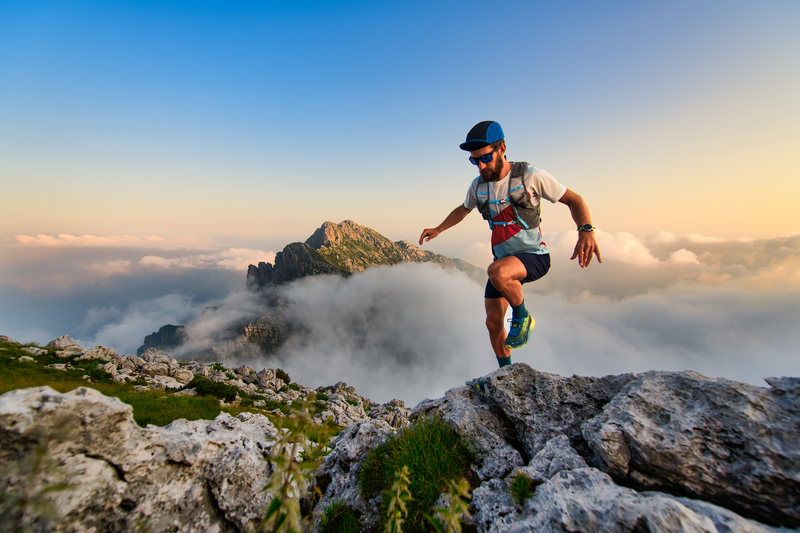
94% of researchers rate our articles as excellent or good
Learn more about the work of our research integrity team to safeguard the quality of each article we publish.
Find out more
ORIGINAL RESEARCH article
Front. Neurol. , 09 October 2024
Sec. Stroke
Volume 15 - 2024 | https://doi.org/10.3389/fneur.2024.1427692
Background: We investigated the potential relationship between age-related conditions, particularly sarcopenia and ischemic stroke (IS), through a two-sample Mendelian randomization (MR) study.
Methods: We conducted a two-sample bidirectional MR study to investigate the relationship between sarcopenia and stroke. Genetic instruments for sarcopenia were derived from the UK Biobank, while data on IS and its subtypes were obtained from the MEGASTROKE consortium. Inverse variance weighting (IVW) served as the primary analytical method. Additionally, heterogeneity and pleiotropy were assessed to ensure the robustness of the findings.
Results: The analysis indicates a negative correlation between appendicular lean mass (ALM) and small vessel stroke (SVS; OR = 0.790, 95% CI: 0.703–0.888, p < 0.001), a positive correlation with cardioembolic stroke (CES; OR = 1.165, 95% CI: 1.058–1.284, p = 0.002), and no causal relationship with any ischemic stroke (AIS) or large artery stroke (LAS). Additionally, SVS is negatively associated with right-hand grip strength (OR = 0.639, 95% CI: 0.437–0.934, p = 0.021), while AIS, LAS, and CES do not exhibit a causal relationship with grip strength. Furthermore, no causal relationship was identified between left-hand grip strength, usual walking pace, and IS or its subtypes. MR analysis reveals only a negative association between CES and usual walking pace (OR = 0.989, 95% CI: 0.980–0.998, p = 0.013), with no associations found between other IS subtypes and sarcopenia-related traits.
Conclusion: This study demonstrates that a reduction in ALM and right-hand grip strength is associated with SVS, whereas decreased ALM may serve as a protective factor against CES. Conversely, our analysis suggests that CES can impact walking speed. Overall, these findings provide valuable insights into the prevention and treatment of these conditions.
Stroke is the second leading cause of death and disability globally (1). In China, the incidence of stroke is rising each year, making it the leading cause of mortality (2, 3). According to a 2019 study, common risk factors for stroke include hypertension, high body mass index (BMI), elevated fasting glucose, air pollution, and smoking (4). However, given the increasing incidence of stroke, it is crucial to explore additional risk factors. Sarcopenia, which has been identified as influencing the incidence and outcomes of stroke, has primarily been studied through observational data (5–8). These observational studies are often subject to confounding factors, complicating the establishment of accurate causal relationships. While the impact of sarcopenia on stroke risk has been explored, most studies have focused on the overall risk of stroke without analyzing specific stroke subtypes. Additionally, several studies have reported a relatively high incidence of sarcopenia following a stroke (9–11), which can adversely affect patient recovery and quality of life. Despite this, the relationship between stroke subtypes and sarcopenia has been largely overlooked, with limited research addressing this area. To fill this gap, we employed the Mendelian randomization (MR) method to investigate the causal relationship between sarcopenia and the risk of various stroke subtypes, providing new insights for clinical prevention and intervention strategies.
Sarcopenia was first introduced in the late 1980s by Rosenberg. The term is derived from Greek, with “sarx” meaning flesh and “penia” meaning loss (12). As the global population ages, sarcopenia has emerged as a significant health concern, with an estimated 500 million people projected to be affected by 2050 (13). Sarcopenia is associated with an increased risk of falls, fractures, disability, weakness, and mortality (14). According to the European Working Group on Sarcopenia in Older People (EWGSOP), sarcopenia is defined by a decline in both muscle mass and function (15). In 2018, the EWGSOP2 updated the diagnostic criteria, proposing a stepwise approach. Initially, muscle strength is assessed, typically through grip strength measurement, with reduced grip strength suggesting possible sarcopenia. Next, appendicular lean mass (ALM) is evaluated using dual-energy X-ray absorptiometry, bioelectrical impedance analysis, computed tomography, or magnetic resonance imaging, with a diagnosis confirmed by decreased muscle mass and quantity. Finally, low physical capacity is used to assess the severity of sarcopenia, with commonly used indicators including gait speed and a timed 400-meter walk; reduced performance on these tests indicates severe sarcopenia (16). Consequently, we selected three variables to evaluate the onset and progression of sarcopenia: ALM, grip strength, and walking pace.
MR is a method used to infer causal relationships between an exposure and an outcome, grounded in Mendel’s Second Law. This approach reduces confounding bias and addresses the limitations inherent in traditional observational studies. By selecting single nucleotide polymorphisms (SNPs) associated with the exposure as instrumental variables (IVs), genetic variation is utilized to derive robust causal inferences between exposure factors and outcomes (17). In this study, we employed a two-sample MR analysis to evaluate the causal relationship between sarcopenia and IS.
The MR study was carried out in two distinct phases. Initially, a forward MR analysis was performed, utilizing three key characteristics of sarcopenia as exposures and various stroke subtypes as outcomes. In the second phase, a reverse MR analysis was conducted, where the roles of exposure and outcome were inverted. To ensure the validity of the MR analysis, three key assumptions must be satisfied (Figure 1): (1) The selected genetic instruments must be strongly associated with the exposure, fulfilling the relevance assumption; (2) The chosen genetic instruments must not be related to any confounding factors, adhering to the independence assumption; (3) The genetic instruments should affect the outcome only through the exposure, satisfying the exclusion restriction assumption (18). For this study, we utilized publicly available, large-scale genome-wide association study (GWAS) meta-data sets that had been previously published. In the original GWAS studies, all participants provided informed consent.
The EWGSOP2 defines the cut-off values for muscle mass as ALM < 20 kg for men and < 15 kg for women, and ALM/height2 < 7.0 kg/m2 for men and < 5.5 kg/m2 for women. The thresholds for low grip strength are <27 kg for men and < 16 kg for women, while the cut-off for walking pace is ≤0.8 m/s (16). ALM was measured using bioelectrical impedance analysis, based on data from the UK Biobank (n = 450,234) (19). GWAS summary data were also obtained from the UK Biobank for left-hand grip strength (n = 461,089) and right-hand grip strength (n = 461,026) (20). Additionally, the UK Biobank collected data on usual walking pace from 459,915 European individuals through touchscreen questionnaires (20).
The stroke GWAS dataset was sourced from the MEGASTROKE consortium and comprises 40,585 cases and 406,111 controls (21). IS is categorized by etiology into large artery stroke (LAS), small vessel stroke (SVS), and cardioembolic stroke (CES) based on the Trial of Org 10,172 in acute stroke treatment classification. To reduce bias from population stratification, all individuals included in the study were of European descent. Table 1 provides detailed information on the GWAS dataset utilized for the MR analysis.
First, SNPs with genome-wide significance (p < 5.0 × 10−8) were selected, and linkage disequilibrium among them was excluded (r2 < 0.001, kb = 10,000). This step ensured the identification of independent SNP loci that met the correlation assumption. Second, palindromic SNPs with intermediate allele frequencies were removed (Supplementary Table S8). Subsequently, we utilized PhenoScanner (http://www.phenoscanner.medschl.cam.ac.uk) to systematically search for the selected SNPs and exclude those associated with the outcome and potential confounding factors, including diabetes, hypertension, dyslipidemia, coronary artery disease, BMI, smoking, and alcohol consumption (Supplementary Table S9) (22), in order to satisfy the second and third assumptions. Additionally, SNPs with an F-statistic greater than 10 were considered significantly associated with the exposure. The F-statistic was calculated using the formula: F = [R2 / (1-R2) × (n-2), R2 = 2 × MAF × (1-MAF) × β2]. N represents the sample size, and MAF represents minor allele frequency. Weak IVs with F values <10 were excluded (23). Finally, MRPRESSO was employed to identify and remove outlier SNPs from the final set of IVs. Proxy SNPs were not included in this MR analysis.
For MR analysis, inverse variance weighting (IVW), weighted median, and MR-Egger were utilized as the primary analytical methods. IVW was employed as the main method, sequentially combining the Wald ratio estimates of each SNP to determine the overall causal effect (24). The weighted median and MR-Egger methods were used as complementary approaches to IVW. When the selected IVs comprise more than half of the total IVs, the weighted median method can reliably provide accurate estimates (25). MR-Egger is capable of detecting and adjusting for horizontal pleiotropy, though it has limited statistical power (26). Therefore, in this study, MR-Egger was applied primarily to test for pleiotropy rather than to evaluate causal effects.
The robustness of the results and potential biases were evaluated using sensitivity analysis methods in the MR study. Heterogeneity was assessed through Cochran’s Q test and funnel plots (27). To evaluate horizontal pleiotropy, the MR-Egger intercept test was conducted by calculating the intercept via linear regression analysis (28). The Leave-One-Out method was also employed, which involves removing individual SNPs and performing MR analysis to determine their influence on the outcomes (29). Additionally, MR-PRESSO was utilized to identify and exclude potential outliers and detect horizontal pleiotropy, thereby minimizing their impact on the causal effect assessment. If MR-PRESSO identified any outliers, the corresponding SNPs were excluded, and MR analysis was re-conducted. The excluded outliers are listed in Supplementary Table S10 (30).
To explore the biological pathways and mechanisms underlying risk genes associated with sarcopenia-related phenotypes, IS, and its subtypes, we performed enrichment analysis using Gene Ontology (GO) and the Kyoto Encyclopedia of Genes and Genomes (KEGG). GO analysis was used to assess gene enrichment within the categories of cellular components, biological processes, and molecular functions. KEGG analysis was conducted to identify gene enrichment in specific metabolic pathways, which is crucial for understanding genomic interactions.
Statistical analysis for this study was performed using R software (version 4.3.2). The TwoSampleMR (version 0.5.8) and MR-PRESSO (version 1.0) packages were applied for MR analysis, and the clusterProfiler (version 4.4.4) package was used for enrichment analysis.
Using sarcopenia-related traits as exposures, 617 SNPs for ALM, 140 SNPs for left-hand grip strength, 161 SNPs for right-hand grip strength, and 36 SNPs for usual walking pace were chosen as IVs (Supplementary Tables S1–S4) for MR analysis. For the reverse MR analysis, with IS and its subtypes as exposures, 7 SNPs for AIS, 3 SNPs for LAS, 4 SNPs for CES, and 0 SNPs for SVS were selected as IVs (Supplementary Tables S5–S7).
The IVW analysis revealed a negative correlation between ALM and SVS (OR = 0.790, 95% CI: 0.703–0.888, p < 0.001), while a positive correlation was observed between ALM and CES (OR = 1.165, 95% CI: 1.058–1.284, p = 0.002). No causal relationship was identified between ALM and either AIS or LAS. Additionally, MR analysis indicated that right-hand grip strength was negatively correlated with SVS (OR = 0.639, 95% CI: 0.437–0.934, p = 0.021), with no significant associations with AIS, LAS, or CES. Furthermore, no causal relationships were found between left-hand grip strength, usual walking pace, and IS or its subtypes. The analysis results are detailed in Table 2, with visual representations of the causal relationships provided in Figure 2. Cochran’s Q test, MR-Egger intercept test, and MR-PRESSO global test indicated no evidence of heterogeneity or horizontal pleiotropy (Table 3). The Supplementary Figure include scatter plots from IVW, MR-Egger, and weighted median methods, along with leave-one-out and funnel plots.
The MR results demonstrated a negative correlation between CES and usual walking pace (OR = 0.989, 95% CI: 0.980–0.998, p = 0.013) (Table 4). However, no significant causal relationships were observed between CES and either ALM or grip strength, as illustrated in Figure 3. Sensitivity analyses, including Cochran’s Q test, MR-Egger intercept test, and MR-PRESSO global test, revealed no evidence of heterogeneity or horizontal pleiotropy (Table 5). Furthermore, the MR analyses did not establish any causal association between AIS, LAS, and sarcopenia-related traits. The Supplementary Figure provide scatter plots from the IVW, MR-Egger, and weighted median methods, along with leave-one-out and funnel plots.
During the enrichment analysis between ALM and CES, right-hand grip strength and SVS, as well as between CES and walking speed, we identified a limited number of significant pathways. As a result, we focused the GO and KEGG enrichment analyses on the SNPs identified by ALM and SVS. The corresponding gene names are listed in Supplementary Table S11. GO analysis revealed that the mitotic cell cycle phase transition, G1/S transition of the mitotic cell cycle, and chondrocyte differentiation are associated with Molecular Function (MF). The transcription regulator complex is linked to Cellular Component (CC), while insulin receptor substrate binding and peptide hormone binding are related to Biological Process (BP). KEGG analysis indicates significant enrichment in pathways related to Human cytomegalovirus infection, Human immunodeficiency virus, and Viral carcinogenesis (Figure 4).
The study assessed the bidirectional causal relationship between sarcopenia and ischemic stroke (IS) using two-sample MR analysis. The results indicated that increased ALM was associated with a reduced risk of SVS but an elevated risk of CES. An inverse causal relationship was found between right-hand grip strength and SVS. Reverse MR analysis identified a correlation solely between CES and usual walking pace.
Sarcopenia and IS are prevalent conditions among the elderly, and some studies have identified a correlation between the two. A longitudinal study conducted over 3.6 years, which included approximately 15,000 Chinese individuals, revealed that after adjusting for confounding factors, the risk of stroke was significantly higher in patients with probable sarcopenia (HR = 1.59, 95% CI: 1.26–2.00, p < 0.001) or confirmed sarcopenia (HR = 1.67, 95% CI: 1.17–2.40, p < 0.01) (5). Furthermore, a meta-analysis involving 63,738,162 participants supported these findings, indicating an increased stroke risk in sarcopenia patients (OR = 1.67, 95% CI: 1.18–2.37, p = 0.004) (7). However, these studies did not examine the influence of sarcopenia on specific stroke subtypes. To address this gap, we expanded upon previous research by utilizing MR analysis to assess the causal effect of genetically predicted sarcopenia on different stroke subtypes.
Inflammation, metabolic disorders, physical inactivity associated with a sedentary lifestyle, hormonal changes related to aging, and endothelial dysfunction collectively contribute to the connection between sarcopenia and stroke. A sedentary lifestyle exacerbates metabolic disorders, endothelial dysfunction, and inflammation levels (31, 32). Insulin resistance and endothelial dysfunction have a reciprocal relationship (33), while the decline in testosterone and estrogen with age further accelerates endothelial dysfunction (34, 35). The interaction of these factors increases the risk of stroke. Sarcopenia is marked by a pro-inflammatory state driven by cytokines and oxidative stress (36), which also plays a significant pathogenic role in stroke (37, 38). Skeletal muscles secrete myokines in response to physical activity, which have anti-inflammatory effects (39). Enhancing muscle mass can promote myokine secretion to combat inflammation (40, 41). An eight-year study demonstrated a negative correlation between grip strength and inflammation (42), and another study found that individuals with low grip strength had elevated C-reactive protein (CRP) levels (OR = 1.60, 95% CI = 1.03–2.49) (43). CRP, as an inflammation marker, is closely associated with stroke risk. This research helps explain how the decline in muscle mass and strength may increase stroke risk through inflammatory pathways. However, our study suggests a more pronounced relationship between muscle issues and SVS, possibly due to inadequate collateral circulation in small arteries.
Additionally, research indicates that sarcopenia is an aging-related syndrome closely associated with immune function (44). This may suggest an increased risk of viral infections in elderly patients (45). Chronic or acute stimulation of the immune system by viral exposure can lead to coagulation dysfunction and subsequently induce IS (46–48). The GO and KEGG enrichment analysis in this study corroborate this finding.
We identified a positive correlation between ALM and CES using MR, which contrasts with the findings for SVS. Causes of CES include atrial fibrillation, heart failure, coronary artery disease, cardiomyopathy, valvular heart disease, and other related conditions (49). To date, only a few clinical studies have examined the relationship between muscle mass and these conditions (50–52), and no studies have specifically addressed CES. Based on our findings, one possible mechanism involves iron metabolism. Previous research has shown that reduced muscle mass is associated with iron deficiency (53). Chang et al. reported that individuals with thrombotic and embolic strokes had a higher incidence of prior iron deficiency diagnoses compared to healthy controls (54). However, this alone does not fully explain the paradoxical result. Enrichment analysis also failed to uncover an effective biological mechanism. Considering that CES primarily results from cardiac conditions, whereas SVS is caused by intrinsic lesions in small cerebral vessels, the differing etiologies may account for the observed outcome variations. Future research should explore the mechanisms linking muscle mass to IS subtypes.
Stroke can lead to systemic muscle mass loss and functional decline through various mechanisms, including prolonged bed rest, motor function loss, feeding difficulties, poor nutritional status, sympathetic activation, inflammatory responses, and nerve damage. These factors contribute to the onset and progression of sarcopenia, thereby increasing the overall burden on patients (55–58). The incidence of gait disorders due to neurological deficits from stroke is notably high and is typically characterized by reduced stride length, decreased walking speed, and impaired balance control. These impairments contribute to difficulties in daily activities and an increased risk of falls (59). Additionally, studies have shown that heart disease is associated with decreased walking speed (60, 61). In our MR study, cardiac causes of CES were found to be negatively correlated with usual walking pace, suggesting that these factors may synergistically contribute to gait disorders. However, due to the limited number of SNPs identified for stroke subtypes in our reverse MR analysis, our results have certain limitations. While our study found a significant relationship between CES and walking speed, the causal effect derived from the limited IVs may be relatively weak. Therefore, future studies should analyze and interpret causal relationships using larger GWAS datasets.
The MR study initially explored the causal relationships between sarcopenia-related traits and IS subtypes, yielding positive findings. Compared to traditional observational studies, MR research minimizes the influence of confounding factors, providing more robust results by accounting for heterogeneity and horizontal pleiotropy. However, several limitations exist. Firstly, unknown confounding factors may still be present, potentially influencing the outcomes. Secondly, the study population was exclusively of European descent, which limits the generalizability of the findings to other populations. Lastly, the proportion of IS subtypes varies across different racial groups, indicating the need for further studies to investigate causal effects in diverse populations.
In summary, our research utilized large-scale gene summary data to identify a bidirectional causal relationship between sarcopenia and IS. Specifically, sarcopenia indicators, such as ALM and right-hand grip strength, were negatively correlated with SVS, while ALM and CES assessment showed a positive correlation. Additionally, CES assessment was negatively correlated with usual walking pace. To further investigate the complex relationship between sarcopenia and stroke, additional studies involving diverse populations and experimental research are required to clarify the underlying mechanisms and improve prevention and treatment strategies.
The datasets presented in this study can be found in online repositories. The names of the repository/repositories and accession number(s) can be found in the article/Supplementary material.
Ethical review and approval was not required for the study on human participants in accordance with the local legislation and institutional requirements. Written informed consent from the patients/participants or patients/participants' legal guardian/next of kin was not required to participate in this study in accordance with the national legislation and the institutional requirements.
F-QM: Data curation, Methodology, Writing – original draft. YZ: Data curation, Methodology, Writing – original draft. X-XB: Data curation, Methodology, Writing – original draft. F-LK: Writing – review & editing, Methodology, Funding acquisition. F-EL: Methodology, Writing – review & editing.
The author(s) declare that financial support was received for the research, authorship, and/or publication of this article. This study was supported by funding from the Natural Science Foundation Project of Jilin Provincial Department of Science and Technology (20220101307JC) for F-LK, and by funding from the health science and technology ability improvement project of Jilin Province (2023LC026) and the Science and Technology Research Project of Education Department of Jilin Province (JJKH20240102KJ) for F-EL.
The authors thank the MEGASTROKE consortium and the UK Biobank study.
The authors declare that the research was conducted in the absence of any commercial or financial relationships that could be construed as a potential conflict of interest.
All claims expressed in this article are solely those of the authors and do not necessarily represent those of their affiliated organizations, or those of the publisher, the editors and the reviewers. Any product that may be evaluated in this article, or claim that may be made by its manufacturer, is not guaranteed or endorsed by the publisher.
The Supplementary material for this article can be found online at: https://www.frontiersin.org/articles/10.3389/fneur.2024.1427692/full#supplementary-material
1. Feigin, VL, Brainin, M, Norrving, B, Martins, S, Sacco, RL, Hacke, W, et al. World stroke organization (WSO): global stroke fact sheet 2022. Int J Stroke. (2022) 17:18–29. doi: 10.1177/17474930211065917
2. Wu, S, Wu, B, Liu, M, Chen, Z, Wang, W, Anderson, CS, et al. Stroke in China: advances and challenges in epidemiology, prevention, and management. Lancet Neurol. (2019) 18:394–405. doi: 10.1016/S1474-4422(18)30500-3
3. Global burden of 369 diseases and injuries in 204 countries and territories, 1990-2019: a systematic analysis for the global burden of disease study 2019. Lancet. (2020) 396:1204–22. doi: 10.1016/S0140-6736(20)30925-9
4. Global, regional, and national burden of stroke and its risk factors, 1990-2019: a systematic analysis for the global burden of disease study 2019. Lancet Neurol. (2021) 20:795–820. doi: 10.1016/S1474-4422(21)00252-0
5. Gao, K, Cao, LF, Ma, WZ, Gao, YJ, Luo, MS, Zhu, J, et al. Association between sarcopenia and cardiovascular disease among middle-aged and older adults: findings from the China health and retirement longitudinal study. EClinicalMedicine. (2022) 44:101264. doi: 10.1016/j.eclinm.2021.101264
6. Park, S, Ham, JO, and Lee, BK. A positive association between stroke risk and sarcopenia in men aged ≥ 50 years, but not women: results from the Korean National Health and nutrition examination survey 2008-2010. J Nutr Health Aging. (2014) 18:806–12. doi: 10.1007/s12603-014-0553-x
7. Fang, M, Liu, C, Liu, Y, Tang, G, Li, C, and Guo, L. Association between sarcopenia with incident cardio-cerebrovascular disease: a systematic review and meta-analysis. Biosci Trends. (2023) 17:293–301. doi: 10.5582/bst.2023.01130
8. Cai, X, Hu, J, Wang, M, Wen, W, Wang, J, Yang, W, et al. Association between the sarcopenia index and the risk of stroke in elderly patients with hypertension: a cohort study. Aging (Albany NY). (2023) 15:2005–32. doi: 10.18632/aging.204587
9. Aydin, T, Kesiktaş, FN, Oren, MM, Erdogan, T, Ahisha, YC, Kizilkurt, T, et al. Sarcopenia in patients following stroke: an overlooked problem. Int J Rehabil Res. (2021) 44:269–75. doi: 10.1097/MRR.0000000000000487
10. Mas, MF, González, J, and Frontera, WR. Stroke and sarcopenia. Curr Phys Med Rehabil Rep. (2020) 8:452–60. doi: 10.1007/s40141-020-00284-2
11. Su, Y, Yuki, M, and Otsuki, M. Prevalence of stroke-related sarcopenia: a systematic review and meta-analysis. J Stroke Cerebrovasc Dis. (2020) 29:105092. doi: 10.1016/j.jstrokecerebrovasdis.2020.105092
12. Rosenberg, IH. Sarcopenia: origins and clinical relevance. J Nutr. (1997) 127:990s–1s. doi: 10.1093/jn/127.5.990S
13. Hida, T, Harada, A, Imagama, S, and Ishiguro, N. Managing sarcopenia and its related-fractures to improve quality of life in geriatric populations. Aging Dis. (2014) 5:226–37. doi: 10.14336/AD.2014.0500226
14. Cruz-Jentoft, AJ, and Sayer, AA. Sarcopenia. Lancet. (2019) 393:2636–46. doi: 10.1016/S0140-6736(19)31138-9
15. Cruz-Jentoft, AJ, Baeyens, JP, Bauer, JM, Boirie, Y, Cederholm, T, Landi, F, et al. Sarcopenia: European consensus on definition and diagnosis: report of the European working group on sarcopenia in older people. Age Ageing. (2010) 39:412–23. doi: 10.1093/ageing/afq034
16. Cruz-Jentoft, AJ, Bahat, G, Bauer, J, Boirie, Y, Bruyère, O, Cederholm, T, et al. Sarcopenia: revised European consensus on definition and diagnosis. Age Ageing. (2019) 48:16–31. doi: 10.1093/ageing/afy169
17. Emdin, CA, Khera, AV, and Kathiresan, S. Mendelian Randomization. JAMA. (2017) 318:1925–6. doi: 10.1001/jama.2017.17219
18. Davies, NM, Holmes, MV, and Davey, SG. Reading Mendelian randomisation studies: a guide, glossary, and checklist for clinicians. BMJ. (2018) 362:k601. doi: 10.1136/bmj.k601
19. Pei, YF, Liu, YZ, Yang, XL, Zhang, H, Feng, GJ, Wei, XT, et al. The genetic architecture of appendicular lean mass characterized by association analysis in the UK Biobank study. Commun Biol. (2020) 3:608. doi: 10.1038/s42003-020-01334-0
20. Mitchell, RE, Elsworth, BL, Mitchell, R, Raistrick, CA, Paternoster, L, Hemani, G, et al. MRC IEU UK Biobank GWAS pipeline version 2.. Univ Bristol. (2019) doi: 10.5523/bris.pnoat8cxo0u52p6ynfaekeigi
21. Malik, R, Chauhan, G, Traylor, M, Sargurupremraj, M, Okada, Y, Mishra, A, et al. Multiancestry genome-wide association study of 520,000 subjects identifies 32 loci associated with stroke and stroke subtypes. Nat Genet. (2018) 50:524–37. doi: 10.1038/s41588-018-0058-3
22. Staley, JR, Blackshaw, J, Kamat, MA, Ellis, S, Surendran, P, Sun, BB, et al. PhenoScanner: a database of human genotype-phenotype associations. Bioinformatics. (2016) 32:3207–9. doi: 10.1093/bioinformatics/btw373
23. Burgess, S, and Thompson, SG. Avoiding bias from weak instruments in Mendelian randomization studies. Int J Epidemiol. (2011) 40:755–64. doi: 10.1093/ije/dyr036
24. Lawlor, DA, Harbord, RM, Sterne, JA, Timpson, N, and Davey, SG. Mendelian randomization: using genes as instruments for making causal inferences in epidemiology. Stat Med. (2008) 27:1133–63. doi: 10.1002/sim.3034
25. Bowden, J, Davey Smith, G, Haycock, PC, and Burgess, S. Consistent estimation in Mendelian randomization with some invalid instruments using a weighted median estimator. Genet Epidemiol. (2016) 40:304–14. doi: 10.1002/gepi.21965
26. Bowden, J, Davey Smith, G, and Burgess, S. Mendelian randomization with invalid instruments: effect estimation and bias detection through egger regression. Int J Epidemiol. (2015) 44:512–25. doi: 10.1093/ije/dyv080
27. Bowden, J, Del Greco, MF, Minelli, C, Zhao, Q, Lawlor, DA, Sheehan, NA, et al. Improving the accuracy of two-sample summary-data Mendelian randomization: moving beyond the NOME assumption. Int J Epidemiol. (2018) 48:728–42. doi: 10.1093/ije/dyy258
28. Burgess, S, and Thompson, SG. Interpreting findings from Mendelian randomization using the MR-egger method. Eur J Epidemiol. (2017) 32:377–89. doi: 10.1007/s10654-017-0255-x
29. Gronau, QF, and Wagenmakers, EJ. Limitations of Bayesian leave-one-out cross-validation for model selection. Comput Brain Behav. (2018) 2:1–11. doi: 10.1007/s42113-018-0011-7
30. Verbanck, M, Chen, CY, Neale, B, and Do, R. Detection of widespread horizontal pleiotropy in causal relationships inferred from Mendelian randomization between complex traits and diseases. Nat Genet. (2018) 50:693–8. doi: 10.1038/s41588-018-0099-7
31. Carter, S, Hartman, Y, Holder, S, Thijssen, DH, and Hopkins, ND. Sedentary behavior and cardiovascular disease risk: mediating mechanisms. Exerc Sport Sci Rev. (2017) 45:80–6. doi: 10.1249/JES.0000000000000106
32. Healy, GN, Wijndaele, K, Dunstan, DW, Shaw, JE, Salmon, J, Zimmet, PZ, et al. Objectively measured sedentary time, physical activity, and metabolic risk: the Australian diabetes, obesity and lifestyle study (AusDiab). Diabetes Care. (2008) 31:369–71. doi: 10.2337/dc07-1795
33. Perticone, M, Maio, R, Gigliotti, S, Shehaj, E, Toscani, AF, Capomolla, A, et al. Mutual effect modification between insulin resistance and endothelial dysfunction in predicting incident heart failure in Hypertensives. Biomedicines. (2023) 11:218. doi: 10.3390/biomedicines11082188
34. Babcock, MC, DuBose, LE, Witten, TL, Stauffer, BL, Hildreth, KL, Schwartz, RS, et al. Oxidative stress and inflammation are associated with age-related endothelial dysfunction in men with Low testosterone. J Clin Endocrinol Metab. (2022) 107:e500–14. doi: 10.1210/clinem/dgab715
35. Lv, Y, Zhang, S, Weng, X, Huang, J, Zhao, H, Dai, X, et al. Estrogen deficiency accelerates postmenopausal atherosclerosis by inducing endothelial cell ferroptosis through inhibiting NRF2/GPX4 pathway. FASEB J. (2023) 37:e22992. doi: 10.1096/fj.202300083R
36. Jensen, GL. Inflammation: roles in aging and sarcopenia. JPEN J Parenter Enteral Nutr. (2008) 32:656–9. doi: 10.1177/0148607108324585
37. Low, A, Mak, E, Rowe, JB, Markus, HS, and O'Brien, JT. Inflammation and cerebral small vessel disease: a systematic review. Ageing Res Rev. (2019) 53:100916. doi: 10.1016/j.arr.2019.100916
38. Regenhardt, RW, Das, AS, Lo, EH, and Caplan, LR. Advances in understanding the pathophysiology of lacunar stroke: a review. JAMA Neurol. (2018) 75:1273–81. doi: 10.1001/jamaneurol.2018.1073
39. Gonzalez-Gil, AM, and Elizondo-Montemayor, L. The role of exercise in the interplay between Myokines, Hepatokines, Osteokines, Adipokines, and modulation of inflammation for energy substrate redistribution and fat mass loss: a review. Nutrients. (2020) 12:1899. doi: 10.3390/nu12061899
40. Minn, YK, and Suk, SH. Higher skeletal muscle mass may protect against ischemic stroke in community-dwelling adults without stroke and dementia: the PRESENT project. BMC Geriatr. (2017) 17:45. doi: 10.1186/s12877-017-0433-4
41. Benatti, FB, and Pedersen, BK. Exercise as an anti-inflammatory therapy for rheumatic diseases-myokine regulation. Nat Rev Rheumatol. (2015) 11:86–97. doi: 10.1038/nrrheum.2014.193
42. Smith, L, Yang, L, and Hamer, M. Handgrip strength, inflammatory markers, and mortality. Scand J Med Sci Sports. (2019) 29:1190–6. doi: 10.1111/sms.13433
43. Liu, G, Xue, Y, Wang, S, Zhang, Y, and Geng, Q. Association between hand grip strength and stroke in China: a prospective cohort study. Aging (Albany NY). (2021) 13:8204–13. doi: 10.18632/aging.202630
44. Zhang, X, Li, H, He, M, Wang, J, Wu, Y, and Li, Y. Immune system and sarcopenia: presented relationship and future perspective. Exp Gerontol. (2022) 164:111823. doi: 10.1016/j.exger.2022.111823
45. Jia, H, Huang, W, Liu, C, Tang, S, Zhang, J, Chen, C, et al. Immunosenescence is a therapeutic target for frailty in older adults: a narrative review. Ann Transl Med. (2022) 10:1142. doi: 10.21037/atm-22-4405
46. Yang, Y, and Tang, H. Aberrant coagulation causes a hyper-inflammatory response in severe influenza pneumonia. Cell Mol Immunol. (2016) 13:432–42. doi: 10.1038/cmi.2016.1
47. Perkins, MV, Joseph, SB, Dittmer, DP, and Mackman, N. Cardiovascular disease and thrombosis in HIV infection. Arterioscler Thromb Vasc Biol. (2023) 43:175–91. doi: 10.1161/ATVBAHA.122.318232
48. Nagel, MA, Mahalingam, R, Cohrs, RJ, and Gilden, D. Virus vasculopathy and stroke: an under-recognized cause and treatment target. Infect Disord Drug Targets. (2010) 10:105–11. doi: 10.2174/187152610790963537
49. Amarenco, P, Bogousslavsky, J, Caplan, LR, Donnan, GA, and Hennerici, MG. New approach to stroke subtyping: the A-S-C-O (phenotypic) classification of stroke. Cerebrovasc Dis. (2009) 27:502–8. doi: 10.1159/000210433
50. Camm, CF, Lacey, B, Massa, MS, Von Ende, A, Gajendragadkar, P, Stiby, A, et al. Independent effects of adiposity measures on risk of atrial fibrillation in men and women: a study of 0.5 million individuals. Int J Epidemiol. (2022) 51:984–95. doi: 10.1093/ije/dyab184
51. Knowles, R, Carter, J, Jebb, SA, Bennett, D, Lewington, S, and Piernas, C. Associations of skeletal muscle mass and fat mass with incident cardiovascular disease and all-cause mortality: a prospective cohort study of UK Biobank participants. J Am Heart Assoc. (2021) 10:e019337. doi: 10.1161/JAHA.120.019337
52. Kim, SR, Lee, G, Choi, S, Oh, YH, Son, JS, Park, M, et al. Changes in predicted lean body mass, appendicular skeletal muscle mass, and body fat mass and cardiovascular disease. J Cachexia Sarcopenia Muscle. (2022) 13:1113–23. doi: 10.1002/jcsm.12962
53. Vinke, JSJ, Gorter, AR, Eisenga, MF, Dam, WA, van der Meer, P, van den Born, J, et al. Iron deficiency is related to lower muscle mass in community-dwelling individuals and impairs myoblast proliferation. J Cachexia Sarcopenia Muscle. (2023) 14:1865–79. doi: 10.1002/jcsm.13277
54. Chang, YL, Hung, SH, Ling, W, Lin, HC, Li, HC, and Chung, SD. Association between ischemic stroke and iron-deficiency anemia: a population-based study. PLoS One. (2013) 8:e82952. doi: 10.1371/journal.pone.0082952
55. Li, W, Yue, T, and Liu, Y. New understanding of the pathogenesis and treatment of stroke-related sarcopenia. Biomed Pharmacother. (2020) 131:110721. doi: 10.1016/j.biopha.2020.110721
56. Yoshimura, Y, Bise, T, Nagano, F, Shimazu, S, Shiraishi, A, Yamaga, M, et al. Systemic inflammation in the recovery stage of stroke: its association with sarcopenia and poor functional rehabilitation outcomes. Prog Rehabil Med. (2018) 3:20180011. doi: 10.2490/prm.20180011
57. Li, S, Gonzalez-Buonomo, J, Ghuman, J, Huang, X, Malik, A, Yozbatiran, N, et al. Aging after stroke: how to define post-stroke sarcopenia and what are its risk factors? Eur J Phys Rehabil Med. (2022) 58:683–92. doi: 10.23736/S1973-9087.22.07514-1
58. Scherbakov, N, Sandek, A, and Doehner, W. Stroke-related sarcopenia: specific characteristics. J Am Med Dir Assoc. (2015) 16:272–6. doi: 10.1016/j.jamda.2014.12.007
59. Stolze, H, Klebe, S, Baecker, C, Zechlin, C, Friege, L, Pohle, S, et al. Prevalence of gait disorders in hospitalized neurological patients. Mov Disord. (2005) 20:89–94. doi: 10.1002/mds.20266
60. Marino, FR, Lessard, DM, Saczynski, JS, McManus, DD, Silverman-Lloyd, LG, Benson, CM, et al. Gait speed and mood, cognition, and quality of life in older adults with atrial fibrillation. J Am Heart Assoc. (2019) 8:e013212. doi: 10.1161/JAHA.119.013212
Keywords: ischemic stroke, sarcopenia, Mendelian randomization, causality, genetics
Citation: Meng F-Q, Zhang Y, Bai X-X, Kong F-L and Li F-E (2024) Ischemic stroke and sarcopenia have an asymmetric bidirectional relationship based on a two-sample Mendelian randomization study. Front. Neurol. 15:1427692. doi: 10.3389/fneur.2024.1427692
Received: 04 May 2024; Accepted: 26 September 2024;
Published: 09 October 2024.
Edited by:
Jean-Claude Baron, University of Cambridge, United KingdomReviewed by:
Stefan Gerner, University Medical Center Giessen, GermanyCopyright © 2024 Meng, Zhang, Bai, Kong and Li. This is an open-access article distributed under the terms of the Creative Commons Attribution License (CC BY). The use, distribution or reproduction in other forums is permitted, provided the original author(s) and the copyright owner(s) are credited and that the original publication in this journal is cited, in accordance with accepted academic practice. No use, distribution or reproduction is permitted which does not comply with these terms.
*Correspondence: Feng-E Li, MTA1OTI1MDYwOUBxcS5jb20=; Fan-Li Kong, a29uZ2ZhbmxpNUAxNjMuY29t
Disclaimer: All claims expressed in this article are solely those of the authors and do not necessarily represent those of their affiliated organizations, or those of the publisher, the editors and the reviewers. Any product that may be evaluated in this article or claim that may be made by its manufacturer is not guaranteed or endorsed by the publisher.
Research integrity at Frontiers
Learn more about the work of our research integrity team to safeguard the quality of each article we publish.