- 1BrainNet, Health and Technology District, Surrey, BC, Canada
- 2Faculty of Individualized Interdisciplinary Studies, Simon Fraser University, Burnaby, BC, Canada
- 3Faculty of Science, Simon Fraser University, Burnaby, BC, Canada
- 4Centre for Neurology Studies, HealthTech Connex, Metro Vancouver, BC, Canada
- 5Cognitive Function Development Institute, Prescott Valley, AZ, United States
- 6Djavad Mowafaghian Centre for Brain Health, Faculty of Medicine, University of British Columbia, Vancouver, BC, Canada
- 7Faculty of Applied Sciences, Simon Fraser University, Burnaby, BC, Canada
Introduction: Cognitive deficits are common in psychiatric and mental health disorders, making the assessment of cognitive function in mental health treatment an important area of research. Cognitive Function Development Therapy (CFDT) is a novel therapeutic modality designed to enhance cognitive function and regulate the autonomic nervous system through targeted exercises and activities focused on attention networks and memory systems. The therapy is tracked and based on Primary Cognitive Function (PCF) scores.
Methods: This retrospective, observational study analyzed real world data from 183 children and adults undergoing CFDT to evaluate changes in cognition over time, incorporating both cognitive performance measures and an exploratory analysis of neurophysiological function. Objective neurophysiological measures in the form of the brain vital signs framework, based in event-related potentials (ERPs), were measured in a small subset of clients to explore the frameworks use in CFDT.
Results: Our findings indicate that CFDT holds promise for improving cognitive performance, as evidenced by increased PCF scores at the group level compared to pre-treatment levels [F (5, 173) = 7.087, p < 0.001, ηp2 = 0.170]. Additionally, a weak effect of age [Spearman’s Rho range: −0.301 to −0.340, p < 0.001] was found to influence the degree of cognitive improvement, suggesting the importance of early intervention for maximizing cognitive gains. The exploratory analysis suggested that CFDT may affect neurophysiological measures of information processing, particularly in basic attention, as reflected in increased amplitude in P300 measures.
Discussion: While these initial findings are encouraging, caution is warranted due to the retrospective nature of the study, though overall, the results suggest a positive impact of CFDT on cognitive function.
Introduction
Cognitive training aims to drive neuroplastic changes in neural systems, which can improve cognitive processes related to emotion regulation and clinical symptoms across various neuropsychiatric disorders (Haas et al., 2021; Thomas et al., 2018; Hochberger et al., 2019; Vinogradov et al., 2012). Unfortunately, traditional therapeutic modalities that address distorted cognitions, focus on emotional expression, or expose individuals to traumatic memories, often fail to modify autonomic dysregulation in response to present day experience (Fisher, 2019). Moreover, where the patient’s presenting symptomology is neurologically based, attempting to treat dysregulation through traditional talk-therapy approaches may elicit an increase in dysregulation rather than a resolution of the distress (McRae et al., 2012). The promise of cognitive training for mental health conditions lies in its ability to enhance cognition and community functioning, although more research is needed to understand its mechanisms and real-world applicability (Keshavan et al., 2014).
Cognitive Function Development Therapy (CFDT)—is a nonpharmaceutical, non-invasive treatment option for individuals aged 6 and above with a wide range of presenting symptoms (including, but not limited to anxiety, attentional problems, depression, psychosis, self-control challenges, and trauma-related conditions). Treatment decisions and outcome expectations of CFDT are based on objective assessment results mapped to five Primary Cognitive Functions (PCFs). These five PCFs include: Attentional Alerting, Attentional Orienting, Attentional Executive, Working Memory, and Encoded Memory (further explained in methods and Supplementary Data 1). Treatment is indirect (e.g., it does not seek to discover and ameliorate “root causes” of distress), thereby reducing the risk of treatment-related trauma or re-traumatization in clients. Rather, CFDT works by presenting targeted, interactive activities that engage and develop the client’s attention system, working memory, and encoded memory, reflected in PCF scores.
Cognitive performance can be improved through targeted training (Mandolesi et al., 2018; Thillier et al., 2023) and has been shown to have an effect on neurophysiological measures (Kariofillis et al., 2014). Therefore, it is important to explore the effects of novel cognitive training programs on neurophysiological measures. The brain vital sign framework (Hajra et al., 2016) utilizes portable and accessible electroencephalography (EEG) to extract well-established event-related potentials (ERPs) as objective neurophysiological indicators of cognitive information processing (Gawryluk and D’Arcy, 2010; Luck, 2014). Recent work has begun to use brain vital signs to track cognitive changes during rehabilitation (Fickling et al., 2020; Kirby et al., 2023), as well as attention training differences in healthy individuals (Smith et al., 2020). The brain vital signs framework elicits and records the N100, P300, and N400 in a portable, rapid and automated device that can be readily integrated at point-of-care (i.e., the NeuroCatch® Platform). Response latency (speed) and amplitude (size) are recorded for each of these three components. Slower and modulated responses, such as those for severe cognitive impairment in dementia (Ighalo et al., 2024) or after brain injury (Fickling et al., 2019b, 2021b; Fickling et al., 2021a), have been shown in previous work. In relation to mental health, the P300 attention related ERP component (Sutton et al., 1967) is commonly studied (Boudarene and Timsit-Berthier, 1997; Araki et al., 2005; Felmingham et al., 2002) and has been previously associated with changes in post-traumatic stress disorder (PTSD) related symptoms in a case study using the brain vital signs framework (Fickling et al., 2020).
The primary objective of this retrospective, observational study was to describe cognitive changes in a sample of children and adults undergoing CFDT and explore potential factors contributing to variation in cognitive changes. Our primary hypothesis was that individuals who have undergone CFDT would show improved cognitive measures as determined by analysis of the primary cognitive functions (PCFs). The secondary exploratory objective of this study was to evaluate changes in brain vital signs measures over time in a subset of program participants, to investigate the neurophysiological effect of CFDT. We anticipated that individuals would exhibit modulation of the P300 component over time in response to cognitive training.
Methods
Experimental paradigm
Real world, de-identified data from 183 clients (Figure 1; demographics in Tables 1, 2) taking part in CFDT at a community mental health clinic (Polara Health, AZ, USA; CFD Services, AZ, USA) from 12/26/2019 through 5/13/2024 and overseen by the Cognitive Function Development Institute (CFDI), a nonprofit organization who developed CFDT, were included in the overall analysis. Clients selected for inclusion in the study were referred to CFDT (clinicians, school counselors, pediatricians, nurse practitioners, other clients, etc.) due to treatment resistance with traditional modalities (e.g., talk therapy) and neurological dysregulation (e.g., threat-related behavior without an observable, external environment threat present). For clinical intake purposes, client diagnoses include anxiety disorder, attention deficit hyperactivity disorder / attention deficit disorder, adjustment disorder, autism spectrum disorder, alcohol use disorder, bipolar disorder, developmental delay, disruptive mood dysregulation disorder, generalized anxiety disorder, impulse control disorder, major depressive disorder, obsessive-compulsive disorder, oppositional defiance disorder, opioid use disorder, persistent depressive disorder, PTSD, reactive attachment disorder, social anxiety disorder, traumatic brain injury, schizophrenia, and schizoaffective disorder. Note: clients may have multiple diagnoses. However, traditional diagnoses are not considered for CFDT purposes. Instead, CFDT treatment protocols are based on the most recent PCF assessment scores. Typical CFD therapy targets the two lowest PCFs with dynamically variable interactive engagements. For example, the therapist may present a pair of integers the client must add. Subsequently, the therapist repeatedly presents an integer and requires the client to add the therapist’s previous and currently presented numbers. If the client can do so, the therapist may simultaneously require the client to identify patterns of images in a densely populated, widely spaced visual field. The presented activities are increased in intensity incrementally until the client demonstrates fatigue, at which point the therapist will reset requirements for client responses. The pattern of fatigue and reset may be repeated two or three times in a typical session, often interlaced with a demonstration of client enjoyment without an easily identifiable reason. Each session is 1 h in duration. Client data were included in the analysis if the client had completed the same cognitive assessment (BFx or Creyos) before and after CFDT and if they received a minimum of 1,440 min of treatment, denoted as “Dosing” in the results (mean therapy duration between assessments [standard deviation]: 250.0 [135.2] days; range: 98–761 days). Final assessment results were taken from the client’s final cognitive assessment after completing CFDT (within 14 days of their final session). In addition, CFDI initiated a search for an objective neurophysiological assessment tool, and, recently started incorporating NeuroCatch as an exploratory tool. Therefore, data from a subset of 18 of these clients (demographics in Table 1) had been evaluated with NeuroCatch at two timepoints (mean therapy duration between scans [standard deviation]: 203.7 [93.0] days; range: 49–388 days).
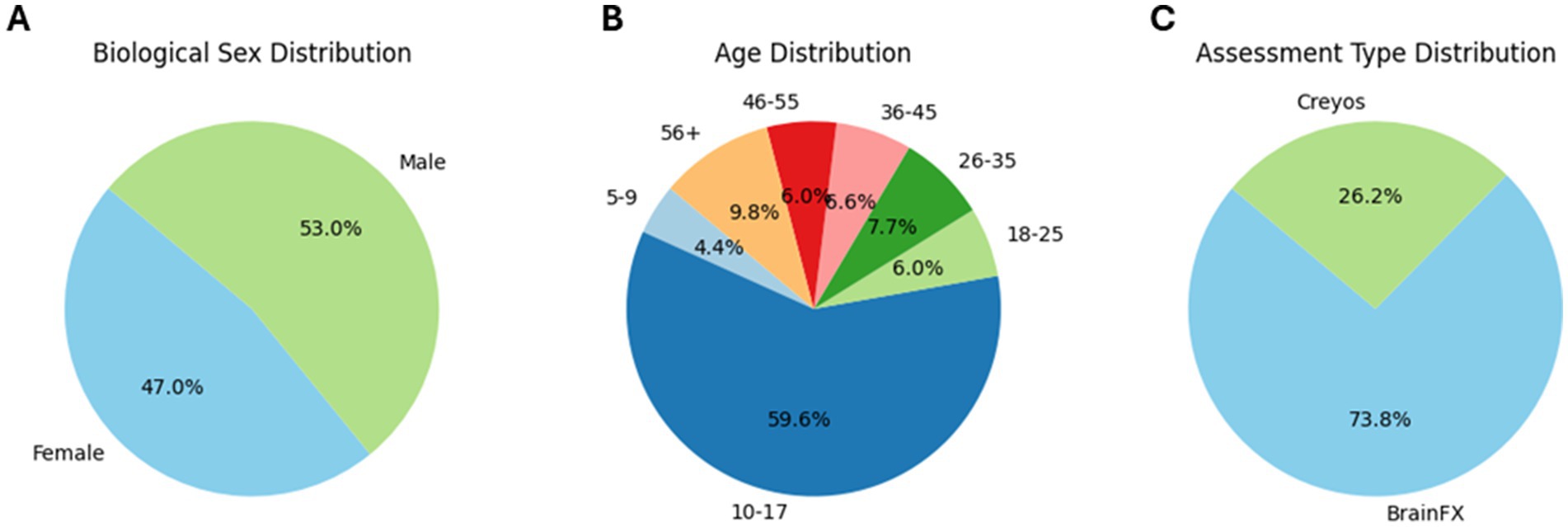
Figure 1. Pie chart of participant demographics: distribution of biological sex (A), distribution of age bins (B), and distribution of assessment type (C).
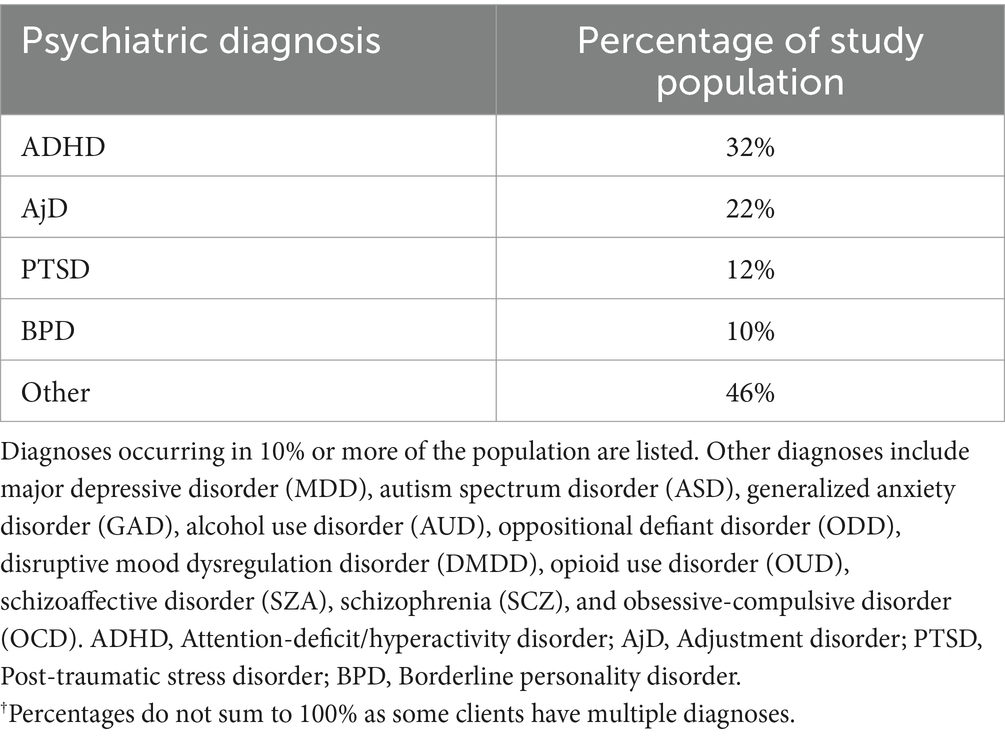
Table 2. Psychiatric diagnoses of study population.†
Primary cognitive function scores
The 5 PCFs used in the CFDT framework are: Attentional Alerting (Callejas et al., 2004; Ex. notice (and name) things in the external or internal environment), Attentional Orienting (Callejas et al., 2004; Ex. select certain items from a larger field of items (select all the cards with a certain feature, like colour, from a messy pile of image cards)), Attentional Executive (Callejas et al., 2004; Ex. call out the characteristics of a card in a certain order amidst a wide or cluttered visual field), Working Memory (Ex. assign a number to a color and compute the value of the images), and Encoded Memory (Ex. recall information such as, patterns shown (then recreated)). Clients were objectively assessed using either the BrainFX® SCREEN (BFx) or the Creyos Health (Creyos) digital cognitive assessments at semi-regular intervals throughout their CFDT program. BFx is a function-focused assessment tool that addresses neurofunction through a digital interface on a tablet. Based on its more comprehensive predecessor the BrainFX® 360 (Searles et al., 2019), the BFx assessment is administered by a healthcare professional in 10–15 min via a touch tablet and has 7 domain-specific tasks. Similarly, the Creyos platform is used to administer 12 online cognitive tests to children, adolescents, and adults. The assessment results were then mapped to PCF scores. PCF mapping is a custom method developed by the CFDI to consolidate multiple scores from different assessment tools into a comprehensive, universal metric. PCF scores are normalized as an adjusted T-score (mean of 0 and standard deviation of 10) and are used to guide treatment decisions and outcome expectations. The mapping process for each of these assessments to PCF scores differs due to BFx and Creyos having a different number of output results and different standardization techniques. BFx was utilized for clients included in this study from December 2019 through June 2024. The CFDI started using Creyos as its primary assessment tool for new clients as of June 2023. The mapping process for each is outlined with more information on the 5 PCFs, as well as the BFx and Creyos tasks used, in Supplementary Data 1.
EEG acquisition and processing
Brain vital signs were recorded using the NeuroCatch® Platform (Version 2.0, NeuroCatch Inc., Surrey, BC). Clients were fitted with a low-density EEG sensor cap (ANT Neuro Waveguard) with standard Ag/AgCl electrodes, and skin-electrode impedances were prepared to below 25 KOhms. Data were recorded from 3 midline electrodes (Fz, Cz, and Pz), with a ground electrode located at AFz, a reference electrode placed on the left earlobe, and a single electrooculogram (EOG) recorded from FPz. The scans took approximately 10 min in total, with brain vital sign testing for about 6 min. All clients received passive repeated auditory stimulation (ear insert headphone) of standard tones (80 dB) and random rare deviant tones (105 dB) ahead of basic spoken word pair primes that either matched or mismatched. The tones stimuli elicited the N100 and P300 and the word pair stimuli elicited the N400.
Recorded EEG traces were processed in Python. EEG data were filtered using a 0.1–20 Hz bandpass and 60 Hz notch filter. Ocular artifacts were corrected using an adaptive filter (He et al., 2004) with the EOG derived from the FPz channel. Stimulus-locked evoked epochs were extracted according to stimulus condition (i.e., standard/deviant tones, congruent/incongruent words). Epochs containing artifacts were rejected using an automated EEG signal-quality index (Fickling et al., 2019a). Artifact-free epochs were averaged for each stimulus condition to form representative ERP waveforms for each participant. The N100, P300, and N400 peaks were automatically detected and manually verified.
Statistical analysis
To assess change in PCF scores, a mixed (between-subject factors: Sex [2: female and male] and Assessment Type [2: BFx and Creyos] and within-subject factors: Timepoint [2: pre- and post-treatment]) repeated measures multivariate analysis of covariance (RM-MANCOVA; covariates: [2: Age and Dosing]) was run. As a post-hoc analysis, all PCF scores were run in separate non-parametric Wilcoxon Signed-Rank tests due to the left skewedness of data. This is a non-parametric statistical test appropriate for non-normal data comparing the same group of subjects before and after an intervention (Woolson, 2005).
To further investigate potential factors affecting the change in PCF scores from pre- to post-treatment, a non-parametric Spearman’s rank correlation was run. Similar to the use of the Wilcoxon Signed-Rank test, non-parametric statistical tests like Spearman’s rank correlation are used for non-normally distributed data. The change in PCF scores were correlated against Age to better understand the effect this measure may be having on the change in PCF scores from pre- to post-treatment. All Wilcoxon Signed-Rank and Spearman’s rank correlation resulting p-values were Bonferroni corrected (5 scores, therefore p-values for each Wilcoxon Signed-Rank and Spearman’s rank correlation run are divided by 5). As Dosing was used to filter participants, this measure was further investigated in Supplementary Data 2.
As an exploratory sub-analysis, brain vital signs results were analyzed similarly to the PCF scores. The N100, P300, and N400 peaks were automatically detected and manually verified. A mixed (between-subject factors: Sex [2: female and male] and within-subject factors: Timepoint [2: scan 1 and scan 2]) repeated measures multivariate analysis of covariance (RM-MANCOVA; covariates: [1: Age]) was run. To ensure homogeneity of variance in this small sample size, Levene’s test of equality of error variance was performed for each brain vital signs component. Additionally, a post-hoc Pearson’s correlation analysis of P300 amplitude change (scan 2 P300 amplitude—scan 1 P300 amplitude) vs. Age was run.
Results
Primary cognitive function scores
On a group level, the 5 PCF scores had a significant increase from pre- to post-treatment. Figure 2 and Table 3 shows the 5 different PCF scores from pre- to post-treatment. RM-MANCOVA results showed a multivariate effect of Timepoint [F (5, 173) = 7.087, p < 0.001, ηp2 = 0.170], Age [F (5, 173) = 2.640, p = 0.025, ηp2 = 0.071], and Assessment Type [F (5, 173) = 11.255, p < 0.001, ηp2 = 0.245]. Additionally, results showed a significant interaction between Timepoint × Age [F (5, 173) = 3.696, p = 0.003, ηp2 = 0.097]. The interaction between Timepoint × Assessment Type was not significant [F (5, 173) = 1.060, p = 0.384, ηp2 = 0.030]. Further MANCOVA results are outlined in Table 4.
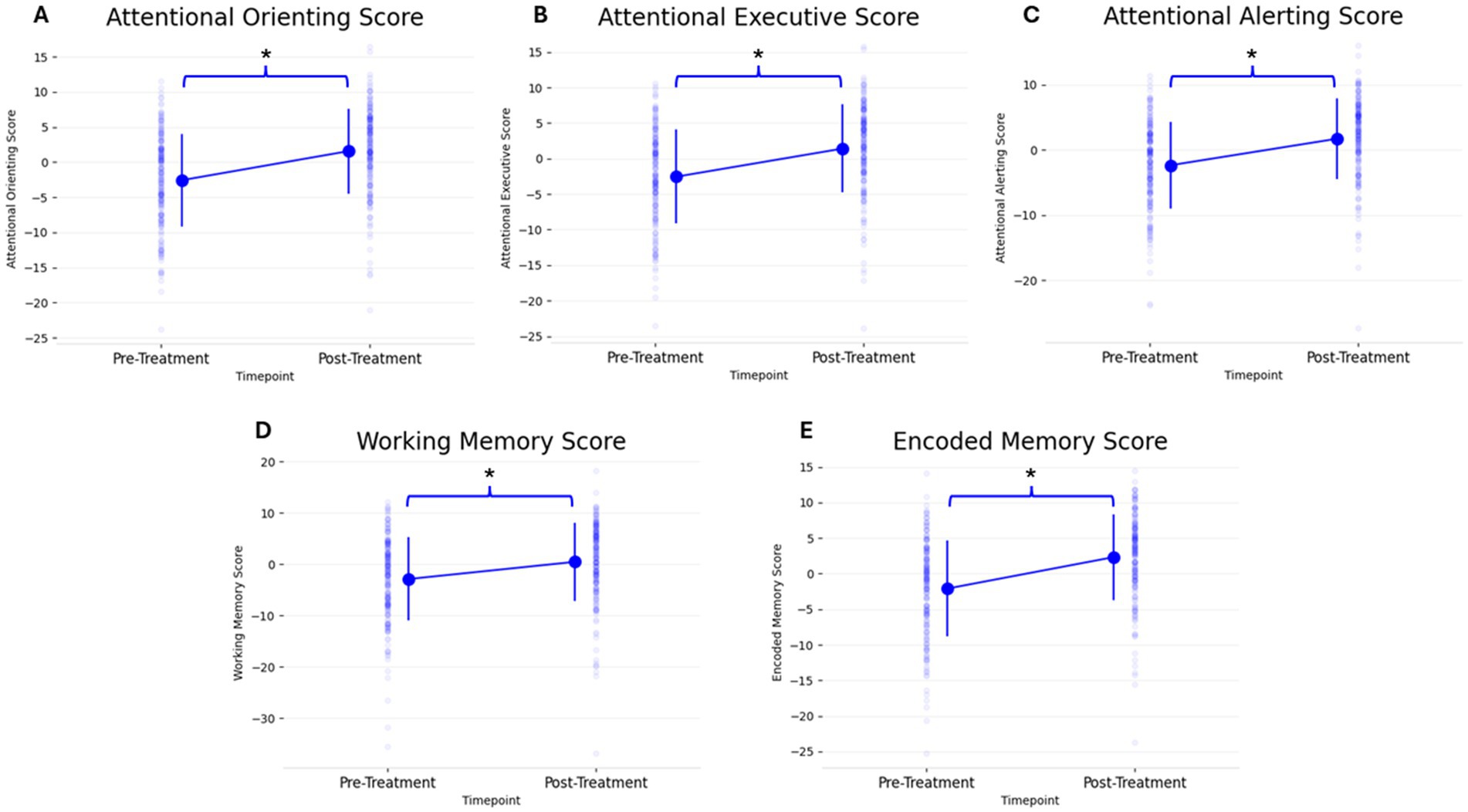
Figure 2. Change in all 5 PCF scores (A: Attentional Orienting, B: Attentional Executive, C: Attentional Alerting, D: Working Memory, E: Encoded Memory) from pre- to post-treatment. Individual scatter plots for each participant are shown faded in the background with group means denoted with large markers, including group standard deviation lines (*p < 0.05).
The PCF scores showed a left-skewed distribution, resulting in violation of a normal distribution. This is a common result in cognitive assessments (Saffari et al., 2024). Therefore, separate non-parametric Wilcoxon Signed-Rank tests were done to analyze each PCF score’s change from pre- to post-treatment. All PCF scores showed a significant change from pre- to post-treatment (Table 5).
Brain vital signs results
Brain vital signs group average waveforms at both timepoints showed a change in the P300 component (Figure 3). This change was confirmed with the RM-MANCOVA, showing a significant increase in P300 amplitude (Figure 3 and Table 6). However, no other brain vital signs components showed significant change (Table 6). Furthermore, all brain vital signs data passed Levene’s test of equality of error variances (all p > 0.05). Visually, brain vital signs waveforms seemed to have a higher group variability at scan 1 compared to scan 2 (shown by wider standard error shading in Figure 3, quantified in Supplementary Data 3).
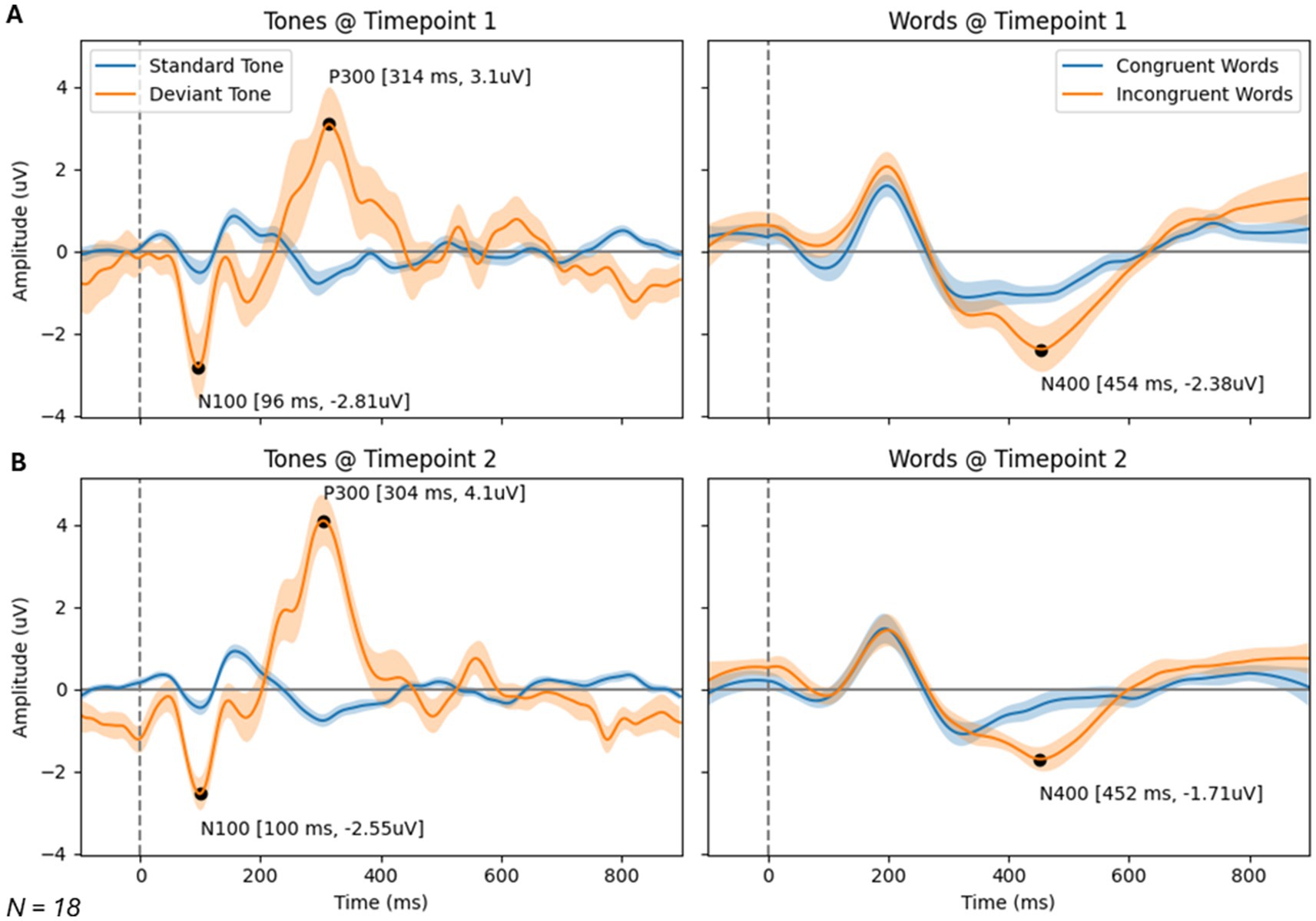
Figure 3. Brain vital signs waveform results at timepoint 1 (A) and 2 (B). Waveforms elicited by standard and deviant tones (left) and congruent and incongruent word pairs (right). N100, P300, and N400 group average peaks are denoted with black dot, as well as a latency (ms) and amplitude (uV) label. A dotted line at time = 0 ms denotes stimuli event onset.
Interestingly, the P300 amplitude showed a significant interaction of Timepoint × Age (Table 7). To further understand this, a post-hoc Pearson’s correlation between Age and P300 amplitude change showed a significant negative correlation (Pearson’s R: −0.590, p < 0.01).
Correlations
Lastly, to further investigate the age effect noted in the primary RM-MANCOVA analyzing PCF scores, Spearman’s rank correlation was investigated. Correlation results showed a significant but weak negative correlation between the change in PCF scores and Age (Table 8; Spearman’s R range: −0.301 to −0.340).
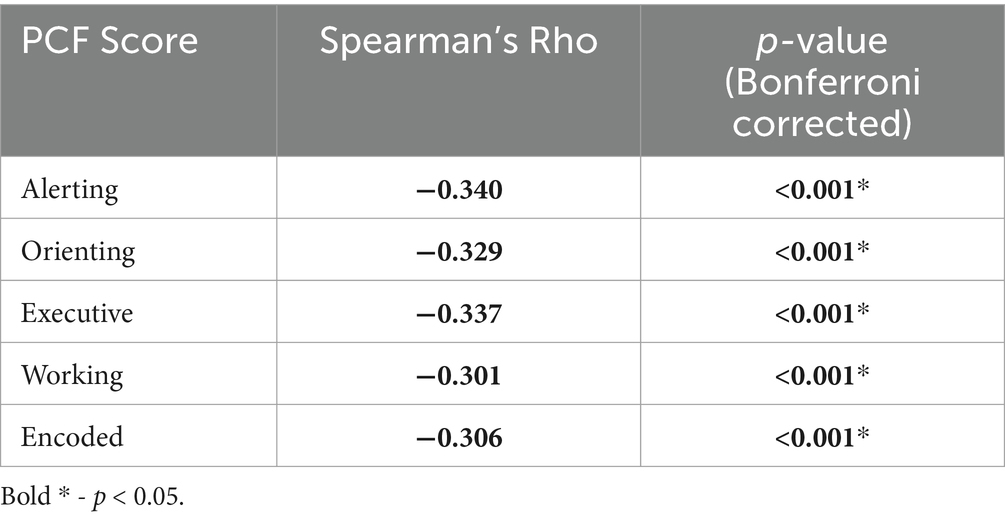
Table 8. Spearman’s correlation results and Bonferroni adjusted p-values for change in PCF scores against Age.
Discussion
The current retrospective, observational study investigated real world data of cognitive performance changes in individuals taking part in CFDT. Specifically, the current study measured cognitive performance with PCF scores, derived from BFx and Creyos assessments before and after a minimum of 1,440 min of CFDT. The results suggest that CFDT improved cognitive performance measured with PCFs. Exploratory analyses suggest that CFDT may also lead to improvements in neurophysiological measures of cognition, measured as a P300 amplitude increase with the brain vital signs framework. Lastly, the results suggest that age may be contributing to variation in cognitive change with a weak, negative relationship between age and cognitive performance, as well as neurophysiological change.
PCF scores
PCF scores, regardless of whether they were derived from BFx or Creyos assessments, improved with CFDT. Psychiatric disorders are commonly related to deficits in cognitive function, such as decreased attention, working memory, and disrupted social cognition (Trivedi, 2006; Wang Y. et al., 2022; Johnsen and Asbjørnsen, 2009; Burri et al., 2013; Kim et al., 2018). The improvement of mental health treatment seems to be a critical area of research as less than 20% of mental healthcare practitioners use or integrate measurement-based care in their practice (Lewis et al., 2018); thus, for those clients who can secure treatment, determining effectiveness of the treatment provided is dependent on highly subjective self-reported levels of patient distress rather than objective test results common with physical health care.
Digital cognitive performance assessment technology is shown to be useful in primary care settings and growing to better detect neuropsychological and cognitive deficits (Libon et al., 2023; Jannati et al., 2024; Banks et al., 2024; Brenkel et al., 2017; Levine et al., 2013). The cognitive tasks used in Creyos have been validated in several large-scale studies examining healthy controls and patient populations (Brenkel et al., 2017; Levine et al., 2013). Brenkl et al. compared cognitive impairment screening of tasks from Creyos with the commonly used Montreal Cognitive Assessment (MoCA). Authors found that the Creyos assessments provided more information that may be able to aid in differentiating levels of cognition in older adults (Brenkel et al., 2017). Cognitive testing also plays a role in cognitive rehabilitation, training, and even pharmacological development (Keefe et al., 2015; Wessels et al., 2021; Bahr et al., 2019; Preiss et al., 2013). Cognitive training offers behaviorally constrained cognitive or socio-affective learning events delivered in a scalable and reproducible manner to potentially improve neural system operations (Keshavan et al., 2014). By identifying specific areas of strength and weakness, cognitive tests can guide personalized programs aimed at improving specific cognitive functions (Preiss et al., 2013; Chen and Vinogradov, 2024). They allow for the monitoring of progress over time, providing feedback that can be used to adjust programs. It is important that cognitive training can affect other outcome variables besides cognitive function, and it implies the importance of better understanding cognitive improvements in mental health therapy (Kim et al., 2018).
Attention, encoded memory, and working memory are well-represented in the literature as individual topics of investigation. However, there exist challenges to leveraging the growing body of literature for clinical application in an integrative care setting. A form of mapping, such as with PCFs, provides an opportunity to track these cognitive functions across multiple cognitive tests (such as BFx and Creyos). It should be anticipated that networks governing a particular cognitive function (e.g., attention) will be coupled via edge interactions with networks that give rise to other cognitive functions (e.g., encoded memory; Bassett and Bullmore, 2017). It can be easily observed, for example, that cognitive functions operate on a coupled basis (Popp et al., 2024). However, the study of individual cognitive functions, as noted above, tends to obscure the possibility of such coupling, let alone interpret clinical applications for improving dysregulation. Conversely, the PCF mapping developed by the CFDI highlights the coupled nature of PCFs and provides actionable information to client-facing treatment providers regarding observable client behavior and therapeutic approaches to achieve the desired, targeted treatment. The PCF mapping method is noteworthy for two reasons. First, it provides an objective “line-in-the-sand” for each identified PCF against which improvements may be measured, as it also showed its robustness across different cognitive tests (no Timepoint*Assessment Type interaction noted). As an adjunct, therapists can readily identify functional areas to target for treatment based on the relative position of PCF scores (e.g., therapists target the two lowest functions for development). Second, graphing the obtained PCF scores produces a limited number of identifiable patterns with demonstratable behavioral explanatory or predictive ability. Therefore, demonstrating a positive change in PCF scores is an important initial step for CFDI to work towards a standardized level of evidence-based mental and cognitive healthcare.
Brain vital signs
Previously, neuroimaging research focused on mental illness showed that cognitive training is associated with both structural and functional brain changes (Subramaniam et al., 2012; Kontis et al., 2013; McClure and Bickel, 2014; Li et al., 2022; González-Alemañy et al., 2024). More specifically to EEG neuroimaging research, the P300 oddball paradigm is one of the most heavily studied ERP components and is most associated with basic attention (Sutton et al., 1967). It is an indicator of the engagement of attentional resources to the stimuli, particularly related to the awareness of an individual’s surrounding environment. In relation to mental health, research suggests that individuals with PTSD exhibit abnormalities in information processing, reflected in ERP measures correlating with illness severity (Javanbakht et al., 2011). This includes a decreased P300 amplitude in groups with PTSD relative to controls (Boudarene and Timsit-Berthier, 1997; Araki et al., 2005; Felmingham et al., 2002). In a previous case study, brain vital signs have been sensitive to changes in the P300 ERP component during physical therapy paired with neuromodulation. These increases in P300 amplitude were paired with self-reported improvements in PTSD symptoms (Fickling et al., 2020). Based on previous research, the N100 and N400 ERP components show promise as biomarkers for psychiatric disorders. The primary finding being that schizophrenic patients show reduced N100 and N400 amplitudes (Jackson et al., 2014; Wang B. et al., 2022), with N400 abnormalities not being specific to schizophrenia, but also characterizing psychosis broadly (Jackson et al., 2014). It is interesting that these responses did not show changes in this subset. As a larger number of studies tend to focus on the P300, the N100 and N400 should be areas of future research on larger groups that are more organized by their diagnoses. The current study builds on this work, replicating the effect of increasing P300 amplitude alongside cognitive training therapy.
A disruption in brain physiology is suspected in several psychiatric disorders (Millan et al., 2012; Wang Y. et al., 2022; Paunova et al., 2023), which may be reflected in an increased neural variability (Menon, 2020; Månsson et al., 2022). Using functional magnetic resonance imaging (fMRI), Månsson et al. showed that task-based brain signal variability, historically considered a source of noise, was the strongest contributor to results of a treatment outcome-based prediction model, focused on measuring improvements in social anxiety. This variability measure also showed high test–retest reliability (Månsson et al., 2022). Speculatively, the variability in event-based neural responses measured with ERPs visually seen in the waveforms in Figure 3 (quantified in Supplementary Data 3) may be a potential area of interest for future biomarkers of psychiatric disorders or for treatment of psychiatric disorders.
Correlations
Interestingly, there seems to be a significant, but weak, negative relationship between increasing age and the magnitude of change in PCF scores. This was both reflected in the significant Timepoint × Age interaction and the significant negative, but weak, Spearman’s rank correlation between each of the 5 PCF scores and age. While the ability to change and learn is kept throughout the lifespan, it is common for this ability to be somewhat lessened in older populations compared to younger populations (Pauwels et al., 2018). If the ability to improve cognitive performance is negatively associated with older age, it is critical for individuals to receive mental healthcare when problems first arise (Wass, 2015). Similar to the PCF result, there seems to be a significant relationship in the change in P300 amplitude and age. This was both reflected in the significant Timepoint × Age interaction and the significant Pearson’s correlation between the change in P300 amplitude and age. Future work may further clarify this with larger studies that are more evenly distributed across age ranges.
Future work and limitations
The present analysis was a retrospective, observational analysis conducted with a diverse (i.e., range of ages, diagnosis types and complexities, and time in program), real world data set, limiting the interpretation of results. In relation to existing cognitive assessment tools used in CFDT (BFx and Creyos), PCF mapping was not affected by which tool was used (shown in the lack of a Timepoint × Assessment Type interaction effect). However, a large main effect of Assessment Type was present on an overall PCF score level. Therefore, while it seems like the change of PCF scores during therapy is not affected by the assessment used, the scores themselves may be on different scales depending on the assessment type used. The potential effect of assessment type used for PCF mapping should be better tested with more evenly weighted groups in future work or CFDT should dominantly focus on the use of a single assessment modality.
It was important to explore the possibility that brain vital signs framework’s objective neurophysiological measurements may be modulated by CFDT, to complement results from digital cognitive assessments. As this was an exploratory sub-analysis, future work can evaluate the effects on brain vital signs changes in larger samples of individuals taking part in CFDT. Additionally, future work may further clarify the connection between P300 amplitude change with larger studies that are more evenly distributed across age ranges. This can also include a correlation between brain vital signs measure changes and PCF score change to better understand the neurophysiological and cognitive performance connection. Future work to understand the connection between neurophysiology and cognitive performance may also look into deriving cognitive workload measures from continuous EEG during cognitive tasks (Mariani et al., 2023; Jia et al., 2021; Barzon et al., 2024). As can be seen from the PCF scores, CFDT emphasizes the importance of attention on primary cognitive function with three of the five PCFs being based in attention. The P300 is mainly associated with attention, therefore the most P300 change may also have the largest baseline attentional deficits, that are targeted in CFDT. As the current dataset is heavily weighted towards children (60% of PCF scores are from 10- to 17-year-olds), a more evenly distributed age range will also be useful for further analysis of the weak correlation between age and PCF and P300 changes.
Lastly, as clients were diagnosed with a range of mental health and/or cognitive concerns (such as PTSD, attention-deficit/hyperactivity disorder, bipolar disorder, etc.) and many clients had more than one diagnosis, the current study did not include ‘diagnosis’ as a predictive variable. Focusing on a particular clinical condition in the future (e.g., only adults with PTSD) may better clarify who CFDT is best suited for. Along with this, it is difficult to attribute the changes noted specifically to CFDT. Adding quality of life data and control groups in a prospective, randomized controlled study will improve the ability to draw more concrete conclusions about the program impact.
Conclusion
The current retrospective study showed that clients taking part in CFDT for a minimum of 1,440 min of therapy improved cognitive performance as measured by PCFs. Given that mental illness is associated to cognitive deficits, it is noteworthy that the CFDT clients increased their cognitive performance during therapy. As an exploratory analysis, we also showed the feasibility and potential usefulness of an objective neurophysiological measure alongside digital cognitive performance measures. Analyses suggest that CFDT may also lead to improvements in neurophysiological measures of cognition, measured as an increase in P300 amplitude with the brain vital signs framework. Lastly, both PCF scores and P300 amplitudes showed a negative relationship between the magnitude of change and age. This may be an important aspect of therapy to investigate as it may stress the importance of seeking treatment as soon as possible to maximize the potential benefit or adjusting the dose of treatment for different ages. Overall, this retrospective, observational study is an important initial step for CFDI to work towards a standardized level of evidence-based mental and cognitive healthcare.
Data availability statement
The datasets presented in this article are not publicly available due to the sensitive nature of the information, which includes personal and health-related data of clinic clients, as well as ethical considerations and privacy agreements with the participants and their care facilities. Requests to access the datasets should be directed to Sonia Brodie, c29uaWFicm9kaWVAaGVhbHRodGVjaGNvbm5leC5jb20=.
Ethics statement
The studies involving humans were approved by Advarra (Pro00079639)—The IRB attests that this submission has been approved by an IRB whose membership complies with the requirements defined in Health Canada regulations, ICH GCP guidelines, FDA regulations at 21 CFR part 56, and HHS regulations at 45 CFR part 46. The IRB carries out its functions in accordance with FDA regulations at 21 CFR parts 50, 56, 312, and 812; HHS regulations at 45 CFR part 46, subparts A-E; good clinical practices; Health Canada regulations; and the Tri-Council Policy Statement: Ethical Conduct for Research Involving Humans, as appropriate to the research. The studies were conducted in accordance with the local legislation and institutional requirements. Written informed consent for participation was not required from the participants or the participants’ legal guardians/next of kin in accordance with the national legislation and institutional requirements.
Author contributions
EK: Conceptualization, Data curation, Formal analysis, Investigation, Visualization, Writing – original draft, Writing – review & editing. BB: Data curation, Writing – review & editing. JB: Data curation, Writing – review & editing. SB: Conceptualization, Writing – original draft, Writing – review & editing. RD’A: Supervision, Writing – review & editing.
Funding
The author(s) declare that financial support was received for the research, authorship, and/or publication of this article. The CFDI funded this study, and contracted EDK, SB, and RCND to conceptualize the study and conduct the analyses.
Acknowledgments
The CFDI would like to thank the following CFD therapists whose direct client-facing work is reflected in the study data: Elyssa Beyst, Cindy Mennenga, John Mennenga, Ehren Falk, Lorna Clark, Scott Mangels, Brenda Fox, Andrew Strachen, Ciarra Barnett, Mekenna Golden, Jennifer Pratt, Stephen Dartt, Mallory Stiles, Amber Voliva, Kathleen Butler, Clover Jacobs, Dinah Lunsford, Rachel Zachary, Shannon Isais, Brittany Keyser, Kate Delafield, and Jeannie Skousen.
Conflict of interest
BB and JB are the co-founders of the Cognitive Function Development Institute, and author RCND® is the co-founder of HealthTech Connex Inc., and the NeuroCatch Platform with financial and/or business interests that may be affected by the research reported in this paper.
The remaining authors declare that the research was conducted in the absence of any commercial or financial relationships that could be construed as a potential conflict of interest.
Generative AI statement
The author(s) declare that no Generative AI was used in the creation of this manuscript.
Publisher’s note
All claims expressed in this article are solely those of the authors and do not necessarily represent those of their affiliated organizations, or those of the publisher, the editors and the reviewers. Any product that may be evaluated in this article, or claim that may be made by its manufacturer, is not guaranteed or endorsed by the publisher.
Supplementary material
The Supplementary material for this article can be found online at: https://www.frontiersin.org/articles/10.3389/fnhum.2024.1508815/full#supplementary-material
References
Araki, T., Kasai, K., Yamasue, H., Kato, N., Kudo, N., Ohtani, T., et al. (2005). Association between lower P300 amplitude and smaller anterior cingulate cortex volume in patients with posttraumatic stress disorder: a study of victims of Tokyo Subway sarin attack. NeuroImage 25, 43–50. doi: 10.1016/j.neuroimage.2004.11.039
Bahr, R., Lopez, A., and Rey, J. A. (2019). Intranasal Esketamine (SpravatoTM) for use in treatment-resistant depression in conjunction with an Oral antidepressant. Pharm. Therap. 44, 340–375
Banks, R., Higgins, C., Greene, B. R., Jannati, A., Gomes-Osman, J., Tobyne, S., et al. (2024). Clinical classification of memory and cognitive impairment with multimodal digital biomarkers. Alzheimers Dement. 16:e12557. doi: 10.1002/dad2.12557
Barzon, G., Ambrosini, E., Vallesi, A., and Suweis, S. (2024). EEG microstate transition cost correlates with task demands. PLoS Comput. Biol. 20:e1012521. doi: 10.1371/journal.pcbi.1012521
Bassett, D. S., and Bullmore, E. T. (2017). Small-world brain networks revisited. Neuroscientist 23, 499–516. doi: 10.1177/1073858416667720
Boudarene, M., and Timsit-Berthier, M. (1997). Interest of events-related potentials in assessment of posttraumatic stress disorder. Ann. N. Y. Acad. Sci. 821, 494–498. doi: 10.1111/j.1749-6632.1997.tb48314.x
Brenkel, M., Shulman, K., Hazan, E., Herrmann, N., and Owen, A. M. (2017). Assessing capacity in the elderly: comparing the MoCA with a novel computerized battery of executive function. Dement. Geriat. Cognit. Disord. Extra 7, 249–256. doi: 10.1159/000478008
Burri, A., Maercker, A., Krammer, S., and Simmen-Janevska, K. (2013). Childhood trauma and PTSD symptoms increase the risk of cognitive impairment in a sample of former indentured child laborers in old age. PLoS One 8:e57826. doi: 10.1371/journal.pone.0057826
Callejas, A., Lupiáñez, J., and Tudela, P. (2004). The three attentional networks: on their Independence and interactions. Brain Cogn. 54, 225–227. doi: 10.1016/j.bandc.2004.02.012
Chen, C. S., and Vinogradov, S. (2024). Personalized cognitive health in psychiatry: current state and the promise of computational methods. Schizophr. Bull. 50, 1028–1038. doi: 10.1093/schbul/sbae108
Felmingham, K. L., Bryant, R. A., Kendall, C., and Gordon, E. (2002). Event-related potential dysfunction in posttraumatic stress disorder: the role of numbing. Psychiatry Res. 109, 171–179. doi: 10.1016/S0165-1781(02)00003-3
Fickling, S. D., Greene, T., Greene, D., Frehlick, Z., Campbell, N., Etheridge, T., et al. (2020). Brain vital signs detect cognitive improvements during combined physical therapy and Neuromodulation in rehabilitation from severe traumatic brain injury: a case report. Front. Hum. Neurosci. 14:347. doi: 10.3389/fnhum.2020.00347
Fickling, Shaun D., Liu, Careesa C., D’Arcy, Ryan C. N., Hajra, Sujoy Ghosh, and Song, Xiaowei. (2019a). “Good data? The EEG quality index for automated assessment of signal quality.” in 2019 IEEE 10th annual information technology, electronics and Mobile communication conference (IEMCON). pp. 0219–0229.
Fickling, S. D., Poel, D. N., Dorman, J. C., D’Arcy, R. C. N., and Munce, T. A. (2021a). Subconcussive changes in youth football players: objective evidence using brain vital signs and instrumented accelerometers. Brain Commun. 4:fcab286. doi: 10.1093/braincomms/fcab286
Fickling, S. D., Smith, A. M., Pawlowski, G., Hajra, S. G., Liu, C. C., Farrell, K., et al. (2019b). Brain vital signs detect concussion-related neurophysiological impairments in ice hockey. Brain 142, 255–262. doi: 10.1093/brain/awy317
Fickling, S. D., Smith, A. M., Stuart, M. J., Dodick, D. W., Farrell, K., Pender, S. C., et al. (2021b). Subconcussive brain vital signs changes predict head-impact exposure in ice hockey players. Brain Commun. 3:fcab019. doi: 10.1093/braincomms/fcab019
Fisher, J. (2019). Sensorimotor psychotherapy in the treatment of trauma. Pract. Innov. 4, 156–165. doi: 10.1037/pri0000096
Gawryluk, J. R., and D’Arcy, R. C. N. (2010). “Electroencephalography: basic concepts and brain applications,” in Handbook of physics in medicine and biology. ed. R. Splinter (Milton Park, UK: Taylor & Francis), 548.
González-Alemañy, E., Ostrosky, F., Lozano, A., Lujan, A., Perez, M., Castañeda, D., et al. (2024). Brain structural change associated with cognitive behavioral therapy in maltreated children. Brain Res. 1825:148702. doi: 10.1016/j.brainres.2023.148702
Haas, S. S., Antonucci, L. A., Wenzel, J., Ruef, A., Biagianti, B., Paolini, M., et al. (2021). A multivariate Neuromonitoring approach to neuroplasticity-based computerized cognitive training in recent onset psychosis. Neuropsychopharmacology 46, 828–835. doi: 10.1038/s41386-020-00877-4
Hajra, G., Sujoy, C. C., Liu, X. S., Fickling, S., Liu, L. E., Pawlowski, G., et al. (2016). Developing brain vital signs: initial framework for monitoring brain function changes over time. Front. Neurosci. 10:211. doi: 10.3389/fnins.2016.00211
He, P., Wilson, G., and Russell, C. (2004). Removal of ocular artifacts from electro-encephalogram by adaptive filtering. Med. Biol. Eng. Comput. 42, 407–412. doi: 10.1007/BF02344717
Hochberger, W. C., Joshi, Y. B., Thomas, M. L., Zhang, W., Bismark, A. W., Treichler, E. B. H., et al. (2019). Neurophysiologic measures of target engagement predict response to auditory-based cognitive training in treatment refractory schizophrenia. Neuropsychopharmacology 44, 606–612. doi: 10.1038/s41386-018-0256-9
Ighalo, J., Kirby, E. D., Song, X., Fickling, S. D., Pawlowski, G., Hajra, S. G., et al. (2024). Brain vital signs as a quantitative measure of cognition: methodological implementation in a care home environment. Heliyon 10:e28982. doi: 10.1016/j.heliyon.2024.e28982
Jackson, F., Foti, D., Kotov, R., Perlman, G., Mathalon, D. H., and Proudfit, G. H. (2014). An incongruent reality: the N400 in relation to psychosis and recovery. Schizophr. Res. 160, 208–215. doi: 10.1016/j.schres.2014.09.039
Jannati, A., Toro-Serey, C., Gomes-Osman, J., Banks, R., Ciesla, M., Showalter, J., et al. (2024). Digital clock and recall is superior to the Mini-mental state examination for the detection of mild cognitive impairment and mild dementia. Alzheimers Res. Ther. 16:2. doi: 10.1186/s13195-023-01367-7
Javanbakht, A., Liberzon, I., Amirsadri, A., Gjini, K., and Boutros, N. N. (2011). Event-related potential studies of post-traumatic stress disorder: a critical review and synthesis. Biol. Mood Anxiety Disord. 1:5. doi: 10.1186/2045-5380-1-5
Jia, W., von Wegner, F., Zhao, M., and Zeng, Y. (2021). Network oscillations imply the highest cognitive workload and lowest cognitive control during idea generation in open-ended creation tasks. Sci. Rep. 11:24277. doi: 10.1038/s41598-021-03577-1
Johnsen, G. E., and Asbjørnsen, A. E. (2009). Verbal learning and memory impairments in posttraumatic stress disorder: the role of encoding strategies. Psychiatry Res. 165, 68–77. doi: 10.1016/j.psychres.2008.01.001
Kariofillis, D., Sartory, G., Kärgel, C., and Müller, B. W. (2014). The effect of cognitive training on evoked potentials in schizophrenia. Schizophrenia Res. Cogn. 1, 180–186. doi: 10.1016/j.scog.2014.07.001
Keefe, R. S. E., Meltzer, H. A., Dgetluck, N., Gawryl, M., Koenig, G., Moebius, H. J., et al. (2015). Randomized, double-blind, placebo-controlled study of Encenicline, an Α7 nicotinic acetylcholine receptor agonist, as a treatment for cognitive impairment in schizophrenia. Neuropsychopharmacology 40, 3053–3060. doi: 10.1038/npp.2015.176
Keshavan, M. S., Vinogradov, S., Rumsey, J., Sherrill, J., and Wagner, A. (2014). Cognitive training in mental disorders: update and future directions. Am. J. Psychiatry 171, 510–522. doi: 10.1176/appi.ajp.2013.13081075
Kim, E. J., Bahk, Y.-C., Hyeonju, O., Lee, W.-H., Lee, J.-S., and Choi, K.-H. (2018). Current status of cognitive remediation for psychiatric disorders: a review. Front. Psychol. 9:461. doi: 10.3389/fpsyt.2018.00461
Kirby, E. D., Jones, C. B., Fickling, S. D., Pawlowski, G., Brodie, S. M., Boyd, L. A., et al. (2023). Real world evidence of improved attention and cognition during physical therapy paired with Neuromodulation: a brain vital signs study. Front. Hum. Neurosci. 17:1209480. doi: 10.3389/fnhum.2023.1209480
Kontis, D., Huddy, V., Reeder, C., Landau, S., and Wykes, T. (2013). Effects of age and cognitive reserve on cognitive remediation therapy outcome in patients with schizophrenia. Am. J. Geriatr. Psychiatry 21, 218–230. doi: 10.1016/j.jagp.2012.12.013
Levine, B., Bacopulos, A., Anderson, N.D., Black, S.E., Davidson, P.S.R., Fitneva, S.A., et al. (2013). “Validation of a novel computerized test battery for automated testing.” in Conference: Canadian Stroke Congress. pp. E196–E196.
Lewis, C. C., Boyd, M., Puspitasari, A., Navarro, E., Howard, J., Kassab, H., et al. (2018). Implementing measurement-based Care in Behavioral Health: a review. JAMA Psychiatry 76:324. doi: 10.1001/jamapsychiatry.2018.3329
Li, J. Y., Huiqin, W., Yuan, S., Wang, C., Wang, Q., Zhong, Y., et al. (2022). A Meta-analysis on neural changes of cognitive training for mental disorders in executive function tasks: increase or decrease brain activation? BMC Psychiatry 22:155. doi: 10.1186/s12888-022-03796-4
Libon, D. J., Matusz, E. F., Cosentino, S., Price, C. C., Swenson, R., Vermeulen, M., et al. (2023). Using digital assessment technology to detect neuropsychological problems in primary care settings. Front. Psychol. 14:1280593. doi: 10.3389/fpsyg.2023.1280593
Luck, S. J. (2014). An introduction to the event-related potential technique. Second Edn. Cambridge, MA, USA: MIT Press.
Mandolesi, L., Polverino, A., Montuori, S., Foti, F., Ferraioli, G., Sorrentino, P., et al. (2018). Effects of physical exercise on cognitive functioning and wellbeing: biological and psychological benefits. Front. Psychol. 9:509. doi: 10.3389/fpsyg.2018.00509
Månsson, K. N. T., Waschke, L., Manzouri, A., Furmark, T., Fischer, H., and Garrett, D. D. (2022). Moment-to-moment brain signal variability reliably predicts psychiatric treatment outcome. Biol. Psychiatry 91, 658–666. doi: 10.1016/j.biopsych.2021.09.026
Mariani, B., Nicoletti, G., Barzon, G., Barajas, M. C. O., Shukla, M., Guevara, R., et al. (2023). Prenatal experience with language shapes the brain. Sci. Adv. 9:eadj3524. doi: 10.1126/sciadv.adj3524
McClure, S. M., and Bickel, W. K. (2014). A dual-systems perspective on addiction: contributions from neuroimaging and cognitive training. Ann. N. Y. Acad. Sci. 1327, 62–78. doi: 10.1111/nyas.12561
McRae, K., Misra, S., Prasad, A. K., Pereira, S. C., and Gross, J. J. (2012). Bottom-up and top-down emotion generation: implications for emotion regulation. Soc. Cogn. Affect. Neurosci. 7, 253–262. doi: 10.1093/scan/nsq103
Menon, V. (2020). Brain networks and cognitive impairment in psychiatric disorders. World Psychiatry 19, 309–310. doi: 10.1002/wps.20799
Millan, M. J., Agid, Y., Brüne, M., Bullmore, E. T., Carter, C. S., Clayton, N. S., et al. (2012). Cognitive dysfunction in psychiatric disorders: characteristics, causes and the quest for improved therapy. Nat. Rev. Drug Discov. 11, 141–168. doi: 10.1038/nrd3628
Paunova, R. K., Stoyanov, D., Ramponi, C., Latypova, A., and Kherif, F. (2023). Disrupted structural brain networks across psychiatric disorders determined using multivariate graph analyses. Eur. Psychiatry 66, S295–S296. doi: 10.1192/j.eurpsy.2023.660
Pauwels, L., Chalavi, S., and Swinnen, S. P. (2018). Aging and brain plasticity. Aging (Albany NY) 10, 1789–1790. doi: 10.18632/aging.101514
Popp, J. L., Thiele, J. A., Faskowitz, J., Seguin, C., Sporns, O., and Hilger, K. (2024). Structural-functional brain network coupling predicts human cognitive ability. NeuroImage 290:120563. doi: 10.1016/j.neuroimage.2024.120563
Preiss, M., Shatil, E., Cermakova, R., Cimermannova, D., and Flesher, I. (2013). Personalized cognitive training in unipolar and bipolar disorder: a study of cognitive functioning. Front. Hum. Neurosci. 7:108. doi: 10.3389/fnhum.2013.00108
Saffari, S. E., Soo, S. A., Mohammadi, R., Ng, K. P., Greene, W., and Kandiah, N. (2024). Modelling the distribution of cognitive outcomes for early-stage neurocognitive disorders: a model comparison approach. Biomedicines 12:393. doi: 10.3390/biomedicines12020393
Searles, C., Farnsworth, J. L., Jubenville, C., Kang, M., and Ragan, B. (2019). Test–retest reliability of the BrainFx 360® performance assessment. Athl. Train. Sports Health Care 11, 183–191. doi: 10.3928/01913913-20181005-01
Smith, C. J., Livingstone, A., Fickling, S. D., Tannouri, P., Campbell, N. K. J., Lakhani, B., et al. (2020). Brain vital signs detect information processing differences when Neuromodulation is used during cognitive skills training. Front. Hum. Neurosci. 14:358. doi: 10.3389/fnhum.2020.00358
Subramaniam, K., Luks, T. L., Fisher, M., Simpson, G. V., Nagarajan, S., and Vinogradov, S. (2012). Computerized cognitive training restores neural activity within the reality monitoring network in schizophrenia. Neuron 73, 842–853. doi: 10.1016/j.neuron.2011.12.024
Sutton, S., Tueting, P., Zubin, J., and John, E. R. (1967). Information delivery and the sensory evoked potential. Science 155, 1436–1439. doi: 10.1126/science.155.3768.1436
Thillier, C., Besombes, N., Agbangla, N. F., and Vitiello, D. (2023). The effects of different types of training on cognitive performance in professional Esports players. J. Elect. Gaming Esports 1:22. doi: 10.1123/jege.2023-0022
Thomas, M. L., Bismark, A. W., Joshi, Y. B., Tarasenko, M., Treichler, E. B. H., Hochberger, W. C., et al. (2018). Targeted cognitive training improves auditory and verbal outcomes among treatment refractory schizophrenia patients mandated to residential care. Schizophr. Res. 202, 378–384. doi: 10.1016/j.schres.2018.07.025
Trivedi, J. K. (2006). Cognitive deficits in psychiatric disorders: current status. Indian J. Psychiatry 48, 10–20. doi: 10.4103/0019-5545.31613
Vinogradov, S., Fisher, M., and de Villers-Sidani, E. (2012). Cognitive training for impaired neural Systems in Neuropsychiatric Illness. Neuropsychopharmacology 37, 43–76. doi: 10.1038/npp.2011.251
Wang, Y., Meng, W., Liu, Z., An, Q., and Xingbin, H. (2022). Cognitive impairment in psychiatric diseases: biomarkers of diagnosis, treatment, and prevention. Front. Cell. Neurosci. 16:1046692. doi: 10.3389/fncel.2022.1046692
Wang, B., Zartaloudi, E., Linden, J. F., and Bramon, E. (2022). Neurophysiology in psychosis: the quest for disease biomarkers. Transl. Psychiatry 12, 1–10. doi: 10.1038/s41398-022-01860-x
Wass, S. V. (2015). Applying cognitive training to target executive functions during early development. Child Neuropsychol. 21, 150–166. doi: 10.1080/09297049.2014.882888
Wessels, A. M., Edgar, C. J., Nathan, P. J., Siemers, E. R., Maruff, P., and Harrison, J. (2021). Cognitive go/no-go decision-making criteria in Alzheimer’s disease drug development. Drug Discov. Today 26, 1330–1336. doi: 10.1016/j.drudis.2021.01.012
Keywords: primary cognitive functions, cognitive training, cognitive testing, EEG, ERPs, brain vital signs
Citation: Kirby ED, Beyst B, Beyst J, Brodie SM and D’Arcy RCN (2024) A retrospective, observational study of real-world clinical data from the Cognitive Function Development Therapy program. Front. Hum. Neurosci. 18:1508815. doi: 10.3389/fnhum.2024.1508815
Edited by:
Daniele Corbo, University of Brescia, ItalyReviewed by:
Giacomo Barzon, University of Padua, ItalyFelix Adochiei, Polytechnic University of Bucharest, Romania
Copyright © 2024 Kirby, Beyst, Beyst, Brodie and D’Arcy. This is an open-access article distributed under the terms of the Creative Commons Attribution License (CC BY). The use, distribution or reproduction in other forums is permitted, provided the original author(s) and the copyright owner(s) are credited and that the original publication in this journal is cited, in accordance with accepted academic practice. No use, distribution or reproduction is permitted which does not comply with these terms.
*Correspondence: Ryan C. N. D’Arcy, cnlhbkBoZWFsdGh0ZWNoY29ubmV4LmNvbQ==