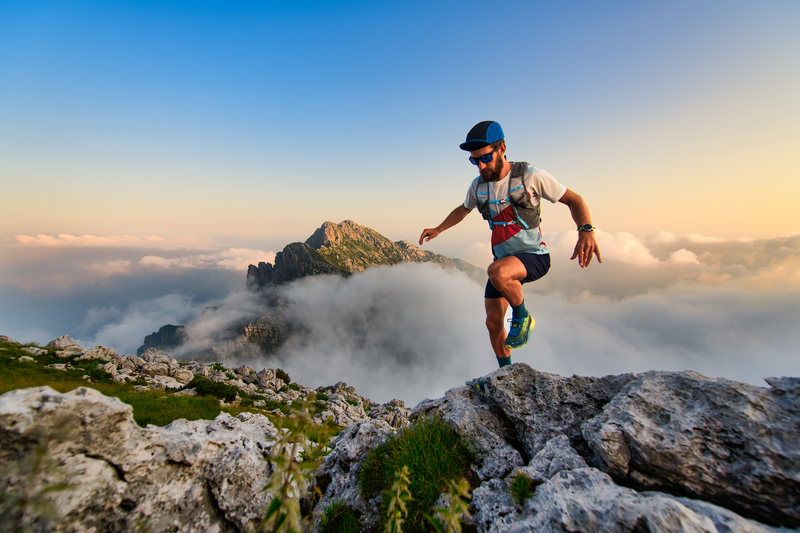
94% of researchers rate our articles as excellent or good
Learn more about the work of our research integrity team to safeguard the quality of each article we publish.
Find out more
REVIEW article
Front. Neurol. , 07 February 2025
Sec. Dementia and Neurodegenerative Diseases
Volume 16 - 2025 | https://doi.org/10.3389/fneur.2025.1496711
This article is part of the Research Topic Post-Stroke Cognitive Decline and Dementia: Unraveling Mechanisms, Models, and Biomarkers View all 7 articles
Vascular cognitive impairment (VCI) is increasingly recognized as the second most prevalent cause of dementia, primarily attributed to vascular risk factors and cerebrovascular disease. Numerous studies suggest that blood biomarkers may play a crucial role in the detection and prognosis of VCI. This study conducted a meta-analysis to evaluate the potential of various blood biomarkers associated with neuronal function as indicators of VCI. We searched four major databases—PubMed, Embase, Web of Science, and the Cochrane Library—up to December 31, 2023, for research on blood biomarkers for VCI. Of the 4,043 studies identified, 30 met the inclusion criteria for this review. The nine peripheral biomarkers analyzed for their association with neuronal function include amyloid beta 42 (Aβ42), amyloid beta 40 (Aβ40), Aβ42/Aβ40 ratio, total Tau (t-Tau), phosphorylated tau 181 (p-tau 181), neurofilament light (NfL), brain-derived neurotrophic factor (BDNF), S100B, and soluble receptor for advanced glycation end products (sRAGE). Our findings reveal that peripheral Aβ42, Aβ42/Aβ40 ratio, NfL, and S100B significantly differ between VCI and non-VCI groups, indicating their potential as blood biomarkers for VCI.
Vascular cognitive impairment (VCI) refers to cognitive deficits associated with cerebrovascular diseases, encompassing a broad range of conditions from vascular mild cognitive impairment to vascular dementia (VaD) (1). It is the second most common cause of dementia after Alzheimer’s disease (AD), accounting for at least 20% of all dementia cases (2). Worldwide, more than 57 million people are affected by dementia, a number projected to exceed 150 million by 2050 (3). Early diagnosis of VCI presents significant challenges, increasing the risk of disability and mortality, which places a substantial burden on both families and society (4, 5). The International Vascular Impairment of Cognition Classification Consensus Study (VICCCS) classifies VCI into mild and severe forms. Severe VCI includes post-stroke dementia, subcortical ischemic vascular dementia, multi-infarct dementia, and mixed dementia (where vascular and neurodegenerative lesions coexist) (6). Clinically, VCI is characterized by attention deficits, impaired information processing, difficulties with complex tasks, and disruptions in thinking and behavior (7). In some cases, patients may also experience mood disorders, such as vascular depression (8, 9).
Cerebral small vessel disease (cSVD) is the predominant pathological foundation of VCI (10). cSVD is categorized into six types, with Type I (arteriosclerosis) and Type II (cerebral amyloid angiopathy) being the most prevalent (11, 12). Emerging evidence indicates that the pathological mechanisms of cSVD involve hypoperfusion/hypoxia, blood–brain barrier (BBB) dysfunction, interstitial fluid (ISF)/cerebrospinal fluid (CSF) drainage obstruction, and vascular inflammation (10). On magnetic resonance imaging (MRI), cSVD typically manifests as white matter hyperintensities (WMHs), cerebral microbleeds (CMBs), subcortical infarcts, lacunes, perivascular space enlargement, and brain atrophy (13). WMH, also known as leukoaraiosis (LA), represents the most common neuroimaging feature of cSVD (14, 15). LA corresponds to specific abnormalities in the white matter, often characterized by multifocal or diffuse changes of varying sizes, primarily located around the ventricles (16). Numerous studies have shown that LA is increasingly prevalent in older adults, with approximately 90% of individuals over 60 exhibiting detectable signs (12). LA has been reported to be closely associated with an elevated risk of cognitive dysfunction, motor gait impairment, stroke, dementia, depression, and even mortality (15, 17–19). The Fazekas scale, based on MRI, is widely utilized in the clinical assessment of LA severity (20). Previous studies, such as the Leukoaraiosis and Disability (LADIS) study, have demonstrated that the severity of LA and the presence of diabetes are independent predictors of cognitive decline in initially non-disabled elderly individuals (21). Another LADIS study suggested that physical activity may reduce the risk of cognitive impairment (primarily VaD) in elderly individuals capable of living independently (22). Consequently, LA is intimately linked to cognitive function (23).
The diagnosis of VCI involves clinical evaluation, neuropsychological testing, and neuroimaging (24). The Montreal Cognitive Assessment (MoCA) and the Mini-Mental State Examination (MMSE) are the most commonly employed neuropsychological tools (25). However, these assessments are influenced by the patient’s age and educational background, and the evaluator’s subjectivity can also affect the accuracy of the results. Additionally, neuroimaging relies on high-quality scans and skilled radiologists, and its high cost limits its widespread clinical application. In contrast, blood biomarkers provide benefits such as accessibility, objectivity, minimal invasiveness, and low testing costs (26). As a result, many studies indicate that blood biomarkers are crucial in diagnosing VCI (24, 27). With the advancement of ultrasensitive technologies, such as single-molecule arrays and electrochemiluminescence analysis, concentrations below femtomolar levels can now be detected, enabling highly sensitive measurements of brain-derived proteins at low concentrations (26). Previous studies have shown that patients with VCI who are amyloid-positive experience a more rapid decline in cognitive function across multiple domains compared to amyloid-negative VCI patients, suggesting that VCI may also involve the pathological mechanisms underlying neurodegenerative diseases (28). Moreover, recent research has identified circulating biomarkers that influence neuronal function, including β-amyloid 42 (Aβ42), phosphorylated tau 181 (p-tau181), neurofilament light (NfL), and S100B, as being associated with VCI (29–32). Therefore, this paper aims to evaluate the potential of circulating biomarkers in predicting VCI, focusing on neuronal function, through a systematic review and meta-analysis, thereby providing some assistance for the early diagnosis and treatment of VCI.
The following keywords were combined for this study: (dementia OR cognitive impairment OR cognitive decline OR cognitive disorder OR cognitive dysfunction OR cognitive deficit) AND (vascular OR strokes OR stroke OR cerebrovascular accident OR brain vascular accident OR apoplexy OR cerebral infarction OR brain infarction OR brain hemorrhage OR cerebral hemorrhage OR hemorrhage) AND (biomarkers OR biomarker OR serum OR plasma OR circulation OR circulating OR peripheral OR whole blood). Four major databases, PubMed, Embase, Web of Science, and Cochrane Library, were searched for all research up until December 31, 2023.
The following were the inclusion criteria for the studies in this systematic review: (1) all biomarkers were derived from blood, including whole blood, serum, and plasma; (2) the study type was case–control, cross-sectional, or cohort; (3) the patients had a confirmed diagnosis of VCI; and (4) the samples included both the VCI group and the control group. The following were the exclusion criteria: (1) reviews, meta-analyses, and systematic reviews; (2) case reports and conference abstracts; (3) animal experimentation studies; (4) intervention experiments; (5) papers written in languages other than English; and (6) lack of access to full text or study data.
Data extraction was conducted independently by two researchers (W.H. and L.L.). The collected data comprised the first author’s surname, the publication year, the country of publication, the study type, the gender ratio, the mean age, the sample size, the diagnostic method for VCI, the sample source, and the biomarkers involved. For biomarkers identified in two or more articles, the mean and standard deviation (SD) of concentrations and the sample size for each group were extracted. Standard methods were used to estimate means and SDs when biomarker concentrations were reported in alternative formats, such as median or interquartile range (33, 34). Any conflicts during the process were resolved through continuous discussions involving all authors.
The quality of the studies included in our review was evaluated using the Newcastle-Ottawa scale. All the studies had scores ranging from 7 to 9, suggesting good quality. This information can be found in Supplementary Table S1. Furthermore, we have previously registered the systematic review program in PROSPERO (registration number CRD42024568815).
The data were analyzed using RevMan 5.4 software. To account for variations in the assays used across different studies, the standardized mean difference (SMD) was selected as the effect size for comparing biomarker levels, along with the corresponding 95% confidence intervals (CIs). Heterogeneity among the included studies was assessed and quantified using the Cochrane Q test and I2 statistic. A random-effects model was applied when I2 exceeded 50%, indicating substantial heterogeneity. Conversely, a fixed-effects model was employed when heterogeneity was deemed insignificant (I2 < 50%). Statistical significance was defined as a p-value ≤0.05.
6,812 studies were identified from various databases: 1,719 from PubMed, 2,179 from Embase, 2,545 from Web of Science, 358 from the Cochrane Library, and 11 from other sources. After removing duplicates, the dataset was reduced to 4,043 studies. After reviewing titles and abstracts, 281 studies were selected for further consideration. Ultimately, 30 articles were included in the final analysis after a comprehensive full-text review (Figure 1).
Table 1 presents data from research on potential blood biomarkers for VCI. The 30 included articles, published between 2005 and 2023, comprise 10 case–control studies (35–44), 11 cohort studies (29–32, 45–51), and 9 cross-sectional studies (52–60). The studies involved participants from Germany, China, Turkey, Singapore, Italy, and Sweden, with sample sizes ranging from 55 to 5,323. Participants were categorized by gender, and the average age was documented. Each study also specified the type and diagnostic criteria of VCI in patients. Furthermore, the type of blood sample (serum or plasma) and the associated blood biomarkers were recorded.
Figure 2 presents the results of a meta-analysis conducted on potential blood biomarkers for VCI that were commonly identified across two or more studies. The analysis incorporated nine biomarkers: Aβ42, Aβ40, Aβ42/Aβ40 ratio, total Tau (t-Tau), p-tau 181, NfL, brain-derived neurotrophic factor (BDNF), S100B, and soluble receptor for advanced glycation end products (sRAGE).
Figure 2. Forest plots for potential biomarkers. Forest plots of the (A) Aβ42, (B) Aβ40, (C) Aβ42/Aβ40, (D) t-Tau, (E) p-tau 181, (F) NfL, (G) BDNF, (H) S100B, (I) sRAGE levels.
As depicted in Figure 2A, we performed a meta-analysis of six studies examining peripheral Aβ42 levels involving 196 VCI patients and 332 non-VCI patients. The results demonstrated that Aβ42 levels were significantly lower in the VCI group compared to the control group (SMD = −0.42, 95% CI = (−0.83, 0.00), p = 0.05). Figure 3A illustrates that the funnel plot was approximately symmetrical, indicating an absence of significant publication bias. A subgroup analysis based on VCI subtypes revealed no significant differences in peripheral Aβ42 levels between the VaD and post-stroke cognitive impairment (PSCI) groups compared to the control group. The overall difference between subgroups was not statistically significant (p = 0.38), as shown in Figure 4A.
We conducted a meta-analysis of two studies assessing peripheral Aβ42/Aβ40 ratios, including 53 VCI patients and 138 non-VCI patients. As presented in Figure 2C, the results indicated that the Aβ42/Aβ40 ratio was significantly lower in the VCI group relative to the control group (SMD = −0.33, 95% CI = (−0.65,-0.01), p = 0.04). The funnel plot, depicted in Figure 3B, suggested no significant publication bias. Given the limited number of studies, further subgroup analysis was not performed.
We conducted a meta-analysis of five studies examining peripheral NfL levels involving 1,325 VCI patients and 1,256 non-VCI patients. As shown in Figure 2F, the meta-analysis results indicate that NfL levels in the VCI group were significantly higher than those in the control group (SMD = 1.41, 95% CI = (0.90, 1.91), p < 0.00001). The funnel plot in Figure 3C displayed poor symmetry, suggesting a certain degree of publication bias. Subgroup analysis based on VCI subtypes revealed that both the VaD group (SMD = 2.01, 95% CI = (0.46, 3.56), p = 0.01) and the PSCI group (SMD = 0.99, 95% CI = (0.84, 1.15), p < 0.00001) had significantly elevated peripheral NfL levels compared to the control group, while the overall subgroup difference was not statistically significant (p = 0.20) (Figure 4B).
We also conducted a meta-analysis of three studies on peripheral S100B levels involving 341 VCI patients and 404 non-VCI patients. The results showed that S100B levels in the VCI group were significantly higher than those in the control group (SMD = 2.64, 95% CI = (0.29, 4.99), p = 0.03) (Figure 2H). Additionally, the funnel plot in Figure 3D indicated no significant publication bias. Due to the limited number of studies, no further subgroup analysis was performed.
As illustrated in Figures 2B,D,E,G,I, there were no significant differences between the two groups in terms of Aβ40 (SMD = 0.16, 95% CI = (−0.13, 0.45), p = 0.28), t-Tau (SMD = −0.20, 95% CI = (−0.73, 0.34), p = 0.48), p-tau181 (SMD = 0.11, 95% CI = (−0.63, 0.85), p = 0.76), BDNF (SMD = −1.78, 95% CI = (−5.34, 1.77), p = 0.33), and sRAGE (SMD = −0.25, 95% CI = (−0.94, 0.43), p = 0.47).
VCI defines the wide spectrum of cognitive disorders caused by different types of cerebrovascular disease and is deemed to be the most common cognitive disorder in the elderly (2). To date, the exact neurochemical basis underlying VCI is not completely clarified. Some of the molecular, biochemical, and electrophysiological abnormalities detected in VCI seem to correlate with disease process and progression. A previous prospective study showed that at least seven different pathologies can predict VCI: large infarcts, lacunar infarcts, microinfarcts, myelin loss, arteriolosclerosis, cerebral amyloid angiopathy (CAA), and perivascular space dilation (61, 62). Growing evidence correlates cerebral hypoperfusion to both cognitive decline and white matter lesions (WMLs) (62). Emerging evidence indicates that various components, such as oxidative stress, neurotransmitter imbalance, neuroinflammation, endothelial dysfunction, and cortical hyperexcitability, play significant roles in VCI (63). Of note, VCI is the only contribution that can be, at least in part, preventable and treatable (64). The search for novel hallmarks of disease process and progression, such as serological, CSF, and instrumental markers, is needed to allow an early, tailored, and accurate screening of VCI patients. Previous meta-analyses have not examined the relationship between VCI and blood biomarkers related to neuronal function. In the present study, we have explored the potential of specific biomarkers associated with neuronal function as indicators. A meta-analysis was conducted on markers examined in two or more of the 30 studies considered.
Cerebral ischemia induces amyloid aggregation, exacerbating inflammatory and neurodegenerative processes in the brain parenchyma, resulting in cognitive dysfunction (65–68). Aβ is a 4 kDa protein produced through the sequential proteolytic cleavage of amyloid precursor protein (APP) by β-secretase and γ-secretase (69). Aβ42 and Aβ40 are the two most prevalent isoforms, with Aβ40 being the most abundant (70, 71). Due to its higher hydrophobicity and propensity to aggregate, Aβ42 demonstrates more significant neurotoxicity, with even low concentrations capable of inducing neuronal death (72) and was considered significant potential for the diagnosis of AD (73–77). Huang et al. previously investigated the potential of common AD biomarkers in predicting VCI (30). In this study, we performed a meta-analysis to assess the potential of five AD biomarkers in individually predicting VCI. Our findings indicate that the peripheral levels of Aβ42 and the Aβ42/Aβ40 ratio in the VCI group were significantly lower than those in the control group, while no significant difference was observed in Aβ40 between the two groups. The results of this study suggest that peripheral Aβ42 has potential as a predictor for VCI, aligning with previous findings (29, 35, 44, 48). However, further subgroup analyses did not reveal statistically significant differences between the VaD and PSCI groups compared to the control group. Therefore, the results of this meta-analysis should be interpreted with caution. Moreover, more extensive cohort studies are necessary to validate the ability of Aβ42 to differentiate VCI from AD. It has been reported that the plasma Aβ42/Aβ40 ratio exhibits the strongest correlation with CSF biomarkers (78). Combining Aβ42 with Aβ40 can account for inter-individual differences in Aβ processing and potential pre-analytical confounding factors (79). Previous studies have reported strong consistency between the plasma Aβ42/Aβ40 ratio and amyloid positron emission tomography (PET) status (80). Although the meta-analysis results for the Aβ42/Aβ40 ratio in this study showed significant differences, only two studies were included, both with small sample sizes. Future research should further investigate the relationship between plasma Aβ42/Aβ40 and VCI.
NfL is a crucial component of the neuronal axonal cytoskeleton and is highly concentrated in neuronal axons (81, 82). Pathological processes that result in neuronal axonal damage lead to the release of NfL into the CSF and, at lower concentrations, into the bloodstream (81, 83). Given the strong correlation between NfL levels in the CSF and peripheral blood, coupled with advancements in quantitative detection techniques for plasma NfL (pNfL), research on the role of pNfL in neurodegenerative diseases and brain injuries has been increasing (31, 84–86). Previous studies have identified circulating NfL as a biomarker for AD (73, 85, 87–89). Recently, NfL has also been associated with VCI (31, 39, 47, 49, 54). This study’s meta-analysis reveals that circulating NfL levels are significantly higher in VCI patients than controls. The levels of circulating NfL were found to have a positive correlation with VCI in all of the included studies. Further subgroup analysis indicates that NfL levels in patients with VaD and PSCI are significantly elevated compared to controls, with the SMD for the VaD group being more significant than that for the PSCI group (2.01 vs. 0.99). We hypothesize that the more severe cognitive dysfunction in VaD results in greater neuronal axonal damage, leading to increased NfL release (90). Additionally, a meta-analysis has shown that CSF NfL levels in VaD patients are significantly higher than in AD patients (91). Recently, two studies have reported that plasma NfL levels in VCI patients are significantly elevated compared to those in the AD group, highlighting its potential to distinguish between VCI and AD (39, 92). Meanwhile, a study established a VCI mouse model and found that treatment with the angiotensin 1-7/MAS receptor agonist could reverse cognitive impairment and significantly reduce NfL levels, suggesting that circulating NfL may be a prognostic biomarker for VCI (93). Another longitudinal study showed that baseline NfL levels in cSVD patients could predict changes in MRI biomarkers, cognitive decline, and dementia over a five-year follow-up period; however, no significant changes in NfL levels were observed during the follow-up (94). In conclusion, we believe that NfL is a promising diagnostic and prognostic biomarker for VCI.
S100B is a calcium-binding protein primarily located in astrocytes and Schwann cells, and it is associated with dystrophic axons within Aβ plaques. Upon central nervous system injury, glial cells become activated, and if the BBB is compromised, S100B can be released into the bloodstream (32, 95). This protein has diverse functions, including regulating protein phosphorylation, cell growth, movement, differentiation, the cell cycle, and transcription processes (96). At nanomolar concentrations, S100B functions as a neurotrophic factor; however, higher micromolar concentrations may induce apoptosis (97). Previous studies have demonstrated an association between peripheral S100B levels and VCI (32, 40, 42, 98). Our meta-analysis indicates that serum S100B levels are significantly elevated in the VCI group compared to the control group. Therefore, we propose S100B as a potential biomarker for VCI.
Our meta-analysis found no significant differences between the two groups in t-Tau and p-tau 181. Notably, among the three studies examining peripheral concentrations of p-tau 181, two reported a positive correlation with VCI, while one found the opposite. Thus, future research should continue to investigate this biomarker. Moreover, the meta-analysis did not reveal any statistically significant differences in BDNF and sRAGE between the groups. It is important to note that the number of studies and the overall sample size included in our analysis were limited, and the results may not fully reflect the roles of these biomarkers in VCI. Therefore, larger, multicenter, prospective cohort studies are needed to better understand the genuine relationship between these biomarkers and VCI.
From the studies reviewed here, it appears evident that the biomarkers of neuronal function were deemed to play a role in VCI. Although some of these pathomechanisms are also shared by AD, the findings seem to converge on the possibility that some changes might be specifically involved in VCI patients. Recent studies have identified an association between neuronal functional circulating biomarkers and “asymptomatic” or “covert” cSVD (ccSVD) (99). For instance, one cohort study demonstrated that brain atrophy and WMH are independently associated with plasma NfL levels in cSVD patients with cognitive impairment (100). Additionally, a longitudinal study revealed that plasma NfL levels were significantly elevated in individuals with moderate to severe cSVD burden compared to those without such burden, and these levels positively correlated with CMBs, lacunar infarcts, and moderate to severe WMH (101). Other studies have also suggested that peripheral NfL levels serve as a valuable low-invasive biomarker that can complement MRI findings and may reflect the severity of the cSVD burden (102–104). In addition, a population-based aging study identified a correlation between a lower plasma Aβ42/Aβ40 ratio and higher plasma p-tau217 levels with CAA in individuals exhibiting CMBs (105). Research conducted by Huss et al. revealed that serum levels of glial fibrillary acidic protein (GFAP) in patients with sporadic cSVD were significantly associated with neurocognitive function. This finding suggests that astrocyte dysfunction may play a critical role in the progression of cSVD (106). GFAP, an intermediate filament protein of the astrocytic cytoskeleton, is a specific marker of reactive astrogliosis (107). A prospective cohort study further indicated that serum GFAP is a promising liquid biomarker for sporadic cSVD, as it correlates with clinical severity and cognitive function (108). Consequently, peripheral neuronal functional biomarkers are also crucial in understanding cSVD.
In addition to circulating biomarkers, recent studies have identified neurophysiological and hemodynamic markers as significant predictors of VCI (109). Research suggests that a reduction in cerebral blood flow (CBF) precedes the clinical onset of VCI, indicating that CBF measurement could aid in the early detection of VCI patients (110, 111). The strong correlation between CBF and neuronal function and metabolism underscores its clinical relevance as a marker of brain function (112). Proper regulation of CBF and normal brain metabolism are essential for maintaining cognitive function (112). MRI-based arterial spin labeling (ASL) method is a non-invasive MRI technique that measures tissue perfusion in capillaries and small arteries (109, 113). ASL is a non-invasive MRI technique that measures tissue perfusion in capillaries and small arteries (109, 113). It can be seamlessly integrated into routine brain MRI scans, requiring only 5 min of scanning time (114). ASL offers several advantages, including non-invasiveness, the absence of radiation or tracer use, high reproducibility, and broader accessibility (115). A recent cohort study demonstrated that a decline in ASL-detected CBF is significantly associated with overall cognitive function in VCI patients (116). Several other studies have reported similar findings, suggesting that ASL-based CBF measurement, as a viable alternative to PET (115), holds promise for the early prediction of VCI (111, 117, 118).
Transcranial Doppler ultrasound (TCD), also a non-invasive examination method, may not match the spatial resolution of functional MRI or PET, but it plays a crucial role in hemodynamic assessment due to its excellent temporal resolution (5 milliseconds), ease of operation, and strong resistance to motion artifacts (109). Previous TCD studies suggested that insufficient cerebral perfusion and high vascular resistance may contribute to the development of VCI (119). Furthermore, a meta-analysis of TCD by Fresnais et al. indicated that, compared to cognitively normal elderly individuals, patients with VaD exhibit significantly reduced cerebral blood velocity (CBV) in the MCA and a significantly increased pulsatility index (PI) (120).
At present, there is a deficiency in effective therapies and methods for VCI (121). Recent research has highlighted the significant role of transcranial magnetic stimulation (TMS) in diagnosing and treating VCI (122). TMS is a non-invasive and relatively safe brain stimulation technique that has garnered attention for its ability to selectively induce electrical currents in specific cortical regions through electromagnetic induction (123). Some studies have found that the motor cortex in patients with VaD is more easily excited compared to the control group (resting motor threshold decreased), suggesting a compensatory mechanism in response to ischemic damage and neuronal loss (124). Additionally, research has demonstrated a significant reduction in short-latency afferent inhibition (SAI), an indicator of central cholinergic transmission, in VCI patients (62, 125, 126). Regarding treatment, studies using VaD mouse models have shown that low-frequency repetitive TMS can ameliorate cognitive deficits by upregulating the release of hippocampal BDNF and enhancing the expression of N-methyl-D-aspartate (NMDA) glutamate receptors (122). A recent meta-analysis also indicates that TMS can improve cognitive abilities and daily living activities in stroke patients. The further search for novel hallmarks of disease process and progression, such as serological, instrumental or CSF markers, is needed to allow an early, tailored, and accurate screening of VCI patients. This will also pave the way to innovative the identification of predictors of drug response and therapeutic strategies.
This study acknowledges several limitations. First, most included studies were conducted on Asian populations, which may introduce racial differences that could affect the research outcomes. Second, certain newly identified biomarkers associated with neuronal function, such as β-secretase 1 (BACE1), neuropeptide Y (NPY), neural cell adhesion molecule (NCAM), neuregulin 1 (NRG1), neuronal pentraxin 2 (NPTX2), and sphingosine-1-phosphate (S1P), were excluded from the meta-analysis due to the limited number of studies and small total sample size. These findings require further validation in future research. Third, the control groups in these studies predominantly consisted of healthy individuals or stroke patients, leaving the question of whether these biomarkers can distinguish between dementia subtypes unanswered. Fourth, due to the limited number of included articles, a subgroup analysis could not be performed for the Aβ42/Aβ40 ratio and S100B biomarkers. Finally, the predictive capacity of a single biomarker for VCI may be restricted, suggesting that future research might benefit from integrating multiple biomarkers to enhance predictive accuracy.
In this study, we found that the levels of circulating NfL and S100B in VCI patients were significantly higher than those in non-VCI patients, while the levels of Aβ42 and the Aβ42/Aβ40 ratio were significantly lower in VCI patients compared to non-VCI patients. Therefore, we suggest clinicians focus on these blood biomarkers and integrate neuroimaging and neuropsychological assessments to evaluate the risk of VCI, which may aid in early detection and timely intervention. Additionally, due to the limited number of studies, some other novel blood biomarkers could not be included in the meta-analysis, and we recommend further validation in future research.
WH: Data curation, Formal analysis, Investigation, Methodology, Writing – original draft. LL: Data curation, Formal analysis, Investigation, Methodology, Writing – original draft. QL: Formal analysis, Investigation, Writing – review & editing. RM: Data curation, Formal analysis, Writing – review & editing. XH: Data curation, Investigation, Writing – review & editing. XD: Data curation, Formal analysis, Investigation, Writing – review & editing. DS: Conceptualization, Formal analysis, Funding acquisition, Investigation, Project administration, Resources, Writing – review & editing.
The author(s) declare that financial support was received for the research, authorship, and/or publication of this article. This work was funded by the Clinical Research Project of Nanjing Drum Tower Hospital (2022-LCYJ-ZX-05).
We thank all participants and clinical staff for supporting and contributing to this study.
The authors declare that the research was conducted in the absence of any commercial or financial relationships that could be construed as a potential conflict of interest.
All claims expressed in this article are solely those of the authors and do not necessarily represent those of their affiliated organizations, or those of the publisher, the editors and the reviewers. Any product that may be evaluated in this article, or claim that may be made by its manufacturer, is not guaranteed or endorsed by the publisher.
The Supplementary material for this article can be found online at: https://www.frontiersin.org/articles/10.3389/fneur.2025.1496711/full#supplementary-material
1. Dichgans, M, and Leys, D. Vascular Cognitive Impairment. Circ Res. (2017) 120:573–91. doi: 10.1161/circresaha.116.308426
2. Badji, A, Youwakim, J, Cooper, A, Westman, E, and Marseglia, A. Vascular cognitive impairment – past, present, and future challenges. Ageing Res Rev. (2023) 90:102042. doi: 10.1016/j.arr.2023.102042
3. Nichols, E, Steinmetz, JD, Vollset, SE, Fukutaki, K, Chalek, J, Abd-Allah, F, et al. Estimation of the global prevalence of dementia in 2019 and forecasted prevalence in 2050: an analysis for the global burden of disease study 2019. Lancet Public Health. (2022) 7:e105–25. doi: 10.1016/s2468-2667(21)00249-8
4. Gao, C-Y, Lian, Y, Zhang, M, Zhang, L-L, Fang, C-Q, Deng, J, et al. Association of Dementia with death after ischemic stroke: a two-year prospective study. Exp Ther Med. (2016) 12:1765–9. doi: 10.3892/etm.2016.3538
5. Li, J, Wang, J, Wu, B, Xu, H, Wu, X, Zhou, L, et al. Association between early cognitive impairment and midterm functional outcomes among Chinese acute ischemic stroke patients: a longitudinal study. Front Neurol. (2020) 11:20. doi: 10.3389/fneur.2020.00020
6. Rundek, T, Tolea, M, Ariko, T, Fagerli, EA, and Camargo, CJ. Vascular Cognitive Impairment (Vci). Neurotherapeutics. (2022) 19:68–88. doi: 10.1007/s13311-021-01170-y
7. Bir, SC, Khan, MW, Javalkar, V, Toledo, EG, and Kelley, RE. Emerging concepts in vascular dementia: a review. J Stroke Cerebrovasc Dis. (2021) 30:105864. doi: 10.1016/j.jstrokecerebrovasdis.2021.105864
8. Bella, R, Ferri, R, Cantone, M, Pennisi, M, Lanza, G, Malaguarnera, G, et al. Motor cortex excitability in vascular depression. Int J Psychophysiol. (2011) 82:248–53. doi: 10.1016/j.ijpsycho.2011.09.006
9. Bella, R, Ferri, R, Lanza, G, Cantone, M, Pennisi, M, Puglisi, V, et al. Tms follow-up study in patients with vascular cognitive impairment-no dementia. Neurosci Lett. (2013) 534:155–9. doi: 10.1016/j.neulet.2012.12.017
10. Inoue, Y, Shue, F, Bu, G, and Kanekiyo, T. Pathophysiology and probable etiology of cerebral small vessel disease in vascular dementia and Alzheimer’s disease. Mol Neurodegener. (2023) 18:46. doi: 10.1186/s13024-023-00640-5
11. Hannawi, Y. Cerebral small vessel disease: a review of the pathophysiological mechanisms. Transl Stroke Res. (2023) 15:1050–69. doi: 10.1007/s12975-023-01195-9
12. Meng, R, Ji, X, Ding, Y, Zhou, D, Ya, J, and Wu, X. Pathogeneses and imaging features of cerebral white matter lesions of vascular origins. Aging Dis. (2021) 12:2031–51. doi: 10.14336/ad.2021.0414
13. van den Brink, H, Doubal, FN, and Duering, M. Advanced Mri in cerebral small vessel disease. Int J Stroke. (2022) 18:28–35. doi: 10.1177/17474930221091879
14. Jochems, ACC, Arteaga, C, Chappell, F, Ritakari, T, Hooley, M, Doubal, F, et al. Longitudinal changes of white matter Hyperintensities in sporadic small vessel disease. Neurology. (2022) 99:e2454–63. doi: 10.1212/wnl.0000000000201205
15. Huang, W-Q, Lin, Q, and Tzeng, C-M. Leukoaraiosis: epidemiology, imaging, risk factors, and management of age-related cerebral white matter Hyperintensities. J Stroke. (2024) 26:131–63. doi: 10.5853/jos.2023.02719
16. Dimaras, T, Merkouris, E, Tsiptsios, D, Christidi, F, Sousanidou, A, Orgianelis, I, et al. Leukoaraiosis as a promising biomarker of stroke recurrence among stroke survivors: a systematic review. Neurol Int. (2023) 15:994–1013. doi: 10.3390/neurolint15030064
17. Tziaka, E, Christidi, F, Tsiptsios, D, Sousanidou, A, Karatzetzou, S, Tsiakiri, A, et al. Leukoaraiosis as a predictor of depression and cognitive impairment among stroke survivors: a systematic review. Neurol Int. (2023) 15:238–72. doi: 10.3390/neurolint15010016
18. Georgakis, MK, Fang, R, Düring, M, Wollenweber, FA, Bode, FJ, Stösser, S, et al. Cerebral small vessel disease burden and cognitive and functional outcomes after stroke: a multicenter prospective cohort study. Alzheimers Dement. (2022) 19:1152–63. doi: 10.1002/alz.12744
19. Fierini, F, Poggesi, A, and Pantoni, L. Leukoaraiosis as an outcome predictor in the acute and subacute phases of stroke. Expert Rev Neurother. (2017) 17:963–75. doi: 10.1080/14737175.2017.1371013
20. Cedres, N, Ferreira, D, Machado, A, Shams, S, Sacuiu, S, Waern, M, et al. Predicting Fazekas scores from automatic segmentations of white matter signal abnormalities. Aging. (2020) 12:894–901. doi: 10.18632/aging.102662
21. Verdelho, A, Madureira, S, Moleiro, C, Ferro, JM, Santos, CO, Erkinjuntti, T, et al. White matter changes and diabetes predict cognitive decline in the elderly. Neurology. (2010) 75:160–7. doi: 10.1212/WNL.0b013e3181e7ca05
22. Verdelho, A, Madureira, S, Ferro, JM, Baezner, H, Blahak, C, Poggesi, A, et al. Physical activity prevents progression for cognitive impairment and vascular dementia. Stroke. (2012) 43:3331–5. doi: 10.1161/strokeaha.112.661793
23. Lam, S, Lipton, RB, Harvey, DJ, Zammit, AR, and Ezzati, A. White matter Hyperintensities and cognition across different Alzheimer's biomarker profiles. J Am Geriatr Soc. (2021) 69:1906–15. doi: 10.1111/jgs.17173
24. Ma, Y, Chen, Y, Yang, T, He, X, Yang, Y, Chen, J, et al. Blood biomarkers for post-stroke cognitive impairment: a systematic review and meta-analysis. J Stroke Cerebrovasc Dis. (2024) 33:107632. doi: 10.1016/j.jstrokecerebrovasdis.2024.107632
25. Gallucci, L, Sperber, C, Monsch, AU, Klöppel, S, Arnold, M, and Umarova, RM. Improving diagnostic accuracy of the Montreal cognitive assessment to identify post-stroke cognitive impairment. Sci Rep. (2024) 14:20125. doi: 10.1038/s41598-024-71184-x
26. Alcolea, D, Beeri, MS, Rojas, JC, Gardner, RC, and Lleó, A. Blood biomarkers in neurodegenerative diseases. Neurology. (2023) 101:172–80. doi: 10.1212/wnl.0000000000207193
27. Kim, KY, Shin, KY, and Chang, K-A. Potential biomarkers for post-stroke cognitive impairment: a systematic review and meta-analysis. Int J Mol Sci. (2022) 23:602. doi: 10.3390/ijms23020602
28. Liu, W, Wong, A, Au, L, Yang, J, Wang, Z, Leung, EYL, et al. Influence of amyloid-Β on cognitive decline after stroke/transient ischemic attack. Stroke. (2015) 46:3074–80. doi: 10.1161/strokeaha.115.010449
29. Chi, N-F, Chao, S-P, Huang, L-K, Chan, L, Chen, Y-R, Chiou, H-Y, et al. Plasma amyloid Beta and tau levels are predictors of post-stroke cognitive impairment: a longitudinal study. Front Neurol. (2019) 10:715. doi: 10.3389/fneur.2019.00715
30. Huang, L-K, Chao, S-P, Hu, C-J, Chien, L-N, Chiou, H-Y, Lo, Y-C, et al. Plasma phosphorylated-tau 181 is a predictor of post-stroke cognitive impairment: a longitudinal study. Front Aging Neurosci. (2022) 14:889101. doi: 10.3389/fnagi.2022.889101
31. Wang, Z, Wang, R, Li, Y, Li, M, Zhang, Y, Jiang, L, et al. Plasma Neurofilament light chain as a predictive biomarker for post-stroke cognitive impairment: a prospective cohort study. Front Aging Neurosci. (2021) 13:631738. doi: 10.3389/fnagi.2021.631738
32. Li, Y, Chen, X, Zhou, R, Xu, W, Wang, X, Chao, W, et al. Correlation between cognitive impairment and homocysteine and S100b protein in patients with progressive ischemic stroke. Neuropsychiatr Dis Treat. (2023) 19:209–17. doi: 10.2147/ndt.S393624
33. Luo, D, Wan, X, Liu, J, and Tong, T. Optimally estimating the sample mean from the sample size, median, mid-range, and/or mid-quartile range. Stat Methods Med Res. (2016) 27:1785–805. doi: 10.1177/0962280216669183
34. Wan, X, Wang, W, Liu, J, and Tong, T. Estimating the sample mean and standard deviation from the sample size, median, range and/or interquartile range. BMC Med Res Methodol. (2014) 14:135. doi: 10.1186/1471-2288-14-135
35. Bibl, M, Esselmann, H, Mollenhauer, B, Weniger, G, Welge, V, Liess, M, et al. Blood-based neurochemical diagnosis of vascular dementia: a pilot study. J Neurochem. (2007) 103:467–74. doi: 10.1111/j.1471-4159.2007.04763.x
36. Cao, L, and Sun, Z. Diagnostic values of serum levels of homocysteine, heat shock protein 70 and high-sensitivity C-reactive protein for predicting vascular cognitive impairment. Neuropsychiatr Dis Treat. (2022) 18:525–33. doi: 10.2147/ndt.S354022
37. Chen, Y-C, Chou, W-H, Fang, C-P, Liu, T-H, Tsou, H-H, Wang, Y, et al. Serum level and activity of Butylcholinesterase: a biomarker for post-stroke dementia. J Clin Med. (2019) 8:1778. doi: 10.3390/jcm8111778
38. Chua, XY, Chai, YL, Chew, WS, Chong, JR, Ang, HL, Xiang, P, et al. Immunomodulatory Sphingosine-1-phosphates as plasma biomarkers of Alzheimer’s disease and vascular cognitive impairment. Alzheimers Res Ther. (2020) 12:122. doi: 10.1186/s13195-020-00694-3
39. Chua, XY, Torta, F, Chong, JR, Venketasubramanian, N, Hilal, S, Wenk, MR, et al. Lipidomics profiling reveals distinct patterns of plasma sphingolipid alterations in Alzheimer’s disease and vascular dementia. Alzheimers Res Ther. (2023) 15:214. doi: 10.1186/s13195-023-01359-7
40. Gao, Q, Fan, Y, Mu, L-Y, Ma, L, Song, Z-Q, and Zhang, Y-N. S100b and Adma in cerebral small vessel disease and cognitive dysfunction. J Neurol Sci. (2015) 354:27–32. doi: 10.1016/j.jns.2015.04.031
41. Liu, J, Li, Q, Peng, T, Zhou, Q, He, B, and Zhu, B. Assessment of four serum biochemical markers in elderly patients with vascular dementia after cerebral infarction and their response to donepezil and Idebenone. J Neurol Surg B Skull Base. (2022) 84:629–36. doi: 10.1055/s-0042-1756500
42. Shi, S, Wang, G, Zhang, K, Zhang, Z, Liang, K, Li, K, et al. Expression of S100β protein in patients with vascular dementia after basal ganglia hemorrhage and its clinical significance. Exp Ther Med. (2017) 13:1917–21. doi: 10.3892/etm.2017.4207
43. Wang, X, Zhang, F, Ma, W, Feng, D, Zhang, J, Xu, J, et al. Increased levels of serum Neuregulin 1 associated with cognitive impairment in vascular dementia. Biomed Res Int. (2020) 2020:1–5. doi: 10.1155/2020/6683747
44. Xu, M, Yang, L, Zhong, Z, and Ye, M. Research on diagnostic markers for post-stroke cognitive impairment. Altern Ther Health Med. (2023) 29:230–5.
45. Dong, W, Lu, Y, Zhai, Y, Bi, Y, Peng, Y, Ju, Z, et al. Plasma neuropeptide Y and cognitive impairment after acute ischemic stroke. J Affect Disord. (2022) 317:221–7. doi: 10.1016/j.jad.2022.08.052
46. Holm, H, Nägga, K, Nilsson, ED, Ricci, F, Melander, O, Hansson, O, et al. High circulating levels of Midregional Proenkephalin a predict vascular dementia: a population-based prospective study. Sci Rep. (2020) 10:8027. doi: 10.1038/s41598-020-64998-y
47. Jiang, L, Wang, Z, Wang, R, Li, M, Zhang, Y, Yang, D, et al. Plasma Neurofilament light chain is associated with cognitive impairment after posterior circulation stroke. Evid Based Complement Alternat Med. (2022) 2022:1–7. doi: 10.1155/2022/2466982
48. Mao, L, Chen, X-H, Zhuang, J-H, Li, P, Xu, Y-X, Zhao, Y-C, et al. Relationship between Β-amyloid protein 1-42, thyroid hormone levels and the risk of cognitive impairment after ischemic stroke. World J Clin Cases. (2020) 8:76–87. doi: 10.12998/wjcc.v8.i1.76
49. Wang, J-H, Huang, J, Guo, F-Q, Wang, F, Yang, S, Yu, N-W, et al. Circulating Neurofilament light predicts cognitive decline in patients with post-stroke subjective cognitive impairment. Front Aging Neurosci. (2021) 13:665981. doi: 10.3389/fnagi.2021.665981
50. You, S, Bi, Y, Miao, M, Bao, A, Du, J, Xu, T, et al. Plasma Sdpp 4 (soluble dipeptidyl Peptidase-4) and cognitive impairment after Noncardioembolic acute ischemic stroke. Stroke. (2023) 54:113–21. doi: 10.1161/strokeaha.122.040798
51. Zhong, C, Lu, Z, Che, B, Qian, S, Zheng, X, Wang, A, et al. Choline pathway nutrients and metabolites and cognitive impairment after acute ischemic stroke. Stroke. (2021) 52:887–95. doi: 10.1161/strokeaha.120.031903
52. Emanuele, E, D’Angelo, A, Tomaino, C, Binetti, G, Ghidoni, R, Politi, P, et al. Circulating levels of soluble receptor for advanced glycation end products in Alzheimer disease and vascular dementia. Arch Neurol. (2005) 62:1734–6. doi: 10.1001/archneur.62.11.1734
53. Liang, F, Jia, J, Wang, S, Qin, W, and Liu, G. Decreased plasma levels of soluble low density lipoprotein receptor-related Protein-1 (Slrp) and the soluble form of the receptor for advanced glycation end products (Srage) in the clinical diagnosis of Alzheimer’s disease. J Clin Neurosci. (2013) 20:357–61. doi: 10.1016/j.jocn.2012.06.005
54. Ma, W, Zhang, J, Xu, J, Feng, D, Wang, X, Zhang, F, et al. Elevated levels of serum Neurofilament light chain associated with cognitive impairment in vascular dementia. Dis Markers. (2020) 2020:1–5. doi: 10.1155/2020/6612871
55. Shao, K, Shan, S, Ru, W, and Ma, C. Association between serum Nptx2 and cognitive function in patients with vascular dementia. Brain Behav. (2020) 10:e01779. doi: 10.1002/brb3.1779
56. Tang, S-C, Yang, K-C, Hu, C-J, Chiou, H-Y, Wu, CC, and Jeng, J-S. Elevated plasma level of soluble form of rage in ischemic stroke patients with dementia. NeuroMolecular Med. (2017) 19:579–83. doi: 10.1007/s12017-017-8471-9
57. Uslu, S, Akarkarasu, ZE, Ozbabalik, D, Ozkan, S, Çolak, O, Demirkan, ES, et al. Levels of amyloid Beta-42, Interleukin-6 and tumor necrosis factor-alpha in Alzheimer’s disease and vascular dementia. Neurochem Res. (2012) 37:1554–9. doi: 10.1007/s11064-012-0750-0
58. Xu, X-Y, Deng, C-Q, Wang, J, Deng, X-J, Xiao, Q, Li, Y, et al. Plasma levels of soluble receptor for advanced glycation end products in Alzheimer's disease. Int J Neurosci. (2016) 127:454–8. doi: 10.1080/00207454.2016.1193861
59. Zhao, J, Lu, W, Li, J, Liu, L, Zhao, X, and Xu, Y. Decreased serum Ncam levels associated with cognitive impairment in vascular dementia. Dis Markers. (2021) 2021:1–6. doi: 10.1155/2021/2792884
60. Zuliani, G, Trentini, A, Rosta, V, Guerrini, R, Pacifico, S, Bonazzi, S, et al. Increased blood Bace 1 activity as a potential common pathogenic factor of vascular dementia and late onset Alzheimer's disease. Sci Rep. (2020) 10:14980. doi: 10.1038/s41598-020-72168-3
61. Skrobot, OA, Attems, J, Esiri, M, Hortobágyi, T, Ironside, JW, Kalaria, RN, et al. Vascular cognitive impairment neuropathology guidelines (Vcing): the contribution of cerebrovascular pathology to cognitive impairment. Brain. (2016) 139:2957–69. doi: 10.1093/brain/aww214
62. Vinciguerra, L, Lanza, G, Puglisi, V, Fisicaro, F, Pennisi, M, Bella, R, et al. Update on the neurobiology of vascular cognitive impairment: from lab to clinic. Int J Mol Sci. (2020) 21:2977. doi: 10.3390/ijms21082977
63. Parfenov, VA, Ostroumova, OD, Ostroumova, TM, Kochetkov, AI, Fateeva, VV, Khacheva, KK, et al. Vascular cognitive impairment: pathophysiological mechanisms, insights into structural basis, and perspectives in specific treatments. Neuropsychiatr Dis Treat. (2019) 15:1381–402. doi: 10.2147/ndt.S197032
64. Azarpazhooh, MR, and Hachinski, V. Vascular cognitive impairment: a preventable component of dementia. Geriatr Neurol. (2019) 167:377–91. doi: 10.1016/B978-0-12-804766-8.00020-0
65. Thiel, A, Cechetto, DF, Heiss, W-D, Hachinski, V, and Whitehead, SN. Amyloid burden, Neuroinflammation, and links to cognitive decline after ischemic stroke. Stroke. (2014) 45:2825–9. doi: 10.1161/strokeaha.114.004285
66. Kaur, M, and Sharma, S. Molecular mechanisms of cognitive impairment associated with stroke. Metab Brain Dis. (2022) 37:279–87. doi: 10.1007/s11011-022-00901-0
67. Gireud-Goss, M, Mack, AF, McCullough, LD, and Urayama, A. Cerebral amyloid Angiopathy and blood-brain barrier dysfunction. Neuroscientist. (2020) 27:668–84. doi: 10.1177/1073858420954811
68. Goulay, R, Mena Romo, L, Hol, EM, and Dijkhuizen, RM. From stroke to dementia: a comprehensive review exposing tight interactions between stroke and amyloid-Β formation. Transl Stroke Res. (2019) 11:601–14. doi: 10.1007/s12975-019-00755-2
69. Hur, J-Y. Γ-secretase in Alzheimer’s disease. Exp Mol Med. (2022) 54:433–46. doi: 10.1038/s12276-022-00754-8
70. Hu, S, Yang, C, and Luo, H. Current trends in blood biomarker detection and imaging for Alzheimer’s disease. Biosens Bioelectron. (2022) 210:114278. doi: 10.1016/j.bios.2022.114278
71. Ring, J, Tadic, J, Ristic, S, Poglitsch, M, Bergmann, M, Radic, N, et al. The Hsp40 chaperone Ydj1 drives amyloid Beta 42 toxicity. EMBO Mol Med. (2022) 14:e13952. doi: 10.15252/emmm.202113952
72. Haass, C, and Selkoe, DJ. Soluble protein oligomers in neurodegeneration: lessons from the Alzheimer's amyloid Β-peptide. Nat Rev Mol Cell Biol. (2007) 8:101–12. doi: 10.1038/nrm2101
73. Dong, Y, Hou, T, Li, Y, Liu, R, Cong, L, Liu, K, et al. Plasma amyloid-Β, Total tau, and Neurofilament light chain across the Alzheimer’s disease clinical Spectrum: a population-based study. J Alzheimers Dis. (2023) 96:845–58. doi: 10.3233/jad-230932
74. Smirnov, DS, Ashton, NJ, Blennow, K, Zetterberg, H, Simrén, J, Lantero-Rodriguez, J, et al. Plasma biomarkers for Alzheimer’s disease in relation to neuropathology and cognitive change. Acta Neuropathol. (2022) 143:487–503. doi: 10.1007/s00401-022-02408-5
75. Li, R-X, Ma, Y-H, Tan, L, and Yu, J-T. Prospective biomarkers of Alzheimer’s disease: a systematic review and Meta-analysis. Ageing Res Rev. (2022) 81:101699. doi: 10.1016/j.arr.2022.101699
76. Brickman, AM, Manly, JJ, Honig, LS, Sanchez, D, Reyes-Dumeyer, D, Lantigua, RA, et al. Plasma P-Tau181, P-Tau217, and other blood-based Alzheimer's disease biomarkers in a multi-ethnic, community study. Alzheimers Dement. (2021) 17:1353–64. doi: 10.1002/alz.12301
77. Guo, Y, Shen, X-N, Wang, H-F, Chen, S-D, Zhang, Y-R, Chen, S-F, et al. The dynamics of plasma biomarkers across the Alzheimer’s continuum. Alzheimers Res Ther. (2023) 15:31. doi: 10.1186/s13195-023-01174-0
78. Verberk, IMW, Slot, RE, Verfaillie, SCJ, Heijst, H, Prins, ND, van Berckel, BNM, et al. Plasma amyloid as Prescreener for the earliest Alzheimer pathological changes. Ann Neurol. (2018) 84:648–58. doi: 10.1002/ana.25334
79. Leuzy, A, Mattsson-Carlgren, N, Palmqvist, S, Janelidze, S, Dage, JL, and Hansson, O. Blood-based biomarkers for Alzheimer's disease. EMBO Mol Med. (2021) 14:e14408. doi: 10.15252/emmm.202114408
80. Li, Y, Schindler, SE, Bollinger, JG, Ovod, V, Mawuenyega, KG, Weiner, MW, et al. Validation of plasma amyloid-Β 42/40 for detecting Alzheimer disease amyloid plaques. Neurology. (2022) 98:e688–99. doi: 10.1212/wnl.0000000000013211
81. Peters, N, van Leijsen, E, Tuladhar, AM, Barro, C, Konieczny, MJ, Ewers, M, et al. Serum Neurofilament light chain is associated with incident lacunes in progressive cerebral small vessel disease. J Stroke. (2020) 22:369–76. doi: 10.5853/jos.2019.02845
82. Gaur, A, Gallagher, D, Herrmann, N, Chen, JJ, Marzolini, S, Oh, P, et al. Neurofilament light chain as a biomarker of global cognition in individuals with possible vascular mild cognitive impairment. J Geriatr Psychiatry Neurol. (2024) 38:62–72. doi: 10.1177/08919887241254469
83. Khalil, M, Teunissen, CE, Lehmann, S, Otto, M, Piehl, F, Ziemssen, T, et al. Neurofilaments as biomarkers in neurological disorders — towards clinical application. Nat Rev Neurol. (2024) 20:269–87. doi: 10.1038/s41582-024-00955-x
84. Baiardi, S, Quadalti, C, Mammana, A, Dellavalle, S, Zenesini, C, Sambati, L, et al. Diagnostic value of plasma P-Tau181, Nfl, and Gfap in a clinical setting cohort of prevalent neurodegenerative dementias. Alzheimers Res Ther. (2022) 14:153. doi: 10.1186/s13195-022-01093-6
85. Cai, H, Pang, Y, Fu, X, Ren, Z, and Jia, L. Plasma biomarkers predict Alzheimer’s disease before clinical onset in Chinese cohorts. Nat Commun. (2023) 14:6747. doi: 10.1038/s41467-023-42596-6
86. Guedes, VA, Kenney, K, Shahim, P, Qu, B-X, Lai, C, Devoto, C, et al. Exosomal Neurofilament light. Neurology. (2020) 94:e2412–23. doi: 10.1212/wnl.0000000000009577
87. Mattsson, N, Cullen, NC, Andreasson, U, Zetterberg, H, and Blennow, K. Association between longitudinal plasma Neurofilament light and neurodegeneration in patients with Alzheimer disease. JAMA Neurol. (2019) 76:791–9. doi: 10.1001/jamaneurol.2019.0765
88. Huang, Y, Li, Y, Xie, F, and Guo, Q. Associations of plasma phosphorylated Tau181 and Neurofilament light chain with brain amyloid burden and cognition in objectively defined subtle cognitive decline patients. CNS Neurosci Ther. (2022) 28:2195–205. doi: 10.1111/cns.13962
89. Planche, V, Bouteloup, V, Pellegrin, I, Mangin, J-F, Dubois, B, Ousset, P-J, et al. Validity and performance of blood biomarkers for Alzheimer disease to predict dementia risk in a large clinic-based cohort. Neurology. (2023) 100:e473–84. doi: 10.1212/wnl.0000000000201479
90. Rajeev, V, Fann, DY, Dinh, QN, Kim, HA, De Silva, TM, Lai, MKP, et al. Pathophysiology of blood brain barrier dysfunction during chronic cerebral Hypoperfusion in vascular cognitive impairment. Theranostics. (2022) 12:1639–58. doi: 10.7150/thno.68304
91. Zhao, Y, Xin, Y, Meng, S, He, Z, and Hu, W. Neurofilament light chain protein in neurodegenerative dementia: a systematic review and network Meta-analysis. Neurosci Biobehav Rev. (2019) 102:123–38. doi: 10.1016/j.neubiorev.2019.04.014
92. Wang, J, Zhang, Y-R, Shen, X-N, Han, J, Cui, M, Tan, L, et al. Deamidation-related blood biomarkers show promise for early diagnostics of neurodegeneration. Biomark Res. (2022) 10:91. doi: 10.1186/s40364-022-00435-8
93. Hoyer-Kimura, C, Konhilas, JP, Mansour, HM, Polt, R, Doyle, KP, Billheimer, D, et al. Neurofilament light: a possible prognostic biomarker for treatment of vascular contributions to cognitive impairment and dementia. J Neuroinflammation. (2021) 18:236. doi: 10.1186/s12974-021-02281-1
94. Egle, M, Loubiere, L, Maceski, A, Kuhle, J, Peters, N, and Markus, HS. Neurofilament light chain predicts future dementia risk in cerebral small vessel disease. J Neurol Neurosurg Psychiatry. (2021) 92:582–9. doi: 10.1136/jnnp-2020-325681
95. Bonomi, CG, Assogna, M, Di Donna, MG, Bernocchi, F, De Lucia, V, Nuccetelli, M, et al. Cerebrospinal fluid Strem-2, Gfap, and Β-S100 in symptomatic sporadic Alzheimer’s disease: microglial, astrocytic, and Apoe contributions along the Alzheimer’s disease continuum. J Alzheimers Dis. (2023) 92:1385–97. doi: 10.3233/jad-221010
96. Kubis-Kubiak, A, Dyba, A, and Piwowar, A. The interplay between diabetes and Alzheimer’s disease—in the hunt for biomarkers. Int J Mol Sci. (2020) 21:2744. doi: 10.3390/ijms21082744
97. Goswami, D, Anuradha, U, Angati, A, Kumari, N, and Singh, RK. Pharmacological and pathological relevance of S100 proteins in neurological disorders. CNS Neurol Disord Drug Targets. (2023) 22:1403–16. doi: 10.2174/1871527322666221128160653
98. Wang, F, Zou, Z-R, Yuan, D, Gong, Y, Zhang, L, Chen, X, et al. Correlation between serum S100β protein levels and cognitive dysfunction in patients with cerebral small vessel disease: a case–control study. Biosci Rep. (2017) 37:BSR20160446. doi: 10.1042/bsr20160446
99. Kong, Q, Xie, X, Wang, Z, Zhang, Y, Zhou, X, Wu, L, et al. Correlations of plasma biomarkers and imaging characteristics of cerebral small vessel disease. Brain Sci. (2024) 14:269. doi: 10.3390/brainsci14030269
100. Chong, JR, Hilal, S, Ashton, NJ, Karikari, TK, Reilhac, A, Vrooman, H, et al. Brain atrophy and white matter Hyperintensities are independently associated with plasma Neurofilament light chain in an Asian cohort of cognitively impaired patients with concomitant cerebral small vessel disease. Alzheimers Dement. (2023) 15:e12396. doi: 10.1002/dad2.12396
101. Qu, Y, Tan, C-C, Shen, X-N, Li, H-Q, Cui, M, Tan, L, et al. Association of Plasma Neurofilament Light with small vessel disease burden in nondemented elderly. Stroke. (2021) 52:896–904. doi: 10.1161/strokeaha.120.030302
102. Sun, Y, Tan, L, Xu, W, Wang, Z-T, Hu, H, Li, J-Q, et al. Plasma Neurofilament light and longitudinal progression of white matter Hyperintensity in elderly persons without dementia. J Alzheimers Dis. (2020) 75:729–37. doi: 10.3233/jad-200022
103. Pinter, D, Gattringer, T, Enzinger, C, Seifert-Held, T, Kneihsl, M, Fandler, S, et al. Longitudinal Mri dynamics of recent small subcortical infarcts and possible predictors. J Cereb Blood Flow Metab. (2018) 39:1669–77. doi: 10.1177/0271678x18775215
104. Gattringer, T, Pinter, D, Enzinger, C, Seifert-Held, T, Kneihsl, M, Fandler, S, et al. Serum Neurofilament light is sensitive to active cerebral small vessel disease. Neurology. (2017) 89:2108–14. doi: 10.1212/wnl.0000000000004645
105. McCarter, SJ, Lesnick, TG, Lowe, VJ, Rabinstein, AA, Przybelski, SA, Algeciras-Schimnich, A, et al. Association between plasma biomarkers of amyloid, tau, and neurodegeneration with cerebral microbleeds. J Alzheimers Dis. (2022) 87:1537–47. doi: 10.3233/jad-220158
106. Huss, A, Abdelhak, A, Mayer, B, Tumani, H, Müller, H-P, Althaus, K, et al. Association of Serum Gfap with functional and neurocognitive outcome in sporadic small vessel disease. Biomedicines. (2022) 10:1869. doi: 10.3390/biomedicines10081869
107. Gonzales, MM, Vela, G, Philip, V, Trevino, H, LaRoche, A, Wang, C-P, et al. Demographic and clinical characteristics associated with serum Gfap levels in an ethnically diverse cohort. Neurology. (2023) 101:e1531–41. doi: 10.1212/wnl.0000000000207706
108. Gattringer, T, Enzinger, C, Pinter, D, Fandler-Höfler, S, Kneihsl, M, Haidegger, M, et al. Serum glial fibrillary acidic protein is sensitive to acute but not chronic tissue damage in cerebral small vessel disease. J Neurol. (2022) 270:320–7. doi: 10.1007/s00415-022-11358-7
109. Malojcic, B, Giannakopoulos, P, Sorond, FA, Azevedo, E, Diomedi, M, Oblak, JP, et al. Ultrasound and dynamic functional imaging in vascular cognitive impairment and Alzheimer’s disease. BMC Med. (2017) 15:27. doi: 10.1186/s12916-017-0799-3
110. Banerjee, G, Wilson, D, Jäger, HR, and Werring, DJ. Novel imaging techniques in cerebral small vessel diseases and vascular cognitive impairment. Biochim Biophys Acta. (2016) 1862:926–38. doi: 10.1016/j.bbadis.2015.12.010
111. Jann, K, Shao, X, Ma, SJ, Cen, SY, D’Orazio, L, Barisano, G, et al. Evaluation of cerebral blood flow measured by 3d Pcasl as biomarker of vascular cognitive impairment and dementia (Vcid) in a cohort of elderly Latinx subjects at risk of small vessel disease. Front Neurosci. (2021) 15:627627. doi: 10.3389/fnins.2021.627627
112. Huang, D, Guo, Y, Guan, X, Pan, L, Zhu, Z, Chen, Z, et al. Recent advances in arterial spin labeling perfusion Mri in patients with vascular cognitive impairment. J Cereb Blood Flow Metab. (2022) 43:173–84. doi: 10.1177/0271678x221135353
113. Padrela, BE, Lorenzini, L, Collij, LE, García, DV, Coomans, E, Ingala, S, et al. Genetic, vascular and amyloid components of cerebral blood flow in a preclinical population. J Cereb Blood Flow Metab. (2023) 43:1726–36. doi: 10.1177/0271678x231178993
114. Kapasouri, EM, Ioannidis, DC, Cameron, D, Vassiliou, VS, and Hornberger, M. The utility of arterial spin labeling Mri in medial temporal lobe as a vascular biomarker in Alzheimer’s disease Spectrum: a systematic review and Meta-analysis. Diagnostics. (2022) 12:2967. doi: 10.3390/diagnostics12122967
115. Zhang, K, Herzog, H, Mauler, J, Filss, C, Okell, TW, Kops, ER, et al. Comparison of cerebral blood flow acquired by simultaneous [15o] water positron emission tomography and arterial spin labeling magnetic resonance imaging. J Cereb Blood Flow Metab. (2014) 34:1373–80. doi: 10.1038/jcbfm.2014.92
116. van Dinther, M, Hooghiemstra, AM, Bron, EE, Versteeg, A, Leeuwis, AE, Kalay, T, et al. Lower cerebral blood flow predicts cognitive decline in patients with vascular cognitive impairment. Alzheimers Dement. (2023) 20:136–44. doi: 10.1002/alz.13408
117. Sun, Y, Cao, W, Ding, W, Wang, Y, Han, X, Zhou, Y, et al. Cerebral blood flow alterations as assessed by 3d Asl in cognitive impairment in patients with subcortical vascular cognitive impairment: a marker for disease severity. Front Aging Neurosci. (2016) 8:8. doi: 10.3389/fnagi.2016.00211
118. Schuff, N, Matsumoto, S, Kmiecik, J, Studholme, C, Du, A, Ezekiel, F, et al. Cerebral blood flow in ischemic vascular dementia and Alzheimer's disease, measured by arterial spin-labeling magnetic resonance imaging. Alzheimers Dement. (2009) 5:454–62. doi: 10.1016/j.jalz.2009.04.1233
119. Ginsberg, SD, Vinciguerra, L, Lanza, G, Puglisi, V, Pennisi, M, Cantone, M, et al. Transcranial Doppler ultrasound in vascular cognitive impairment-no dementia. PLoS One. (2019) 14:e0216162. doi: 10.1371/journal.pone.0216162
120. Fresnais, D, Ihle-Hansen, H, Lundström, E, Andersson, ÅG, and Fure, B. Cerebrovascular hemodynamics in cognitive impairment and dementia: a systematic review and Meta-analysis of transcranial Doppler studies. Dement Geriatr Cogn Disord. (2023) 52:277–95. doi: 10.1159/000535422
121. Biesbroek, JM, and Biessels, GJ. Diagnosing vascular cognitive impairment: current challenges and future perspectives. Int J Stroke. (2022) 18:36–43. doi: 10.1177/17474930211073387
122. Cantone, M, Lanza, G, Fisicaro, F, Pennisi, M, Bella, R, Di Lazzaro, V, et al. Evaluation and treatment of vascular cognitive impairment by transcranial magnetic stimulation. Neural Plast. (2020) 2020:1–17. doi: 10.1155/2020/8820881
123. Valero-Cabré, A, Amengual, JL, Stengel, C, Pascual-Leone, A, and Coubard, OA. Transcranial magnetic stimulation in basic and clinical neuroscience: a comprehensive review of fundamental principles and novel insights. Neurosci Biobehav Rev. (2017) 83:381–404. doi: 10.1016/j.neubiorev.2017.10.006
124. Di Lazzaro, V, Bella, R, Benussi, A, Bologna, M, Borroni, B, Capone, F, et al. Diagnostic contribution and therapeutic perspectives of transcranial magnetic stimulation in dementia. Clin Neurophysiol. (2021) 132:2568–607. doi: 10.1016/j.clinph.2021.05.035
125. Nardone, R, Bergmann, J, Tezzon, F, Ladurner, G, and Golaszewski, S. Cholinergic dysfunction in subcortical Ischaemic vascular dementia: a transcranial magnetic stimulation study. J Neural Transm. (2008) 115:737–43. doi: 10.1007/s00702-007-0003-6
Keywords: vascular cognitive impairment, biomarkers, blood, neuronal function, systematic review
Citation: Huang W, Liao L, Liu Q, Ma R, He X, Du X and Sha D (2025) Blood biomarkers for vascular cognitive impairment based on neuronal function: a systematic review and meta-analysis. Front. Neurol. 16:1496711. doi: 10.3389/fneur.2025.1496711
Received: 15 September 2024; Accepted: 28 January 2025;
Published: 07 February 2025.
Edited by:
Paco Herson, The Ohio State University, United StatesReviewed by:
Mariagiovanna Cantone, Gaspare Rodolico Hospital, ItalyCopyright © 2025 Huang, Liao, Liu, Ma, He, Du and Sha. This is an open-access article distributed under the terms of the Creative Commons Attribution License (CC BY). The use, distribution or reproduction in other forums is permitted, provided the original author(s) and the copyright owner(s) are credited and that the original publication in this journal is cited, in accordance with accepted academic practice. No use, distribution or reproduction is permitted which does not comply with these terms.
*Correspondence: Dujuan Sha, dGJ3ZW4wOTEyQDEyNi5jb20=
†These authors have contributed equally to this work
Disclaimer: All claims expressed in this article are solely those of the authors and do not necessarily represent those of their affiliated organizations, or those of the publisher, the editors and the reviewers. Any product that may be evaluated in this article or claim that may be made by its manufacturer is not guaranteed or endorsed by the publisher.
Research integrity at Frontiers
Learn more about the work of our research integrity team to safeguard the quality of each article we publish.