- 1Department of Cognitive Neuroscience, Donders Institute for Brain, Cognition and Behaviour, Radboud University Medical Centre, Nijmegen, Netherlands
- 2Karakter Child and Adolescent Psychiatry University Center, Nijmegen, Netherlands
- 3Center of Neurodevelopmental Disorders (KIND), Centre for Psychiatry Research, Department of Women's and Children's Health, Karolinska Institutet, Solna, Sweden
- 4Child and Adolescent Psychiatry, Stockholm Health Care Services, Stockholm, Sweden
- 5Curtin Autism Research Group, School of Occupational Therapy, Social Work and Speech Pathology, Curtin University, Perth, WA, Australia
- 6Department of Child and Adolescent Psychiatry and Psychotherapy, Central Institute of Mental Health, Medical Faculty Mannheim/Heidelberg University, Mannheim, Germany
- 7Department of Child and Adolescent Psychiatry and Psychotherapy, Psychiatric Hospital, University of Zurich, Zurich, Switzerland
- 8Department of Psychiatry, Hospital de Clinicas de Porto Alegre, Federal University of Rio Grande do Sul, Porto Alegre, Brazil
- 9National Institute of Developmental Psychiatry for Children and Adolescents, São Paulo, Brazil
- 10Centre for Innovation in Mental Health, Academic Unit of Psychology, Faculty of Environmental and Life Sciences, University of Southampton, Southampton, United Kingdom
- 11Clinical and Experimental Sciences (CNS and Psychiatry), Faculty of Medicine, University of Southampton, Southampton, United Kingdom
- 12Solent National Health System Trust, Southampton, United Kingdom
- 13Hassenfeld Children's Hospital at NYU Langone, New York University Child Study Center, New York, NY, United States
- 14Division of Psychiatry and Applied Psychology, School of Medicine, University of Nottingham, Nottingham, United Kingdom
- 15Departments of Paediatrics and Psychiatry, Royal Children's Hospital, University of Melbourne, Melbourne, VIC, Australia
- 16Departments of Psychiatry, Neuroscience and Physiology, SUNY Upstate Medical University, Syracuse, New York, NY, United States
- 17Departments of Human Genetics and Psychiatry, Donders Institute for Brain, Cognition and Behaviour, Radboud University Medical Center, Nijmegen, Netherlands
- 18Medice Arzneimittel Pütter GmbH & Co. KG, Iserlohn, Germany
- 19Department of Cognitive Neuroscience, Donders Institute for Brain, Cognition and Behaviour, Radboud University Medical Center, Nijmegen, Netherlands
- 20King's College London, Social, Genetic and Developmental Psychiatry Centre, Institute of Psychiatry, Psychology and Neuroscience, London, United Kingdom
- 21Amsterdam University Medical Center, Location VUMc, Amsterdam, Netherlands
- 22PsyQ, Expertise Center Adult ADHD, The Hague, Netherlands
- 23Department of Psychiatry, Donders Institute for Brain, Cognition and Behaviour, Radboud University Medical Center, Nijmegen, Netherlands
- 24Department of Psychiatry, Icahn School of Medicine at Mount Sinai, New York, NY, United States
- 25Department of Psychiatry, Faculdade de Medicina FMUSP, Universidade de São Paulo, São Paulo, Brazil
- 26ADHD Outpatient Program and Developmental Psychiatry Program, Hospital de Clinica de Porto Alegre, Federal University of Rio Grande do Sul, Porto Alegre, Brazil
- 27Department of Child and Adolescent Psychiatry, Institute of Psychiatry, Psychology and Neuroscience, King's College London, United Kingdom
- 28Department of Psychiatry and Behavioral Sciences, Seattle, WA, United States
- 29Department of Public Health and Pediatric Sciences, Section of Child and Adolescent Neuropsychiatry, University of Turin, Turin, Italy
- 30Department of Public Health, Johns Hopkins University, Baltimore, MA, United States
- 31GuzelGunler Clinic, Istanbul, Turkey
- 32Yale Child Study Center, New Haven, CT, United States
- 33Institute for Forensic Psychology and Psychiatry, Neurocenter, Saarland, Germany
- 34Department of Child and Adolescent Psychiatry, Psychosomatics and Psychotherapy, Medical Faculty of the University of Cologne, Cologne, Germany
- 35School for Child and Adolescent Cognitive Behavioural Therapy, University Hospital of Cologne, Cologne, Germany
Attention-Deficit Hyperactivity Disorder (ADHD) is a complex and heterogeneous neurodevelopmental condition for which curative treatments are lacking. Whilst pharmacological treatments are generally effective and safe, there is considerable inter-individual variability among patients regarding treatment response, required dose, and tolerability. Many of the non-pharmacological treatments, which are preferred to drug-treatment by some patients, either lack efficacy for core symptoms or are associated with small effect sizes. No evidence-based decision tools are currently available to allocate pharmacological or psychosocial treatments based on the patient's clinical, environmental, cognitive, genetic, or biological characteristics. We systematically reviewed potential biomarkers that may help in diagnosing ADHD and/or stratifying ADHD into more homogeneous subgroups and/or predict clinical course, treatment response, and long-term outcome across the lifespan. Most work involved exploratory studies with cognitive, actigraphic and EEG diagnostic markers to predict ADHD, along with relatively few studies exploring markers to subtype ADHD and predict response to treatment. There is a critical need for multisite prospective carefully designed experimentally controlled or observational studies to identify biomarkers that index inter-individual variability and/or predict treatment response.
Introduction
Since the earliest times in medicine, doctors and patients have faced key questions, such as which signs and symptoms contribute to establishing a diagnosis, which treatment is likely to be the most effective and least harmful, which course of illness to expect, and if there will be full recovery? Throughout history, answers have been sought and provided through a mixture of natural, spiritual and moral perspectives. Only in the last decades have these questions been addressed by applying systematic measurement of mostly physical, neurocognitive, and behavioral symptoms. The subsequent development of laboratory and imaging techniques to analyze and visualize the interior of the body and the brain have further revolutionized clinical medicine. Today, we are at the transition to an additional level of medical insight known as precision, stratification, or personalized medicine (PM). This perspective paper aims to describe the status quo and path toward PM for Attention-Deficit Hyperactivity Disorder (ADHD).
Precision Medicine
Medicine in the consultation room or at the bedside is directed at an individual patient and thereby per se individualized. In a more narrow sense, PM aims to offer evidence-based tailored assessment and treatment to each patient and thereby is an advancing field of healthcare that is informed by each person's unique clinical, genetic/genomic, biomarker, and environmental information (Ginsburg and Willard, 2009). These unique personal characteristics are the basis for treatment, prediction, and prevention. The ambitions of PM therefore go beyond the provision of optimal clinical care and extend to population medicine, screening of high-risk individuals, prevention and personal lifestyle recommendations. In this way, PM forms an integrated, coordinated, evidence-based approach across the continuum from health to disease (Ginsburg and Willard, 2009; Jameson and Longo, 2015). Some prefer the term precision or stratification medicine instead of PM because ultimately recommendations and decisions in clinical practice will be targeted to well defined groups of patients with very similar clinical, genetic, or biomarker profiles rather than be directed at the N = 1 level.
The goals of PM can be achieved in several ways. The use of diagnostic markers may lead to improved diagnostic accuracy and reduce misclassification, thereby reducing medical shopping, delivering of unnecessary or harmful treatments, and optimizing the use of limited resources. Treatments targeted at the individual level are intended to produce higher response rates, fewer side effects and/or faster responses to treatment and speed up well-being and reduce functional impairment. Successful examples of PM are currently most commonly found in oncology and internal medicine (Hamburg and Collins, 2010; Jameson and Longo, 2015).
However, PM for psychiatric disorders faces several challenges. The most important of these is heterogeneity which is manifest at several levels in psychiatric disorders: phenotypically, with comorbidity being the rule rather than the exception, and aetiologically, with a complex genetic architecture based on different types of genetic variants and gene-environment interplay (Geschwind and Flint, 2015; Boyle et al., 2017), and diverse brain alterations (Faraone et al., 2015). There is no stable, agreed upon, and biologically valid concept of any psychiatric disorder (Kapur et al., 2012), which makes the current taxonomy an unclear basis for informed biological research. Attempts to define more biologically homogeneous subgroups (“biotypes”) (Drysdale et al., 2017) or pathophysiological dimensions of psychiatric disorders are under way (Cuthbert, 2014), but still have to deliver (Wium-Andersen et al., 2017).
Biomarkers
The success of PM depends on identifying biomarkers, especially stratification biomarkers. A biomarker measures the presence or severity of a condition, its biological subtypes (also called biotypes), course or treatment response; it can be a physical, chemical, biologic, genetic, cognitive, psychological or environmental measure (see Table 1) (Drucker and Krapfenbauer, 2013). Unfortunately, many attempts to identify, validate and implement biomarkers have failed, for example because of lack of (adherence to) rigorous standardized operating procedures, small sample sizes and failures of replication (Drucker and Krapfenbauer, 2013). To be implementable in the clinic, a potential biomarker should be validated across independent data sets, and be reproducible, reliable, specific, sensitive, clinically relevant and feasible (Drucker and Krapfenbauer, 2013; Fusar-Poli et al., 2018).
Developing and validating biomarkers requires high-quality study designs. Biomarker discovery may start with retrospective analysis of randomized controlled trial (RCT) datasets for moderator effects. However, most such retrospective analyses are underpowered and/or lack a hypothesis; which is why findings need to be confirmed in prospective designs. The field of oncology has generated advanced designs for testing biomarkers (Freidlin and Korn, 2014). Examples are enrichment designs, in which individuals with a positive biomarker are oversampled and/or biomarker-positive and -negative individuals are balanced; next only biomarker-positive individuals are randomized to a new vs. a standard treatment, or biomarker-positive and biomarker-negative individuals are randomized in a stratified way to new vs. standard treatment. In the latter case, the investigators can be blinded as to the biomarker status of the participants (Mandrekar and Sargent, 2009).
ADHD
ADHD is a common neurodevelopmental disorder of childhood and has a worldwide prevalence of around 5% (Polanczyk et al., 2007). Persistence into adulthood – either as a full clinical diagnosis or as a subthreshold condition with residual functional impairment is frequent, and adult ADHD has a worldwide prevalence of 2.8% (Fayyad et al., 2017). The categorical diagnosis of ADHD as defined by the DSM-5 (APA, 2013) and ICD-10 (WHO) (WHO, 1992) is based on the presence of pervasive, persistent symptoms of inattention and/or hyperactivity/impulsivity that typically arise early in life and result in significant functional impairment. Current approaches to subtyping in ADHD are limited. The current definition distinguishes three subtypes or presentations, inattentive, hyperactive/impulsive and combined. However, these subtypes appear to be far from stable, and transitions between them are frequent and common (Lahey et al., 2005). A more recent, but equally contentious subgrouping approach for ADHD focuses on age of onset. Contrary to existing definitions which describe ADHD as presenting early in life, there may be a group of individuals in which onset is delayed until adolescence or young adulthood (Asherson et al., 2016; Cooper et al., 2018; Sibley et al., 2018; Asherson and Agnew-Blais, 2019; Breda et al., 2021; Ilbegi et al., 2021; Riglin et al., 2022). However, studies are not consistent as to whether and to what extent late-onset ADHD cases are due to misdiagnosis or underdiagnosis of childhood ADHD symptoms, strong supportive environments in childhood, can be better explained by other (comorbid) disorders, are due to change of rater over time, or are indeed true late-onset cases (Taylor et al., 2021). It is also unclear whether early and late onset ADHD reflect a different balance of genetic and environmental risk factors and share the same underlying neural mechanisms (Asherson and Agnew-Blais, 2019). Pending further study, this could suggest age of onset as a further method for subgrouping patients with ADHD.
There are several critical clinical decision points that may present potential targets for a PM approach to ADHD. The most obvious is predicting treatment response. At present, medication is recognized as the most effective treatment for ADHD (NICE, 2018). However, many clinicians and families prefer to start treatment with non-pharmacological interventions to avoid the potential adverse effects of medication. Unfortunately, meta-analyses have demonstrated limited efficacy for most non-pharmacological treatments by reporting small to modest effect sizes for reducing core ADHD symptoms (Sonuga-Barke et al., 2013; Daley et al., 2014; Cortese et al., 2015, 2016; Oliva et al., 2021; Yang et al., 2021; Groenman et al., 2022). Stronger effects were found on cognitive measures, with modest to large effect sizes for the impact of physical exercise on inhibition and cognitive flexibility (Lambez et al., 2020; Liang et al., 2021). The Achilles heel of most non-pharmacological intervention studies is the lack of blinded raters, lack of an active/sham control group and, even when outcome measures are based on objective laboratory tests, not blinding researchers during data collection and data analysis. Few if any studies have investigated whether there are subgroups of those with ADHD who respond robustly to these treatments.
The main medication treatments for ADHD [methylphenidate (MPH), amphetamines, lisdexamphetamine, atomoxetine (ATX), guanfacine] are associated with moderate to large effect sizes across multiple trials in children older than 6; however, not every individual with ADHD responds well to every medication (Hodgkins et al., 2012), 10–20% of patients do not respond to medication at all, and there is considerable inter-individual variability in tolerability and important adverse effects such as growth problems and hypertension (Faraone et al., 2008). There is currently no clear evidence to guide decision making about who will respond best to and/or tolerate which treatment approach. Further, it is currently not possible to predict course or outcome at the level of the individual patient. It would, for example, be of considerable clinical interest to predict which hyperactive toddlers go on to have full-blown ADHD at school entry, which of those school-age children with ADHD will continue to have problems in adolescence and adulthood, and who will develop which comorbidities and problematic functional outcomes (Caye et al., 2016). Further, while some individuals with subthreshold ADHD symptoms will develop a full clinical syndrome and some may require early treatment, we have no predictors of which subthreshold cases require intensive monitoring and/or treatment (Biederman et al., 2018).
Systematic Search
A systematic review was undertaken of randomized controlled trials (RCTs) or non randomized studies exploring cognitive, physiological (EEG/ERP), MRI, genetic and clinical measures that may serve as biomarkers to predict diagnosis, stratify ADHD into more homogenous subgroups and/or predict response to treatment and/or predict course (see Supplementary Materials for details of the protocol and search strategy). The search led to the identification of 71 papers, of which 65 were retained after checks for reporting sufficient statistical information. Manual search through PubMed and review papers led to identify another 7 relevant papers. The vast majority of papers reported on EEG (11), cognitive/actigraphic (26) and MRI-based (18) diagnostic markers. Fewer papers described markers to predict response to treatment (11, of which 5 EEG/ERP studies, 3 cognition, 1 NIRS, 1 SPECT and 1 MRI) and biologically subtype ADHD (6) (see Table 2 for a summary of the diagnostic marker studies, and Table 3 for a summary of the other marker studies). The studies are also discussed in the text sections below.
The next sections incorporate the results of the search and provide short state-of-the-art summaries of what is known regarding markers for diagnosis and treatment selection in ADHD.
Clinical Aspects
Inattention and hyperactivity/impulsivity symptoms follow specific developmental courses and have different relationships to co-occurring disorders. The data are mixed as to whether the nature of presenting symptoms predicts differential response to treatment with medication (Solanto et al., 2009). Severity of ADHD symptoms and associated oppositional defiant disorder or conduct disorder may be associated with worse response to treatment (Chazan et al., 2011). Comorbid anxiety and depressive disorders are not associated with reduced effects of stimulants on ADHD symptoms (Gadow et al., 2002). Children with associated tic disorders or autism spectrum disorder (ASD) also have a favorable response to stimulants (Bloch et al., 2009; Reichow et al., 2013). However, the reduction of ADHD symptoms in children with tic disorders and ASD is generally smaller than reported for ADHD without tics or ASD (Cortese et al., 2018), and emergence of tics and adverse events are more common in these populations (Bloch et al., 2009; Reichow et al., 2013). Comorbid bipolar disorder (BD) and substance use disorder (SUD) are associated with increased severity of ADHD and generally of poorer prognosis (Goldstein et al., 2017; Luo and Levin, 2017). The presence of these disorders does not preclude the use of stimulants, but when offered, stimulant treatment should be preceded by mood stabilizers for those with BD and assessment of abuse liability and drug interactions for those with SUD. In adults with ADHD, comorbidity with personality disorders is associated with a lower response rate to stimulants and a more complex clinical course (Robison et al., 2010). Subtyping of ADHD by interindividual differences in temperament and in particular by emotional regulation/dysregulation has been proposed as a promising way to parse the disorder's clinical and cognitive heterogeneity and develop models to predict treatment response and course (Karalunas et al., 2014, 2019; Nigg et al., 2020).
Genetics and Pharmacogenetics
ADHD is a highly heritable disorder with a complex genetic architecture (Faraone and Larsson, 2019; Brikell et al., 2021). Most cases seem to be due to multiple genetic variants (of mostly limited effect size), in interaction with environmental risk factors. Given this polygenic nature of ADHD, use of genetic testing is still limited in diagnostic assessments of ADHD. However, recent evidence suggesting a role for more penetrant rare genetic mutations in a subgroup of patients (Demontis et al., 2016; Kim et al., 2017; Ganna et al., 2018) may lead to an increased use of genetic diagnostic testing. If their accuracy can be dramatically improved, polygenic risk scores (PRS) may become important for polygenic ADHD cases, since PRS have been shown to predict symptom severity and comorbidity in ADHD (Hamshere et al., 2013; Stergiakouli et al., 2015; Ronald et al., 2021) and are informative regarding symptom persistence (Riglin et al., 2016; Agnew-Blais et al., 2021). Epigenetic profiling of a set of ADHD risk genes was claimed to predict ADHD among Chinese children with 93% accuracy but this has not been replicated (Xu et al., 2015).
Pharmacogenetics is the study of genetic variability in medication response. Numerous studies of stimulant response have been conducted, primarily with genes related to catecholamine functions (Froehlich et al., 2010). Effect sizes have been small, and few consistent findings have been reported (Hart et al., 2013; Myer et al., 2017). Given the poor adherence to ADHD medications, perhaps a more appropriate target for pharmacogenetic studies is tolerability (Joensen et al., 2017). Despite a paucity of studies and large between-study heterogeneity, associations have been found between adverse events and several genetic variants (see Table 4). Indeed, maintenance of effects and tolerability are key-drivers of long-term outcome and may be more salient targets for genetic studies than acute symptom reduction. Since the majority of common adverse events are dose-related, pharmacogenetic studies associated with inter-individual variability in drug metabolism hold considerable promise, such as CYP2D6 for amphetamines and ATX (Bach et al., 1999; Yu et al., 2016), and Carboxylesterase-1 gene (CES1) which is the sole enzyme involved in MPH metabolism (Stage et al., 2017). A regularly updated database of pharmacogenetic findings can be accessed via the Clinical Pharmacogenetics Implementation Consortium: https://cpicpgx.org/.
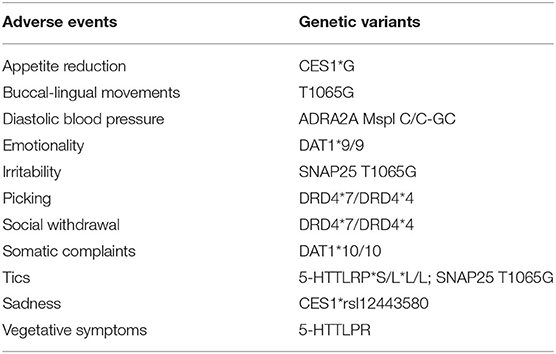
Table 4. Associations between adverse events to ADHD medication and genetic variants (Joensen et al., 2017).
Metabolomics and Neurochemistry
A meta-analysis reviewed 71 studies of metabolites in monoaminergic transmission pathways, 18 studies of heavy metals, 16 studies of substance or chemical exposures, 29 studies of trace elements, 24 studies of essential fatty acids, 22 studies of hypothalamic pituitary axis pathway metabolites, 15 studies of growth and thyroid function, 7 studies of oxidative stress, and 3 studies of cytokine imbalance (Scassellati et al., 2012). Because the lack of availability of brain tissue in ADHD, these were all studies of peripheral samples: plasma, serum, urine, saliva, blood, platelets, red blood cells, cerebrospinal fluid, and hair. Of all biomarkers meta-analyzed, eight were statistically significantly associated with ADHD after correcting for multiple comparisons: norepinephrine (NE) in urine, 3-methoxy-4-hydroxyphenylethylene glycol (MHPG) in urine, monoamine oxidase (MAO) in platelets, normetanephrine (NM) in urine, metanephrine (MET) in urine, ferritin (FE) in serum, zinc (ZN) in serum/plasma/urine, and cortisol in saliva. NE, MHPG, MAO and cortisol were also associated with drug response and ADHD symptom severity. Given the consistency of findings for NE, MHPG and MAO, it is possible that lower MAO activity interferes with the degradation of NE leading to lower levels of MHPG. Urinary “MAO-NE-MHPG” levels could provide a biochemical marker profile for ADHD (Scassellati et al., 2012). Yet, because prior studies of these biomarkers have focused on group effects rather than on diagnostic accuracy and individual prediction, more work is needed to define a valid and accurate biomarker profile from this triad. A study observed serum levels of sphingomyelins to be significantly lower in ADHD patients compared to controls with a separation at the individual level with 79% sensitivity and 78% specificity (Henríquez-Henríquez et al., 2015).
Neurophysiology
Electroencephalogram (EEG) markers such as theta and beta oscillations at rest reflecting arousal, alertness, orienting and activation (Clarke et al., 2013; McLoughlin et al., 2022) and task-related cognitive event-related potentials (ERPs) reflecting preparation (contingent negative variation, CNV), attention and inhibition (earlier P100, N100, P200, N200 and later P300 components) reveal moderate deviations mainly for later ERPs and heterogeneity in ADHD at the level of functional brain systems (Kaiser et al., 2020). Consistent subgroups based on these EEG markers include clusters showing increased theta and reduced beta activity, increased slower activity resembling what is seen in developmental delay, or increased beta activity; fewer consistent subgroups were reported for ERPs (Barry et al., 2003). These markers partly cluster independently of clinical DSM-based subtypes and comorbid diagnostic categories (Mazaheri et al., 2014).
The search identified 11 studies that used EEG-based measures and in particular the power of the frequency bands and the ratio of the theta to beta power (TBR) to predict a diagnosis of ADHD in case-control designs (see Table 2). TBR was initially hypothesized to be a measure of central arousal; later, it was reconsidered to be more an index of cognitive processing and executive control (Clarke et al., 2019). The EEG diagnostic work however suffers from a lack of replication in independent large samples and standardization of data collection (eye open vs. eyes closed condition) (Clarke et al., 2020). Further, the results of the various studies differ but, in terms of sensitivity and specificity, mostly fall behind criteria for application as clinical test (accuracy > 0.90). In terms of subtyping ADHD, cluster analysis of resting-state EEG data showed three clusters (maturational lag, hypoarousal, and excess beta-hyperarousal) (Magee et al., 2005) but differences in event-related potentials (ERPs) between these clusters on a separate odd-ball task were only minimal (Brown et al., 2005).
Longitudinal work has identified consistent neurophysiological patterns related to differential outcomes. Children with ADHD persisting into adulthood show increased beta and reduced frontal theta EEG at rest (Clarke et al., 2011), and ERP markers for reduced cognitive preparation and error processing (Doehnert et al., 2013; Cheung et al., 2016; Michelini et al., 2016).
Studies predicting treatment response using EEG-based markers have focused on neurofeedback and medication. Neurofeedback studies indicate that increased theta predicts response to theta-modulating neurofeedback (Gevensleben et al., 2009; Janssen et al., 2016), and parietal alpha plus a strong CNV reflecting cognitive preparation together explain nearly 30% of the variance in slow cortical potential neurofeedback outcome. Recent work describes that a high individual peak frequency (iAPF) of the EEG is associated with higher remissions rates both to neurofeedback and medication treatment (Voetterl et al., 2022). Medication studies report that increased theta and absence of frontal slowing is associated with better stimulant response in some (Chabot et al., 1999; Clarke et al., 2002; Arns et al., 2008) but not all (Janssen et al., 2016) studies. Changes of the P3b component of the ERP after administration of a single dose of MPH separated medication responders and non-responders with 81% accuracy (Young et al., 1995). In the largest study to date, theta/beta ratio did not predict MPH response, and frontal alpha slowing predicted non-response only in a male adolescent subgroup (Arns et al., 2018). The response to ATX was predicted by stronger cognitive N2 activity (Griffiths et al., 2017). The EEG and ERP markers predicting pharmacological and non-pharmacological treatment response partly overlap. However, given the limitations of retrospective analyses of treatment response, independent and large controlled prospective multicenter studies with guideline based diagnosis and treatment are needed to clarify the promise of EEG based marker for PM in ADHD (Dopfner et al., 2017).
Neurocognition
Many studies have used the omission and commission error scores of a Continuous Performance Task (CPT) often in combination with actigraphy or movement assessments to index the inattention, impulsivity and hyperactivity symptom domain to develop cognitive diagnostic markers predicting ADHD (Table 2). Other measures used are tests of working memory, attention, other executive functioning tests, eye vergence to index visual orienting, reaction time characteristics, and quality of handwriting evaluated by a blinded graphologist (Table 2). Cognitive tests have also been explored for differentiating “true ADHD” from feigned ADHD and malingering (Quinn, 2003; Berger et al., 2021). Predictive statistics vary and for a couple of studies comes close to being clinically relevant. Stringent replication in independent samples however is lacking. A diagnostic randomized single-blind study showed that the Qbtest (a combination of CPT and movement analysis by an infrared measurement system) as an add-on to the standard clinical assessment approach did improve clinical decision-making and was associated with faster diagnostic decisions, a shorter clinical consultation time and higher clinician confidence in the diagnosis, compared to standard clinical assessment only (Hollis et al., 2018).
Psychological factors such as compliance, suggestibility, motivation, coping strategies, and cognitive capacities are all important predictors of treatment success and should be considered when taking a whole person approach to PM (Cutica et al., 2014). Several approaches to stratification of ADHD with the aim of producing more homogenous neurocognitive subgroups have been described ADHD (Fair et al., 2012; Coghill et al., 2014; van Hulst et al., 2015; Leikauf et al., 2017; Mostert et al., 2018). The most ambitious study applied unsupervised machine learning on a battery of cognitive tests in 116 children with ADHD and identified two biotypes that were validated by EEG and ECG measures and differential response to medication. One biotype was labeled impulsive cognition and characterized by high rates of commission errors and shorter reaction time, greater EEG slow wave (theta/delta) power and greater resting heart rate. The second biotype was labeled inattentive cognition and was marked by high rates of omission errors, longer and more variable reaction time and lower EEG fast wave (beta) power. The biotypes did not differentially predict the clinical response to atomoxetine, but only within the inattentive cognitive biotype a strong correlation was observed between change in ADHD symptoms and change in cognitive measures such as verbal memory immediate recall (Leikauf et al., 2017). This approach is informative for PM approaches to predict treatment response for both medication and non-pharmacological (cognitive) interventions. Further research could lead to matching specific cognitive and educational interventions to particular cognitive deficits (van der Donk et al., 2013). Moreover, neurocognitive predictors of longitudinal course and outcome in ADHD may give hints to PM approaches. A study investigated the predictive value for ADHD course and severity of eight cognitive domains; the authors reported better baseline working memory to be correlated with less ADHD severity at follow up 6 years later, whilst reduced reaction time variability was associated with better global functioning (van Lieshout et al., 2017).
Studies on the prediction of treatment response from neurocognitive measures are limited. Performance on an oddball and working memory test (Hermens et al., 2005) and variability of response time (Lee et al., 2009) did separate responders from non-responders to stimulants with about 85 and 70% accuracy respectively. Machine learning analyses on a large set of pre-treatment measures learned that the performance on the Stroop test together with information on candidate genes polymorphisms and clinical characteristics predicted stimulant response with 84.6% accuracy (Kim et al., 2015). Performance on a delayed matching to sample task (Coghill et al., 2007) and on the go/no go task were significantly associated with MPH response (Johnston et al., 2015). For future research, it will be important to integrate neurocognitive data with other biomarkers across the different levels of analysis. Further, it is important to focus on neurocognitive tasks that are conceptualized from a neuroscience perspective, and have shown to be dependent on particular neuroanatomical, and neurophysiological substrates, such as the CANTAB battery of tasks (Fried et al., 2015).
Brain Imaging
Many studies have attempted to predict ADHD diagnoses from neuroimaging data, with some claiming high accuracy (see Table 2). Most studies performed various sorts of machine learning using features extracted from resting-state MRI, structural MRI or a combination of these, while one used task-based MRI, one the hemodynamic response assessed by fNIRS, and another a combination of PET-scan imaging of the serotonin-transporter and serotonergic genes. Methodological limitations such as mostly small sample sizes and lack of out of sample validation lead to adjust these claims (Wolfers et al., 2015). The largest predictive study derives from the ENIGMA ADHD consortium's structural MRI data and was able to separate ADHD from non-ADHD individuals at the group level by machine learning, but not with sufficient specificity to be considered clinically useful for individual prediction (Zhang-James et al., 2021).
Few studies have examined predictors of treatment response from brain imaging data. Resting-state regional blood flow measured by SPECT pre-treatment (Cho et al., 2007) and by hemodynamic response to single doses of stimulants assessed by NIRS (Ishii-Takahashi et al., 2014) were associated with the response to stimulants. Robust improvement with MPH was associated with greater reduction in dopamine transporter (DAT) density in putamen, measured by Tc-99m] TRODAT-1SPECT (Akay et al., 2018). Other research used imaging together with genotype and clinical/demographic factors to determine the brain basis of improvement with MPH treatment; in younger children and lower treatment levels, carriers of the DRD4 7R allele showed decreased frontal cortex volumes - suggesting that youth with the DRD4 7R genotype might be sensitive to cortical remodeling after low dose stimulant treatment (Schweren et al., 2016). Another study examined the activation profiles of fMRI scans obtained while performing an inhibitory control task off treatment to predict response to treatment with MPH and ATX (Schulz et al., 2017). Improvement with ATX was predicted by relatively higher activation in motor cortex off medication, while superior response to MPH over ATX was predicted by elevated caudate activation (Schulz et al., 2017). A longitudinal study of resting-state connectivity and response to stimulant treatment found that decreasing connectivity within the cingulo-opercular network with increasing age was associated with a better treatment response to stimulants (Norman et al., 2021).
Another application of the PM approach has used structural MRI to characterize ADHD symptom profiles and/or to predict persistence vs. remission over time. In longitudinal designs, persistent ADHD relative to remitting ADHD was associated with a thinner cortex in the medial occipital cortex, insula, parahippocampus, and prefrontal regions (Proal et al., 2011). Adults with persistent ADHD were characterized by greater cortical thinning in the medial and dorsolateral prefrontal cortex; moreover, the rate of cortical thinning was correlated with the number of inattention symptoms (Shaw et al., 2013). Loss of cerebellar volume over time was further associated with worse outcome (Mackie et al., 2007). A study using longitudinal voxel-based morphometry found that low volume in fronto-cerebellar brain regions involved in cognitive control and larger amygdala volume were associated with persistence of ADHD, while low volume in the visual and auditory cortices was associated with remission over time (Adamo, 2018).
Given the variability in phenotypic expression across individuals with ADHD, imaging studies may have their greatest utility for determining biomarkers of subgroups within the larger ADHD population, which may have predictive value in relation to course, differential response and tolerability, and possibly even dose. Three studies have made a start by using resting-state MRI data to biologically subtype ADHD using different processing and data analytic pipelines (Gates et al., 2014; Costa Dias et al., 2015; Lin et al., 2018). Further work in this direction including out of sample validation is needed.
Environmental Factors
Multiple indicators of psychosocial adversity, including family adversity, low income, and low socioeconomic status are associated with ADHD (Dopfner et al., 2008; Thapar et al., 2013; Russell et al., 2016). Maternal nicotine and alcohol use during pregnancy and exposure to pesticides and lead may influence the development of ADHD symptoms (Linnet et al., 2003; Sciberras et al., 2017). However, many of these environmental effects are not specific to ADHD and/or may disappear when genetic influences are controlled for (Rice et al., 2018). Extreme forms of early deprivation can result in ADHD-type symptoms (Rutter et al., 2007; Kennedy et al., 2016). Studies examining the influence of psychosocial adversity on pharmacological, psychosocial or combined treatment have produced unclear results (Handen et al., 1994; Thomson and Varley, 1998). Single-parent status, low income, and education are associated with a poorer behavioral treatment response in children with ADHD, lower adherence, and higher attrition for treatments (Firestone and Witt, 1982). Poor adherence to pharmacological treatment is predicted by high maternal psychological distress, indifferent parenting, maternal overprotection/control, poor family support, decreased interaction with parents, and increased problems at home (Gau et al., 2006).
Moderator analyses conducted in the Multimodal Treatment Study of ADHD (MTA) showed that, for families from the lowest socioeconomic strata, who required welfare support, only Combined Treatment of medication and psychosocial intervention yielded meaningful benefits with respect to the outcome of teacher-reported social skills (MTA Cooperative Group, 1999). For children receiving either medication or combined treatment, caregiver symptoms of depression predicted worse outcome than for those with less-depressed parents (Owens et al., 2003; Hinshaw, 2007). In behavioral interventions for children with ADHD, parenting self-efficacy plays a moderating role in decreasing comorbid behavioral problems, but not in decreasing ADHD symptoms (Hinshaw, 2007). In behavioral interventions for children with ADHD parenting self-efficacy plays a moderating role in decreasing comorbid behavioral problems, but not in decreasing ADHD symptoms (van den Hoofdakker et al., 2010).
Sex
ADHD is more common in boys than girls, and the male preponderance is higher for hyperactivity/-impulsivity than for inattentiveness (Greven et al., 2018). In adulthood, the sex ratio is more even (Greven et al., 2018). It is unclear, whether course and long-term prognosis differ between boys and girls with ADHD. Although sex is an important aspect in the clinical manifestation of ADHD, research on PM according to sex is relatively immature (Greven et al., 2018). Sex-specific aspects of treatment include a focus on sex-specific comorbidities as well as treatment response. For example, adolescent girls with ADHD have increased risk for early sexual activity, sexually transmitted diseases and teenage pregnancy, compared to girls without ADHD (Heerde et al., 2015; Snyder, 2015). Up to now, it appears that sex-specific factors do not play a strong role in the pharmacological and non-pharmacological treatment of ADHD. The safety and response to medication is overall comparable between males and females with ADHD (Storebo et al., 2015), but see (Sonuga-Barke et al., 2007). In the context of PM it is important to train teachers and professionals in recognizing sex-specific manifestations of ADHD.
Patient Preferences
It is important to take patients' preferences into account, since patients/families receiving the treatment of their choice tend to show better treatment adherence and clinical outcomes (Swift et al., 2011). Patient preference is particularly relevant in PM for ADHD since novel treatment options, such as nutritional interventions, computerized cognitive training, neurofeedback, and mindfulness training are increasingly considered (Stevenson et al., 2014; Cortese et al., 2015, 2016), and weighing the pros and cons of the various treatment options becomes more complex. Several methods are available that can help clarify treatment preference, such as the discrete choice experiment or the best-worst scaling method (Schatz et al., 2015).
Health Economics
ADHD has a profound negative impact on costs for health care both at the individual and the societal level (Le et al., 2014). Misdiagnosis or selection of an intervention not suitable or harmful to the individual patient will compromise cost-effective treatment of ADHD, as ineffective treatments and wastage of resources boost health care expenditures (Akhmetov et al., 2015). Further, an initial diagnosis at a much older age that had been missed earlier is associated with substantially higher health care and societal costs (Daley et al., 2015). PM holds the potential to lower health care-related costs, if patients immediately receive those therapies and interventions that are most likely be safe and effective for them (Abadi-Korek et al., 2013). However, to remain profitable for manufacturers, targeting smaller subpopulations identified by specific assessment methods, e.g. genomic testing, with highly specific therapies may also increase treatment expenses (Faulkner et al., 2012). Across Europe and the United States, there is significant variation and fragmentation with respect to regulations regarding licensing and reimbursement of PM technologies, and national health care systems are limited in their ability to adequately evaluate and provide access to personalized diagnostics and treatments brought to the market (Akhmetov et al., 2015). Reliable guidance for the design of studies that combine regulatory and reimbursement needs is lacking for novel PM technologies in many countries, though there is guidance from the Federal Drug Administration for clinical pharmacogenomics studies (FDA, 2013). Such guidance is mandatory for industry to gain a clear picture of the evidence required to receive market authorization and reimbursement within the public insurance systems. Clarifying areas of uncertainty and moving toward standard regulatory and reimbursement practices on a multi-national level will facilitate the broader adoption of PM into clinical practice (Faulkner et al., 2012).
Ethical Considerations
PM inevitably also faces ethical challenges (Evers, 2009; Lewis et al., 2014). First, PM must be accurate in order to not exclude individuals with ADHD from potentially effective care, and prioritize others arbitrarily, respectively. Second, stratification based on biomarkers may disadvantage those individuals who are excluded from the specified subgroups; see for example the discussion about use of polygenic risk scores (Martin et al., 2019). These might include ethnicities or minorities, who are already disadvantaged, which may increase stigmatization. Third, testing for a biomarker may also yield incidental findings that may either distract from or delay administration of the most effective treatment, and increase expense from unnecessary assessment, or cause more distress than relief to some patients. Fourth, biomarker studies also raise basic questions regarding access to health care, as private and public insurers could force individuals with ADHD first to conduct tests to determine coverage of therapies.
A Roadmap Toward Personalized Medicine in ADHD
There are many tasks and challenges to negotiate in developing and implementing PM for ADHD. This needs to involve a variety of research designs and strategies, coupled with both hypothesis-free (for hypothesis generation) and hypothesis-driven (for hypothesis testing). Firstly, big data science, such as exploitation of large case-registry data bases, will be useful for exploratory analyses and hypothesis generation with regard to clinical and family-genetic markers of course and outcome. Infrastructure such as the United Kingdom Biobank also offers unprecedented opportunities for exploratory analyses capitalizing on access to genetic, brain imaging and other biological data and soon also to information on ADHD symptoms scores (Sudlow et al., 2015). Birth cohort or other population-based studies with a longitudinal design such as IMAGEN, ALSPAC and Generation-R provide additional opportunities for exploratory and discovery analyses on dimensional measures of ADHD (Golding, 2004; Schumann et al., 2010; Kooijman et al., 2016).
Secondly, the cornerstone will be to conduct prospective longitudinal cohort studies for the identification of valid, reliable, and clinically useful predictors, mediators, and moderators of clinical course, treatment response and long-term outcome across the age range. These will be observational within-subject studies of multi-site clinical ADHD cohorts that should involve careful documentation of treatments provided, assessment of clinical responses, and incorporation of potential biomarkers for diagnostic and/or stratification and/or prediction purposes. Outcomes studied might range from diagnostic accuracy to prediction of type of treatment, dosage needed, treatment response, various functional real-world outcomes, and course, but should also include cost-efficiency. There need to be discovery and validation cohorts, and studies across subgroups to take account of ethnic and cultural diversity, and allow for dissection of findings by age and sex.
Thirdly, incorporation of potential biomarkers in pharmaceutical industry-sponsored or investigator-initiated RCTs at baseline and eventually endpoint would be another powerful strategy to move forward. Special designs with enrichment strategies will improve statistical power for biomarker analyses (Freidlin and Korn, 2014). Further, as classical study designs are poor at addressing ADHD heterogeneity, future clinical studies using novel designs, e.g. stepped-care designs, are also required as to complement to the more standard efficacy RCTs (Lenze et al., 2020).
Fourthly, biomarker studies might benefit from a stronger orientation toward the dimensional perspective on ADHD and the Research Domain Criteria (RDoC) framework (Cuthbert, 2014). The goal of RDoC is to understand the nature of psychiatric disorders in terms of varying degrees of dysfunction in general psychological/biological systems or dimensions that cut across traditional diagnostic boundaries. The dimensions directly relevant for ADHD are, of course, the clinical domains of inattention and hyperactivity-impulsivity, but also executive functioning, reward processing, and arousal and regulation. An example is work on the overlap between ADHD and autism spectrum disorder (ASD) that showed that combined ADHD-ASD cases could be subtyped according to strong vs. weak visuo-spatial working memory (van der Meer et al., 2012, 2017).
An essential requirement is to establish rigorous standard operating procedures (SOPs) across sites for biomarker assessment, including achieving standards for test-retest reliability, across-site reproducibility and accuracy. Feasibility will also be an issue, as, for example, MRI biomarkers may be scientifically relevant as probes into the neural underpinnings of ADHD but less implementable into clinical practice. Appropriate statistical techniques for predictive modeling that may exploit the potential of advances machine learning approaches should be used (Wong et al., 2017; Fusar-Poli et al., 2018; Quaak et al., 2021). These can aid in the integration of complex multi-level data to achieve excellent predictive performance by taking into account the non-linear relationships and interactions that connect the set of predictors and the response variable (Fusar-Poli et al., 2018). Ecological momentary assessment devices allow, for example, collection of a huge amount of individualized real-time data within the natural environment (Perna et al., 2018). Probably, given the heterogeneity of ADHD, multiple biomarker panels may be more promising because the as yet unknown underlying pathophysiology may be reflected in different indices across individuals at multiple levels of assessment (behavior, cognition, brain structure and function, metabolomics, proteomics). For the latter, cutting-edge work on metabolomics and proteomics in ADHD is relatively absent and in need of upgrading. Biomarker research is an iterative process, where findings will improve our understanding of the underlying pathophysiology and conceptualization of ADHD. In turn, this will facilitate new studies on biomarkers discovery and validation.
Before implementation to routine clinical practice, rigorous evaluation of efficacy, and cost-effectiveness of PM diagnostic and treatment algorithms will be needed.
Author Contributions
JB, TB, SC, LR, and SF developed the protocol for the search. AC and DL identified and retrieved the papers. TB, NC, and SC extracted the papers. JB and TB wrote the final version. All authors contributed to the writing of sections of the manuscript and contributed to the article and approved the submitted version.
Funding
This work was supported by an unrestricted educational grant by Medice. BF is supported by a personal grant from the Innovation Program of the Netherlands Organization for Scientific Research (NWO; grant 016-130-669). GP is supported by São Paulo Research Foundation (FAPESP; grant 2016/22455-8) and National Council for Scientific and Technological Development (CNPq; grant 310582/2017-2). LR is supported by the National Council for Scientific and Technological Development (CNPq; grant 308294/2018-1). Medice was not involved in the study design, collection, analysis, interpretation of data, the writing of this article or the decision to submit it for publication.
Conflict of Interest
JB has been in the past 3 years a consultant to/member of advisory board of / and/or speaker for Shire, Roche, Medice, and Servier. He is not an employee of any of these companies, and not a stock shareholder of any of these companies. He has no other financial or material support, including expert testimony, patents, royalties. LR has received grant or research support from, served as a consultant to, and served on the speakers' bureau of Eli Lilly and Co., Janssen, Medice, Novartis and Shire. The ADHD and Juvenile Bipolar Disorder Outpatient Programs chaired by LR have received unrestricted educational and research support from the following pharmaceutical companies: Eli Lilly and Co., Janssen, and Novartis. LR has received authorship royalties from Oxford Press and ArtMed and travel grants from Shire to take part in the 2018 APA annual meeting and from Novartis to take part of the 2016 AACAP annual meeting. Barbara Franke has received educational speaking fees from Medice and Shire. SB discloses that he has in the last 5 years acted as an author, consultant or lecturer for Shire, Medice, Roche, Eli Lilly, Prima Psychiatry, GLGroup, System Analytic, Ability Partner, Kompetento, Expo Medica, Clarion Healthcare, and Prophase. He receives royalties for text books and diagnostic tools from Huber/Hogrefe, Kohlhammer and UTB. DB serves as an unpaid scientific advisor for an EU-funded neurofeedback trial unrelated to the present work. TB served in an advisory or consultancy role for Actelion, Hexal Pharma, Lilly, Lundbeck, Medice, Neurim Pharmaceuticals, Novartis, Shire. He received conference support or speaker's fee by Lilly, Medice, Novartis and Shire. He has been involved in clinical trials conducted by Shire & Viforpharma. He received royalties from Hogrefe, Kohlhammer, CIP Medien, Oxford University Press. The present work is unrelated to the above grants and relationships. DC served in an advisory or consultancy role for Medice, Novartis, Shire/Takeda. He received conference support or speaker's fee by Medice, Servier and Shire/Takeda. He has been involved in clinical trials conducted by Shire. He received royalties from Oxford University Press. The present work is unrelated to the above grants and relationships. In the past year, SF received income, potential income, travel expenses continuing education support and/or research support from Tris, Otsuka, Arbor, Ironshore, Shire, Akili, Enzymotec, Sunovion, Supernus and Genomind. With his institution, he has US patent US20130217707 A1 for the use of sodium-hydrogen exchange inhibitors in the treatment of ADHD. In previous years, he received support from: Shire, Ironshore, Neurovance, Alcobra, Rhodes, CogCubed, KemPharm, Enzymotec, Akili, Neurolifesciences, Lundbeck/Takeda, Otsuka, McNeil, Janssen, Novartis, Pfizer and Eli Lilly. He also receives royalties from books published by Guilford Press: Straight Talk about Your Child's Mental Health, Oxford University Press: Schizophrenia: The Facts and Elsevier: ADHD: Non-Pharmacologic Interventions. He is principal investigator of www.adhdinadults.com. Guilherme V. Polanczyk has been in the past 3 years a consultant, member of advisory board, and/or speaker for Shire/Takeda and Medice. He received travel expenses for continuing education support from Shire/Takeda and royalties from Editora Manole. In the past three years, Jeffrey Newcorn is/has been an advisor and/or consultant for Adlon Therapeutics, Akili Interactive, Alcobra, Arbor, Cingulate Therapeutics, Enzymotec, KemPharm, Lundbeck/Takeda, Medice, NLS, Rhodes, Shire, and Supernus. He was a DSMB member for Pfizer and Sunovion, and received research funds from Enzymotec, Otsuka, Shire and Supernus. He also has received speaker fees from Shire for disease-state presentations, and served as a consultant for the US National Football League. BV has served as consultant to Medice and Teva Pharm. Markus Gleitz is an employee of Medice Arzneimittel Pütter GmbH & Co. KG. MS received research support from Supernus, Shire, and Akili Interactive labs and in the past year has served in a advisory or consultancy capacity to Genomind, NLS, Shire/Takeda, Supernus, and Sunovian.
The remaining authors declare that the research was conducted in the absence of any commercial or financial relationships that could be construed as a potential conflict of interest.
The reviewer KAJ declared a shared affiliation with the author DC to the handling editor at the time of review.
Publisher's Note
All claims expressed in this article are solely those of the authors and do not necessarily represent those of their affiliated organizations, or those of the publisher, the editors and the reviewers. Any product that may be evaluated in this article, or claim that may be made by its manufacturer, is not guaranteed or endorsed by the publisher.
Supplementary Material
The Supplementary Material for this article can be found online at: https://www.frontiersin.org/articles/10.3389/fnbeh.2022.900981/full#supplementary-material
References
Abadi-Korek, I., Glazer, J., Granados, A., Luxenburg, O., Trusheim, M. R., Hakak, N., et al. (2013). Personalized medicine and health economics: is small the new big? A white paper. Israel Med. Assoc. J. 15, 602–607.
Adamo, N. (2018). “The underlying pathways to ADHD and its long-term outcomes: a study of genetic risk factors and cognitive-brain markers”, in Social Genetic and Developmental Psychiatry Centre. London, King's College London.
Agnew-Blais, J. C., Belsky, D. W., Caspi, A., Danese, A., Moffitt, T. E., Polanczyk, G. V., et al. (2021). Polygenic risk and the course of attention-deficit/hyperactivity disorder from childhood to young adulthood: findings from a nationally representative cohort. J. Am. Acad. Child. Adolesc. Psychiatry. 60, 1147–1156. doi: 10.1016/j.jaac.2020.12.033
Akay, A. P., Kaya, G. C., Kose, S., Yazicioglu, C. E., Erkuran, H. O., Guney, S. A., et al. (2018). Genetic imaging study with [Tc-(99m)] TRODAT-1 SPECT in adolescents with ADHD using OROS-methylphenidate. Progr Neuro-Psychopharmacol Biol. Psychiat. 86, 294–300. doi: 10.1016/j.pnpbp.2018.04.008
Akhmetov, I., Ramaswamy, R., and Thimmaraju, P. K. (2015). Market access advancements and challenges in “drug-companion diagnostic test” co-development in Europe. J. Personalized Med. 5, 213–228. doi: 10.3390/jpm5020213
APA (American Psychiatric Association). (2013). Diagnostic and Statistical Manual of Mental Disorders DSM 5-−5th edn. Washington DC, American Psychiatric Association.
Arns, M., Gunkelman, J., Breteler, M., and Spronk, D. (2008). EEG phenotypes predict treatment outcome to stimulants in children with ADHD. J. Integr. Neurosci. 7, 421–438. doi: 10.1142/S0219635208001897
Arns, M., Vollebregt, M. A., Palmer, D., Spooner, C., Gordon, E., Kohn, M., et al. (2018). Electroencephalographic biomarkers as predictors of methylphenidate response in attention-deficit/hyperactivity disorder. Eur. Neuropsychopharmacol. 28, 881–891. doi: 10.1016/j.euroneuro.2018.06.002
Asherson, P., and Agnew-Blais, J. (2019). Annual Research Review: Does late-onset attention-deficit/hyperactivity disorder exist? J. Child Psychol. Psychiatry. 60, 333–352. doi: 10.1111/jcpp.13020
Asherson, P., Buitelaar, J., Faraone, S. V., and Rohde, L. A. (2016). Adult attention-deficit hyperactivity disorder: key conceptual issues. Lancet Psychiatry. 3, 568–578. doi: 10.1016/S2215-0366(16)30032-3
Bach, M. V., Coutts, R. T., and Baker, G. B. (1999). Involvement of CYP2D6 in the in vitro metabolism of amphetamine, two N-alkylamphetamines and their 4-methoxylated derivatives. Xenobiotica; Fate Foreign Comp. Biol. Syst. 29, 719–732. doi: 10.1080/004982599238344
Barry, R. J., Clarke, A. R., and Johnstone, S. J. (2003). A review of electrophysiology in attention-deficit/hyperactivity disorder: I. Qualitative and quantitative electroencephalography. Clin. Neurophysiol. 114, 171–183. doi: 10.1016/S1388-2457(02)00362-0
Berger, C., Lev, A., Braw, Y., Elbaum, T., Wagner, M., and Rassovsky, Y. (2021). Detection of feigned ADHD using the MOXO-d-CPT. J. Atten. Disord. 25, 1032–1047. doi: 10.1177/1087054719864656
Biederman, J., Fitzgerald, M., Kirova, A. M., Woodworth, K. Y., Biederman, I., and Faraone, S. V. (2018). Further Evidence of Morbidity and Dysfunction Associated With Subsyndromal ADHD in clinically referred children. J. Clin. Psychiatry. 79. doi: 10.4088/JCP.17m11870
Bloch, M. H., Panza, K. E., Landeros-Weisenberger, A., and Leckman, J. F. (2009). Meta-analysis: treatment of attention-deficit/hyperactivity disorder in children with comorbid tic disorders. J. Am. Acad. Child Adolesc. Psychiat. 48, 884–893. doi: 10.1097/CHI.0b013e3181b26e9f
Bohland, J. W., Saperstein, S., Pereira, F., Rapin, J., and Grady, L. (2012). Network, anatomical, and non-imaging measures for the prediction of ADHD diagnosis in individual subjects. Front. Syst. Neurosci. 6, 78. doi: 10.3389/fnsys.2012.00078
Boyle, E. A., Li, Y. I., and Pritchard, J. K. (2017). An expanded view of complex traits: from polygenic to omnigenic. Cell. 169, 1177–1186. doi: 10.1016/j.cell.2017.05.038
Breda, V., Rohde, L. A., Menezes, A. M. B., Anselmi, L., Caye, A., Rovaris, D. L., et al. (2021). The neurodevelopmental nature of attention-deficit hyperactivity disorder in adults. Br. J. Psychiatry. 218, 43–50. doi: 10.1192/bjp.2020.200
Brikell, I., Burton, C., Mota, N. R., and Martin, J. (2021). Insights into attention-deficit/hyperactivity disorder from recent genetic studies. Psychol. Med. 51, 2274–2286. doi: 10.1017/S0033291721000982
Brown, C. R., Clarke, A. R., Barry, R. J., McCarthy, R., Selikowitz, M., and Magee, C. (2005). Event-related potentials in attention-deficit/hyperactivity disorder of the predominantly inattentive type: an investigation of EEG-defined subtypes. Int. J. Psychophysiol. 58, 94–107. doi: 10.1016/j.ijpsycho.2005.03.012
Caye, A., Spadini, A. V., Karam, R. G., Grevet, E. H., Rovaris, D. L., Bau, C. H., et al. (2016). Predictors of persistence of ADHD into adulthood: a systematic review of the literature and meta-analysis. Eur. Child Adolesc. Psychiat. 25, 1151–1159. doi: 10.1007/s00787-016-0831-8
Chabot, R. J., Orgill, A. A., Crawford, G., Harris, M. J., and Serfontein, G. (1999). Behavioral and electrophysiologic predictors of treatment response to stimulants in children with attention disorders. J. Child Neurol. 14, 343–351. doi: 10.1177/088307389901400601
Chabot, R. J., and Serfontein, G. (1996). Quantitative electroencephalographic profiles of children with attention deficit disorder. Biol Psychiatry. 40, 951–963. doi: 10.1016/0006-3223(95)00576-5
Chazan, R., Borowski, C., Pianca, T., Ludwig, H., Rohde, L. A., and Polanczyk, G. (2011). Do phenotypic characteristics, parental psychopathology, family functioning, and environmental stressors have a role in the response to methylphenidate in children with attention-deficit/hyperactivity disorder? A naturalistic study from a developing country. J. Clin. Psychopharmacol. 31, 309–317. doi: 10.1097/JCP.0b013e318217b4df
Chen, M., Li, H., Wang, J., Dillman, J. R., Parikh, N. A., and He, L. (2019). A multichannel deep neural network model analyzing multiscale functional brain connectome data for attention deficit hyperactivity disorder detection. Radiol. Artif. Intellig. 2, e190012. doi: 10.1148/ryai.2019190012
Cheng, W., Ji, X., Zhang, J., and Feng, J. (2012). Individual classification of ADHD patients by integrating multiscale neuroimaging markers and advanced pattern recognition techniques. Front. Syst. Neurosci. 6, 58. doi: 10.3389/fnsys.2012.00058
Cheung, C. H. M., Rijsdijk, F., McLoughlin, G., Brandeis, D., Banaschewski, T., Asherson, P., and Kuntsi, J. (2016). Cognitive and neurophysiological markers of ADHD persistence and remission. British J. Psychiatry. 208, 548–555. doi: 10.1192/bjp.bp.114.145185
Cho, S. C., Hwang, J. W., Kim, B. N., Lee, H. Y., Kim, H. W., Lee, J. S., et al. (2007). The relationship between regional cerebral blood flow and response to methylphenidate in children with attention-deficit hyperactivity disorder: comparison between non-responders to methylphenidate and responders. J. Psychiatr. Res. 41, 459–465. doi: 10.1016/j.jpsychires.2006.05.011
Clarke, A. R., Barry, R. J., Dupuy, F. E., McCarthy, R., Selikowitz, M., and Heaven, P. C. L. (2011). Childhood EEG as a predictor of adult attention-deficit/hyperactivity disorder. Clin. Neurophysiol. 122, 73–80. doi: 10.1016/j.clinph.2010.05.032
Clarke, A. R., Barry, R. J., Dupuy, F. E., McCarthy, R., Selikowitz, M., and Johnstone, S. J. (2013). Excess beta activity in the EEG of children with attention-deficit/hyperactivity disorder: a disorder of arousal? Int. J. Psychophysiol. 89, 314–319. doi: 10.1016/j.ijpsycho.2013.04.009
Clarke, A. R., Barry, R. J., and Johnstone, S. (2020). Resting state EEG power research in Attention-Deficit/Hyperactivity Disorder: A review update. Clin. Neurophysiol. 131, 1463–1479. doi: 10.1016/j.clinph.2020.03.029
Clarke, A. R., Barry, R. J., Karamacoska, D., and Johnstone, S. J. T. (2019). Theta/Beta Ratio: A marker of Arousal or Cognitive Processing Capacity? Appl. Psychophysiol. Biofeedback. 44, 123–129. doi: 10.1007/s10484-018-09428-6
Clarke, A. R., Barry, R. J., McCarthy, R., and Selikowitz, M. (2002). EEG differences between good and poor responders to methylphenidate and dexamphetamine in children with attention-deficit/hyperactivity disorder. Clin. Neurophysiol. 113, 194–205. doi: 10.1016/S1388-2457(01)00736-2
Coghill, D. R., Rhodes, S. M., and Matthews, K. (2007). The neuropsychological effects of chronic methylphenidate on drug-naive boys with attention-deficit/hyperactivity disorder. Biol. Psychiat. 62, 954–962. doi: 10.1016/j.biopsych.2006.12.030
Coghill, D. R., Seth, S., and Matthews, K. (2014). A comprehensive assessment of memory, delay aversion, timing, inhibition, decision making and variability in attention deficit hyperactivity disorder: advancing beyond the three-pathway models. Psychol. Med. 44, 1989–2001. doi: 10.1017/S0033291713002547
Cohen, R., Cohen-Kroitoru, B., Halevy, A., Aharoni, S., Aizenberg, I., and Shuper, A. (2019). Handwriting in children with Attention Deficient Hyperactive Disorder: role of graphology. BMC Pediatrics 19, 484. doi: 10.1186/s12887-019-1854-3
Colby, J. B., Rudie, J. D., Brown, J. A., Douglas, P. K., Cohen, M. S., and Shehzad, Z. (2012). Insights into multimodal imaging classification of ADHD. Front. Syst. Neurosci. 6, 59. doi: 10.3389/fnsys.2012.00059
Cooper, M., Hammerton, G., Collishaw, S., Langley, K., Thapar, A., Dalsgaard, S., et al. (2018). Investigating late-onset ADHD: a population cohort investigation. J. Child Psychol. Psychiatry. 59, 1105–1113. doi: 10.1111/jcpp.12911
Cortese, S., Adamo, N., Del Giovane, C., Mohr-Jensen, C., Hayes, A. J., Carucci, S., et al. (2018). Comparative efficacy and tolerability of medications for attention-deficit hyperactivity disorder in children, adolescents, and adults: a systematic review and network meta-analysis. Lancet Psychiatry. 5, 727–738. doi: 10.1016/S2215-0366(18)30269-4
Cortese, S., Ferrin, M., Brandeis, D., Buitelaar, J., Daley, D., Dittmann, R. W., et al. (2015). Cognitive training for attention-deficit/hyperactivity disorder: meta-analysis of clinical and neuropsychological outcomes from randomized controlled trials. J. Am. Acad. Child. Adolesc. Psychiatry. 54, 164–174. doi: 10.1016/j.jaac.2014.12.010
Cortese, S., Ferrin, M., Brandeis, D., Holtmann, M., Aggensteiner, P., Daley, D., et al. (2016). Neurofeedback for attention-deficit/hyperactivity disorder: meta-analysis of clinical and neuropsychological outcomes from randomized controlled trials. J. Am. Acad. Child. Adolesc, Psychiatry. 55, 444–455. doi: 10.1016/j.jaac.2016.03.007
Costa Dias, T. G., Iyer, S. P., Carpenter, S. D., Cary, R. P., Wilson, V. B., Mitchell, S. H., et al. (2015). Characterizing heterogeneity in children with and without ADHD based on reward system connectivity. Develop. Cogn. Neurosci. 11, 155–174. doi: 10.1016/j.dcn.2014.12.005
Cuthbert, B. N. (2014). The RDoC framework: facilitating transition from ICD/DSM to dimensional approaches that integrate neuroscience and psychopathology. World Psychiat. 13, 28–35. doi: 10.1002/wps.20087
Cutica, I., Vie, G. M., and Pravettoni, G. (2014). Personalised medicine: the cognitive side of patients. Eur. J. Internal Med. 25, 685–688. doi: 10.1016/j.ejim.2014.07.002
Dai, D., Wang, J., Hua, J., and He, H. (2012). Classification of ADHD children through multimodal magnetic resonance imaging. Front. Syst. Neurosci. 6, 63. doi: 10.3389/fnsys.2012.00063
Daley, D., Jacobsen, R., Lange, A.-.M, Sørensen, A., and Walldorf, J. (2015). Costing Adult Attention Deficit Hyperactivity Disorder. Impact on the Individual and Society. Oxford, Oxford University Press. doi: 10.1093/acprof:oso/9780198745556.001.0001
Daley, D., van der Oord, S., Ferrin, M., Danckaerts, M., Doepfner, M., Cortese, S., et al. (2014). Behavioral interventions in attention-deficit/hyperactivity disorder: a meta-analysis of randomized controlled trials across multiple outcome domains. J. Am. Acad. Child. Adolesc. Psychiatry. 53, 835–847. e835. doi: 10.1016/j.jaac.2014.05.013
Demontis, D., Lescai, F., Borglum, A., Glerup, S., Ostergaard, S. D., Mors, O., et al. (2016). Reveals Increased Burden of Rare Functional and Disruptive Variants in Candidate Risk Genes in Individuals With Persistent Attention-Deficit/Hyperactivity Disorder. J. Am. Acad. Child Adolesc. Psychiat. 55, 521–523. doi: 10.1016/j.jaac.2016.03.009
Dey, S., Rao, A. R., and Shah, M. (2014). Attributed graph distance measure for automatic detection of attention deficit hyperactive disordered subjects. Front. Neural Circ. 8, 64. doi: 10.3389/fncir.2014.00064
Doehnert, M., Brandeis, D., Schneider, G., Drechsler, R., and Steinhausen, H. C. (2013). A neurophysiological marker of impaired preparation in an 11-year follow-up study of Attention-Deficit/Hyperactivity Disorder (ADHD). J. Child Psychol. Psychiat. 54, 260–270. doi: 10.1111/j.1469-7610.2012.02572.x
Dopfner, M., Breuer, D., Wille, N., Erhart, M., and Ravens-Sieberer, U. (2008). How often do children meet ICD-10/DSM-IV criteria of attention deficit-/hyperactivity disorder and hyperkinetic disorder? Parent-based prevalence rates in a national sample–results of the BELLA study. Eur. Child Adolesc. Psychiatry. 17, 59–70. doi: 10.1007/s00787-008-1007-y
Dopfner, M., Hautmann, C., Dose, C., Banaschewski, T., Becker, K., Brandeis, D., et al. (2017). ESCAschool study: trial protocol of an adaptive treatment approach for school-age children with ADHD including two randomised trials. BMC Psychiatry. 17, 269. doi: 10.1186/s12888-017-1433-9
dos Santos Siqueira, A., Biazoli Junior, C. E., Comfort, W. E., Rohde, L. A., and Sato, J. R. (2014). Abnormal functional resting-state networks in ADHD: graph theory and pattern recognition analysis of fMRI data. BioMed. Res. Int. 2014, 380531. doi: 10.1155/2014/380531
Drucker, E., and Krapfenbauer, K. (2013). Pitfalls and limitations in translation from biomarker discovery to clinical utility in predictive and personalised medicine. EPMA J. 4, 7. doi: 10.1186/1878-5085-4-7
Drysdale, A. T., Grosenick, L., Downar, J., Dunlop, K., Mansouri, F., Meng, Y., et al. (2017). Resting-state connectivity biomarkers define neurophysiological subtypes of depression. Nat. Med. 23, 28–38. doi: 10.1038/nm.4246
Edebol, H., Helldin, L., and Norlander, T. (2012). Objective Measures of Behavior Manifestations in Adult ADHD and Differentiation from Participants with Bipolar II Disorder, Borderline Personality Disorder, Participants with Disconfirmed ADHD as Well as Normative Participants. Clin. Pract Epidemiol Mental Health. 8, 134–143. doi: 10.2174/1745017901208010134
Edebol, H., Helldin, L., and Norlander, T. (2013). Measuring adult Attention Deficit Hyperactivity Disorder using the Quantified Behavior Test Plus. PsyCh J. 2, 48–62. doi: 10.1002/pchj.17
Eloyan, A., Muschelli, J., and Nebel, M. B. (2012). Automated diagnoses of attention deficit hyperactive disorder using magnetic resonance imaging. Front. Syst. Neurosci. 6, 61. doi: 10.3389/fnsys.2012.00061
Esposito, F. L., Varela, P., Alkan, E. O., Morata, I., De la Osa, N., and Ezpeleta, L. (2016). Validation of BGaze method supporting ADHD diagnosis. Eur. Congress Psychiat. 33S:S135. doi: 10.1016/j.eurpsy.2016.01.211
Evers, K. (2009). Personalized medicine in psychiatry: ethical challenges and opportunities. Dial. Clin. Neurosci. 11, 427–434. doi: 10.31887/DCNS.2009.11.4/kevers
Fair, D. A., Bathula, D., Nikolas, M. A., and Nigg, J. T. (2012). Distinct neuropsychological subgroups in typically developing youth inform heterogeneity in children with ADHD. Proc. Natl. Acad. Sci. U S A. 109, 6769–6774. doi: 10.1073/pnas.1115365109
Faraone, S. V., Asherson, P., Banaschewski, T., Biederman, J., Buitelaar, J. K., Ramos-Quiroga, J. A., et al. (2015). Attention-deficit/hyperactivity disorder. Nat. Rev. Dis. Prim. 1, 15020. doi: 10.1038/nrdp.2015.20
Faraone, S. V., Biederman, J., Morley, C. P., and Spencer, T. J. (2008). Effect of stimulants on height and weight: a review of the literature. J. Am. Acad. Child Adolesc. Psychiat. 47, 994–1009. doi: 10.1097/CHI.0b013e31817e0ea7
Faraone, S. V., and Larsson, H. (2019). Genetics of attention deficit hyperactivity disorder. Molec. Psychiatry. 24, 562–75. doi: 10.1038/s41380-018-0070-0
Faulkner, E., Annemans, L., Garrison, L., Helfand, M., Holtorf, A. P., Hornberger, J., et al. (2012). Challenges in the development and reimbursement of personalized medicine-payer and manufacturer perspectives and implications for health economics and outcomes research: a report of the ISPOR personalized medicine special interest group. Value Health. 15, 1162–1171. doi: 10.1016/j.jval.2012.05.006
Fayyad, J., Sampson, N. A., Hwang, I., Adamowski, T., Aguilar-Gaxiola, S., Al-Hamzawi, A., et al. (2017). The descriptive epidemiology of DSM-IV Adult ADHD in the world health organization world mental health surveys. Attent. Deficit Hyperact. Disord. 9, 47–65. doi: 10.1007/s12402-016-0208-3
FDA. (2013). Available online at: https://www.fda.gov/downloads/Drugs/GuidanceComplianceRegulatoryInformation/Guidances/UCM337169.pdf (accessed May 29, 2022).
Firestone, P., and Witt, J. E. (1982). Characteristics of families completing and prematurely discontinuing a behavioral parent-training program. J. Pediatric Psychol. 7, 209–222. doi: 10.1093/jpepsy/7.2.209
Freidlin, B., and Korn, E. L. (2014). Biomarker enrichment strategies: matching trial design to biomarker credentials. Nat. Rev. Clin. Oncol. 11, 81–90. doi: 10.1038/nrclinonc.2013.218
Fried, R., Hirshfeld-Becker, D., Petty, C., Batchelder, H., and Biederman, J. (2015). How informative is the CANTAB to assess executive functioning in children with ADHD? A controlled study. J. Attent. Disord. 19, 468–475. doi: 10.1177/1087054712457038
Froehlich, T. E., McGough, J. J., and Stein, M. A. (2010). Progress and promise of attention-deficit hyperactivity disorder pharmacogenetics. CNS drugs. 24, 99–117. doi: 10.2165/11530290-000000000-00000
Fuermaier, A. B., Tucha, O., and Koerts, J. (2016). The Development of an Embedded Figures Test for the Detection of Feigned Attention Deficit Hyperactivity Disorder in Adulthood. PLoS ONE. 11, e0164297. doi: 10.1371/journal.pone.0164297
Fuermaier, A. B. M., Tucha, O., and Koerts, J. (2017). Noncredible cognitive performance at clinical evaluation of adult ADHD: An embedded validity indicator in a visuospatial working memory test. Psychol. Assessment 29, 1466–1479. doi: 10.1037/pas0000534
Fusar-Poli, P., Hijazi, Z., Stahl, D., and Steyerberg, E. W. (2018). The science of prognosis in psychiatry: a review. JAMA Psychiat. 75, 1289–1297. doi: 10.1001/jamapsychiatry.2018.2530
Gadow, K. D., Nolan, E. E., Sverd, J., Sprafkin, J., and Schwartz, J. (2002). Anxiety and depression symptoms and response to methylphenidate in children with attention-deficit hyperactivity disorder and tic disorder. J. Clin. Psychopharmacol. 22, 267–274. doi: 10.1097/00004714-200206000-00007
Ganna, A., Satterstrom, F. K., Zekavat, S. M., Das, I., Kurki, M. I., Churchhouse, C., et al. (2018). Quantifying the Impact of Rare and Ultra-rare Coding Variation across the Phenotypic Spectrum. Am. J. Hum. Genet. 102, 1204–1211. doi: 10.1016/j.ajhg.2018.05.002
Gates, K. M., Molenaar, P. C., Iyer, S. P., Nigg, J. T., and Fair, D. A. (2014). Organizing heterogeneous samples using community detection of GIMME-derived resting state functional networks. PLoS ONE. 9, e91322. doi: 10.1371/journal.pone.0091322
Gau, S. S., Shen, H. Y., Chou, M. C., Tang, C. S., Chiu, Y. N., and Gau, C. S. (2006). Determinants of adherence to methylphenidate and the impact of poor adherence on maternal and family measures. J. Child Adolesc. Psychopharmacol. 16, 286–297. doi: 10.1089/cap.2006.16.286
Gehricke, J. G., Kruggel, F., and Thampipop, T. (2017). The brain anatomy of attention-deficit/hyperactivity disorder in young adults - a magnetic resonance imaging study. PLoS ONE 12, e0175433. doi: 10.1371/journal.pone.0175433
Geschwind, D. H., and Flint, J. (2015). Genetics and genomics of psychiatric disease. Science. 349, 1489–1494. doi: 10.1126/science.aaa8954
Gevensleben, H., Holl, B., Albrecht, B., Schlamp, D., Kratz, O., Studer, P., et al. (2009). Distinct EEG effects related to neurofeedback training in children with ADHD: A randomized controlled trial. Int. J. Psychophysiol. 74, 149–157. doi: 10.1016/j.ijpsycho.2009.08.005
Gilbert, H., Qin, L., Li, D., Zhang, X., and Johnstone, S. J. (2016). Aiding the diagnosis of AD/HD in childhood: Using actigraphy and a continuous performance test to objectively quantify symptoms. Res. Dev. Disabil. 59, 35–42. doi: 10.1016/j.ridd.2016.07.013
Ginsburg, G. S., and Willard, H. F. (2009). Genomic and personalized medicine: foundations and applications. Transl. Res. 154, 277–287. doi: 10.1016/j.trsl.2009.09.005
Golding, J. (2004). The Avon Longitudinal Study of Parents and Children (ALSPAC)–study design and collaborative opportunities. Eur. J. Endocrinol. 151, U119–123. doi: 10.1530/eje.0.151u119
Goldstein, B. I., Birmaher, B., Carlson, G. A., DelBello, M. P., Findling, R. L., Fristad, M., et al. (2017). The International Society for Bipolar Disorders Task Force report on pediatric bipolar disorder: knowledge to date and directions for future research. Bipolar Disor. 19, 524–543. doi: 10.1111/bdi.12556
Greven, C., Richards, J. S., and Buitelaar, J. (2018). Sex differences in ADHD. in Oxford Textbook of Attention Deficit Hyperactivity Disorder. Edited by Banaschewski T, Coghill D, Zuddas A. Oxford, Oxford University Press. p. 154–161. doi: 10.1093/med/9780198739258.003.0016
Griffiths, K. R., Jurigova, B. G., Leikauf, J. E., Palmer, D., Clarke, S. D., Tsang, T. W., et al. (2017). A signature of attention-elicited electrocortical activity distinguishes response from non-response to the non-stimulant atomoxetine in children and adolescents with ADHD. J. Attent. Diso. 1087054717733044. doi: 10.1177/1087054717733044
Grodzinsky, G. M., and Barkley, R. A. (1999). Predictive power of frontal lobe tests in the diagnosis of attention deficit hyperactivity disorder. Clin. Neuropsychol. 13, 12–21. doi: 10.1076/clin.13.1.12.1983
Groenman, A. P., Hornstra, R., Hoekstra, P. J., Steenhuis, L., Aghebati, A., Boyer, B. E., et al. (2022). An individual participant data meta-analysis: behavioral treatments for children and adolescents with attention-deficit/hyperactivity disorder. J. Am. Acad. Child Adolesc. Psychiatry. 61, 144–158. doi: 10.1016/j.jaac.2021.02.024
Groom, M. J., Young, Z., Hall, C. L., Gillott, A., and Hollis, C. (2016). The incremental validity of a computerised assessment added to clinical rating scales to differentiate adult ADHD from autism spectrum disorder. Psychiatry Res. 243, 168–173. doi: 10.1016/j.psychres.2016.06.042
Gupta, R., Kar, B. R., and Srinivasan, N. (2011). Cognitive-motivational deficits in ADHD: development of a classification system. Child Neuropsychol. 17, 67–81. doi: 10.1080/09297049.2010.524152
Hamburg, M. A., and Collins, F. S. (2010). The path to personalized medicine. New Engl. J. Med. 363, 301–304. doi: 10.1056/NEJMp1006304
Hamshere, M. L., Langley, K., Martin, J., Agha, S. S., Stergiakouli, E., Anney, R. J., et al. (2013). High loading of polygenic risk for ADHD in children with comorbid aggression. Am. J. Psychiatry. 170, 909–916. doi: 10.1176/appi.ajp.2013.12081129
Handen, B. L., Janosky, J., McAuliffe, S., Breaux, A. M., and Feldman, H. (1994). Prediction of response to methylphenidate among children with ADHD and mental retardation. J. Am. Acad. Child Adolesc. Psychiat. 33, 1185–1193. doi: 10.1097/00004583-199410000-00014
Hart, A. B., de Wit, H., and Palmer, A. A. (2013). Candidate gene studies of a promising intermediate phenotype: failure to replicate. Neuropsychopharmacol. 38, 802–816. doi: 10.1038/npp.2012.245
Hart, H., Chantiluke, K., and Cubillo, A. I. (2014). Pattern classification of response inhibition in ADHD: toward the development of neurobiological markers for ADHD. Hum. Brain Mapp. 35, 3083–3094. doi: 10.1002/hbm.22386
Heerde, J. A., Scholes-Balog, K. E., and Hemphill, S. A. (2015). Associations between youth homelessness, sexual offenses, sexual victimization, and sexual risk behaviors: a systematic literature review. Arch. Sex. Behav. 44, 181–212. doi: 10.1007/s10508-014-0375-2
Henríquez-Henríquez, M. P., Solari, S., Quiroga, T., Kim, B. I., Deckelbaum, R. J., and Worgall, T. S. (2015). Low serum sphingolipids in children with attention deficit-hyperactivity disorder. Front. Neurosci. 9, 300. doi: 10.3389/fnins.2015.00300
Hermens, D. F., Cooper, N. J., Kohn, M., Clarke, S., and Gordon, E. (2005). Predicting stimulant medication response in ADHD: evidence from an integrated profile of neuropsychological, psychophysiological and clinical factors. J. Integr. Neurosci. 4, 107–121. doi: 10.1142/S0219635205000653
Hinshaw, S. P. (2007). Moderators and mediators of treatment outcome for youth with ADHD: understanding for whom and how interventions work. J. Pediatric Psychol. 32, 664–675. doi: 10.1093/jpepsy/jsl055
Hodgkins, P., Shaw, M., Coghill, D., and Hechtman, L. (2012). Amfetamine and methylphenidate medications for attention-deficit/hyperactivity disorder: complementary treatment options. Eur. Child Adolesc. Psychiatry. 21, 477–492. doi: 10.1007/s00787-012-0286-5
Hollis, C., Hall, C. L., Guo, B., James, M., Boadu, J., Groom, M. J., et al. (2018). The impact of a computerised test of attention and activity (QbTest) on diagnostic decision-making in children and young people with suspected attention deficit hyperactivity disorder: single-blind randomised controlled trial. J. Child. Psychol. Psychiatry. 59, 1298–1308. doi: 10.1111/jcpp.12921
Hult, N., Kadesj,ö, J., Kadesj,ö, B., Gillberg, C., and Billstedt, E. (2018). ADHD and the qbtest: diagnostic validity of qbtest. J. Atten. Disord. 22, 1074–1080. doi: 10.1177/1087054715595697
Ilbegi, S., Buitelaar, J. K., Hoekstra, P. J., Hartman, C. A., Franke, B., Faraone, S. V., et al. (2021). Neurocognitive markers of late-onset ADHD: a 6-year longitudinal study. J. Child Psychol. Psychiatry. 62, 244–252. doi: 10.1111/jcpp.13272
Ishii-Takahashi, A., Takizawa, R., and Nishimura, Y. (2014). Prefrontal activation during inhibitory control measured by near-infrared spectroscopy for differentiating between autism spectrum disorders and attention deficit hyperactivity disorder in adults. NeuroImage Clin. 4, 53–63. doi: 10.1016/j.nicl.2013.10.002
Jameson, J. L., and Longo, D. L. (2015). Precision medicine–personalized, problematic, and promising. New Engl. J. Med. 372, 2229–2234. doi: 10.1056/NEJMsb1503104
Janssen, T. W. P., Bink, M., Gelad,é, K., Mourik, R., Maras, A., and Oosterlaan, J. (2016). A randomized controlled trial into the effects of neurofeedback, methylphenidate, and physical activity on EEG power spectra in children with ADHD. J. Child Psychol. Psychiat. 57, 633–644. doi: 10.1111/jcpp.12517
Jasinski, L. J., Harp, J. P., Berry, D. T., Shandera-Ochsner, A. L., Mason, L. H., and Ranseen, J. D. (2011). Using symptom validity tests to detect malingered ADHD in college students. Clin. Neuropsychol. 25, 1415–1428. doi: 10.1080/13854046.2011.630024
Joensen, B., Meyer, M., and Aagaard, L. (2017). Specific genes associated with adverse events of methylphenidate use in the pediatric population: a systematic literature review. J. Res Phar. Pract. 6, 65–72. doi: 10.4103/jrpp.JRPP_16_161
Johansson, V., Norén Selinus, E., and Kuja-Halkola, R. (2021). The Quantified Behavioral Test Failed to Differentiate ADHD in Adolescents With Neurodevelopmental Problems. J. Atten. Disord. 25, 312–321. doi: 10.1177/1087054718787034
Johnston, B. A., Coghill, D., Matthews, K., and Steele, J. D. (2015). Predicting methylphenidate response in attention deficit hyperactivity disorder: a preliminary study. J. Psychopharmacol. 29, 24–30. doi: 10.1177/0269881114548438
Juselius Baghdassarian, E., Nilsson Markhed, M., Lindström, E., Nilsson, B. M., and Lewander, T. (2018). Auditory brainstem response (ABR) profiling tests as diagnostic support for schizophrenia and adult attention-deficit hyperactivity disorder (ADHD). Acta Neuropsychiatr. 30, 137–147. doi: 10.1017/neu.2017.24
Kaiser, A., Aggensteiner, P. M., Baumeister, S., Holz, N. E., Banaschewski, T., and Brandeis, D. (2020). Earlier versus later cognitive event-related potentials (ERPs) in attention-deficit/hyperactivity disorder (ADHD): a meta-analysis. Neurosci. Biobehav. Rev. 112, 117–134. doi: 10.1016/j.neubiorev.2020.01.019
Kapur, S., Phillips, A. G., and Insel, T. R. (2012). Why has it taken so long for biological psychiatry to develop clinical tests and what to do about it? Molec. Psychiat. 17, 1174–1179. doi: 10.1038/mp.2012.105
Karalunas, S. L., Fair, D., Musser, E. D., Aykes, K., Iyer, S. P., and Nigg, J. T. (2014). Subtyping attention-deficit/hyperactivity disorder using temperament dimensions: toward biologically based nosologic criteria. JAMA Psychiatry. 71, 1015–1024. doi: 10.1001/jamapsychiatry.2014.763
Karalunas, S. L., Gustafsson, H. C., Fair, D., Musser, E. D., and Nigg, J. T. (2019). Do we need an irritable subtype of ADHD? Replication and extension of a promising temperament profile approach to ADHD subtyping. Psychol. Assess. 31, 236–247. doi: 10.1037/pas0000664
Katz, L. J., Wood, D. S., Goldstein, G., Auchenbach, R. C., and Geckle, M. (1998). The utility of neuropsychological tests in evaluation of Attention-Deficit/ Hyperactivity Disorder (ADHD) versus depression in adults. Assessment. 5, 45–52. doi: 10.1177/107319119800500107
Kautzky, A., Vanicek, T., and Philippe, C. (2020). Machine learning classification of ADHD and HC by multimodal serotonergic data. Translat. Psychiat. 10, 104. doi: 10.1038/s41398-020-0781-2
Kennedy, M., Kreppner, J., Knights, N., Kumsta, R., Maughan, B., Golm, D., et al. (2016). Early severe institutional deprivation is associated with a persistent variant of adult attention-deficit/hyperactivity disorder: clinical presentation, developmental continuities and life circumstances in the English and Romanian Adoptees study. J. Child Psychol. Psychiat. All. Disc. 57, 1113–1125. doi: 10.1111/jcpp.12576
Kessler, D., Angstadt, M., and Sripada, C. (2016). Growth Charting of Brain Connectivity Networks and the Identification of Attention Impairment in Youth. JAMA Psychiat. 73, 481–489. doi: 10.1001/jamapsychiatry.2016.0088
Kim, D. S., Burt, A. A., Ranchalis, J. E., Wilmot, B., Smith, J. D., Patterson, K. E., et al. (2017). of Washington Center for Mendelian G. Sequencing of sporadic Attention-Deficit Hyperactivity Disorder (ADHD) identifies novel and potentially pathogenic de novo variants and excludes overlap with genes associated with autism spectrum disorder. Am. J. Med. Genet. B Neuropsychiatr. Genet. 174, 381–389. doi: 10.1002/ajmg.b.32527
Kim, J. W., Sharma, V., and Ryan, N. D. (2015). Predicting methylphenidate response in ADHD using machine learning approaches. Int. J. Neuropsychopharmacol. 18, pyv052. doi: 10.1093/ijnp/pyv052
Kooijman, M. N., Kruithof, C. J., van Duijn, C. M., Duijts, L., Franco, O. H., van, IMH, et al. (2016). The Generation R Study: design and cohort update 2017. Eur. J. Endocrinol. 31, 1243–1264. doi: 10.1007/s10654-016-0224-9
Lahey, B. B., Pelham, W. E., Loney, J., Lee, S. S., and Willcutt, E. (2005). Instability of the DSM-IV Subtypes of ADHD from preschool through elementary school. Arch. Gen. Psychiat. 62, 896–902. doi: 10.1001/archpsyc.62.8.896
Lambez, B., Harwood-Gross, A., Golumbic, E. Z., and Rassovsky, Y. (2020). Non-pharmacological interventions for cognitive difficulties in ADHD: a systematic review and meta-analysis. J. Psychiatr Res. 120, 40–55. doi: 10.1016/j.jpsychires.2019.10.007
Le, H. H., Hodgkins, P., Postma, M. J., Kahle, J., Sikirica, V., Setyawan, J., et al. (2014). Economic impact of childhood/adolescent ADHD in a European setting: the Netherlands as a reference case. Eur. Child Adolesc. Psychiatry. 23, 587–598. doi: 10.1007/s00787-013-0477-8
Lee, S. H., Song, D. H., Kim, B. N., Joung, Y. S., Ha, E. H., Cheon, K. A., et al. (2009). Variability of response time as a predictor of methylphenidate treatment response in korean children with attention deficit hyperactivity disorder. Yonsei Med. J. 50, 650–655. doi: 10.3349/ymj.2009.50.5.650
Leikauf, J. E. G., Saggar, K. R., Hong, M., Clarke, D. S., Efron, S., Tsang, D., et al. (2017). Identification of biotypes in Attention-Deficit/Hyperactivity Disorder, a report from a randomized, controlled trial. Personal. Med. Psychiat. 3, 8–17. doi: 10.1016/j.pmip.2017.02.001
Lenze, E. J., Rodebaugh, T. L., and Nicol, G. E. (2020). A framework for advancing precision medicine in clinical trials for mental disorders. JAMA Psychiatry. 77, 663–664. doi: 10.1001/jamapsychiatry.2020.0114
Lewis, J., Lipworth, W., and Kerridge, I. (2014). Ethics, evidence and economics in the pursuit of “personalized medicine”. J. Personal. Med. 4, 137–146. doi: 10.3390/jpm4020137
Li, Y., Tang, Y., Liu, B., Long, S., Sun, G., Shen, L., et al. (2005). Electroencephalogram diagnosis and biofeedback treatment for the child with attention deficit hyperactivity disorder. Chin. J. Clin. Rehabilit. 9, 236–237.
Liang, X., Li, R., Wong, S. H., Sum, R. K., and Sit, C. H. (2021). The impact of exercise interventions concerning executive functions of children and adolescents with attention-deficit/hyperactive disorder: a systematic review and meta-analysis. Int. J. Behav. Nutr. Phys. Activ. 18, 68. doi: 10.1186/s12966-021-01135-6
Liechti, M. D., Valko, L., and Müller, U. C. (2013). Diagnostic value of resting electroencephalogram in attention-deficit/hyperactivity disorder across the lifespan. Brain Topogr. 26, 135–151. doi: 10.1007/s10548-012-0258-6
Lim, L., Marquand, A., and Cubillo, A. A. (2013). Disorder-specific predictive classification of adolescents with attention deficit hyperactivity disorder (ADHD) relative to autism using structural magnetic resonance imaging. PLoS ONE 8, e63660. doi: 10.1371/journal.pone.0063660
Lin, H. Y., Cocchi, L., Zalesky, A., Lv, J., Perry, A., Tseng, W. I., et al. (2018). Brain-behavior patterns define a dimensional biotype in medication-naïve adults with attention-deficit hyperactivity disorder. Psychol. Med. 48, 2399–2408. doi: 10.1017/S0033291718000028
Linnet, K. M., Dalsgaard, S., Obel, C., Wisborg, K., Henriksen, T. B., Rodriguez, A., et al. (2003). Maternal lifestyle factors in pregnancy risk of attention deficit hyperactivity disorder and associated behaviors: review of the current evidence. Am. J. Psychiatry. 160, 1028–1040. doi: 10.1176/appi.ajp.160.6.1028
López Villalobos, J. A., Serrano Pintado, I., Sánchez-Mateos, J. D., de Llano, J. M., Sánchez Azón, M. I., and Alberola López, S. (2011). Utility of a statistical model of cognitive styles in attention deficit hyperactivity disorder. Psicothema. 23, 818–823.
Luo, S. X., and Levin, F. R. (2017). Towards precision addiction treatment: new findings in co-morbid substance use and attention-deficit hyperactivity disorders. Curr. Psychiatry Rep. 19, 14. doi: 10.1007/s11920-017-0769-7
Mackie, S., Shaw, P., Lenroot, R., Pierson, R., Greenstein, D. K., Nugent, T. F., et al. (2007). Cerebellar development and clinical outcome in attention deficit hyperactivity disorder. Am. J. Psychiat. 164, 647–655. doi: 10.1176/ajp.2007.164.4.647
Magee, C. A., Clarke, A. R., Barry, R. J., McCarthy, R., and Selikowitz, M. (2005). Examining the diagnostic utility of EEG power measures in children with attention deficit/hyperactivity disorder. Clin. Neurophysiol. 116, 1033–1040. doi: 10.1016/j.clinph.2004.12.007
Mandrekar, S. J., and Sargent, D. J. (2009). Clinical trial designs for predictive biomarker validation: theoretical considerations and practical challenges. J. Clin. Oncol. 27, 4027–4034. doi: 10.1200/JCO.2009.22.3701
Martin, A. R., Kanai, M., Kamatani, Y., Okada, Y., Neale, B. M., and Daly, M. J. (2019). Clinical use of current polygenic risk scores may exacerbate health disparities. Nat. Genet. 51, 584–591. doi: 10.1038/s41588-019-0379-x
Matier-sharma, K. P., Newcorn, J. H., Sharma, V., and Halperin, J. M. (1995). Differential diagnosis of ADHD: Are objective measures of attention, impulsivity, and activity level helpful? Child Neuropsychol. 1, 118–127. doi: 10.1080/09297049508402243
Mazaheri, A., Fassbender, C., Coffey-Corina, S., Hartanto, T. A., Schweitzer, J. B., and Mangun, G. R. (2014). Differential oscillatory electroencephalogram between attention-deficit/hyperactivity disorder subtypes and typically developing adolescents. Biol. Psychiatry. 76, 422–429. doi: 10.1016/j.biopsych.2013.08.023
McLoughlin, G., Gyurkovics, M., Palmer, J., and Makeig, S. (2022). Midfrontal theta activity in psychiatric illness: an index of cognitive vulnerabilities across disorders. Biol. Psychiatry. 91, 173–182. doi: 10.1016/j.biopsych.2021.08.020
Michelini, G., Kitsune, G. L., Cheung, C. H. M., Brandeis, D., Banaschewski, T., Asherson, P., et al. (2016). ADHD remission is linked to better neurophysiological error detection and attention-vigilance processes. Biol. Psychiatry. 80, 923–932. doi: 10.1016/j.biopsych.2016.06.021
Monastra, V. J., Lubar, J. F., and Linden, M. (1999). Assessing attention deficit hyperactivity disorder via quantitative electroencephalography: an initial validation study. Neuropsychology 13, 424–433. doi: 10.1037/0894-4105.13.3.424
Monastra, V. J., Lubar, J. F., and Linden, M. (2001). The development of a quantitative electroencephalographic scanning process for attention deficit-hyperactivity disorder: reliability and validity studies. Neuropsychology 15, 136–44. doi: 10.1037/0894-4105.15.1.136
Monden, Y., Dan, I., and Nagashima, M. (2015). Individual classification of ADHD children by right prefrontal hemodynamic responses during a go/no-go task as assessed by fNIRS. NeuroImage Clin. 9, 1–12. doi: 10.1016/j.nicl.2015.06.011
Mostert, J. C., Hoogman, M., Onnink, A. M. H., van Rooij, D., von Rhein, D., van Hulzen, K. J. E., et al. (2018). similar subgroups based on cognitive performance parse heterogeneity in adults with ADHD and healthy controls. J. Atten. Disord. 22, 281–292. doi: 10.1177/1087054715602332
MTA Cooperative Group (1999). Moderators and mediators of treatment response for children with attention-deficit/hyperactivity disorder: the Multimodal Treatment Study of children with Attention-deficit/hyperactivity disorder. Arch. General Psychiat. 56, 1088–1096. doi: 10.1001/archpsyc.56.12.1088
Myer, N. M., Boland, J. R., and Faraone, S. V. (2017). Pharmacogenetics predictors of methylphenidate efficacy in childhood ADHD. Molec. Psychiat. 23, 1929–36. doi: 10.1038/mp.2017.234
NICE. (2018). Attention Deficit Hyperactivity Disorder: Diagnosis and Management (Nice guideline NG87). National Institute for Health and Care Excellence (NICE).
Nielsen, N. P., and Wiig, E. H. (2011). AQT cognitive speed and processing efficiency differentiate adults with and without ADHD: a preliminary study. Int. J. Psychiat. Clin. Pract. 15, 219–227. doi: 10.3109/13651501.2011.582538
Nigg, J. T., Karalunas, S. L., Feczko, E., and Fair, D. A. (2020). Toward a Revised Nosology for Attention-Deficit/Hyperactivity Disorder Heterogeneity. Biol. Psychiat. Cogn. Neurosci. Neuroimag. 5, 726–737. doi: 10.1016/j.bpsc.2020.02.005
Norman, L. J., Sudre, G., Bouyssi-Kobar, M., Sharp, W., and Shaw, P. (2021). A Longitudinal Study of Resting-State Connectivity and Response to Psychostimulant Treatment in ADHD. Am. J. Psychiatry. 178, 744–751. doi: 10.1176/appi.ajp.2021.20091342
Ogrim, G., Kropotov, J., and Hestad, K. (2012). The quantitative EEG theta/beta ratio in attention deficit/hyperactivity disorder and normal controls: sensitivity, specificity, and behavioral correlates. Psychiatry Res. 198, 482–488. doi: 10.1016/j.psychres.2011.12.041
Oliva, F., Malandrone, F., di Girolamo, G., Mirabella, S., Colombi, N., Carletto, S., et al. (2021). The efficacy of mindfulness-based interventions in attention-deficit/hyperactivity disorder beyond core symptoms: a systematic review, meta-analysis, and meta-regression. J. Affect. Disord. 292, 475–486. doi: 10.1016/j.jad.2021.05.068
Owens, E. B., Hinshaw, S. P., Kraemer, H. C., Arnold, L. E., Abikoff, H. B., Cantwell, D. P., et al. (2003). Which treatment for whom for ADHD? Moderators of treatment response in the MTA. J. Consult. Clin. Psychol. 71, 540–552. doi: 10.1037/0022-006X.71.3.540
Perna, G., Grassi, M., Caldirola, D., and Nemeroff, C. B. (2018). The revolution of personalized psychiatry: will technology make it happen sooner? Psychol. Med. 48, 705–713. doi: 10.1017/S0033291717002859
Polanczyk, G., de Lima, M. S., Horta, B. L., Biederman, J., and Rohde, L. A. (2007). The worldwide prevalence of ADHD: a systematic review and metaregression analysis. Am. J. Psychiat. 164, 942–948. doi: 10.1176/ajp.2007.164.6.942
Proal, E., Reiss, P. T., Klein, R. G., Mannuzza, S., Gotimer, K., Ramos-Olazagasti, M. A., et al. (2011). Brain gray matter deficits at 33-year follow-up in adults with attention-deficit/hyperactivity disorder established in childhood. Arch. General Psychiatry. 68, 1122–1134. doi: 10.1001/archgenpsychiatry.2011.117
Quaak, M., van de Mortel, L., Thomas, R. M., and van Wingen, G. (2021). Deep learning applications for the classification of psychiatric disorders using neuroimaging data: systematic review and meta-analysis. NeuroImage Clin. 30, 102584. doi: 10.1016/j.nicl.2021.102584
Quinn, C. A. (2003). Detection of malingering in assessment of adult ADHD. Arch. Clin. Neuropsychol. 18, 379–395. doi: 10.1093/arclin/18.4.379
Quintana, H., Snyder, S. M., Purnell, W., Aponte, C., and Sita, J. (2007). Comparison of a standard psychiatric evaluation to rating scales and EEG in the differential diagnosis of attention-deficit/hyperactivity disorder. Psychiatry Res 152, 211–222. doi: 10.1016/j.psychres.2006.04.015
Reichow, B., Volkmar, F. R., and Bloch, M. H. (2013). Systematic review and meta-analysis of pharmacological treatment of the symptoms of attention-deficit/hyperactivity disorder in children with pervasive developmental disorders. J. Autism Develop. Diso. 43, 2435–2441. doi: 10.1007/s10803-013-1793-z
Rice, F., Langley, K., Woodford, C., Davey Smith, G., and Thapar, A. (2018). Identifying the contribution of prenatal risk factors to offspring development and psychopathology: What designs to use and a critique of literature on maternal smoking and stress in pregnancy. Develop. Psychopathol. 30, 1107–1128. doi: 10.1017/S0954579418000421
Riglin, L., Collishaw, S., Thapar, A. K., Dalsgaard, S., Langley, K., Smith, G. D., et al. (2016). Association of genetic risk variants with attention-deficit/hyperactivity disorder trajectories in the general population. JAMA Psychiatry. 73, 1285–1292. doi: 10.1001/jamapsychiatry.2016.2817
Riglin, L., Wootton, R. E., Livingston, L. A., Agnew-Blais, J., Arseneault, L., Blakey, R., et al. (2022). “Late-onset” ADHD symptoms in young adulthood: Is this ADHD? J Atten Disord. doi: 10.1177/10870547211066486
Robison, R. J., Reimherr, F. W., Gale, P. D., Marchant, B. K., Williams, E. D., Soni, P., et al. (2010). Personality disorders in ADHD Part 2: The effect of symptoms of personality disorder on response to treatment with OROS methylphenidate in adults with ADHD. Ann. Clin. Psychiat. 22, 94–102.
Ronald, A., de Bode, N., and Polderman, T. J. C. (2021). Systematic review: how the attention-deficit/hyperactivity disorder polygenic risk score adds to our understanding of ADHD and associated traits. J. Am. Acad. Child. Adolesc. Psychiatry. 60, 1234–1277. doi: 10.1016/j.jaac.2021.01.019
Russell, A. E., Ford, T., Williams, R., and Russell, G. (2016). The Association Between Socioeconomic Disadvantage and Attention Deficit/Hyperactivity Disorder (ADHD): A Systematic Review. Child Psychiat Human Develop. 47, 440–458. doi: 10.1007/s10578-015-0578-3
Rutter, M., Kreppner, J., Croft, C., Murin, M., Colvert, E., Beckett, C., et al. (2007). Early adolescent outcomes of institutionally deprived and non-deprived adoptees. III. Quasi-autism. J. Child Psychol. Psychiat. All. Disc. 48, 1200–1207. doi: 10.1111/j.1469-7610.2007.01792.x
Scassellati, C., Bonvicini, C., Faraone, S. V., and Gennarelli, M. (2012). Biomarkers and attention-deficit/hyperactivity disorder: a systematic review and meta-analyses. J. Am. Acad. Child Adolesc. Psychiat. 51, 1003–1019.e1020. doi: 10.1016/j.jaac.2012.08.015
Schatz, N. K., Fabiano, G. A., Cunningham, C. E., dosReis, S., Waschbusch, D. A., Jerome, S., et al. (2015). Systematic review of patients' and parents' preferences for ADHD treatment options and processes of care. Patient. 8, 483–497. doi: 10.1007/s40271-015-0112-5
Schulz, K. P., Bedard, A. V., Fan, J., Hildebrandt, T. B., Stein, M. A., Ivanov, I., et al. (2017). Striatal activation predicts differential therapeutic responses to methylphenidate and atomoxetine. J. Am. Acad. Child Adolesc. Psychiat. 56, 602–609.e602. doi: 10.1016/j.jaac.2017.04.005
Schumann, G., Loth, E., Banaschewski, T., Barbot, A., Barker, G., Buchel, C., et al. (2010). The IMAGEN study: reinforcement-related behaviour in normal brain function and psychopathology. Molec. Psychiatry. 15, 1128–1139. doi: 10.1038/mp.2010.4
Schweren, L. J., Hartman, C. A., Zwiers, M. P., Heslenfeld, D. J., Franke, B., Oosterlaan, J., et al. (2016). Stimulant treatment history predicts frontal-striatal structural connectivity in adolescents with attention-deficit/hyperactivity disorder. Eur. Neuropsychopharmacol. 26, 674–683. doi: 10.1016/j.euroneuro.2016.02.007
Sciberras, E., Mulraney, M., Silva, D., and Coghill, D. (2017). Prenatal risk factors and the etiology of ADHD-review of existing evidence. Curr. Psychiatry Rep. 19, 1. doi: 10.1007/s11920-017-0753-2
Sen, B., Borle, N. C., Greiner, R., and Brown, M. R. G. (2018). A general prediction model for the detection of ADHD and Autism using structural and functional MRI. PLoS ONE 13, e0194856. doi: 10.1371/journal.pone.0194856
Shaw, P., Malek, M., Watson, B., Greenstein, D., de Rossi, P., and Sharp, W. (2013). Trajectories of cerebral cortical development in childhood and adolescence and adult attention-deficit/hyperactivity disorder. Biol. Psychiat. 74, 599–606. doi: 10.1016/j.biopsych.2013.04.007
Sibley, M. H., Rohde, L. A., Swanson, J. M., Hechtman, L. T., Molina, B. S. G., Mitchell, J. T., et al. (2018). Reconsidered with comprehensive repeated assessments between ages 10 and 25. Am. J. Psychiatry. 175, 140–149. doi: 10.1176/appi.ajp.2017.17030298
Sidhu, G. S., Asgarian, N., Greiner, R., and Brown, M. R. (2012). Kernel Principal Component Analysis for dimensionality reduction in fMRI-based diagnosis of ADHD. Front. Syst. Neurosci. 6, 74. doi: 10.3389/fnsys.2012.00074
Snyder, J. A. (2015). The link between ADHD and the risk of sexual victimization among college women: expanding the lifestyles/routine activities framework. Violence Against Women. 21, 1364–1384. doi: 10.1177/1077801215593647
Snyder, S. M., Quintana, H., Sexson, S. B., Knott, P., Haque, A. F., and Reynolds, D. A. (2008). Blinded, multi-center validation of EEG and rating scales in identifying ADHD within a clinical sample. Psychiatry Res. 159, 346–358. doi: 10.1016/j.psychres.2007.05.006
Snyder, S. M., Rugino, T. A., Hornig, M., and Stein, M. A. (2015). Integration of an EEG biomarker with a clinician's ADHD evaluation. Brain Behav. 5, e00330. doi: 10.1002/brb3.330
Solanto, M., Newcorn, J., Vail, L., Gilbert, S., Ivanov, I., and Lara, R. (2009). Stimulant drug response in the predominantly inattentive and combined subtypes of attention-deficit/hyperactivity disorder. J. Child Adolesc. Psychopharmacol. 19, 663–671. doi: 10.1089/cap.2009.0033
Solanto, M. V., Etefia, K., and Marks, D. J. (2004). The utility of self-report measures and the continuous performance test in the diagnosis of ADHD in adults. CNS Spectrums 9, 649–659. doi: 10.1017/S1092852900001929
Soliva, J. C., Fauquet, J., and Bielsa, A. (2010). Quantitative MR analysis of caudate abnormalities in pediatric ADHD: proposal for a diagnostic test. Psychiatry Res. 182, 238–243. doi: 10.1016/j.pscychresns.2010.01.013
Sonuga-Barke, E. J., Brandeis, D., Cortese, S., Daley, D., Ferrin, M., Holtmann, M., et al. (2013). Nonpharmacological interventions for ADHD: systematic review and meta-analyses of randomized controlled trials of dietary and psychological treatments. Am. J. Psychiatry. 170, 275–289. doi: 10.1176/appi.ajp.2012.12070991
Sonuga-Barke, E. J., Coghill, D., Markowitz, J. S., Swanson, J. M., Vandenberghe, M., and Hatch, S. J. (2007). Sex differences in the response of children with ADHD to once-daily formulations of methylphenidate. J. Am. Acad. Child Adolesc. Psychiat. 46, 701–710. doi: 10.1097/chi.0b013e31804659f1
Stage, C., Jurgens, G., Guski, L. S., Thomsen, R., Bjerre, D., Ferrero-Miliani, L., et al. (2017). The impact of CES1 genotypes on the pharmacokinetics of methylphenidate in healthy Danish subjects. Br. J. Clin. Pharmacol. 83, 1506–1514. doi: 10.1111/bcp.13237
Stergiakouli, E., Martin, J., Hamshere, M. L., Langley, K., Evans, D. M., St Pourcain, B., et al. (2015). Shared genetic influences between attention-deficit/hyperactivity disorder (ADHD) traits in children and clinical ADHD. J. Am. Acad. Child Adolesc. Psychiat. 54, 322–327. doi: 10.1016/j.jaac.2015.01.010
Stevenson, J., Buitelaar, J., Cortese, S., Ferrin, M., Konofal, E., Lecendreux, M., et al. (2014). Research review: the role of diet in the treatment of attention-deficit/hyperactivity disorder–an appraisal of the evidence on efficacy and recommendations on the design of future studies. J. Child Psychol. Psychiatry. 55, 416–427. doi: 10.1111/jcpp.12215
Storebo, O. J., Ramstad, E., Krogh, H. B., Nilausen, T. D., Skoog, M., Holmskov, M., et al. (2015). Methylphenidate for children and adolescents with attention deficit hyperactivity disorder (ADHD). Cochrane Datab. System. Rev. 11, Cd009885. doi: 10.1002/14651858.CD009885.pub2
Studerus, E., Corbisiero, S., and Mazzariello, N. (2018). Can neuropsychological testing facilitate differential diagnosis between at-risk mental state (ARMS) for psychosis and adult attention-deficit/hyperactivity disorder (ADHD)? Eur. Psychiatr. 52, 38–44. doi: 10.1016/j.eurpsy.2018.02.006
Sudlow, C., Gallacher, J., Allen, N., Beral, V., Burton, P., Danesh, J., et al. (2015). UK biobank: an open access resource for identifying the causes of a wide range of complex diseases of middle and old age. PLoS Med. 12, e1001779. doi: 10.1371/journal.pmed.1001779
Swift, J. K., Callahan, J. L., and Vollmer, B. M. (2011). Preferences. J. Clin. Psychol. 67, 155–165. doi: 10.1002/jclp.20759
Taylor, L. E., Kaplan-Kahn, E. A., Lighthall, R. A., and Antshel, K. M. (2021). Adult-Onset ADHD: a critical analysis and alternative explanations. Child Psychiatry Hum Dev. doi: 10.1007/s10578-021-01159-w
Thapar, A., Cooper, M., Eyre, O., and Langley, K. (2013). What have we learnt about the causes of ADHD? J. Child Psychol. Psychiat. Allied Disc. 54, 3–16. doi: 10.1111/j.1469-7610.2012.02611.x
Thomson, J. B., and Varley, C. K. (1998). Prediction of stimulant response in children with attention-deficit/hyperactivity disorder. J. Child Adolesc. Psychopharmacol. 8, 125–132. doi: 10.1089/cap.1998.8.125
Unal, M., O'Mahony, E., Dunne, C., Meagher, D., and Adamis, D. (2019). The clinical utility of three visual attention tests to distinguish adults with ADHD from normal controls. Rivista di psichiatria 54, 211–217.
van den Hoofdakker, B. J., Nauta, M. H., van der Veen-Mulders, L., Sytema, S., Emmelkamp, P. M., Minderaa, R. B., et al. (2010). Behavioral parent training as an adjunct to routine care in children with attention-deficit/hyperactivity disorder: moderators of treatment response. J. Pediatric Psychol. 35, 317–326. doi: 10.1093/jpepsy/jsp060
van der Donk, M. L., Hiemstra-Beernink, A. C., Tjeenk-Kalff, A. C., van der Leij, A. V., and Lindauer, R. J. (2013). Interventions to improve executive functioning and working memory in school-aged children with AD(H)D: a randomised controlled trial and stepped-care approach. BMC Psychiatry. 13, 23. doi: 10.1186/1471-244X-13-23
van der Meer, J. M., Oerlemans, A. M., van Steijn, D. J., Lappenschaar, M. G., de Sonneville, L. M., Buitelaar, J. K., et al. (2012). Are autism spectrum disorder and attention-deficit/hyperactivity disorder different manifestations of one overarching disorder? Cognitive and symptom evidence from a clinical and population-based sample. J. Am. Acad. Child. Adolesc. Psychiatry. 51, 1160–1172.e1163. doi: 10.1016/j.jaac.2012.08.024
van der Meer, J. M. J., Lappenschaar, M. G. A., Hartman, C. A., Greven, C. U., Buitelaar, J. K., and Rommelse, N. N. J. (2017). Homogeneous Combinations of ASD-ADHD traits and their cognitive and behavioral correlates in a population-based sample. J Atten. Disord. 21, 753–763. doi: 10.1177/1087054714533194
van Hulst, B. M., de Zeeuw, P., and Durston, S. (2015). Distinct neuropsychological profiles within ADHD: a latent class analysis of cognitive control, reward sensitivity and timing. Psychol. Med. 45, 735–745. doi: 10.1017/S0033291714001792
van Lieshout, M., Luman, M., Twisk, J. W., Faraone, S. V., Heslenfeld, D. J., Hartman, C. A., et al. (2017). Neurocognitive Predictors of ADHD outcome: a 6-year follow-up study. J. Abnorm. Child. Psychol. 45, 261–272. doi: 10.1007/s10802-016-0175-3
Voetterl, H., van Wingen, G., Michelini, G., Griffiths, K. R., Gordon, E., DeBeus, R., et al. (2022). Brainmarker-I differentially predicts remission to various attention-deficit/hyperactivity disorder treatments: a blinded discovery, transfer and validation study. Biol. Psychiat. Cogn. Neurosci. Neuroimag. doi: 10.1016/j.bpsc.2022.02.007
WHO. (1992). The ICD-10 Classification of Mental and Behavioral Disorders: Clinical Descriptions and Diagnostic Guidelines. Geneva, WHO.
Wiig, E. H., and Nielsen, N. P. (2012). A quick test of cognitive speed for comparing processing speed to differentiate adult psychiatric referrals with and without attention-deficit/hyperactivity disorders. Primary Care Compan. CNS Diso. 14. doi: 10.4088/PCC.11m01273
Wium-Andersen, I. K., Vinberg, M., Kessing, L. V., and McIntyre, R. S. (2017). Personalized medicine in psychiatry. Nordic J. Psychiat. 71, 12–19. doi: 10.1080/08039488.2016.1216163
Wolfers, T., Buitelaar, J. K., Beckmann, C. F., Franke, B., and Marquand, A. F. (2015). From estimating activation locality to predicting disorder: A review of pattern recognition for neuroimaging-based psychiatric diagnostics. Neurosci. Biobehav. Rev. 57, 328–349. doi: 10.1016/j.neubiorev.2015.08.001
Wong, H. K., Tiffin, P. A., Chappell, M. J., Nichols, T. E., Welsh, P. R., Doyle, O. M., et al. (2017). Personalized medication response prediction for attention-deficit hyperactivity disorder: learning in the model space vs. learning in the data space. Front. Physiol. 8, 199. doi: 10.3389/fphys.2017.00199
Xu, Y., Chen, X. T., Luo, M., Tang, Y., Zhang, G., Wu, D., et al. (2015). Multiple epigenetic factors predict the attention deficit/hyperactivity disorder among the Chinese Han children. J. Psychiatr. Res. 64, 40–50. doi: 10.1016/j.jpsychires.2015.03.006
Yang, K. H., Lane, H. Y., Chang, Y. C., and Tzang, R. F. (2021). Exploring the effects of pharmacological, psychosocial, and alternative/complementary interventions in children and adolescents with attention-deficit/hyperactivity disorder: meta-regression approach. Int. J. Neuropsychopharmacol. 24, 776–786. doi: 10.1093/ijnp/pyab034
Young, E. S., Perros, P., Price, G. W., and Sadler, T. (1995). Acute challenge ERP as a prognostic of stimulant therapy outcome in attention-deficit hyperactivity disorder. Biol. Psychiatry. 37, 25–33. doi: 10.1016/0006-3223(94)00075-E
Yu, G., Li, G. F., and Markowitz, J.S. (2016). Atomoxetine: a review of its pharmacokinetics and pharmacogenomics relative to drug disposition. J. Child Adolesc Psychopharmacol. 26, 314–326. doi: 10.1089/cap.2015.0137
Zelnik, N., Bennett-Back, O., Miari, W., Goez, H. R., and Fattal-Valevski, A. (2012). Is the test of variables of attention reliable for the diagnosis of attention-deficit hyperactivity disorder (ADHD)? J. Child Neurol. 27, 703–707. doi: 10.1177/0883073811423821
Zhang-James, Y., Helminen, E. C., Liu, J., ENIGMA-ADHD Working Group Franke, B., Hoogman, M., et al. (2021). Evidence for similar structural brain anomalies in youth and adult attention-deficit/hyperactivity disorder: a machine learning analysis. Transl. Psychiatry 11:82. doi: 10.1038/s41398-021-01201-4
Keywords: Attention-Deficit Hyperactivity Disorder (ADHD), precision medicine, biomarker, heterogeneity, inter-individual variability
Citation: Buitelaar J, Bölte S, Brandeis D, Caye A, Christmann N, Cortese S, Coghill D, Faraone SV, Franke B, Gleitz M, Greven CU, Kooij S, Leffa DT, Rommelse N, Newcorn JH, Polanczyk GV, Rohde LA, Simonoff E, Stein M, Vitiello B, Yazgan Y, Roesler M, Doepfner M and Banaschewski T (2022) Toward Precision Medicine in ADHD. Front. Behav. Neurosci. 16:900981. doi: 10.3389/fnbeh.2022.900981
Received: 21 March 2022; Accepted: 16 May 2022;
Published: 06 July 2022.
Edited by:
Salman Zubedat, Technion Israel Institute of Technology, IsraelReviewed by:
Katherine A. Johnson, The University of Melbourne, AustraliaBrandy L. Callahan, University of Calgary, Canada
Copyright © 2022 Buitelaar, Bölte, Brandeis, Caye, Christmann, Cortese, Coghill, Faraone, Franke, Gleitz, Greven, Kooij, Leffa, Rommelse, Newcorn, Polanczyk, Rohde, Simonoff, Stein, Vitiello, Yazgan, Roesler, Doepfner and Banaschewski. This is an open-access article distributed under the terms of the Creative Commons Attribution License (CC BY). The use, distribution or reproduction in other forums is permitted, provided the original author(s) and the copyright owner(s) are credited and that the original publication in this journal is cited, in accordance with accepted academic practice. No use, distribution or reproduction is permitted which does not comply with these terms.
*Correspondence: Jan Buitelaar, SmFuLkJ1aXRlbGFhckByYWRib3VkdW1jLm5s