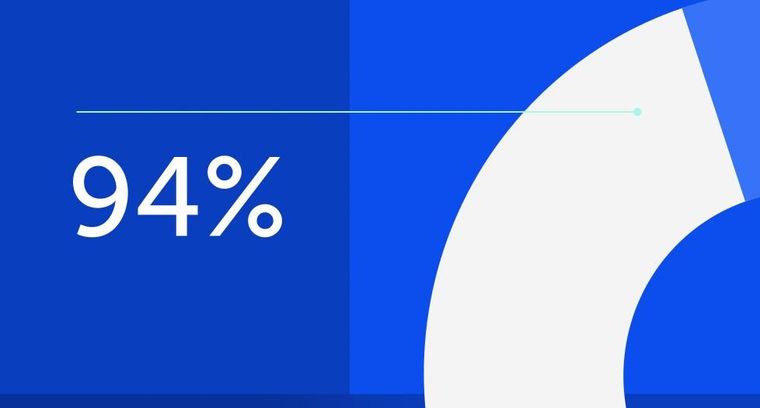
94% of researchers rate our articles as excellent or good
Learn more about the work of our research integrity team to safeguard the quality of each article we publish.
Find out more
ORIGINAL RESEARCH article
Front. Mol. Biosci., 23 March 2021
Sec. Molecular Diagnostics and Therapeutics
Volume 8 - 2021 | https://doi.org/10.3389/fmolb.2021.622643
Background: Limited immunotherapy options are approved for the treatment of cervical cancer and only 10–25% of patients respond effectively to checkpoint inhibition monotherapy. To aid the development of novel therapeutic immune targets, we aimed to explore survival-associated immune biomarkers and co-expressed immune networks in cervical cancer.
Methods: Using The Cancer Genome Atlas (TCGA) Cervical Squamous Cell Carcinoma (CESC) data (n = 304), we performed weighted gene co-expression network analysis (WGCNA), and determined which co-expressed immune-related genes and networks are associated with survival probability in CESC patients under conventional therapy. A “Pan-Immune Score” and “Immune Suppression Score” was generated based on expression of survival-associated co-expressed immune networks and immune suppressive genes, which were subsequently tested for association with survival probablity using the TCGA Head Neck Squamous Cell Carcinoma (HNSCC) data (n = 528), representing a second SCC cancer type.
Results: In CESC, WGCNA identified a co-expression module enriched in immune response related genes, including 462 genes where high expression was associated with increased survival probability, and enriched for genes associated with T cell receptor, cytokine and chemokine signaling. However, a high level of expression of 43 of the genes in this module was associated with decreased survival probability but were not enriched in particular pathways. Separately, we identified 20 genes associated with immune suppression including inhibitory immune checkpoint and regulatory T cell-related genes, where high expression was associated with increased survival probability. Expression of these 20 immune suppressive genes (represented as “Immune Suppression Score”) was highly correlated with expression of overall survival-associated immune genes (represented as “Pan-Immune Score”). However, high expression of seven immune suppression genes, including TWEAK-R, CD73, IL1 family and TGFb family genes, was significantly associated with decreased survival probability. Both scores also significantly associated with survival probability in HNSCC, and correlated with the previously established “Immunophenoscore.”
Conclusion: CESC and HNSCC tumors expressing genes predictive of T cell infiltrates (hot tumors) have a better prognosis, despite simultaneous expression of many immune inhibitory genes, than tumors lacking expression of genes associated with T cell infiltrates (cold tumors) whether or not these tumor express immune inhibitory genes.
Cervical squamous cell carcinoma (CESC) resulted in an estimated 311,000 deaths and 570,000 new cases in 2018 worldwide, representing a major health burden (Paul et al., 2019). Virtually all CESC cases arise from infection by oncogenic strains of the human papillomavirus (HPV), which is also the causative agent of other anogenital and oropharyngeal cancers (Zhou et al., 2019), such as a proportion of head and neck squamous cell carcinoma (HNSCC). In contrast, HPV-negative HNSCCs are primarily caused by heavy smoking and/or drinking (Johnson et al., 2020). While a preventive HPV vaccine exists, CESC and HPV-positive HNSCC-associated deaths continue to occur in low and middle-income countries, where the vaccine remains unaffordable. Regular cervical screening facilitates early detection, but 5-years survival of women with stage III CESC lies below 40% (Otter et al., 2019). Meanwhile, the cases of HNSCC rise continually with 450,000 deaths in 2018 (Johnson et al., 2020). Therefore, CESC and HNSCC will likely remain significant global health issues.
The immune system plays an important role in the prevention and recognition of cancer. Different patients with the same cancer type have widely differenting tumor immune infiltrates. Tumors with significant tumor infiltrating lymphocytes (TILs) are characterized as “hot” and those with limited infiltrates are referred to as “cold” (Galon and Bruni, 2019). For many cancers, including colorectal cancer (Narayanan et al., 2018) and cervical cancer (De Vos van Steenwijk et al., 2013), hot tumors are associated with better survival. A “cold” tumor may reflect lack of tumor specific antigens, defective antigen presentation, lack of T cell activation or deficiencies in migration of immune cells to the tumor site (Bonaventura et al., 2019). This simplified categorization of “hot” and “cold” tumors however doesn’t take the mechanisms of active immune suppression which are commonly observed within tumors into consideration. Particularly, inhibitory immune molecules displayed by cancer and/or immune cells can contribute to prevention of tumor cell-targeted effector T cell responses. The heterogeneic nature of cancers including CESC thus requires methods for tumor stratification for prognostic purposes beyond the classical Tumor/Nodes/Metastasis (TNM) staging system, including the consensus Immunoscore (Pagès et al., 2018; Angell et al., 2020). Identification of prognostic immune-related gene signatures and the “Immunophenoscore” facilitated retrospective patient stratification in CESC (Charoentong et al., 2017; Thorsson et al., 2018; Yang et al., 2019; Nie et al., 2020; Qi et al., 2020) and will potentially guide immunotherapy selection to increase survival outcomes. However, identification of co-expressed immune networks will help to identify optimal targets for therapy. To the best of our knowledge, there is no report comprehensively evaluating the co-expression of immune-related genes and networks in CESC that are significantly associated with survival probability.
Herein, we utilized weighted gene co-expression network analysis (WGCNA) to explore the central immune networks associated with probability of survival for patients receiving conventional therapy. Using the TCGA-CESC dataset, we identified a novel co-expression Immune Module, comprising immune genes for which high expression was associated with increased survival probability. The survival-associated co-expressed immune gene landscape clustered in pathways of T cell activity and infiltration promoting chemokine and cytokine networks, and contained many genes associated with immune-suppression. High expression of 20 inhibitory immune genes was paradoxically associated with increased survival probability, of which 16 were co-expressed in the WGCNA Immune Module, while only high expression of seven inhibitory genes was associated with decreased survival probability. Based on the identification of survival-associated stimulating and inhibitory immune genes in CESC associating with increased survival probability, we established an overall “Pan-Immune Score” and an “Immune Suppression Score” respectively, which were highly positively correlated in both primary CESC and HNSCC. These scores were also highly positively correlated with the previously proposed “Immunophenoscore” (Charoentong et al., 2017). Overall, this study shows that phenotypic stratification of CESC and HNSCC tumors based on co-expressed immune networks or expression of specific immune suppressive genes unexpectedly reveals a hierarchy of survival probability, in which “hot” tumors with significant T cell infiltrates that co-express present mechanisms of specific immune suppression have a better prognosis than “cold” tumors lacking T cell infiltrates.
The TCGA-CESC clinical data was obtained from the GDC legacy database using the TCGAbiolinks R package (https://bioconductor.org/packages/release/bioc/html/TCGAbiolinks.html). The legacy mRNA TCGA-CESC expression data (Illumina HiSeqV2) was manually downloaded from XenaBrowser (https://tcga.xenahubs.net/download/TCGA.CESC.sampleMap/HiSeqV2.gz). Both clinical and expression data was filtered to include “Primary Tumor” sample types only, using the unique descriptive barcode assigned to each patient, 304 patients remained after applying this filter. The clinicopathological data of this cohort has been described previously (Song et al., 2019). We also downloaded the normalized gene expression and clinical data of TCGA-HNSCC from XenaBrowser (https://tcga.xenahubs.net/download/TCGA.HNSC.sampleMap/HiSeqV2_PANCAN.gz). Similar to the cervical cancer data, TCGA-HNSCC was filtered to include “Primary Tumor” sample types only.
We developed a weighted co-expression network of the TCGA-CESC expression data using the WGCNA package in R (Langfelder and Horvath, 2008). The co-expression was measured between the genes in the TCGA-CESC data by using a Pearson correlation function. The adjacency matrix was constructed by raising the co-expression similarity power to β = 6. From the adjacency matrix, we built the topological overlap matrix (TOM) to consider topological similarity and a corresponding dissimilarity matrix (1-TOM) to form clusters. The hclust function was used to perform hierarchical clustering using the dissimilarity matrix. The flashClust package was used to determine the outliers (Langfelder and Horvath, 2012). We used the dynamic tree cutting algorithm with parameters deep split = 2, minimum gene modules = 20, cut height = 0.6 to detect highly distinct gene modules. Module Eigengene expressions (ME) represented by the first principal component (PC1) were calculated to determine the expression of each module. Clinical data was correlated with Eigengene expression of modules and gene significance (GS) was determined. A quantitative method of module membership was determined by correlating ME of modules with gene expression of modules. Colors were randomly assigned to modules. To provide gene ontology (GO) enrichment analysis for modules, the top three gene ontology processes were determined for each module using enrichGO function in the clusterProfiler package (Yu et al., 2012). To identify immune-related modules, GO terms containing the text “immune” were searched. In this research only one module (palevioletred1) was identified as Immune Module.
KMSA was performed using customized R scripts (http://www.r-project.org/). Patients’ gene expression, clinical and scoring data was matched using the assigned patient barcodes. For each gene expression or score, patients were assigned to two groups; Upper Quartile (UQ) and Lower Quartile (LQ) based on whether their gene expression or score values were in the bottom 25% or the top 25% of gene expression or score. KMSA was performed with the R package Survminer (https://cran.r-project.org/web/packages/survminer/index.html) using default arguments and Rho = 0, and the R package Survival (https://cran.r-project.org/web/packages/survival/index.html) using default settings to extract a log rank test p-value for overall survival of up to 1825 days (5 years). p-values of significant associations were −log10 transformed for visualization.
Significant survival-associated genes were sorted into groups of high and low expression in CESC patients with increased survival outcomes. The resulting gene lists were separately analyzed using Enrichr (http://amp.pharm.mssm.edu/Enrichr/) followed by mapping to human KEGG 2019 Human pathways and GO Biological Process 2018 ontologies.
PPIs were predicted using the Search Tool for the Retrieval of Interacting Genes (STRING) (https://string-db.org/) based on a threshold of interaction score >0.9. Hub genes were identified as genes with the highest degree of connectivity for the top enriched pathways.
To establish an “Immune Suppression Score,” we created an immune suppression gene list consisting of 50 genes and including checkpoint inhibitory genes, Treg-associated genes and immune-suppressive cytokines, which was tested for survival association using KMSA. The “Immune Suppression Score” was created based on 20 of these genes for which high expression was associated with increased survival probability (Supplementary File S1). To establish a “Pan-Immune Score,” we used the 462 immune genes identified from the WGCNA palevioletred1 Immune Module for which high expression was associated with increased survival probability, excluding immune suppression genes which were part of the “Immune Suppression Score” to ensure both scores are independent (Supplementary File S1).
The establishment of the “Pan-Immune Score” and “Immune Suppression Score” was performed using a method developed by Foroutan and colleagues (Foroutan et al., 2018). Specifically, each sample in the TCGA-CESC and TCGA-HNSCC expression data was ranked by increasing transcript abundance using the rankgenes function in the singscore package (Foroutan et al., 2018). The mean rank was calculated and normalized against the minimum and maximum value of the rank in all samples in the gene expression datasets. A high “Pan-Immune Score” and “Immune Suppression Score” represents that a particular sample are in concordant with the immune and immune suppression gene pattern. Individual scores for each TCGA CESC and HNSCC sample are reported in Supplementary File S1. We compared 5-years survival probability of the upper and lower quartiles of the “Pan-Immune Score” and “Immune Suppression Score” using KMSA.
“Immunophenoscore” data (IPS1-4) for CESC and HNSCC was retrieved from www.tcia.at and are reported in Supplementary File S1. Briefly, IPS1 is calculated based on gene or metagene expression in four catagories (effector cells, suppressive cells, MHC-related molecules, immunomodulators) (Charoentong et al., 2017). An average z-score based on gene expression in each category was calculated and, and was subsequently positively weighted for catagories “effector cells,” “MHC-related molecules” and for stimulatory “immunomodulator” genes, and negatively weighted for the catagory “suppressive cells” and for inhibitory “immunomodulator” genes. Three additional IPS scores (IPS2-4) were calculated with the aim to facilitate predicting response to checkpoint treatment, where the inhibitory “immunomodulator” gene to be blocked was positively weighted instead (IPS2 positivly weighted expression of CTLA-4; IPS3 positively weighted expression of PD1/PDL1/PDL2; IPS4 positivly weighted expression of CTLA-4/PD1/PDL1/PDL2).
Pearson R correlation analysis (PRCA) was used to determine correlation between WGCNA modules with gene significance, between “Pan-Immune Score,” “Immune Suppression Score” and the “Immunophenoscore” (IPS1-4). Spearman correlation analysis was used to determine correlation between survival-associated immune genes and FIGO stage.
Immune pathway gene lists were generated using KEGG (http://www.genome.jp/kegg/). KEGG pathways analyzed were: NF-kappa B signaling pathway (hsa04064), Antigen Processing and Presentation (hsa04612), B cell Receptor Signaling Pathway (hsa04662), C-Type lectin receptor signaling pathway (hsa04625), Cell Adhesion (hsa04514), Chemokine Signaling (hsa04062), Complement and Coagulation Cascades (hsa04610), Jak-STAT signaling pathway (hsa04630), Cytosolic DNA-sensing pathway (hsa04623), Extracellular Matrix (hsa04512), Fc Epsilon RI signaling pathway (hsa04664), Fc Gamma R-mediated phagocytosis (hsa04666), Hematopoietic Cell Lineage (hsa04640), IL-17 Signaling Pathway (hsa04657), Intestinal Immune Network for IgA Production (hsa04672), Leukocyte Transendothelial Migration (hsa04670), Natural Killer Cell Mediated Cytotoxicity (hsa04650), NOD-like receptor signaling Pathway (hsa04621), Platelet Activation (hsa04611), RIG-I-like Receptor Signaling Pathway (has04622), T Cell Receptor Signaling (hsa04660), Th1 and Th2 Differentiation (hsa04658), Th17 Differentiation (hsa04659), Toll-like Receptor Signaling (hsa04620), Toll and Imd Signaling Pathway (hsa04624). Genes were analyzed for survival association using KMSA.
We sought to identify co-expressed immune genes and networks predictive of probability of survival for patients with CESC. Weighted gene co-expression network analysis (WGCNA) across all 20,530 genes of TCGA data for 304 patients with CESC using a co-expression threshold of 0.6 resulted in 21 co-expression modules (Figures 1A,B), with generally weak intermodule correlation, with the exception of the gray and greenyellow module (Figure 1C), validating the chosen co-expression threshold as appropriate for module stratification. Gene Ontology (GO) analysis showed that the palevioletred1 module was enriched in immune receptor and cytokine receptor activity (Table 1), while correlation analysis identified that this module was significantly associated with days to death for the subjects in the TCGA cohort, but associated with no other recorded clinical features (Figures 1D,E).
FIGURE 1. Idenditifaction of a co-expression Immune Module which correlates with CESC survival probability. (A) Flow chart describing analysis pipeline and summary of results. (B) Weighted gene co-expression network analysis (WGCNA) was used to identify survival-associated co-expressed immune networks in the TCGA CESC data. Clustering of module eigengenes with a threshold of 0.6 resulted in 21 modules. (C) Correlation was performed between eigengene expressions of each module with each other to represent association of modules with each other. (D) Correlation analysis of modules eigengene expression with clinical features. (E) Correlation analysis comparing the palevioletred1 Immune Module to clinical feature days_to_death.
TABLE 1. Enriched GO biological processes for each WGCNA module. Only top three are shown. NA: not applicable, no GO ID was identified.
The palevioletred1 Immune Module comprised a total of 1,485 genes (Figure 1A). As this module showed significant correlation with days to death, and the strongest GO enrichment in immune related processes, we assessed each of the genes of this module for significant association with 5-years survival probability using Kaplan Maier Survival analysis (KMSA) and comparing top and bottom quartiles of gene expression. From this module, we identified 462 genes where high level expression was significantly associated with increased 5-years survival probability and 43 genes where high level expression was significantly associated with decreased 5-years survival probability (Figure 1A; Supplementary File S1). A majority of the 462 genes where high level expression was associated with increased 5-years survival were linked to immune processes according to GO and KEGG pathway analysis (Table 2), whereas the 43 co-expressed genes where high level expression was significantly associated with decreased 5-years survival probability were not particularly representative of any GO or KEGG pathway (data not shown). To determine genes with increased connectivity from amongst the 462 genes associated with increased 5-years survival probability, we built a protein-protein interaction (PPI) network. These genes organized into three major hubs overlapping with the T cell receptor signaling, chemokine signaling and cytokine-cytokine receptor interaction KEGG pathways (Figure 2A). Central genes of these hubs were LCK, CD3G, PTPN6, CD3D, and CD4 in the T cell receptor signaling pathway; GNG2, GNGT2, CXCR4, CCR5, and CXCR3 in chemokine signaling pathway; and CXCR4, IL2RB, IL2RA, CCR5, and CXCR3 in the cytokine-cytokine receptor interaction pathway (Figure 2B). CXCL9-CXCR3 interaction and CCL5 are crucial for T cell function and infiltration into tumors (Duan et al., 2020), and higher level of expression of these genes might result in improved anti-tumor immunity in patients with better survival outcomes. In contrast, the 43 highly co-expressed genes in patients with decreased survival outcomes arranged themselves randomly without evident connection hubs (data not shown), indicating that there is no specific immune network which is highly expressed in patients with decreased survival probability.
FIGURE 2. Protein-protein interaction (PPI) network identifies hub network genes promoting increased CESC survival probability. Co-expressed survival-associated immune genes of the WGCNA palevioletred1 Immune Module were analyzed for PPI. (A) Shown is the network of 462 highly expressed genes in patients of increased survival probability (excluding non-connected genes). (B) Hub genes were identified as genes with highest connectivity in a particular KEGG pathway.
TABLE 2. Enriched GO biological processes and KEGG pathways of 462 highly expressed genes in subjects with increased 5-years survival probability.
To test whether clinical features contributed to survival probability in the TGCA CESC cohort, we performed correlation analysis for age, body-mass-index, and use of tobacco products, and none proved predictive (Figure 3A). No difference in survival probability was observed for patients presenting with FIGO stages I, II, and III CESC, whereas FIGO stage IV presentation was as expected associated with decreased survival (Figure 3A). To test whether FIGO staging correlated with the expression of survival-associated immune genes, we performed a Spearman Correlation analysis for every of the 505 survival-associated genes of the WGCNA palevioletred2 Immune Module, identifying 51 significant FIGO-stage correlated genes, although with weak correlation (Figure 3B). 45 of these 51 genes had a weak negative correlation with FIGO stage, and were largely independent in a PPI analysis (not shown).
FIGURE 3. CESC FIGO stage is associated with tumour-promoting proinflammatory cytokines. (A) Clinical features were tested for association with survival. For BMI, four groups were defined based on diagnostic BMI values (underweight <18.5, normal 18.5–24.9, overweight 25–30, obese >30). For smoking, the lower and upper quartile of the clinical feature pack_years_smoked were compared. (B) Genes of the WGCNA palevioletred1 Immune Module (n = 505) which significantly associated with 5-years survival probability with cervical cancer were analyzed for correlation with FIGO stage using Spearman correlation analysis. Genes were ordered from largest to smallest correlation coefficient r (left panel). p-values were–log10 transformed and plotted as heatmap (right panel). Significant genes at the top are positively correlated with FIGO stage and significant genes at the bottom are negatively correlated with FIGO stage.
To identify survival-associated immune genes that associated with FIGO staging but were not part of the co-expression Immune Module, we further carried out a knowledge based analysis of immune genes from recognized KEGG immune pathways for association with survival. From these, we identified 220 genes significantly associated with 5-years survival probability, of which 155 were associated with higher survival probability when expressed at high level, while 65 were associated with lower survival probability when expressed at high level (Supplementary Figure S1A,B). Also, 108 of the 220 genes overlapped with the WGCNA palevioletred2 Immune Module. We tested FIGO stage correlation with these 220 survival-associated immune genes, and identified 27 significant FIGO-stage correlated genes (Supplementary Figure S2). Although not part of the WGCNA palevioletred2 Immune Module, we identified IL1A, IL1B and TGFB1 as positively correlated with FIGO stage, suggesting a contribution of inflammation to cervical cancer progression. The level of expression of MHC class II genes and IL12A was negatively correlated with FIGO stage, indicating that tumors with an increased FIGO stage might be depleted of antigen-presenting cells.
Progressing tumors express inhibitory immune molecules and/or regulatory T cells (Tregs). Inhibitory molecule targeting has revolutionized cancer immunotherapy, but less than 20% of cervical cancer patients are responsive to current immune checkpoint inhibition therapy (Sharma et al., 2017). We created a knowledge-based list of 50 immune-suppression associated genes (Figure 1A; (Supplementary File S1) including many inhibitory immune checkpoint genes, Treg associated genes and soluble immune-suppressive mediators, and found that high expression of 20 of these genes and low expression of seven was significantly associated with increased 5-years survival probability (Figure 4A). Genes where high expression associated with better survival encoded B7H4, Galactin-9, HVEM, TACI, BAFF, BAFF-R, CTLA4, LAG3, TIGIT, TRAIL, as well as Treg associated genes FOXP3, KLRG1, and IL10RA, and soluble mediators IDO1 and IDO2 (Figure 4B). In addition to classical tumor-promoting TGFB family and IL1 family genes, immune checkpoints CD73 (NT5E) and TWEAK-R (TNFRSF12A) were identified as immune suppressive genes for which high expression was associated with a decreased survival probability, suggesting that these molecules might be targets for immunotherapy for subjects with this CESC phenotype. The WGCNA co-expression network also contained 16 of the 20 survival-associated immune suppressive genes, including 11 immune checkpoint genes, Treg associated genes as well as IDO1 and IDO2 (Supplementary File S1).
FIGURE 4. High expression of multiple immune suppression genes is associated with increased 5-years survival probability with CESC. A custom built list of 50 immune suppressive genes including immune checkpoint inhibitor genes, Treg related genes and soluble mediators was analyzed for significant association with 5-years survival probability. (A) Number of genes where high or low expression was associated with increased 5-years survival probability. (B)p-values of significant immune suppression genes were −log10 transformed. Genes for which low expression was associated with increased survival probability were further transformed to a negative value.
To assess the relationship between immunity-promoting and immune-suppressive gene activity in CESC, we built scores using a method developed by Foroutan and colleagues (Foroutan et al., 2018). A “Immune Suppression Score” was constructed based on the previously identified 20 immune-suppressive genes described in Figure 4B, for which high expression was associated with increased survival probability (Supplementary File S1). An overall “Pan-Immune Score” was built based on the 462 survival-associated genes of the palevioletred1 Immune Module excluding any overlapping immune-suppressive genes present in the “Immune Suppression Score” to ensure both scores are independent (Supplementary File S1). We subsequently compared 5-years survival probability for subjects of the upper (high) and lower (low) score quartiles. CESC subjects with a high “Pan-Immune Score” had significantly increased 5-years survival probability compared to subjects with a low “Pan-Immune Score” (Figure 5A). Interestingly, CESC subjects with a high “Immune Suppression Score” also had significantly increased 5-years survival probability compared to subjects with a low “Immune Suppression Score” (Figure 5B). “Pan-Immune Score” and “Immune Suppression Score” were highly positively correlated in CESC (Figure 5C). We tested the applicability of the TCGA CESC-derived-“Pan-Immune Score” and “Immune Suppression Score” to data from the TCGA HNSCC cohort. As observed for CESC, a high “Pan-Immune Score” was associated with increased 5-years survival probability in HNSCC, indicating that survival probability in HNSCC is also linked to a hot/cold tumor phenotype (Figure 5D). A high “Immune Suppression Score” was also significantly associated with increased survival probability in HNSCC (Figure 5E), and as observed in the CESC cohort data, “Pan-Immune Score” and “Immune Suppression Score” were also highly positively correlated in HNSCC (Figure 5F). These data suggest that CESC and HNSCC patients with increased survival probability express higher levels of immune-suppressive genes as a consequence of overall immune activity within the tumor.
FIGURE 5. “Pan-Immune Score” and “Immune Suppression Score” predicting 5-years survival probability with CESC and HNSCC are highly correlated. (A, D) Subjects were assigned a “Pan-Immune Score” according to expression of survival-associated immune genes of the WGCNA palevioletred1 Immune Module, and 5-years survival probability of the upper and lower quartiles of the “Pan-Immune Score” representing high and low scores was compared in the TCGA-CESC (A) and TCGA-HNSCC (D) data. (B, E) Subjects were assigned an “Immune Suppression Score” according to expression of survival-associated immune suppression genes described in Figure 1B, and 5-years survival probability of the upper and lower quartiles of the “Immune Suppression Score” representing high and low scores was compared in the TCGA-CESC (B) and TCGA-HNSCC (E) data. (C, F) Correlation analysis of “Pan-Immune Score” and “Immune Suppression Score” in the TCGA-CESC (C) and TCGA-HNSCC (F) data.
To compare these findings with previously established immune scores, we assessed correlation of the “Pan-Immune Score” and “Immune Suppression Score” with the “Immunophenoscores” IPS1-4 which have been shown to predict response to immune checkpoint inhibition therapy in melanoma patients (Charoentong et al., 2017). In both CESC and HNSCC, both “Pan-Immune Score” and “Immune Suppression Score” were positively and significantly correlated with IPS2-4, with the strongest correlation observed with IPS4 (Table 3; Figures 6A,B). IPS2-4, in contrast to IPS1, are positively weighted for expression of CTLA4 (IPS2), PD1/PDL1/PDL2 (IPS3) or CTLA4/PD1/PDL1/PDL2 (IPS4). The lack of positive or strong correlation of IPS1 with the here established scores is likely due to IPS1 weighing inhibitory immunomodulators negative, resulting in a lower score of samples expressing high levels of these molecules, while the “Immune Suppressive Score” and “Pan-Immune Score” weigh expression of any immune-related genes positively toward the score.
TABLE 3. Correlation of IPS1-4 with “Pan-Immune Score” and “Immune Suppression Score” in CESC and HNSCC. R: Correlation efficient; p: p-value.
FIGURE 6. “Pan-Immune Score” and “Immune Suppression Score” are positively correlated with “Immunophenoscore.” Correlation analysis of “Pan-Immune Score” and “Immune Suppression Score” with “Immunophenoscore” IPS4 in TCGA-CESC (A) and TCGA-HNSCC (B).
Combined with the observation that tumors presenting with an overall increased immune gene co-expression are likely to demonstrate an increased probability of 5-years survival, these data suggest that CESC and HNSCC tumors with high levels of expression of specific immune-suppressive genes are more associated with a high probability of 5-years survival than CESC and HNSCC tumors lacking any immune related gene expression.
The current consensus is that cancers of most organs and tissues are extensively heterogeneous, consistent with the observation that only a limited proportion of patients with any particular tumor type respond to any specific immunotherapy. Advancements in high-dimensional screening technologies have enabled consideration of personalized cancer immunotherapy, optimized for the phenotype of the patient’s tumor. We aimed in the current study to determine the co-expressed immune gene networks within CESC tumors that correlate with survival, and to analyze survival-associated immune genes which are related to immune suppression, in an attempt to subdivide primary CESC tumors by immune phenotype, which may guide appropriate immunotherapy selection.
Dominant co-expressed immune networks associated with increased survival probability were T cell related, as well as enriched in pathways of cytokine and chemokine signaling. Others have predicted the tumor-infiltrating immune cell composition in the TCGA CESC dataset using the metagene CIBERSORT approach, and found in line with our data that an enrichment of T cells was associated with increased survival probability (Wang et al., 2019). In addition to CD8 T cells, high infiltration of resting but not activated mast cells, stationary dendritic cells, and activated CD4 T cells in CESC were also found related to better survival (Yang et al., 2019; Nie et al., 2020). Resting mast cells and naïve B cells were recently selected as prognostic signatures (Che et al., 2020), where SCC patients were stratified into low and high risk groups. The low risk patients with high proportions of these two immune cells had better survival rates than high risk patients. Low risk patients significantly benefited from chemotherapeutic and immunotherapeutic treatments. In addition to tumor-infiltrating immune cells, immune-related gene sets such as RIPOR2, DAAM2, SORBS1, and CXCL8 (Mei et al., 2020), or LTA, TFRC, TYK2, DLL4, CSK, JUND, NFATC4, SBDS, FLT1, IL17RD, IL3RA, SDC1, PLAU (Ding et al., 2020), were proposed as prognostic genes to stratify CESC patients. However, neither of these studies took co-expression of immune networks into consideration.
Subjects with primary CESC tumors expressing the lowest quartile of gene expression of co-expressed immune networks, suggestive of a “cold” tumor phenotype, had the lowest survival probability. Additionally, subjects with the lowest survival probability expressed very few immune-related genes at high levels, many of which are known to promote tumor progression, including IL1A, IL1B, and TGFB, and which were also correlated with increased FIGO stage. In a previous study, using immune related gene pairs (IRGPs), 29 IRGPs (47 genes) were proposed as prognostic immune markers to stratify CESC patients into low and high risk groups, and IL1B was also correlated with CESC development in the current study (Nie et al., 2020).
The current paradigm separates tumors into “hot,” with a substantial immune and inflammatory cell infiltrate, “cold,” lacking such an infiltrate, and “altered” phenotypes (Galon and Bruni, 2019). The “altered” phenotypes can be further divided into “altered-excluded” and “altered-immunosuppressed.” The former describes tumors containing immune cells in the tumor margin, but not within the tumor bed, and the latter describes tumors containing an overall intermediate “Immunoscore” (defined by CD3 and CD8 immunohistochemistry) while also expressing immune-suppressive molecules such as TGFβ and inhibitory immune checkpoint genes. When analyzing the TCGA CESC data for association of immune suppression genes with survival, including inhibitory immune checkpoint genes, we observed that subjects with tumors expressing the lowest levels of multiple inhibitory immune checkpoint and other immune suppression genes had the lowest survival probability, indicating that the tumor phenotype stratification can be ordered into a hierarchy. Our data suggests that subjects with “cold” CESC tumors have worse survival probabilities compared to subjects with “immunosuppressed” CESC tumors, at least when considering expression of the particular immunosuppressive genes outlined in this study. Our data further suggests that an “altered-immunosuppressed” classification is oversimplified and requires more precise characterization based on the expression of specific immune-suppressive molecules. Subjects with the top 25% of “Immune Suppression Score” had the best survival probability in both CESC and HNSCC. Furthermore, the high correlation between the overall “Pan-Immune Score” and the “Immune Suppression Score” demonstrates that CESC and HNSCC tumors with the highest levels of immune activity also express the highest levels of particular inhibitory immune checkpoint genes and Treg associated genes, and despite this are associated paradoxically with the best survival probability following conventional therapy. We therefore hypothesize that a combined specific immune checkpoint inhibitor therapy and conventional therapy will likely further increase survival outcomes of CESC and HNSCC patients who already have high levels of immune gene expression within the tumor as indicator of tumor immune cell infiltration and priming of an anti-tumor T cell response, but might not benefit patients with “cold” tumors, as they lack an adaptive immune infiltrate, and testing this hypothesis should be an aim of future trials of immunotherapy.
Our analysis identified 20 inhibitory immune modulator genes for which high expression was associated with increased survival. In a recent report, eight inhibitory immune modulators in SCC were also identified as prognostic makers (Che et al., 2020), of which IDO1, TIGIT, ICOS, and LAG3 were consistent with our observation. We also identified seven genes, including TWEAK-R and CD73, for which high expression was associated with decreased survival, and we observed a comparable pattern in HNSCC. TWEAK-R is overexpressed in other multiple cancers and treatment with anti-TWEAK-R antibodies attracted effector immune cells infiltrating into tumors in mice (Ye et al., 2017). Targeting CD73 using antibodies or small molecule inhibitors has been proposed as immunotherapy to enhance T cell infiltration, and has been evaluated in different clinical settings, including cervical cancer (Arab and Hadjati, 2019). Hence, these targets represent promising therapy options for patients presenting with an overall “cold” tumor phenotype but expressing high levels of these molecules.
The most widely studied checkpoint inhibitors in cervical cancer target PD-1 and PD-L1, although response rates when given as monotherapy are below 20%. While neither PD-1 nor PD-L1 gene expression levels were associated with survival probability in the TCGA-CESC dataset, others have detected a significant correlation between expression of membrane PD-1 measured by immunohistochemistry with survival in patients with recurrent cervical cancer (Roszik et al., 2018). A recent study showed that high expression of PD-L1 on immune cells was associated with decreased survival, while high expression of PD-L1 on tumor cells was associated with increased survival (Chen et al., 2020), indicating another level of complexity of how these molecules effect outcomes. Lack of association of PD-1/PD-L1 gene expression at presentation with survival in this cohort has thus no implications for use of checkpoint inhibitors targeting these molecules as they may be induced by other therapies including HPV oncoprotein targeted vaccination.
Immunotherapy will need to be optimized to increase survival probability for patients presenting a “cold” tumor phenotype (Bonaventura et al., 2019). HPV-induced cervical cancers can be immunologically “cold” despite expressing virus derived antigens, implying that a cold phenotype can also arise from defects in antigen presentation. In this study we observed that the expression of MHC class II genes was negatively correlated to FIGO stage, indicating that antigen presentation can decline with tumor progression. Absence of MHC class I gene expression by tumors could also be associated with a “cold” tumor phenotype, but we did not identify any survival associated MHC class I genes in this data set. We speculate that patients with “cold” CESC and HNSCC tumors might benefit from a multi-step therapy approach (Frazer and Chandra, 2019) by: 1) conversion of “cold” to “hot” tumors using local radiation therapy to induce immunogenic cell death, and/or intra-tumor application of adjuvant (if achievable), 2) priming of a systemic tumor-targeted T cell response using HPV oncoprotein targeted vaccination for HPV + CESC and HNSCC (Chandra et al., 2017), 3) immune checkpoint inhibition to prolong tumor- and therapy-induced immune responses, 4) promotion of T cell homing to the tumor by blockade of TGFβ (Mariathasan et al., 2018), 5) anti-angiogenesis therapy by VEGF blockage, and 6) inhibition of tumor cell dissemination by targeting the central focal adhesion pathway (Budhwani et al., 2020). Proof of principle studies have shown that “cold” to “hot” tumor conversion can be achieved, for example by intra-tumor delivery of oncolytic viruses such as Talimogene laherparpvec (T-VEC), an engineered herpes simplex virus which selectively infects cancer cells and expresses the dendritic cell attractant granulocyte-macrophage colony-stimulating factor (GM-CSF) (Johnson et al., 2015). T-VEC is currently approved for use in non-operable melanoma lesions, with an overall response rate when given as monotherapy of 26.4% (Andtbacka et al., 2015). However, combination with PD-1 checkpoint blockade increased the overall response rate to 62% (Ribas et al., 2017), underlining the potential that lies in well-orchestrated combination immunotherapies. Targeting of the autophagy-related protein PIK3C3 (Vps34) has recently been demonstrated as a novel strategy to fire up “cold” tumors (Noman et al., 2020). A multi-faceted trial combining four of the above outlined approaches is currently underway in patients with checkpoint naïve advanced HPV-associated malignancies (NTC04287868). This study is testing a combination of a therapeutic HPV vaccine together with tumor-targeted IL-12 as “cold” to “hot” tumor converter (Strauss et al., 2019), and a bi-functional fusion protein blocking PD-L1 and TGFβ (Strauss et al., 2018) which has demonstrated enhanced efficacy in preclinical models of HPV-associated disease (Rumfield et al., 2020). Combination therapies may deliver better outcomes, but will also likely increase the risk of adverse and off target effects.
The data presented here and by others supports the hypothesis that the magnitude of a T cell-associated immune signature is predictive of prognosis in CESC and HNSCC. Our future outlook predicts that cancer patients will eventually have access to high-dimensional technologies to identify their individual tumor/immune landscape in detail and allow best suited drug selection from an extensive cancer- and immunotherapy drug palette.
The original contributions presented in the study are included in the article/Supplementary Material, further inquiries can be directed to the corresponding author.
Ethical review and approval were not required for the study on human participants in accordance with the local legislation and institutional requirements. Written informed consent for participation was not required for this study in accordance with the national legislation and the institutional requirements.
MB, AM, and JC conceptualized the study. MB, GT, and AM collected the data. MB, GT, MY, AM, and JC analyzed the data. IF and JC supervised the study. JC wrote the first draft of the manuscript. All authors revised and edited the manuscript.
MY holds a National Health and Medical Research Council (NHMRC) Early Career Fellowship (APP1124265). Prof Ian Frazer holds an NHMRC Senior Investigator Grant (APP1173927) and an NHMRC Development Grant (APP2000135), and funding from the Merchant Foundation and the Metal Manufacturing Industries.
The authors declare that the research was conducted in the absence of any commercial or financial relationships that could be construed as a potential conflict of interest.
This research was carried out at the Translational Research Institute, Woolloongabba, QLD, Australia. The Translational Research Institute is supported by a grant from the Australian Government.
The Supplementary Material for this article can be found online at: https://www.frontiersin.org/articles/10.3389/fmolb.2021.622643/full#supplementary-material.
Andtbacka, R. H., Kaufman, H. L., Collichio, F., Amatruda, T., Senzer, N., Chesney, J., et al. (2015). Talimogene laherparepvec improves durable response rate in patients with advanced melanoma. J. Clin. Oncol. 33 (25), 2780–2788. doi:10.1200/JCO.2014.58.3377
Angell, H. K., Bruni, D., Barrett, J. C., Herbst, R., and Galon, J. (2020). The Immunoscore: colon cancer and beyond. Clin. Cancer Res. 26 (2), 332–339. doi:10.1158/1078-0432.CCR-18-1851
Arab, S., and Hadjati, J. (2019). Adenosine blockage in tumor microenvironment and improvement of cancer immunotherapy. Immune Netw. 19 (4), e23. doi:10.4110/in.2019.19.e23
Bonaventura, P., Shekarian, T., Alcazer, V., Valladeau-Guilemond, J., Valsesia-Wittmann, S., Amigorena, S., et al. (2019). Cold tumors: a therapeutic challenge for immunotherapy. Front. Immunol. 10, 168. doi:10.3389/fimmu.2019.00168
Budhwani, M., Lukowski, S. W., Porceddu, S. V., Frazer, I. H., and Chandra, J. (2020). Dysregulation of stemness pathways in HPV mediated cervical malignant transformation identifies potential oncotherapy targets. Front. Cell. Infect. Microbiol. 10, 307. doi:10.3389/fcimb.2020.00307
Chandra, J., Dutton, J. L., Li, B., Woo, W. P., Xu, Y., Tolley, L. K., et al. (2017). DNA vaccine encoding HPV16 oncogenes E6 and E7 induces potent cell-mediated and humoral immunity which protects in tumor challenge and drives E7-expressing skin graft rejection. J. Immunother. 40 (2), 62–70. doi:10.1097/CJI.0000000000000156
Charoentong, P., Finotello, F., Angelova, M., Mayer, C., Efremova, M., Rieder, D., et al. (2017). Pan-cancer immunogenomic analyses reveal genotype-immunophenotype relationships and predictors of response to checkpoint blockade. Cell Rep. 18 (1), 248–262. doi:10.1016/j.celrep.2016.12.019
Che, Y., Luo, Z., Zhang, C., Sun, N., Gao, S., and He, J. (2020). Immune signature of tumor-infiltrating immune cells predicts the prognosis and therapeutic effects in squamous cell carcinoma. Int. Immunopharmacol. 87, 106802. doi:10.1016/j.intimp.2020.106802
Chen, H., Xia, B., Zheng, T., and Lou, G. (2020). Immunoscore system combining CD8 and PD-1/PD-L1: a novel approach that predicts the clinical outcomes for cervical cancer. Int. J. Biol. Marker 35 (1), 65–73. doi:10.1177/1724600819888771
De Vos van Steenwijk, P. J., Ramwadhdoebe, T. H., Goedemans, R., Doorduijn, E. M., van Ham, J. J., Gorter, A., et al. (2013). Tumor-infiltrating CD14-positive myeloid cells and CD8-positive T-cells prolong survival in patients with cervical carcinoma. Int. J. Cancer 133 (12), 2884–2894. doi:10.1002/ijc.28309
Ding, H., Fan, G. L., Yi, Y. X., Zhang, W., Xiong, X. X., and Mahgoub, O. K. (2020). Prognostic implications of immune-related genes’ (IRGs) signature models in cervical cancer and endometrial cancer. Front. Genet. 11, 725. doi:10.3389/fgene.2020.00725
Duan, Q., Zhang, H., Zheng, J., and Zhang, L. (2020). Turning cold into hot: firing up the tumor microenvironment. Trends Cancer 6 (7), 605–618. doi:10.1016/j.trecan.2020.02.022
Foroutan, M., Bhuva, D. D., Lyu, R., Horan, K., Cursons, J., and Davis, M. J. (2018). Single sample scoring of molecular phenotypes. BMC Bioinformatics 19 (1), 404. doi:10.1186/s12859-018-2435-4
Frazer, I. H., and Chandra, J. (2019). Immunotherapy for HPV-associated head and neck cancer. Papillomavirus Res. 8, 100176. doi:10.1016/j.pvr.2019.100176
Galon, J., and Bruni, D. (2019). Approaches to treat immune hot, altered and cold tumours with combination immunotherapies. Nat. Rev. Drug Discov. 18 (3), 197–218. doi:10.1038/s41573-018-0007-y
Johnson, D. B., Puzanov, I., and Kelley, M. C. (2015). Talimogene laherparepvec (T-VEC) for the treatment of advanced melanoma. Immunotherapy 7 (6), 611–619. doi:10.2217/imt.15.35
Johnson, D. E., Burtness, B., Leemans, C. R., Lui, V. W. Y., Bauman, J. E., and Grandis, J. R. (2020). Head and neck squamous cell carcinoma. Nat. Rev. Dis. Primers 6 (1), 1–22. doi:10.1038/s41572-020-00224-3
Langfelder, P., and Horvath, S. (2012). Fast R functions for robust correlations and hierarchical clustering. J. Stat. Softw. 46 (11), i11. doi:10.18637/jss.v046.i11
Langfelder, P., and Horvath, S. (2008). WGCNA: an R package for weighted correlation network analysis. BMC Bioinformatics 9 (1), 559. doi:10.1186/1471-2105-9-559
Mariathasan, S., Turley, S. J., Nickles, D., Castiglioni, A., Yuen, K., Wang, Y., et al. (2018). TGFβ attenuates tumour response to PD-L1 blockade by contributing to exclusion of T cells. Nature 554 (7693), 544–548. doi:10.1038/nature25501
Mei, J., Xing, Y., Lv, J., Gu, D., Pan, J., Zhang, Y., et al. (2020). Construction of an immune-related gene signature for prediction of prognosis in patients with cervical cancer. Int. Immunopharmacol. 88, 106882. doi:10.1016/j.intimp.2020.106882
Narayanan, S., Kawaguchi, T., Yan, L., Peng, X., Qi, Q., and Takabe, K. (2018). Cytolytic activity score to assess anticancer immunity in colorectal cancer. Ann. Surg. Oncol. 25 (8), 2323–2331. doi:10.1245/s10434-018-6506-6
Nie, H., Bu, F., Xu, J., Li, T., and Huang, J. (2020). 29 immune-related genes pairs signature predict the prognosis of cervical cancer patients. Sci. Rep. 10 (1), 1–16. doi:10.1038/s41598-020-70500-5
Noman, M. Z., Parpal, S., Van Moer, K., Xiao, M., Yu, Y., Viklund, J., et al. (2020). Inhibition of Vps34 reprograms cold into hot inflamed tumors and improves anti-PD-1/PD-L1 immunotherapy. Sci. Adv. 6 (18), eaax7881. doi:10.1126/sciadv.aax7881
Otter, S. J., Chatterjee, J., Stewart, A. J., and Michael, A. (2019). The role of biomarkers for the prediction of response to checkpoint immunotherapy and the rationale for the use of checkpoint immunotherapy in cervical cancer. Clin. Oncol. (R. Coll. Radiol.) 31 (12), 834–843. doi:10.1016/j.clon.2019.07.003
Pagès, F., Mlecnik, B., Marliot, F., Bindea, G., Ou, F. S., Bifulco, C., et al. (2018). International validation of the consensus immunoscore for the classification of colon cancer: a prognostic and accuracy study. Lancet 391 (10135), 2128–2139. doi:10.1016/S0140-6736(18)30789-X
Paul, A. C., Anjua, J., Ana, O., and Denny, L. (2019). Cervical cancer. Lancet 393 (10167), 169–182. doi:10.1016/S0140-6736(18)32470-X
Qi, X., Fu, Y., Zhang, M., Lu, C., Wang, Y., Peng, J., et al. (2020). An innovative immune score-based prognostic nomogram for patients with cervical cancer. Biomed. Res. Int. 2020, 1–9. doi:10.1155/2020/8882576
Ribas, A., Dummer, R., Puzanov, I., VanderWalde, A., Andtbacka, R. H. I., Michielin, O., et al. (2017). Oncolytic virotherapy promotes intratumoral T cell infiltration and improves anti-PD-1 immunotherapy. Cell 170 (6), 1109–1119.e10. doi:10.1016/j.cell.2017.08.027
Roszik, J., Ring, K. L., Wani, K. M., Lazar, A. J., Yemelyanova, A. V., Soliman, P. T., et al. (2018). Gene expression analysis identifies novel targets for cervical cancer therapy. Front. Immunol. 9, 2102. doi:10.3389/fimmu.2018.02102
Rumfield, C. S., Pellom, S. T., Morillon Ii, Y. M., Schlom, J., and Jochems, C. (2020). Immunomodulation to enhance the efficacy of an HPV therapeutic vaccine. J. Immunother. Cancer 8 (1), e000612. doi:10.1136/jitc-2020-000612
Sharma, P., Hu-Lieskovan, S., Wargo, J. A., and Ribas, A. (2017). Primary, adaptive, and acquired resistance to cancer immunotherapy. Cell 168 (4), 707–723. doi:10.1016/j.cell.2017.01.017
Song, L., Liu, S., Yao, H., Zhang, L., Li, Y., Xu, D., et al. (2019). MiR-362-3p is downregulated by promoter methylation and independently predicts shorter OS of cervical squamous cell carcinoma. Biomed. Pharmacother. 115, 108944. doi:10.1016/j.biopha.2019.108944
Strauss, J., Heery, C. R., Kim, J. W., Jochems, C., Donahue, R. N., Montgomery, A. S., et al. (2019). First-in-Human phase I trial of a tumor-targeted cytokine (NHS-IL12) in subjects with metastatic solid tumors. Clin. Cancer Res. 25 (1), 99–109. doi:10.1158/1078-0432.CCR-18-1512
Strauss, J., Heery, C. R., Schlom, J., Madan, R. A., Cao, L., Kang, Z., et al. (2018). Phase I trial of M7824 (MSB0011359C), a bifunctional fusion protein targeting PD-L1 and TGFβ, in advanced solid tumors. Clin. Cancer Res. 24 (6), 1287–1295. doi:10.1158/1078-0432.CCR-17-2653
Thorsson, V., Gibbs, D. L., Brown, S. D., Wolf, D., Bortone, D. S., Ou Yang, T. H., et al. (2018). The immune landscape of cancer. Immunity 48 (4), 812–830.e14. doi:10.1016/j.immuni.2018.03.023
Wang, J., Li, Z., Gao, A., Wen, Q., and Sun, Y. (2019). The prognostic landscape of tumor-infiltrating immune cells in cervical cancer. Biomed. Pharmacother. 120, 109444. doi:10.1016/j.biopha.2019.109444
Yang, S., Wu, Y., Deng, Y., Zhou, L., Yang, P., Zheng, Y., et al. (2019). Identification of a prognostic immune signature for cervical cancer to predict survival and response to immune checkpoint inhibitors. Oncoimmunology 8 (12), e1659094. doi:10.1080/2162402X.2019.1659094
Ye, S., Fox, M. I., Belmar, N. A., Sho, M., Chao, D. T., Choi, D., et al. (2017). Enavatuzumab, a humanized anti-TWEAK receptor monoclonal antibody, exerts antitumor activity through attracting and activating innate immune effector cells. J. Immunol. Res. 2017, 5737159. doi:10.1155/2017/5737159
Yu, G., Wang, L. G., Han, Y., and He, Q. Y. (2012). clusterProfiler: an R package for comparing biological themes among gene clusters. OMICS 16 (5), 284–287. doi:10.1089/omi.2011.0118
Keywords: cervical cancer, head and neck cancer, inflamed tumours, immune inhibition, immune checkpoints, prognosis
Citation: Budhwani M, Turrell G, Yu M, Frazer IH, Mehdi AM and Chandra J (2021) Immune-Inhibitory Gene Expression is Positively Correlated with Overall Immune Activity and Predicts Increased Survival Probability of Cervical and Head and Neck Cancer Patients. Front. Mol. Biosci. 8:622643. doi: 10.3389/fmolb.2021.622643
Received: 15 November 2020; Accepted: 05 February 2021;
Published: 23 March 2021.
Edited by:
Matteo Becatti, University of Firenze, ItalyReviewed by:
Gyan Bhanot, The State University of New Jersey-Busch Campus, United StatesCopyright © 2021 Budhwani, Turrell, Yu, Frazer, Mehdi and Chandra. This is an open-access article distributed under the terms of the Creative Commons Attribution License (CC BY). The use, distribution or reproduction in other forums is permitted, provided the original author(s) and the copyright owner(s) are credited and that the original publication in this journal is cited, in accordance with accepted academic practice. No use, distribution or reproduction is permitted which does not comply with these terms.
*Correspondence: Janin Chandra, j.chandra@uq.edu.au
†These authors share first authorship
‡These authors share senior authorship
Disclaimer: All claims expressed in this article are solely those of the authors and do not necessarily represent those of their affiliated organizations, or those of the publisher, the editors and the reviewers. Any product that may be evaluated in this article or claim that may be made by its manufacturer is not guaranteed or endorsed by the publisher.
Research integrity at Frontiers
Learn more about the work of our research integrity team to safeguard the quality of each article we publish.