- 1Department of Neurology, The First Hospital of Jilin University, Changchun, China
- 2Department of Neurosurgery, Northern Jiangsu People's Hospital, Clinical Medical College of Yangzhou University, Yangzhou, China
- 3Department of Vascular Ultrasonography, Xuanwu Hospital, Capital Medical University, Beijing, China
- 4Beijing Diagnostic Center of Vascular Ultrasound, Beijing, China
- 5Department of Neurology, Linyi People's Hospital, Linyi, China
- 6Department of Neurology, Changchun People's Hospital, Changchun, China
Background: We aimed to explore whether transcranial Doppler (TCD) combined with quantitative electroencephalography (QEEG) can improve prognosis evaluation in patients with a large hemispheric infarction (LHI) and to establish an accurate prognosis prediction model.
Methods: We prospectively assessed 90-day mortality in patients with LHI. Brain function was monitored using TCD-QEEG at the bedside of the patient.
Results: Of the 59 (55.3 ± 10.6 years; 17 men) enrolled patients, 37 (67.3%) patients died within 90 days. The Cox regression analyses revealed that the Glasgow Coma Scale (GCS) score ≤ 8 [hazard ratio (HR), 3.228; 95% CI, 1.335–7.801; p = 0.009], TCD-terminal internal carotid artery as the offending vessel (HR, 3.830; 95% CI, 1.301–11.271; p = 0.015), and QEEG-a (delta + theta)/(alpha + beta) ratio ≥ 3 (HR, 3.647; 95% CI, 1.170–11.373; p = 0.026) independently predicted survival duration. Combining these three factors yielded an area under the receiver operating characteristic curve of 0.905 and had better predictive accuracy than those of individual variables (p < 0.05).
Conclusion: TCD and QEEG complement the GCS score to create a reliable multimodal method for monitoring prognosis in patients with LHI.
Introduction
Large hemispheric infarction (LHI) is a devastating disease associated with high mortality and poor outcomes (1). Severe neurological deficit, disturbance of consciousness, and even cerebral hernia often occur in the early stage of the disease and the mortality rate is as high as 80% in patients receiving conservative treatment (2, 3). Imaging examination can help to identify patients with LHI and facilitate monitoring disease progression (4). However, the complexity and uncertainty of condition of the patients do not allow frequent reexamination and serial imaging might be difficult to perform in critically ill patients (5, 6). Establishing methods to identify the brain functional status of patients with LHI at the bedside, in a non-invasive and objective way in the early stage of disease, and identifying the factors predicting poor prognosis in patients with LHI are urgent clinical goals.
Large hemispheric infarction is usually caused by acute occlusion of the internal carotid artery (ICA) or middle cerebral artery (MCA). The brain is critically dependent on the continuous delivery of oxygen and glucose via the blood flow (7). Acute vascular occlusion interrupts the blood supply to the brain and can cause irreparable brain damage, resulting in cell death due to energy failure (8). As a rapid and non-invasive blood flow examination method (9, 10), transcranial Doppler (TCD) can monitor changes in the blood flow spectral waveforms, blood flow direction and velocity, and the pulsatility index (PI), allowing evaluation of the offending vessels and collateral branches (11, 12); TCD has been widely used in patients with ischemic stroke. Compared with diagnosis using cerebral angiography, the diagnosis of intracranial ICA and MCA mainstem occlusion using TCD showed a sensitivity and specificity of 95.0 and 92.0%, respectively (13). TCD plays an important role in the evaluation of patients with LHI due to its unique ability to evaluate hemodynamics in real time (14, 15).
In addition, in patients with a poor prognosis of LHI, the ischemic core region is significantly larger, cerebral blood perfusion is worse, and irreversible neuronal damage is more serious (16). Electroencephalograms (EEGs) can reflect changes in the metabolism and electrical activity of cortical neurons (17). When cerebral blood flow (CBF) becomes compromised, the EEG shows a loss of fast frequencies and an increase in slow frequencies. As CBF continues to decline toward the infarct threshold, irreversible damage occurs in neurons and the EEG becomes silent (18–20). Quantitative EEGs (QEEGs) transform the basic elements of EEGs into various quantitative parameters, being more objective and simpler than traditional EEGs for assessing brain damage in patients (21, 22). Most QEEG indicators [including relative alpha, theta, delta power, delta/alpha ratio (DAR), the (delta + theta)/(alpha + beta) ratio (DTABR), and brain symmetry index (BSI)] have been reported to be significantly associated with patient outcomes (23–27). These parameters showed a better prediction effect than the National Institutes of Health Stroke Scale (NIHSS) and ischemic volume (28, 29). The past two decades have seen rapid development in QEEG for the assessment of ischemic stroke outcomes and QEEG is considered as a powerful tool for prognostic prediction (30, 31).
The combination of TCD and QEEG as a new multimodality monitoring method is of major interest, as together, they describe stroke-related blood perfusion injury and neuronal function damage. In this study, we sought to explore whether it is possible to obtain more accurate and comprehensive prognostic predictors for patients with LHI by TCD-QEEG. Identifying such predictors is pivotal for developing risk-optimized treatment strategies and deciding on healthcare resource allocation.
Materials and Methods
Subjects
We conducted a prospective observational study between July 2018 and December 2019. Patients with LHI admitted to the Department of Neurology, First Hospital of Jilin University, China, were eligible for enrollment. The inclusion criteria were as follows: (1) admission time ≤ 72 h after LHI onset; (2) presence of LHI as confirmed by an experienced neurologist; (3) CT and/or MRI documenting infarction in at least two-thirds of the unilateral MCA territory and at least part of the basal ganglia, with or without additional ipsilateral infarction of the anterior or posterior cerebral artery (32); and (4) carotid ultrasound and TCD examination. The exclusion criteria were as follows: (1) patients who dropped out of the study; (2) inaccessible ultrasound windows; (3) intravascular thrombolysis or stent therapy; (4) significant contralateral infarction; (5) space-occupying hemorrhagic transformation of the infarct; (6) surgical decompression; and (7) intake of central nervous system depressants including sedatives, narcotics, antidepressants, antipsychotics, and antiepileptics. We also recruited 29 healthy age- and sex-matched controls (62 ± 10 years; 20 males), with normal brain MRI findings, no metabolic derangements, and normal central nervous system functioning.
This study was approved by the Ethics Committee of the First Hospital of Jilin University, China (NO. 2018-406) and conformed to the tenets of the Declaration of Helsinki. A written informed consent was obtained from the participants or next of kin of the participants before the commencement of this study.
Clinical Data
The following variables of patients admitted to the neurological intensive care unit were recorded during monitoring: demographic characteristics, risk factors, vital signs, laboratory indices, the Glasgow Coma Scale (GCS) score, the NIHSS score, infarction side, and time from onset to monitoring. All the patients were treated according to the Guidelines for the Early Management of Patients with Acute Ischemic Stroke 2019 of the American Heart Association/American Stroke Association and received regular clinical care and postdischarge guidance (33).
Patients were followed-up 90 days after stroke onset by telephonic interview. The modified Rankin Scale score was used to determine the prognoses of the patients. The survival duration after disease onset was also recorded, if the patient had died.
Non-Invasive Vascular Ultrasound Examination
The TCD criteria for the diagnosis of lesions at different locations were based on the criteria published by the European Society of Neurosonology and Cerebral Hemodynamics (34). When the site of severe stenosis or occlusion was located in the MCA, the primary TCD criterion was a blood flow spectrum in line with the thrombolysis in brain ischemia (TIBI) grades 0–4 at depths of < 45 mm (M2 segment) and 45–65 mm (M1 segment). For the detection of severe stenosis or occlusion in the terminal ICA (TICA), the primary TCD criteria included: (1) a blood flow spectrum in line with the TIBI grades 0–4 at a depth of 60–70 mm and (2) cross-filling of the anterior communicating artery (ACoA) or collateral flow in the posterior communicating artery (PCoA) indicated by increased velocity and carotid compression tests. When the site of severe stenosis or occlusion was located in the proximal ICA, the primary TCD criteria included: (1) cross-filling of the ACoA or collateral flow in the PCoA indicated by increased velocity and carotid compression tests; (2) reversed ophthalmic artery (OA) flow; and (3) delayed systolic flow acceleration, or blunted ipsilateral MCA, and mean flow velocity > 20 cm/s.
The TCD examination was performed using an EMS-9A (Delica, Shenzhen, China) device. To improve accuracy, the TCD-based diagnosis was referenced to the results of carotid ultrasound performed using the Philips CX50 ultrasound machine (Philips, Bothell, Washington, USA) (35).
Transcranial Doppler-QEEG Measurements
Quantitative brain function monitoring using NSD version 1.0 (NSD-8100; Delica, Birsfelden, Switzerland) was performed with patients in the supine position and the head of the bed raised by 15–30°. TCD was performed using 2-Hz pulsed-wave probes fixed to each of the temporal windows of the patient via a helmet. A depth of 50–60 mm was used to acquire optimal MCA signals. Simultaneously, EEG was performed using Ag/silver chloride (AgCl) scalp electrodes positioned at 16 sites defined by the International 10–20 system, which uses 16 channels (Fp1, Fp2, F3, F4, F7, F8, C3, C4, T3, T4, T5, T6, P3, P4, O1, and O2) with the frontal central zero (Fz) electrode pole used for grounding and Cz, A1, and A2 used as references. The background EEG signal acquisition parameters were set as follows: time baseline, 30 mm/s; sensitivity, 10 μv/mm; electrode impedance maintained below 10 KΩ; sampling frequency, 250 Hz; and data filtering (high pass, 0.3 Hz; low pass, 30 Hz) (36). Each participant rested with their eyes closed until clear and stable data were achieved and data were then recorded for over 30 min. Every participant underwent monitoring only once.
Transcranial Doppler-QEEG Original Data and Data Analysis
All the clearly readable TCD waveforms were used in the calculations. The following variables of flow velocity were analyzed from the TCD-QEEG machine: systolic flow velocity (Vs), diastolic flow velocity (Vd), mean velocity (Vm), and PI from the affected and unaffected hemispheres. The flow velocity of the MCA was classified into five stages according to TIBI grades 0–4 for further statistical analysis (37).
Electroencephalography artifact interpretation was performed independently by two physicians qualified in EEG interpretation. Artifact-free 5-min EEG segments were selected manually and quantitatively analyzed offline using MATLAB (MathWorks, Natick, Massachusetts, USA). The power spectral density was calculated using Fast Fourier transform for each electrode over the 1–30 Hz range. Power spectral density was calculated using Welch's averaged, modified periodogram spectral estimation method, with a 2-s Hamming window and 50% overlap. The frequency resolution was 0.5 Hz. From the resulting power spectra for each electrode, the absolute band power was summed across the delta (1–3 Hz), theta (4–7 Hz), alpha (8–13 Hz), and beta (14–30 Hz) bands (inclusive). Relative band power [relative delta power (RDP), relative theta power (RTP), relative alpha power (RAP), and relative beta power (RBP)] were calculated as the ratio of the summed absolute band power to the total summed power across the 1–30 Hz range (36). DAR and DTABR were calculated as the ratio of absolute power for the respective frequency bands of interest. The BSI was calculated as reported in previous studies (38, 39). Statistical differences in the above indicators among healthy controls, survivors, and non-survivors were analyzed.
Statistical Analysis
A blinded analysis was performed for each patient. Data are reported as means and SDs of normally distributed variables and as medians and interquartile ranges (IQRs) for non-normally distributed variables. Categorical variables are presented as percentages. The Student's t-test was used for normally distributed variables. Non-parametric tests were used for non-normally distributed variables. Categorical variables were compared using the chi-squared test. After a preliminary analysis of prognoses of the patient, a survival analysis was performed using the duration of survival at 90 days after LHI onset. The parameters that had stronger correlations with the outcome under investigation were dichotomized for further analysis. Consequently, statistically significant (p < 0.05) parameters in the above analysis were entered into the Cox proportional-hazards model. The most economical model was obtained by backward stepwise elimination of the non-significant factors. The significance of variables in the multivariate models was evaluated using the Kaplan–Meier curves with log-rank tests. The Kaplan–Meier curves were plotted using the survminer package of RStudio (RStudio Incorporation, Boston, Massachusetts, USA) (https://CRAN.R-project.org/package=survminer). Moreover, receiver operating characteristic (ROC) curve analysis was used to assess the predictive ability of variables in the final model based on the area under the ROC curve (AUROC). The ROC curves were compared using DeLong's test.
All the statistical testing was two-tailed and a p < 0.05 was considered as statistically significant. Statistical analyses were performed using the SPSS version 22.0 (SPSS Incorporation, Chicago, Illinois, USA), RStudio version 1.2.1335 (RStudio Incorporation, Boston, Massachusetts, USA), and MedCalc version 19.0.7 (MedCalc Software, Mariakerke, Belgium, UK).
Results
Baseline Demographics
A total of 70 patients were originally recruited. Among them, 11 patients were excluded-−2 patients were lost to follow-up, 4 patients had disturbed EEG data, and 5 patients had poor penetration in the temporal window. The remaining 59 patients with LHI (63 ± 11.2 years; 40 males) were included in this study. Of these, 37 (67.3%) patients died by the 90-day follow-up. Characteristics of the patient are shown in Table 1. No significant differences were observed between survivors and non-survivors in terms of demographics or risk factors. With respect to the indicators of vital signs, heart rate (p = 0.010), respiratory rate (p = 0.013), and oxygen saturation (p = 0.021) differed significantly according to patient outcomes. The GCS (p = 0.001) and the NIHSS scores (p < 0.001) were significantly associated with mortality.
Non-Invasive Vascular Ultrasound Examination
As shown in Table 2, prognosis correlated significantly with the offending vessel (p = 0.005). Among the patients who had died, the offending vessel was most often the TICA [7 of 8 (87.5%) patients], followed by the MCA [8 of 22 (36.4%) patients], and the proximal ICA [7 of 29 (24.1%) patients]. Thus, the TICA as the offending vessel was strongly associated with the mortality rate.
Table 2 shows the results of statistical analysis for the involvement of collateral vessels in ICA stenosis/occlusion. There were no significant differences between groups according to the presence or absence of collateral circulation, the presence or absence of the ACoA, or the PCoA compensated through the circle of Willis. Importantly, of the 23 patients who survived 90 days, 10 patients had a collateral branch of the OA. In contrast, only 1 patient among the 14 patients who died within 90 days had a collateral branch of the OA. The presence of the collateral branch of the OA was significantly associated with mortality (p = 0.027).
Original TCD-QEEG Data
Figure 1 shows the CT and TCD-QEEG images of representative patients.
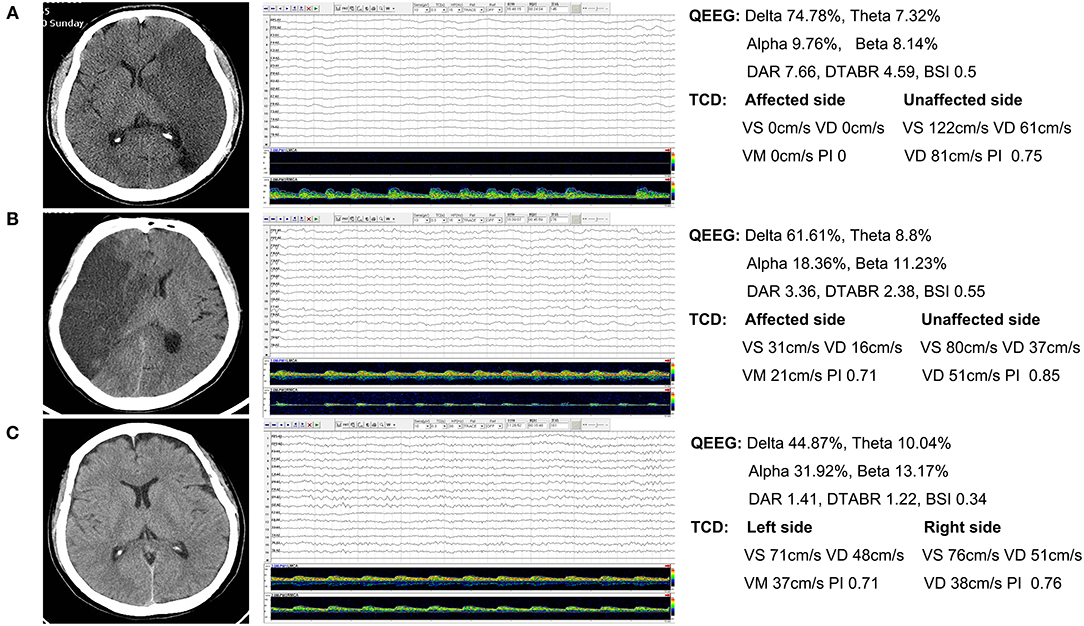
Figure 1. Representative patients. (A) Nonsurvivor: QEEG image shows that the slower delta frequency band is significantly increased and the faster alpha frequency band is significantly decreased. The DAR and DTABR are increased. The TCD image shows that the flow velocity of the affected side is notably decreased. (B) Survivor: QEEG and TCD images show similar changes. (C) Healthy control: QEEG and TCD images show normal findings. QEEG, quantitative electroencephalography; DAR, delta/alpha ratio; DTABR, (delta + theta)/(alpha + beta) ratio; BSI, brain symmetry index; TCD, transcranial Doppler; Vs, systolic flow velocity; Vd, diastolic flow velocity; Vm, mean velocity; PI, pulsatility index.
Figure 2 and Table 3 show the Vs, Vd, Vm, and PI of the MCA on TCD. On the affected side, the Vs, Vd, and Vm were lower in patients than in the healthy controls (p < 0.05). On the unaffected side, the Vs, Vm, and PI were higher in the patients (p < 0.05). Non-survivors and survivors did not show significant differences in the aforementioned indicators. Blood flow velocity in the MCA, which was further classified into five stages according to the TIBI grades (0–4), did not significantly affect the clinical outcomes of the patients.
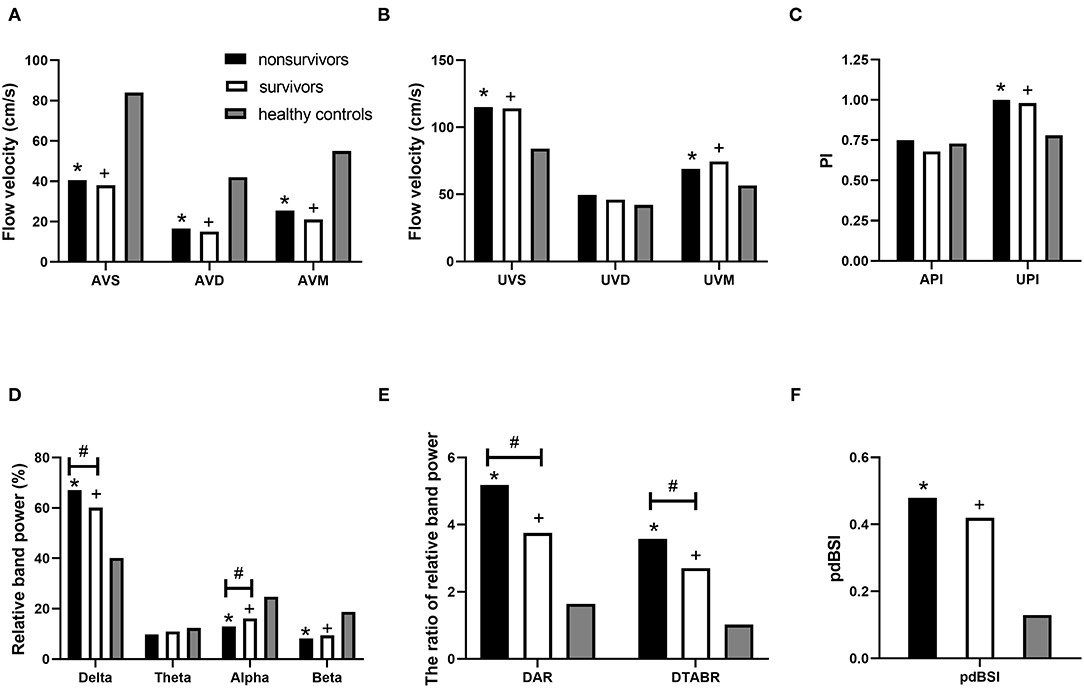
Figure 2. TCD-QEEG parameters for nonsurvivors, survivors, and healthy controls. TCD parameters: (A) AVS, AVM, and AVD; (B) UVS, UVM, and UVD; and (C) API and UPI. QEEG parameters: (D) relative delta power, relative theta power, relative alpha power, and relative beta power; (E) DAR and DTABR; and (F) BSI. *p < 0.05 for nonsurvivors vs. healthy controls; +p < 0.05 for survivors vs. healthy controls; #p < 0.05 for nonsurvivors vs. survivors. API, affected pulsatility index; AVD, affected diastolic flow velocity; AVM, affected mean flow velocity; AVS, affected hemisphere systolic flow velocity; DAR, delta/alpha ratio; DTABR, (delta + theta)/(alpha + beta) ratio; BSI, brain symmetry index; QEEG, quantitative electroencephalography; TCD, transcranial Doppler; UPI, unaffected pulsatility index; UVD, unaffected diastolic flow velocity; UVM, unaffected mean flow velocity; UVS, unaffected hemisphere systolic flow velocity.
Figure 2 and Table 3 list the QEEG indicators. RDP, DAR, DTABR, and BSI were higher, while RAP and RBP were lower in patients than in healthy controls (p < 0.05). In both the non-survivors and survivors, higher RDP, DAR, and DTABR and lower RAP were associated with mortality (p < 0.05).
Survival Analysis
The Cox proportional-hazards model incorporated all the variables with p < 0.05 from the above analyses. With respect to QEEG variables, DAR and DTABR were selected for further analysis: patients were divided into two groups based on a threshold DAR of 4.0 or a threshold DTABR of 3.0. The final Cox proportional-hazards model incorporated the GCS score, the offending vessel, and DTABR. As shown in Figure 3, the GCS score of ≤ 8 [hazard ratio (HR), 3.228; 95% CI, 1.335–7.801; p = 0.009], the TICA as the offending vessel (HR, 3.830; 95% CI, 1.301–11.271; p = 0.015), and DTABR ≥ 3 (HR, 3.647; 95% CI, 1.170–11.373; p = 0.026) are the three independent indicators of survival duration identified.
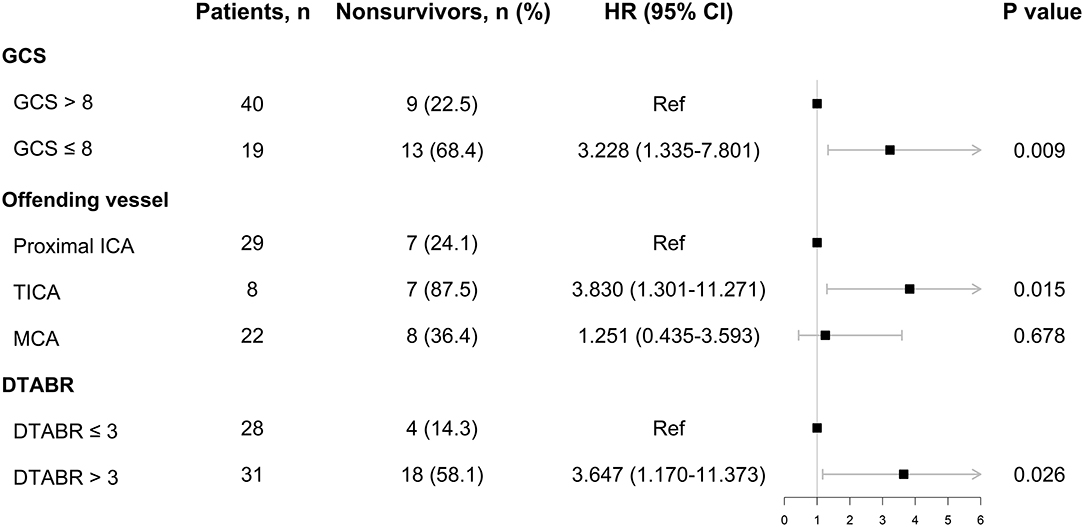
Figure 3. The Cox proportional-hazards model. DTABR, (delta + theta)/(alpha + beta) ratio; GCS, Glasgow Coma Scale; HR, hazard ratio; ICA, internal carotid artery; MCA, middle cerebral artery; TICA, terminal ICA.
The Kaplan–Meier curves were plotted for the GCS score, the offending vessels, and DTABR (Figure 4; log-rank p < 0.001, p = 0.001, and p < 0.001, respectively). The median survival time in those with the GCS ≤ 8 was 44 days, with TICA as the offending vessel was 12 days, and with a DTABR > 3 was 55 days.
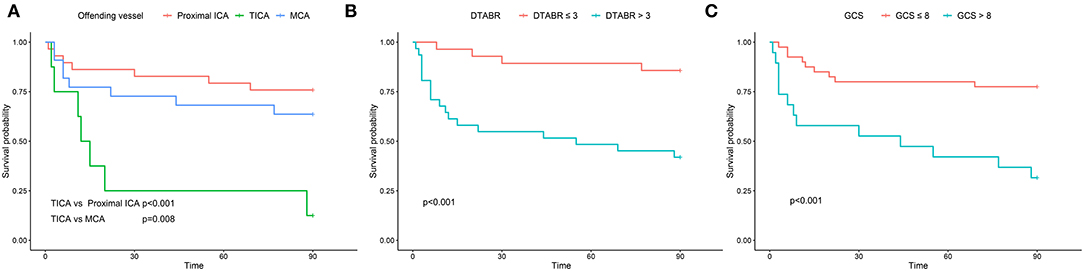
Figure 4. The Kaplan–Meier survival curves for the training cohort. (A) The Kaplan-Meier survival curves for the offending vessel. (B) The Kaplan-Meier survival curves for DTABR. (C) The Kaplan-Meier survival curves for GCS. ICA, internal carotid artery; TICA, terminal internal carotid artery; MCA, middle cerebral artery; DTABR, (delta + theta)/(alpha + beta) ratio; GCS, Glasgow Coma Scale.
Receiver Operating Characteristic Curves
To determine whether TCD-QEEG variables combined with the GCS score in the model improved outcome prediction, we compared the ROC curves for four models. The first model was obtained using the GCS score, the second model was obtained using the TCD (the offending vessel), the third model was obtained by using the QEEG (DTABR), and the final model included the combination of GCS, TCD, and QEEG. The AUROC for the model that included GCS, TCD, and QEEG was 0.905; the sensitivity of this combination for predicting mortality within 90 days was 77.27% and specificity was 89.19%. Comparison of the ROC curves showed that the efficacy of the combination of GCS, TCD, and QEEG for predicting mortality in patients with LHI was better than that of the GCS score (AUROC 0.714; p = 0.0003), TCD (AUROC 0.693; p = 0.0009), or QEEG (AUROC 0.733; p = 0.0040). Therefore, the contribution of the final model was significant (Figure 5).
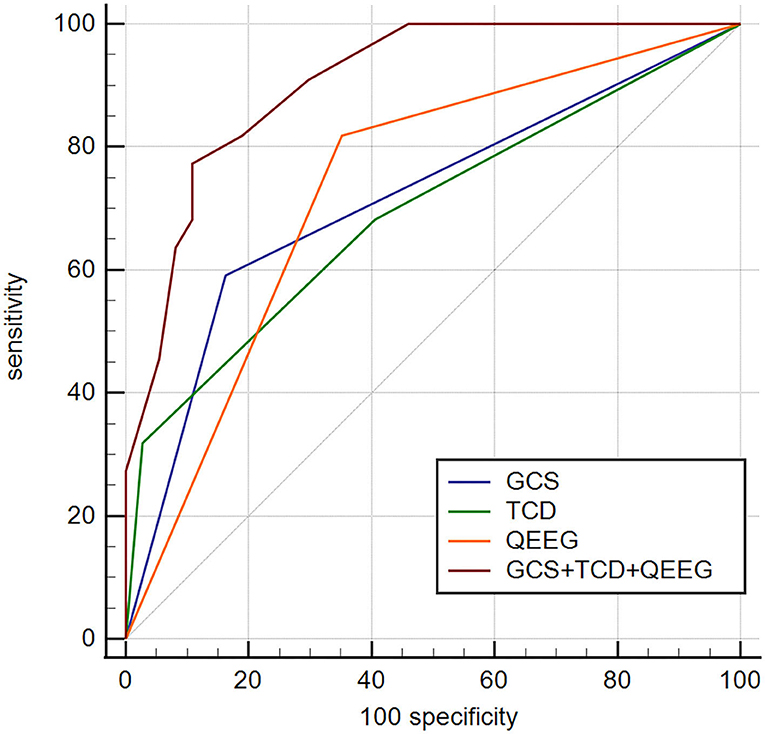
Figure 5. Comparison of ROC curves to predict outcome in this cohort between four models. GCS, AUROC 0.715 (0.582–0.824); TCD—offending vessel, AUROC 0.693 (0.560–0.807); QEEG—DTABR, AUROC 0.733 (0.602–0.840); GCS + TCD + QEEG, AUROC 0.905 (0.801–0.966). p < 0.05 for GCS + TCD (offending vessel) + QEEG (DTABR) comparison with GCS, offending vessel (independent predictor of TCD), and DTABR (independent predictor of QEEG). ROC, receiver operating characteristic; GCS, Glasgow Coma Scale; AUROC, area under the ROC curve; TCD, transcranial Doppler, QEEG, quantitative electroencephalography; DTABR, (delta + theta)/(alpha + beta) ratio; VD, diastolic flow velocity.
Discussion
This study used a combination of TCD and QEEG to assess the prognosis of patients with LHI by evaluating changes in brain function; such an approach has not been used previously. We used TCD-QEEG as a new bedside monitoring approach and confirmed that the GCS score, TCD, and QEEG were independent predictors of 90-day mortality. Moreover, we showed that the combination of the GCS score, DTABR from the QEEG, and the offending vessel from TCD together showed a marked potential for predicting the prognosis in patients with LHI. Our findings emphasize the importance of monitoring the damage of CBF and neuronal activity when evaluating the prognosis of patients with LHI.
The GCS score is the most widely used indicator of the severity of consciousness disturbance (40). Many studies have shown that the GCS score was associated with a poor prognosis (41, 42). Patients with LHI usually demonstrate a severe disturbance of consciousness (43) and for patients with LHI, the baseline GCS score at admission has been significantly associated with death (44). We found that the GCS score of ≤ 8 significantly affected the duration of survival in patients with LHI. However, the subjectivity of the surgeons and dysphasia, mechanical ventilation, or sedation of patients may bias the results (45–47). Therefore, for a more accurate and comprehensive evaluation of patients, we suggest that the GCS score should be combined with the other variables included in our multivariate model to predict prognosis, as other studies have suggested previously (48, 49).
Quantitative electroencephalography, as a non-invasive and economical bedside monitoring method, can reflect the decrease of CBF and the change of neuron damage (50). The significant QEEG characteristics of patients with LHI are increased delta activity, decreased beta and alpha activity, and a decrease in the overall background activity (51). Additionally, Sheorajpanday et al. found that BSI is an independent predictor of acute anterior circulation syndrome (28). In this study, we found that the RDP, RAP, RBP, DAR, DTABR, and BSI of patients with LHI are significantly different from those of healthy controls. When comparing EEG findings with clinical outcomes, depression of alpha frequency together with continuous delta activity was a predictor of poor outcome (52). DAR and DTABR have important predictive value in the evaluation of patients with LHI (26, 27, 53). Compared with DAR and DTABR as a marker of global brain function, it can also be used to evaluate theta and beta activities. It can independently predict mortality in patients with LHI (28, 29). The data in this study support previous findings that RAP, RDP, DAR, and DTABR are all related to the mortality of patients with LHI within 90 days. In order to promote clinical translation and utility of the study findings, it is necessary to formulate standard ranges or standard values of variables. Studies have shown that the DAR threshold of 3.7 can distinguish ischemic stroke from healthy brains (53). In a study of 24 patients with lacunar cerebral infarction, DTABR < 1 had 100% specificity for the absence, while DTABR > 3.5 had 100% sensitivity for the presence of such lesions (54). This study found that DTABR > 3 was an independent predictor of survival duration in patients with LHI and could reliably distinguish patients with a poor prognosis. The application of QEEG is, thus, an indispensable part of multimodal monitoring in the clinical management of patients with severe LHI.
Patients with LHI primarily have three offending vessels: the proximal ICA, TICA, and MCA. In this study, TCD detected that severe stenosis or occlusion of the TICA was an independent predictor of the duration of survival in patients with LHI. The mortality rate in patients with TICA as the offending vessel was about 3.8 times that of patients with the proximal ICA as the offending vessel. Other researchers have also found that patients with ≥ 50% stenosis or occlusion, as a result of TICA atherosclerosis, had a higher risk of stroke and death and their long-term prognosis was poor compared to that of patients with proximal ICA involvement (55, 56). Moreover, patients with a hyperdense TICA sign caused by a thrombus also had a more severe initial neurological deficit and a worse prognosis (57). We reasoned that differences in prognosis between patients with TICA and proximal ICA might be related to compensation by the OA. A previous study has demonstrated that when the ICA is occluded, cerebral perfusion strongly depends on the contribution of the ipsilateral external carotid artery (58). This indicates that, in future clinical work, clinicians should pay more attention to the occurrence of severe TICA stenosis or occlusion, since TICA lesions are associated with a poor prognosis.
Pathophysiological changes in patients with LHI remain problematic. BSI was found to be an indicator of bilateral hemispheric damage symmetry (38, 59). In this study, BSI was only statistically significantly different between patients with LHI and healthy controls, but there was no difference between survivors and non-survivors. In the study of Bentes et al. (29), DTABR and RAP, rather than BSI, were the best QEEG indicators of prognosis in patients with LHI. Therefore, the predictive effect of BSI requires further studies in patients with LHI. Monitoring of dynamic changes in variables may provide more insight. In addition, TCD monitoring in the first 6 or 12 h after admission has shown that lack of blood flow or asymmetric flow velocity in the MCA is an independent predictor of poor prognosis (60, 61). This study found no predictive significance, perhaps due to later monitoring. It is possible that cerebrovascular recanalization or early reocclusion had occurred; therefore, in this study, the offending vessels, rather than flow velocity, were the ultimate predictor.
The results of this study should be interpreted with caution, given its limitations. First, this study had a small sample size. Second, although participants with LHI were included, specific differences in the volume of the cerebral infarction may also have influenced the outcome. Third, this study found only a predictive effect of TCD-QEEG on mortality of the patient; the predictive effect of TCD-QEEG on the quality of life of the patient warrants further investigation. Finally, the brain function of patients should be dynamically monitored at an earlier point in future studies to determine if changes therein are associated with outcomes.
Our results show that the GCS score, QEEG, and TCD can independently predict poor prognosis in patients with LHI, which has not been reported previously. Combining the clinical assessment scale and multimodal monitoring methods yielded the best prognostic prediction model that could provide more accurate prognostic information on mortality risk. Based on the advantages of non-invasive and bedside monitoring, this is expected to become a routine method to evaluate the prognosis of patients with LHI.
Data Availability Statement
The raw data supporting the conclusions of this article will be made available by the authors, without undue reservation.
Ethics Statement
The studies involving human participants were reviewed and approved by the Ethics Committee of the First Hospital of Jilin University, China (NO. 2018-406). The patients/participants provided their written informed consent to participate in this study.
Author Contributions
YQ and LW contributed to the conceptualization, methodology, formal analysis and investigation, and writing–original draft preparation. YX contributed to the conceptualization, methodology, formal analysis and investigation, writing–review and editing, funding acquisition, and resources. JZ, YCa, and LL contributed to the methodology, formal analysis, and investigation. YCh contributed to the conceptualization, writing–review and editing, funding acquisition, resources, and supervision. All the authors read and approved the final manuscript.
Funding
This study was supported by the National Natural Science Foundation of China (Grant No. 82102066 and No. 81971620) and the Natural Science Foundation of Jilin Science and Technology Department (Grant No. 20210101254JC and 20200201522JC).
Conflict of Interest
The authors declare that the research was conducted in the absence of any commercial or financial relationships that could be construed as a potential conflict of interest.
Publisher's Note
All claims expressed in this article are solely those of the authors and do not necessarily represent those of their affiliated organizations, or those of the publisher, the editors and the reviewers. Any product that may be evaluated in this article, or claim that may be made by its manufacturer, is not guaranteed or endorsed by the publisher.
Acknowledgments
We thank all the participants involved in this study and the patients and their families for their participation and cooperation.
References
1. Hacke W, Schwab S, Horn M, Spranger M, De Georgia M, von Kummer R. ‘Malignant' middle cerebral artery territory infarction: clinical course and prognostic signs. Arch Neurol. (1996) 53:309–15. doi: 10.1001/archneur.1996.00550040037012
2. Heiss W-D. Malignant MCA infarction: pathophysiology and imaging for early diagnosis and management decisions. Cerebrovasc Dis. (2016) 41:1–7. doi: 10.1159/000441627
3. Li J, Zhang P, Wu S, Yi X, Wang C, Liu M. Factors associated with favourable outcome in large hemispheric infarctions. BMC Neurol. (2018) 18:152. doi: 10.1186/s12883-018-1148-7
4. Zha AM, Sari M, Torbey MT. Recommendations for management of large hemispheric infarction. Curr Opin Crit Care. (2015) 21:91–8. doi: 10.1097/MCC.0000000000000184
5. Minnerup J, Wersching H, Ringelstein EB, Heindel W, Niederstadt T, Schilling M, et al. Prediction of malignant middle cerebral artery infarction using computed tomography-based intracranial volume reserve measurements. Stroke. (2011) 42:3403–9. doi: 10.1161/STROKEAHA.111.619734
6. Wijdicks EFM, Sheth KN, Carter BS, Greer DM, Kasner SE, Kimberly WT, et al. Recommendations for the management of cerebral and cerebellar infarction with swelling: a statement for healthcare professionals from the American heart association/American stroke association. Stroke. (2014) 45:1222–38. doi: 10.1161/01.str.0000441965.15164.d6
7. Iadecola C, Anrather J. The immunology of stroke: from mechanisms to translation. Nat Med. (2011) 17:796–808. doi: 10.1038/nm.2399
8. Moskowitz MA, Lo EH, Iadecola C. The science of stroke: mechanisms in search of treatments. Neuron. (2010) 67:181–98. doi: 10.1016/j.neuron.2010.07.002
9. Alexandrov AV, Demchuk AM, Wein TH, Grotta JC. Yield of transcranial doppler in acute cerebral ischemia. Stroke. (1999) 30:1604–9. doi: 10.1161/01.STR.30.8.1604
10. Egido JA, Sánchez C. Neurosonology in cerebral ischemia: future application of transcranial doppler in acute stroke. Cerebrovasc Dis. (2001) 11:15–9. doi: 10.1159/000049121
11. Wong KS Li H, Chan YL, Ahuja A, Lam WW, Wong A, et al. Use of transcranial doppler ultrasound to predict outcome in patients with intracranial large-artery occlusive disease. Stroke. (2000) 31:2641–7. doi: 10.1161/01.STR.31.11.2641
12. Bouzat P, Almeras L, Manhes P, Sanders L, Levrat A, David JS, et al. Transcranial doppler to predict neurologic outcome after mild to moderate traumatic brain injury. Anesthesiology. (2016) 125:346–54. doi: 10.1097/ALN.0000000000001165
13. Camerlingo M, Casto L, Censori B, Ferraro B, Gazzaniga GC, Mamoli A. Transcranial doppler in acute ischemic stroke of the middle cerebral artery territories. Acta Neurol Scand. (1993) 88:108–11. doi: 10.1111/j.1600-0404.1993.tb04200.x
14. Guan J, Zhang S, Zhou Q, Li C, Lu Z. Usefulness of transcranial doppler ultrasound in evaluating cervical–cranial collateral circulations. Interv Neurol. (2013) 2:8–18. doi: 10.1159/000354732
15. Liu LP, Xu AD, Wong LKS, Wang DZ, Wang YJ. Expert consensus group of the evaluation & intervention of collateral circulation for ischemic stroke. Chinese consensus statement on the evaluation and intervention of collateral circulation for ischemic stroke CNS. Neurosci Ther. (2014) 20:202–8. doi: 10.1111/cns.12226
16. Dohmen C, Bosche B, Graf R, Staub F, Kracht L, Sobesky J, et al. Prediction of malignant course in MCA infarction by PET and microdialysis. Stroke. (2003) 34:2152–8. doi: 10.1161/01.STR.0000083624.74929.32
17. Nagata K, Tagawa K, Hiroi S, Shishido F, Uemura K. Electroencephalographic correlates of blood flow and oxygen metabolism provided by positron emission tomography in patients with cerebral infarction. Electroencephalogr Clin Neurophysiol. (1989) 72:16–30. doi: 10.1016/0013-4694(89)90027-8
18. Sharbrough FW, Messick JM, Sundt TM. Correlation of Continuous Electroencephalograms with Cerebral Blood Flow Measurements during Carotid Endarterectomy. Stroke. (1973) 4:674–83. doi: 10.1161/01.STR.4.4.674
19. Hossmann KA. Viability thresholds and the penumbra of focal ischemia. Ann Neurol. (1994) 36:557–65. doi: 10.1002/ana.410360404
20. Foreman B, Claassen J. Quantitative EEG for the detection of rain ischemia. Crit Care. (2012) 16:216. doi: 10.1186/cc11230
21. Sainio K, Stenberg D, Keskimäki I, Muuronen A, Kaste M. Visual and spectral EEG analysis in the evaluation of the outcome in patients with ischemic brain infarction. Electroencephalogr Clin Neurophysiol. (1983) 56:117–24. doi: 10.1016/0013-4694(83)90066-4
22. Jiang M, Su Y, Liu G, Chen W, Gao D. Predicting the non-survival outcome of large hemispheric infarction patients via quantitative electroencephalography: superiority to visual electroencephalography and the glasgow coma scale. Neurosci Lett. (2019) 706:88–92. doi: 10.1016/j.neulet.2019.05.007
23. Finnigan SP, Rose SE, Walsh M, Griffin M, Janke AL, McMahon KL, et al. Correlation of quantitative EEG in acute ischemic stroke with 30-day NIHSS score: comparison with diffusion and perfusion MRI. Stroke. (2004) 35:899–903. doi: 10.1161/01.STR.0000122622.73916.d2
24. Cuspineda E, Machado C, Galán L, Aubert E, Alvarez MA, Llopis F, et al. QEEG prognostic value in acute stroke. Clin EEG Neurosci. (2007) 38:155–60. doi: 10.1177/155005940703800312
25. Burghaus L, Hilker R, Dohmen C, Bosche B, Winhuisen L, Galldiks N, et al. Early electroencephalography in acute ischemic stroke: prediction of a malignant course? Clin Neurol Neurosurg. (2007) 109:45–9. doi: 10.1016/j.clineuro.2006.06.003
26. Finnigan SP, Walsh M, Rose SE, Chalk JB. Quantitative EEG indices of sub-acute ischaemic stroke correlate with clinical outcomes. Clin Neurophysiol. (2007) 118:2525–32. doi: 10.1016/j.clinph.2007.07.021
27. Sheorajpanday RV, Nagels G, Weeren AJ, van Putten MJ, De Deyn PP. Quantitative EEG in ischemic stroke: correlation with functional status after 6 months. Clin Neurophysiol. (2011) 122:874–83. doi: 10.1016/j.clinph.2010.07.028
28. Sheorajpanday RV, Nagels G, Weeren AJ, De Surgeloose D, De Deyn PP. Additional value of quantitative EEG in acute anterior circulation syndrome of presumed ischemic origin. Clin Neurophysiol. (2010) 121:1719–25. doi: 10.1016/j.clinph.2009.10.037
29. Bentes C, Peralta AR, Viana P, Martins H, Morgado C, Casimiro C, et al. Quantitative EEG and functional outcome following acute ischemic stroke. Clin Neurophysiol. (2018) 129:1680–7. doi: 10.1016/j.clinph.2018.05.021
30. Finnigan S, van Putten MJAM. EEG in Ischaemic stroke: quantitative EEG can uniquely inform (sub-)acute prognoses and clinical management. Clin Neurophysiol. (2013) 124:10–9. doi: 10.1016/j.clinph.2012.07.003
31. Doerrfuss JI, Kilic T, Ahmadi M, Holtkamp M, Weber JE. Quantitative and qualitative EEG as a prediction tool for outcome and complications in acute stroke patients. Clin EEG Neurosci. (2020) 51:121–9. doi: 10.1177/1550059419875916
32. Jüttler E, Schwab S, Schmiedek P, Unterberg A, Hennerici M, Woitzik J, et al. Decompressive surgery for the treatment of malignant infarction of the middle cerebral artery (DESTINY): a randomized controlled trial. Stroke. (2007) 38:2518–25. doi: 10.1161/STROKEAHA.107.485649
33. Powers WJ, Rabinstein AA, Ackerson T, Adeoye OM, Bambakidis NC, Becker K, et al. Guidelines for the early management of patients with acute ischemic stroke: 2019 update to the 2018 guidelines for the early management of acute ischemic stroke: a guideline for healthcare professionals from the American Heart Association/American Stroke Association. Stroke. (2019) 50:e344–418. doi: 10.1161/STR.0000000000000211
34. Csiba L, Baracchini C. Manual of Neurosonology. Cambridge University Press. (2016). doi: 10.1017/CBO9781107447905
35. Chernyshev OY, Garami Z, Calleja S, Song J, Campbell MS, Noser EA, et al. Yield and accuracy of urgent combined carotid/transcranial ultrasound testing in acute cerebral ischemia. Stroke. (2005) 36:32–7. doi: 10.1161/01.STR.0000150496.27584.e3
36. Chen Y, Wang L, Zhang J, Wang S, Qi Y, Cao J, et al. Monitoring of patients with brainstem hemorrhage: a simultaneous study of quantitative electroencephalography and transcranial doppler. Clin Neurophysiol. (2021) 132:946–52. doi: 10.1016/j.clinph.2020.12.026
37. Demchuk AM, Burgin WS, Christou I, Felberg RA, Barber PA, Hill MD, et al. Thrombolysis in brain ischemia (TIBI) transcranial doppler flow grades predict clinical severity, early recovery, and mortality in patients treated with intravenous tissue plasminogen activator. Stroke. (2001) 32:89–93. doi: 10.1161/01.STR.32.1.89
38. Sheorajpanday RV, Nagels G, Weeren AJ, van Putten MJ, De Deyn PP. Reproducibility and clinical relevance of quantitative EEG parameters in cerebral ischemia: a basic approach. Clin Neurophysiol. (2009) 120:845–55. doi: 10.1016/j.clinph.2009.02.171
39. Chen Y, Xu W, Wang L, Yin X, Cao J, Deng F, et al. Transcranial doppler combined with quantitative EEG brain function monitoring and outcome prediction in patients with severe acute intracerebral hemorrhage. Crit Care. (2018) 22:36. doi: 10.1186/s13054-018-1951-y
40. Teasdale G, Jennett B. Assessment of coma and impaired consciousness. A practical scale. Lancet. (1974) 2:81–4. doi: 10.1016/S0140-6736(74)91639-0
41. Miah MT, Hoque AA, Khan RR, Nur Z, Mahbub MS, Rony RI, et al. The glasgow coma scale following acute stroke and in-hospital outcome: an observational study. J Med. (2009) 10:11–4. doi: 10.3329/jom.v10i3.2009
42. Mansour OY, Megahed MM, Elghany EHSA. Acute ischemic stroke prognostication, comparison between glasgow coma score, nihs scale and full outline of unresponsiveness score in intensive care unit. Alexandria Med J. (2015) 51:247–53. doi: 10.1016/j.ajme.2014.10.002
43. Li J, Zhang P, Wu S, Liu J, Tao W, Wang D, et al. Impaired consciousness at stroke onset in large hemisphere infarction: incidence, risk factors and outcome. Sci Rep. (2020) 10:1–9. doi: 10.1038/s41598-020-70172-1
44. Tsai C-L, Chu H, Peng G-S, Ma HI, Cheng CA, Hueng DY. Preoperative APACHE II and GCS scores as predictors of outcomes in patients with malignant MCA infarction after decompressive hemicraniectomy. Neurol India. (2012) 60:608–12. doi: 10.4103/0028-3886.105195
45. Rowley G, Fielding K. Reliability and accuracy of the glasgow coma scale with experienced and inexperienced users. Lancet. (1991) 337:535–8. doi: 10.1016/0140-6736(91)91309-I
46. Weir CJ, Bradford APJ, Lees KR. The prognostic value of the components of the glasgow coma scale following acute stroke. QJM. (2003) 96:67–74. doi: 10.1093/qjmed/hcg008
47. Reith FCM, Van den Brande R, Synnot A, Gruen R, Maas AI. The reliability of the glasgow coma scale: a systematic review. Intensive Care Med. (2016) 42:3–15. doi: 10.1007/s00134-015-4124-3
48. Teasdale G, Maas A, Lecky F, Manley G, Stocchetti N, Murray G. The glasgow coma scale at 40 years: standing the test of time. Lancet Neurol. (2014) 13:844–54. doi: 10.1016/S1474-4422(14)70120-6
49. Rivera Lara L, Püttgen HA. Multimodality monitoring in the neurocritical care unit. Continuum. (2018) 24:1776–88. doi: 10.1212/CON.0000000000000671
50. Faught E. Current role of electroencephalography in cerebral ischemia. Stroke. (1993) 24:609–13. doi: 10.1161/01.STR.24.4.609
51. Cuspineda E, Machado C, Aubert E, Galán L, Llopis F, Avila Y. Predicting outcome in acute stroke: a comparison between QEEG and the canadian neurological scale. Clin Electroencephalogr. (2003) 34:1–4. doi: 10.1177/155005940303400104
52. Cillessen JP, van Huffelen AC, Kappelle LJ, Algra A, van Gijn J. Electroencephalography improves the prediction of functional outcome in the acute stage of cerebral ischemia. Stroke. (1994) 25:1968–72. doi: 10.1161/01.STR.25.10.1968
53. Finnigan S, Wong A, Read S. Defining abnormal slow EEG Activity in acute ischaemic stroke: delta/alpha ratio as an optimal QEEG index. Clin Neurophysiol. (2016) 127:1452–9. doi: 10.1016/j.clinph.2015.07.014
54. Sheorajpanday RVA, Nagels G, Weeren AJTM, De Deyn PP. Quantitative EEG in ischemic stroke: correlation with infarct volume and functional status in posterior circulation and lacunar syndromes. Clin Neurophysiol. (2011) 122:884–90. doi: 10.1016/j.clinph.2010.08.020
55. Marzewski DJ, Furlan AJ, St Louis P, Little JR, Modic MT, Williams G. Intracranial internal carotid artery stenosis: longterm prognosis. Stroke. (1982) 13:821–4. doi: 10.1161/01.STR.13.6.821
56. Chen H, Hong H, Xing S, Liu G, Zhang A, Tan S, et al. Intracranial versus extracranial symptomatic carotid atherosclerosis in Chinese patients: risk factors, stroke mechanisms, and long-term prognosis. J Stroke Cerebrovasc Dis. (2015) 24:2632–9. doi: 10.1016/j.jstrokecerebrovasdis.2015.07.019
57. Ozdemir O, Leung A, Bussiére M, Hachinski V, Pelz D. Hyperdense internal carotid artery sign: a CT sign of acute ischemia. Stroke. (2008) 39:201–6. doi: 10.1161/STROKEAHA.107.505230
58. van Laar PJ, van der Grond J, Bremmer JP, Klijn CJ, Hendrikse J. Assessment of the contribution of the external carotid artery to brain perfusion in patients with internal carotid artery occlusion. Stroke. (2008) 39:3003–8. doi: 10.1161/STROKEAHA.108.514265
59. van Putten MJ, Peters JM, Mulder SM, de Haas JA, Bruijninckx CM, Tavy DL, et al. Brain Symmetry Index (BSI) for online EEG monitoring in carotid endarterectomy. Clin Neurophysiol. (2004) 115:1189–94. doi: 10.1016/j.clinph.2003.12.002
60. Toni D, Fiorelli M, Zanette EM, Sacchetti ML, Salerno A, Argentino C, et al. Early spontaneous improvement and deterioration of ischemic stroke patients. A serial study with transcranial doppler ultrasonography. Stroke. (1998) 29:1144–8. doi: 10.1161/01.STR.29.6.1144
Keywords: glasgow coma scale, large hemispheric infarction, multimodal monitoring, prognosis, quantitative electroencephalography, transcranial doppler
Citation: Qi Y, Xing Y, Wang L, Zhang J, Cao Y, Liu L and Chen Y (2021) Multimodal Monitoring in Large Hemispheric Infarction: Quantitative Electroencephalography Combined With Transcranial Doppler for Prognosis Prediction. Front. Neurol. 12:724571. doi: 10.3389/fneur.2021.724571
Received: 13 June 2021; Accepted: 08 November 2021;
Published: 08 December 2021.
Edited by:
Laszlo Csiba, University of Debrecen, HungaryReviewed by:
Branko Malojcic, University Hospital Centre Zagreb, CroatiaNagy Zoltán, Semmelweis University, Hungary
Copyright © 2021 Qi, Xing, Wang, Zhang, Cao, Liu and Chen. This is an open-access article distributed under the terms of the Creative Commons Attribution License (CC BY). The use, distribution or reproduction in other forums is permitted, provided the original author(s) and the copyright owner(s) are credited and that the original publication in this journal is cited, in accordance with accepted academic practice. No use, distribution or reproduction is permitted which does not comply with these terms.
*Correspondence: Ying Chen, cheny187080119@jlu.edu.cn
†These authors have contributed equally to this work