M., et al. (2016). Crowdsourcing reproducible seizure forecasting in human and canine epilepsy. Brain 139, 1713–1722. doi: 10.1093/brain/aww045
Burrello, A., Cavigelli, L., Schindler, K., Benini, L., and Rahimi, A. (2019). Laelaps: an energy-efficient seizure detection algorithm from long-term human iEEG recordings without false alarms. In: 2019 design, Automation and Test in Europe Conference and Exhibition (DATE), IEEE, pp. 752–757.
Burrello, A., Schindler, K., Benini, L., and Rahimi, A. (2018). One-shot learning for iEEG seizure detection using end-to-end binary operations: local binary patterns with hyperdimensional computing. In: 2018 IEEE biomedical circuits and systems conference (BioCAS), pp. 1–4.
Butt, U. M., Letchmunan, S., Hassan, F. H., and Koh, T. W. (2024). Leveraging transfer learning with deep learning for crime prediction. PLoS One 19:e0296486. doi: 10.1371/journal.pone.0296486
Cao, J., Zhu, J., Hu, W., and Kummert, A. (2019). Epileptic signal classification with deep EEG features by stacked CNNs. IEEE Trans. Cogn. Dev. Syst. 12, 709–722. doi: 10.1109/tcds.2019.2936441
Chandani, M., and Kumar, A. (2018). EEG signal processing for epileptic seizure prediction by using MLPNN and SVM classifiers. Am. J. Inf. Sci. Technol. 2, 36–41. doi: 10.11648/j.ajist.20180202.12
Chen, X., Wang, Q., Hu, C., and Wang, C. (2024). A stock market decision-making framework based on CMR-DQN. Appl. Sci. 14:6881. doi: 10.3390/app14166881
Chen, W., Wang, Y., Ren, Y., Jiang, H., Du, G., Zhang, J., et al. (2023). An automated detection of epileptic seizures EEG using CNN classifier based on feature fusion with high accuracy. BMC Med. Inform. Decis. Mak. 23:96. doi: 10.1186/s12911-023-02180-w
Cho, K., Van Merriënboer, B., Bahdanau, D., and Bengio, Y. (2014). On the properties of neural machine translation: encoder-decoder approaches. arxiv :4012. doi: 10.3115/v1/W14-4012
Choi, W., Kim, M. J., Yum, M. S., and Jeong, D. H. (2022). Deep convolutional gated recurrent unit combined with attention mechanism to classify pre-ictal from interictal EEG with minimized number of channels. J. Pers. Med. 12:763. doi: 10.3390/jpm12050763
Craley, J., Johnson, E., and Venkataraman, A. (2019). Integrating convolutional neural networks and probabilistic graphical modeling for epileptic seizure detection in multichannel EEG. In: information processing in medical imaging: 26th international conference, IPMI 2019, Hong Kong, China, June 2–7, 2019: roceedings 26. Springer International Publishing, pp. 291–303.
Daoud, H., and Bayoumi, M. A. (2019). Efficient epileptic seizure prediction based on deep learning. IEEE Trans. Biomed. Circuits Syst. 13, 804–813. doi: 10.1109/TBCAS.2019.2929053
Das, S., Mumu, S. A., Akhand, M. A. H., Salam, A., and Kamal, M. A. S. (2024). Epileptic seizure detection from decomposed EEG signal through 1D and 2D feature representation and convolutional neural network. Information 15:256. doi: 10.3390/info15050256
De Santana Correia, A., and Colombini, E. L. (2022). Attention: lease! A survey of neural attention models in deep learning. Artif. Intell. Rev. 55, 6037–6124. doi: 10.1007/s10462-022-10148-x
De Vos, M., Deburchgraeve, W., Cherian, P. J., Matic, V., Swarte, R. M., Govaert, P., et al. (2011). Automated artifact removal as preprocessing refines neonatal seizure detection. Clin. Neurophysiol. 122, 2345–2354. doi: 10.1016/j.clinph.2011.04.026
Detti, D. (2020). Siena scalp EEG Database (version 1.0.0): hysioNet. Available at: https://physionet.org/content/siena-scalp-eeg/1.0.0/.
Du, Y., Li, G., Wu, M., and Chen, F. (2024). Unsupervised multivariate feature-based adaptive clustering analysis of epileptic EEG signals. Brain Sci. 14:342. doi: 10.3390/brainsci14040342
Ein Shoka, A. A., Dessouky, M. M., El-Sayed, A., and Hemdan, E. E. D. (2023). EEG seizure detection: concepts, techniques, challenges, and future trends. Multimed. Tools Appl. 82, 42021–42051. doi: 10.1007/s11042-023-15052-2
Fathallah, M., Sakr, M., and Eletriby, S. (2023). Stabilizing and improving training of generative adversarial networks through identity blocks and modified loss function. IEEE Access 11, 43276–43285. doi: 10.1109/ACCESS.2023.3272032
Fei, K., Wang, W., Yang, Q., and Tang, S. (2017). Chaos feature study in fractional Fourier domain for preictal prediction of epileptic seizure. Neurocomputing 249, 290–298. doi: 10.1016/j.neucom.2017.04.019
Francois, C. (2022). “Machine learning fundamentals” in The Python Deep Learning. ed. T. Xie (Beijing: People’s Post and Telecommunications Press), 124–125.
Ganti, B., Chaitanya, G., Balamurugan, R. S., Nagaraj, N., Balasubramanian, K., and Pati, S. (2022). Time-series generative adversarial network approach of deep learning improves seizure detection from the human thalamic SEEG. Front. Neurol. 13:755094. doi: 10.3389/fneur.2022.755094
Gao, Y., Gao, B., Chen, Q., Liu, J., and Zhang, Y. (2020). Deep convolutional neural network-based epileptic electroencephalogram (EEG) signal classification. Front. Neurol. 11:375. doi: 10.3389/fneur.2020.00375
Gao, B., Zhou, J., Yang, Y., Chi, J., and Yuan, Q. (2022). Generative adversarial network and convolutional neural network-based EEG imbalanced classification model for seizure detection. Biocybern. Biomed. Eng. 42, 1–15. doi: 10.1016/j.bbe.2021.11.002
Goldberger, A., Amaral, L., Glass, L., Hausdorff, J., Ivanov, C., Mark, R., et al. (2000). PhysioBank: hysioToolkit, and PhysioNet: components of a new research resource for complex physiologic signals. Circulation 101, e215–e220. doi: 10.1161/01.cir.101.23.e215
Goodfellow, I., Pouget-Abadie, J., Mirza, M., Xu, B., Warde-Farley, D., Ozair, S., et al. (2014). Generative adversarial nets. ArXiv [Preprint]. Available at: https://arxiv.org/pdf/1406.2661v1 (Accessed September 27, 2024).
Goodfellow, I., Pouget-Abadie, J., Mirza, M., Xu, B., Warde-Farley, D., Ozair, S., et al. (2020). Generative adversarial networks. Commun. ACM 63, 139–144. doi: 10.1145/3422622
Harati, A., Lopez, S., Obeid, I., Jacobson, M., Tobochnik, S., and Picone, J. Data from: The TUH EEG CORPUS: A big data resource for automated EEG interpretation. (2014). Available at: https://www.researchgate.net/publication/276921148_The_TUH_EEG_CORPUS_A_big_data_resource_for_automated_EEG_interpretation.
Hassan, J., Reza, M. S., Ahmed, S. U., Anik, N. H., and Khan, M. O. (2024). EEG workload estimation and classification: a systematic review. J. Neural Eng. 16:705. doi: 10.1088/1741-2552/ad705e
Hassan, A. R., Subasi, A., and Zhang, Y. (2020). Epilepsy seizure detection using complete ensemble empirical mode decomposition with adaptive noise. Knowl.-Based Syst. 191:105333. doi: 10.1016/j.knosys.2019.105333
He, K., Zhang, X., Ren, S., and Sun, J. (2016). Deep residual learning for image recognition. In: Proceedings of the IEEE conference on computer vision and pattern recognition, pp. 770–778.
Hosseini, M. P., Tran, T. X., Pompili, D., Elisevich, K., and Soltanian-Zadeh, H. (2020). Multimodal data analysis of epileptic EEG and rs-fMRI via deep learning and edge computing. Artif. Intell. Med. 104:101813. doi: 10.1016/j.artmed.2020.101813
Hu, W., Cao, J., Lai, X., and Liu, J. (2023). Mean amplitude spectrum based epileptic state classification for seizure prediction using convolutional neural networks. J. Ambient. Intell. Humaniz. Comput. 14, 15485–15495. doi: 10.1007/s12652-019-01220-6
Hu, X., Yuan, S., Xu, F., Leng, Y., Yuan, K., and Yuan, Q. (2020). Scalp EEG classification using deep bi-LSTM network for seizure detection. Comput. Biol. Med. 124:103919. doi: 10.1016/j.compbiomed.2020.103919
Huang, N. E., Shen, Z., Long, S. R., Wu, M. C., Shih, H. H., Zheng, Q., et al. (1998). The empirical mode decomposition and the Hilbert spectrum for nonlinear and non-stationary time series analysis. Proc. R. Soc. London A Math Phys Eng Sci 454, 903–995. doi: 10.1098/rspa.1998.0193
Huang, L., Zhou, K., Chen, S., Chen, Y., and Zhang, J. (2024). Automatic detection of epilepsy from EEGs using a temporal convolutional network with a self-attention layer. Biomed. Eng. Online 23:50. doi: 10.1186/s12938-024-01244-w
Hussain, W., Sadiq, M. T., Siuly, S., and Rehman, A. U. (2021). Epileptic seizure detection using 1 D-convolutional long short-term memory neural networks. Appl. Acoust. 177:107941. doi: 10.1016/j.apacoust.2021.107941
Ihle, M., Feldwisch-Drentrup, H., Teixeira, C. A., Witon, A., Schelter, B., Timmer, J., et al. (2012). EPILEPSIAE–A European epilepsy database. Comput. Methods Prog. Biomed. 106, 127–138. doi: 10.1016/j.cmpb.2010.08.011
Indurani, P., and Vandana, S. (2023). Improving deep learning for seizure detection using GAN with Cramer distance and a temporal-spatial- frequency loss function. Int. J. Recent Innov. Trends Comput. Commun. 11, 424–432. doi: 10.17762/ijritcc.v11i6s.6949
Islam, M. S., El-Hajj, A. M., Alawieh, H., Dawy, Z., Abbas, N., and El-Imad, J. (2020). EEG mobility artifact removal for ambulatory epileptic seizure prediction applications. Biomed. Signal Process. Control 55:101638. doi: 10.1016/j.bspc.2019.101638
Jana, G. C., Agrawal, A., Pattnaik, K., and Sain, M. (2022). DWT-EMD feature level fusion based approach over multi and single channel EEG signals for seizure detection. Diagnostics 12:324. doi: 10.3390/diagnostics12020324
Jana, R., and Mukherjee, I. (2023). Efficient seizure prediction and EEG channel selection based on multi-objective optimization. IEEE Access 11, 54112–54121. doi: 10.1109/ACCESS.2023.3281450
Jemal, I., Abou-Abbas, L., Henni, K., Mitiche, A., and Mezghani, N. (2024). Domain adaptation for EEG-based, cross-subject epileptic seizure prediction. Front. Neuroinform. 18:1303380. doi: 10.3389/fninf.2024.1303380
Ji, H., Xu, T., Xue, T., Xu, T., Yan, Z., Liu, Y., et al. (2023). An effective fusion model for seizure prediction: GAMRNN. Front. Neurosci. 17:1246995. doi: 10.3389/fnins.2023.1246995
Jibon, F. A., Jamil Chowdhury, A. R., Miraz, M. H., Jin, H. H., Khandaker, M. U., Sultana, S., et al. (2024). Sequential graph convolutional network and DeepRNN based hybrid framework for epileptic seizure detection from EEG signal. Digit. Health 10:49874. doi: 10.1177/20552076241249874
Kapoor, B., Nagpal, B., Jain, P. K., Abraham, A., and Gabralla, L. A. (2023). Epileptic seizure prediction based on hybrid seek optimization tuned ensemble classifier using EEG signals. Sensors 23:423. doi: 10.3390/s23010423
Karabiber Cura, O., Kocaaslan Atli, S., Türe, H. S., and Akan, A. (2020). Epileptic seizure classifications using empirical mode decomposition and its derivative. Biomed. Eng. Online 19, 1–22. doi: 10.1186/s12938-020-0754-y
Khan, H., Marcuse, L., Fields, M., Swann, K., and Yener, B. (2017). Focal onset seizure prediction using convolutional networks. IEEE Trans. Biomed. Eng. 65, 2109–2118. doi: 10.1109/TBME.2017.2785401
Klatt, J., Feldwisch-Drentrup, H., Ihle, M., Navarro, V., Neufang, M., Teixeira, C., et al. (2012). The EPILEPSIAE database: An extensive electroencephalography database of epilepsy patients. Epilepsia 53, 1669–1676. doi: 10.1111/j.1528-1167.2012.03564.x
Lakehal, M. R., and Ferdi, Y. (2024). Baseline wander and power line interference removal from physiological signals using fractional notch filter optimized through genetic algorithm. Arab. J. Sci. Eng. 1, 1–21. doi: 10.1007/s13369-024-09145-9
Lan, C., Zhao, S., Chen, H., Zhang, L., Yang, Y., Fan, Z., et al. (2024). Single-channel speech enhancement algorithm based on ME-MGCRN in low signal-to-noise scenario. IEEE Access.
Li, H., Dong, X., Zhong, X., Li, C., Cui, H., and Zhou, W. (2024). End-to-end model for automatic seizure detection using supervised contrastive learning. Eng. Appl. Artif. Intell. 133:108665. doi: 10.1016/j.engappai.2024.108665
Li, L., Zhang, H., Liu, X., Li, J., Li, L., Liu, D., et al. (2023). Detection method of absence seizures based on Resnet and bidirectional GRU. Acta Epileptol. 5:7. doi: 10.1186/s42494-022-00117-w
Li, C., Zhao, Y., Song, R., Liu, X., Qian, R., and Chen, X. (2022). Patient-specific seizure prediction from electroencephalogram signal via multichannel feedback capsule network. IEEE Trans. Cogn. Dev. Syst. 15, 1360–1370. doi: 10.1109/tcds.2022.3212019
Liang, W., Pei, H., Cai, Q., and Wang, Y. (2020). Scalp EEG epileptogenic zone recognition and localization based on long-term recurrent convolutional network. Neurocomputing 396, 569–576. doi: 10.1016/j.neucom.2018.10.108
Liu, Y. H., Chen, L., Li, X. W., Wu, Y. C., Liu, S., Wang, J. J., et al. (2022). Epilepsy detection with artificial neural network based on as-fabricated neuromorphic chip platform. AIP Adv. 12:761. doi: 10.1063/5.0075761
Liu, X., Li, C., Lou, X., Kong, H., Li, X., Li, Z., et al. (2024). Epileptic seizure prediction based on EEG using pseudo-three-dimensional CNN. Front. Neuroinform. 18:1354436. doi: 10.3389/fninf.2024.1354436
Liu, G., Tian, L., Wen, Y., Yu, W., and Zhou, W. (2024). Cosine convolutional neural network and its application for seizure detection. Neural Netw. 174:106267. doi: 10.1016/j.neunet.2024.106267
Liu, X., Wang, J., Shang, J., Liu, J., Dai, L., and Yuan, S. (2022). Epileptic seizure detection based on variational mode decomposition and deep forest using EEG signals. Brain Sci. 12:1275. doi: 10.3390/brainsci12101275
Liu, G., Xiao, R., Xu, L., and Cai, J. (2021). Minireview of epilepsy detection techniques based on electroencephalogram signals. Front. Syst. Neurosci. 15:685387. doi: 10.3389/fnsys.2021.685387
Lopes, F., Leal, A., Medeiros, J., Pinto, M. F., Dourado, A., Dümpelmann, M., et al. (2021). Automatic electroencephalogram artifact removal using deep convolutional neural networks. IEEE Access 9, 149955–149970. doi: 10.1109/ACCESS.2021.3125728
Ma, Y., Huang, Z., Su, J., Shi, H., Wang, D., Jia, S., et al. (2023). A multi-channel feature fusion CNN-BI-LSTM epilepsy EEG classification and prediction model based on attention mechanism. IEEE Access 11, 62855–62864. doi: 10.1109/ACCESS.2023.3287927
Maillard, L., and Ramantani, G. (2017). New recommendations of the IFCN: from scalp EEG to electrical brain imaging. Clin. Neurophysiol. 128, 2068–2069. doi: 10.1016/j.clinph.2017.07.413
Maiwald, T., Winterhalder, M., Aschenbrenner-Scheibe, R., Voss, H. U., Schulze-Bonhage, A., and Timmer, J. (2004). Comparison of three nonlinear seizure prediction methods by means of the seizure prediction characteristic. Phys D 194, 357–368. doi: 10.1016/j.physd.2004.02.013
Mallick, S., and Baths, V. (2024). Novel deep learning framework for detection of epileptic seizures using EEG signals. Front. Comput. Neurosci. 18:1340251. doi: 10.3389/fncom.2024.1340251
Martini, M. L., Valliani, A. A., Sun, C., Costa, A. B., Zhao, S., Panov, F., et al. (2021). Deep anomaly detection of seizures with paired stereoelectroencephalography and video recordings. Sci. Re 11:7482. doi: 10.1038/s41598-021-86891-y
Moctezuma, L. A., and Molinas, M. (2020). Classification of low-density EEG for epileptic seizures by energy and fractal features based on EMD. J. Biomed. Res. 34:180. doi: 10.7555/JBR.33.20190009
Nasiri, S., and Clifford, G. D. (2021). Generalizable seizure detection model using generating transferable adversarial features. IEEE Signal Process. Lett. 28, 568–572. doi: 10.1109/LS2021.3060967
Ngiam, J., Khosla, A., Kim, M., Nam, J., Lee, H., and Ng, A. Y. (2011). Multimodal deep learning. In: Proceedings of the 28th international conference on machine learning (ICML-11), pp. 689–696.
Osorio, I., Frei, M. G., and Wilkinson, S. B. (1998). Real-time automated detection and quantitative analysis of seizures and short-term prediction of clinical onset. Epilepsia 39, 615–627. doi: 10.1111/j.1528-1157.1998.tb01430.x
Ozcan, A. R., and Erturk, S. (2019). Seizure prediction in scalp EEG using 3D convolutional neural networks with an image-based approach. IEEE Trans. Neural Syst. Rehabil. Eng. 27, 2284–2293. doi: 10.1109/TNSRE.2019.2943707
Palanisamy, K. K., and Rengaraj, A. (2024). Early detection of stress and anxiety based seizures in position data augmented EEG signal using hybrid deep learning algorithms. IEEE Access 12, 35351–35365. doi: 10.1109/ACCESS.2024.3365192
Pan, S. J., and Yang, Q. (2009). A survey on transfer learning. IEEE Trans. Knowl. Data Eng. 22, 1345–1359. doi: 10.1109/TKDE.2009.191
Pan, Y., Zhou, X., Dong, F., Wu, J., Xu, Y., and Zheng, S. (2022). Epileptic seizure detection with hybrid time-frequency EEG input: a deep learning approach. Comput. Math. Methods Med. 2022, 1–14. doi: 10.1155/2022/8724536
Parija, S., Dash, P. K., and Bisoi, R. (2020). Multi-kernel-based random vector functional link network with decomposed features for epileptic EEG signal classification. IET Signal Process. 14, 162–174. doi: 10.1049/iet-spr.2019.0277
Pelkonen, A., Mzezewa, R., Sukki, L., Ryynänen, T., Kreutzer, J., Hyvärinen, T., et al. (2020). A modular brain-on-a-chip for modelling epileptic seizures with functionally connected human neuronal networks. Biosens. Bioelectron. 168:112553. doi: 10.1016/j.bios.2020.112553
Peng, J., Xue-Jun, Z., and Zhi-Xin, S. (2021). eEpileptic electroencephalogram signal classification method based on elastic variational mode decomposition. Acta Phys. Sin. 70:018702. doi: 10.7498/aps.70.20200904
Perucca, E. (2021). The pharmacological treatment of epilepsy: recent advances and future perspectives. Acta Epileptol. 3:22. doi: 10.1186/s42494-021-00055-z
Pham, T. D. (2021). Time–frequency time–space LSTM for robust classification of physiological signals. Sci. Re 11:6936. doi: 10.1038/s41598-021-86432-7
Prathaban, B. P., and Balasubramanian, R. (2021). Dynamic learning framework for epileptic seizure prediction using sparsity based EEG reconstruction with optimized CNN classifier. Expert Syst. Appl. 170:114533. doi: 10.1016/j.eswa.2020.114533
Puka, K., Widjaja, E., and Smith, M. L. (2017). The influence of patient, caregiver, and family factors on symptoms of anxiety and depression in children and adolescents with intractable epilepsy. Epilepsy Behav. 67, 45–50. doi: 10.1016/j.yebeh.2016.12.011
Qin, Y. F., Fu, X., Li, X. K., and Li, H. J. (2024). ADAMS simulation and HHT feature extraction method for bearing faults of coal shearer. PRO 12:164. doi: 10.3390/pr12010164
Qiu, Y., Zhou, W., Yu, N., and Du, P. (2018). Denoising sparse autoencoder-based ictal EEG classification. IEEE Trans. Neural Syst. Rehabil. Eng. 26, 1717–1726. doi: 10.1109/TNSRE.2018.2864306
Ra, J. S., and Li, T. (2023). A novel epileptic seizure prediction method based on synchroextracting transform and 1-dimensional convolutional neural network. Comput. Methods Prog. Biomed. 240:107678. doi: 10.1016/j.cmpb.2023.107678
Raghu, S., Sriraam, N., Vasudeva Rao, S., Hegde, A. S., and Kubben, P. L. (2020). Automated detection of epileptic seizures using successive decomposition index and support vector machine classifier in long-term EEG. Neural Comput. & Applic. 32, 8965–8984. doi: 10.1007/s00521-019-04389-1
Rasheed, K., Qadir, J., O’Brien, T. J., Kuhlmann, L., and Razi, A. (2021). A generative model to synthesize EEG data for epileptic seizure prediction. IEEE Trans. Neural Syst. Rehabil. Eng. 29, 2322–2332. doi: 10.1109/TNSRE.2021.3125023
Rukhsar, S., and Tiwari, A. K. (2023). Lightweight convolution transformer for cross-patient seizure detection in multi-channel EEG signals. Comput. Methods Prog. Biomed. 242:107856. doi: 10.1016/j.cmpb.2023.107856
Sabour, S., Frosst, N., and Hinton, G. E. (2017). Dynamic routing between capsules. Adv. Neural Inf. Proces. Syst. 30:11. doi: 10.48550/arXiv.1710.09829
Sardouie, S. H., Shamsollahi, M. B., Albera, L., and Merlet, I. (2014). Denoising of ictal EEG data using semi-blind source separation methods based on time-frequency priors. IEEE J. Biomed. Health Inform. 19, 839–847. doi: 10.1109/JBHI.2014.2336797
Scheffer, I. E., Berkovic, S., Capovilla, G., Connolly, M. B., French, J., Guilhoto, L., et al. (2017). ILAE classification of the epilepsies: position paper of the ILAE Commission for Classification and Terminology. Epilepsia 58, 512–521. doi: 10.1111/epi.13709
Sengupta, A., Ye, Y., Wang, R., Liu, C., and Roy, K. (2019). Going deeper in spiking neural networks: VGG and residual architectures. Front. Neurosci. 13:95. doi: 10.3389/fnins.2019.00095
Shafiezadeh, S., Duma, G. M., Mento, G., Danieli, A., Antoniazzi, L., Del Popolo Cristaldi, F., et al. (2024). Calibrating deep learning classifiers for patient-independent electroencephalogram seizure forecasting. Sensors 24:2863. doi: 10.3390/s24092863
Sharma, R. K. (2017). EEG signal denoising based on wavelet transform. In: 2017 international conference of electronics, communication and aerospace technology (ICECA), 1, pp. 758–761.
Shi, S., and Liu, W. (2023). B2-ViT net: broad vision transformer network with broad attention for seizure prediction. IEEE Trans. Neural Syst. Rehabil. Eng. 32, 178–188. doi: 10.1109/TNSRE.2023.3245654
Shoeb, A. H. (2009). Application of machine learning to epileptic seizure onset detection and treatment Doctoral dissertation, Massachusetts Institute of Technology.
Singh, K., and Malhotra, J. (2022). Two-layer LSTM network-based prediction of epileptic seizures using EEG spectral features. Complex Intell. Syst. 8, 2405–2418. doi: 10.1007/s40747-021-00627-z
Sirpal, P., Kassab, A., Pouliot, P., Nguyen, D. K., and Lesage, F. (2019). fNIRS improves seizure detection in multimodal EEG-fNIRS recordings. J. Biomed. Opt. 24, –051408. doi: 10.1117/1.JBO.24.5.051408
Song, T., Wang, J., Huo, J., Wei, W., Han, R., Xu, D., et al. (2023). Prediction of significant wave height based on EEMD and deep learning. Front. Mar. Sci. 10:1089357. doi: 10.3389/fmars.2023.1089357
Stevenson, N. J. (2019). Data from: a dataset of neonatal EEG recordings with seizure annotations. Sci. Data 6:190039. doi: 10.1038/sdata.2019.39
Subasi, A., Jukic, S., and Kevric, J. (2019). Comparison of EMD, DWT and WPD for the localization of epileptogenic foci using random forest classifier. Measurement 146, 846–855. doi: 10.1016/j.measurement.2019.07.026
Sun, Y., and Chen, X. (2022). Automatic detection of epilepsy based on entropy feature fusion and convolutional neural network. Oxidative Med. Cell. Longev. 2022:1322826. doi: 10.1155/2024/9847185
Sun, B., Lv, J. J., Rui, L. G., Yang, Y. X., Chen, Y. G., Ma, C., et al. (2021). Seizure prediction in scalp EEG based channel attention dual-input convolutional neural network. Phys. A Stat. Mech. Appl. 584:126376. doi: 10.1016/j.physa.2021.126376
Sunaryono, D., Sarno, R., Siswantoro, J., Purwitasari, D., Sabilla, S. I., Susilo, R. I., et al. (2022). Hybrid one-dimensional CNN and DNN model for classification epileptic seizure. Int. J. Intell. Eng. Syst. 16, 492–502. doi: 10.22266/ijies2022.1231.44
Swami, S., Panigrahi, B., Nara, S., and Bhatia, M. (2016). Gandhi T. Data from: EEG Epilepsy Datasets. Available at: https://www.researchgate.net/publication/308719109_EEG_Epilepsy_Datasets.
Takahashi, H., Emami, A., Shinozaki, T., Kunii, N., Matsuo, T., and Kawai, K. (2020). Convolutional neural network with autoencoder-assisted multiclass labelling for seizure detection based on scalp electroencephalography. Comput. Biol. Med. 125:104016. doi: 10.1016/j.compbiomed.2020.104016
The SWEC-ETHZ iEEG Database and Algorithms Overview . (2024). Available at: http://ieeg-swez.ethz.ch/ (Accessed August 16, 2024).
Toraman, S. (2020). Preictal and Interictal recognition for epileptic seizure prediction using pre-trained 2DCNN models. Traitement Signal 37:617. doi: 10.18280/ts.370617
Toraman, S. (2021). Automatic recognition of preictal and interictal EEG signals using 1D-capsule networks. Comput. Electr. Eng. 91:107033. doi: 10.1016/j.compeleceng.2021.107033
Truong, N. D., Kuhlmann, L., Bonyadi, M. R., Querlioz, D., Zhou, L., and Kavehei, O. (2019). Epileptic seizure forecasting with generative adversarial networks. IEEE Access 7, 143999–144009. doi: 10.1109/ACCESS.2019.2944691
Truong, N. D., Nguyen, A. D., Kuhlmann, L., Bonyadi, M. R., Yang, J., Ippolito, S., et al. (2018). Convolutional neural networks for seizure prediction using intracranial and scalp electroencephalogram. Neural Netw. 105, 104–111. doi: 10.1016/j.neunet.2018.04.018
Truong, N. D., Nguyen, A. D., Kuhlmann, L., Bonyadi, M. R., Yang, J., and Kavehei, O. (2017). A generalised seizure prediction with convolutional neural networks for intracranial and scalp electroencephalogram data analysis. arxiv 2017:01976. doi: 10.48550/arXiv.1707.01976
Tsiouris, Κ. Μ., Pezoulas, V. C., Zervakis, M., Konitsiotis, S., Koutsouris, D. D., and Fotiadis, D. I. (2018). A long short-term memory deep learning network for the prediction of epileptic seizures using EEG signals. Comput. Biol. Med. 99, 24–37. doi: 10.1016/j.compbiomed.2018.05.019
Tuncer, E., and Bolat, E. D. (2022). Classification of epileptic seizures from electroencephalogram (EEG) data using bidirectional short-term memory (bi-LSTM) network architecture. Biomed. Signal Process. Control 73:103462. doi: 10.1016/j.bspc.2021.103462
Uddin, Z., Altaf, M., Ahmad, A., Qamar, A., and Orakzai, F. A. (2023). Isolation of multiple electrocardiogram artifacts using independent vector analysis. PeerJ Comput. Sci. 9:e1189. doi: 10.7717/peerj-cs.1189
Ullah, I., Hussain, M., and Aboalsamh, H. (2018). An automated system for epilepsy detection using EEG brain signals based on deep learning approach. Expert Syst. Appl. 107, 61–71. doi: 10.1016/j.eswa.2018.04.021
Usman, S. M., Khalid, S., and Aslam, M. H. (2020). Epileptic seizures prediction using deep learning techniques. IEEE Access 8, 39998–40007. doi: 10.1109/ACCESS.2020.2976866
Wan, S., Dong, F., Zhang, X., Wu, W., and Li, J. (2023). Fault voiceprint signal diagnosis method of power transformer based on Mixup data enhancement. Sensors 23:3341. doi: 10.3390/s23063341
Wang, D., Lian, J., Cheng, H., and Zhou, Y. (2024). Music-evoked emotions classification using vision transformer in EEG signals. Front. Psychol. 15:1275142. doi: 10.3389/fpsyg.2024.1275142
Wang, Z., Song, X., Chen, L., Nan, J., Sun, Y., Pang, M., et al. (2024). Research progress of epileptic seizure prediction methods based on EEG. Cogn. Neurodyn. 1, 1–20.
Wang, X., Wang, X., Liu, W., Chang, Z., Kärkkäinen, T., and Cong, F. (2021). One dimensional convolutional neural networks for seizure onset detection using long-term scalp and intracranial EEG. Neurocomputing 459, 212–222. doi: 10.1016/j.neucom.2021.06.048
Wang, B., Xu, Y., Peng, S., Wang, H., and Li, F. (2024). Detection method of epileptic seizures using a neural network model based on multimodal dual-stream networks. Sensors 24:3360. doi: 10.3390/s24113360
Wang, C., Yi, H., Wang, W., and Valliappan, P. (2019). Lesion localization algorithm of high-frequency epileptic signal based on Teager energy operator. Biomed. Signal Process. Control 47, 262–275. doi: 10.1016/j.bspc.2018.08.033
Watanabe, R. G. S., Thais, M. E. R., Marmentini, E. L., Freitas, T. G., Wolf, P., and Lin, K. (2024). Theory of mind in epilepsy. Epilepsy Behav. 158:109910. doi: 10.1016/j.yebeh.2024.109910
Wei, L., Boutouil, H., Gerbatin, R. R., Mamad, O., Heiland, M., Reschke, C. R., et al. (2021). Detection of spontaneous seizures in EEGs in multiple experimental mouse models of epilepsy. J. Neural Eng. 18:056060. doi: 10.1088/1741-2552/ac2ca0
Wei, X., Zhou, L., Chen, Z., Zhang, L., and Zhou, Y. (2018). Automatic seizure detection using three-dimensional CNN based on multi-channel EEG. BMC Med. Inform. Decis. Mak. 18, 71–80. doi: 10.1186/s12911-018-0693-8
Wei, X., Zhou, L., Zhang, Z., Chen, Z., and Zhou, Y. (2019). Early prediction of epileptic seizures using a long-term recurrent convolutional network. J. Neurosci. Methods 327:108395. doi: 10.1016/j.jneumeth.2019.108395
Wei, Z., Zou, J., Zhang, J., and Xu, J. (2019). Automatic epileptic EEG detection using convolutional neural network with improvements in time-domain. Biomed. Signal Process. Control 53:101551. doi: 10.1016/j.bspc.2019.04.028
Weiss, K., Khoshgoftaar, T. M., and Wang, D. (2016). A survey of transfer learning. J. Big Data 3, 1–40. doi: 10.1186/s40537-016-0043-6
Wen, T., and Zhang, Z. (2018). Deep convolution neural network and autoencoders-based unsupervised feature learning of EEG signals. IEEE Access 6, 25399–25410. doi: 10.1109/ACCESS.2018.2833746
World Health Organization . (2024). Epilepsy. Available at: https://www.who.int/news-room/fact-sheets/detail/epilepsy (Accessed August 12, 2024)
Xin, Q., Hu, S., Liu, S., Zhao, L., and Zhang, Y. D. (2022). An attention-based wavelet convolution neural network for epilepsy EEG classification. IEEE Trans. Neural Syst. Rehabil. Eng. 30, 957–966. doi: 10.1109/TNSRE.2022.3166181
Xu, Y., Yang, J., Ming, W., Wang, S., and Sawan, M. (2024). Shorter latency of real-time epileptic seizure detection via probabilistic prediction. Expert Syst. Appl. 236:121359. doi: 10.1016/j.eswa.2023.121359
Xu, X. F., Zhuang, X. N., Xue, C., Chen, Z. Y., Wu, Y. C., and Guo, G. P. (2024). An efficient quantum algorithm for independent component analysis. New J. Phys. 26:073030. doi: 10.1088/1367-2630/ad5e16
Yan, J., Li, J., Xu, H., Yu, Y., and Xu, T. (2022). Seizure prediction based on transformer using scalp electroencephalogram. Appl. Sci. 12:4158. doi: 10.3390/app12094158
Yan, Y., Wu, X., Li, C., He, Y., Zhang, Z., Li, H., et al. (2022). Topological EEG nonlinear dynamics analysis for emotion recognition. IEEE Trans. Cogn. Dev. Syst. 15, 625–638. doi: 10.1109/tcds.2022.3174209
Yang, Y., Luan, T., Yu, Z., Zhang, M., Li, F., Chen, X., et al. (2024). Technological vanguard: the outstanding performance of the LTY-CNN model for the early prediction of epileptic seizures. J. Transl. Med. 22:162. doi: 10.1186/s12967-024-04945-x
Yang, Y., Sarkis, R. A., El Atrache, R., Loddenkemper, T., and Meisel, C. (2021). Video-based detection of generalized tonic-clonic seizures using deep learning. IEEE J. Biomed. Health Inform. 25, 2997–3008. doi: 10.1109/JBHI.2021.3049649
Yang, W., Xue, L., Chen, J., Wang, Y., Ding, S., and Zhang, H. (2024). Classification of recurrent depression using brain CT images through feature fusion. J. Radiat. Res. Appl. Sci. 17:100986. doi: 10.1016/j.jrras.2024.100986
Yedurkar, D. P., and Metkar, S. P. (2020). Multiresolution approach for artifacts removal and localization of seizure onset zone in epileptic EEG signal. Biomed. Signal Process. Control 57:101794. doi: 10.1016/j.bspc.2019.101794
Yuan, Y., Xun, G., Jia, K., and Zhang, A. (2018). A multi-view deep learning framework for EEG seizure detection. IEEE J. Biomed. Health Inform. 23, 83–94. doi: 10.1109/JBHI.2018.2871678
Zhang, S., Chen, D., Ranjan, R., Ke, H., Tang, Y., and Zomaya, A. Y. (2021). A lightweight solution to epileptic seizure prediction based on EEG synchronization measurement. J. Supercomput. 77, 3914–3932. doi: 10.1007/s11227-020-03426-4
Zhang, Z., Lin, B. S., Peng, C. W., and Lin, B. S. (2024a). Multi-modal sleep stage classification with two-stream encoder-decoder. IEEE Trans. Neural Syst. Rehabil. Eng. 32, 2096–2105. doi: 10.1109/TNSRE.2024.3394738
Zhang, Z., and Parhi, K. K. (2016). Low-complexity seizure prediction from iEEG/sEEG using spectral power and ratios of spectral power. IEEE Trans. Biomed. Circuits Syst. 10, 693–706. doi: 10.1109/TBCAS.2015.2477264
Zhang, Z., Xiao, M., Ji, T., Jiang, Y., Lin, T., Zhou, X., et al. (2024b). Efficient and generalizable cross-patient epileptic seizure detection through a spiking neural network. Front. Neurosci. 17:1303564. doi: 10.3389/fnins.2023.1303564
Zhang, Y., Yang, S., Liu, Y., Zhang, Y., Han, B., and Zhou, F. (2018). Integration of 24 feature types to accurately detect and predict seizures using scalp EEG signals. Sensors 18:1372. doi: 10.3390/s18051372
Zhang, Y., Yao, S., Yang, R., Liu, X., Qiu, W., Han, L., et al. (2022). Epileptic seizure detection based on bidirectional gated recurrent unit network. IEEE Trans. Neural Syst. Rehabil. Eng. 30, 135–145. doi: 10.1109/TNSRE.2022.3143540
Zhang, J., Zheng, S., Chen, W., Du, G., Fu, Q., and Jiang, H. (2024). A scheme combining feature fusion and hybrid deep learning models for epileptic seizure detection and prediction. Sci. Re 14:16916. doi: 10.1038/s41598-024-67855-4
Zhao, Y., Li, C., Liu, X., Qian, R., Song, R., and Chen, X. (2022). Patient-specific seizure prediction via adder network and supervised contrastive learning. IEEE Trans. Neural Syst. Rehabil. Eng. 30, 1536–1547. doi: 10.1109/TNSRE.2022.3180155
Zhao, W., Wang, W. F., Patnaik, L. M., Zhang, B. C., Weng, S. J., Xiao, S. X., et al. (2024). Residual and bidirectional LSTM for epileptic seizure detection. Front. Comput. Neurosci. 18:1415967. doi: 10.3389/fncom.2024.1415967
Zhou, Y., You, J., Zhu, F., Bragin, A., Engel, J., and Li, L. (2021). Automatic electrophysiological noise reduction and epileptic seizure detection for Stereoelectroencephalography. In: 2021 43rd annual international conference of the IEEE engineering in Medicine and Biology Society (EMBC), pp. 107–112.
Zhou, W., Zheng, W., Feng, Y., and Li, X. (2024). LMA-EEGNet: a lightweight multi-attention network for neonatal seizure detection using EEG signals. Electronics 13:2354. doi: 10.3390/electronics13122354
Keywords: preprocessing, feature extraction, epilepsy detection, epilepsy prediction, deep learning
Citation: Zhang X, Zhang X, Huang Q and Chen F (2024) A review of epilepsy detection and prediction methods based on EEG signal processing and deep learning. Front. Neurosci. 18:1468967. doi: 10.3389/fnins.2024.1468967
Edited by:
Zhongbo Sun, Changchun University of Technology, ChinaReviewed by:
Mingjie Dong, Beijing University of Technology, ChinaJiliang Zhang, The University of Sheffield, United Kingdom
Copyright © 2024 Zhang, Zhang, Huang and Chen. This is an open-access article distributed under the terms of the Creative Commons Attribution License (CC BY). The use, distribution or reproduction in other forums is permitted, provided the original author(s) and the copyright owner(s) are credited and that the original publication in this journal is cited, in accordance with accepted academic practice. No use, distribution or reproduction is permitted which does not comply with these terms.
*Correspondence: Fuming Chen, Y2ZtNTc2MkAxMjYuY29t
†These authors have contributed equally to this work