- 1Tri-Institutional Center for Translational Research in Neuroimaging and Data Science (TReNDS) Georgia State University, Georgia Institute of Technology, Emory University, Atlanta, GA, United States
- 2Department of Computer Science, Georgia State University, Atlanta, GA, United States
- 3Neuroscience Institute, Georgia State University, Atlanta, GA, United States
Human adolescence marks a crucial phase of extensive brain development, highly susceptible to environmental influences. Employing brain age estimation to assess individual brain aging, we categorized individuals (N = 7,435, aged 9–10 years old) from the Adolescent Brain and Cognitive Development (ABCD) cohort into groups exhibiting either accelerated or delayed brain maturation, where the accelerated group also displayed increased cognitive performance compared to their delayed counterparts. A 4-way multi-set canonical correlation analysis integrating three modalities of brain metrics (gray matter density, brain morphological measures, and functional network connectivity) with nine environmental factors unveiled a significant 4-way canonical correlation between linked patterns of neural features, air pollution, area crime, and population density. Correlations among the three brain modalities were notably strong (ranging from 0.65 to 0.77), linking reduced gray matter density in the middle temporal gyrus and precuneus to decreased volumes in the left medial orbitofrontal cortex paired with increased cortical thickness in the right supramarginal and bilateral occipital regions, as well as increased functional connectivity in occipital sub-regions. These specific brain characteristics were significantly more pronounced in the accelerated brain aging group compared to the delayed group. Additionally, these brain regions exhibited significant associations with air pollution, area crime, and population density, where lower air pollution and higher area crime and population density were correlated to brain variations more prominently in the accelerated brain aging group.
1 Introduction
During adolescence, the brain experiences rapid development, second only to infancy (Arain et al., 2013). Studies of brain structure using MRI have shown that gray matter exhibits a pre-adolescence increase, followed by a steady decrease into adulthood (Blakemore and Choudhury, 2006). In contrast, white matter density increases roughly linearly until young adulthood (Paus et al., 1999). This general pattern varies across brain regions in terms of rate and time (Giedd et al., 1999; Sowell et al., 2001; Gogtay et al., 2004), and is accompanied by synaptic pruning (Huttenlocher and Dabholkar, 1997), and prolonged increases in myelination (Miller et al., 2012). Functional brain imaging studies also demonstrated that brain responses to stimuli such as sensory inputs, affection, rewards, or demands, change during adolescence (Casey et al., 2005; Laruelle et al., 2002; Kwon et al., 2002; Rubia et al., 2000). Such multidimensional changes in the brain also provide the biological foundation for the maturation of adolescent cognitive ability, which is why cognitive performance is often thought to reflect brain maturation (Dosenbach et al., 2010).
This period of rapid brain maturation has been shown to also be a period of vulnerability to environmental factors (Green et al., 2010). The complex relationship between the brain, behavior, and environmental factors has been well-established (Modabbernia et al., 2021; Xu et al., 2023). Much of the latest research has used the Adolescent Brain and Cognitive Development Study (ABCD) to investigate the effects of environment on brain development. Consistently, researchers using this large adolescent cohort (N = 11 k), have found that environmental measures such as neighborhood disadvantage, school environment, and socioeconomic disadvantage are associated with increased functional connectivity (Rakesh et al., 2021, 2023) and reductions in global cortical thickness (Rakesh et al., 2022; Hackman et al., 2021; Taylor et al., 2020). A longitudinal study of the same cohort, looking at these relationships at baseline and year two, found similar associations between changes in brain connectivity and negative environmental factors, suggesting that accelerated maturation of the brain may be an adaptive response to adversity (Brieant et al., 2021).
Beyond the ABCD cohort, other studies have established the relationship between multiple environmental factors, on a variety of scales, with brain maturation, health, and cognitive development. The factors include air pollution (Cipriani et al., 2018), urbanization (Lederbogen et al., 2013; Sampson et al., 2020), negative and unstable family relationships (Bush et al., 2020), and stressful life events (Gapp et al., 2014; Herzberg and Gunnar, 2020). Specifically, on a macro scale, higher air pollution has been associated with a thinner cortex in the precuneus and rostral middle frontal regions, with partially mediating effects on impaired inhibitory control (Guxens et al., 2018), as well as lower functional integration and segregation in key brain networks in school-age children (Pujol et al., 2016). Population density, closely related to urbanicity, has been consistently associated with affective symptoms, including elevated depression (Sampson et al., 2020). Urbanicity is positively correlated with cerebellar volume and negatively correlated with medial prefrontal cortex volume in young people (Xu et al., 2022). Recent research shows that people living in areas with higher ratios of green space exhibit stronger parietal and insular activation during stress, whereas exposure to more air pollution leads to weaker activation in the same brain areas (Dimitrov-Discher et al., 2022). Meso-scale environmental factors like deprived neighborhoods, often characterized by area deprivation, area crime rates, or limited access to school education, are known to have negative effects on young people. These include increased chronic stress (Jorgensen et al., 2023), increased risk of childhood mental health problems, (Baranyi et al., 2021; Sui et al., 2022; Alderton et al., 2019), anxiety, depression (Barnett et al., 2018; Thapaliya et al., 2024), and increased suicidal tendencies (Cairns et al., 2017). Moreover, previous studies on micro-scale environmental factors have established strong links, such as smaller gray matter volume in focal regions resulting from socioeconomic status deprivation (Jeong et al., 2023) and neighborhood disadvantage being associated with lower cortical thickness in brain regions like the cuneus and lateral occipital cortex (Rakesh et al., 2022). A good school environment was also associated with decreased connectivity between the cingulo-opercular network and the default mode network (Rakesh et al., 2023). At the same time, various types of early life adversity were linked to noticeable effects on the brain, including extended activation of prefrontal–hippocampal–amygdala circuits (Smith and Pollak, 2020; Herzberg and Gunnar, 2020).
Despite the depth of our understanding regarding these relationships between environmental factors and specific brain regional alterations, there has been very little research on how they might be related to brain maturation in a holistic way. To estimate the relative brain maturation, neuronal features derived from MRI have been used to create an objective, biological measure, referred to as brain age estimation (Franke and Gaser, 2019; Franke et al., 2010). The gap between estimated brain age and chronological age, referred to as delta age, is commonly used to indicate an individual's brain aging process. Both accelerated and delayed brain aging have been associated with various symptoms of mental illness as well as health conditions (Baecker et al., 2021; Ramduny et al., 2022; Dunlop et al., 2021; Casanova et al., 2022; Phillips et al., 2023). Hereafter, we will refer to the positive/negative gaps between estimated brain age and chronological age as accelerated/delayed brain aging (Peng et al., 2021), indicating the individual differences in brain aging/maturation when compared to the estimated norm of the same age subjects. The validity of brain age estimation has been verified in various cohorts (Baecker et al., 2021) where the brain age gap has been shown to be a reliable biomarker for abnormal brain development, resilient aging process, or risk of mental illness (Franke and Gaser, 2019; Kaufmann et al., 2017; Konrad et al., 2013). In particular, children and adolescent studies, including ours (Ray et al., 2021), have demonstrated that estimated brain age not only reflects age-related changes but is also indicative of cognitive maturation (Ray et al., 2021; Basodi et al., 2021, 2022; Liem et al., 2017; Dosenbach et al., 2010). Accelerated brain age (brain age older than chronological age) is significantly associated with faster information processing speeds and higher verbal comprehension compared to the delayed brain age group (Ray et al., 2023).
Brain age estimation models are effective at selecting brain features that contribute to the accurate estimation of brain age, but they cannot provide coherent information regarding whole brain development patterns. For instance, interrelated bilateral gray matter density in the frontal cortex may both show similar growth patterns, but only one might be selected for the estimation of brain age (Ray et al., 2021). In our previous work, we built a reliable brain age estimation model that identified adolescents in the ABCD Study who were experiencing accelerated or delayed brain maturation (Ray et al., 2023). To fully understand the whole brain patterns related to accelerated vs. delayed brain maturation, as well as investigate the influence of environmental factors, in this study we have applied a multi-set canonical correlation analysis (mCCA) (Li et al., 2009; Zhuang et al., 2020) to overcome the limitations associated with brain age estimation models. Our study is focused on: (i) identifying whole-brain patterns linked to accelerated or delayed brain aging, (ii) exploring the impact of various environmental factors on these brain patterns, (iii) and providing an understanding of how environmental factors may contribute to brain maturation. We seek to reveal, at least partially, the complex interplay between the brain and multi-scale environmental factors, providing further understanding of how brain maturation may be influenced by environments.
2 Data and method
2.1 Participants
We analyzed the data collected from the ABCD study https://abcdstudy.org (Hagler et al., 2019), which is a 10-year-long study on participants initially recruited at the age of nine to ten from 21 sites across the United States. Along with multisession structural and functional brain MRI scans, the ABCD study also includes key demographic information, including gender, race, socio-economic background, cognitive development, and a mental and physical health assessment of the subjects. Written informed consent from the parents and assent from the child were obtained for each participant, with approval from the Institutional Review Board (IRB). The ABCD dataset is provided by the National Institute of Mental Health Data Archive (NDA) https://nda.nih.gov/. The NDA shares the ABCD data as an open-source dataset, collected from a wide range of research projects across various scientific domains, to enable collaborative science and discovery. In this study, we used data from the ABCD baseline, which contains 11,875 participants, to select a subpopulation with accelerated or delayed brain age.
In our prior study, we developed a refined brain age model to robustly and accurately estimate the brain age of ABCD participants within a narrow age range (9–10 years) (Ray et al., 2024, 2023). The refined model was constructed with two modules. The first module is the brain age model pre-trained with 1,417 subjects aged 8–22 years from the Philadelphia Neurodevelopmental Cohort (PNC) (Satterthwaite et al., 2016), which leveraged the wider age range of PNC data to ensure broader prediction power. After pre-training the first module, the second module was trained by utilizing the ABCD baseline data with a narrow age range (9–10 years) to account for unexplained residual variation (the difference between the prediction of the first module and actual age). The refined brain age estimation was finally obtained by subtracting the estimated residuals from the broader predicted age, thereby improving model accuracy. The final refined model showed the best performance on both ABCD baseline and year-two data with a mean absolute error of 0.49 and 0.48 years, respectively. Furthermore, the brain age gap yielded by the refined model demonstrated significant associations with participants' information processing speed and verbal comprehension ability on the baseline data. In this study, we have applied the model to ABCD full baseline data to estimate participants' brain age. The brain age gaps (estimated brain age—chronological age) were computed (see Supplementary Figure 1) and were found to roughly follow a normal distribution. We identified 7,435 participants whose brain age gap was 0.41 standard deviation away from the mean after z transformation, partitioned into accelerated brain age group (z>0.41:N = 3, 755) and delayed brain age group (z < −0.41:N = 3, 680). The threshold of 0.41 was chosen to include roughly the bottom and top third of the cohort. In our analysis, we specifically targeted these two subgroups to identify brain patterns with significant differences between the two groups and linked to environmental factors. We did not use all participants to emphasize the factors contributing most to the variations between accelerated and delayed brain age. In the data quality control steps, we removed samples following the inclusion recommendation of ABCD release version 5 for T1 weighted sMRI and rs-fMRI and removed samples with missing values in the environmental factors. Finally, we have 4,264 samples (Accelerated group: N = 2,149, Delayed group: N = 2,115) for our analysis. The two groups listed in Table 1 had no significant differences in age, gender, and race.
2.2 sMRI data preprocessing and feature generation
We extracted three types of brain features: (i) gray matter density of 100 independent components derived from independent component analysis (ICA) (Xu et al., 2009) of gray matter images, (ii) 152 brain morphological features derived from FreeSurfer version v5.3 (Khan et al., 2008), and (iii) 1,378 static functional network connectivity (sFNC) values (Saha et al., 2022) derived from resting state functional MRI.
Gray matter (GM) density maps were generated by preprocessing the T1-weighted sMRI images using the Statistical Parametric Mapping 12 (SPM12) (Ashburner et al., 2014) software toolbox. Six types of tissue maps (gray matter, white matter, CSF, bone, soft tissue, and others) were created in Montreal Neuroimaging Institute (MNI) space after jointly segmenting and spatially normalizing the T1-weighted sMRI images using SPM12 default tissue probability maps. The gray matter maps were then smoothed using a 6 mm3 Gaussian kernel. Finally, we applied quality control on the individual gray matter maps and selected those correlated to the group mean gray matter map at correlation ≥0.9. After quality control, the gray matter maps were then masked to only include voxels with a gray matter density value >0.2. We then applied independent component analysis (ICA) (Bell and Sejnowski, 1995; Amari, 1998) to extract 100 brain components. ICA decomposes the gray matter data into a linear combination of maximally independent components, called source-based morphometry (SBM) (Xu et al., 2009). Each component as a brain network identifies a network of voxels with covarying gray matter patterns and often these components resemble those in resting fMRI data (Luo et al., 2020). The ICA loadings reflect how these brain networks are expressed across subjects, which are used as one type of brain feature in this study, referred to as gray matter density of 100 independent components. In addition, the brain morphological measures derived by FreeSurfer version v5.3 (Khan et al., 2008) are provided by the ABCD study (data release version 5). We selected 152 measures as the second type of brain features, which included the estimated total intracranial volume, cortical thickness and cortical volume, and subcortical volume of the human brain based on the Desikan atlas (Desikan et al., 2006).
2.3 fMRI data preprocessing and functional network connectivity (sFNC) features
We conducted preprocessing on the raw resting-state fMRI data using a combination of the FMRIB Software Library (FSL) v6.0 (Jenkinson et al., 2012; Smith et al., 2004; Woolrich et al., 2009) toolbox and the Statistical Parametric Mapping (SPM) 12 toolbox within the MATLAB 2020b environment. The preprocessing steps involved: (i) correcting for rigid body motion; (ii) addressing distortion; (iii) eliminating dummy scans; (iv) normalizing the data to standard Montreal Neurological Institute space; and (v) applying smoothing with a 6 mm Gaussian kernel. We conducted data quality control on the preprocessed fMRI data using the Neuromark framework (Fu et al., 2023). The FSL MCFLIRT tool was employed to rectify any rigid body motion observed in the subject's head during the fMRI scanning. After correcting head motion, distortion correction in the fMRI images was performed using field map files. These files were obtained by acquiring phase encoding in both the anterior-posterior (AP) and posterior-anterior (PA) directions with the FSL tool topup. The distortion present in the fMRI volume was then addressed by applying the output field map coefficients using the FSL tool applytopup. After distortion correction, 10 initial scans with significant signal changes were discarded to help the tissue gain a steady state of radiofrequency excitation. Then wrapping and smoothening of the fMRI data were done with MNI space 3 × 3 × 3 mm3 spatial resolution and a Gaussian kernel with a full width at half maximum (FWHM) of 6 mm. We employed the Neuromark_fMRI_1.0 (Du et al., 2020) network templates to extract intrinsic connectivity networks (ICNs) and corresponding time courses (TCs) through an entirely automated spatially restricted ICA method. These templates were obtained based on replicated networks estimated from two healthy control datasets, the human connectome project (Van Essen et al., 2012) and the genomics super struct project (Holmes et al., 2015). More details of the Neuromark framework and templates can be found in the GIFT toolbox http://trendscenter.org/software/gift and at http://trendscenter.org/data (Correa et al., 2005). These spatial priors have been established to be highly consistent between pipelines and across various datasets and populations. We obtained 53 intrinsic connectivity networks for each subject by implementing this approach. To address any confounding effects, such as the greater degree of head motion present in pediatric images, we included four additional post-processing steps to regulate the remaining noise in the TCs of ICNs. These steps involved detrending linear, quadratic, and cubic trends, eliminating detected outliers, implementing multiple regression on the head motion parameters, and bandpass filtering. After the post-processing phase, Pearson correlation coefficients between post-processed TCs were calculated to measure the static functional network connectivity (sFNC) among 53 ICNs. The 53 × 53 symmetric sFNC matrix was then flattened, and 1,378 correlation values from off-diagonal elements were extracted. These form the third type of brain features of this study.
2.4 Environmental factors
In our study, we utilized a total of nine environmental factors spanning macro, meso, and micro scales (Thapaliya et al., 2021, 2023) from the ABCD Cohort, both because of their established relevance in the literature and our own group's previous work (Thapaliya et al., 2021, 2024) with these factors elucidating the complex relationship between the brain and the environment. We are aware that more environmental variables are now available, which are unexplored in our current research and can be utilized in future studies. The factors we included are air pollution, population density, area crime, neighborhood safety, school safety, household income, family conflict, early life stress (ELS), and area deprivation. Each factor was derived by aggregating multiple related variables to construct a comprehensive measure through summation, with variables reversed, if necessary, to maintain consistency in the direction of the effect. A higher value in air pollution, population density, area crime, family conflict, ELS, and area deprivation implies a negative or unfavorable direction. Conversely, higher values in neighborhood safety, school safety, and household income indicate a positive or desirable direction, such as a safe neighborhood and school environment and better socio-economic status. A detailed explanation of the relevant variables of each environmental factor is presented in Supplementary material.
2.5 Cognitive measures
In this study, we utilized three cognitive composite scores from the NIH Toolbox Cognition Battery (Akshoomoff et al., 2013) for our analysis. The three main cognitive summary scores are: (i) crystallized cognition composite score (combination of picture vocabulary and oral reading recognition tests), which reflects crystallized cognition based on past learning experiences. (ii) fluid cognition composite score (includes tests that evaluate fluid abilities like executive function, working memory, attention, and processing speed), which demonstrates the ability for new learning and information processing in unexplored situations. (iii) total cognitive function composite score, which provides a comprehensive measure of general cognitive abilities by combining both the crystallized and fluid cognition composite scores.
2.6 Statistical analysis
We utilized multi-set canonical correlation analysis to identify the distinct multivariate patterns of different brain feature sets, as well as environmental factors, for the accelerated and delayed subpopulation. Canonical correlation analysis (CCA) is a statistical approach, first proposed by Hotelling (Hardoon et al., 2004) in 1936, which tries to find pairs of linear projections for different views in such a way that the correlation between them is maximized.
If we have two data matrices and where n denotes the number of samples and dx, dy indicate feature dimensions for data matrices X and Y, respectively. CCA will find m pairs of linear projections. and represent the canonical weight matrices for two data matrices X and Y. The correlation between ath pair of canonical projections and are maximized as in Equation 1. Equation 1 can be simplified as Equation 2.
The mCCA approach basically extends the concept of the general form of CCA in order to find correlated patterns among more than two views. Multi-view CCA aims to maximize the sum of pairwise canonical correlations via optimizing canonical vectors of all views. ci is the regularization parameter to be defined.
In Equations 2, 3, the orthogonality constraint ensures that every pair of canonical variables is orthogonal/uncorrelated with another pair of canonical variables.
To investigate the covariation patterns of the brain and the environment in this subpopulation, we conducted a 4-way mCCA analysis using the environmental factors and three types of brain features. Specifically, mCCA was applied to the subject gray matter loadings of 100 independent components, 152 morphological measures, 1,378 sFNC values, and nine environmental factors. The data were split as follows: 80% for training and 20% for testing, with a stratified 3-fold cross-validation in the training set to select the ci parameter and avoid overfitting issues. We used the CCA-Zoo (Chapman and Wang, 2021) package for our analysis. To be conservative, we selected the number of canonical variable sets based on the smallest dataset. Since the top four principal components explained nearly 90% of the variance in the smallest input data matrix of nine environmental factors, we applied mCCA with four sets of canonical variables in the training data to derive correlated brain and environmental variables. Thus, only those brain patterns potentially associated with environmental factors were extracted. The derived latent variables were directly projected into the testing data to verify their associations. In order to test for significant mean differences between the accelerated and delayed groups, we applied the analysis of variance (ANOVA) method on latent variables. To help further understanding of our findings, we examined the canonical weight and shared variance percentage of each original feature to identify its contribution to the canonical latent variables. Canonical weights are the values in the canonical vectors W in Equation 3. The shared variance percentage is calculated as the square of the correlation between an original observed feature and its corresponding canonical variable (r2).
To test the stability and robustness of the mCCA results, we applied the mCCA analysis 100 times, each time randomly splitting the data into an 80/20 split for training and hold-out test data. The mCCA model was trained on the training data and tested on the hold-out data. We then reported the average canonical weights, the variance explained, and the frequency of a given feature that was among the “Top 5” list across the 100 mCCA models on the hold-out test data. For illustration purposes, related brain areas for the top five (arbitrary selection) features based on the shared variance percentage from the three brain datasets (ICA, FreeSurfer, and sFNC) are plotted using Talairach Daemon software from the GIFT toolbox and the Desikan atlas and displayed in the results section (Rachakonda et al., 2007).
To test the associations with cognition and brain maturation group, we implemented linear mixed-effects regression models using cognitive measures (NIH Toolbox Fluid Cognition Composite Score, Crystallized Cognition Composite Score, and Total Cognition Composite Score) as the dependent variable, with age, gender (Male = 0, Female = 1), and brain maturation group (Accelerated = 3, Delayed = 2) as fixed-effect independent variables. ABCD Family ID nested within ABCD site information was included as random-effect variables. We included each subject's actual age and gender as predictors, along with the developmental group, to control for the known effects of age and gender on cognition during development. Additionally, we conducted linear mixed-effects regression analyses on our 20% test data to examine associations with cognitive measures and brain-related canonical variables, using the cognitive score as the dependent variable and age, gender, and brain-related canonical variables as fixed-effect independent variables, while ABCD Family ID nested within ABCD site information were included as random effect variables.
3 Results
In our 4-way mCCA analysis, four sets of canonical variables were extracted during the training, while each set comprised of four canonical latent variables: three latent brain variables and one latent environmental variable. All four canonical variables within each set were significantly correlated (p-values that survived FDR correction) during the training phase. The direct projection of derived latent variables into the 20% hold-out testing data revealed that all four sets of canonical latent variables were significantly cross-correlated within each set in the testing data as well (see Table 2). Since results from the testing data are more impartial, hereafter we present only those results. The correlations between canonical variables of the brain features were high with r-values ranging from 0.88 to 0.55. In contrast, the correlations of environmental factors with brain features were low, but statistically significant, with r-values ranging between 0.37 and 0.09.
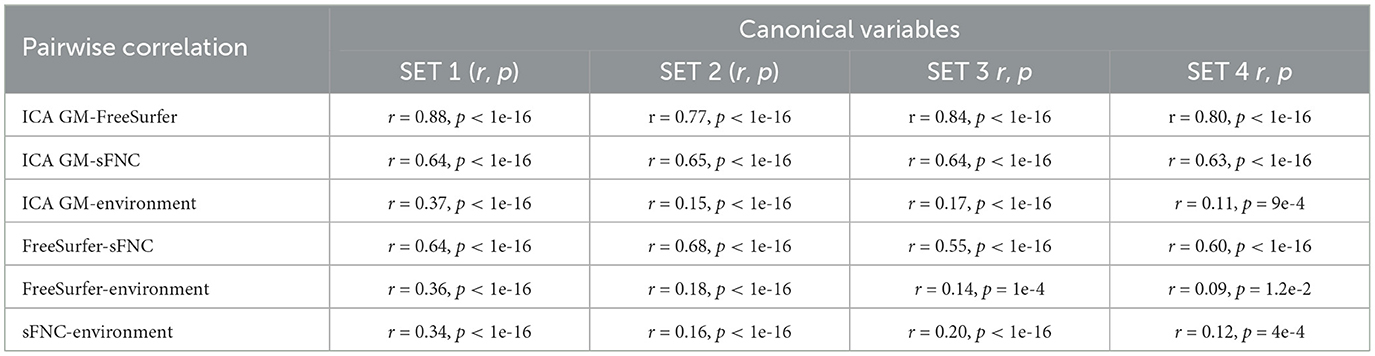
Table 2. Pairwise correlation of four sets of canonical variables (results from the 20% testing data).
A detailed report of the brain regions and associated environmental variables is presented in Supplementary Tables 1–8. The 1st derived environmental variable highlights the positive effect of household income (shared variance percentage of 71.95%, canonical weight of 0.16) and the negative effect of area deprivation (shared variance percentage of 68.56%, canonical weight of −0.14) (see Supplementary Table 2). The 2nd environmental variable highlights air pollution and area crime (see Supplementary Table 4). The 3rd environmental variable emphasizes area deprivation (shared variance percentage of 77.11%, canonical weight of 0.12) in contrast to household income (shared variance percentage of 44.15%, canonical weight of −0.02) and neighborhood safety (shared variance percentage of 44.95%, canonical weight of −0.04) (see Supplementary Table 6). The 4th environmental variable highlights relatively small effects of negative household income (shared variance percentage of 64.16%, canonical weight of −0.10) and positive area deprivation (shared variance percentage of 49.04%, canonical weight of 0.06) (see Supplementary Table 8). All four environmental variables are associated with specific brain features (see Supplementary Tables 1–8).
We further examined whether the derived canonical variables had differences between the accelerated and delayed brain age groups. The 2nd set of canonical variables showed consistently significant differences in the three brain-related canonical latent variables, where the accelerated group had higher values than the delayed group. The environmental canonical latent variable did not show group differences in all four sets. We presented the results of the testing data in Table 3. Given our interest is in identifying brain patterns related to brain development, the 2nd set of canonical variables became our focus hereafter. We presented their four-way associations on testing data in Figure 1. The figure displays the pairwise canonical correlation coefficients among the four canonical latent variables, as well as the top three features contributing to each of the canonical variables for illustration purposes. Based on the percentage of shared variance calculated using the testing data, we have identified the top five contributing features for each canonical variable. Table 4 provides feature names, canonical weights, and shared variance percentages. The resulting brain areas corresponding to these top five features are depicted collectively in Figure 2, with green indicating gray matter ICA components, red representing FreeSurfer morphological features, and blue denoting sFNC.
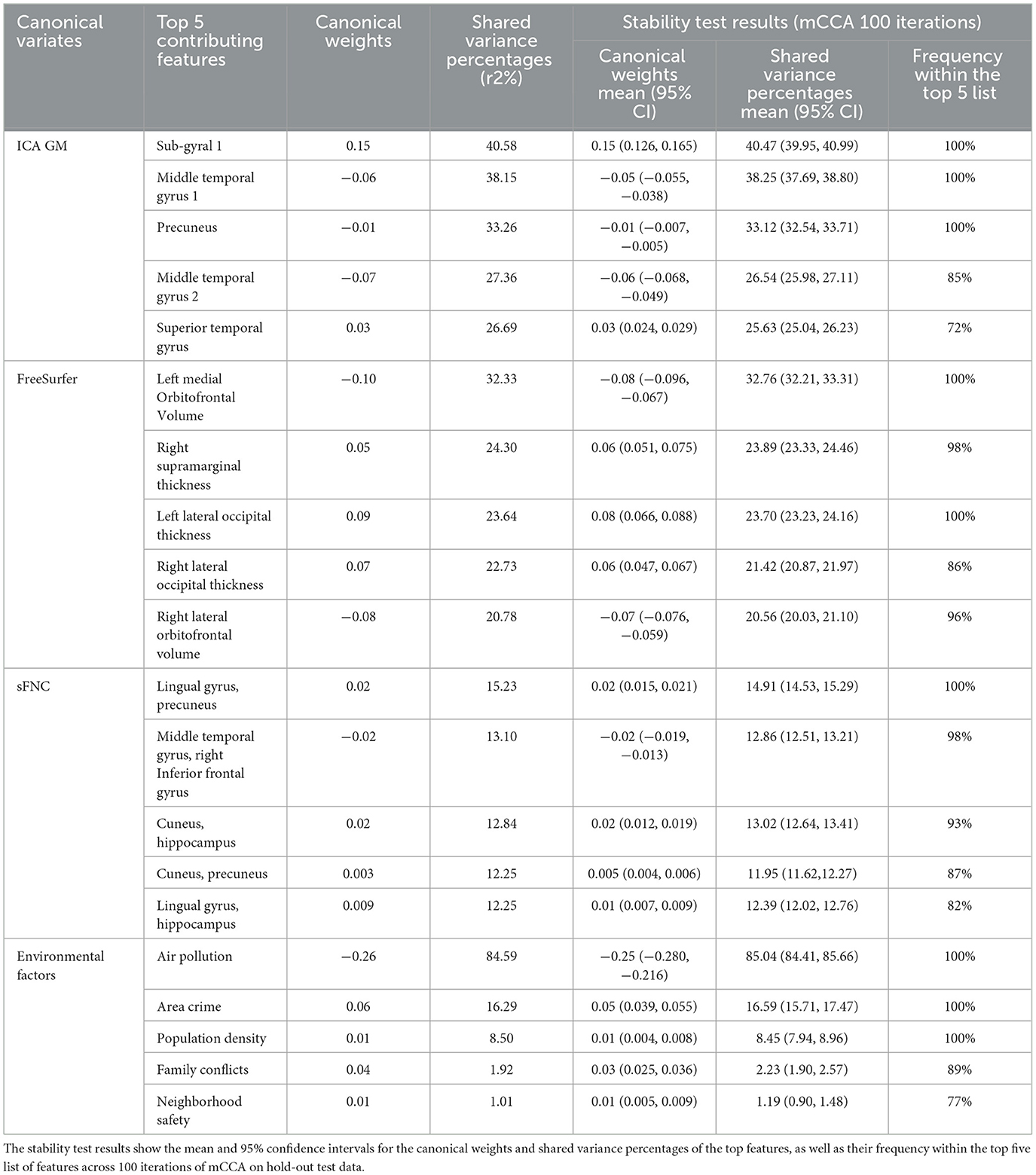
Table 4. The top five contributing features of the 2nd set of canonical variables, along with their canonical weights and shared variance percentages in the testing data, are presented.
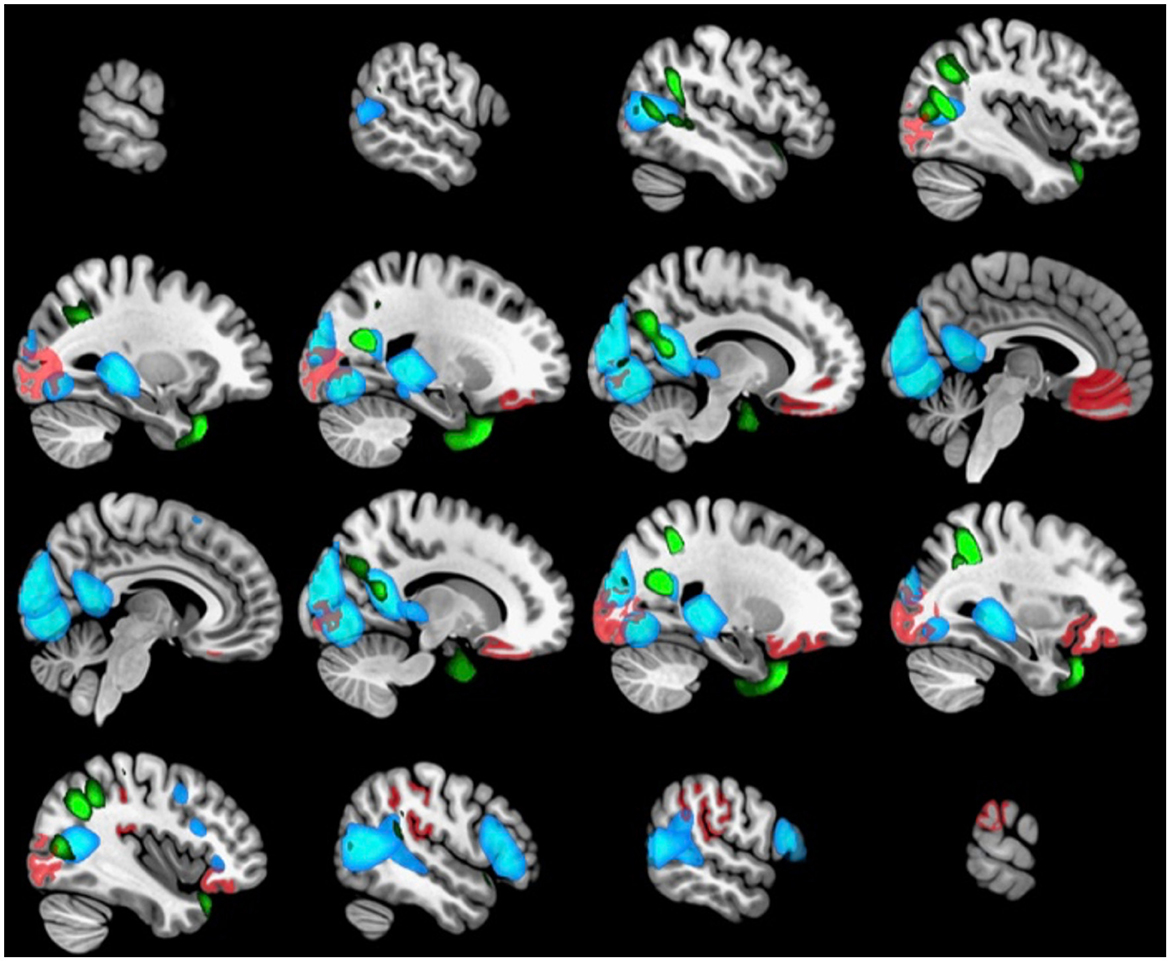
Figure 2. The brain regions associated with the top five contributing features of the 2nd set of brain canonical variables (ICA GM in green, FreeSurfer in red, sFNC in blue).
We performed linear mixed-effect regression model analyses on the accelerated and delayed brain age group to test the associations with cognitive measures and the brain maturation group. The results showed a t−value:4.24, p < 1e−16 for the Total composite score, t−value:4.19, p < 1e−16 for the Fluid composite score, and t = 2.63, p = 0.009 for the Crystallized composite score, suggesting the accelerated age group showed significantly higher performance on cognitive tests, consistent with accelerated brain maturation. Moreover, the association analyses between cognitive scores and brain-related canonical variables showed that the 1st set of brain canonical variables were all significantly associated with all three cognitive composite scores, passing FDR correction. Sets 2, 3, and 4 brain canonical variables were not associated with cognitive measures. The detailed association results are listed in Supplementary Table 9.
4 Discussion and conclusion
Our study aims to explore the multifaceted brain features that are associated with brain maturation and their relationship with environmental factors. From the ABCD cohort, we have identified a subpopulation of participants with either accelerated or delayed brain age using the brain age estimation model from our prior study. These two groups showed significant differences in cognitive measures, with the accelerated brain age group performing higher than the delayed group on cognitive measures, even after adjusting for biological age. To study environmental effects on multimodal brain features, we performed a 4-way mCCA analysis on regional gray matter density, morphometric measures, resting-state functional network connectivity, and environmental factors from nine domains. The 4-way mCCA analysis revealed highly related patterns between the three types of brain measures and their relationship with the environment.
Four sets of 4-way canonical variable correlations were verified (all four latent variables were significantly correlated in the testing data), and each set highlights a different environmental association with brain patterns. While the 1st, 3rd, and 4th sets present mainly effects from household income, area deprivation, and neighborhood, the 2nd set presents effects of air pollution and area crime at large. The 2nd set of brain canonical variables also demonstrates significant mean differences between the accelerated and delayed groups. The 1st set of brain canonical variables are also positively associated with all three cognitive scores (see Supplementary Table 9). Given they relate significantly to household income, which is one of the most studied environmental factors with abundant evidence for associations with brain and cognition (Tomasi and Volkow, 2021, 2023), we are not surprised to observe this particular set of brain canonical variables associated with overall cognition. The lack of cognitive association of other sets of brain variables warrants further investigation on more specific cognitive ability, such as processing speed, inhibition, etc., in addition to over composite scores. Though all four sets of brain-environmental associations are valid and important, given our research interest, we focus our discussion on the associations among brain and environmental latent variables from the 2nd set of canonical variables.
To improve the interpretation of how the original observed features contribute to the latent variables, we presented the canonical weights assigned to each variable and the percentage of variance explained. The magnitude of the weight indicates the degree of contribution of the feature to the latent variable. Features with positive weights contribute to the canonical variable positively, while features with negative weights contribute inversely. However, interpreting the importance of a feature based on its canonical weight is subject to the limitation of the beta weights in regression analyses. Small weight can either suggest that the corresponding feature is nonessential, or its contribution has been partially explained by other features due to high multicollinearity. This limitation applies only to the interpretation of the CCA components, not to their extraction since multicollinearity is not a concern for extracting CCA components. CCA is designed to extract multivariate linearly related patterns within each dataset, ensuring that these linear patterns are correlated across datasets. To address the limitation inherent in canonical weights, we also examined the percentage of variance shared which represents the degree to which the observed feature shares variance with the canonical variable.
The accelerated brain age group displayed higher values across all three canonical variables of brain measures compared to the delayed group. First, the ICA gray matter density of the top regions contributed highly to the ICA latent variable. Notably, the first and last contributing regions exhibited positive canonical weights, while the remaining three areas had negative canonical weights (refer to Table 4). Positive weights indicated increased gray matter density in sub-gyral and superior temporal gyrus regions, whereas negative weights indicated reduced gray matter density in middle temporal gyrus and precuneus regions, resulting in higher values of the canonical variable. The sub-gyral region stood out as the most prominent contributor, accounting for 40.58% of the shared variance, followed by the middle temporal gyrus with 38.15%. Therefore, reduced gray matter density in the middle temporal gyrus and precuneus, along with increased density in the superior temporal region, albeit to a lesser extent, contributed to elevated values in the latent gray matter variable. The accelerated group exhibited higher values than the delayed group, implying potentially greater reductions in gray matter density overall, consistent with advanced brain maturation observed during this stage of adolescence (Arain et al., 2013; Giedd et al., 1999; Gogtay and Thompson, 2010; Paus, 2005; Whitford et al., 2007).
Typically, maturation of gray matter volume appears first as linear loss in the dorsal parietal cortices, frontal and occipital poles, then progresses rostrally through the frontal cortex as quadratic and cubic gray matter volume loss (Gogtay et al., 2004). This reduction in gray matter volume is accompanied by increases in cortical thickness in the frontal and parieto-occipital regions as the brain matures (Sowell et al., 2004). Our results are in alignment with the current understanding of the maturation of gray matter volume and cortical thickness. The top five features that contributed to the latent morphological variable were decreases in gray matter volume in the orbitofrontal regions (left medial, and right lateral) and increases in cortical thickness in the right supramarginal region and bilateral occipital regions. The accelerated maturation group demonstrated higher values in this latent variable, confirming that reduction in gray matter volume in the orbitofrontal cortex, alongside increases in cortical thickness in the supramarginal and lateral occipital cortex are associated with the accelerated brain age.
Maturation of functional connectivity during adolescence is marked by increased segregation between short-range connectivity as well as increased integration of long-range connectivity (Fair et al., 2007). This is seen in decreases in connectivity between the anterior PFC, dorsolateral PFC, and the frontoparietal control network in conjunction with increases in connectivity between the dorsal ACC, the medial superior frontal cortex, and the cingula-opercular control network (Stevens et al., 2009). Consistent with this understanding of adolescent maturation, our study showed positive contributions to the latent variable from the functional connectivity of the occipital regions (cuneus, precuneus, and lingual gyrus), while connectivity between the middle temporal gyrus and right inferior frontal gyrus contributed negatively. The accelerated maturation group again had higher values with regard to the latent variable, confirming that they are experiencing accelerated brain maturation.
All three latent brain variables in our study were highly correlated, confirming our expectation that changes in gray matter density, cortical thickness, and functional connectivity are tightly coupled and covary during brain development. Figure 2 also illustrates anatomical regions from the three latent variables that are concentrated in the posterior part of the brain (occipital, temporal, and parietal cortices), accompanied by the inferior orbital part of the frontal cortex. Our results suggest the accelerated brain age group may be experiencing accelerated gray matter density reduction in the middle temporal gyrus and precuneus, volume reduction in the orbitofrontal cortex, as well as increased thickness in the supramarginal region, and increased functional connectivity across cortical-subcortical regions.
The environmental variable linked with the second set of brain patterns reveals that air pollution has the highest shared variance percentage at 84.59% and a canonical weight of −0.26, positioning it as the primary contributing feature (see Table 4). Following air pollution, area crime, and population density emerge as significant contributors to the environmental canonical variable, with canonical weights of 0.06 and 0.01, and shared variance percentages of 16.29 and 8.50%, respectively. Low levels of air pollution coupled with higher area crime and population density result in a heightened latent variable that exhibits positive associations with all three latent brain variables. Although there are no discernible group differences in the environmental variable, its positive correlations with brain variables suggest that exposure to an environment characterized by low air pollution and elevated area crime and population density is associated with patterns of brain regions that include reductions in gray matter and enhanced functional connectivity, regions which are more prominent in the accelerated brain aging group. It seems counterintuitive that lower air pollution, a desirable environmental factor, would be coupled with unfavorable or harsh neighborhood conditions like higher crime rate and population density. However, in terms of brain development/maturation both favorable and unfavorable factors can work in the same direction. Prior research has suggested that individuals may mature faster when exposed to harsh social environmental conditions (Hedderich et al., 2021), and brain development trajectory may be altered by air pollution in a time-sensitive manner (Herting et al., 2024). The lack of group difference in environmental variables could be interpreted in two ways. One is that environmental variables stimulate brain development in specific patterns but with small effect sizes, so large samples are needed to verify environmental effects. The other is that environmental variables are only associated with the part of the variance in the brain patterns not showing brain development differences, and the part showing brain development difference is contributed by something else, such that more investigations on other environmental factors are warranted. In the literature air pollution (specifically fine particulate PM2.5) has been reported to have a positive correlation with gray matter volumetric changes across various brain regions, such as the medial orbitofrontal cortex, while a negative association between air pollution and gray matter volume is observed in regions like the superior temporal gyrus (Miller et al., 2022). Our result showed that a low level of ambient air pollution is linked to a reduction of gray matter density in the middle temporal gyrus and the precuneus, as well as a reduction of gray matter volume in the left medial orbitofrontal cortex and the right lateral orbitofrontal cortex. Moreover, low air pollution is associated with increased gray matter density in the sub-gyral, superior temporal gyrus, and increased cortical thickness in the right supramarginal, bilateral occipital brain regions.
The limitations of our study are also opportunities for future research. Even though our study shows significant associations between environmental factors and brain maturation, we note that these results are only observational. These findings could be affected by potential confounders such as genetics, culture, or other unexplored demographic elements. The mCCA method assumes a linear relationship between observed features and the latent variables, as well as a linear correlation between different datasets. The method will fail to capture hidden relationships if they are complex nonlinear interactions. All our findings are based on ABCD baseline data with a cross-sectional analytical design. A longitudinal analysis of the ABCD cohort that tracks the changes in the multivariate relationships between brain-environment will paint a more complete and likely more complex picture.
In sum, our research leveraged brain age estimation in a large developmental cohort and revealed neuronal structural and functional variations associated with accelerated vs. delayed brain maturation. Furthermore, we provided evidence of associations between environmental factors and brain maturation, suggesting that such factors may modulate neuronal variations. However, the influence of other confounders should be considered. Future longitudinal studies on brain development could further unveil the dynamic trajectory of environmental factors.
Data availability statement
The data analyzed in this study is subject to the following licenses/restrictions: data used in the preparation of this manuscript were obtained from the Adolescent Brain Cognitive Development (ABCD) Study (https://abcdstudy.org) shared by National Institute of Mental Health Data Archive (NDA) (https://nda.nih.gov/). Requests to access these datasets should be directed to https://abcdstudy.org and https://nda.nih.gov/.
Ethics statement
Ethical review and approval was not required for the study on human participants in accordance with the local legislation and institutional requirements. Written informed consent from the patients/participants or patients/participants' legal guardian/next of kin was not required to participate in this study in accordance with the national legislation and the institutional requirements.
Author contributions
BR: Conceptualization, Data curation, Formal analysis, Investigation, Methodology, Project administration, Software, Validation, Visualization, Writing – original draft, Writing – review & editing. DJ: Writing – original draft, Writing – review & editing. PS: Writing – original draft, Writing – review & editing. BT: Writing – original draft, Writing – review & editing. RS: Writing – original draft, Writing – review & editing. BF: Writing – original draft, Writing – review & editing. ZF: Writing – original draft, Writing – review & editing, Data curation. JC: Writing – original draft, Writing – review & editing. VC: Funding acquisition, Resources, Supervision, Writing – original draft, Writing – review & editing. JL: Conceptualization, Funding acquisition, Methodology, Project administration, Resources, Supervision, Validation, Writing – original draft, Writing – review & editing.
Funding
The author(s) declare financial support was received for the research, authorship, and/or publication of this article. This work was supported by the National Institutes of Health (NIH; R01DA049238) and the National Science Foundation of the USA (NSF; 2112455) to VC and JL.
Conflict of interest
The authors declare that the research was conducted in the absence of any commercial or financial relationships that could be construed as a potential conflict of interest.
The author(s) declared that they were an editorial board member of Frontiers, at the time of submission. This had no impact on the peer review process and the final decision.
Publisher's note
All claims expressed in this article are solely those of the authors and do not necessarily represent those of their affiliated organizations, or those of the publisher, the editors and the reviewers. Any product that may be evaluated in this article, or claim that may be made by its manufacturer, is not guaranteed or endorsed by the publisher.
Supplementary material
The Supplementary Material for this article can be found online at: https://www.frontiersin.org/articles/10.3389/fnimg.2024.1390409/full#supplementary-material
References
Akshoomoff, N., Beaumont, J. L., Bauer, P. J., Dikmen, S. S., Gershon, R. C., Mungas, D., et al. (2013). VIII. NIH toolbox cognition battery (CB): composite scores of crystallized, fluid, and overall cognition. Monogr. Soc. Res. Child Dev. 78, 119–132. doi: 10.1111/mono.12038
Alderton, A., Villanueva, K., O'Connor, M., Boulangé, C., and Badland, H. (2019). Reducing inequities in early childhood mental health: How might the neighborhood built environment help close the gap? A systematic search and critical review. Int. J. Environ. Res. Public Health 16:1516. doi: 10.3390/ijerph16091516
Amari, S.-I. (1998). Natural gradient works efficiently in learning. Neural Comput. 10, 251–276. doi: 10.1162/089976698300017746
Arain, M., Haque, M., Johal, L., Mathur, P., Nel, W., Rais, A., et al. (2013). Maturation of the adolescent brain. Neuropsychiatr. Dis. Treat. 9, 449–461. doi: 10.2147/NDT.S39776
Ashburner, J., Barnes, G., Chen, C.-C., Daunizeau, J., Flandin, G., Friston, K., et al. (2014). SPM12 Manual. London: Wellcome Trust Centre for Neuroimaging.
Baecker, L., Garcia-Dias, R., Vieira, S., Scarpazza, C., and Mechelli, A. (2021). Machine learning for brain age prediction: Introduction to methods and clinical applications. EBioMedicine 72:103600. doi: 10.1016/j.ebiom.2021.103600
Baranyi, G., Di Marco, M. H., Russ, T. C., Dibben, C., and Pearce, J. (2021). The impact of neighbourhood crime on mental health: a systematic review and meta-analysis. Soc. Sci. Med. 282:114106. doi: 10.1016/j.socscimed.2021.114106
Barnett, A., Zhang, C. J., Johnston, J. M., and Cerin, E. (2018). Relationships between the neighborhood environment and depression in older adults: a systematic review and meta-analysis. Int. Psychogeriatr. 30, 1153–1176. doi: 10.1017/S104161021700271X
Basodi, S., Raja, R., Ray, B., Gazula, H., Liu, J., Verner, E., et al. (2021). Federation of brain age estimation in structural neuroimaging data. Annu. Int. Conf. IEEE Eng. Med. Biol. Soc. 2021, 3854–3857. doi: 10.1109/EMBC46164.2021.9629865
Basodi, S., Raja, R., Ray, B., Gazula, H., Sarwate, A. D., Plis, S., et al. (2022). Decentralized brain age estimation using mri data. Neuroinformatics 20, 981–990. doi: 10.1007/s12021-022-09570-x
Bell, A. J., and Sejnowski, T. J. (1995). An information-maximization approach to blind separation and blind deconvolution. Neural Comput. 7, 1129–1159. doi: 10.1162/neco.1995.7.6.1129
Blakemore, S. J., and Choudhury, S. (2006). Development of the adolescent brain: implications for executive function and social cognition. J. Child Psychol. Psychiatry 47, 296–312. doi: 10.1111/j.1469-7610.2006.01611.x
Brieant, A. E., Sisk, L. M., and Gee, D. G. (2021). Associations among negative life events, changes in cortico-limbic connectivity, and psychopathology in the abcd study. Dev. Cogn. Neurosci. 52:101022. doi: 10.1016/j.dcn.2021.101022
Bush, N. R., Wakschlag, L. S., LeWinn, K. Z., Hertz-Picciotto, I., Nozadi, S. S., Pieper, S., et al. (2020). Family environment, neurodevelopmental risk, and the environmental influences on child health outcomes (echo) initiative: looking back and moving forward. Front. Psychiatry 11:547. doi: 10.3389/fpsyt.2020.00547
Cairns, J.-M., Graham, E., and Bambra, C. (2017). Area-level socioeconomic disadvantage and suicidal behaviour in europe: a systematic review. Soc. Sci. Med. 192, 102–111. doi: 10.1016/j.socscimed.2017.09.034
Casanova, R., Anderson, A., Barnard, R., Walker, K., Hughes, T., Kritchevsky, S., et al. (2022). Accelerated brain aging is associated with mortality across race. Innovat. Aging 6(Suppl. 1):784. doi: 10.1093/geroni/igac059.2834
Casey, B., Tottenham, N., Liston, C., and Durston, S. (2005). Imaging the developing brain: what have we learned about cognitive development? Trends Cogn. Sci. 9, 104–110. doi: 10.1016/j.tics.2005.01.011
Chapman, J., and Wang, H.-T. (2021). CCA-zoo: a collection of regularized, deep learning based, kernel, and probabilistic cca methods in a scikit-learn style framework. J. Open Source Softw. 6:3823. doi: 10.21105/joss.03823
Cipriani, G., Danti, S., Carlesi, C., and Borin, G. (2018). Danger in the air: air pollution and cognitive dysfunction. Am. J. Alzheimers Dis. Other Dement. 33, 333–341. doi: 10.1177/1533317518777859
Correa, N., Adali, T., Li, Y.-O., and Calhoun, V. D. (2005). “Comparison of blind source separation algorithms for fMRI using a new matlab toolbox: gift,” in Proceedings.(ICASSP'05). IEEE International Conference on Acoustics, Speech, and Signal Processing, 2005, Vol. 5 (Philadelphia, PA: IEEE), v-401.
Desikan, R. S., Ségonne, F., Fischl, B., Quinn, B. T., Dickerson, B. C., Blacker, D., et al. (2006). An automated labeling system for subdividing the human cerebral cortex on mri scans into gyral based regions of interest. Neuroimage 31, 968–980. doi: 10.1016/j.neuroimage.2006.01.021
Dimitrov-Discher, A., Wenzel, J., Kabisch, N., Hemmerling, J., Bunz, M., Schöndorf, J., et al. (2022). Residential green space and air pollution are associated with brain activation in a social-stress paradigm. Sci. Rep. 12:10614. doi: 10.1038/s41598-022-14659-z
Dosenbach, N. U., Nardos, B., Cohen, A. L., Fair, D. A., Power, J. D., Church, J. A., et al. (2010). Prediction of individual brain maturity using fmri. Science 329, 1358–1361. doi: 10.1126/science.1194144
Du, Y., Fu, Z., Sui, J., Gao, S., Xing, Y., Lin, D., et al. (2020). Neuromark: An automated and adaptive ica based pipeline to identify reproducible fmri markers of brain disorders. Neuroimage Clin. 28:102375. doi: 10.1016/j.nicl.2020.102375
Dunlop, K., Victoria, L. W., Downar, J., Gunning, F. M., and Liston, C. (2021). Accelerated brain aging predicts impulsivity and symptom severity in depression. Neuropsychopharmacology 46, 911–919. doi: 10.1038/s41386-021-00967-x
Fair, D. A., Dosenbach, N. U., Church, J. A., Cohen, A. L., Brahmbhatt, S., Miezin, F. M., et al. (2007). Development of distinct control networks through segregation and integration. Proc. Nat. Acad. Sci. U. S. A. 104, 13507–13512. doi: 10.1073/pnas.0705843104
Franke, K., and Gaser, C. (2019). Ten years of brainage as a neuroimaging biomarker of brain aging: what insights have we gained? Front. Neurol. 10:789. doi: 10.3389/fneur.2019.00789
Franke, K., Ziegler, G., Klöppel, S., Gaser, C., Initiative, A. D. N., et al. (2010). Estimating the age of healthy subjects from t1-weighted mri scans using kernel methods: exploring the influence of various parameters. Neuroimage 50, 883–892. doi: 10.1016/j.neuroimage.2010.01.005
Fu, Z., Liu, J., Salman, M. S., Sui, J., and Calhoun, V. D. (2023). Functional connectivity uniqueness and variability? Linkages with cognitive and psychiatric problems in children. Nat. Mental Health 1, 956–970. doi: 10.1038/s44220-023-00151-8
Gapp, K., Woldemichael, B. T., Bohacek, J., and Mansuy, I. (2014). Epigenetic regulation in neurodevelopment and neurodegenerative diseases. Neuroscience 264, 99–111. doi: 10.1016/j.neuroscience.2012.11.040
Giedd, J. N., Blumenthal, J., Jeffries, N. O., Castellanos, F. X., Liu, H., Zijdenbos, A., et al. (1999). Brain development during childhood and adolescence: a longitudinal MRI study. Nat. Neurosci. 2, 861–863. doi: 10.1038/13158
Gogtay, N., Giedd, J. N., Lusk, L., Hayashi, K. M., Greenstein, D., Vaituzis, A. C., et al. (2004). Dynamic mapping of human cortical development during childhood through early adulthood. Proc. Nat. Acad. Sci. U. S. A. 101, 8174–8179. doi: 10.1073/pnas.0402680101
Gogtay, N., and Thompson, P. M. (2010). Mapping gray matter development: implications for typical development and vulnerability to psychopathology. Brain Cogn. 72, 6–15. doi: 10.1016/j.bandc.2009.08.009
Green, J. G., McLaughlin, K. A., Berglund, P. A., Gruber, M. J., Sampson, N. A., Zaslavsky, A. M., et al. (2010). Childhood adversities and adult psychiatric disorders in the national comorbidity survey replication I: associations with first onset of DSM-IV disorders. Arch. Gen. Psychiatry 67, 113–123. doi: 10.1001/archgenpsychiatry.2009.186
Guxens, M., Lubczyńska, M. J., Muetzel, R. L., Dalmau-Bueno, A., Jaddoe, V. W., Hoek, G., et al. (2018). Air pollution exposure during fetal life, brain morphology, and cognitive function in school-age children. Biol. Psychiatry 84, 295–303. doi: 10.1016/j.biopsych.2018.01.016
Hackman, D. A., Cserbik, D., Chen, J. C., Berhane, K., Minaravesh, B., McConnell, R., et al. (2021). Association of local variation in neighborhood disadvantage in metropolitan areas with youth neurocognition and brain structure. JAMA Pediatr. 175, e210426. doi: 10.1001/jamapediatrics.2021.0426
Hagler, D. J., Hatton, S., Cornejo, M. D., Makowski, C., Fair, D. A., Dick, A. S., et al. (2019). Image processing and analysis methods for the adolescent brain cognitive development study. Neuroimage 202:116091. doi: 10.1016/j.neuroimage.2019.116091
Hardoon, D. R., Szedmak, S., and Shawe-Taylor, J. (2004). Canonical correlation analysis: an overview with application to learning methods. Neural Comput. 16, 2639–2664. doi: 10.1162/0899766042321814
Hedderich, D. M., Menegaux, A., Schmitz-Koep, B., Nuttall, R., Zimmermann, J., Schneider, S. C., et al. (2021). Increased brain age gap estimate (brainage) in young adults after premature birth. Front. Aging Neurosci. 13:653365. doi: 10.3389/fnagi.2021.653365
Herting, M. M., Bottenhorn, K. L., and Cotter, D. L. (2024). Outdoor air pollution and brain development in childhood and adolescence. Trends Neurosci. 47, 593–607. doi: 10.1016/j.tins.2024.06.008
Herzberg, M. P., and Gunnar, M. R. (2020). Early life stress and brain function: activity and connectivity associated with processing emotion and reward. Neuroimage 209:116493. doi: 10.1016/j.neuroimage.2019.116493
Holmes, C., Levy, M., Smith, A., Pinne, S., and Neese, P. (2015). A model for creating a supportive trauma-informed culture for children in preschool settings. J. Child Fam. Stud. 24, 1650–1659. doi: 10.1007/s10826-014-9968-6
Huttenlocher, P. R., and Dabholkar, A. S. (1997). Regional differences in synaptogenesis in human cerebral cortex. J. Comp. Neurol. 387, 167–178. doi: 10.1002/(SICI)1096-9861(19971020)387:2<167::AID-CNE1>3.0.CO;2-Z
Jenkinson, M., Beckmann, C. F., Behrens, T. E., Woolrich, M. W., and Smith, S. M. (2012). FSL. Neuroimage 62, 782–790. doi: 10.1016/j.neuroimage.2011.09.015
Jeong, H. J., Moore, T. M., Durham, E. L., Reimann, G. E., Dupont, R. M., Cardenas-Iniguez, C., et al. (2023). General and specific factors of environmental stress and their associations with brain structure and dimensions of psychopathology. Biol. Psychiatry Glob. Open Sci. 3, 480–489. doi: 10.1016/j.bpsgos.2022.04.004
Jorgensen, N. A., Muscatell, K. A., McCormick, E. M., Prinstein, M. J., Lindquist, K. A., and Telzer, E. H. (2023). Neighborhood disadvantage, race/ethnicity and neural sensitivity to social threat and reward among adolescents. Soc. Cogn. Affect. Neurosci. 18:nsac053. doi: 10.1093/scan/nsac053
Kaufmann, T., Alnaes, D., Doan, N. T., Brandt, C. L., Andreassen, O. A., and Westlye, L. T. (2017). Delayed stabilization and individualization in connectome development are related to psychiatric disorders. Nat. Neurosci. 20, 513–515. doi: 10.1038/nn.4511
Khan, A. R., Wang, L., and Beg, M. F. (2008). Freesurfer-initiated fully-automated subcortical brain segmentation in mri using large deformation diffeomorphic metric mapping. Neuroimage 41, 735–746. doi: 10.1016/j.neuroimage.2008.03.024
Konrad, K., Firk, C., and Uhlhaas, P. J. (2013). Brain development during adolescence: neuroscientific insights into this developmental period. Dtsch. Arztebl. Int. 110, 425–431. doi: 10.3238/arztebl.2013.0425
Kwon, H., Reiss, A. L., and Menon, V. (2002). Neural basis of protracted developmental changes in visuo-spatial working memory. Proc. Natl. Acad. Sci. U. S. A. 99, 13336–13341. doi: 10.1073/pnas.162486399
Laruelle, M., Kegeles, L., Zea-Ponce, Y., Mawlawi, O., Martinez, D., Abi-Dargham, A., et al. (2002). Amphetamine-induced dopamine release in patients with schizotypal personality disorders studied by spect and [1231] ibzm. Neuroimage 16, S61–S61.
Lederbogen, F., Haddad, L., and Meyer-Lindenberg, A. (2013). Urban social stress-risk factor for mental disorders. The case of schizophrenia. Environ. Pollut. 183, 2–6. doi: 10.1016/j.envpol.2013.05.046
Li, Y. O., Adali, T., Wang, W., and Calhoun, V. D. (2009). Joint blind source separation by multi-set canonical correlation analysis. IEEE Trans. Signal Process. 57, 3918–3929. doi: 10.1109/TSP.2009.2021636
Liem, F., Varoquaux, G., Kynast, J., Beyer, F., Kharabian Masouleh, S., Huntenburg, J. M., et al. (2017). Predicting brain-age from multimodal imaging data captures cognitive impairment. Neuroimage 148, 179–188. doi: 10.1016/j.neuroimage.2016.11.005
Luo, N., Sui, J., Abrol, A., Chen, J., Turner, J. A., Damaraju, E., et al. (2020). Structural brain architectures match intrinsic functional networks and vary across domains: a study from 15 000+ individuals. Cereb. Cortex 30, 5460–5470. doi: 10.1093/cercor/bhaa127
Miller, D. J., Duka, T., Stimpson, C. D., Schapiro, S. J., Baze, W. B., McArthur, M. J., et al. (2012). Prolonged myelination in human neocortical evolution. Proc. Natl. Acad. Sci. U. S. A. 109, 16480–16485. doi: 10.1073/pnas.1117943109
Miller, J. G., Dennis, E. L., Heft-Neal, S., Jo, B., and Gotlib, I. H. (2022). Fine particulate air pollution, early life stress, and their interactive effects on adolescent structural brain development: a longitudinal tensor-based morphometry study. Cereb. Cortex 32, 2156–2169. doi: 10.1093/cercor/bhab346
Modabbernia, A., Janiri, D., Doucet, G. E., Reichenberg, A., and Frangou, S. (2021). Multivariate patterns of brain-behavior-environment associations in the adolescent brain and cognitive development study. Biol. Psychiatry 89, 510–520. doi: 10.1016/j.biopsych.2020.08.014
Paus, T. (2005). Mapping brain maturation and cognitive development during adolescence. Trends Cogn. Sci. 9, 60–68. doi: 10.1016/j.tics.2004.12.008
Paus, T., Zijdenbos, A., Worsley, K., Collins, D. L., Blumenthal, J., Giedd, J. N., et al. (1999). Structural maturation of neural pathways in children and adolescents: in vivo study. Science 283, 1908–1911. doi: 10.1126/science.283.5409.1908
Peng, H., Gong, W., Beckmann, C. F., Vedaldi, A., and Smith, S. M. (2021). Accurate brain age prediction with lightweight deep neural networks. Med. Image Anal. 68:101871. doi: 10.1016/j.media.2020.101871
Phillips, N. S., Baedke, J. L., Williams, A., Ji, Q., Zhang, S., Scoggins, M., et al. (2023). Accelerated brain age and associated neurocognitive impairments in adult survivors of childhood cancer. J. Clin. Oncol. 41(16_suppl), 10028–10028. doi: 10.1200/JCO.2023.41.16_suppl.10028
Pujol, J., Martínez-Vilavella, G., Macià, D., Fenoll, R., Alvarez-Pedrerol, M., Rivas, I., et al. (2016). Traffic pollution exposure is associated with altered brain connectivity in school children. Neuroimage 129, 175–184. doi: 10.1016/j.neuroimage.2016.01.036
Rachakonda, S., Egolf, E., Correa, N., and Calhoun, V. (2007). Group ICA of fMRI toolbox (gift) manual. Dostupnez.
Rakesh, D., Zalesky, A., and Whittle, S. (2021). Similar but distinct - effects of different socioeconomic indicators on resting state functional connectivity: findings from the adolescent brain cognitive development (abcd) study(r). Dev. Cogn. Neurosci. 51:101005. doi: 10.1016/j.dcn.2021.101005
Rakesh, D., Zalesky, A., and Whittle, S. (2022). Assessment of parent income and education, neighborhood disadvantage, and child brain structure. JAMA Netw. Open 5:e2226208. doi: 10.1001/jamanetworkopen.2022.26208
Rakesh, D., Zalesky, A., and Whittle, S. (2023). The role of school environment in brain structure, connectivity, and mental health in children: a multimodal investigation. Biol. Psychiatry Cogn. Neurosci. Neuroimaging 8, 32–41. doi: 10.1016/j.bpsc.2022.01.006
Ramduny, J., Bastiani, M., Huedepohl, R., Sotiropoulos, S. N., and Chechlacz, M. (2022). The association between inadequate sleep and accelerated brain ageing. Neurobiol. Aging 114, 1–14. doi: 10.1016/j.neurobiolaging.2022.02.005
Ray, B., Chen, J., Fu, Z., Suresh, P., Thapaliya, B., Farahdel, B., et al. (2023). Replication and refinement of brain age model for adolescent development. bioRxiv. doi: 10.1101/2023.08.16.553472
Ray, B., Chen, J., Fu, Z., Suresh, P., Thapaliya, B., Farahdel, B., et al. (2024). “Replication and refinement of brain age model for adolescent development,” in 2024 IEEE International Symposium on Biomedical Imaging (ISBI) (Athens: IEEE), 1–5. doi: 10.1109/ISBI56570.2024.10635532
Ray, B., Duan, K., Chen, J., Fu, Z., Suresh, P., Johnson, S., et al. (2021). Multimodal brain age prediction with feature selection and comparison. Annu. Int. Conf. IEEE Eng. Med. Biol. Soc. 2021, 3858–3864. doi: 10.1109/EMBC46164.2021.9631007
Rubia, K., Overmeyer, S., Taylor, E., Brammer, M., Williams, S. C., Simmons, A., et al. (2000). Functional frontalisation with age: mapping neurodevelopmental trajectories with fMRI. Neurosci. Biobehav. Rev. 24, 13–19. doi: 10.1016/S0149-7634(99)00055-X
Saha, R., Saha, D. K., Rahaman, M. A., Fu, Z., and Calhoun, V. D. (2022). “Longitudinal whole-brain functional network change patterns over a two-year period in the abcd data,” in 2022 IEEE 19th International Symposium on Biomedical Imaging (ISBI) (Kolkata: IEEE), 1–4. doi: 10.1109/ISBI52829.2022.9761647
Sampson, L., Ettman, C. K., and Galea, S. (2020). Urbanization, urbanicity, and depression: a review of the recent global literature. Curr. Opin. Psychiatry 33, 233–244. doi: 10.1097/YCO.0000000000000588
Satterthwaite, T. D., Connolly, J. J., Ruparel, K., Calkins, M. E., Jackson, C., Elliott, M. A., et al. (2016). The philadelphia neurodevelopmental cohort: a publicly available resource for the study of normal and abnormal brain development in youth. Neuroimage 124(Pt B), 1115–1119. doi: 10.1016/j.neuroimage.2015.03.056
Smith, K. E., and Pollak, S. D. (2020). Early life stress and development: potential mechanisms for adverse outcomes. J. Neurodev. Disord. 12, 1–15. doi: 10.1186/s11689-020-09337-y
Smith, S. M., Jenkinson, M., Woolrich, M. W., Beckmann, C. F., Behrens, T. E., Johansen-Berg, H., et al. (2004). Advances in functional and structural mr image analysis and implementation as fsl. Neuroimage 23(Suppl. 1), S208–S219. doi: 10.1016/j.neuroimage.2004.07.051
Sowell, E. R., Thompson, P. M., Leonard, C. M., Welcome, S. E., Kan, E., and Toga, A. W. (2004). Longitudinal mapping of cortical thickness and brain growth in normal children. J. Neurosci. 24, 8223–8231. doi: 10.1523/JNEUROSCI.1798-04.2004
Sowell, E. R., Thompson, P. M., Tessner, K. D., and Toga, A. W. (2001). Mapping continued brain growth and gray matter density reduction in dorsal frontal cortex: inverse relationships during postadolescent brain maturation. J. Neurosci. 21, 8819–8829. doi: 10.1523/JNEUROSCI.21-22-08819.2001
Stevens, M. C., Pearlson, G. D., and Calhoun, V. D. (2009). Changes in the interaction of resting-state neural networks from adolescence to adulthood. Hum. Brain Mapp. 30, 2356–2366. doi: 10.1002/hbm.20673
Sui, Y., Ettema, D., and Helbich, M. (2022). Longitudinal associations between the neighborhood social, natural, and built environment and mental health: A systematic review with meta-analyses. Health Place 77:102893. doi: 10.1016/j.healthplace.2022.102893
Taylor, R. L., Cooper, S. R., Jackson, J. J., and Barch, D. M. (2020). Assessment of neighborhood poverty, cognitive function, and prefrontal and hippocampal volumes in children. JAMA Netw. Open 3:e2023774. doi: 10.1001/jamanetworkopen.2020.23774
Thapaliya, B., Calhoun, V. D., and Liu, J. (2021). “Environmental and genome-wide association study on children anxiety and depression,” in 2021 IEEE International Conference on Bioinformatics and Biomedicine (BIBM) (Houston, TX: IEEE), 2330–2337. doi: 10.1109/BIBM52615.2021.9669291
Thapaliya, B., Ray, B., Farahdel, B., Suresh, P., Sapkota, R., consortium, I., et al. (2023). Cross-continental environmental and genome-wide association study on children and adolescent anxiety and depression. medRxiv. doi: 10.21203/rs.3.rs-2744140/v1
Thapaliya, B., Ray, B., Farahdel, B., Suresh, P., Sapkota, R., Holla, B., et al. (2024). Cross-continental environmental and genome-wide association study on children and adolescent anxiety and depression. Front. Psychiatry 15:1384298. doi: 10.3389/fpsyt.2024.1384298
Tomasi, D., and Volkow, N. D. (2021). Associations of family income with cognition and brain structure in usa children: prevention implications. Mol. Psychiatry 26, 6619–6629. doi: 10.1038/s41380-021-01130-0
Tomasi, D., and Volkow, N. D. (2023). Effects of family income on brain functional connectivity in us children: associations with cognition. Mol. Psychiatry 28, 4195–4202. doi: 10.1038/s41380-023-02222-9
Van Essen, D. C., Ugurbil, K., Auerbach, E., Barch, D., Behrens, T. E., Bucholz, R., et al. (2012). The human connectome project: a data acquisition perspective. Neuroimage 62, 2222–2231. doi: 10.1016/j.neuroimage.2012.02.018
Whitford, T. J., Rennie, C. J., Grieve, S. M., Clark, C. R., Gordon, E., and Williams, L. M. (2007). Brain maturation in adolescence: concurrent changes in neuroanatomy and neurophysiology. Hum. Brain Mapp. 28, 228–237. doi: 10.1002/hbm.20273
Woolrich, M. W., Jbabdi, S., Patenaude, B., Chappell, M., Makni, S., Behrens, T., et al. (2009). Bayesian analysis of neuroimaging data in fsl. Neuroimage 45(1 Suppl), S173–S186. doi: 10.1016/j.neuroimage.2008.10.055
Xu, J., Liu, N., Polemiti, E., Garcia-Mondragon, L., Tang, J., Liu, X., et al. (2023). Effects of urban living environments on mental health in adults. Nat. Med. 29, 1456–1467. doi: 10.1038/s41591-023-02365-w
Xu, J., Liu, X., Li, Q., Goldblatt, R., Qin, W., Liu, F., et al. (2022). Global urbanicity is associated with brain and behaviour in young people. Nat. Hum. Behav. 6, 279–293. doi: 10.1038/s41562-021-01204-7
Xu, L., Groth, K. M., Pearlson, G., Schretlen, D. J., and Calhoun, V. D. (2009). Source-based morphometry: the use of independent component analysis to identify gray matter differences with application to schizophrenia. Hum. Brain Mapp. 30, 711–724. doi: 10.1002/hbm.20540
Keywords: adolescence, brain development, multivariate, multi-set canonical correlation analysis, structural MRI, functional MRI, environmental factors
Citation: Ray B, Jensen D, Suresh P, Thapaliya B, Sapkota R, Farahdel B, Fu Z, Chen J, Calhoun VD and Liu J (2024) Adolescent brain maturation associated with environmental factors: a multivariate analysis. Front. Neuroimaging 3:1390409. doi: 10.3389/fnimg.2024.1390409
Received: 23 February 2024; Accepted: 29 October 2024;
Published: 19 November 2024.
Edited by:
Michael Harms, Washington University in St. Louis, United StatesReviewed by:
Rachel Minke Brouwer, VU Amsterdam, NetherlandsNiousha Dehestani, Deakin University, Australia
Copyright © 2024 Ray, Jensen, Suresh, Thapaliya, Sapkota, Farahdel, Fu, Chen, Calhoun and Liu. This is an open-access article distributed under the terms of the Creative Commons Attribution License (CC BY). The use, distribution or reproduction in other forums is permitted, provided the original author(s) and the copyright owner(s) are credited and that the original publication in this journal is cited, in accordance with accepted academic practice. No use, distribution or reproduction is permitted which does not comply with these terms.
*Correspondence: Jingyu Liu, amxpdTc1QGdzdS5lZHU=