- 1Community Health Service Center, Geriatric Hospital Affiliated to Wuhan University of Science and Technology, Wuhan, China
- 2Brain Science and Advanced Technology Institute, School of Medicine, Wuhan University of Science and Technology, Wuhan, China
- 3School of Public Health, Wuhan University of Science and Technology, Wuhan, China
- 4Community Health Service Center, Wuchang Hospital, Wuhan, China
Ribonucleic acid (RNA) methylation is the most abundant modification in biological systems, accounting for 60% of all RNA modifications, and affects multiple aspects of RNA (including mRNAs, tRNAs, rRNAs, microRNAs, and long non-coding RNAs). Dysregulation of RNA methylation causes many developmental diseases through various mechanisms mediated by N6-methyladenosine (m6A), 5-methylcytosine (m5C), N1-methyladenosine (m1A), 5-hydroxymethylcytosine (hm5C), and pseudouridine (Ψ). The emerging tools of RNA methylation can be used as diagnostic, preventive, and therapeutic markers. Here, we review the accumulated discoveries to date regarding the biological function and dynamic regulation of RNA methylation/modification, as well as the most popularly used techniques applied for profiling RNA epitranscriptome, to provide new ideas for growth and development.
1 Introduction
Ribonucleic acid (RNA) modification plays an important role in linking deoxyribonucleic acid (DNA) to proteins during the transmission of genetic information. More than 100 post-transcriptional RNA modifications have been identified in important biological processes in viruses, archaea, bacteria, and eukaryotes. Methylation accounts for 60% of the total RNA modifications. RNA methylation was discovered as early as the 1970s (Cantara et al., 2011), and it is widely distributed in messenger RNA (mRNA), transfer RNA (tRNA), ribosomal RNA (rRNA), small nuclear RNA (snRNA), small nucleolar RNA (snoRNA), and micro RNA (El Yacoubi et al., 2012). Currently, various distinct modifications in natural RNA have been characterized: N1-methyladenosine (m1A), N6-methyladenosine (m6A), N6, 2′-O-dimethyladenosine (m6Am), 5-methylcytosine (m5C), 5-hydroxymethylcytosine (hm5C), pseudouridine (Ψ), and Adenosine to inosine editing (A-to-I editing), etc. (Roundtree et al., 2017a; Walkley and Li, 2017; Zhao et al., 2020). Among these, m6A, m5C, m1A, and Ψ have been well studied (Figure 1). Compared with DNA methylation, RNA methylation is more complex and diverse, participates in the regulation of many more biological processes, and determines the diversification of RNA post-transcriptional modifications. Mutations in approximately half of the currently known RNA methylation enzymes have been linked to human diseases, including cancer, cardiovascular diseases, congenital genetic disabilities, metabolic diseases, neurological disorders, and mitochondrial-related defects (Jonkhout et al., 2017; Barbieri and Kouzarides, 2020). Here, we review the discoveries to date regarding the dynamic regulation and key roles of RNA methylation.
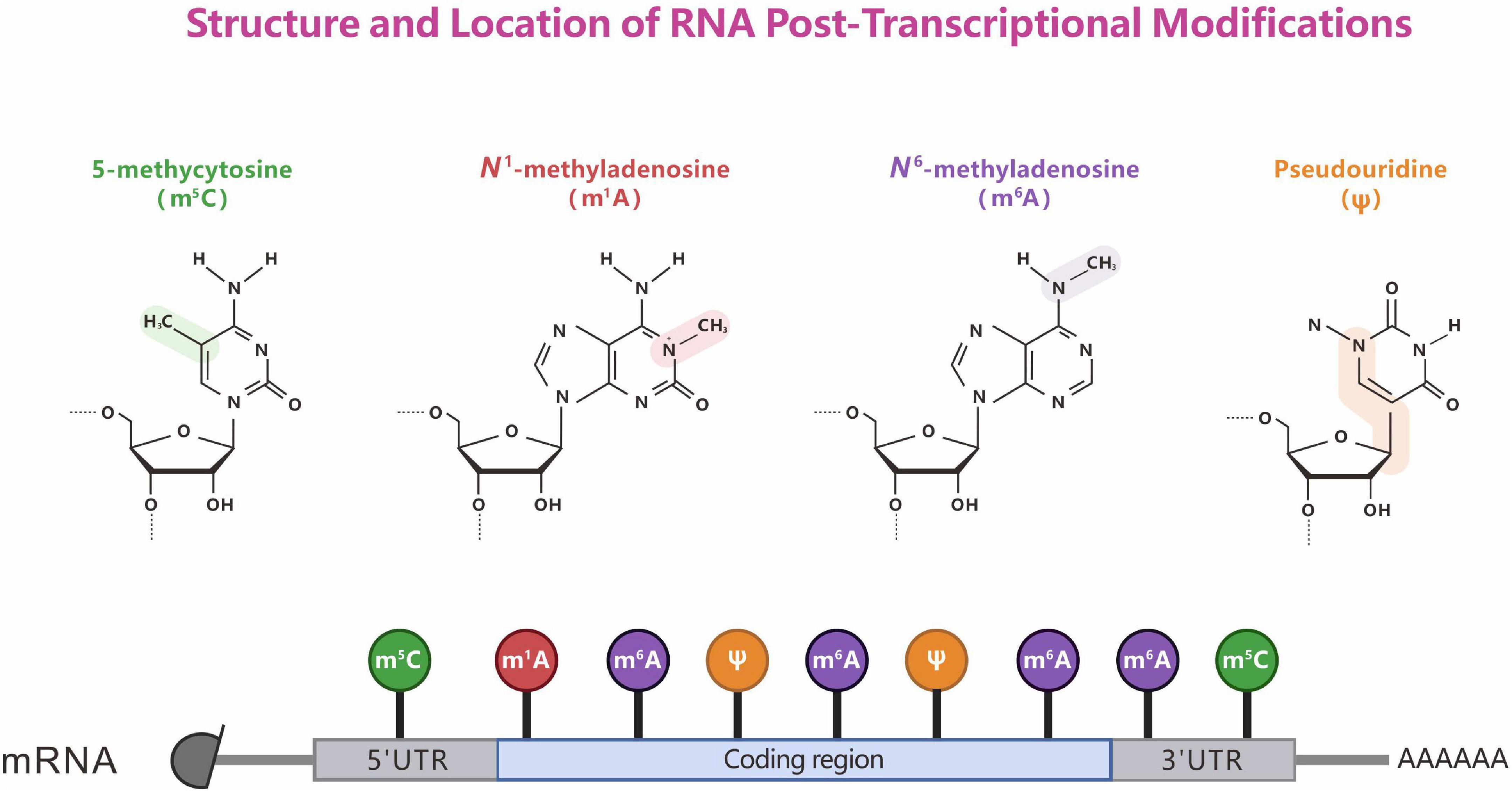
Figure 1. Structure and location of representative RNA post-transcriptional modifications. The chemical properties of RNA modifications (top). The known sites (bottom) of m6A, m1A, m5C, and Ψ modification on mRNA.
2 Biological patterns of RNA methylation/modification
2.1 N6-methyladenosine (m6A)
N6-methyladenosine (m6A), the most abundant methylation modification in eukaryotic mRNA, is the most thoroughly studied type of RNA modification (Schwartz et al., 2013). m6A has been found in many eukaryotes, ranging from yeast, Arabidopsis, and Drosophila to mammals and even in viruses (Krug et al., 1976; Schwartz et al., 2013; Luo et al., 2014; Lin et al., 2017; Guo et al., 2018). In mammals, m6A is widely distributed in multiple tissues, with the highest level of m6A in the brain, kidney, and liver (Meyer et al., 2012). Although the existence of m6A on RNA was identified as early as the 1970s, little was known about its precise location, temporal dynamics, and regulation until 2011 (Desrosiers et al., 1974). With the identification of the first demethylase fat mass and obesity-associated protein (FTO) of RNA m6A and the development of antibody enrichment and high-throughput sequencing technology, accurate mapping of the transcriptome-level m6A distribution has been achieved (Jia et al., 2011). The sequence near the m6A methylation site on mRNA is highly conserved and mainly occurs on the adenine of RRm6ACH, where R = G/A (G > A) and H = U/A/C (U > A > C) (Harper et al., 1990). In addition, whole-transcriptome m6A sequencing and comprehensive bioinformatics analysis of human and mouse samples revealed that m6A modifications are species-specific (Liu et al., 2020a). m6A methylation is a reversible dynamic modification in many species and is jointly regulated by methyltransferases (writers), demethylases (erasers), and binding proteins (readers). The main components of the methyltransferase complex that have been identified include methyltransferase 3 (METTL3), methyltransferase 14 (METTL14), Wilms’ tumor 1-associating protein (WTAP), and KIAA1429 (vir-like m6A methyltransferase associated) (Oerum et al., 2021). The demethylases FTO and AlkB homolog 5 (ALKBH5) can reverse methylation (Figure 2; Liu et al., 2013; Zheng et al., 2013).
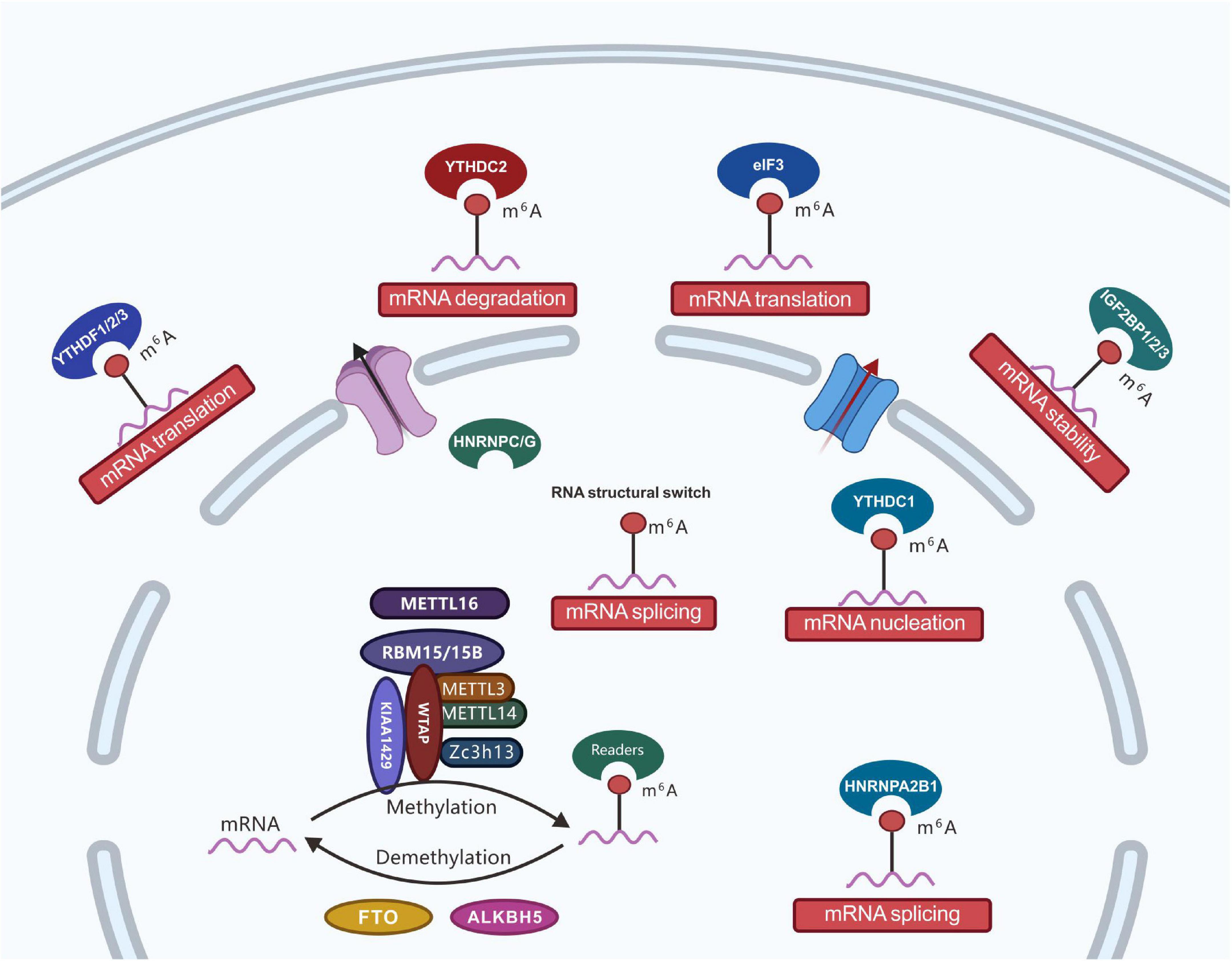
Figure 2. Regulatory roles of m6A effector proteins. The m6A effectors, including writer proteins (i.e., m6A methyltransferase complex: core subunits METTL3 and METTL14 and additional adaptors proteins including WTAP, ZC3H13, VIRMA, METTL16, RBM15/15B, HAKAI, and KIAA1429); eraser proteins (i.e., RNA demethylases: FTO and ALKBH5), and reader (three classes of reader proteins: ➀ YTH-domain-containing proteins, including YTHDC1, YTHDC2, YTHDF1, YTHDF2, and YTHDF3; ➁ proteins favoring RNA-binding events, including HNRNPA2B1, HNRNPC, and HNRNPG; ➂ RNA binding proteins, including eIF3, IGF2BP1, IGF2BP2, and IGF2BP3). With the development of RNA modification detection technology, m6A modifications have been determined to functionally regulate the transcriptome of eukaryotes and processes, such as mRNA stability, splicing, nucleation, localization, and translation.
The biological function of m6A is mainly through the post-transcriptional regulation of RNA by m6A-binding proteins. Currently known binding proteins include the YT521-B homology (YTH) domain proteins (YTHDF1, YTHDF2, YTHDF3, YTHDC1, and YTHDC2) and nuclear heterogeneous ribonucleoprotein HNRNP family proteins (HNRNPA2B1, HNRNPC, and HNRNPG) (Alarcón et al., 2015; Roundtree et al., 2017b). With the development of RNA modification detection technology, m6A modifications have been determined to functionally regulate the transcriptome of eukaryotes and processes such as mRNA stability, splicing, nucleation, localization, and translation (Figure 2). Furthermore, m6A is involved in a variety of biological processes such as stem cell differentiation, cell division, gametogenesis, and biological rhythms. Under the catalytic regulation of relevant enzymes, m6A participates in various diseases, including tumors, obesity, and infertility (Jiang et al., 2021).
Knocking out the m6A demethylase gene ALKBH5 accelerates the nuclear export of mRNA (Zheng et al., 2013), whereas RNAi silencing of the core of the m6A methyltransferase complex METTL3 inhibits the nuclear transport process (Fustin et al., 2013), thereby demonstrating that m6A modification can promote nuclear transfer. The nuclear export of mRNA is a bridge connecting mRNA processing in the nucleus and translation in the cytoplasm. Further, nuclear export is coupled with each step of pre-mRNA processing, and only properly processed mRNA is nucleated and translated into protein.
As an exocyclic ring amine involved in Watson Crick base pairing, the rotation direction of Watson Crick base pairing of m6A is opposite to the U force of the carbon-nitrogen bond, showing the methyl group in the reverse conformation, which destabilizes the RNA double strand to local unstructured transcripts (Roost et al., 2015). m6A tends to weaken these structures. This modification is enriched in the 3′-untranslated region (UTR) and alternative splicing exons and introns and is related to mRNA preprocessing, such as splicing regulation and polyadenylation (Meyer et al., 2012). Structural switches, including helices with buried binding sites, can be refolded to allow access to their respective protein partners (Liu et al., 2015). The formation of hybrid structures, such as miRNA-target interactions, is also affected by m6A (Brummer et al., 2017).
2.2 5-methylcytosine (m5C)
RNA m5C modifications were discovered more than 40 years ago. Similar to m6A, RNA m5C modifications are dynamically reversible. Methyltransferase uses S-adenosyl methionine (SAM) as a methyl donor to methylate cytosine (C) to form m5C (Figure 3). The distribution and function of m5C may be species- and tissue-specific. Bisulfite treatment combined with transcriptome sequencing has identified m5C modification sites in thousands of mRNAs in HeLa cells (Squires et al., 2012). m5C antibody immunoprecipitation combined with bisulfite sequencing allowed the identification of multiple m5C modifications in archaeal mRNA. The conserved sequence of AU (m5C) GANGU was consistent with the m5C conserved sequence in archaeal rRNA (Edelheit et al., 2013). The discovery of the m5C methyltransferase NSUN2 (NOP2/Sun RNA methyltransferase family member 2) and the binding protein ALYREF (Aly/REF export factor) also proved that RNA m5C modifications have dynamic reversibility (Yang et al., 2017b). RNA m5C methylation is widespread in cells and plays an important role in various physiological (Flores et al., 2017) and pathological processes, such as tumors (Huang et al., 2021; Nombela et al., 2021), neurological disorders (Van Haute et al., 2019), viral infections (Wnuk et al., 2020), and organism ontogeny (Flores et al., 2017). However, research on RNA m5C methylation is still in its infancy.
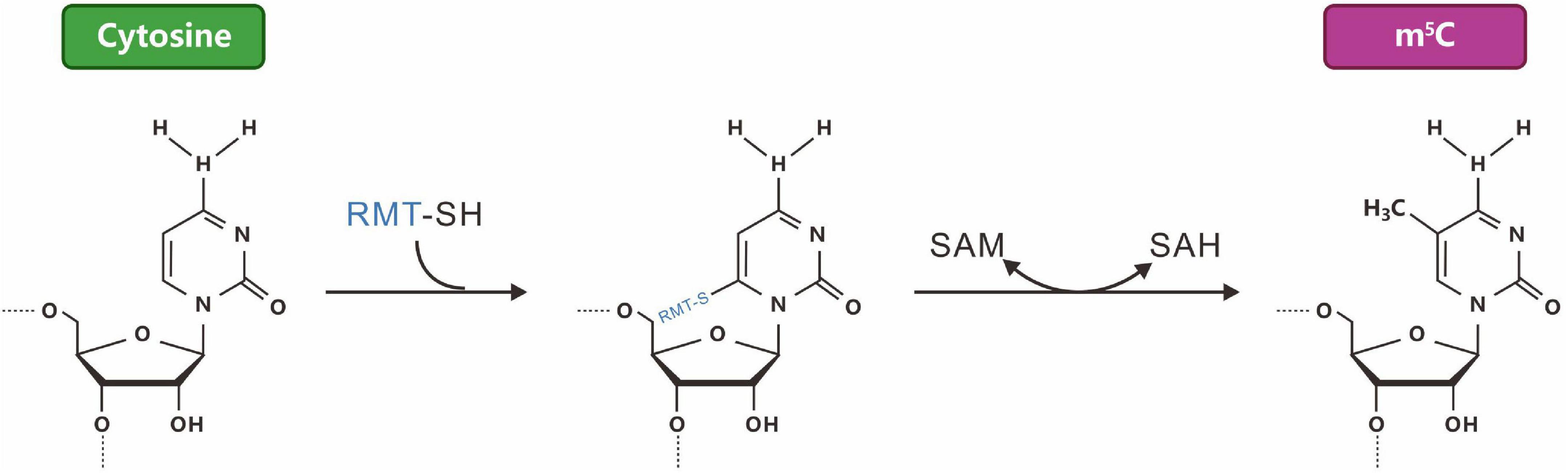
Figure 3. The reaction mechanism of a typical m5C-RNA cytosine methyltransferase [m(5)C-RMTs, in blue]. M5C, formed by the methylation of carbon 5 of cytosine.
RNA m5C modification can be recognized by the transporter linker protein ALYREF, which promotes the transport of related mRNA out of the nucleus. Knockout of the m5C-modified methyltransferase gene NSUN2 blocks the shuttling of the ALYREF protein between the nucleus and cytoplasm (Yang et al., 2017b). In addition, the patterns of m5C distribution on mRNA in relation to cis-acting regulatory motifs and miRNA/RISC-binding sites suggest that this modification may be involved in the post-transcriptional regulation of mRNA metabolism (Squires et al., 2012). NSUN2-mediated methylation is required to process non-coding vault RNAs (vtRNAs) into small vault RNAs (svRNAs); however, consequences in the downstream coding transcripts have not emerged because of the defect of RNA m5C modification (Hussain et al., 2013). ALYREF can recognize m5C in mRNA via a methyl-specific RNA-binding motif and regulate the export of bound transcripts in an NSUN2-dependent manner (Yang et al., 2017b), whereas hm5C, derived from the Tet-dependent oxidation of m6C, preferentially marks mRNAs within coding regions and favors the translation of Drosophila transcripts (Fu et al., 2014; Delatte et al., 2016).
2.3 Other RNA methylation/modifications
In addition to m6A and m5C, other RNA modifications, including m1A, m6Am, hm5C, and pseudouridine (Ψ), have been a hot field of research in recent years.
2.3.1 N1-methyladenosine (m1A)
RNA m1A methylation was first discovered in non-coding RNAs such as rRNA and tRNA and is widely present in prokaryotic and eukaryotic mRNAs. Unlike m6A methylation, m1A methylation occurs at the N1 position of the adenosine base group. It carries a positive charge by blocking Watson-Crick base-pairing under physiological conditions. Thus, it can drastically alter protein-RNA interactions and RNA secondary structures through electrostatic effects. m1A maps uniquely to positions near the translation start and first splice sites in coding transcripts and correlates with the upregulation of translation (Dominissini et al., 2016). m1A can be removed by ALKBH3 and is responsive to various types of cellular stress (Li et al., 2015a). However, the methyltransferases and binding proteins of m1A remain unknown; hence, its specific function and mechanism of action need to be further explored.
In mRNA, m1A exists in the highly structured 5′UTRs, indicating that it may change the predicted secondary structure (Dominissini et al., 2016; Li et al., 2016c). In the loop structure, this positive charge may stabilize interactions with the RNA phosphate backbone. The methylation level of m1A in transcripts is related to increased translation, which may be due to the availability or direct recruitment of initiation factors and extension factors. The positive charge of this modification enables it to adapt to specific protein RNA and unique RNA-RNA interactions, and its biological effects are still unclear.
2.3.2 N6, 2′-O-dimethyladenosine (m6Am)
The N6, 2′-O-dimethyladenosine (m6Am) modification was found at the first nucleotide of certain mRNAs (Crain et al., 1978). m6Am is formed by the combination of 2′-O-methyltransferase (2′-O-MTase) and 2′-O-methyladenosine-N6-methyltransferase. Adenosine is methylated by 2′-O-methyltransferase (2′-O-MTase) to form Am, which can then be methylated by 2′-O-methyladenosine-N6-methyltransferase to form m6Am (Wei et al., 1975). Compared to m6A, the m6Am level in RNA is very low. Studies in H1-ESCs and GM12878 cells found that H1-ESC poly(A) + RNAs contained approximately three m6A nucleotides per 105 nucleotides compared to ∼100 m6A nucleotides per 105 nucleotides, revealing 33 times more m6A compared to m6Am (Molinie et al., 2016). m6Am and m6A share similar chemical characteristics; m6Am can be detected by m6A-seq, and it can be demethylated by FTO and is involved in the regulation of mRNA stability (Mauer et al., 2016). Chen et al. (2020a) demonstrated that METTL4 mediates the N6-methylating process of Am30 on U2 small nuclear RNA (snRNA) under an AAG motif in vitro and in vivo (Chen et al., 2020a). However, the binding protein of m6Am and molecular mechanisms involved in regulating its biological functions require further study.
2.3.3 5-hydroxymethylcytosine (hm5C)
Similar to m5C in DNA, m5C in RNA can be oxidized by ten-eleven translocation (Tet)-family enzymes to hm5C (Figure 4; Fu et al., 2014). The Tet family of Fe(II)- and 2-oxoglutarate-dependent dioxygenases can induce the oxidation of m5C to yield hm5C (Kriaucionis and Heintz, 2009; Ito et al., 2011). In Drosophila melanogaster, which lacks DNA hydroxymethylation, hm5C is present in greater than 1,500 mRNAs, and hm5C modification is mainly distributed in the exons of mRNA and contains CU-enriched conserved motifs (Delatte et al., 2016). hm5C modification can promote the translation efficiency of mRNA, which is significantly higher than that of RNA without hm5C modifications. After TET knockout, the level of hm5C modification in RNA is reduced, resulting in the abnormal development of the Drosophila brain (Delatte et al., 2016).
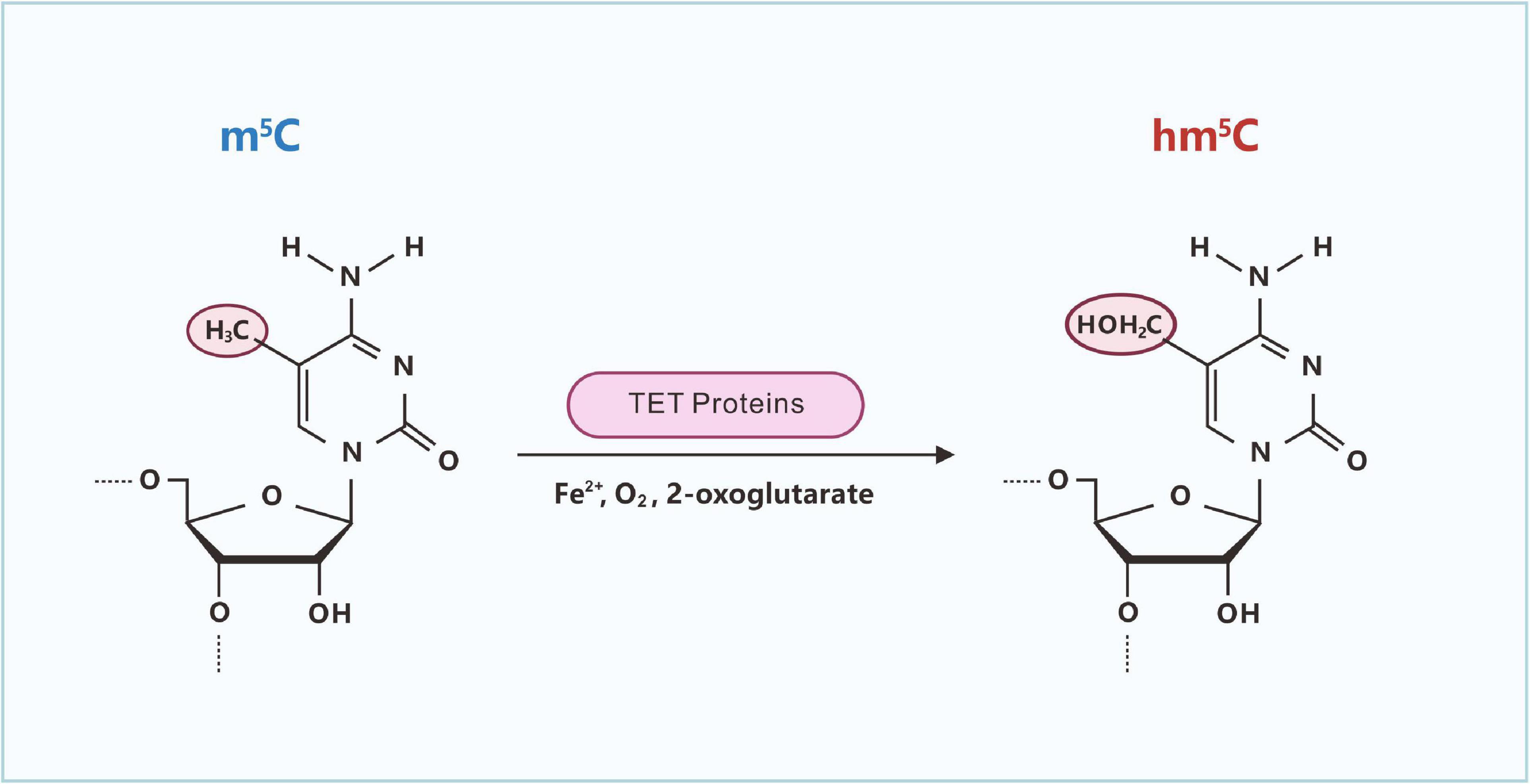
Figure 4. Ten-eleven translocation (Tet)-catalyzed formation of hm5C in RNA. The Tet family of Fe(II)- and 2-oxoglutarate-dependent dioxygenases can induce the oxidation of m5C to yield hm5C.
2.3.4 Pseudouridine (Ψ)
In addition to methylated modifications, there are some unmethylated modifications of RNA, such as ψ (sometimes referred to as pseudouracil). It is one of the most abundant forms of post-transcriptional RNA modifications, widely present in cellular RNA, and highly conserved among species. ψ is formed by the sequence-specific isomerization of uracil (U) (Figure 5), which is abundant in tRNA and rRNA (Goodman et al., 1968). Ψ is known to affect the secondary structure of RNA, and its function in altering stop codon read-through may also be biologically relevant (Karijolich and Yu, 2010; Fernandez et al., 2013).
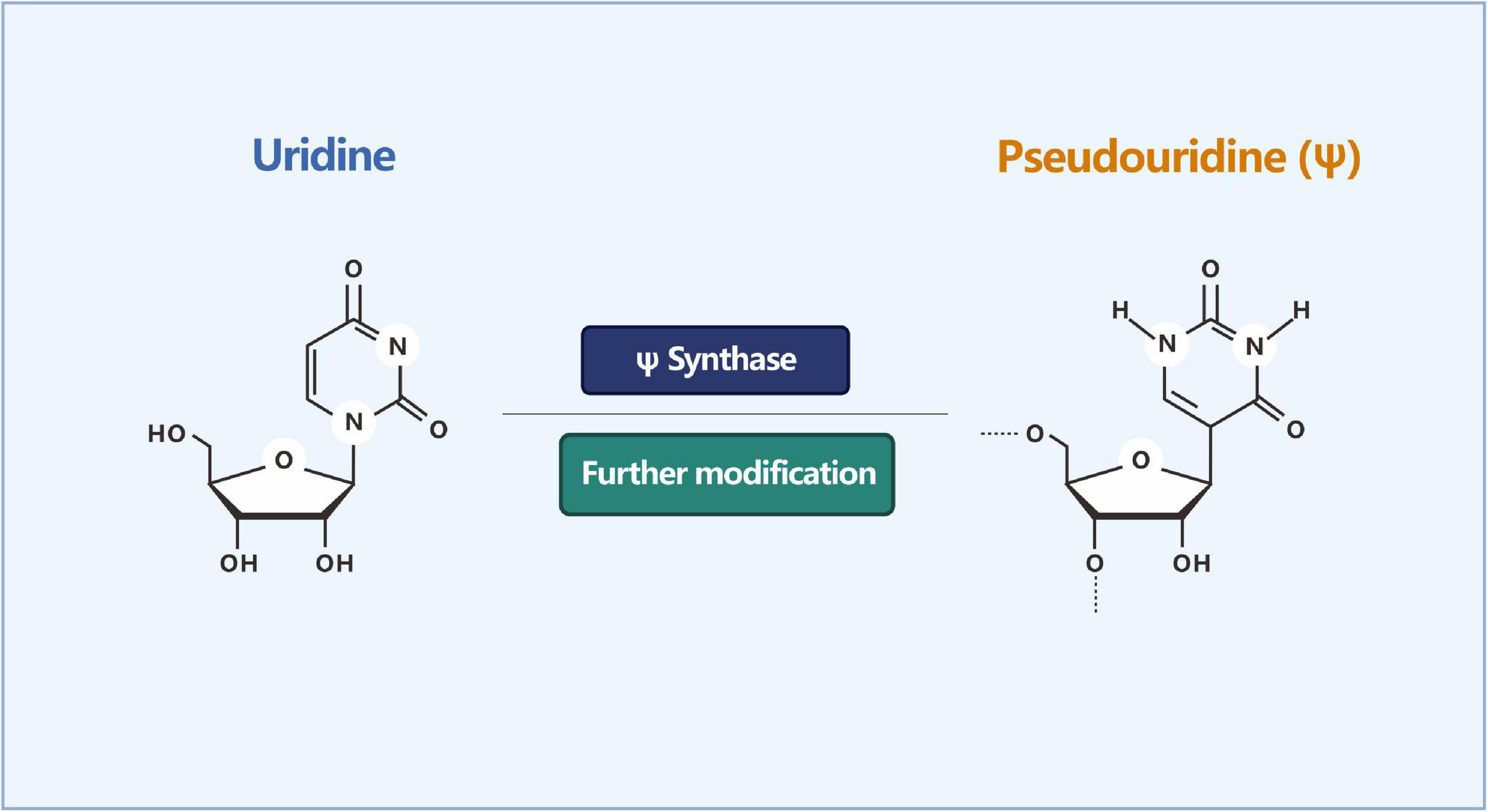
Figure 5. Isomerization reaction of uridine into pseudouridine (Ψ). ψ is formed by the sequence-specific isomerization of uracil (U).
3 Dynamic regulation of RNA methylation/modifications
3.1 Methyltransferase-writers
3.1.1 Core m6A methyltransferase complex components: METTL3, METTL14, and WTAP
The RNA m6A methyltransferase holoenzyme complex consists of at least two multicomponent factors of the whole methyltransferase called MT-A (∼ 200 kDa) and MT-B (∼ 800 kDa). However, only the METTL3 (methyltransferase like 3, ∼ 70 kDa) protein has been identified, and the subunit alone had no enzymatic activity (Tuck, 1992). METTL3 is widely present in various human tissues, especially in the testes (Leach and Tuck, 2001). METTL3 has two key domains that are used to combine SAM and catalyze the formation of m6A (Figure 6). Knocking down METTL3 causes apoptosis in human HeLa and HepG2 cells, accompanied by a significant decrease in m6A levels (Dominissini et al., 2012). Apart from the METTL3 component, other components of the methyltransferase complex have not been comprehensively studied. In 2014, an evolutionary analysis of the METTL3 family revealed the METTL14 and METTL4 genes, which are highly homologous to different families of METTL3 (Bujnicki et al., 2002). METTL14 is another component in the m6A methyltransferase complex with enzymatic activity (Liu et al., 2013). In HeLa and HEK 293FT cells, knocking down METTL14 resulted in a decrease in total mRNA of m6A content, while METTL14 and METTL3 interacted with each other. In vitro size exclusion chromatography (gel filtration) experiments and two-dimensional gel electrophoresis analysis showed that METTL14 and METTL3 can form a stable complex at a 1:1 ratio. In vitro experiments on the activity of enzymes involved in m6A formation showed that although a single METTL14 had slightly higher enzymatic activity than METTL3, the heterodimer formed by METTL14 and METTL3 with a strong preference for substrates had the highest enzymatic activity for the RNA of the stem-loop structure, which has no obvious secondary structures. It preferentially methylates GGACU, which is consistent with the conserved sequence of m6A distribution reported previously (Liu et al., 2013).
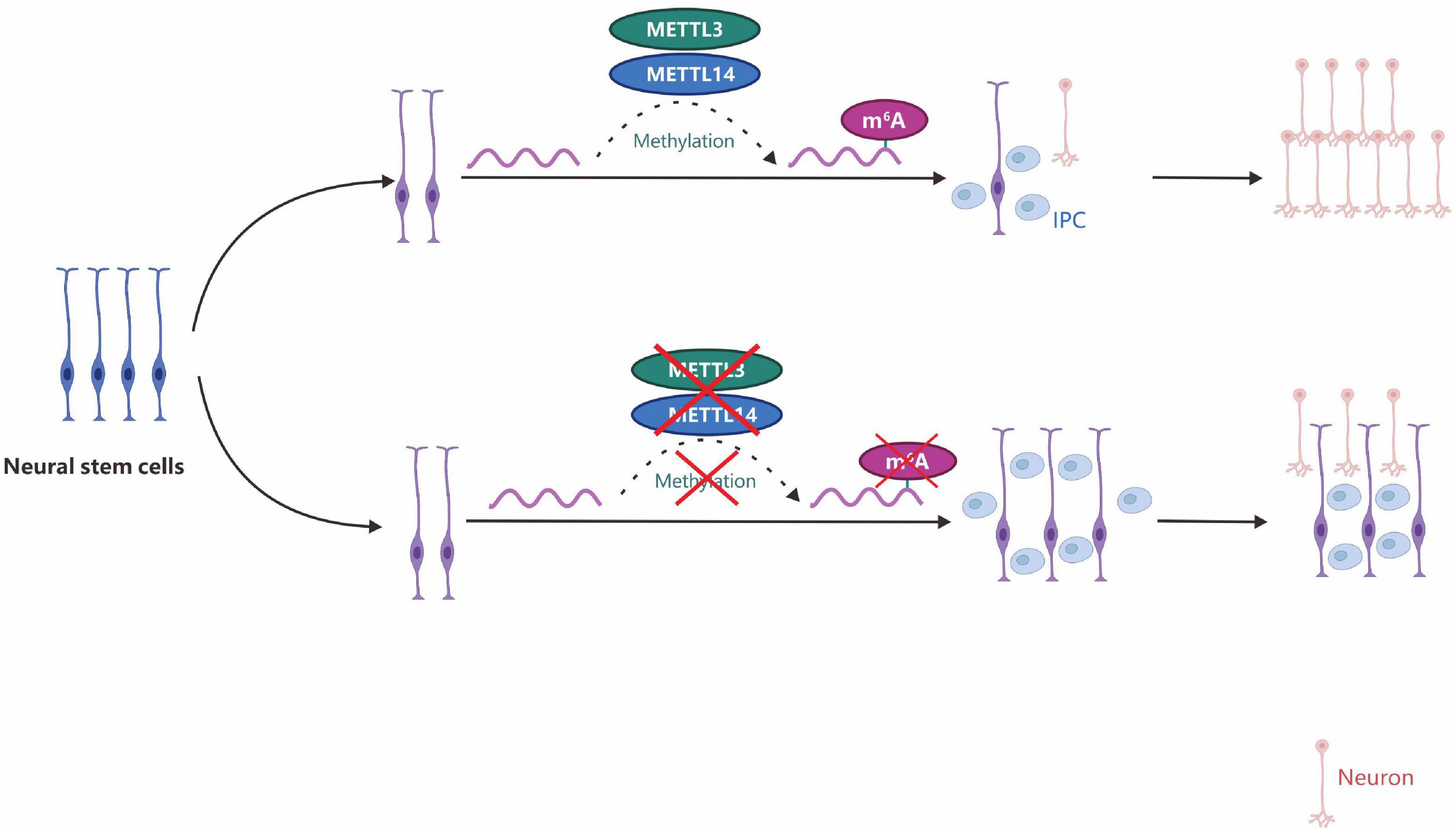
Figure 6. m6A depletion by METTL3/14 gene knockdown can promote the proliferation of neural stem cells and lead to prolonged cell cycle progression and maintenance of radial glial cells. IPC, intermediate progenitor cells.
The third component of the mammalian m6A methyltransferase complex is WTAP (Ping et al., 2014b). WTAP plays a key role in transcriptional and post-transcriptional regulation and is an oncogene whose expression is elevated in several types of human tumors. Through a yeast two-hybrid experiment, FIP37 (FKBP12 interacting protein 37, At3g54170, which encodes a human WTAP homologous gene) was found in Arabidopsis as a protein that interacts with methylthioadenosine (MTA) (Zhong et al., 2008). In HeLa cells, WTAP can co-precipitate with the METTL3-METTL14 heterodimer, and their combined effect is weaker than the interaction between METTL3 and METTL14 (Liu et al., 2013). WTAP lacks a catalytic region and has no methyltransferase activity, but the interaction between WTAP and METTL3-METTL14 can localize the methyltransferase complex in nuclear spots (Ping et al., 2014a), thereby regulating the binding of the methyltransferase complex to the target RNA, which ultimately affects the m6A level. Downregulating WTAP can suppress the localization of METTL3 in the nucleus and reduce m6A levels. The field has demonstrated that the consistency of the RNA substrates of the WTAP, METTL3, and METTL14 combination reached 36% using immunoprecipitation (PAR-CLIP) experiments (Liu et al., 2013). The conserved sequence of RNA binding is the same as the previously reported conserved sequence of m6A, and the three sites of RNA binding are mainly located in the intergenic and intron regions, indicating that m6A is generated from the precursor RNA. Silencing of the methyltransferase complex increases the expression of bound RNA, indicating that m6A is inversely related to gene expression (Batista et al., 2014; Schwartz et al., 2014a). Because WTAP is a splicing factor, knocking down WTAP or METTL3 can result in the formation of different isoforms of RNA containing m6A (Dominissini et al., 2012; Ping et al., 2014a), indicating that m6A affects alternative splicing of RNA.
Taken together, the discovery of METTL3, METTL14, and WTAP, which are important active center components in the m6A methyltransferase complex (Yao et al., 2020), has provided many new insights into m6A, laying an important foundation for revealing the biological function of m6A in RNA.
3.1.2 Other m6A methyltransferase complex components
3.1.2.1 KIAA1429
KIAA1429 is an important methyltransferase that participates in m6A modifications (Schwartz et al., 2014b). Based on the protein immunoprecipitation-mass spectrometry analysis of the core component of m6A methyltransferase, KIAA1429 may be a new subunit component of the m6A methyltransferase complex to catalyze the formation of m6A in mRNA (Schwartz et al., 2014). In Drosophila, the homologous gene of KIAA1429 interacts with the homologous gene of WTAP and regulates the pre-mRNA selective splicing of the important gene sex lethal (Sxl), which controls sex determination (Ortega et al., 2003). In human A549 cells, deletion of KIAA1429 can cause a sharp drop in the m6A peak, indicating that KIAA1429 plays an important role in the methyltransferase complex (Schwartz et al., 2014). Another study found that KIAA1429 can recruit METTL3/METTL14/WTAP, the catalytic core of the m6A methyltransferase complex, to achieve site-specific regulation of m6A levels in mRNA (Yue et al., 2018).
3.1.2.2 RNA binding motif protein 15(B) (RBM15/RBM15B)
RBM15/15B, a protein-coding gene, is a part of the WTAP-METTL3 m6A methyltransferase complex that interacts with WTAP to recruit the complex to target mRNAs. RBM15 and RBM15B can participate in catalyzing the formation of m6A on mRNA and non-coding RNA X-inactive specific transcripts (XIST). Further, immunoprecipitation experiments showed that RBM15 and RBM15B could bind and recruit the WTAP-METTL3 complex to specific target sites. RBM15/RBM15B consists of an RNA recognition domain, as per iCLIP (individual nucleotide resolution crosslinking and immunoprecipitation) sequencing and single miCLIP (m6A individual nucleotide resolution crosslinking and immunoprecipitation). Sequencing analysis of the base resolution revealed that the RBM15/RBM15B-binding site was significantly enriched near the m6A methylation modification site (Patil et al., 2016). In addition, the homologous protein Nito of RBM15 was also identified as a component of the methylase complex in Drosophila (Lence et al., 2016).
3.1.2.3 METTL16
METTL16, a homologous protein of METTL3, can mediate the formation of m6A in U6 snRNA. A 2017 study showed that METTL16 could also mediate the methylation of mRNA and regulate the intracellular levels of SAM. METTL16 contains methylation marks within the conserved UACm6AGAGAA sequence in the 3′UTR hairpins of the mRNA MAT2A and a special type of RNA structure (Figure 7; Pendleton et al., 2017).
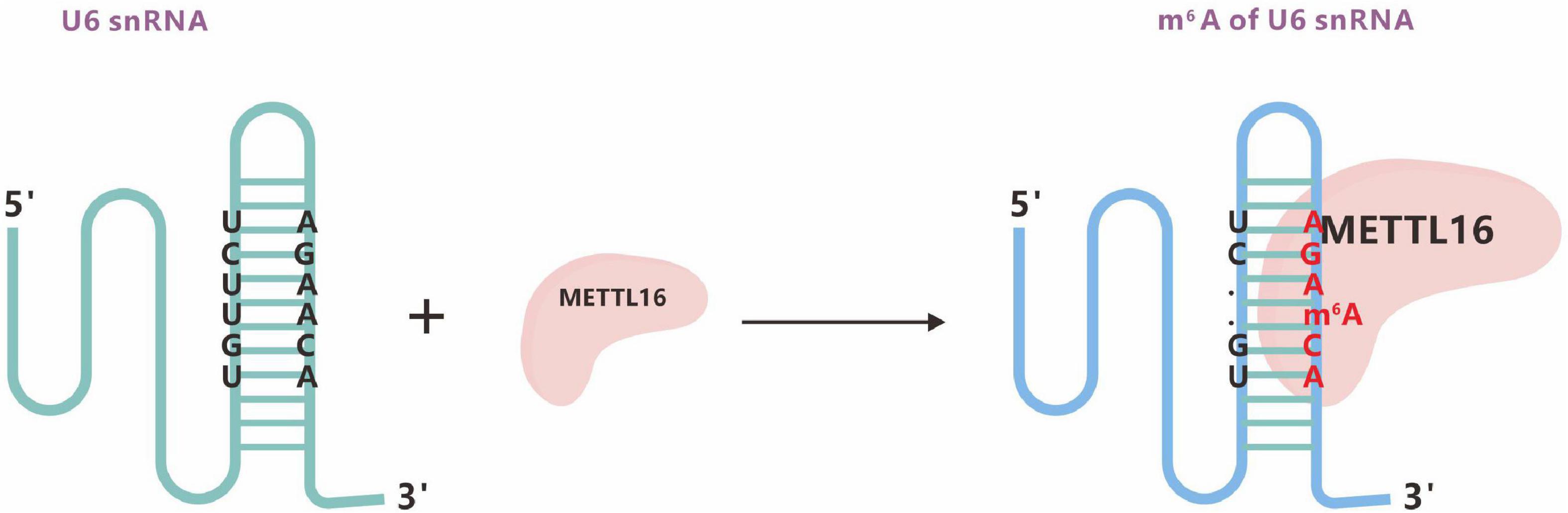
Figure 7. The m6A methyltransferase METTL16 binds U6 snRNA. m6A typically locates at a DRACH motif, where D denotes A, G, or U; R denotes A or G; and H denotes A, C, or U.
3.1.2.4 Zinc finger CCCH-type containing 13 (Zc3h13)
Zc3h13, a critical RNA m6A regulator, plays an important role in modulating RNA m6A methylation in the nucleus (Wen et al., 2018). In 2018, three studies almost simultaneously described Zc3h13 as a new component of the m6A methyltransferase complex that regulates m6A modification. Wen et al. (2018) found that mouse Zc3h13 can stabilize the nuclear localization of the Zc3h13-WTAP-Virilizer-Hakai complex and regulate the self-renewal of mouse embryonic stem cells (mESCs) by promoting m6A methylation (Figure 8). Knuckles et al. (2018) further clarified that mouse Zc3h13 and its Drosophila homologous gene CG7358 (named Flacc) mediate the interaction between RBM15/Nito and WTAP/Fl (2)d, thereby promoting m6A modification of Mrna. They also found that Flacc regulates sex determination and dosage compensation in Drosophila by regulating alternative splicing of the Sxl gene. Guo et al. (2018) identified the CG7358 gene of Drosophila (named Xio) and its involvement in the sex determination pathway of Drosophila through regulating the alternative splicing of Sxl via m6A modification.
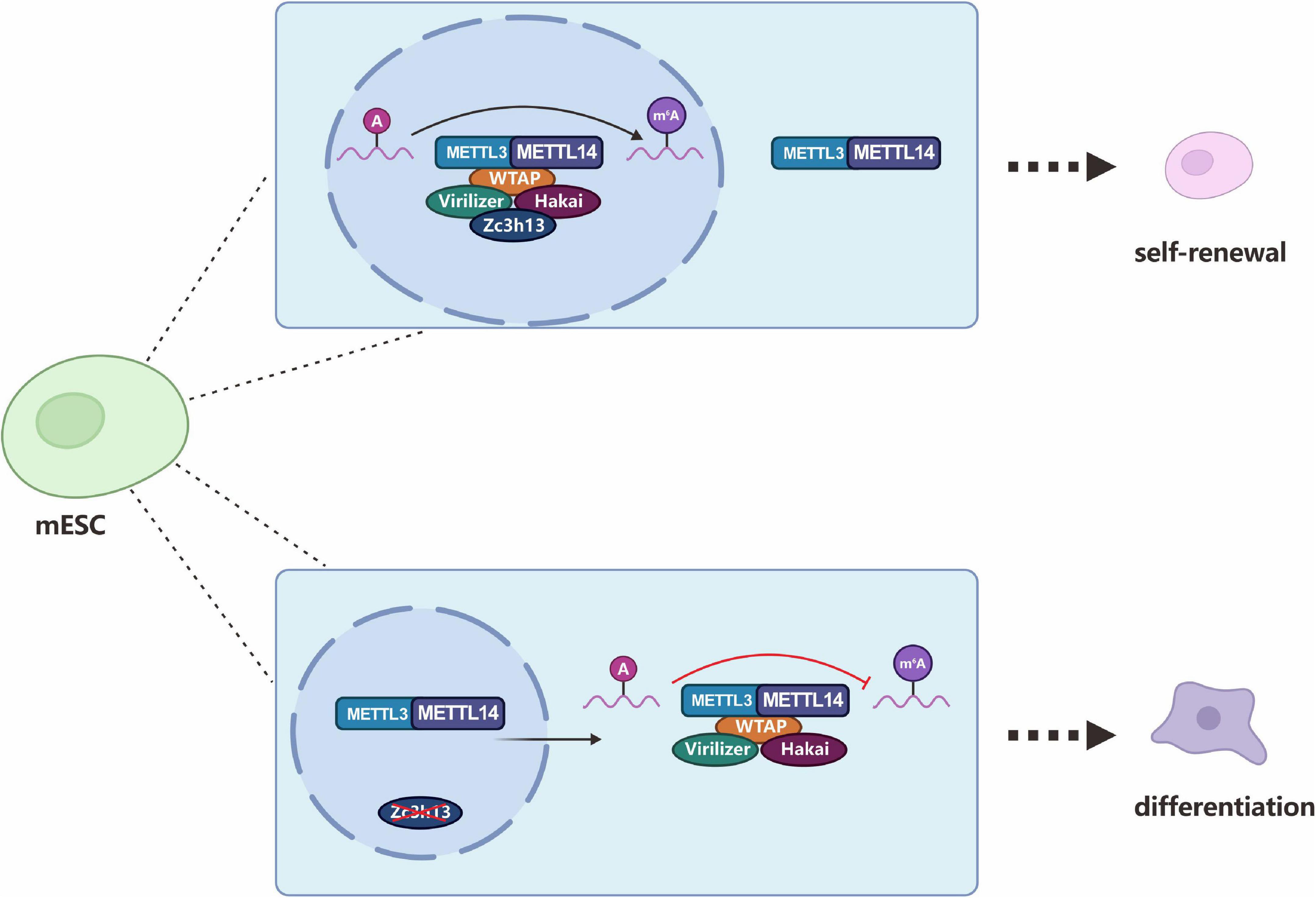
Figure 8. Zc3h13 modulates RNA m6A methylation in the nucleus. Zc3h13 anchors WTAP, Virilizer, and Hakai in the nucleus to facilitate m6A methylation and to regulate mESC self-renewal. Upon Zc3h13 knockdown, a great majority of WTAP, Virilizer, and Hakai translocate to the cytoplasm to inhibit m6A methylation.
In addition to the above-mentioned members, there are other methyltransferase components involved in the selective recognition of methylation sites and division of labor to ensure fine post-transcriptional regulation.
3.1.3 m5C methyltransferase: NSUN2 and DNMT2
The identified RNA m5C-specific methyltransferases include the NSUN (NOL1/NOP2/SUN) family proteins and DNMT2 (DNA methyltransferase 2), which belong to the superfamily of Rosman-folded enzymes and are characterized by the conserved cysteine residue SAM. The RNA m5C methyltransferase that has been studied the most recently is the NSUN family, which acts as a methyl donor to catalyze the transfer of methyl groups to cytosine residues of different RNA substrates (Bujnicki et al., 2004). Among the nine members of this family, many have catalytic and release sites for methyltransferases, particularly NSUN2 (also known as Trm4). NSUN2, encoded by the NSUN2 gene on chromosome 5p15.31-33, is a nucleolar RNA methyltransferase that catalyzes the m5C methylation of various RNAs such as mRNA, tRNA, and ncRNA (Figure 9). Ultrahigh-performance liquid chromatography-triple quadrupole mass spectrometry with multiple-reaction monitoring (UHPLC-QQQ-MRM-MS/MS) studies have found that NSUN2 is an mRNA-specific m5C methyltransferase, and its catalytic activity depends on the C271 (cysteine 271) and C321 (cysteine 321) sites, where C321 catalyzes the methylation of cytosine by binding to the cytosine pyrimidine ring to form a covalent bond, and C271 mediates the release of RNA. High-throughput sequencing analysis also showed that NSUN2 knockdown significantly reduced mRNA m5C modification (Yang et al., 2017b).
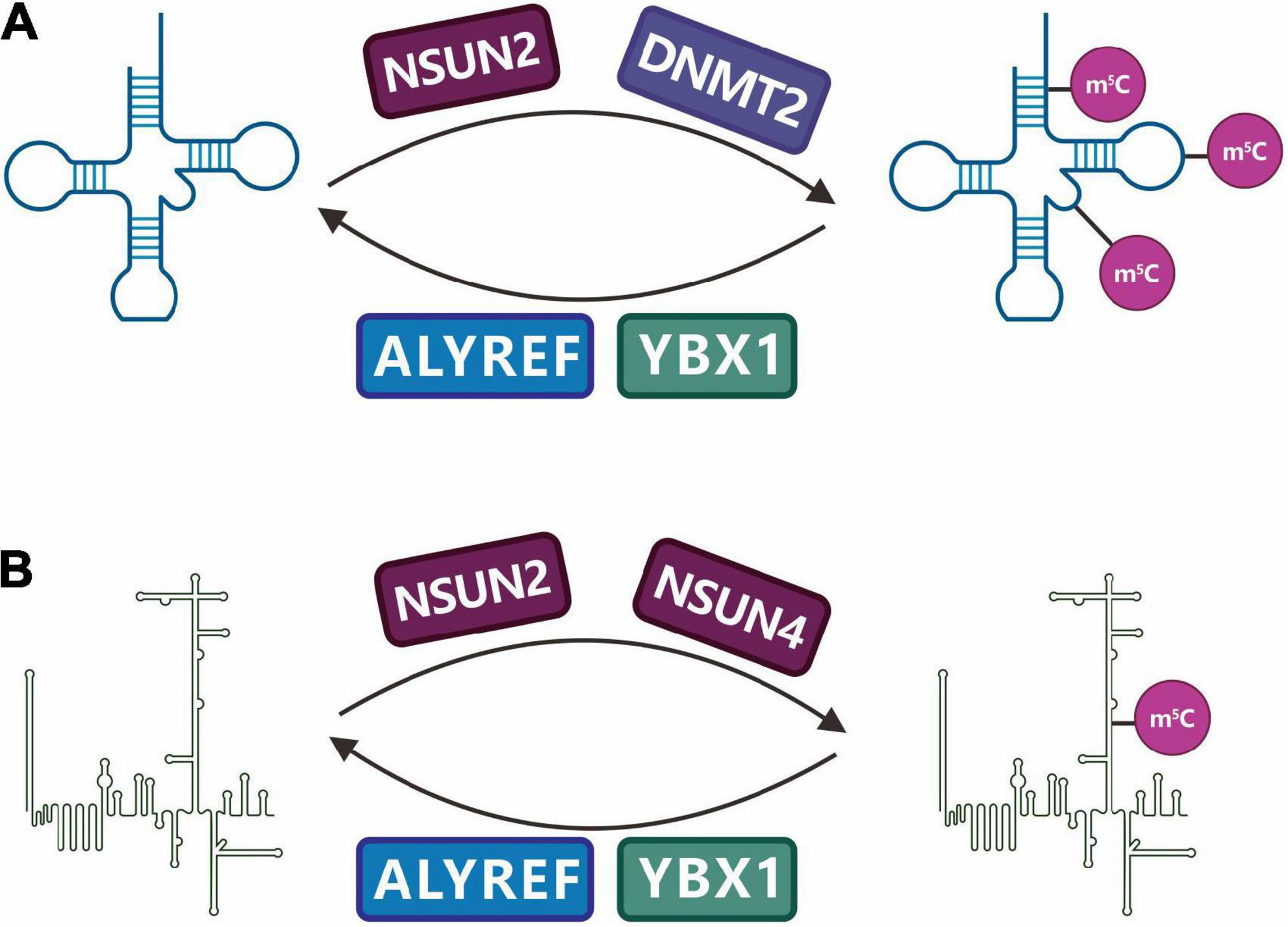
Figure 9. Regulation and function of RNA m5C methylation. C5-methylcytidine (m5C) is a common modification in (A) tRNAs and (B) other non-coding RNAs (ncRNAs). NSUN family enzymes (NSUN2, NSUN4) and DNMT2 have been identified as m5C writers, while ALYREF and YBX1 have been identified as m5C readers.
In addition to NSUN2, DNMT2 is an RNA m5C methyltransferase that plays a role in multiple species. It was initially considered a DNA methyltransferase, but the latest research shows that human DNMT2 cannot catalyze DNA methylation but can catalyze miRNA methylation and the changes in the C38 methyl group of tRNAAsp (aspartatetRNA) (Figure 9; Goll et al., 2006).
3.2 Demethylase-erasers
3.2.1 m6A demethylase: FTO and ALKBH5
During brain development, a dynamic change in m6A levels in RNA has been observed (Meyer et al., 2012), suggesting the existence of RNA m6A demethylases. Two novel mRNA m6A demethylases (FTO and ALKBH5) have recently been identified, confirming the dynamic regulation of m6A (Jia et al., 2011; Zheng et al., 2013).
The FTO gene is a major regulator of metabolism and energy utilization (Church et al., 2009, 2010; Fischer et al., 2009). It is a member of the Fe(II)- and oxoglutarate-dependent AlkB oxygenase family and was originally shown to catalyze the oxidative demethylation of methylated thymidine and uracil (Gerken et al., 2007; Jia et al., 2008). Overexpressing FTO in mice causes excessive energy intake and fattening; knocking out FTO makes mice thinner and grow slowly and causes teratogenic and lethal phenomena. In addition, FTO is closely related to diseases such as type 2 diabetes, cancer, and dementia (Boissel et al., 2009; Fu et al., 2013; Zhao et al., 2014). FTO can remove 3mT and m3U from single-stranded DNA and RNA (Jia et al., 2008), but its activity is very weak, and these nucleic acid modifications are rarely found in the body. It is generally believed that these are not the actual substrates for FTO. In 2011, m6A was reported to be an enzyme substrate for FTO (Jia et al., 2011). By simulating the demethylation reaction conditions in the physiological environment in vitro, the wild-type and mutant proteins of the obesity gene FTO were incubated with methylated substrates, and various methylation forms were analyzed using mass spectrometry and high-performance liquid phase technology. FTO had a demethylation function for m6A on single-stranded RNA, and the enzyme kinetic data indicated that the activity of FTO on m6A was much higher than that on m3U. In vivo experiments showed that the m6A content of mRNA in cells with knockdown of the FTO gene increased by approximately 23%; overexpression of FTO reduced the m6A content of mRNA by approximately 18% (Jia et al., 2011). Both in vivo and in vitro data indicate that the actual demethylase substrate of FTO is m6A on nuclear RNA. MeRIP-seq analysis of FTO-knockout brain tissue showed that the m6A level was almost unaffected, but the m6A level of certain specific mRNAs was significantly increased, indicating that FTO acts only on specific mRNA substrates (Hess et al., 2013). Epidemiological fluorescence experiments indicated that FTO is partially located in nuclear spots, suggesting that nuclear RNA is the main substrate of FTO (Jia et al., 2011).
Soon after FTO was discovered, a study reported a second m6A demethylase, ALKBH5, in mammals (Zheng et al., 2013). Integrating mass spectrometry, cell biology, genomics, bioinformatics, and model biology revealed the catalytic m6A demethylation activity of ALKBH5 (RNA demethylase). As the second identified m6A demethylase, ALKBH5 has m6A demethylase activity comparable to that of FTO and preferentially acts on the m6A site of conserved motifs. Unlike the oxidative demethylation of FTO, ALKBH5 can directly catalyze the removal of m6A without generating intermediate products (Zheng et al., 2013). In addition to FTO and ALKBH5, more m6A demethylases are yet to be discovered.
3.3 Binding proteins-readers
3.3.1 m6A readers
The discovery of m6A methyltransferase and demethylase in RNA means that m6A is a reversible chemical modification that can dynamically regulate physiological development processes. It is currently known that m6A-binding proteins in mammals are mainly of families containing YTH (YT521-B homology) domains, including YTHDF1, YTHDF2, YTHDF3, YTHDC1, YTHDC2, and heterogeneous nuclear ribonucleoproteins (HNRNP). Readers mainly work by directly or indirectly binding RNA. Direct RNA binding is mediated by a reader-specific recognition modification domain such as the YTH family proteins, including YTHDF1, YTHDF2, YTHDF3, YTHDC1, and YTHDC2, which can directly bind to methylation modifications of the target genes in the nucleus and cytoplasm (Figure 2). It can affect mRNA modifications, including mRNA splicing, nuclear export, translation, and mRNA degradation (Nachtergaele and He, 2017; Zhao et al., 2017). Indirect RNA binding relies on methylation-induced RNA unwinding, thereby exposing hidden protein-binding motifs and making proteins more likely to bind to RNA. HNRNP, known as heterogeneous nuclear ribonucleoproteins and indirect RNA-binding proteins, is a complex of proteins and RNA found in the nucleus of cells during gene transcription and subsequent post-transcriptional modification of newly synthesized RNA. It is involved in various cellular functions, including mRNA stability (Zhao et al., 2009), mRNA transport (Ma et al., 2018), miRNA maturation (Guil and Cáceres, 2007), and telomere biogenesis (Labranche et al., 1998).
3.3.1.1 YT521-B homology domain family members
YTH is a newly discovered domain that can bind to short, denatured single-stranded RNA (Zhang et al., 2010). The human genome contains at least five YTH domain-containing proteins: YTHDF1–3 and YTHDC1–2. Some YTH proteins contain a low-complexity region (LCR) that can form a phase separation of the protein (Ries et al., 2019), causing the cytoplasmic YTHDF-m6A-RNA polymer to become processing bodies (P-bodies). P-bodies are cytoplasmic ribonucleoprotein (RNP) granules, which are aggregates of multiple functional proteins and RNA in the cytoplasm (Wang et al., 2014). The binding capacity of the YTH domain to RNA containing conserved m6A sequences is approximately 1 μmol/L (Wang et al., 2014; Xu et al., 2014, 2015; Zhu et al., 2014).
In 2014, the m6A-binding protein YTHDF2 (YTH domain family, member 2) was first reported to mediate the degradation of m6A-modified mRNA (Wang et al., 2014). Photoactivatable ribonucleoside–enhanced crosslinking and PAR-CLIP sequencing results indicated that YTHDF2 mainly binds to mRNA and some long non-coding RNA, and the binding site is mainly in the 3′UTR rich in GAC sequences, which is very similar to the distribution characteristics of m6A. In general, YTHDF2 colocalizes with the deadenylation and decapsidase complex in the cell, which can direct the RNA substrate to the cytoplasmic subcellular organelle P-body that mediates RNA degradation (Wang et al., 2014). Subsequent studies further showed that YTHDF2 accelerated the degradation of m6A-modified transcripts by recruiting CCR4-NOT adenylate complexes (Du et al., 2016), primarily comprising subunits with deadenylase activity (CNOT6, CNOT6 L, CNOT7, or CNOT8) and regulatory NOT modules (CNOT1, CNOT2, CNOT3, CNOT9, CNOT10, and CNOT11).
Similar to YTHDF2, the second identified m6A-binding protein is YTHDF1 (YTH domain family, member 1), which is also a cytoplasmic protein that can promote the translation efficiency of the m6A-mRNA it binds. Ribosomal map analysis showed that YTHDF1 promotes the binding of substrate RNA to ribosomes, which is conducive to RNA translation (Wang et al., 2015). In addition, YTHDF1 promotes translation initiation, which, in turn, promotes protein synthesis. Downregulation of YTHDF1 will result in a reduction in substrate RNA translation efficiency, and the magnitude of the reduction is directly related to how much YTHDF1 is downregulated. The discovery of the YTHDF1 function indicates that m6A, as a dynamic RNA marker, can effectively regulate protein production.
YTHDC1, an m6A-binding protein in the nucleus, recognizes and binds m6A-containing RNAs and can mediate m6A-regulated mRNA splicing (Xiao et al., 2016). YTHDC1 is located at the YT body of the nucleus. In vitro electrophoretic mobility shift assay (EMSA) experiments showed significant binding of YTHDC1 to RNA containing m6A modifications, while in vivo photoactivatable ribonucleoside enhanced crosslinking and PAR-CLIP sequencing showed that the binding motif of YTHDC1 is consistent with the conserved motif RRACH of m6A. Most of the binding sites were located near the stop codon, which is also consistent with the distribution characteristics of m6A. YTHDC1 can interact with the splicing factor SR protein to regulate the alternative splicing of m6A-containing exons (Xiao et al., 2016). YTHDC1 also promotes XIST-mediated X-chromosome silencing by identifying the m6A modification site on the non-coding gene XIST (Patil et al., 2016). In HeLa cells, YTHDC1 interacts with SRSF3 and the RNA export factor 1 (NXF1) to promote the export of m6A-modified mRNA (Roundtree et al., 2017b).
YTHDF3 interacts with YTHDF1 and YTHDF2 to enhance the binding ability of YTHDF1 or YTHDF2 to RNA containing an m6A-modified substrate, thereby promoting RNA translation or degradation. YTHDF3 may also interact with other proteins to exert cell-specific regulatory functions (Li et al., 2017; Ni et al., 2019).
YTHDC2 enhances the translation efficiency of target mRNA by binding to the m6A-conserved motif (Wojtas et al., 2017). YTHDC2 also plays an important role in spermatogenesis by interacting with the meiosis-specific protein MEIOC to affect substrate stability (Abby et al., 2016; Soh et al., 2017). A study using infertile male and female mice with YTHDC2 knockout presented a defective phenotype during meiosis phase I (Tanabe et al., 2016). In addition to containing the YTH domain, YTHDC2 also contains an RNA-binding domain, a helicase domain, and two ankyrin repeats, which may play a role in the recruitment of RNA secondary structures, RNA binding proteins, and other interacting proteins (Abby et al., 2016; Tanabe et al., 2016). This also suggests that YTHDC2 may play multiple roles in various biological processes.
3.3.1.2 HNRNP family members
The HNRNP protein family is mainly distributed in the nucleus and is an RNA-binding protein that participates in processes such as precursor RNA splicing, transportation, and translation (Han et al., 2010). HNRNPA2B1, a member of the HNRNP family, can bind m6A-modified RNA transcripts both in vivo and in vitro. However, it remains controversial whether HNRNPA2B1 is an m6A-binding protein. Alarcón et al. (2015) found that HNRNPA2B1 can directly bind to m6A modification sites and regulate alternative splicing and pri miRNA processing, while Wu et al. (2018) found that HNRNPA2B1 does not directly bind m6A but acts as a converter based on structural studies. In addition, two other HNRNP family proteins, HNRNPC and HNRNPG, can regulate the processing of RNA transcripts containing m6A modifications. Unlike HNRNPA2B1, HNRNPC and HNRNPG do not directly bind to the m6A site but mediate the alternative splicing process of transcripts containing m6A by recognizing and binding m6A-dependent structural switches (Liu et al., 2015, 2017).
Additionally, studies based on RNA pull-down experiments have detected other potential m6A binding proteins, including ELAV-like RNA binding protein 1 (ELAVL1, also called HuR), FMRP translational regulator 1 (FMR1), leucine-rich pentatricopeptide repeat containing (LRPPRC), and insulin-like growth factor 2 mRNA-binding proteins (IGF2BPs).
3.3.2 m5C-binding proteins: ALYREF
To identify the binding protein of m5C, two oligonucleotide substrates, with and without m5C, were designed. Through oligonucleotide enrichment combined with protein profiling, the mRNA was identified as a nuclear functional complex component. ALYREF binds to m5C-modified RNA oligonucleotides. EMSA and RIP combined with HPLC and sequencing technology demonstrated that ALYREF’s K171 (lysine 171) mutation can significantly reduce its ability to bind m5C, thereby reducing its ability to bind RNA. Further, fluorescence in situ hybridization technology (FISH) showed that the efficiency of mRNA nucleation decreased with the knockdown of NSUN2 and ALYREF. However, backfilling experiments demonstrated that only the wild-type NSUN2 and ALYREF could reverse the reduction in mRNA nucleation caused by the knockdown of NSUN2 and ALYREF, suggesting that m5C plays an important regulatory role in the process of mRNA nucleation (Shi et al., 2017; Yang et al., 2017a; Fan et al., 2019).
4 Biological processing of RNA methylation
4.1 m6A and neural stem cells
m6A is distributed in distinct developmental stages in the brain and controls the self-renewal of neural stem cells (NSCs) and their differentiation into neurons (Yoon et al., 2017; Du et al., 2019; Zhu et al., 2020). In METTL3/METTL14 gene knockout mice, m6A can promote the proliferation of NSCs and prolong the cell cycle of radial glial cells (Figure 6; Yoon et al., 2017; Wang et al., 2018b). Such regulation may promote the development of stem cell and gene-targeted therapies for Alzheimer’s, Parkinson’s, and cognitive-related neurological diseases.
4.2 m6A and synaptic functions
m6A modifications also contribute to the local regulation of synaptic functions (Flamand and Meyer, 2019). Experimental studies have shown that FTO protein in neurons can shift between the nucleus, cell body, and dendrites (including synapses) and can lead to changes in local RNA methylation kinetics (Meyer et al., 2012; Chang et al., 2017). Methylated transcripts are highly biased toward neuronal genes and functions such as synaptic functions. The m6A transcriptome is spatially regulated in different brain regions. At the single neuron level, m6A-modified RNA and its interaction groups diffuse to specific structures such as axons, dendrites, pre-synaptic nerve endings, and dendritic spines. This spatial distribution supports the m6A’s functional library to control synapses, touch transmission, and plasticity. Transcriptomic analysis of m6A in a mammalian brain revealed a specific bias of m6A toward neuronal genes rather than glial cell genes. The functional classification of m6A-seq analysis in the entire mouse brain, midbrain, cortex, and cerebellum has been identified. Most m6A-target genes are involved in nervous system development, synaptic transmission, and post-synaptic function. Other studies have reported the localization of m6A in axons and its role in axon growth. Transcripts encoding the axon elongation factor growth-associated protein-43 (GAP-43), a specific marker for axonal regeneration, were identified as targets for m6A modifications. The local translation of these transcripts is negatively regulated by m6A and can be regulated by FTO in axons (Geuens et al., 2016; Yu et al., 2018), showing that RNA methylation also plays an important role in the development and function of synapses.
4.3 m6A regulates nervous system development
Among the various tissues and organs, the brain has the most abundant expression of m6A and its recognition proteins. m6A-related regulatory proteins perform important functions in the cerebral cortex, such as synaptic function, axon regeneration, neural stem cell self-renewal, and cerebellar development (Yoon et al., 2017). The m6A modification plays an irreplaceable regulatory role in the development and function of the nervous system. Drosophila with a knockout of a yeast m6A methyltransferase encoded by the Ime4 gene can be born and survive until adulthood; however, the life span of the fruit fly is shortened and shows obvious abnormal behavior, suggesting that the deletion of m6A modification affects the function of the fruit fly nervous system (Lence et al., 2016). A specific knockout of METTL14 in the central nervous system of mice can affect the development of the mouse cerebral cortex (Yoon et al., 2017). Moreover, the deletion of the YTHDF2 gene in mice leads to an increase in the overall level of m6A, preventing the differentiation of neural stem cells and the formation of neuronal axis dendrites from entering the RNA degradation pathway, resulting in the asymmetric division of neural stem cells in the cerebral cortex (Li et al., 2018b). When neural precursor cells are missing in mice, the differentiation of neurons is affected, resulting in the slower development of the forebrain cortex.
Except for the cerebral cortex, cerebellar RNA m6A patterns and levels are particularly prominent. The dynamic process of m6A methylation and demethylation occurs throughout the entire developmental process of the cerebellum after birth, and the lack of ALKBH5 in a low-pressure and low-oxygen environment causes the m6A level of genes involved in the regulation of cerebellar development to be disordered, speeding up the process of RNA export and leading to a marked lag in cerebellar development (Ma et al., 2018). The specific knockout of METTL3 in the CNS causes severe motor dysfunction in mice during lactation and leads to death. Anatomical and pathological examination revealed that the deletion of m6A methylation caused by METTL3 knockout severely affected the development of the cerebral cortex and cerebellum, resulting in thinning of the cerebral cortex and dysplasia of the cerebellum (Wang et al., 2018a). The absence of m6A also causes disordered gene expression regulation during the differentiation and maturation of granule neurons in the cerebellum, resulting in severe apoptosis of newborn granule neurons, revealing that METTL3-mediated m6A modification plays an important role in the development of the mammalian central nervous system (Wang et al., 2018a).
4.4 m5C is involved in brain development
m5C-modified methyltransferase NOP2/Sun RNA methyltransferase 2 (NSUN2), a protein-coding gene, also regulates neuronal development. In a developing mouse brain, the absence of NSUN2 did not affect RG cells but delayed their differentiation into superior neurons. NSUN2 is expressed in early neuroectodermal cells and can differentiate into various region-specific neuronal and glial cell types (Li et al., 2005). Mutations or deletions in the m5C methylase genes NSUN2 and NSUN3 can cause defects in the nervous system. NSUN2 mutations are associated with autosomal recessive mental retardation (Khan et al., 2012). In the mouse brain, NSUN2 is localized in the nucleolus of Purkinje cells in the cerebellum. The mutated NSUN2 cannot function normally because it cannot converge in the nucleolus. Further research revealed that the methylation level of tRNA in the brains of NSUN2-deficient mice decreased, the cerebral cortex thickened, the number of intermediate progenitors increased, and the number of upper-layer neurons decreased. In this process, the loss of NSUN2 may result in a reduction in the size of the hindbrain due to the failure to produce sufficient numbers of differentiated neurons, while the migration ability of human neuroepithelial stem cells with NSUN2 loss is significantly reduced, which also suggests that NSUN2-dependent tRNA methylation is essential for brain development. The differentiation and migration of neural progenitor cells thus play an important role (Flores et al., 2017). In addition, the inactivation of NSUN3 in mouse embryonic stem cells can further lead to impaired differentiation of the neuroectodermal lineage (Trixl et al., 2018). The summary of RNA modifications is presented in Table 1.
5 Methods to detect RNA modifications
5.1 Wet-lab approaches applied for profiling RNA epitranscriptome
Targeted approaches for detecting RNA modifications have been well established. These include roughly four types (Vandivier and Gregory, 2017): (1) direct sequencing through nucleotide labeling and chromatography, (2) mass spectrometry, (3) detecting the stalling and termination of reverse transcriptase (RT), and (4) direct measurement of changes in base pairing. We have summarized these targeted technologies for detecting specific modifications (Li et al., 2016b; Helm and Motorin, 2017; Lv et al., 2021). Recently, the progress in combining existing biochemical techniques and high-throughput sequencing has been rapid in covalent RNA modification studies. These technologies include: (1) high-throughput sequencing + antibody pull-down and (2) high-throughput sequencing + chemical conversion and chemical adduct coupling methods (Vandivier and Gregory, 2017). (3) Single-molecule real time (SMRT) technology using nanowells and nanopore sequencing (Furlan et al., 2021). Recently developed in silico methods that detect RT errors in high-throughput RNA sequencing data and high-throughput single molecule sequencing data may read transcriptome and extratranscriptome information simultaneously (Helm and Motorin, 2017; Vandivier and Gregory, 2017). These methods are summarized in Table 2.
These high-throughput mapping methods have enabled the characterization of the epitranscriptome in diverse cellular environments. However, further technical improvements are needed to improve the resolution and sensitivity of these methods. Following the recent discovery of more writers, readers, and erasers of the epitranscriptome, as well as the detailed analysis of the known epitranscriptome, new research directions will emerge, which may lead to new treatment strategies. In the future, improved high-throughput technology should help improve spatial resolution, provide chemometric data, and possibly detect new mRNA modifications in the transcriptome.
5.2 Applied computational approaches for profiling RNA epitranscriptome
Although wet-lab approaches can obtain relatively accurate mRNA modification information, they are time-consuming, costly, and difficult to conduct. Given that RNA sequence numbers show explosive growth in the post-genomic era, wet-lab approaches are obviously not suitable for systematic and in-depth analysis of the relevant mechanisms and functions of RNA methylation modification. Therefore, many researchers have developed predictive and computational tools for identifying epigenetic modifications (Chen et al., 2020c; Guo et al., 2021), which have evolved rapidly in recent years (Rehman et al., 2021b,2022b; Liao et al., 2022). These tools are mainly based on machine learning (ML) or deep learning (DL) algorithms (Abbas et al., 2022). The information for most detection tools and databases has been described in recent reviews published by Chen et al. (2019b), El Allali et al. (2021) and Wang et al. (2022b). We have integrated the information from those studies with the most recent advances; this information is listed in Table 3. Furthermore, we categorized emerging tools into two types that identify single modification and multiple RNA methylation sites, respectively, based on the year that the tool developed (Table 3).
5.2.1 Prediction tools for identifying m6A sites
Dao et al. (2020) established the iRNA-m6A tool based on the Support Vector Machine (SVM) with fivefold cross-validation test. It can accurate identify m6A sites with the data from high-throughput sequencing techniques in multiple human, mouse, and rat tissues. Liu et al. (2020c) further developed the im6A-TS-CNN tool and improved the results of iRNA-m6A using a convolutional neural network (CNN). Most recently, deep neural network (DNN)-based m6A site computation models, such as TS-m6A-DL, iMethyl-Deep, DNN-M6A, EDLm6APred, and DL-M6A, have been developed. These tools can achieve the identification of m6A methylation sites across species, and are specific to tissue and even RNA types (Mahmoudi et al., 2020; Abbas et al., 2021; Zhang et al., 2021a,b; Rehman et al., 2022a). Kortel et al. (2021) developed m6Aboost by combining ML with miCLIP (m6A individual-nucleotide resolution UV crosslinking and immunoprecipitation) to significantly improve the detection of m6A sites. miCLIP is a kind of antibody-based approach for m6A site mapping with single-nucleotide resolution. Zhang et al. (2021c) developed a novel predictor named M6A-GSMS based on the GBDT (Gradient Boosting Decision Tree) and stacking learning to identify m6A sites (Zhang et al., 2021c). At the same time, Wang et al. (2021) proposed the m6AGE predictor that combines sequence-derived features and graph embeddings for m6A site prediction. Recently, Rehman et al. (2021a) has made use of artificial intelligence to produce an effective model, the m6A-NeuralTool, which can be utilized for speedy and efficient identification of N6-methyladenosine sites (Rehman et al., 2021a). m6A TSHub is a comprehensive online platform established by Song et al. (2022a), which provides a web server constructed by multi-instance deep neural networks with gated attention for high-accuracy prediction of m6A methylation sites, named as m6A-TSFinder (Song et al., 2022a).
5.2.2 Prediction tools for identifying m5C sites
Lv et al. (2020a) established a typical predictor named as iRNA-m5C based on the best features and random forest algorithm, to identify m5C sites in Homo sapiens, Mus musculus, Saccharomyces cerevisiae, and Arabidopsis thaliana (Lv et al., 2020a). Li et al. (2022b) developed a model to identify m5C based on a deep fusion approach with an improved residual network via 10-fold cross-validation and independent set testing, which shows a considerable improvement compared to previous tools (Li et al., 2022b).
5.2.3 Prediction tools for identifying m1A sites
Yao et al. (2022) built the framework m1ARegpred (m1A regulators substrate prediction), m1ARegpred was achieved based on ML and the combination of sequence-derived and genome-derived features. Suleman and Khan (2022) developed an extreme gradient boost predictor named as m1A-Pred for the prediction of modified m1A sites.
5.2.4 Prediction tools for identifying m6Am sites
Jiang et al. (2022) presented the m6AmPred, the first web server, for in silico identification of m6Am sites from the primary sequences of RNA. m6AmPred was built upon the XgbDart (eXtreme Gradient Boosting with Dart algorithm) and EIIP-PseEIIP encoding scheme. Luo et al. (2022) proposed an ensemble DL framework, named as DLm6Am, to identify m6Am sites. DLm6Am consists of three similar base classifiers, each of which contains a multi-head attention module, an embedding module with two parallel DL sub-modules, a CNN and a BiLSTM (Bidirectional long short-term memory), and a prediction module. Compared with the existing state-of-the-art m6Am prediction tool, m6AmPred and MultiRM show superior performance (Luo et al., 2022).
5.2.5 Prediction tools for identifying hm5C sites
Zhang and Shi (2021) designed a novel and powerful model called iR5hmcSC for identifying hm5C. iR5hmcSC can achieve high-throughput identification of hm5C (Zhang and Shi, 2021). Shi et al. (2022) designed a model called R5hmCFDV. R5hmCFDV showed higher accuracy than iR5hmcSC does in the 10-fold cross-validation.
5.2.6 Prediction tools for identifying Ψ sites
Zhuang et al. (2021) built PseUdeep, an RNA Pseudouridine Site Identification framework with DL Algorithm. PseUdeep outperformed the best traditional ML model available, which was evaluated through 10-fold cross-validation and two independent testing data sets (Zhuang et al., 2021).
5.2.7 Prediction tools for multi-modification sites
Wang et al. (2022a) combined convolutional CNN and BiLSTM, and developed an ensemble multiscale DL predictor, EMDLP. It can identify RNA methylation sites by NLP (natural language processing) and DL way, and also take better advantage of the local and global information for site prediction. Abbas et al. (2022) proposed a unified DL model named as ZayyuNet, which can efficiently receive large inputs and achieve better performance based on its SpinalNet architecture that was inspired by the human somatosensory system.
5.3 Benchmark datasets related to RNA methylation
It is important to understand the methylation-related databases involved in developing these tools. We summarized the database information involved in methylation modification (Table 4) and sorted it into either single site or multiple site modification based on the year that the database was established in. Databases related to methylation modification are under development from comprehensive databases to more detailed and specialized ones. The following are the emerged databases of methylation modifications associated with some specific diseases and fields: (1) After m6A methylation-related genes based on The Cancer Genome Atlas (TCGA) were used to predict the prognosis of hepatocellular carcinoma (Group et al., 2020; Liu et al., 2020b; Li et al., 2021b), cancer-related methylation modification databases have begun to emerge, including, Lnc2Cancer 3.0 and OncoDB (Gao et al., 2021; Tang et al., 2022); (2) Osteoarthritis-omics and molecular biomarkers (OAOB), which are a group of database containing differential molecular biomarkers related to osteoarthritis (Li et al., 2022a); (3) Other than disease, Pan et al. (2022) established an integrative multi-omic database (iMOMdb) of Asian pregnant women providing the first blood-based multi-omic analysis of pregnant women in Asia. This database contains high-resolution genotypes, DNA methylation, and transcriptome profiles, and fills the knowledge gap of complex traits in populations of Asian ancestry; (4) Gao et al. (2022) developed AgingBank, an experimentally supported multiomics database of information related to aging in multiple species; (5) ProMetheusDB, a database generated by analyzing and sorting cell culture experiments data using ML tools from the protein perspective (Massignani et al., 2022); (6) compendium of protein lysine modifications (CPLM 4.0), a post-translational modification (PTMs) database (Zhang et al., 2022); (7) tRNA-related databases containing high-throughput tRNA sequencing data (Sajek et al., 2020); (8) RNAWRE and RM2 Target are two databases focusing on information of writers, readers, and erasers (Nie et al., 2020; Bao et al., 2022); and (9) SyStemCell, a multiple-levels experimental database for stem cell research (Yu et al., 2012). With the emergence of these specialized and multi-angle RNA methylation related databases, the traditional databases are also constantly updated and developed. Song et al. (2022a) established a comprehensive online platform, m6A-TSHub, to reveal context-specific m6A methylation and gene mutations that may regulate m6A epigenetic markers. Ma et al. (2022) proposed the M5C Atlas, a database for the comprehensive collection and annotation of RNA5 methylcytosine. Zhou et al. (2022) built ASMdb, a DNA modification database containing RNA sequencing data. Nevertheless, at present, specific databases for methylation modification such as m1A, m6Am, hm5C, and Ψ are still relatively lacking, which requires further improvement and development by researchers.
6 Conclusion and outlook
To provide biochemical researchers with the latest progress in RNA methylation, we reviewed the findings to date on the dynamic regulation and key roles of RNA methylation. We found that combining biochemical technology with high-throughput sequencing has made rapid progress in understanding the form and function of RNA modification, especially in mRNA and lncRNA. We mainly discussed m6A, m5C, m1A, pseudouridine, and 2’OMe. Other types of modifications that can be detected but are not clearly defined were not included. We also discussed the advantages and disadvantages of the detecting method based on high-throughput sequencing. It is worth mentioning that the databases related to RNA methylation modification, as well as the prediction and identification tools for RNA methylation sites developed with the help of these databases, have played an increasingly important role in RNA methylation modification research. We found that establishing a powerful method to investigate transcriptome modification is key to understanding post-transcriptional regulation. We emphasized that RNA methylation modification plays a key role in neural stem cells, synaptic functions, nervous system development, and brain development. Future research should focus on the role and mechanism of RNA methylation in neurodevelopmental disorders, which will greatly contribute to the prevention and treatment of developmental diseases.
Author contributions
JZ and HL were responsible for gathering information and drawing the illustrations. YZ contributed to information interpretation, editing, and critical revision of the manuscript. WT, Y-QC, JD, S-YB, and Z-XW were responsible for revision of the manuscript. All authors read and approved the final manuscript.
Funding
This study was financially supported by the Ministry of Science and Technology of China (2020YFC2006000) and the National Natural Science Foundation of China (81870901 and 82071272). The funders had no role in the design, analysis, or writing of this manuscript.
Conflict of interest
The authors declare that the research was conducted in the absence of any commercial or financial relationships that could be construed as a potential conflict of interest.
Publisher’s note
All claims expressed in this article are solely those of the authors and do not necessarily represent those of their affiliated organizations, or those of the publisher, the editors and the reviewers. Any product that may be evaluated in this article, or claim that may be made by its manufacturer, is not guaranteed or endorsed by the publisher.
References
Abbas, Z., Tayara, H., and Chong, K. T. (2022). ZayyuNet - A unified deep learning model for the identification of epigenetic modifications using raw genomic sequences. IEEE ACM Trans. Comput. Biol. Bioinform. 19, 2533–2544. doi: 10.1109/Tcbb.2021.3083789
Abbas, Z., Tayara, H., Zou, Q., and Chong, K. T. (2021). Ts-m6A-Dl: Tissue-specific identification of N6-methyladenosine sites using a universal deep learning model. Comput. Struct. Biotechnol. J. 19, 4619–4625. doi: 10.1016/j.csbj.2021.08.014
Abby, E., Tourpin, S., Ribeiro, J., Daniel, K., Messiaen, S., Moison, D., et al. (2016). Implementation of meiosis prophase I programme requires a conserved retinoid-independent stabilizer of meiotic transcripts. Nat. Commun. 7:10324. doi: 10.1038/ncomms10324
Ahmed, S., Hossain, Z., Uddin, M., Taherzadeh, G., Sharma, A., Shatabda, S., et al. (2020). Accurate prediction of Rna 5-hydroxymethylcytosine modification by utilizing novel position-specific gapped k-mer descriptors. Comput. Struct. Biotechnol. J. 18, 3528–3538. doi: 10.1016/j.csbj.2020.10.032
Akbar, S., and Hayat, M. (2018). iMethyl-Sttnc: Identification of N(6)-methyladenosine sites by extending the idea of Saac into Chou’s Pseaac to formulate Rna sequences. J. Theory Biol. 455, 205–211. doi: 10.1016/j.jtbi.2018.07.018
Alam, W., Ali, S. D., Tayara, H., and Chong, K. T. (2020). A Cnn-Based Rna N6-methyladenosine site predictor for multiple species using heterogeneous features representation. IEEE Access. 8, 138203–138209. doi: 10.1109/access.2020.3002995
Alarcón, C. R., Goodarzi, H., Lee, H., Liu, X., Tavazoie, S., and Tavazoie, S. F. (2015). Hnrnpa2B1 Is a Mediator of m(6)A-Dependent nuclear rna processing events. Cell 162, 1299–1308. doi: 10.1016/j.cell.2015.08.011
Ali, S. D., Kim, J. H., Tayara, H., and Chong, K. T. (2021). Prediction of Rna 5-Hydroxymethylcytosine modifications using deep learning. IEEE Access. 9, 8491–8496. doi: 10.1109/access.2021.3049146
Aziz, A. Z. B., and Hasan, M. A. M. (2020). “A mixed convolution neural network for identifying rna pseudouridine sites,” in Ieee Region 10 Symposium (Tensymp), (Dhaka: Ieee), 799–802.
Aziz, A. Z. B., Hasan, M. A. M., and Shin, J. (2021). Identification of Rna pseudouridine sites using deep learning approaches. PLoS One 16:e0247511. doi: 10.1371/journal.pone.0247511
Bao, X., Zhang, Y., Li, H., Teng, Y., Ma, L., Chen, Z., et al. (2022). Rm2Target: A comprehensive database for targets of writers, erasers and readers of Rna modifications. Nucleic Acids Res. doi: 10.1093/nar/gkac945
Barbieri, I., and Kouzarides, T. (2020). Role of Rna modifications in cancer. Nat. Rev. Cancer 20, 303–322. doi: 10.1038/s41568-020-0253-2
Barrett, T., Wilhite, S. E., Ledoux, P., Evangelista, C., Kim, I. F., Tomashevsky, M., et al. (2013). Ncbi Geo: Archive for functional genomics data sets–update. Nucleic Acids Res. 41, D991–D995. doi: 10.1093/nar/gks1193
Batista, P. J., Molinie, B., Wang, J., Qu, K., Zhang, J., Li, L., et al. (2014). m(6)A Rna modification controls cell fate transition in mammalian embryonic stem cells. Cell Stem Cell 15, 707–719. doi: 10.1016/j.stem.2014.09.019
Bi, Y., Jin, D., and Jia, C. (2020). EnsemPseU: Identifying pseudouridine sites with an ensemble approach. IEEE Access. 8, 79376–79382. doi: 10.1109/access.2020.2989469
Boissel, S., Reish, O., Proulx, K., Kawagoe-Takaki, H., Sedgwick, B., Yeo, G. S., et al. (2009). Loss-of-function mutation in the dioxygenase-encoding Fto gene causes severe growth retardation and multiple malformations. Am. J. Hum. Genet. 85, 106–111. doi: 10.1016/j.ajhg.2009.06.002
Brummer, A., Yang, Y., Chan, T. W., and Xiao, X. (2017). Structure-mediated modulation of mrna abundance by A-to-I editing. Nat. Commun. 8:1255. doi: 10.1038/s41467-017-01459-7
Bujnicki, J. M., Feder, M., Ayres, C. L., and Redman, K. L. (2004). Sequence-structure-function studies of trna:m5C methyltransferase Trm4p and its relationship to Dna:m5C and Rna:m5U methyltransferases. Nucleic Acids Res. 32, 2453–2463. doi: 10.1093/nar/gkh564
Bujnicki, J., Feder, M., Radlinska, M., and Blumenthal, R. (2002). Structure prediction and phylogenetic analysis of a functionally diverse family of proteins homologous to the Mt-A70 subunit of the human mrna: m6A methyltran sferase. J. Mol. Evol. 55, 431–444. doi: 10.1007/s00239-002-2339-8
Cantara, W. A., Crain, P. F., Rozenski, J., Mccloskey, J. A., Harris, K. A., Zhang, X., et al. (2011). The Rna Modification Database. Rnamdb: 2011 update. Nucleic Acids Res. 39, D195–D201. doi: 10.1093/nar/gkq1028
Chai, D., Jia, C., Zheng, J., Zou, Q., and Li, F. (2021). Staem5: A novel computational approachfor accurate prediction of m5C site. Mol. Ther. Nucleic Acids 26, 1027–1034. doi: 10.1016/j.omtn.2021.10.012
Chang, M., Lv, H., Zhang, W., Ma, C., He, X., Zhao, S., et al. (2017). Region-specific Rna m(6)A methylation represents a new layer of control in the gene regulatory network in the mouse brain. Open Biol. 7:170166. doi: 10.1098/rsob.170166
Chen, H., Gu, L., Orellana, E. A., Wang, Y., Guo, J., Liu, Q., et al. (2020a). Mettl4 is an snrna m(6)Am methyltransferase that regulates Rna splicing. Cell Res. 30, 544–547. doi: 10.1038/s41422-019-0270-4
Chen, K., Wei, Z., Zhang, Q., Wu, X., Rong, R., Lu, Z., et al. (2019a). Whistle: A high-accuracy map of the human N6-methyladenosine (m6A) epitranscriptome predicted using a machine learning approach. Nucleic Acids Res. 47:e41. doi: 10.1093/nar/gkz074
Chen, W., Ding, H., Zhou, X., Lin, H., and Chou, K. C. (2018a). irna(m6A)-Psednc: Identifying N(6)-methyladenosine sites using pseudo dinucleotide composition. Anal. Biochem. 561-562, 59–65. doi: 10.1016/j.ab.2018.09.002
Chen, W., Feng, P., Ding, H., and Lin, H. (2016a). Identifying N (6)-methyladenosine sites in the Arabidopsis thaliana transcriptome. Mol. Genet. Genomics 291, 2225–2229. doi: 10.1007/s00438-016-1243-7
Chen, W., Feng, P., Ding, H., Lin, H., and Chou, K. C. (2015a). irna-Methyl: Identifying N(6)-methyladenosine sites using pseudo nucleotide composition. Anal. Biochem. 490, 26–33. doi: 10.1016/j.ab.2015.08.021
Chen, W., Feng, P., Tang, H., Ding, H., and Lin, H. (2016b). Rampred: Identifying the N(1)-methyladenosine sites in eukaryotic transcriptomes. Sci. Rep. 6:31080. doi: 10.1038/srep31080
Chen, W., Feng, P., Yang, H., Ding, H., Lin, H., and Chou, K. C. (2018b). irna-3typeA: Identifying Three types of modification at Rna’s adenosine sites. Mol. Ther. Nucleic Acids 11, 468–474. doi: 10.1016/j.omtn.2018.03.012
Chen, W., Tang, H., and Lin, H. (2017a). Methyrna: A web server for identification of N(6)-methyladenosine sites. J. Biomol. Struct. Dyn. 35, 683–687. doi: 10.1080/07391102.2016.1157761
Chen, W., Tang, H., Ye, J., Lin, H., and Chou, K. C. (2016c). irna-PseU: Identifying Rna pseudouridine sites. Mol. Ther. Nucleic Acids 5:e332. doi: 10.1038/mtna.2016.37
Chen, W., Tran, H., Liang, Z., Lin, H., and Zhang, L. (2015b). Identification and analysis of the N(6)-methyladenosine in the Saccharomyces cerevisiae transcriptome. Sci. Rep. 5:13859. doi: 10.1038/srep13859
Chen, W., Xing, P., and Zou, Q. (2017b). Detecting N6-methyladenosine sites from Rna transcriptomes using ensemble support vector machines. Sci. Rep. 7:40242. doi: 10.1038/srep40242
Chen, X., Sun, Y. Z., Liu, H., Zhang, L., Li, J. Q., and Meng, J. (2019b). Rna methylation and diseases: Experimental results, databases, Web servers and computational models. Brief Bioinform. 20, 896–917. doi: 10.1093/bib/bbx142
Chen, X., Xiong, Y., Liu, Y., Chen, Y., Bi, S., and Zhu, X. (2020b). m5cpred-Svm: A novel method for predicting m5C sites of Rna. BMC Bioinform. 21:489. doi: 10.1186/s12859-020-03828-4
Chen, Z., Zhao, P., Li, F., Wang, Y., Smith, A. I., Webb, G. I., et al. (2020c). Comprehensive review and assessment of computational methods for predicting Rna post-transcriptional modification sites from Rna sequences. Brief Bioinform. 21, 1676–1696. doi: 10.1093/bib/bbz112
Church, C., Lee, S., Bagg, E. A., Mctaggart, J. S., Deacon, R., Gerken, T., et al. (2009). A mouse model for the metabolic effects of the human fat mass and obesity associated Fto gene. PLoS Genet. 5:e1000599. doi: 10.1371/journal.pgen.1000599
Church, C., Moir, L., Mcmurray, F., Girard, C., Banks, G. T., Teboul, L., et al. (2010). Overexpression of Fto leads to increased food intake and results in obesity. Nat. Genet. 42, 1086–1092. doi: 10.1038/ng.713
Crain, P., Choi, Y., Busch, H., and McCloskey, J. (1978). Characterization of N6, O2′-dimethyladenosine from nuclear Rna of Novikoff hepatoma. Nucleic Acids Res. 5, 771–776. doi: 10.1093/nar/5.3.771
Croft, D., O’kelly, G., Wu, G., Haw, R., Gillespie, M., Matthews, L., et al. (2011). Reactome: A database of reactions, pathways and biological processes. Nucleic Acids Res. 39, D691–D697. doi: 10.1093/nar/gkq1018
Dao, F. Y., Lv, H., Yang, Y. H., Zulfiqar, H., Gao, H., and Lin, H. (2020). Computational identification of N6-methyladenosine sites in multiple tissues of mammals. Comput. Struct. Biotechnol. J. 18, 1084–1091. doi: 10.1016/j.csbj.2020.04.015
Delatte, B., Wang, F., Ngoc, L., Collignon, E., Bonvin, E., Deplus, R., et al. (2016). Transcriptome-wide distribution and function of Rna hydroxymethylcytosine. Science 351, 282–285. doi: 10.1126/science.aac5253
Deng, S., Zhang, H., Zhu, K., Li, X., Ye, Y., Li, R., et al. (2021). M6A2Target: A comprehensive database for targets of m6A writers, erasers and readers. Brief Bioinform. 22:bbaa055. doi: 10.1093/bib/bbaa055
Desrosiers, R., Friderici, K., and Rottman, F. (1974). Identification of methylated nucleosides in messengerrna from Novikoff Hepatoma cells. Proc. Natl. Acad. Sci. U.S.A. 71, 3971–3975. doi: 10.1073/pnas.71.10.3971
Dominissini, D., Moshitch-Moshkovitz, S., Schwartz, S., Salmon-Divon, M., Ungar, L., Osenberg, S., et al. (2012). Topology of the human and mouse m6A Rna methylomes revealed by m6A-seq. Nature 485, 201–206. doi: 10.1038/nature11112
Dominissini, D., Nachtergaele, S., Moshitch-Moshkovitz, S., Peer, E., Kol, N., Ben-Haim, M. S., et al. (2016). The dynamic N(1)-methyladenosine methylome in eukaryotic messenger Rna. Nature 530, 441–446. doi: 10.1038/nature16998
Dou, L., Li, X., Ding, H., Xu, L., and Xiang, H. (2020a). irna-m5C_Nb: A novel predictor to identify rna 5-methylcytosine sites based on the naive bayes classifier. IEEE Access. 8, 84906–84917. doi: 10.1109/access.2020.2991477
Dou, L., Li, X., Ding, H., Xu, L., and Xiang, H. (2020b). Prediction of m5C modifications in rna sequences by combining multiple sequence features. Mol. Ther. Nucleic Acids 21, 332–342. doi: 10.1016/j.omtn.2020.06.004
Du, H., Zhao, Y., He, J., Zhang, Y., Xi, H., Liu, M., et al. (2016). Ythdf2 destabilizes m(6)A-containing Rna through direct recruitment of the Ccr4-Not deadenylase complex. Nat. Commun. 7:12626. doi: 10.1038/ncomms12626
Du, K. Z., Zhang, L. B., Lee, T., and Sun, T. (2019). m(6)A Rna methylation controls neural development and is involved in human diseases. Mol. Neurobiol. 56, 1596–1606. doi: 10.1007/s12035-018-1138-1
Dunin-Horkawicz, S., Czerwoniec, A., Gajda, M. J., Feder, M., Grosjean, H., and Bujnicki, J. M. (2006). Modomics: A database of Rna modification pathways. Nucleic Acids Res. 34, D145–D149. doi: 10.1093/nar/gkj084
Edelheit, S., Schwartz, S., Mumbach, M. R., Wurtzel, O., and Sorek, R. (2013). Transcriptome-wide mapping of 5-methylcytidine Rna modifications in bacteria, archaea, and yeast reveals m5C within archaeal mrnas. PLoS Genet. 9:e1003602. doi: 10.1371/journal.pgen.1003602
El Allali, A., Elhamraoui, Z., and Daoud, R. (2021). Machine learning applications in Rna modification sites prediction. Comput. Struct. Biotechnol. J. 19, 5510–5524. doi: 10.1016/j.csbj.2021.09.025
El Yacoubi, B., Bailly, M., and De Crecy-Lagard, V. (2012). Biosynthesis and function of posttranscriptional modifications of transfer Rnas. Annu. Rev. Genet. 46, 69–95. doi: 10.1146/annurev-genet-110711-155641
Fan, J., Wang, K., Du, X., Wang, J., Chen, S., Wang, Y., et al. (2019). Alyref links 3′-end processing to nuclear export of non-polyadenylated mrnas. EMBO J. 38:e99910. doi: 10.15252/embj.201899910
Fang, T., Zhang, Z., Sun, R., Zhu, L., He, J., Huang, B., et al. (2019). Rnam5cpred: Prediction of Rna 5-methylcytosine sites based on three different kinds of nucleotide composition. Mol. Ther. Nucleic Acids 18, 739–747. doi: 10.1016/j.omtn.2019.10.008
Feng, P., Ding, H., Chen, W., and Lin, H. (2016). Identifying Rna 5-methylcytosine sites via pseudo nucleotide compositions. Mol. Biosyst. 12, 3307–3311. doi: 10.1039/c6mb00471g
Feng, P., Ding, H., Yang, H., Chen, W., Lin, H., and Chou, K. C. (2017). irna-PseColl: Identifying the occurrence sites of different rna modifications by incorporating collective effects of nucleotides into pseknc. Mol. Ther. Nucleic Acids 7, 155–163. doi: 10.1016/j.omtn.2017.03.006
Fernandez, I. S., Ng, C. L., Kelley, A. C., Wu, G., Yu, Y. T., and Ramakrishnan, V. (2013). Unusual base pairing during the decoding of a stop codon by the ribosome. Nature 500, 107–110. doi: 10.1038/nature12302
Fischer, J., Koch, L., Emmerling, C., Vierkotten, J., Peters, T., Bruning, J. C., et al. (2009). Inactivation of the Fto gene protects from obesity. Nature 458, 894–898. doi: 10.1038/nature07848
Flamand, M. N., and Meyer, K. D. (2019). The epitranscriptome and synaptic plasticity. Curr. Opin. Neurobiol. 59, 41–48. doi: 10.1016/j.conb.2019.04.007
Flores, J. V., Cordero-Espinoza, L., Oeztuerk-Winder, F., Andersson-Rolf, A., Selmi, T., Blanco, S., et al. (2017). Cytosine-5 Rna methylation regulates neural stem cell differentiation and motility. Stem Cell Rep. 8, 112–124. doi: 10.1016/j.stemcr.2016.11.014
Fu, L., Guerrero, C. R., Zhong, N., Amato, N. J., Liu, Y., Liu, S., et al. (2014). Tet-mediated formation of 5-hydroxymethylcytosine in Rna. J. Am. Chem. Soc. 136, 11582–11585. doi: 10.1021/ja505305z
Fu, Y., Jia, G., Pang, X., Wang, R. N., Wang, X., Li, C. J., et al. (2013). Fto-mediated formation of N6-hydroxymethyladenosine and N6-formyladenosine in mammalian Rna. Nat. Commun. 4:1798. doi: 10.1038/ncomms2822
Furlan, M., Delgado-Tejedor, A., Mulroney, L., Pelizzola, M., Novoa, E. M., and Leonardi, T. (2021). Computational methods for Rna modification detection from nanopore direct Rna sequencing data. RNA Biol. 18, 31–40. doi: 10.1080/15476286.2021.1978215
Fustin, J. M., Doi, M., Yamaguchi, Y., Hida, H., Nishimura, S., Yoshida, M., et al. (2013). Rna-methylation-dependent Rna processing controls the speed of the circadian clock. Cell 155, 793–806. doi: 10.1016/j.cell.2013.10.026
Gao, Y., Shang, S., Guo, S., Li, X., Zhou, H., Liu, H., et al. (2021). Lnc2Cancer 3.0: An updated resource for experimentally supported lncrna/circrna cancer associations and web tools based on Rna-seq and scrna-seq data. Nucleic Acids Res. 49, D1251–D1258. doi: 10.1093/nar/gkaa1006
Gao, Y., Shang, S., Guo, S., Wang, X., Zhou, H., Sun, Y., et al. (2022). AgingBank: A manually curated knowledgebase and high-throughput analysis platform that provides experimentally supported multi-omics data relevant to aging in multiple species. Brief Bioinform. 23:bbac438. doi: 10.1093/bib/bbac438
Gerken, T., Girard, C. A., Tung, Y. C., Webby, C. J., Saudek, V., Hewitson, K. S., et al. (2007). The obesity-associated Fto gene encodes a 2-oxoglutarate-dependent nucleic acid demethylase. Science 318, 1469–1472. doi: 10.1126/science.1151710
Geuens, T., Bouhy, D., and Timmerman, V. (2016). The hnrnp family: Insights into their role in health and disease. Hum. Genet. 135, 851–867. doi: 10.1007/s00439-016-1683-5
Goll, M. G., Kirpekar, F., Maggert, K. A., Yoder, J. A., Hsieh, C. L., Zhang, X., et al. (2006). Methylation of trnaasp by the Dna methyltransferase homolog Dnmt2. Science 311, 395–398. doi: 10.1126/science.1120976
Goodman, H. M., Abelson, J., Landy, A., Brenner, S., and Smith, J. D. (1968). Amber suppression: A nucleotide change in the anticodon of a tyrosine transfer Rna. Nature 217, 1019–1024. doi: 10.1038/2171019a0
Group, P. T. C., Calabrese, C., Davidson, N. R., Demircioglu, D., Fonseca, N. A., He, Y., et al. (2020). Genomic basis for Rna alterations in cancer. Nature 578, 129–136. doi: 10.1038/s41586-020-1970-0
Guil, S., and Cáceres, J. F. (2007). The multifunctional Rna-binding protein hnrnp A1 is required for processing of miR-18a. Nat. Struct. Mol. Biol. 14, 591–596. doi: 10.1038/nsmb1250
Guo, G., Pan, K., Fang, S., Ye, L., Tong, X., Wang, Z., et al. (2021). Advances in mrna 5-methylcytosine modifications: Detection, effectors, biological functions, and clinical relevance. Mol. Ther. Nucleic Acids 26, 575–593. doi: 10.1016/j.omtn.2021.08.020
Guo, J., Tang, H. W., Li, J., Perrimon, N., and Yan, D. (2018). Xio is a component of the Drosophila sex determination pathway and Rna N(6)-methyladenosine methyltransferase complex. Proc. Natl. Acad. Sci. U.S.A. 115, 3674–3679. doi: 10.1073/pnas.1720945115
Han, S. P., Tang, Y. H., and Smith, R. (2010). Functional diversity of the hnrnps: Past, present and perspectives. Biochem. J. 430, 379–392. doi: 10.1042/Bj20100396
Han, Y., Feng, J., Xia, L., Dong, X., Zhang, X., Zhang, S., et al. (2019). Cvm6A: A visualization and exploration database for m(6)As in cell lines. Cells 8:168. doi: 10.3390/cells8020168
Harper, J. E., Miceli, S. M., Roberts, R. J., and Manley, J. L. (1990). Sequence specificity of the human mrna N6-adenosine methylase in vitro. Nucleic Acids Res. 18, 5735–5741. doi: 10.1093/nar/18.19.5735
He, J., Fang, T., Zhang, Z., Huang, B., Zhu, X., and Xiong, Y. (2018). Pseui: Pseudouridine sites identification based on Rna sequence information. BMC Bioinform. 19:306. doi: 10.1186/s12859-018-2321-0
Helm, M., and Motorin, Y. (2017). Detecting Rna modifications in the epitranscriptome: Predict and validate. Nat. Rev. Genet. 18, 275–291. doi: 10.1038/nrg.2016.169
Hess, M. E., Hess, S., Meyer, K. D., Verhagen, L. A., Koch, L., Bronneke, H. S., et al. (2013). The fat mass and obesity associated gene (Fto) regulates activity of the dopaminergic midbrain circuitry. Nat. Neurosci. 16, 1042–1048. doi: 10.1038/nn.3449
Huang, Y., He, N., Chen, Y., Chen, Z., and Li, L. (2018). Bermp: A cross-species classifier for predicting m(6)A sites by integrating a deep learning algorithm and a random forest approach. Int. J. Biol. Sci. 14, 1669–1677. doi: 10.7150/ijbs.27819
Huang, Z., Pan, J., Wang, H., Du, X., Xu, Y., Wang, Z., et al. (2021). Prognostic significance and tumor immune microenvironment heterogenicity of m5C Rna methylation regulators in triple-negative breast cancer. Front. Cell Dev. Biol. 9:657547. doi: 10.3389/fcell.2021.657547
Hussain, S., Sajini, A. A., Blanco, S., Dietmann, S., Lombard, P., Sugimoto, Y., et al. (2013). Nsun2-mediated cytosine-5 methylation of vault noncoding Rna determines its processing into regulatory small Rnas. Cell Rep. 4, 255–261. doi: 10.1016/j.celrep.2013.06.029
Ito, S., Shen, L., Dai, Q., Wu, S. C., Collins, L. B., Swenberg, J. A., et al. (2011). Tet proteins can convert 5-methylcytosine to 5-Formylcytosine and 5-Carboxylcytosine. Science 333, 1300–1303. doi: 10.1126/science.1210597
Jia, C. Z., Zhang, J. J., and Gu, W. Z. (2016). Rna-MethylPred: A high-accuracy predictor to identify N6-methyladenosine in Rna. Anal. Biochem. 510, 72–75. doi: 10.1016/j.ab.2016.06.012
Jia, G., Fu, Y., Zhao, X., Dai, Q., Zheng, G., Yang, Y., et al. (2011). N6-methyladenosine in nuclear Rna is a major substrate of the obesity-associated Fto. Nat. Chem. Biol. 7, 885–887. doi: 10.1038/nchembio.687
Jia, G., Yang, C. G., Yang, S., Jian, X., Yi, C., Zhou, Z., et al. (2008). Oxidative demethylation of 3-methylthymine and 3-methyluracil in single-stranded Dna and Rna by mouse and human Fto. FEBS Lett. 582, 3313–3319. doi: 10.1016/j.febslet.2008.08.019
Jiang, J., Song, B., Chen, K., Lu, Z., Rong, R., Zhong, Y., et al. (2022). m6AmPred: Identifying Rna N6, 2′-O-dimethyladenosine (m(6)Am) sites based on sequence-derived information. Methods 203, 328–334. doi: 10.1016/j.ymeth.2021.01.007
Jiang, S., Xie, Y., He, Z., Zhang, Y., Zhao, Y., Chen, L., et al. (2018). m6asnp: A tool for annotating genetic variants by m6A function. Gigascience 7:giy035. doi: 10.1093/gigascience/giy035
Jiang, X., Liu, B., Nie, Z., Duan, L., Xiong, Q., Jin, Z., et al. (2021). The role of m6A modification in the biological functions and diseases. Signal Transduct Target Ther. 6:74. doi: 10.1038/s41392-020-00450-x
Jonkhout, N., Tran, J., Smith, M. A., Schonrock, N., Mattick, J. S., and Novoa, E. M. (2017). The Rna modification landscape in human disease. RNA 23, 1754–1769. doi: 10.1261/rna.063503.117
Karijolich, J., and Yu, Y. T. (2010). Spliceosomal snrna modifications and their function. RNA Biol. 7, 192–204. doi: 10.4161/rna.7.2.11207
Khan, A., Rehman, H. U., Habib, U., and Ijaz, U. (2020a). Detecting N6-methyladenosine sites from Rna transcriptomes using random forest. J. Comput. Sci. 47:40242. doi: 10.1016/j.jocs.2020.101238
Khan, M. A., Rafiq, M. A., Noor, A., Hussain, S., Flores, J. V., Rupp, V., et al. (2012). Mutation in Nsun2, which encodes an Rna methyltransferase, causes autosomal-recessive intellectual disability. Am. J. Hum. Genet. 90, 856–863. doi: 10.1016/j.ajhg.2012.03.023
Khan, S. M., He, F., Wang, D., Chen, Y., and Xu, D. (2020b). Mu-Pseudeep: A deep learning method for prediction of pseudouridine sites. Comput. Struct. Biotechnol. J. 18, 1877–1883. doi: 10.1016/j.csbj.2020.07.010
Kiran, A. M., O’mahony, J. J., Sanjeev, K., and Baranov, P. V. (2013). Darned in 2013: Inclusion of model organisms and linking with Wikipedia. Nucleic Acids Res. 41, D258–D261. doi: 10.1093/nar/gks961
Knuckles, P., Lence, T., Haussmann, I. U., Jacob, D., Kreim, N., Carl, S. H., et al. (2018). Zc3h13/Flacc is required for adenosine methylation by bridging the mrna-binding factor Rbm15/Spenito to the m(6)A machinery component Wtap/Fl(2)d. Genes Dev. 32, 415–429. doi: 10.1101/gad.309146.117
Kortel, N., Ruckle, C., Zhou, Y., Busch, A., Hoch-Kraft, P., Sutandy, F. X. R., et al. (2021). Deep and accurate detection of m6A Rna modifications using miclip2 and m6Aboost machine learning. Nucleic Acids Res. 49:e92. doi: 10.1093/nar/gkab485
Kriaucionis, S., and Heintz, N. (2009). The Nuclear Dna Base 5-Hydroxymethylcytosine is present in purkinje neurons and the brain. Science 324, 929–930. doi: 10.1126/science.1169786
Krug, R., Morgan, M., and Shatkin, A. (1976). Influenza viral mrna con- tains internal N6 -methyladenosine and 5′-terminal 7-methyl- guanosine in cap structures. J. Virol. 20, 45–53. doi: 10.1128/Jvi.20.1.45-53.1976
Labranche, H., Dupuis, S., Ben-David, Y., Bani, M. R., Wellinger, R. J., and Chabot, B. (1998). Telomere elongation by hnrnp A1 and a derivative that interacts with telomeric repeats and telomerase. Nat. Genet. 19, 199–202. doi: 10.1038/575
Leach, R., and Tuck, M. (2001). Expression of the mrna (N6-adeno sine)-methyltransferase S-adenosyl-L-methionine binding subunit mrna in cultured cells. Int. J. Biochem. Cell Biol. 33, 984–999. doi: 10.1016/s1357-2725(01)00071-1
Lence, T., Akhtar, J., Bayer, M., Schmid, K., Spindler, L., Ho, C. H., et al. (2016). m6A modulates neuronal functions and sex determination in Drosophila. Nature 540, 242–247. doi: 10.1038/nature20568
Li, A., Chen, Y. S., Ping, X. L., Yang, X., Xiao, W., Yang, Y., et al. (2017). Cytoplasmic m(6)A reader Ythdf3 promotes mrna translation. Cell Res. 27, 444–447. doi: 10.1038/cr.2017.10
Li, F., Guo, X., Jin, P., Chen, J., Xiang, D., Song, J., et al. (2021a). Porpoise: A new approach for accurate prediction of Rna pseudouridine sites. Brief Bioinform. 22:bbab245. doi: 10.1093/bib/bbab245
Li, G. Q., Liu, Z., Shen, H. B., and Yu, D. J. (2016a). TargetM6A: Identifying N(6)-methyladenosine sites from Rna sequences via position-specific nucleotide propensities and a support vector machine. IEEE Trans. Nanobiosci. 15, 674–682. doi: 10.1109/Tnb.2016.2599115
Li, J., Huang, Y., Yang, X., Zhou, Y., and Zhou, Y. (2018a). Rnam5Cfinder: A web-server for predicting Rna 5-methylcytosine (m5C) sites based on random forest. Sci. Rep. 8:17299. doi: 10.1038/s41598-018-35502-4
Li, J., Yang, X., Chu, Q., Xie, L., Ding, Y., Xu, X., et al. (2022a). Multi-omics molecular biomarkers and database of osteoarthritis. Database (Oxford) 2022:baac052. doi: 10.1093/database/baac052
Li, M., Zhao, X., Wang, W., Shi, H., Pan, Q., Lu, Z., et al. (2018b). Ythdf2-mediated m(6)A mrna clearance modulates neural development in mice. Genome Biol. 19:69. doi: 10.1186/s13059-018-1436-y
Li, X. J., Du, Z. W., Zarnowska, E. D., Pankratz, M., Hansen, L. O., Pearce, R. A., et al. (2005). Specification of motoneurons from human embryonic stem cells. Nat. Biotechnol. 23, 215–221. doi: 10.1038/nbt1063
Li, X., Xiong, X., and Yi, C. (2016b). Epitranscriptome sequencing technologies: Decoding Rna modifications. Nat. Methods 14, 23–31. doi: 10.1038/nmeth.4110
Li, X., Xiong, X., Wang, K., Wang, L., Shu, X., Ma, S., et al. (2016c). Transcriptome-wide mapping reveals reversible and dynamic N1-methyladenosine methylome. Nat. Chem. Biol. 12, 311–316. doi: 10.1038/nchembio.2040
Li, X., Zhang, S., and Shi, H. (2022b). An improved residual network using deep fusion for identifying Rna 5-methylcytosine sites. Bioinformatics 38, 4271–4277. doi: 10.1093/bioinformatics/btac532
Li, X., Zhu, P., Ma, S., Song, J., Bai, J., Sun, F., et al. (2015a). Chemical pulldown reveals dynamic pseudouridylation of the mammalian transcriptome. Nat. Chem. Biol. 11, 592–597. doi: 10.1038/nchembio.1836
Li, Y., Qi, D., Zhu, B., and Ye, X. (2021b). Analysis of m6A Rna methylation-related genes in liver hepatocellular carcinoma and their correlation with survival. Int. J. Mol. Sci. 22:1474. doi: 10.3390/ijms22031474
Li, Y.-H., Zhang, G., and Cui, Q. (2015b). Ppus: A web server to predict Pus-specific pseudouridine sites: Table 1. Bioinformatics 31, 3362–3364. doi: 10.1093/bioinformatics/btv366
Liao, J., Wang, Q., Wu, F., and Huang, Z. (2022). In silico methods for identification of potential active sites of therapeutic targets. Molecules 27:7103. doi: 10.3390/molecules27207103
Lin, Z., Hsu, P. J., Xing, X., Fang, J., Lu, Z., Zou, Q., et al. (2017). Mettl3-/Mettl14-mediated mrna N(6)-methyladenosine modulates murine spermatogenesis. Cell Res. 27, 1216–1230. doi: 10.1038/cr.2017.117
Liu, H., Wang, H., Wei, Z., Zhang, S., Hua, G., Zhang, S. W., et al. (2018). MeT-Db V2.0: Elucidating context-specific functions of N6-methyl-adenosine methyltranscriptome. Nucleic Acids Res. 46, D281–D287. doi: 10.1093/nar/gkx1080
Liu, J., Li, K., Cai, J., Zhang, M., Zhang, X., Xiong, X., et al. (2020a). Landscape and regulation of m(6)A and m(6)Am methylome across human and mouse tissues. Mol. Cell 77, 426–440e6. doi: 10.1016/j.molcel.2019.09.032
Liu, J., Sun, G., Pan, S., Qin, M., Ouyang, R., Li, Z., et al. (2020b). The cancer genome atlas (Tcga) based m(6)A methylation-related genes predict prognosis in hepatocellular carcinoma. Bioengineered 11, 759–768. doi: 10.1080/21655979.2020.1787764
Liu, J., Yue, Y., Han, D., Wang, X., Fu, Y., Zhang, L., et al. (2013). A Mettl3–Mettl14 complex mediates mammalian nuclear Rna N6-adenosine methylation. Nat. Chem. Biol. 10, 93–95. doi: 10.1038/nchembio.1432
Liu, K., and Chen, W. (2020). imrm: A platform for simultaneously identifying multiple kinds of Rna modifications. Bioinformatics 36, 3336–3342. doi: 10.1093/bioinformatics/btaa155
Liu, K., Cao, L., Du, P., and Chen, W. (2020c). im6A-Ts-Cnn: Identifying the N(6)-Methyladenine site in multiple tissues by using the convolutional neural network. Mol. Ther. Nucleic Acids 21, 1044–1049. doi: 10.1016/j.omtn.2020.07.034
Liu, K., Chen, W., and Lin, H. (2020d). Xg-PseU: An eXtreme Gradient Boosting based method for identifying pseudouridine sites. Mol. Genet. Genomics 295, 13–21. doi: 10.1007/s00438-019-01600-9
Liu, L., Lei, X., Fang, Z., Tang, Y., Meng, J., and Wei, Z. (2020e). Lithophone: Improving lncrna methylation site prediction using an ensemble predictor. Front. Genet. 11:545. doi: 10.3389/fgene.2020.00545
Liu, L., Lei, X., Meng, J., and Wei, Z. (2020f). Isgm1A: Integration of sequence features and genomic features to improve the prediction of human m1A Rna methylation sites. IEEE Access. 8, 81971–81977. doi: 10.1109/access.2020.2991070
Liu, L., Lei, X., Meng, J., and Wei, Z. (2020g). Witmsg: Large-scale prediction of human intronic m(6)A Rna methylation sites from sequence and genomic features. Curr. Genomics 21, 67–76. doi: 10.2174/1389202921666200211104140
Liu, N., Dai, Q., Zheng, G., He, C., Parisien, M., and Pan, T. (2015). N(6)-methyladenosine-dependent Rna structural switches regulate Rna-protein interactions. Nature 518, 560–564. doi: 10.1038/nature14234
Liu, N., Zhou, K. I., Parisien, M., Dai, Q., Diatchenko, L., and Pan, T. (2017). N6-methyladenosine alters Rna structure to regulate binding of a low-complexity protein. Nucleic Acids Res. 45, 6051–6063. doi: 10.1093/nar/gkx141
Liu, Q., and Gregory, R. I. (2019). Rnamod: An integrated system for the annotation of mrna modifications. Nucleic Acids Res. 47, W548–W555. doi: 10.1093/nar/gkz479
Liu, S., Zhu, A., He, C., and Chen, M. (2020h). Repic: A database for exploring the N(6)-methyladenosine methylome. Genome Biol. 21:100. doi: 10.1186/s13059-020-02012-4
Liu, Y., Chen, D., Su, R., Chen, W., and Wei, L. (2020i). irna5hmC: The first predictor to identify Rna 5-hydroxymethylcytosine modifications using machine learning. Front. Bioeng. Biotechnol. 8:227. doi: 10.3389/fbioe.2020.00227
Liu, Z., Xiao, X., Yu, D. J., Jia, J., Qiu, W. R., and Chou, K. C. (2016). prnam-Pc: Predicting N(6)-methyladenosine sites in Rna sequences via physical-chemical properties. Anal. Biochem. 497, 60–67. doi: 10.1016/j.ab.2015.12.017
Lo Giudice, C., Pesole, G., and Picardi, E. (2018). Redidb 3.0: A comprehensive collection of Rna editing events in plant organellar genomes. Front. Plant Sci. 9:482. doi: 10.3389/fpls.2018.00482
Luo, G. Z., Macqueen, A., Zheng, G., Duan, H., Dore, L. C., Lu, Z., et al. (2014). Unique features of the m6A methylome in Arabidopsis thaliana. Nat. Commun. 5:5630. doi: 10.1038/ncomms6630
Luo, X., Li, H., Liang, J., Zhao, Q., Xie, Y., Ren, J., et al. (2021). Rmvar: An updated database of functional variants involved in Rna modifications. Nucleic Acids Res. 49, D1405–D1412. doi: 10.1093/nar/gkaa811
Luo, Z., Su, W., Lou, L., Qiu, W., Xiao, X., and Xu, Z. (2022). Dlm6Am: A deep-learning-based tool for identifying N6,2′-O-Dimethyladenosine sites in rna sequences. Int. J. Mol. Sci. 23:11026. doi: 10.3390/ijms231911026
Lv, H., Dao, F. Y., Zhang, D., Yang, H., and Lin, H. (2021). Advances in mapping the epigenetic modifications of 5-methylcytosine (5mC), N6-methyladenine (6mA), and N4-methylcytosine (4mC). Biotechnol. Bioeng. 118, 4204–4216. doi: 10.1002/bit.27911
Lv, H., Zhang, Z. M., Li, S. H., Tan, J. X., Chen, W., and Lin, H. (2020a). Evaluation of different computational methods on 5-methylcytosine sites identification. Brief Bioinform. 21, 982–995. doi: 10.1093/bib/bbz048
Lv, Z., Zhang, J., Ding, H., and Zou, Q. (2020b). Rf-PseU: A random forest predictor for rna pseudouridine sites. Front. Bioeng. Biotechnol. 8:134. doi: 10.3389/fbioe.2020.00134
Ma, C., Chang, M., Lv, H., Zhang, Z. W., Zhang, W., He, X., et al. (2018). Rna m(6)A methylation participates in regulation of postnatal development of the mouse cerebellum. Genome Biol. 19:68. doi: 10.1186/s13059-018-1435-z
Ma, J., Song, B., Wei, Z., Huang, D., Zhang, Y., Su, J., et al. (2022). m5C-Atlas: A comprehensive database for decoding and annotating the 5-methylcytosine (m5C) epitranscriptome. Nucleic Acids Res. 50, D196–D203. doi: 10.1093/nar/gkab1075
Mahmoudi, O., Wahab, A., and Chong, K. T. (2020). iMethyl-Deep: N6 methyladenosine identification of yeast genome with automatic feature extraction technique by using deep learning algorithm. Genes (Basel) 11:529. doi: 10.3390/genes11050529
Massignani, E., Giambruno, R., Maniaci, M., Nicosia, L., Yadav, A., Cuomo, A., et al. (2022). ProMetheusdb: An in-depth analysis of the high-quality human Methyl-proteome. Mol. Cell Proteomics 21:100243. doi: 10.1016/j.mcpro.2022.100243
Mauer, J., Luo, X., Blanjoie, A., Jiao, X., Grozhik, A. V., Patil, D. P., et al. (2016). Reversible methylation of m6Am in the 5′ cap controls mrna stability. Nature 541, 371–375. doi: 10.1038/nature21022
Meyer, K. D., Saletore, Y., Zumbo, P., Elemento, O., Mason, C. E., and Jaffrey, S. R. (2012). Comprehensive analysis of mrna methylation reveals enrichment in 3′. Utrs and near stop codons. Cell 149, 1635–1646. doi: 10.1016/j.cell.2012.05.003
Molinie, B., Wang, J., Lim, K. S., Hillebrand, R., Lu, Z. X., Van Wittenberghe, N., et al. (2016). m(6)A-Laic-seq reveals the census and complexity of the m(6)A epitranscriptome. Nat. Methods 13, 692–698. doi: 10.1038/nmeth.3898
Mu, Y., Zhang, R., Wang, L., and Liu, X. (2020). iPseU-Layer: Identifying Rna pseudouridine sites using layered ensemble model. Interdiscip. Sci. 12, 193–203. doi: 10.1007/s12539-020-00362-y
Nachtergaele, S., and He, C. (2017). The emerging biology of Rna post-transcriptional modifications. RNA Biol. 14, 156–163. doi: 10.1080/15476286.2016.1267096
Nazari, I., Tahir, M., Tayara, H., and Chong, K. T. (2019). iN6-Methyl (5-step): Identifying Rna N6-methyladenosine sites using deep learning mode via Chou’s 5-step rules and Chou’s general Pseknc. Chemometr. Intell. Lab. Syst. 193:103811. doi: 10.1016/j.chemolab.2019.103811
Nguyen-Vo, T.-H., Nguyen, Q. H., Do, T. T. T., Nguyen, T.-N., Rahardja, S., and Nguyen, B. P. (2019). iPseU-Ncp: Identifying Rna pseudouridine sites using random forest and Ncp-encoded features. BMC Genomics 20:971. doi: 10.1186/s12864-019-6357-y
Ni, W., Yao, S., Zhou, Y., Liu, Y., Huang, P., Zhou, A., et al. (2019). Long noncoding Rna Gas5 inhibits progression of colorectal cancer by interacting with and triggering Yap phosphorylation and degradation and is negatively regulated by the m(6)A reader Ythdf3. Mol. Cancer 18:143. doi: 10.1186/s12943-019-1079-y
Nie, F., Feng, P., Song, X., Wu, M., Tang, Q., and Chen, W. (2020). Rnawre: A resource of writers, readers and erasers of Rna modifications. Database (Oxford) 2020:baaa049. doi: 10.1093/database/baaa049
Nombela, P., Miguel-Lopez, B., and Blanco, S. (2021). The role of m(6)A, m(5)C and Psi Rna modifications in cancer: Novel therapeutic opportunities. Mol. Cancer 20:18. doi: 10.1186/s12943-020-01263-w
Oerum, S., Meynier, V., Catala, M., and Tisne, C. (2021). A comprehensive review of m6A/m6Am Rna methyltransferase structures. Nucleic Acids Res. 49, 7239–7255. doi: 10.1093/nar/gkab378
Ortega, A., Niksic, M., Bachi, A., Wilm, M., Sanchez, L., Hastie, N., et al. (2003). Biochemical function of female-lethal (2)D/Wilms’ tumor suppressor-1-associated proteins in alternative pre-mrna splicing. J. Biol. Chem. 278, 3040–3047. doi: 10.1074/jbc.M210737200
Pan, H., Tan, P. F., Lim, I. Y., Huan, J., Teh, A. L., Chen, L., et al. (2022). Integrative multi-omics database (imomdb) of Asian pregnant women. Hum. Mol. Genet. 31, 3051–3067. doi: 10.1093/hmg/ddac079
Panwar, B., and Raghava, G. P. S. (2014). Prediction of uridine modifications in trna sequences. BMC Bioinform. 15:326. doi: 10.1186/1471-2105-15-326
Patil, D. P., Chen, C. K., Pickering, B. F., Chow, A., Jackson, C., Guttman, M., et al. (2016). m(6)A Rna methylation promotes Xist-mediated transcriptional repression. Nature 537, 369–373. doi: 10.1038/nature19342
Pendleton, K. E., Chen, B., Liu, K., Hunter, O. V., Xie, Y., Tu, B. P., et al. (2017). The U6 snrna m(6)A Methyltransferase Mettl16 regulates sam synthetase intron retention. Cell 169, 824–835e14. doi: 10.1016/j.cell.2017.05.003
Ping, X. L., Sun, B. F., Wang, L., Xiao, W., Yang, X., Wang, W. J., et al. (2014b). Mammalian Wtap is a regulatory subunit of the Rna N6-methyladenosine methyltransferase. Cell Res. 24, 177–189.
Ping, X.-L., Sun, B.-F., Wang, L., Xiao, W., Yang, X., Wang, W.-J., et al. (2014a). Mammalian Wtap is a regulatory subunit of the Rna N6-methyladenosine methyltransferase. Cell Res. 24, 177–189. doi: 10.1038/cr.2014.3
Qiang, X., Chen, H., Ye, X., Su, R., and Wei, L. (2018). M6amrfs: Robust prediction of N6-methyladenosine sites with sequence-based features in multiple species. Front. Genet. 9:495. doi: 10.3389/fgene.2018.00495
Qiu, W. R., Jiang, S. Y., Xu, Z. C., Xiao, X., and Chou, K. C. (2017). irnam5C-Psednc: Identifying Rna 5-methylcytosine sites by incorporating physical-chemical properties into pseudo dinucleotide composition. Oncotarget 8, 41178–41188. doi: 10.18632/oncotarget.17104
Rehman, M. U., Hong, K. J., Tayara, H., and Chong, K. T. (2021a). m6A-NeuralTool: Convolution neural tool for Rna N6-methyladenosine site identification in different species. IEEE Access. 9, 17779–17786. doi: 10.1109/access.2021.3054361
Rehman, M. U., Tayara, H., and Chong, K. T. (2021b). Dcnn-4mC: Densely connected neural network based N4-methylcytosine site prediction in multiple species. Comput. Struct. Biotechnol. J. 19, 6009–6019. doi: 10.1016/j.csbj.2021.10.034
Rehman, M. U., Tayara, H., and Chong, K. T. (2022a). “Dl-M6A: Identification of N6-methyladenosine sites in mammals using deep learning based on different encoding schemes,” in IEEE/ACM Transactions on computational biology and bioinformatics, (Piscataway: IEEE). doi: 10.1109/Tcbb.2022.3192572
Rehman, M. U., Tayara, H., Zou, Q., and Chong, K. T. (2022b). i6mA-Caps: A CapsuleNet-based framework for identifying Dna N6-methyladenine sites. Bioinformatics 38, 3885–3891. doi: 10.1093/bioinformatics/btac434
Ries, R. J., Zaccara, S., Klein, P., Olarerin-George, A., Namkoong, S., Pickering, B. F., et al. (2019). m(6)A enhances the phase separation potential of mrna. Nature 571, 424–428. doi: 10.1038/s41586-019-1374-1
Roost, C., Lynch, S. R., Batista, P. J., Qu, K., Chang, H. Y., and Kool, E. T. (2015). Structure and thermodynamics of N6-methyladenosine in Rna: A spring-loaded base modification. J. Am. Chem. Soc. 137, 2107–2115. doi: 10.1021/ja513080v
Roundtree, I. A., Evans, M. E., Pan, T., and He, C. (2017a). Dynamic Rna modifications in gene expression regulation. Cell 169, 1187–1200. doi: 10.1016/j.cell.2017.05.045
Roundtree, I. A., Luo, G. Z., Zhang, Z., Wang, X., Zhou, T., Cui, Y., et al. (2017b). Ythdc1 mediates nuclear export of N(6)-methyladenosine methylated mrnas. Elife 6:e31311. doi: 10.7554/eLife.31311
Ryvkin, P., Leung, Y. Y., Silverman, I. M., Childress, M., Valladares, O., Dragomir, I., et al. (2013). Hamr: High-throughput annotation of modified ribonucleotides. RNA 19, 1684–1692. doi: 10.1261/rna.036806.112
Sabooh, M. F., Iqbal, N., Khan, M., Khan, M., and Maqbool, H. F. (2018). Identifying 5-methylcytosine sites in Rna sequence using composite encoding feature into Chou’s Pseknc. J. Theory Biol. 452, 1–9. doi: 10.1016/j.jtbi.2018.04.037
Sajek, M. P., Wozniak, T., Sprinzl, M., Jaruzelska, J., and Barciszewski, J. (2020). T-psi-C: User friendly database of trna sequences and structures. Nucleic Acids Res. 48, D256–D260. doi: 10.1093/nar/gkz922
Schwartz, S., Agarwala, S. D., Mumbach, M. R., Jovanovic, M., Mertins, P., Shishkin, A., et al. (2013). High-resolution mapping reveals a conserved, widespread, dynamic mrna methylation program in yeast meiosis. Cell 155, 1409–1421. doi: 10.1016/j.cell.2013.10.047
Schwartz, S., Bernstein, D. A., Mumbach, M. R., Jovanovic, M., Herbst, R. H., Leon-Ricardo, B. X., et al. (2014a). Transcriptome-wide mapping reveals widespread dynamic-regulated pseudouridylation of ncrna and mrna. Cell 159, 148–162. doi: 10.1016/j.cell.2014.08.028
Schwartz, S., Mumbach, M. R., Jovanovic, M., Wang, T., Maciag, K., Bushkin, G. G., et al. (2014b). Perturbation of m6A writers reveals two distinct classes of mrna methylation at Internal and 5′ Sites. Cell Rep. 8, 284–296. doi: 10.1016/j.celrep.2014.05.048
Schwartz, S., Mumbach, M., Jovanovic, M., Wang, T., Maciag, K., Bushkin, G., et al. (2014). Perturbation of m6A writers reveals two distinct classes of mrna methylation at internal and 5′ sites. Cell Rep. 8, 284–296.
Shi, H., Zhang, S., and Li, X. (2022). R5hmcfdv: Computational identification of Rna 5-hydroxymethylcytosine based on deep feature fusion and deep voting. Brief Bioinform. 23:bbac341. doi: 10.1093/bib/bbac341
Shi, M., Zhang, H., Wu, X., He, Z., Wang, L., Yin, S., et al. (2017). Alyref mainly binds to the 5′ and the 3′ regions of the mrna in vivo. Nucleic Acids Res. 45, 9640–9653. doi: 10.1093/nar/gkx597
Soh, Y. Q. S., Mikedis, M. M., Kojima, M., Godfrey, A. K., De Rooij, D. G., and Page, D. C. (2017). Meioc maintains an extended meiotic prophase I in mice. PLoS Genet. 13:e1006704. doi: 10.1371/journal.pgen.1006704
Song, B., Chen, K., Tang, Y., Ma, J., Meng, J., and Wei, Z. (2020a). Psi-Mouse: Predicting mouse pseudouridine sites from sequence and genome-derived features. Evol. Bioinform. 16:1176934320925752. doi: 10.1177/1176934320925752
Song, B., Chen, K., Tang, Y., Wei, Z., Su, J., De Magalhaes, J. P., et al. (2021a). Consrm: Collection and large-scale prediction of the evolutionarily conserved Rna methylation sites, with implications for the functional epitranscriptome. Brief Bioinform. 22:bbab088. doi: 10.1093/bib/bbab088
Song, B., Huang, D., Zhang, Y., Wei, Z., Su, J., Pedro de Magalhães, J., et al. (2022a). m6A-TSHub: Unveiling the context-specific m6A methylation and m6A-affecting mutations in 23 human tissues. Genom. Proteom. Bioinform. doi: 10.1016/j.gpb.2022.09.001
Song, B., Wang, X., Liang, Z., Ma, J., Huang, D., Wang, Y., et al. (2022b). Rmdisease V2.0: An updated database of genetic variants that affect Rna modifications with disease and trait implication. Nucleic Acids Res. doi: 10.1093/nar/gkac750
Song, B., Tang, Y., Wei, Z., Liu, G., Su, J., Meng, J., et al. (2020b). Piano: A web server for Pseudouridine-Site (Psi) identification and functional annotation. Front. Genet. 11:88. doi: 10.3389/fgene.2020.00088
Song, J., Zhai, J., Bian, E., Song, Y., Yu, J., and Ma, C. (2018). Transcriptome-wide annotation of m(5)C Rna modifications using machine learning. Front. Plant Sci. 9:519. doi: 10.3389/fpls.2018.00519
Song, Z., Huang, D., Song, B., Chen, K., Song, Y., Liu, G., et al. (2021b). Attention-based multi-label neural networks for integrated prediction and interpretation of twelve widely occurring Rna modifications. Nat. Commun. 12:4011. doi: 10.1038/s41467-021-24313-3
Squires, J. E., Patel, H. R., Nousch, M., Sibbritt, T., Humphreys, D. T., Parker, B. J., et al. (2012). Widespread occurrence of 5-methylcytosine in human coding and non-coding Rna. Nucleic Acids Res. 40, 5023–5033. doi: 10.1093/nar/gks144
Suleman, M. T., and Khan, Y. D. (2022). m1A-pred: Prediction of Modified 1-methyladenosine Sites in Rna sequences through artificial intelligence. Comb. Chem. High. Throughput Screen 25, 2473–2484. doi: 10.2174/1386207325666220617152743
Sun, P. P., Chen, Y. B., Liu, B., Gao, Y. X., Han, Y., He, F., et al. (2019). Deepmrmp: A new predictor for multiple types of Rna modification sites using deep learning. Math. Biosci. Eng. 16, 6231–6241. doi: 10.3934/mbe.2019310
Tahir, M., Hayat, M., and Chong, K. T. (2020). Prediction of N6-methyladenosine sites using convolution neural network model based on distributed feature representations. Neural Netw. 129, 385–391. doi: 10.1016/j.neunet.2020.05.027
Tahir, M., Hayat, M., and Chong, K. T. (2021). A convolution neural network-based computational model to identify the occurrence sites of various Rna modifications by fusing varied features. Chemometr. Intell. Lab. Syst. 211:104233. doi: 10.1016/j.chemolab.2021.104233
Tahir, M., Tayara, H., and Chong, K. T. (2019). iPseU-Cnn: Identifying Rna pseudouridine sites using convolutional neural networks. Mol. Ther. Nucleic Acids 16, 463–470. doi: 10.1016/j.omtn.2019.03.010
Tanabe, A., Tanikawa, K., Tsunetomi, M., Takai, K., Ikeda, H., Konno, J., et al. (2016). Rna helicase Ythdc2 promotes cancer metastasis via the enhancement of the efficiency by which Hif-1alpha mrna is translated. Cancer Lett. 376, 34–42. doi: 10.1016/j.canlet.2016.02.022
Tang, G., Cho, M., and Wang, X. (2022). Oncodb: An interactive online database for analysis of gene expression and viral infection in cancer. Nucleic Acids Res. 50, D1334–D1339. doi: 10.1093/nar/gkab970
Tang, Y., Chen, K., Song, B., Ma, J., Wu, X., Xu, Q., et al. (2021). m6A-Atlas: A comprehensive knowledgebase for unraveling the N6-methyladenosine (m6A) epitranscriptome. Nucleic Acids Res. 49, D134–D143. doi: 10.1093/nar/gkaa692
Trixl, L., Amort, T., Wille, A., Zinni, M., Ebner, S., Hechenberger, C., et al. (2018). Rna cytosine methyltransferase Nsun3 regulates embryonic stem cell differentiation by promoting mitochondrial activity. Cell Mol. Life Sci. 75, 1483–1497. doi: 10.1007/s00018-017-2700-0
Tuck, M. T. (1992). Partial purification of a 6-methyladenine mrna methyltransferase which modifies internal adenine residues. Biochem. J. 288 (Pt 1), 233–240. doi: 10.1042/bj2880233
Uyar, B., Yusuf, D., Wurmus, R., Rajewsky, N., Ohler, U., and Akalin, A. (2017). Rcas: An Rna centric annotation system for transcriptome-wide regions of interest. Nucleic Acids Res. 45:e91. doi: 10.1093/nar/gkx120
Van Haute, L., Lee, S. Y., Mccann, B. J., Powell, C. A., Bansal, D., Vasiliauskaite, L., et al. (2019). Nsun2 introduces 5-methylcytosines in mammalian mitochondrial trnas. Nucleic Acids Res. 47, 8720–8733. doi: 10.1093/nar/gkz559
Vandivier, L. E., and Gregory, B. D. (2017). Reading the Epitranscriptome: New techniques and perspectives. Enzymes 41, 269–298. doi: 10.1016/bs.enz.2017.03.004
Walkley, C. R., and Li, J. B. (2017). Rewriting the transcriptome: Adenosine-to-inosine Rna editing by Adars. Genome Biol. 18:205. doi: 10.1186/s13059-017-1347-3
Wang, C. X., Cui, G. S., Liu, X., Xu, K., Wang, M., Zhang, X. X., et al. (2018a). Mettl3-mediated m6A modification is required for cerebellar development. PLoS Biol. 16:e2004880. doi: 10.1371/journal.pbio.2004880
Wang, H., Liu, H., Huang, T., Li, G., Zhang, L., and Sun, Y. (2022a). Emdlp: Ensemble multiscale deep learning model for Rna methylation site prediction. BMC Bioinform. 23:221. doi: 10.1186/s12859-022-04756-1
Wang, H., Wang, S., Zhang, Y., Bi, S., and Zhu, X. (2022b). A brief review of machine learning methods for Rna methylation sites prediction. Methods 203, 399–421. doi: 10.1016/j.ymeth.2022.03.001
Wang, J., and Zhang, S. (2021). Pa-PseU: An incremental passive-aggressive based method for identifying Rna pseudouridine sites via Chou’s 5-steps rule. Chemometr. Intell. Lab. Syst. 210:104250. doi: 10.1016/j.chemolab.2021.104250
Wang, X., and Yan, R. (2018). RfathM6A: A new tool for predicting m(6)A sites in Arabidopsis thaliana. Plant Mol. Biol. 96, 327–337. doi: 10.1007/s11103-018-0698-9
Wang, X., Lu, Z., Gomez, A., Hon, G. C., Yue, Y., Han, D., et al. (2014). N6-methyladenosine-dependent regulation of messenger Rna stability. Nature 505, 117–120. doi: 10.1038/nature12730
Wang, X., Zhao, B. S., Roundtree, I. A., Lu, Z., Han, D., Ma, H., et al. (2015). N6-methyladenosine modulates messenger Rna translation efficiency. Cell 161, 1388–1399. doi: 10.1016/j.cell.2015.05.014
Wang, Y., Guo, R., Huang, L., Yang, S., Hu, X., and He, K. (2021). m6age: A Predictor for N6-methyladenosine sites identification utilizing sequence characteristics and graph embedding-based geometrical information. Front. Genet. 12:670852. doi: 10.3389/fgene.2021.670852
Wang, Y., Li, Y., Yue, M., Wang, J., Kumar, S., Wechsler-Reya, R. J., et al. (2018b). N(6)-methyladenosine Rna modification regulates embryonic neural stem cell self-renewal through histone modifications. Nat. Neurosci. 21, 195–206. doi: 10.1038/s41593-017-0057-1
Wang, Z., Jensen, M. A., and Zenklusen, J. C. (2016). A practical guide to the cancer genome atlas (Tcga). Methods Mol. Biol. 1418, 111–141. doi: 10.1007/978-1-4939-3578-9_6
Wei, C., Gershowitz, A., and Moss, B. (1975). N6, O2′-dimethyladenosine a novel methylated ribonucleoside next to the 5′ terminal of animal cell and virus mrnas. Nature 257, 251–253. doi: 10.1038/257251a0
Wei, L., Chen, H., and Su, R. (2018). M6apred-El: A sequence-based predictor for identifying n6-methyladenosine sites using ensemble learning. Mol. Ther. Nucleic Acids 12, 635–644. doi: 10.1016/j.omtn.2018.07.004
Wei, L., Su, R., Wang, B., Li, X., Zou, Q., and Gao, X. (2019). Integration of deep feature representations and handcrafted features to improve the prediction of N6-methyladenosine sites. Neurocomputing 324, 3–9. doi: 10.1016/j.neucom.2018.04.082
Wen, J., Lv, R., Ma, H., Shen, H., He, C., Wang, J., et al. (2018). Zc3h13 Regulates nuclear Rna m(6)A methylation and mouse embryonic stem cell self-renewal. Mol. Cell 69, 1028–1038e6. doi: 10.1016/j.molcel.2018.02.015
Wnuk, M., Slipek, P., Dziedzic, M., and Lewinska, A. (2020). The Roles of Host 5-methylcytosine Rna methyltransferases during viral infections. Int. J. Mol. Sci. 21:8176. doi: 10.3390/ijms21218176
Wojtas, M. N., Pandey, R. R., Mendel, M., Homolka, D., Sachidanandam, R., and Pillai, R. S. (2017). Regulation of m(6)A Transcripts by the 3′–>5′ Rna Helicase Ythdc2 Is Essential for a successful meiotic program in the mammalian germline. Mol. Cell 68, 374–387e12. doi: 10.1016/j.molcel.2017.09.021
Wu, B., Su, S., Patil, D. P., Liu, H., Gan, J., Jaffrey, S. R., et al. (2018). Molecular basis for the specific and multivariant recognitions of Rna substrates by human hnrnp A2/B1. Nat. Commun. 9:420. doi: 10.1038/s41467-017-02770-z
Xiang, S., Liu, K., Yan, Z., Zhang, Y., and Sun, Z. (2016a). RnamethPre: A web server for the prediction and query of mrna m6A Sites. PLoS One 11:e0162707. doi: 10.1371/journal.pone.0162707
Xiang, S., Yan, Z., Liu, K., Zhang, Y., and Sun, Z. (2016b). AthMethPre: A web server for the prediction and query of mrna m(6)A sites in Arabidopsis thaliana. Mol. Biosyst. 12, 3333–3337. doi: 10.1039/c6mb00536e
Xiao, W., Adhikari, S., Dahal, U., Chen, Y. S., Hao, Y. J., Sun, B. F., et al. (2016). Nuclear m(6)A reader ythdc1 regulates mrna splicing. Mol. Cell 61, 507–519. doi: 10.1016/j.molcel.2016.01.012
Xing, P., Su, R., Guo, F., and Wei, L. (2017). Identifying N(6)-methyladenosine sites using multi-interval nucleotide pair position specificity and support vector machine. Sci. Rep. 7:46757. doi: 10.1038/srep46757
Xu, C., Liu, K., Ahmed, H., Loppnau, P., Schapira, M., and Min, J. (2015). Structural Basis for the Discriminative Recognition of N6-Methyladenosine Rna by the Human Yt521-B homology domain family of proteins. J. Biol. Chem. 290, 24902–24913. doi: 10.1074/jbc.M115.680389
Xu, C., Wang, X., Liu, K., Roundtree, I. A., Tempel, W., Li, Y., et al. (2014). Structural basis for selective binding of m6A Rna by the Ythdc1 Yth domain. Nat. Chem. Biol. 10, 927–929. doi: 10.1038/nchembio.1654
Xuan, J. J., Sun, W. J., Lin, P. H., Zhou, K. R., Liu, S., Zheng, L. L., et al. (2018). Rmbase v2.0: Deciphering the map of Rna modifications from epitranscriptome sequencing data. Nucleic Acids Res. 46, D327–D334. doi: 10.1093/nar/gkx934
Yang, X., Yang, Y., Sun, B. F., Chen, Y. S., Xu, J. W., Lai, W. Y., et al. (2017a). 5-methylcytosine promotes mrna export - Nsun2 as the methyltransferase and Alyref as an m(5)C reader. Cell Res. 27, 606–625. doi: 10.1038/cr.2017.55
Yang, Y., Hsu, P. J., Chen, Y.-S., and Yang, Y.-G. (2017b). 5-methylcytosine promotes mrna export — Nsun2 as the methyltransferase and Alyref as an m5C reader. Cell Res. 28, 616–624. doi: 10.1038/s41422-018-0040-8
Yao, J. H., Lin, M. X., Liao, W. J., Fan, W. J., Xu, X. X., Shi, H., et al. (2022). M1aregpred: Epitranscriptome target prediction of N1-methyladenosine (m1A) regulators based on sequencing features and genomic features. Front. Biosci. 27:269. doi: 10.31083/j.fbl2709269
Yao, W., Han, X., Ge, M., Chen, C., Xiao, X., Li, H., et al. (2020). N(6)-methyladenosine (m(6)A) methylation in ischemia-reperfusion injury. Cell Death Dis. 11:478. doi: 10.1038/s41419-020-2686-7
Yoon, K. J., Ringeling, F. R., Vissers, C., Jacob, F., Pokrass, M., Jimenez-Cyrus, D., et al. (2017). Temporal control of mammalian cortical neurogenesis by m(6)A METHYLATION. Cell 171, 877–889e17. doi: 10.1016/j.cell.2017.09.003
Yu, J., Chen, M., Huang, H., Zhu, J., Song, H., Zhu, J., et al. (2018). Dynamic m6A modification regulates local translation of mrna in axons. Nucleic Acids Res. 46, 1412–1423. doi: 10.1093/nar/gkx1182
Yu, J., Xing, X., Zeng, L., Sun, J., Li, W., Sun, H., et al. (2012). SyStemCell: A database populated with multiple levels of experimental data from stem cell differentiation research. PLoS One 7:e35230. doi: 10.1371/journal.pone.0035230
Yue, Y., Liu, J., Cui, X., Cao, J., Luo, G., Zhang, Z., et al. (2018). Virma mediates preferential m(6)A mrna methylation in 3′utr and near stop codon and associates with alternative polyadenylation. Cell Discov. 4:10. doi: 10.1038/s41421-018-0019-0
Zhang, J., Feng, P., Lin, H., and Chen, W. (2018a). Identifying Rna N(6)-methyladenosine sites in Escherichia coli Genome. Front. Microbiol. 9:955. doi: 10.3389/fmicb.2018.00955
Zhang, L., Li, G., Li, X., Wang, H., Chen, S., and Liu, H. (2021a). Edlm(6)Apred: Ensemble deep learning approach for mrna m(6)A site prediction. BMC Bioinform. 22:288. doi: 10.1186/s12859-021-04206-4
Zhang, L., Qin, X., Liu, M., Xu, Z., and Liu, G. (2021b). Dnn-m6A: A cross-species method for identifying Rna N6-methyladenosine sites based on deep neural network with multi-information fusion. Genes (Basel) 12:354. doi: 10.3390/genes12030354
Zhang, S., Wang, J., Li, X., and Liang, Y. (2021c). M6A-Gsms: Computational identification of N(6)-methyladenosine sites with Gbdt and stacking learning in multiple species. J. Biomol. Struct. Dyn. 1–12. doi: 10.1080/07391102.2021.1970628
Zhang, M., Sun, J.-W., Liu, Z., Ren, M.-W., Shen, H.-B., and Yu, D.-J. (2016). Improving N6-methyladenosine site prediction with heuristic selection of nucleotide physical–chemical properties. Anal. Biochem. 508, 104–113. doi: 10.1016/j.ab.2016.06.001
Zhang, M., Xu, Y., Li, L., Liu, Z., Yang, X., and Yu, D. J. (2018b). Accurate Rna 5-methylcytosine site prediction based on heuristic physical-chemical properties reduction and classifier ensemble. Anal. Biochem. 550, 41–48. doi: 10.1016/j.ab.2018.03.027
Zhang, S. Y., Zhang, S. W., Fan, X. N., Zhang, T., Meng, J., and Huang, Y. (2019). Fundmdeep-m6A: Identification and prioritization of functional differential m6A methylation genes. Bioinformatics 35, i90–i98. doi: 10.1093/bioinformatics/btz316
Zhang, S., and Shi, H. (2021). iR5hmcsc: Identifying Rna 5-hydroxymethylcytosine with multiple features based on stacking learning. Comput. Biol. Chem. 95:107583. doi: 10.1016/j.compbiolchem.2021.107583
Zhang, W., Tan, X., Lin, S., Gou, Y., Han, C., Zhang, C., et al. (2022). Cplm 4.0: An updated database with rich annotations for protein lysine modifications. Nucleic Acids Res. 50, D451–D459. doi: 10.1093/nar/gkab849
Zhang, Y., and Hamada, M. (2018). DeepM6aseq: Prediction and characterization of m6A-containing sequences using deep learning. BMC Bioinform. 19:524. doi: 10.1186/s12859-018-2516-4
Zhang, Z., Theler, D., Kaminska, K. H., Hiller, M., De La Grange, P., Pudimat, R., et al. (2010). The Yth domain is a novel Rna binding domain. J. Biol. Chem. 285, 14701–14710. doi: 10.1074/jbc.M110.104711
Zhao, B. S., Roundtree, I. A., and He, C. (2017). Post-transcriptional gene regulation by mrna modifications. Nat. Rev. Mol. Cell Biol. 18, 31–42. doi: 10.1038/nrm.2016.132
Zhao, L.-Y., Song, J., Liu, Y., Song, C.-X., and Yi, C. (2020). Mapping the epigenetic modifications of Dna and Rna. Protein Cell 11, 792–808. doi: 10.1007/s13238-020-00733-7
Zhao, T. T., Graber, T. E., Jordan, L. E., Cloutier, M., Lewis, S. M., Goulet, I., et al. (2009). hnrnp A1 regulates Uv-induced Nf-kappaB signalling through destabilization of ciap1 mrna. Cell Death Differ. 16, 244–252. doi: 10.1038/cdd.2008.146
Zhao, X., Yang, Y., Sun, B. F., Shi, Y., Yang, X., Xiao, W., et al. (2014). Fto-dependent demethylation of N6-methyladenosine regulates mrna splicing and is required for adipogenesis. Cell Res. 24, 1403–1419. doi: 10.1038/cr.2014.151
Zhao, Z., Peng, H., Lan, C., Zheng, Y., Fang, L., and Li, J. (2018). Imbalance learning for the prediction of N(6)-Methylation sites in mrnas. BMC Genomics 19:574. doi: 10.1186/s12864-018-4928-y
Zhen, D., Wu, Y., Zhang, Y., Chen, K., Song, B., Xu, H., et al. (2020). m(6)A Reader: Epitranscriptome target prediction and functional characterization of N (6)-Methyladenosine (m(6)A) Readers. Front. Cell Dev. Biol. 8:741. doi: 10.3389/fcell.2020.00741
Zheng, G., Dahl, J. A., Niu, Y., Fedorcsak, P., Huang, C. M., Li, C. J., et al. (2013). Alkbh5 is a mammalian Rna demethylase that impacts Rna metabolism and mouse fertility. Mol. Cell 49, 18–29. doi: 10.1016/j.molcel.2012.10.015
Zheng, Y., Nie, P., Peng, D., He, Z., Liu, M., Xie, Y., et al. (2018). m6avar: A database of functional variants involved in m6A modification. Nucleic Acids Res. 46, D139–D145. doi: 10.1093/nar/gkx895
Zhong, S., Li, H., Bodi, Z., Button, J., Vespa, L., Herzog, M., et al. (2008). Mta is an Arabidopsis messenger Rna adenosine methylase and interacts with a homolog of a sex-specific splicing factor. Plant Cell 20, 1278–1288. doi: 10.1105/tpc.108.058883
Zhou, Q., Guan, P., Zhu, Z., Cheng, S., Zhou, C., Wang, H., et al. (2022). Asmdb: A comprehensive database for allele-specific Dna methylation in diverse organisms. Nucleic Acids Res. 50, D60–D71. doi: 10.1093/nar/gkab937
Zhou, Y., Zeng, P., Li, Y. H., Zhang, Z., and Cui, Q. (2016). Sramp: Prediction of mammalian N6-methyladenosine (m6A) sites based on sequence-derived features. Nucleic Acids Res. 44:e91. doi: 10.1093/nar/gkw104
Zhu, T., Roundtree, I. A., Wang, P., Wang, X., Wang, L., Sun, C., et al. (2014). Crystal structure of the Yth domain of Ythdf2 reveals mechanism for recognition of N6-methyladenosine. Cell Res. 24, 1493–1496. doi: 10.1038/cr.2014.152
Zhu, Z. M., Huo, F. C., and Pei, D. S. (2020). Function and evolution of Rna N6-methyladenosine modification. Int. J. Biol. Sci. 16, 1929–1940. doi: 10.7150/ijbs.45231
Zhuang, J., Liu, D., Lin, M., Qiu, W., Liu, J., and Chen, S. (2021). PseUdeep: Rna Pseudouridine site identification with deep learning algorithm. Front. Genet. 12:773882. doi: 10.3389/fgene.2021.773882
Zhuang, Y. Y., Liu, H. J., Song, X., Ju, Y., and Peng, H. (2019). A linear regression predictor for identifying N(6)-methyladenosine sites using frequent gapped K-mer pattern. Mol. Ther. Nucleic Acids 18, 673–680. doi: 10.1016/j.omtn.2019.10.001
Keywords: RNA methylation, N6-methyladenosine, 5-methylcytosine, 5-hydroxymethylcytosine, N1-methyladenosine, pseudouridine
Citation: Zou J, Liu H, Tan W, Chen Y-q, Dong J, Bai S-y, Wu Z-x and Zeng Y (2022) Dynamic regulation and key roles of ribonucleic acid methylation. Front. Cell. Neurosci. 16:1058083. doi: 10.3389/fncel.2022.1058083
Received: 30 September 2022; Accepted: 28 November 2022;
Published: 19 December 2022.
Edited by:
Mario Gomes-Pereira, Institut National de la Santé et de la Recherche Médicale (INSERM), FranceReviewed by:
Bowen Song, University of Liverpool, United KingdomKil To Chong, Jeonbuk National University, South Korea
Copyright © 2022 Zou, Liu, Tan, Chen, Dong, Bai, Wu and Zeng. This is an open-access article distributed under the terms of the Creative Commons Attribution License (CC BY). The use, distribution or reproduction in other forums is permitted, provided the original author(s) and the copyright owner(s) are credited and that the original publication in this journal is cited, in accordance with accepted academic practice. No use, distribution or reproduction is permitted which does not comply with these terms.
*Correspondence: Yan Zeng, emVuZ3lhbjY4QHd1c3QuZWR1LmNu
†These authors share first authorship