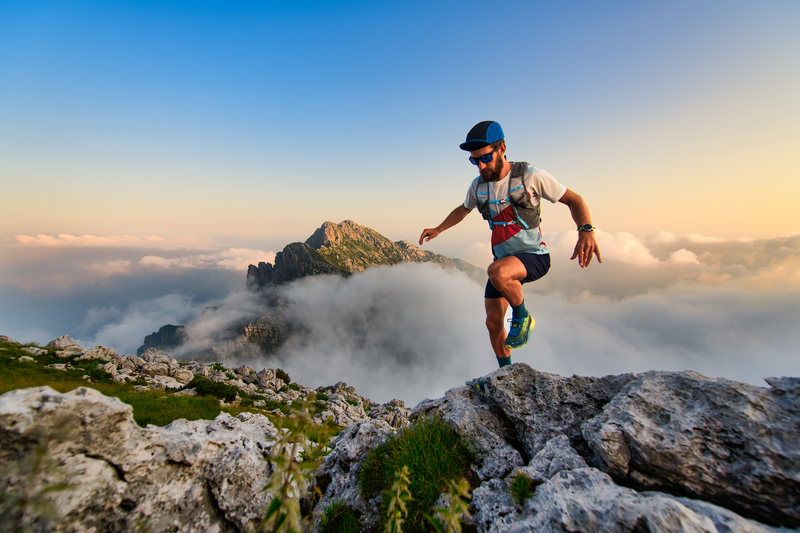
94% of researchers rate our articles as excellent or good
Learn more about the work of our research integrity team to safeguard the quality of each article we publish.
Find out more
SYSTEMATIC REVIEW article
Front. Aging Neurosci. , 25 October 2021
Sec. Parkinson’s Disease and Aging-related Movement Disorders
Volume 13 - 2021 | https://doi.org/10.3389/fnagi.2021.716102
This article is part of the Research Topic Technological Advancements in Aging and Neurological Conditions to Improve Physical Activity, Cognitive Functions, and Postural Control View all 15 articles
Assessing the progression of movement disorders such as Parkinson's Disease (PD) is key in adjusting therapeutic interventions. However, current methods are still based on subjective factors such as visual observation, resulting in significant inter-rater variability on clinical scales such as UPDRS. Recent studies show the potential of sensor-based methods to address this limitation. The goal of this systematic review is to provide an up-to-date analysis of contactless sensor-based methods to estimate hand dexterity UPDRS scores in PD patients. Two hundred and twenty-four abstracts were screened and nine articles selected for analysis. Evidence obtained in a cumulative cohort of n = 187 patients and 1, 385 samples indicates that contactless sensors, particularly the Leap Motion Controller (LMC), can be used to assess UPDRS hand motor tasks 3.4, 3.5, 3.6, 3.15, and 3.17, although accuracy varies. Early evidence shows that sensor-based methods have clinical potential and might, after refinement, complement, or serve as a support to subjective assessment procedures. Given the nature of UPDRS assessment, future studies should observe whether LMC classification error falls within inter-rater variability for clinician-measured UPDRS scores to validate its clinical utility. Conversely, variables relevant to LMC classification such as power spectral densities or movement opening and closing speeds could set the basis for the design of more objective expert systems to assess hand dexterity in PD.
Parkinson's Disease (PD) is a movement disorder caused by the degeneration of the dopaminergic neurons of the substantia nigra pars compacta, a reduction of striatal dopamine, and is characterized by the potential presence of Lewy bodies (Jameson, 2018). PD requires constant monitoring to track progression and perform therapeutic adjustments. Monitoring is currently performed with questionnaires such as the Unified PD Rating Scale (UPDRS) (Goetz et al., 2008).
UPDRS rates different aspects of PD through visual observation of a series of tasks. These tasks are designed to monitor, among others, the most important symptoms of PD, also known as cardinal signs: resting tremors, asymmetry, bradykinesia, and a positive response to dopaminergic replacement therapy. In the case of hand dexterity, these tasks are related to bradykinesia and hand tremors, performing tasks such as finger tapping. UPDRS then rates these tasks on scales from zero (no symptoms) to four (patient is unable to perform the task), through visual observation. While the criteria to identify zeroes and fours are mostly clear, intermediate scores are considerably more ambiguous, which irrevocably leads to sensibility and reliability problems (Patrick et al., 2001). UPDRS is commonly complemented with patient diaries, which, although helpful, can also be biased by the subjective view of the patient (Hauser et al., 2004). The fact that this ambiguity introduces variability in assessments is well-documented (Meara et al., 1999; Patrick et al., 2001). Furthermore, the relationship between PD and similar conditions also accompanied by hand tremor, such as Essential Tremor (ET), is still unclear (Jimenez-Jimenez et al., 2012).
A solution to minimize subjectivity is to introduce sensor-based measurements (Chaudhry et al., 2006), which provide a reproducible and objective assessment of hand tremor and bradykinesia. The Leap Motion Controller (LMC) has been proposed for this task (Garcia-Agundez et al., 2019). Capturing hand movements via contactless sensors has the potential to reduce ambiguity, providing neurologists with more objective assessments of hand dexterity that may lead to more accurate therapeutic adjustments. At the same time, variables that provide meaningful information for the estimation of UPDRS scores could be used to establish more objective assessment scales, allowing for a finer resolution in hand dexterity assessment and better adjusted pharmacologic therapies.
The goal of this article is to provide a systematic review of recent advances in hand dexterity assessment using contactless sensors in PD patients, in the domains of hand tremor and bradykinesia. This review aims to provide further insight into the feasibility and reliability of this paradigm, as well as suggesting best practice guidelines for both engineers and clinicians on how to proceed from this point.
As a basis for this systematic review, we searched the databases Pubmed, ScienceDirect, IEEE Xplore, and Cochrane for articles matching the search query:
(Parkinson OR Tremor) AND (Leap Motion OR Contactless OR Infared OR Lidar)
on March 31, 2021. This search yielded the following results:
• 28 matches in Pubmed
• 168 in ScienceDirect, including 10 duplicates
• 18 in IEEE Xplore, including 5 duplicates
• 3 in Cochrane, including 3 duplicates
The search was complemented by seven additional articles selected from the references of search matches, yielding 224 abstracts for screening. The abstracts of these matches were filtered according to the following criteria:
1. Research articles
2. Related to PD
3. Related to hand tremor or bradykinesia
This filtering reduced the abstracts to 47 full-text articles assessed for eligibility. These full-text articles were selected for analysis if they met the following inclusion criteria:
1. Articles presenting a method to measure hand tremor or bradykinesia using a contactless approach
2. In patients with PD
3. Aiming to link sensor data to clinical functional performance scores (MDS-UPDRS-III or similar)
Conversely, articles were excluded if they met at least one of the following exclusion criteria:
1. Articles not related to hand tremor or bradykinesia. Thirteen articles were excluded with this criterion.
2. Articles without participants (technical or otherwise conceptual papers). Seven further exclusions.
3. Articles aiming to test a novel rehabilitation tool or otherwise not linking sensor data to clinical functional performance scores. Nine further exclusions.
4. Articles not using contactless sensors. Three further exclusions.
5. Articles aiming to classify between PD patients and controls exclusively and not to assess symptom severity. Six further exclusions.
Finally resulting in n=9 articles for the qualitative and quantitative analysis (Khan et al., 2014; Butt et al., 2017, 2018; Lugo et al., 2017; Cakmak et al., 2018; Lee et al., 2019; Vivar et al., 2019; Williams et al., 2020a,b). Of the selected articles, six were first identified in the Pubmed search, one in ScienceDirect, and two were selected from the additional articles. This procedure was conducted in accordance with the PRISMA guidelines. A.G. was responsible for the selection and data collection process. The following data were sought from the articles: cohort data, procedure data (assessment method, sensor implementation), classification data, and classification accuracy. No studies are clinical trials and no bias assessment was conducted. The PRISMA flow diagram is included in Figure 1.
All identified articles use some form of video source, including hand detection and tracking. Six of the nine articles use the LMC (Butt et al., 2017, 2018; Lugo et al., 2017; Cakmak et al., 2018; Lee et al., 2019; Vivar et al., 2019), while three use other video sources (Khan et al., 2014; Williams et al., 2020a,b). Essentially, all studies follow the same structure: given a dataset of PD patients performing a certain MDS-UPDRS III task (e.g., finger tapping) rated by one or more neurologists and captured with a sensor, the resulting task score (or a linear regression model) is inferred using points of interest of the hand, defined by a series of features, with a classification method, as depicted in Figure 2.
The identified studies implement one or more of the following UPDRS specific tasks:
• Task 3.4, Finger Tapping: The patient taps the index finger on the thumb 10 times as quickly and as big as possible. Out of the nine identified studies, seven analyse this task (Khan et al., 2014; Butt et al., 2017, 2018; Cakmak et al., 2018; Lee et al., 2019; Williams et al., 2020a,b).
• Task 3.5, Hand Movements: The patient makes a tight fist, then opens the hand 10 times as fully and as quickly as possible. Out of the nine studies, three analyse this task (Butt et al., 2017, 2018; Lee et al., 2019).
• Task 3.6, Pronation-Supination: The patient extends the arm with the palm down, then runs the palm up and down alternately 10 times as fast and as fully as possible. The three same studies as above analyse this task (Butt et al., 2017, 2018; Lee et al., 2019).
• Task 3.15, Postural Tremor: The patient stretches the arm with the palms down. Tremor in this posture is observed for 10 s. Out of the nine studies, four analyse this task (Butt et al., 2017, 2018; Lugo et al., 2017; Vivar et al., 2019).
• Task 3.17, Kinetic Tremor: The patient performs at least three finger-to-nose maneuvers. Tremor in this movement is observed. Out of the nine studies, two analyse this task (Lugo et al., 2017; Vivar et al., 2019).
Table 1 summarizes the implemented tasks and signal of interest of each study. With the exception of task 3.5, the choice of hand region of interest for each task is consistent.
Table 2 presents the study devices, cohorts, total number, and type of samples, as well as the main study goals. The studies either aim to predict the UPDRS rating of a given sample (Khan et al., 2014; Lugo et al., 2017; Vivar et al., 2019; Williams et al., 2020a) or build a linear regression model relating variables extracted from the signals described in Table 1 and UPDRS scores (Butt et al., 2017, 2018; Cakmak et al., 2018; Lee et al., 2019; Williams et al., 2020b). In this study, we refer to sample as an instance of either hand of a PD patient performing a UPDRS task. Cumulatively, the nine identified studies have a cohort of n = 187 patients and 1, 385 samples.
In the following, we divide the qualitative analysis into two subsections. Section 3.1 compares the results of studies that aim to evaluate the scores of UPDRS tasks related to tremor, 3.15 (Postural Tremor) and/or 3.16 (Kinetic Tremor), while section 3.2 compares the results of studies that aim to evaluate bradykinesia with Tasks 3.4 (Finger Tapping), 3.5 (Hand Movements), and 3.6 (Pronation-Supination).
Four studies aimed to assess hand tremor in PD using contactless sensors (Butt et al., 2017, 2018; Lugo et al., 2017; Vivar et al., 2019). All studies used the LMC and either the center of the palm (Lugo et al., 2017; Vivar et al., 2019) or changes in fingertip velocity (Butt et al., 2017, 2018). Butt et al. suggest the use of a 14 Hz lowpass filter, which should not affect the detection of Parkinsonian tremors. The studies also differed greatly in the choice of variables, as well as in the resulting accuracy, if classification was attempted.
Vivar et al. (2019) proposed the use of histogram-based variables, computing an addition and subtraction of data points within a sliding window of 449 samples that advances through the data. Standard features are then computed from these histograms, with contrast and homogeneity providing the best performance. This yielded the best performance in this task group, with an accuracy over 97% classifying scores of 0, 1, and 2.
Lugo et al. (2017) performed a similar study, using a significantly shorter windowing of 15 frames, as well as a different choice of variables. The resulting performance was worse at 74%, albeit the sample size was larger and a patient with a score of 3 on both hands was included.
Finally, Butt et al. (2017, 2018) did not aim to estimate UPDRS scores but rather find variables correlated with said scores. The first study found no correlations between the chosen variables (signal strength and power in the 8–12 Hz band). The second study used the same variables and identified a correlation of R = 0.59 with signal strength.
Table 3 summarizes the differences in these studies. Overall, data indicate that detecting resting tremor is feasible, but kinetic tremor is more difficult to identify.
Seven studies aimed to assess at least one UPDRS task related to bradykinesia using contactless sensors (Khan et al., 2014; Butt et al., 2017, 2018; Cakmak et al., 2018; Lee et al., 2019; Williams et al., 2020a,b). These studies used a mixture of LMC and video, and differed greatly in choice of signals and variables. As all of these studies implemented Task 3.4 (Finger Tapping) but only three included additional tasks (Butt et al., 2017, 2018; Lee et al., 2019). Table 4 summarizes the results for all tasks. The following subsections offer a detailed analysis of each task.
With the exception of Khan et al. (2014), all studies used the Euclidean distance between the tip of the index finger and the thumb as signal of interest. All studies are also reasonably consistent in the choice of variables: number of repetitions, amplitudes, variability of amplitude (particularly a decrease in amplitude with subsequent repetitions), speeds (generally considered as opening and closing speeds separately), accelerations, and frequency domain analysis. We can divide these seven studies into two groups: two that classify UPDRS scores (Khan et al., 2014; Williams et al., 2020a) and five that use linear regression instead (Butt et al., 2017, 2018; Cakmak et al., 2018; Lee et al., 2019; Williams et al., 2020b).
The two studies aiming at classification (Khan et al., 2014; Williams et al., 2020a) used video instead of a LMC. Interestingly, the resolution and frequency employed by Khan et al. (2014) is significantly lower, with a smaller number of participants but a significantly larger number of samples and a more complex classification task, as they aim to classify ternary scores of 0, 1, and 2 instead of classifying scores binarily as ≤ 1 vs. >1 (Williams et al., 2020a). Both obtained the best results when using support vector machines, with overall accuracies of 82% for Khan (Khan et al., 2014) and 84% for Williams (Williams et al., 2020a).
The remaining five studies used linear regression (Butt et al., 2017, 2018; Cakmak et al., 2018; Lee et al., 2019; Williams et al., 2020b). Some, but not all studies report the correlation of each of the variables individually. Overall, correlated variables fall within the [0.5, 0.6] range, with Butt et al. (2018) reporting significantly higher correlations for opening (R = 0.836) and closing (R = 0.804) speeds. Table 5 provides a direct comparison of the correlations of these studies. Overall, data indicate that assessing UPDRS scores with video is feasible, and opening and closing speeds show good correlations with UPDRS scores.
Concerning Task 3.5 (Hand Movements), no classification has been implemented yet. Lee et al. (2019) explored the correlation of a 120 Hz linearly interpolated signal analyzed through amplitudes, frequencies, velocities, and slopes. The number of participants was small (eight), but a large number of samples was collected by measuring with and without deep brain stimulation. They employed the angle between the fingers and the palm as signal of interest. As they did not explore the regression coefficients on each task individually but rather build a global linear regression model, only the velocity of Task 3.5 is reported as showing a relevant correlation of R = 0.69.
Butt et al. (2017, 2018) also implemented this task in their two studies, using the Euclidean distance between palm and fingertips. Again employing a 14 Hz lowpass filter, they explored a very similar set of variables, using number of repetitions, speeds, the variability of frequency and amplitude, and power spectral density. They do report the individual correlation of each of the explored variables, showing significant correlations in most variables. Interestingly, the correlations vary substantially between both studies.
Table 5 offers a comparison between the correlations of these three studies. Overall, data indicate good correlations for opening and closing speeds. No study has attempted to classify UPDRS scores so far.
The same three studies as in the previous subsection implemented Task 3.6, using the same variables as in the previous task but focusing on a different point of the hand, the roll angle of the palm. All three studies report worse results with Task 3.6, as summarized in Table 5. Overall, data only shows good correlations for amplitude and variability of amplitude. No study has attempted to classify UPDRS scores so far.
In this systematic review, we analyzed recent advances in sensor-based, UPDRS-inspired tremor and bradykinesia assessment in PD patients.
Concerning tremor, it seems that the coordinates of the palm center are a good predictor of UPDRS scores. Larger windows as well as statistical variables seem to be a better choice. Although the studies did not include patients with higher scores (three and four) classifying these should be easier as tremor is expected to be more severe. Although the limited number of studies does not yield definite conclusions, it would seem that classifying tremor UPDRS scores is nearly as accurate as classifying PD patients and healthy controls.
Figure 3 summarizes the number of samples, studies and sample-weighted correlations of all UPDRS bradykinesia tasks. The number of repetitions, opening and closing speeds, combined with changes in amplitude as the task progresses, seem to best characterize the rating in Task 3.4 (Finger Tapping). Implemented classification schemes in this scenario can already achieve excellent results, with accuracies over 80% when discriminating scores of 0, 1, and 2. As is the case with tremor, including higher scores would probably not decrease accuracy as these represent patients that are either almost (3) or fully (4) incapable of performing the task.
Figure 3. Number of samples and (number of articles) using different variables and UPDRS bradykinesia tasks (top) and sample-weighted correlations (bottom).
For Tasks 3.5 (Hand Movements) and 3.6 (Pronation-Supination) no full classification has been implemented yet. Early results seem to suggest that this task is more difficult to rate, as correlations between variables and neurologist ratings are somewhat lower, in the 0.5–0.6 range. A significant exception is variability of amplitude, which seemed to perform better in Butt et al. (2017).
In this study, we limited ourselves to contactless sensors because we believe the advantages of this approach are significant. However, contactless sensors cannot provide a comprehensive method to measure and quantify all motor symptoms of PD, since they cannot assess the stiffness and rigidity of the arms and legs. They are also more limited than electromyography, which provides richer information on muscular activity. On the positive side, they do not require any adjustment to the patient or any interaction other than the performance of the manual tasks, providing an ideal setup to monitor some of the motor symptoms of PD remotely as an addition, rather than a substitution, of more comprehensive PD assessment methods. Other contactless approaches, such as e.g., Lidar, remain to be explored. Finally, the LMC also presents the additional limitations of infrared sensors, such as measurement noise. Numerous authors indicate that the LMC is fallible depending on environmental light and dirt on the lens being present.
In spite of the limitations of this study, and considering the number of relevant studies is still small, available early evidence points to the LMC offering a feasible, objective alternative to visual observation to capture and rate some features of hand motility in PD, as well as in other related diseases. Evidence shows that sensor-based methods have clinical potential and might, after refinement, complement, or even replace subjective assessment procedures, not only in patient care but as an additional outcome measure in the clinical trials of disease-modifying treatments. A significant advantage of a sensor-based approach is that a linear regression model could provide a much higher resolution than current UPDRS assessment. Apart from this advantage, a sensor-based assessment also shows potential to link objective tremor and bradykinesia assessment to dopaminergic replacement therapy (DRT) dosage directly. In this sense, a more accurately adjusted dose might help maximize the period in which DRT is effective as dosage needs to be subsequently increased and OFF periods become longer.
Nevertheless, a substantial number of additional studies in several domains are required. Future research should focus on including more than one clinician rating, as well as procedure standardization. Once pilot trials achieve UPDRS classification predictions that fall within the inter-rater range, designing expert systems that offer a much finer resolution of tremor and bradykinesia should become feasible.
The original contributions presented in the study are included in the article/supplementary material, further inquiries can be directed to the corresponding author/s.
Both authors listed have made a substantial, direct, and intellectual contribution to the work, and approved it for publication.
The authors declare that the research was conducted in the absence of any commercial or financial relationships that could be construed as a potential conflict of interest.
All claims expressed in this article are solely those of the authors and do not necessarily represent those of their affiliated organizations, or those of the publisher, the editors and the reviewers. Any product that may be evaluated in this article, or claim that may be made by its manufacturer, is not guaranteed or endorsed by the publisher.
Butt, A. H., Rovini, E., Dolciotti, C., Bongioanni, P., De Petris, G., and Cavallo, F. (2017). “Leap motion evaluation for assessment of upper limb motor skills in Parkinson's disease,” in 2017 International Conference on Rehabilitation Robotics (ICORR) (IEEE) (London), 116–121. doi: 10.1109/ICORR.2017.8009232
Butt, A. H., Rovini, E., Dolciotti, C., De Petris, G., Bongioanni, P., Carboncini, M., et al. (2018). Objective and automatic classification of Parkinson disease with leap motion controller. Biomed. Eng. Online 17, 1–21. doi: 10.1186/s12938-018-0600-7
Cakmak, Y. Ö., Ölc,ek, S. C., Özsoy, B., and Gökc,ay, D. (2018). “Quantitative measurement of bradykinesia in Parkinson's disease using commercially available leap motion,” in BIOSIGNALS (Funchal), 227–232 doi: 10.5220/0006655402270232
Chaudhry, B., Wang, J., Wu, S., Maglione, M., Mojica, W., Roth, E., et al. (2006). Systematic review: impact of health information technology on quality, efficiency, and costs of medical care. Ann. Intern. Med. 144, 742–752. doi: 10.7326/0003-4819-144-10-200605160-00125
Garcia-Agundez, A., Goosses, M, Konrad, R., Becker, H., Göbel, S., et al. (2019). “Pdpuzzletable: a leap motion exergame for dual-tasking rehabilitation in Parkinson's disease. Design and study protocol,” in Joint International Conference on Entertainment Computing and Serious Games (Arequipa: Springer), 402–406. doi: 10.1007/978-3-030-34644-7_35
Goetz, C. G., Tilley, B. C., Shaftman, S. R., Stebbins, G. T., Fahn, S., Martinez-Martin, P., et al. (2008). Movement disorder society-sponsored revision of the unified Parkinson's disease rating scale (MDSUPDRS): scale presentation and clinimetric testing results. Mov. Disord. 23, 2129–2170 doi: 10.1002/mds.22340
Hauser, R. A., Deckers, F., and Lehert, P. (2004). Parkinson's disease home diary: further validation and implications for clinical trials. Mov. Disord. 19, 1409–1413 doi: 10.1002/mds.20248
Jameson, J. L. (2018). Harrison's Principles of Internal Medicine. New York, NY: McGraw-Hill Education.
Jimenez-Jimenez, F. J., Alonso-Navarro, H., Garc'ia-Mart'in, E., and Agu'ndez, J. A. (2012). The relationship between Parkinson's disease and essential tremor: review of clinical, epidemiologic, genetic, neuroimaging and neuropathological data, and data on the presence of cardinal signs of parkinsonism in essential tremor. Tremor Other Hyperkinet. Mov. 2:tre-02-75-409-3. doi: 10.5334/tohm.118
Khan, T., Nyholm, D., Westin, J., and Dougherty, M. (2014). A computer vision framework for finger tapping evaluation in Parkinson's disease. Artif. Intell. Med. 60, 27–40. doi: 10.1016/j.artmed.2013.11.004
Lee, W. L., Sinclair, N. C., Jones, M., Tan, J. L., Proud, E. L., Peppard, R., et al. (2019). Objective evaluation of bradykinesia in Parkinson's disease using an inexpensive marker-less motion tracking system. Physiol. Meas. 40:014004. doi: 10.1088/1361-6579/aafef2
Lugo, G., Ibarra-Manzano, M., Ba, F., and Cheng, I. (2017). “Virtual reality and hand tracking system as a medical tool to evaluate patients with Parkinson's,” in Proceedings of the 11th EAI International Conference on Pervasive Computing Technologies for Healthcare (Barcelona), 405–408. doi: 10.1145/3154862.3154924
Meara, J., Bhowmick, B. K., and Hobson, P. (1999). Accuracy of diagnosis in patients with presumed Parkinson's disease. Age Ageing 28, 99–102. doi: 10.1093/ageing/28.2.99
Patrick, S. K., Denington, A. A., Gauthier, M. J., Gillard, D. M., and Prochazka, A. (2001). Quantification of the UPDRS rigidity scale. IEEE Trans. Neural Syst. Rehabil. Eng. 9, 31–41. doi: 10.1109/7333.918274
Vivar, G., Almanza-Ojeda, D.-L., Cheng, I., Gomez, J. C., Andrade-Lucio, J. A., and Ibarra-Manzano, M.-A. (2019). Contrast and homogeneity feature analysis for classifying tremor levels in Parkinson's disease patients. Sensors 19:2072. doi: 10.3390/s19092072
Williams, S., Relton, S. D., Fang, H., Alty, J., Qahwaji, R., Graham, C. D., et al. (2020a). Supervised classification of bradykinesia in Parkinson's disease from smartphone videos. Artif. Intell. Med. 110:101966. doi: 10.1016/j.artmed.2020.101966
Keywords: bradykinesia, Parkinson's disease, UPDRS, leap motion, contactless
Citation: Garcia-Agundez A and Eickhoff C (2021) Towards Objective Quantification of Hand Tremors and Bradykinesia Using Contactless Sensors: A Systematic Review. Front. Aging Neurosci. 13:716102. doi: 10.3389/fnagi.2021.716102
Received: 28 May 2021; Accepted: 27 September 2021;
Published: 25 October 2021.
Edited by:
Emma Stanmore, The University of Manchester, United KingdomReviewed by:
Yousef Salimpour, Johns Hopkins University, United StatesCopyright © 2021 Garcia-Agundez and Eickhoff. This is an open-access article distributed under the terms of the Creative Commons Attribution License (CC BY). The use, distribution or reproduction in other forums is permitted, provided the original author(s) and the copyright owner(s) are credited and that the original publication in this journal is cited, in accordance with accepted academic practice. No use, distribution or reproduction is permitted which does not comply with these terms.
*Correspondence: Augusto Garcia-Agundez, YXVndXN0b19nYXJjaWFAYnJvd24uZWR1
Disclaimer: All claims expressed in this article are solely those of the authors and do not necessarily represent those of their affiliated organizations, or those of the publisher, the editors and the reviewers. Any product that may be evaluated in this article or claim that may be made by its manufacturer is not guaranteed or endorsed by the publisher.
Research integrity at Frontiers
Learn more about the work of our research integrity team to safeguard the quality of each article we publish.