- 1Centre for Systems Modelling and Quantitative Biomedicine (SMQB), University of Birmingham, Birmingham, United Kingdom
- 2Institute of Metabolism and Systems Research (IMSR), University of Birmingham, Birmingham, United Kingdom
- 3Centre of Membrane Proteins and Receptors (COMPARE), University of Birmingham, Birmingham, United Kingdom
- 4Oxford Centre for Diabetes, Endocrinology, and Metabolism (OCDEM), Radcliffe Department of Medicine, Churchill Hospital, University of Oxford, Oxford, United Kingdom
- 5NIHR Oxford Biomedical Research Centre, Radcliffe Department of Medicine, Churchill Hospital, University of Oxford, Oxford, United Kingdom
- 6Living Systems Institute, University of Exeter, Exeter, United Kingdom
- 7EPSRC Hub for Quantitative Modelling in Healthcare, University of Exeter, Exeter, United Kingdom
- 8College of Engineering, Mathematics and Physical Sciences, University of Exeter, Exeter, United Kingdom
We study the impact of spatial distribution of heterogeneity on collective dynamics in gap-junction coupled beta-cell networks comprised on cells from two populations that differ in their intrinsic excitability. Initially, these populations are uniformly and randomly distributed throughout the networks. We develop and apply an iterative algorithm for perturbing the arrangement of the network such that cells from the same population are increasingly likely to be adjacent to one another. We find that the global input strength, or network drive, necessary to transition the network from a state of quiescence to a state of synchronised and oscillatory activity decreases as network sortedness increases. Moreover, for weak coupling, we find that regimes of partial synchronisation and wave propagation arise, which depend both on network drive and network sortedness. We then demonstrate the utility of this algorithm for studying the distribution of heterogeneity in general networks, for which we use Watts–Strogatz networks as a case study. This work highlights the importance of heterogeneity in node dynamics in establishing collective rhythms in complex, excitable networks and has implications for a wide range of real-world systems that exhibit such heterogeneity.
1 Introduction
Many non-linear systems exhibit excitable behaviour, whereby they exhibit large-amplitude oscillations in response to small-amplitude, transient perturbations. One prominent example in biology is electrical excitability in cells, which underlies the function of neurons (Izhikevich, 2000; De Maesschalck and Wechselberger, 2015; Wedgwood et al., 2021), cardiac cells (Majumder et al., 2018; Barrio et al., 2020), pituitary cells (Sanchez-Cardenas et al., 2010; Hodson et al., 2012) and pancreatic beta cells (Bertram et al., 2007; McKenna et al., 2016). Moreover, the study of these systems is broadly applicable, and such excitable dynamics are also observed in semiconductor lasers (Terrien et al., 2020; Terrien et al., 2021), social media networks (Mathiesen et al., 2013), epidemiology (Vannucchi and Boccaletti, 2004), and wildfires (Punckt et al., 2015). When excitable units are combined into networks, they can generate complex rhythms (Bittihn et al., 2017; Fretter et al., 2017; Hörning et al., 2017). Interestingly, such networks may also generate dynamics that occur over low-dimensional manifolds of the full system (Ashwin and Swift, 1992; Watanabe and Strogatz, 1993; Ott and Antonsen, 2009; Bick et al., 2020). For example, neurons in the pre-Bötzinger complex fire synchronously to induce the inspiratory and expiratory phases during breathing (Wittmeier et al., 2008; Gaiteri and Rubin, 2011).
Heterogeneity is ubiquitous in natural systems. Whilst often portrayed as a undesirable attribute, it can play an important role in governing network dynamics (Manchanda et al., 2017; Delgado et al., 2018; Lambert and Vanni, 2018). For example, neurons may coarsely be stratified into excitatory and inhibitory groups, with the former promoting firing behaviour in other neurons and the latter suppressing it. When coupled, these neuronal subtypes give rise to a variety of behaviours, including synchronisation, and enable the network to respond differentially to incoming inputs (Börgers and Kopell, 2003; Börgers et al., 2005; Kopell et al., 2010). The classification of neuronal subtypes is becoming ever finer (Gouwens et al., 2019; Lipovsek et al., 2021) and it remains an open question as to how this heterogeneity governs overall brain dynamics. Even when networks comprise only a single unit type, heterogeneity may still impact the global dynamics. For example, if the natural frequencies of nodes in a coupled oscillator network are too far apart, the network will be unable to synchronise and will instead display more complex rhythms (Ottino-Löffler and Strogatz, 2016). In pancreatic islets, heterogeneity has increasingly been acknowledged as crucial for healthy glucose metabolism (Nasteska et al., 2021), and classification of beta-cell populations in particular is an area of active investigation. Heterogeneity in cell-intrinsic properties (i.e., excitability, metabolic activity, and genetic profiles), network properties, and functional properties have all been demonstrated (Johnston et al., 2016; Westacott et al., 2017; Nasteska et al., 2021; Kravets et al., 2022; Šterk et al., 2023).
Here, we explore transitions between quiescent states and collective oscillations in coupled networks of heterogeneous, excitable nodes. For the node dynamics, we treat two types of system, the first being the FitzHugh–Nagumo (FHN) model, which is a prototypical model of electrical excitability in biological cells (Fitzhugh, 1961; Nagumo, 1962), is often used to investigate collective network dynamics, and has been used as a model for beta cells (Scialla et al., 2021; Pedersen et al., 2022). For the second, we consider a conductance-based model of pancreatic beta cells designed to more closely reproduce the signalling dynamics in these cells (Sherman et al., 1988). These cells remain at rest until they receive a significantly large electrical impulse or, in the case of beta cells, the extracellular concentration of glucose surpasses a threshold value (Ashcroft Frances M and Rorsman, 1989; Braun et al., 2008).
For the past 40 years, based on empirical evidence from rodent islets, it has generally been assumed that beta cells form a syncticium, in which activity of an entire islet of Langerhans can be described by considering the dynamics of a single cell (Dolenšek et al., 2013; Podobnik et al., 2020; Satin et al., 2020). A number of studies have challenged this perspective in recent years, in particular highlighting that some ‘leader cells’ disproportionately influence the activity of the entire network which is made up primarily of ‘follower cells’ (Johnston et al., 2016; Westacott et al., 2017; Salem et al., 2019; Benninger and Kravets, 2021). In particular, one hypothesis suggests that beta cells can be grouped into two overarching classes based on their degree of excitability. This then suggests that islets are composed of a small number (∼10%) of highly excitable cells, with the remainder being less excitable (Benninger and Hodson, 2018).
To this end, we diffusively couple cells having two different degrees of excitability according to two different network architecture classes. The first of these comes from the Watts–Strogatz model, which allows for the generation of connected graphs, with consistent mean degree, and a parameter for adjusting the balance between local and long-range connections. This form of coupling allows one to explore architectures ranging from regular to small world graphs and, as such, is well-suited to investigations of the general types of graphs that occur in the natural world (Watts and Strogatz, 1998). While these graphs are not directly relevant to the electrical coupling architecture of pancreatic beta-cell networks, there is potential that the dynamics of beta-cell networks share common features with small-world networks. For example, small-worldness has be observed in the functional connectivity of pancreatic beta-cell Ca2+ signals (Stožer et al., 2013), and long-range connections have been proposed in mathematical modelling studies (Barua and Goel, 2016). The second model architecture was designed to mimic the spatial configuration of electrical coupling (i.e., connexin36 gap junctions) within beta-cell networks. Cells are locally coupled via gap junctions (Benninger et al., 2011; Rorsman and Braun, 2013) and spatially arranged in a three-dimensonal lattice embedded within a sphere.
With these network structures in mind, we explore how the spatial organisation of the two subpopulations affects the propensity of the whole network to oscillate in a synchronous fashion. The work of Westacott et al. (2017) recently demonstrated that β-cells with similar electrical excitability are spatially correlated within pancreatic islets. Motivated by this finding, we develop a metric for evaluating the degree of this spatial non-uniformity in β-cell networks and an algorithm for generating non-uniform networks with a specified value of this metric. We demonstrate that spatial correlation in heterogeneity, or network sortedness, plays a critical role in modulating collective dynamics in these networks. Moreover, we show that these metrics and algorithms can be applied to networks that do not have an explicit notion of space (e.g., Watts–Strogatz networks) and that network sortedness is similarly important in defining collective dynamics on these general network architectures. This work aims to demonstrate the importance of cellular organisation when studying heterogeneity in pancreatic β-cell networks (and in networks more generally) through a case study in heterogeneous excitability. Furthermore, it provides a set of tools for introducing correlated heterogeneities in arbitrary networks and studying their impact on dynamics. This spatial organisation is likely to be of particular relevance to the study of human islets, since these have been shown possess a highly non-trivial architecture, particularly when compared with rodent islets, on which most of our knowledge of beta cell function is based (Cabrera et al., 2006).
The remainder of the manuscript is arranged as follows: In Section 2, we describe the single cell dynamics and graph structures, introduce a metric that captures how sorted a network is with respect to its heterogeneity, and present an algorithm that can generate networks with arbitrary sortedness. In Section 3, we investigate how dynamic transitions to synchronous activity depend on the degree of sortedness in the network and end in Section 4 with concluding remarks.
2 Methods
2.1 Mathematical model
2.1.1 Fitzhugh-Nagumo model (FHN)
The Fitzhugh-Nagumo model is a minimal model of an excitable unit. It exhibits relaxation oscillations when the external stimulus parameter, I, exceeds a critical value, where a Hopf bifurcation occurs. Here, we consider a network of N diffusively-coupled excitable nodes, which may, for example, be cells, each of which is described by the following model.
Here, V models the fast upstroke characteristic of excitability whilst W models the slow recovery (i.e., negative feedback). We replace the regular stimulus current term, I, with GI, where I is now the maximum stimulus current to a node, and thus defines the excitability of the node, whereas G ∈ [0, 1] is the degree of drive to the network. This allows us to consider a heterogeneous network model based on the excitability of nodes, but whilst including a network-wide drive parameter. A list of parameters is included in Supplementary Table S1.
2.1.2 Sherman-Rinzel-Keizer model (SRK)
The electrical activity of pancreatic beta cells is proportional to the extracellular concentration of glucose. For sufficiently high extracellular glucose, the cells exhibit bursting dynamics, in which their voltage periodically switches between high frequency oscillations and quiescence. The high frequency oscillations in voltage are correlated with the secretion of insulin from these cells, so that these bursting dynamics are tightly coupled to the cells’ functional role. Here, we consider a network of N diffusively-coupled excitable cells, each of which is described by the three-variable model.
The variable V represents the membrane voltage of a β-cell, n is the activation variable for K+ ion channels, and c is the cytosolic Ca2+. This system was adapted from the Sherman–Rinzel–Keizer model, which describes the dynamics of electrical activity in pancreatic β-cells in the presence of glucose (Sherman et al., 1988). The intrinsic dynamics of the voltage, V given by Eq. 3 are driven by K+ (IK), Ca2+ (ICa), and Ca2+-activated K+ (IK−Ca) ionic currents, with a rate governed by the whole cell capacitance given by Cm. These currents are described via.
In Eqs 6–9,
The dynamics for n, m, and h follow exponential decay to their state values given by
at a rate given by the voltage-dependent time constant.
for n. In Eq. 10, Vx represents the activation (inactivation) thresholds for m and n (h) and Sx represents the sensitivity of the channels around this point. Finally, Eq. 5 describes the evolution of the concentration of cytosolic Ca2+, which decays and is pumped out of the cell following a combined linear process with rate kCa and enters the cell via the Ca2+ ion channel at a rate given by the scale factor α. The parameter f specifies the fraction of free to bound Ca2+ in the cell, where bound Ca2+ plays no role in the relevant dynamics in our model.
To expose the dependence of our system on glucose, we introduced a hyperpolarising leak current given by Eq. 9 that explicitly depends on the glucose concentration G. For an isolated cell (i.e., without coupling) with the parameters specified in Supplementary Table S2, the system describing each node exhibits steady state behaviour for low G and passes through a bifurcation as G ∈ [0, 1] is increased, as shown in Figure 1.
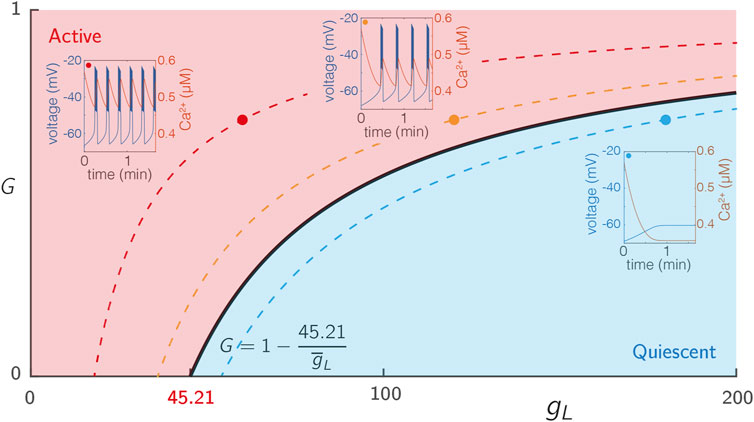
FIGURE 1. Excitability of single SRK cells. The voltage traces from 3 cells with varying levels of intrinsic excitability
The bursting dynamics in our model are of the fold-homoclinic type under the classification specified in (Izhikevich, 2000). This classification is based on separation of the full system into a fast subsystem (Eqs 3, 4) and a slow subsystem (Eq. 5), treating the slow subsystem variables (in this case, c) as parameters in the fast subsystem. During each bursting cycle, the slow evolution of c pushes the fast subsystem through bifurcations that initiate and terminate oscillatory behaviour. In particular, when c decreases to a small enough value, the fast subsystem passes through a fold bifurcation in which a stable steady state and a saddle steady state collide and annihilate one another. Following this, the system exhibits stable periodic activity, during which c increases according to Eq. 5. When c increases to a sufficiently large value, the fast subsystem passes through a homoclinic bifurcation that destroys the periodic orbit and the system returns to the original stable steady state. Following this, c decreases until it once again reaches the fold point and the cycle repeats.
2.2 Model simulations
Simulations were conducted using Matlab 2019B. The dynamical systems were solved using ode15s, the relative tolerance set to 10–5, and explicit Jacobians were provided. The code was run on the University of Birmingham BlueBEAR HPC running RedHat 8.3 (x86_64) (see http://www.birmingham.ac.uk/bear for more details). Each set of simulations ran over 16 cores using a maximum of 128 GB RAM (32 GB was sufficient in most cases). All code used in the project is freely available for download from: github.com/dgalvis/network_spatial.
2.2.1 Initial conditions
For the FHN model, the initial conditions
For the SRK model, the initial conditions
The term
2.2.2 Excitability and drive in the single-cell FHN model
The term GI is used to represent node excitability (I) and network drive (G). A Hopf bifurcation occurs at GI = 0.33. During the study, we partition the nodes into two sub-populations based on their excitability, with one population being highly excitable and the other being significantly less so.
2.2.3 Excitability and drive in the single-cell SRK model
The ionic current IL (Eq. 9) is a hyperpolarising current that can be used to adjust the excitability of each cell and to determine the activation level of the network model. In particular, the maximum conductance
2.2.4 Graph structure and coupling
In this work, we consider two types of graph structure. The first is the Watts-Strogatz model of graph generation to create small-world graphs (WS graphs). We use N = 1000 nodes, a mean degree of D = 12, and unless stated, we use a rewiring probability of β = 0.2. The second type is motivated by pancreatic β-cell networks, which are arranged into roughly spherical clusters called islets of Langerhans (which also encompass other cell types that are disregarded in our model), which each contain ∼ 1,000 β-cells. To capture this, we arrange N = 1, 018 nodes on a hexagonal close packed (hcp) lattice embedded within a sphere (βC graph). Each node is connected to all of its nearest-neighbours so that the number of connections of nodes away from the boundary of the sphere is equal to the coordination number 12 whilst nodes on the boundary have fewer connections. This type of connectivity scheme is commonly employed in β-cell network modelling studies (Benninger et al., 2014; Westacott et al., 2017; Dwulet et al., 2019, 2021), as it qualitatively captures the network architecture. In reality, pancreatic islet architecture is more complex in terms of islet shape, cell shape, and the layout of cells in the tissue (Stožer et al., 2013; Šterk et al., 2023); all of which could be considered as additional sources of heterogeneity. Moreover, there may be heterogeneity in the number of connections that β-cells form amongst each other (Cabrera et al., 2006). We choose to employ this homogeneous lattice structure so that we could consider heterogeneity in cell-intrinsic excitability alone without introducing additional sources of heterogeneity due to the graph structure.
The dominant form of coupling between beta cells in the islets is through gap junctions, which allow small molecules, including charged ions to pass directly from a cell to its adjacent neighbours. Mathematically, this is represented through the inclusion of the diffusive term Icoup,i in Eq. 1 or Eq. 3 (we use the same coupling for both FHN and SRK models) given by
where Ji is the set of all nodes to which node i is coupled.
Together, the two models for node dynamics (FHN and SRK models) and the two types of graph structure (WS and βC graphs) give rise to four combinations of network models, which we denote by WS-FHN, WS-SRK, βC-FHN, and βC-SRK. Note that throughout this work, we use the term graph to refer specifically to the set of links between nodes. We use the term network or network model to refer to the entirety of the model including choice of dynamics on the node (FHN or SRK), connectivity structure, and choice of parameters.
2.2.5 Heterogeneity
For the FHN model, we consider networks consisting of two sub-populations of nodes distinguished by their excitability (i.e., by their I values). Population 1 is highly excitable (I = 2) and population 2 is less excitable (I = 1). Similarly for the SRK model, we consider networks consisting of two sub-populations of nodes distinguished by their excitability (i.e., by their
2.3 Measuring sortedness
2.3.1 Definition
To track the degree of sortedness in the network, we define a node sortedness measure that, for a given node, measures the proportion of neighbours that are of the same population type. For a general network with nodes attributed to
where the population sets Pk contain the indices of the nodes within population k = 1, … , K and form a partition over the node indices {1, 2, … , N}, Ji is the set of indices of nodes that are coupled to node i,
Finally, the network sortedness is defined as
where
2.3.2 Modified network sortedness for beta cell networks
For a βC lattice network,
where J = 12 is the number of connections that interior lattice nodes possess. For nodes with |Ji| < J (i.e., nodes on the domain boundary) the additional term in Eq. 18 compared to Eq. 15 incorporates a further J − |Ji| connections to population 2 nodes for the purposes of calculating node sortedness values. This procedure is equivalent to assuming that the lattice defining our domain is embedded within a larger lattice of population 2 nodes.
2.4 Modifying network sortedness
2.4.1 The sorting algorithm
Here, we describe our approach for generating networks with different network sortedness. The algorithm works by exchanging the population type of nodes from different populations randomly to increase (or decrease)
On each iteration, a, of the algorithm, pairs of nodes (from different populations) are sampled without replacement from a joint probability density function (pdf) P(X = i, Y = j) = f (i, j), i ∈ P1, j ∈ P2, where X and Y are random integer variables indicating the node selected from population 1 and 2, respectively. The population types of these nodes are then exchanged, that is, if i ∈ P1 and j ∈ P2, then i is added to P2 and removed from P1 and vice versa for j. The network sortedness (Eq. 17) is then recomputed for the adjusted population sets. If the exchange leads to an increase (decrease) in
Figure 2 depicts a WS network (Figure 2A, top row) and a βC network (Figure 2B, top row) at five iterations of the sorting algorithm. The WS network can be described by a set of nodes equispaced along a ring, and when the rewiring probability is small, the majority of connections are between nodes that are close to one another on the ring. For our choice of D = 12 (and β = 0.2), the majority of connections will occur between an arbitrary node and the D/2 = 6 closest nodes in either direction. Figure 2A shows that as
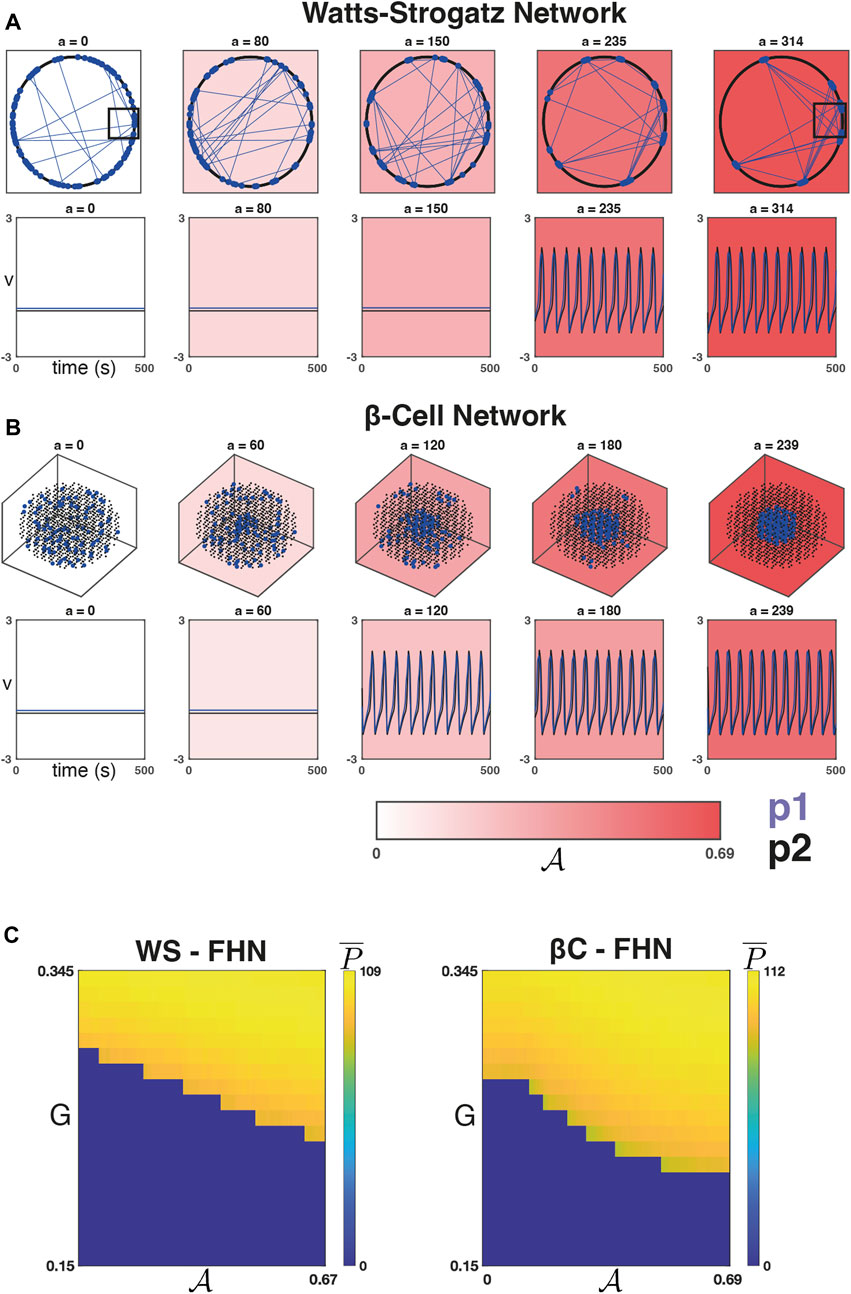
FIGURE 2. Dynamics on networks defined by the sorting algorithm. (A) Top Row: Five iterations of the sorting algorithm on a WS graph (with β = 0.2, D = 12). The majority of connections in this graph
2.4.2 Node selection probabilities
In this section, we formulate the node selection pdf used in the network sortedness adjustment algorithm. We assume that the selection of node from P1 is independent of the selection of node from P2:
One choice would set f1 and f2 to be uniform over P1 and P2, respectively. This is the choice we take for the WS networks.
Empirical observations of the algorithm applied to the βC networks, however, demonstrate that clusters of population 1 nodes tend to form at the edge of the domain. As discussed in Section 2.3.2, we wish to avoid this scenario. The tendency for clusters to form near the edge occurs because of the spherical nature of our lattice domain. In particular, a uniform choice for f1 and f2 means that nodes at the centre of the domain are less likely to be selected under a uniformly random sampling of indices than those at the edge because the number of nodes in the network increases superlinearly with respect to the domain radius. Therefore, we derive choices for
Denote the radial distance from the origin of node
where
Pseudocode for the βC network generation and network sortedness manipulation algorithm is provided in the Supplementary Section S2.
2.5 Evaluation of collective dynamics
To characterise the network dynamics, we consider two features based on the Ca2+ trajectories across all nodes, namely, the mean number of peaks
3 Results
3.1 For strong coupling, increasing sortedness decreases the necessary drive for a phase transition from quiescence into global and synchronised activity
We ran the sorting algorithm to convergence once on a typical WS network and again on the βC network to produce the sets
Figure 2C shows the average number of peaks
We next verified that this observed relationship is robust to changes in
We found that for each of the four combinations of network models, increasing
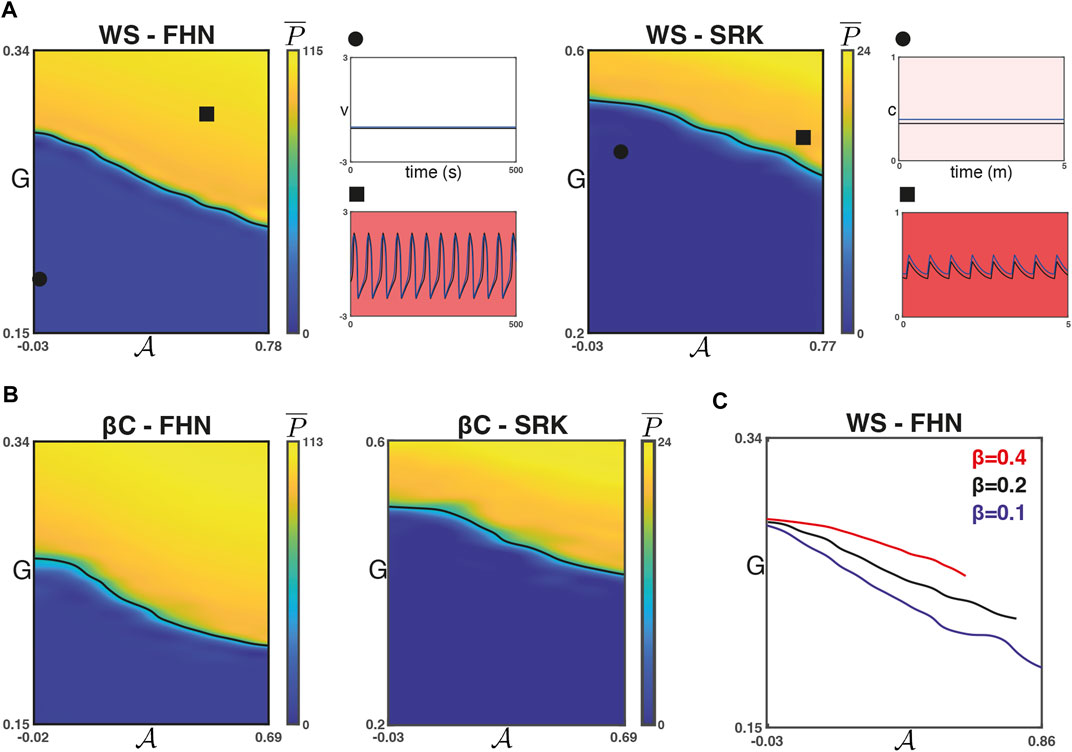
FIGURE 3. Phase transitions with respect to
For the WS networks, the rewiring parameter β is related to the small-worldness of the network and inversely related to the regularity of the graph. Moreover, the maximum possible value of
3.2 Weak coupling and high sortedness leads to wave propagation in the FHN networks
Next, we studied the influence of coupling strength on the relationship between
For the WS-FHN networks, we found that the intra-population isosurfaces (e.g.,
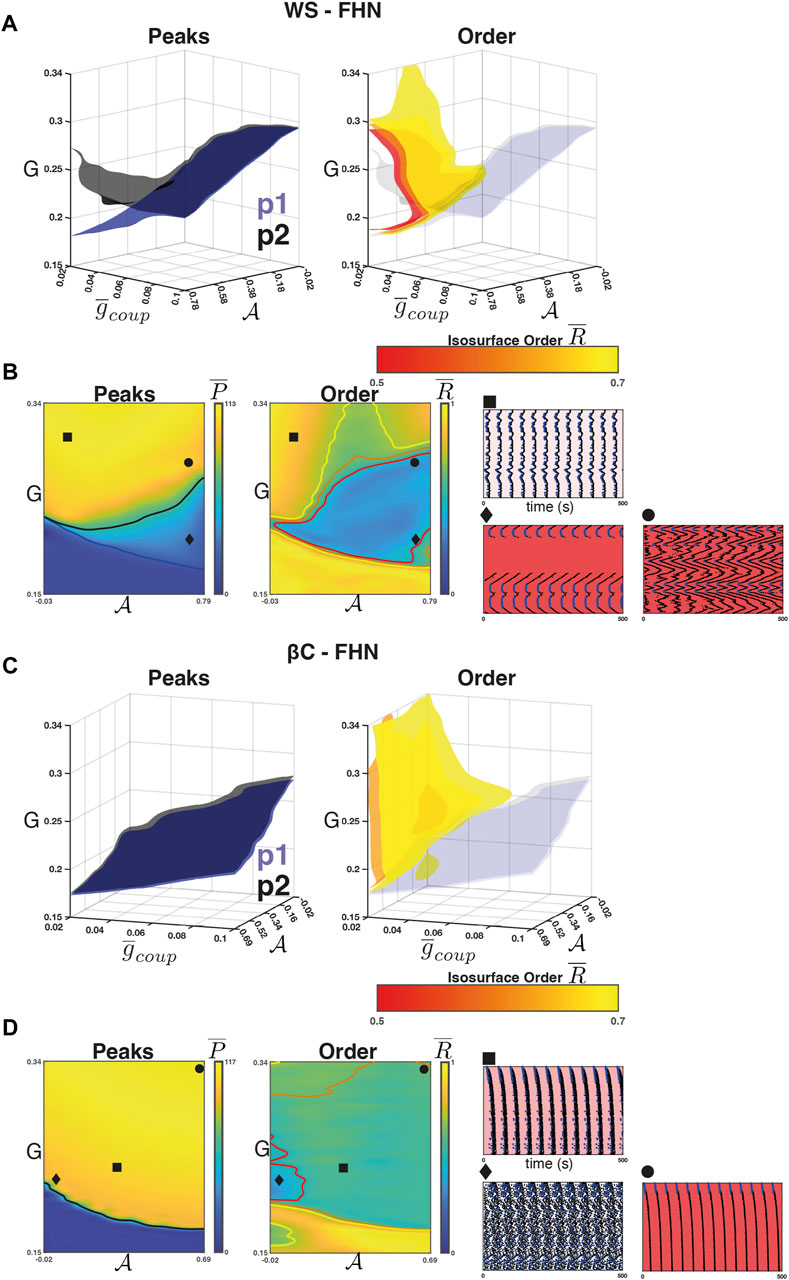
FIGURE 4. Weak coupling in the FHN networks. (A) Left panel: The isosurfaces for the
Figure 4A (right) shows the isosurfaces
For the βC-FHN networks, we repeated the experiment as described above. In this case, however, the raster plots are arranged by radial distance from the centre of the lattice (Figure 4D rasters). Unlike in the case of WS-FHN networks,
3.3 Weak coupling and high sortedness leads to resonance in the SRK networks
For the WS-SRK networks, we found that the intra-population isosurfaces corresponding to the phase transition between quiescence and activity separate for weak coupling and high
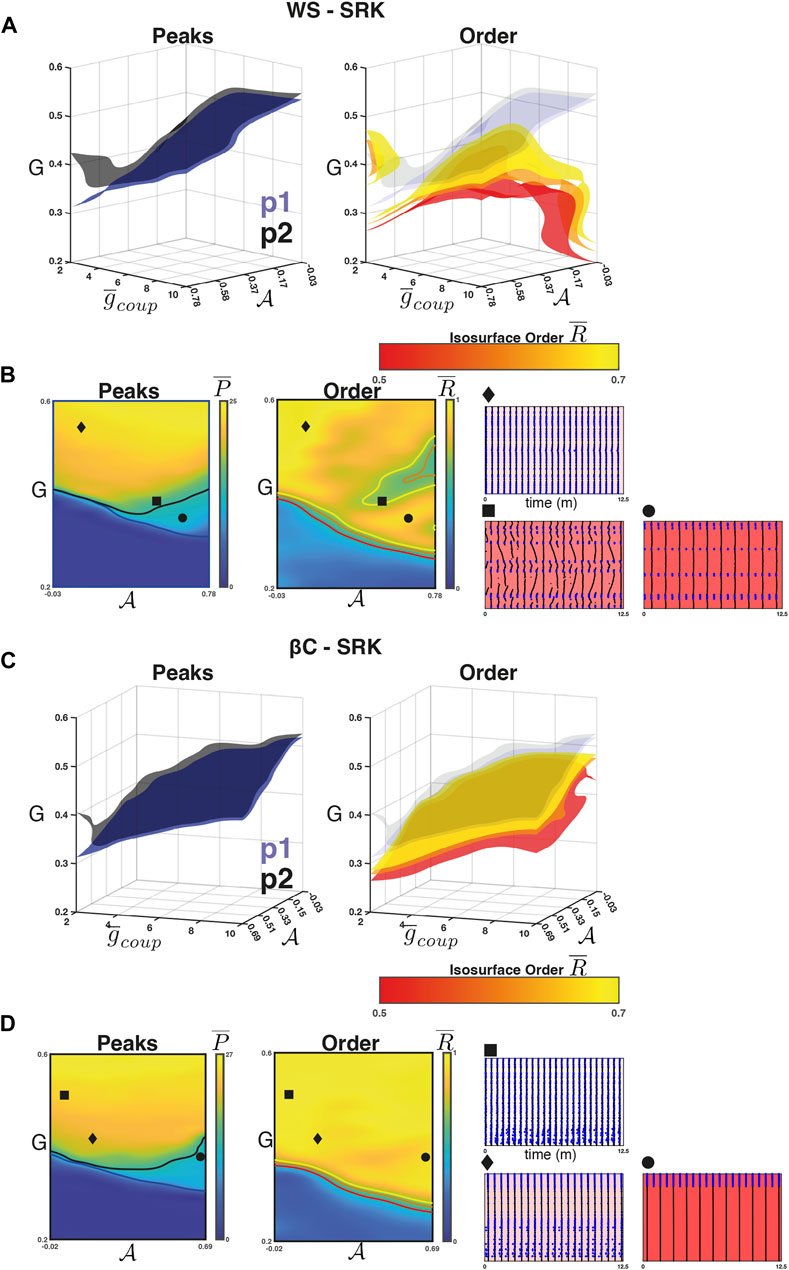
FIGURE 5. Weak coupling in the SRK networks. (A) Left panel: The isosurfaces for the
Figure 5A (right) shows the isosurfaces
For the βC-SRK networks, we repeated the experiment and found that a 2:1 resonance regime emerged in this case as well. Figures 5C, D (left panel) shows the separation in
4 Discussion
In this manuscript, we demonstrated how transitions to globally-coordinated activity are dependent on the degree of sortedness in population excitability. We used two prototypical models of cellular excitability where a small population was highly excitable, whilst a larger population was less excitable. This scenario has recently been hypothesised to be relevant in beta cell networks, in particular in the description of “hubs,” populations displaying high functional connectivity (Johnston et al., 2016), ‘wave-initiators’ (also known as “leaders”) (Šterk et al., 2023), and “first-responders” (Kravets et al., 2022). As the global drive to the network was increased, activity across the network transitioned from a globally inactive state to one in which subsets of nodes became active and synchronised their activity. By perturbing the spatial distribution of the highly excitable population, we showed that the drive strength at which such transitions occur is dependent on the sortedness of the network. Moreover, we highlighted the presence of other types of network solution, including partially synchronised states, slow waves, and 2:1 resonant states and explored how these depended on sortedness. These results have implications for insulin secretion in the pancreatic islets of Langerhans, and more general implications regarding transitions to synchrony and other forms of collective dynamics in networks of coupled, excitable units.
Pulsatile insulin secretion is a key component of healthy islet function and is driven by the coordinated electrical activity of β-cell populations (Bertram et al., 2007). In turn, the coordination of electrical activity is facilitated by local cell-cell communication through the gap junctional network (Ravier et al., 2005). Recently, many studies have demonstrated the existence of β-cell heterogeneity and its importance in determining network dynamics (Stožer et al., 2013; Benninger et al., 2014; Johnston et al., 2016; Westacott et al., 2017; Salem et al., 2019; Benninger and Kravets, 2021; Nasteska et al., 2021; Kravets et al., 2022; Šterk et al., 2023). Thus, a key question in this work has been to study the potential impact of the cytoarchitecture (e.g., spatial layout of heterogeneity) in modulating these dynamics. Our work suggests that it may be key to fully understanding the impact of heterogeneity on collective dynamics. We chose to focus on one form of heterogeneity, the cell-intrinsic excitability, as a case study; however, it is likely that cytoarchitecture of β-cell networks will modulate the impact of heterogeneity more generally.
We focussed on heterogeneity in cell-intrinsic excitability because of its critical role in delineating subpopulations of pancreatic beta cell networks. High excitability is a feature of both the first-responder cells in the transient phase of glucose-stimulated insulin secretion (GSIS) (Salem et al., 2019; Benninger and Kravets, 2021; Kravets et al., 2022) and the wave-initiator population during the pulsatile phase of GSIS (Benninger et al., 2014). The existence of these populations has been heavily implicated as a feature of healthy pancreatic islets, as has their critical roles in driving the collective dynamics required for GSIS. However, defining these subpopulations is complex because they likely arise as an emergent property of the network, relying on an interplay of both cell-intrinsic properties (i.e., excitability and metabolism) and network properties (i.e., coupling strength and non-uniformity of heterogeneity) (Benninger et al., 2014; Westacott et al., 2017; Salem et al., 2019; Kravets et al., 2022; Šterk et al., 2023). For example, it appears that both first-responder cells (Kravets et al., 2022) and wave-initiator cells (Šterk et al., 2023) may have weaker local connectivity, a property that would isolate them from the hyperpolarising influence of less-excitable cells and could improve network responsiveness. Although our model did not include heterogeneous coupling; we did observe that weaker coupling lowers the activation threshold of the network (Figures 4A, 5A). Likewise, we demonstrated that the spatial aggregation of highly-excitable nodes, a network property previously demonstrated by Westacott et al. (2017), can also have this effect. On the other hand, our work also illustrates potential tradeoffs in these network features. When coupling is too weak, the network can fail to synchronise and waves can fail to propagate. When sortedness is too high, excitable clusters can fail to recruit the whole network.
A variety of simplifications have been used in modelling studies to provide insight into the collective dynamics of heterogeneous coupled oscillators. For example, in specific cases of heterogeneity under the assumption of isotropic coupling and in the limit of infinitely many nodes, the network state can be mapped exactly to a low dimensional description, in which boundaries representing dynamical transitions can be found in closed form (Watanabe and Strogatz, 1993; Ott and Antonsen, 2009; Montbrió et al., 2015). For networks with a large, but not infinite number of nodes, and with general types of structure and heterogeneity, such a transformation may not be available, meaning that exact prediction of transitions between dynamical states is largely intractable. Our approach was to perform Monte-Carlo sampling across networks and initial conditions to elucidate common behaviours and trends regarding such transitions, taking advantage of the robustness of results across trials. In spite of the analytical intractability of the system, our results are consistent with the notion that the dynamics of the full network could be projected to a low dimensional manifold (e.g., the manifold representing full synchronisation of the subpopulations). In this regard, there are a number of approaches under active development, such as polynomial chaos expansion (Ghanem and Red-Horse, 2017), dynamic mode decomposition (Kutz et al., 2016), and proper orthogonal decomposition (Berkooz et al., 1993), that may facilitate construction of an approximate low dimensional system that captures the core dynamical features of the full heterogeneous network, thus allowing for a deeper exploration of network state transitions in the future.
To perform our study, we developed an algorithm that perturbs the sortedness of the network in a directed manner. The algorithm can be adapted to a range of domain geometries and network architectures, as showcased in our studies of Watts–Strogatz networks. Although our study focussed on conditions in which there are only two different populations, Section 2 discusses how our metrics can be extended to networks with more population types. Moreover, while we focussed on the case where the cells were homogeneous within a subpopulation, small changes to the algorithm could allow for the study of multimodal parameter distributions. We hope that this work will facilitate further study into the interplay of node-intrinsic heterogeneity and network features in driving the function of excitable networks, in particular beta-cell networks. To this end, future work will focus on adapting the sortedness algorithm to multiple parameters, which would allow us to further interrogate the importance of coupling strength, metabolic activity, electrical excitability, and neighbourhood on the emergence of functional roles in heterogeneous, excitable networks.
Data availability statement
The datasets presented in this study can be found in online repositories. The names of the repository/repositories and accession number(s) can be found below: https://github.com/dgalvis/network_spatial.
Author contributions
DG, DH, and KW conceived and designed the research; DG and KW developed the algorithm, model, and codebase; DG, DH, and KW interpreted the results; DG and KW prepared the figures; DG and KW drafted the manuscript; DG, DH, and KW edited and revised the manuscript.
Funding
DG acknowledges funding from the University of Birmingham Dynamic Investment Fund and the EPSRC Centre grant EP/N014391/2. DH was supported by MRC (MR/N00275X/1 and MR/S025618/1) and Diabetes UK (17/0005681) Project Grants, as well as a UKRI ERC Frontier Research Guarantee Grant (EP/X026833/1). This project has received funding from the European Research Council (ERC) under the European Union’s Horizon 2020 research and innovation programme (Starting Grant 715884 to DH). KW acknowledges funding from the MRC Fellowship MR/P01478X/1 and the Hub for Quantitative Modelling in Healthcare EP/T017856/1.
Conflict of interest
The authors declare that the research was conducted in the absence of any commercial or financial relationships that could be construed as a potential conflict of interest.
Publisher’s note
All claims expressed in this article are solely those of the authors and do not necessarily represent those of their affiliated organizations, or those of the publisher, the editors and the reviewers. Any product that may be evaluated in this article, or claim that may be made by its manufacturer, is not guaranteed or endorsed by the publisher.
Supplementary material
The Supplementary Material for this article can be found online at: https://www.frontiersin.org/articles/10.3389/fnetp.2023.1170930/full#supplementary-material
References
Ashcroft Frances, M., and Rorsman, P. (1989). Electrophysiology of the pancreatic beta cell. Prog. Biophysics Mol. Biol. 54, 87–143. doi:10.1016/0079-6107(89)90013-8
Ashwin, P., and Swift, J. W. (1992). The dynamics of n weakly coupled identical oscillators. J. Nonlinear Sci. 2, 69–108. doi:10.1007/bf02429852
Barrio, R., Coombes, S., Desroches, M., Fenton, F., Luther, S., and Pueyo, E. (2020). Excitable dynamics in neural and cardiac systems. Commun. Nonlinear Sci. Numer. Simul. 86, 105275. doi:10.1016/j.cnsns.2020.105275
Barua, A. K., and Goel, P. (2016). Isles within islets: The lattice origin of small-world networks in pancreatic tissues. Phys. D. Nonlinear Phenom. 315, 49–57. doi:10.1016/J.PHYSD.2015.07.009
Benninger, R. K., and Hodson, D. J. (2018). New understanding of β-cell heterogeneity and in situ islet function. Diabetes 67, 537–547. doi:10.2337/dbi17-0040
Benninger, R. K., Hutchens, T., Head, W. S., McCaughey, M. J., Zhang, M., Marchand, S. J. L., et al. (2014). Intrinsic islet heterogeneity and gap junction coupling determine spatiotemporal Ca²⁺ wave dynamics. Biophysical J. 107, 2723–2733. doi:10.1016/J.BPJ.2014.10.048
Benninger, R. K., and Kravets, V. (2021). The physiological role of β-cell heterogeneity in pancreatic islet function. Nat. Rev. Endocrinol. 18, 0123456789–123456822. doi:10.1038/s41574-021-00568-0
Benninger, R. K. P., Head, W. S., Zhang, M., Satin, L. S., and Piston, D. W. (2011). Gap junctions and other mechanisms of cell-cell communication regulate basal insulin secretion in the pancreatic islet. J. Physiology 589, 5453–5466. doi:10.1113/jphysiol.2011.218909
Berkooz, G., Holmes, P., and Lumley, J. L. (1993). The proper orthogonal decomposition in the analysis of turbulent flows. Annu. Rev. Fluid Mech. 25, 539–575. doi:10.1146/annurev.fl.25.010193.002543
Bertram, R., Satin, L. S., Pedersen, M. G., Luciani, D. S., and Sherman, A. (2007). Interaction of glycolysis and mitochondrial respiration in metabolic oscillations of pancreatic islets. Biophysical J. 92, 1544–1555. doi:10.1529/biophysj.106.097154
Bick, C., Goodfellow, M., Laing, C. R., and Martens, E. A. (2020). Understanding the dynamics of biological and neural oscillator networks through exact mean-field reductions: A review. J. Math. Neurosci. 10, 9. doi:10.1186/s13408-020-00086-9
Bittihn, P., Berg, S., Parlitz, U., and Luther, S. (2017). Emergent dynamics of spatio-temporal chaos in a heterogeneous excitable medium. Chaos 27, 093931. doi:10.1063/1.4999604
Börgers, C., Epstein, S., and Kopell, N. J. (2005). Background gamma rhythmicity and attention in cortical local circuits: A computational study. Proc. Natl. Acad. Sci. U. S. A. 102, 7002–7007. doi:10.1073/pnas.0502366102
Börgers, C., and Kopell, N. (2003). Synchronization in networks of excitatory and inhibitory neurons with sparse, random connectivity. Neural Comput. 15, 509–538. doi:10.1162/089976603321192059
Braun, M., Ramracheya, R., Bengtsson, M., Zhang, Q., Karanauskaite, J., Partridge, C., et al. (2008). Voltage-gated ion channels in human pancreatic beta-cells: Electrophysiological characterization and role in insulin secretion. Diabetes 57, 1618–1628. doi:10.2337/db07-0991
Cabrera, O., Berman, D. M., Kenyon, N. S., Ricordi, C., Berggren, P.-O., and Caicedo, A. (2006). The unique cytoarchitecture of human pancreatic islets has implications for islet cell function. Proc. Natl. Acad. Sci. U. S. A. 103, 2334–2339. doi:10.1073/pnas.0510790103
De Maesschalck, P., and Wechselberger, M. (2015). Neural excitability and singular bifurcations. J. Math. Neurosci. 5, 29. doi:10.1186/s13408-015-0029-2
Delgado, M. d. M., Miranda, M., Alvarez, S. J., Gurarie, E., Fagan, W. F., Penteriani, V., et al. (2018). The importance of individual variation in the dynamics of animal collective movements. Philosophical Trans. R. Soc. B Biol. Sci. 373, 20170008. doi:10.1098/rstb.2017.0008
Dolenšek, J., Stožer, A., Klemen, M. S., Miller, E. W., and Rupnik, M. S. (2013). The relationship between membrane potential and calcium dynamics in glucose-stimulated beta cell syncytium in acute mouse pancreas tissue slices. PLoS ONE 8, 823744–e82416. doi:10.1371/journal.pone.0082374
Dwulet, J. A. M., Ludin, N. W., Piscopio, R. A., Schleicher, W. E., Moua, O., Westacott, M. J., et al. (2019). How heterogeneity in glucokinase and gap-junction coupling determines the islet [ca2+] response. Biophysical J. 117, 2188–2203. doi:10.1016/J.BPJ.2019.10.037
Dwulet, J. M., Briggs, J. K., and Benninger, R. K. (2021). Small subpopulations of β-cells do not drive islet oscillatory [Ca2+] dynamics via gap junction communication. PLoS Comput. Biol. 17, 10089488–e1009031. doi:10.1371/journal.pcbi.1008948
Ermentrout, B. (2002). Simulating, analysing and animating dynamical systems: A guide to xppaut for researchers and students (siam).
Fitzhugh, R. (1961). Impulses and physiological states in theoretical models of nerve membrane. Biophysical J. 1, 445–466. doi:10.1016/s0006-3495(61)86902-6
Fretter, C., Lesne, A., Hilgetag, C. C., and Hütt, M. T. (2017). Topological determinants of self-sustained activity in a simple model of excitable dynamics on graphs. Sci. Rep. 7, 42340–42411. doi:10.1038/srep42340
Gaiteri, C., and Rubin, J. E. (2011). The interaction of intrinsic dynamics and network topology in determining network burst synchrony. Front. Comput. Neurosci. 5, 10. doi:10.3389/fncom.2011.00010
Ghanem, R., and Red-Horse, J. (2017). Polynomial chaos: Modeling, estimation, and approximation. Cham: Springer International Publishing, 521–551. doi:10.1007/978-3-319-12385-1_13
Gouwens, N. W., Sorensen, S. A., Berg, J., Lee, C., Jarsky, T., Ting, J., et al. (2019). Classification of electrophysiological and morphological neuron types in the mouse visual cortex. Nat. Neurosci. 22, 1182–1195. doi:10.1038/s41593-019-0417-0
Hodson, D. J., Schaeffer, M., Romanò, N., Fontanaud, P., Lafont, C., Birkenstock, J., et al. (2012). Existence of long-lasting experience-dependent plasticity in endocrine cell networks. Nat. Commun. 3, 605. doi:10.1038/ncomms1612
Hörning, M., Blanchard, F., Isomura, A., and Yoshikawa, K. (2017). Dynamics of spatiotemporal line defects and chaos control in complex excitable systems. Sci. Rep. 7, 7757–7810. doi:10.1038/s41598-017-08011-z
Izhikevich, E. M. (2000). Neural excitability, spiking and bursting. Int. J. Bifurcation Chaos 10, 1171–1266. doi:10.1142/s0218127400000840
Johnston, N. R., Mitchell, R. K., Haythorne, E., Trauner, D., Rutter, G. A., Hodson, D. J., et al. (2016). Beta cell hubs dictate pancreatic islet responses to Glucose. Cell Metab. 24, 389–401. doi:10.1016/j.cmet.2016.06.020
Kopell, N., Kramer, M. A., Malerba, P., and Whittington, M. A. (2010). Are different rhythms good for different functions? Front. Hum. Neurosci. 4, 187–189. doi:10.3389/fnhum.2010.00187
Kravets, V., Dwulet, J. A. M., Schleicher, W. E., Hodson, D. J., Davis, A. M., Pyle, L., et al. (2022). Functional architecture of pancreatic islets identifies a population of first responder cells that drive the first-phase calcium response. PLOS Biol. 20, e3001761. doi:10.1371/JOURNAL.PBIO.3001761
Kutz, J. N., Brunton, S. L., Brunton, B., and Proctor, J. L. (2016). Dynamic mode decomposition: Data-driven modelling of complex systems. SIAM.
Lambert, D., and Vanni, F. (2018). Complexity and heterogeneity in a dynamic network. Chaos, Solit. Fractals 108, 94–103. doi:10.1016/j.chaos.2018.01.024
Lipovsek, M., Bardy, C., Cadwell, C. R., Hadley, K., Kobak, D., and Tripathy, S. J. (2021). Patch-seq: Past, present, and future. J. Neurosci. 41, 937–946. doi:10.1523/JNEUROSCI.1653-20.2020
Majumder, R., Feola, I., Teplenin, A. S., de Vries, A. A., Panfilov, A. V., and Pijnappels, D. A. (2018). Optogenetics enables real-time spatiotemporal control over spiral wave dynamics in an excitable cardiac system. eLife 7, 410766–e41155. doi:10.7554/eLife.41076
Manchanda, K., Bose, A., and Ramaswamy, R. (2017). Collective dynamics in heterogeneous networks of neuronal cellular automata. Phys. A Stat. Mech. its Appl. 487, 111–124. doi:10.1016/j.physa.2017.06.021
Mathiesen, J., Angheluta, L., Ahlgren, P. T., and Jensen, M. H. (2013). Excitable human dynamics driven by extrinsic events in massive communities. Proc. Natl. Acad. Sci. U. S. A. 110, 17259–17262. doi:10.1073/pnas.1304179110
McKenna, J. P., Ha, J., Merrins, M. J., Satin, L. S., Sherman, A., and Bertram, R. (2016). Ca2+ effects on ATP production and consumption have regulatory roles on oscillatory islet activity. Biophysical J. 110, 733–742. doi:10.1016/j.bpj.2015.11.3526
Montbrió, E., Pazó, D., and Roxin, A. (2015). Macroscopic description for networks of spiking neurons. Phys. Rev. X 5, 021028–21115. doi:10.1103/PhysRevX.5.021028
Nagumo, J., Arimoto, S., and Yoshizawa, S. (1962). An active pulse transmission line simulating nerve axon. Proc. IEEE 117, 2061–2070. doi:10.1109/jrproc.1962.288235
Nasteska, D., Fine, N. H., Ashford, F. B., Cuozzo, F., Viloria, K., Smith, G., et al. (2021). Pdx1low mafalow beta-cells contribute to islet function and insulin release. Nat. Commun. 12, 674–719. doi:10.1038/s41467-020-20632-z
Ott, E., and Antonsen, T. M. (2009). Long time evolution of phase oscillator systems. Chaos 19, 023117. doi:10.1063/1.3136851
Ottino-Löffler, B., and Strogatz, S. H. (2016). Kuramoto model with uniformly spaced frequencies: Finite- N asymptotics of the locking threshold. Phys. Rev. E 93, 062220. doi:10.1103/PhysRevE.93.062220
Pedersen, M. G., Brøns, M., and Sørensen, M. P. (2022). Amplitude-modulated spiking as a novel route to bursting: Coupling-induced mixed-mode oscillations by symmetry breaking. Chaos Interdiscip. J. Nonlinear Sci. 32, 013121. doi:10.1063/5.0072497
Podobnik, B., Korošak, D., Skelin Klemen, M., Stožer, A., Dolenšek, J., Slak Rupnik, M., et al. (2020). β cells operate collectively to help maintain glucose homeostasis. Biophysical J. 118, 2588–2595. doi:10.1016/j.bpj.2020.04.005
Punckt, C., Bodega, P. S., Kaira, P., and Rotermund, H. H. (2015). Wildfires in the lab: Simple experiment and models for the exploration of excitable dynamics. J. Chem. Educ. 92, 1330–1337. doi:10.1021/ed500714f
Ravier, M. A., ldenagel, M. G., Charollais, A., Gjinovci, A., Caille, D., hl, G. S., et al. (2005). Loss of connexin36 channels alters beta-cell coupling, islet synchronization of glucose-induced Ca2+ and insulin oscillations, and basal insulin release. DIABETES 54, 1798–1807. doi:10.2337/diabetes.54.6.1798
Rorsman, P., and Braun, M. (2013). Regulation of insulin secretion in human pancreatic islets. Annu. Rev. Physiology 75, 155–179. doi:10.1146/annurev-physiol-030212-183754
Salem, V., Silva, L., Suba, K., Georgiadou, E., Neda Mousavy Gharavy, S., Akhtar, N., et al. (2019). Leader β-cells coordinate ca2+ dynamics across pancreatic islets in vivo. Nat. Metab. 1, 615–629. doi:10.1038/s42255-019-0075-2
Sanchez-Cardenas, C., Fontanaud, P., He, Z., Lafont, C., Meunier, A. C., Schaeffer, M., et al. (2010). Pituitary growth hormone network responses are sexually dimorphic and regulated by gonadal steroids in adulthood. Proc. Natl. Acad. Sci. U. S. A. 107, 21878–21883. doi:10.1073/pnas.1010849107
Satin, L. S., Zhang, Q., and Rorsman, P. (2020). Take Me to Your Leader”: An electrophysiological appraisal of the role of hub cells in pancreatic islets. Diabetes 69, 830–836. doi:10.2337/dbi19-0012
Scialla, S., Loppini, A., Patriarca, M., and Heinsalu, E. (2021). Hubs, diversity, and synchronization in fitzhugh-nagumo oscillator networks: Resonance effects and biophysical implications. Phys. Rev. E 103, 052211. doi:10.1103/PhysRevE.103.052211
Sherman, A., Rinzel, J., and Keizer, J. (1988). Emergence of organized bursting in clusters of pancreatic beta-cells by channel sharing. Biophysical J. 54, 411–425. doi:10.1016/S0006-3495(88)82975-8
Šterk, M., Dolenšek, J., Klemen, M. S., Bombek, L. K., Leitgeb, E. P., Kerčmar, J., et al. (2023). Functional characteristics of hub and wave initiator cells in beta cell networks. Biophysical J. 122, 784–801. doi:10.1016/j.bpj.2023.01.039
Stožer, A., Gosak, M., Dolenšek, J., Perc, M., Marhl, M., Rupnik, M. S., et al. (2013). Functional connectivity in islets of langerhans from mouse pancreas tissue slices. PLoS Comput. Biol. 9, 1002923. doi:10.1371/journal.pcbi.1002923
Terrien, S., Pammi, V. A., Broderick, N. G. R., Braive, R., Beaudoin, G., Sagnes, I., et al. (2020). Equalization of pulse timings in an excitable microlaser system with delay. Phys. Rev. Res. 2, 023012. doi:10.1103/physrevresearch.2.023012
Terrien, S., Pammi, V. A., Krauskopf, B., Broderick, N. G., and Barbay, S. (2021). Pulse-timing symmetry breaking in an excitable optical system with delay. Phys. Rev. E 103, 012210. doi:10.1103/PhysRevE.103.012210
Vannucchi, F., and Boccaletti, S. (2004). Chaotic spreading of epidemics in complex networks of excitable units. Math. Biosci. Eng. 1, 49–55. doi:10.3934/mbe.2004.1.49
Watanabe, S., and Strogatz, S. H. (1993). Integrability of a globally coupled oscillator array. Phys. Rev. Lett. 70, 2391–2394. doi:10.1103/PhysRevLett.70.2391
Watts, D. J., and Strogatz, S. H. (1998). Collective dynamics of ‘small-world’ networks. Nature 393, 440–442. doi:10.1038/30918
Wedgwood, K. C., Słowiński, P., Manson, J., Tsaneva-Atanasova, K., and Krauskopf, B. (2021). Robust spike timing in an excitable cell with delayed feedback. J. R. Soc. Interface 18, 20210029. doi:10.1098/rsif.2021.0029
Westacott, M. J., Ludin, N. W., and Benninger, R. K. (2017). Spatially organized β-cell subpopulations control electrical dynamics across islets of langerhans. Biophysical J. 113, 1093–1108. doi:10.1016/j.bpj.2017.07.021
Keywords: excitable systems, collective dynamics, beta cell, sortedness, heterogeneous networks, phase transitions
Citation: Galvis D, Hodson DJ and Wedgwood KCA (2023) Spatial distribution of heterogeneity as a modulator of collective dynamics in pancreatic beta-cell networks and beyond. Front. Netw. Physiol. 3:1170930. doi: 10.3389/fnetp.2023.1170930
Received: 21 February 2023; Accepted: 17 March 2023;
Published: 24 March 2023.
Edited by:
Marko Gosak, University of Maribor, SloveniaReviewed by:
Shangbin Chen, Huazhong University of Science and Technology, ChinaStefano Scialla, National Institute of Chemical Physics and Biophysics, Estonia
Copyright © 2023 Galvis, Hodson and Wedgwood. This is an open-access article distributed under the terms of the Creative Commons Attribution License (CC BY). The use, distribution or reproduction in other forums is permitted, provided the original author(s) and the copyright owner(s) are credited and that the original publication in this journal is cited, in accordance with accepted academic practice. No use, distribution or reproduction is permitted which does not comply with these terms.
*Correspondence: Daniel Galvis, d.galvis@bham.ac.uk