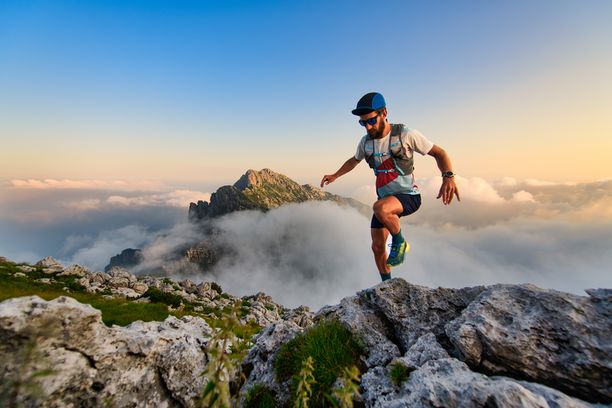
94% of researchers rate our articles as excellent or good
Learn more about the work of our research integrity team to safeguard the quality of each article we publish.
Find out more
ORIGINAL RESEARCH article
Front. Microbiol., 06 November 2024
Sec. Systems Microbiology
Volume 15 - 2024 | https://doi.org/10.3389/fmicb.2024.1495432
This article is part of the Research TopicArtificial Intelligence in Pathogenic Microorganism ResearchView all 14 articles
Objective: By extracting early chest CT radiomic features of COVID-19 patients, we explored their correlation with laboratory indicators and oxygenation index (PaO2/FiO2), thereby developed an Artificial Intelligence (AI) model based on radiomic features to predict the deterioration of oxygenation function in COVID-19 patients.
Methods: This retrospective study included 384 patients with COVID-19, whose baseline information, laboratory indicators, oxygenation-related parameters, and non-enhanced chest CT images were collected. Utilizing the PaO2/FiO2 stratification proposed by the Berlin criteria, patients were divided into 4 groups, and differences in laboratory indicators among these groups were compared. Radiomic features were extracted, and their correlations with laboratory indicators and the PaO2/FiO2 were analyzed, respectively. Finally, an AI model was developed using the PaO2/FiO2 threshold of less than 200 mmHg as the label, and the model’s performance was assessed using the area under the receiver operating characteristic curve (AUC), sensitivity and specificity. Group datas comparison was analyzed using SPSS software, and radiomic features were extracted using Python-based Pyradiomics.
Results: There were no statistically significant differences in baseline characteristics among the groups. Radiomic features showed differences in all 4 groups, while the differences in laboratory indicators were inconsistent, with some PaO2/FiO2 groups showed differences and others not. Regardless of whether laboratory indicators demonstrated differences across different PaO2/FiO2 groups, they could all be captured by radiomic features. Consequently, we chose radiomic features as variables to establish an AI model based on chest CT radiomic features. On the training set, the model achieved an AUC of 0.8137 (95% CI [0.7631–0.8612]), accuracy of 0.7249, sensitivity of 0.6626 and specificity of 0.8208. On the validation set, the model achieved an AUC of 0.8273 (95% CI [0.7475–0.9005]), accuracy of 0.7739, sensitivity of 0.7429 and specificity of 0.8222.
Conclusion: This study found that the early chest CT radiomic features of COVID-19 patients are strongly associated not only with early laboratory indicators but also with the lowest PaO2/FiO2. Consequently, we developed an AI model based on CT radiomic features to predict deterioration in oxygenation function, which can provide a reliable basis for further clinical management and treatment.
Coronavirus Disease 2019 (COVID-19) is caused by Severe Acute Respiratory Syndrome Coronavirus 2 (SARS-CoV-2) and has become a global pandemic threatening worldwide health (Sudre et al., 2021). SARS-CoV-2 infection can affect multiple organs and presents a variety of clinical symptoms (Wang et al., 2023). In the pathogenesis of COVID-19, a key factor is the dysregulation of immune inflammation (Xu et al., 2020). SARS-CoV-2 primarily enters respiratory epithelial cells by binding to angiotensin-converting enzyme 2 (ACE-2), triggering an immune inflammatory responses that results in varying degrees of damage to the alveolar epithelium, formation of hyaline membranes, and lung consolidation (Camporota et al., 2022; Caso et al., 2020; Qin et al., 2023). Therefore, the clinical symptoms of patients infected with COVID-19 exhibit significant heterogeneity; some patients are asymptomatic or exhibit only mild upper respiratory symptoms, while others may develop respiratory distress, potentially progressing to Acute Respiratory Distress Syndrome (ARDS) (Poston et al., 2020). The lungs are the organs most affected early and severely by COVID-19, and the rapid deterioration in respiratory function due to lung damage is a major cause of the high mortality rate in COVID-19 patients (Torres-Castro et al., 2021; Huang et al., 2020).
Clinically, the PaO2/FiO2 is used to represent oxygenation function and serves as a reliable predictor of acute lung injury (Matsubara et al., 2024). Since oxygenation dysfunction is an independent risk factor for progression to severe/critical COVID-19, deterioration in the PaO2/FiO2 provides an important basis for early clinical identification of worsening conditions in COVID-19 patients (Zhang et al., 2021). However, some critically ill patients may have mild clinical manifestations early in the disease, which do not correspond to the degree of oxygenation dysfunction due to severe lung damage (Tobin et al., 2020). Several laboratory indicators, such as lymphocytes, neutrophils, and pro-inflammatory cytokines, have been studied for predicting disease worsening and severe outcomes in COVID-19 patients (Del Valle et al., 2020; Zhao et al., 2020). Although these indicators reflect the immune-inflammatory status after SARS-CoV-2 infection, they are not directly indicative of oxygenation function and the extent of lung damage. Research by Fatima N et al. suggested a good correlation between early chest CT images and the PaO2/FiO2 in COVID-19 patients, indicating that chest CT can effectively assess the extent of lung damage and has potential for predicting severe cases of COVID-19 (Fatima et al., 2023; Liu F. et al., 2020).
Currently, semi-quantitative chest CT scoring systems have been developed to predict the severity and clinical outcomes of COVID-19 patients. However, these systems require radiologists to visually assess all chest CT images, which introduces considerable human error and prevents precise assessment (Wasilewski et al., 2020). Additionally, manual annotation of all infected areas for training leads to a substantial workload, making routine application challenging (Arian et al., 2023). To improve the sensitivity of COVID-19 assessment, AI-assisted quantitative analysis of chest CT is emerging as a new trend (Shaikh et al., 2021). Limited existing AI studies have extracted features such as lung lesion volume, inflammation area, and lesion density from chest CT images, with sample sizes generally around 100 cases, which limits comprehensive assessment of lung damage (Zhang et al., 2020; Pu et al., 2021; Pang et al., 2021). There is a pressing need to extract more lung features from larger samples to develop AI models that meet clinical needs for predicting severe lung damage in COVID-19 patients. Currently, researches based on AI primarily focus on employing AI techniques to analyze the different imaging findings presented in chest CT images of COVID-19 patients in order to predict disease severity and prognosis (Arian et al., 2023; Cai et al., 2020). There is a lack of comparative studies regarding oxygenation function and chest CT images using AI.
Therefore, this study will analyze the early chest CT radiomic features of COVID-19 patients using the PaO2/FiO2 as a stratification standard, exploring the correlation between early laboratory indicators, early chest CT radiomic features, and the PaO2/FiO2. We aim to establish an AI model to predict the extent of lung injury and deterioration in oxygenation function, providing a reliable basis for the early clinical management and treatment of COVID-19 patients.
This retrospective study included patients admitted to our hospital from January 1, 2023, to June 1, 2024, with a diagnosis of novel coronavirus infection.
Inclusion criteria:
1. Diagnosed with novel coronavirus infection upon admission (National Health Commission, 2023).
2. Underwent CT examination on the day of admission and multiple blood gas analyses during the hospital stay.
3. Aged ≥18 years.
Cases that may interfere with this study or where obtaining imaging data is challenging will be excluded, including:
1. Patients requiring mechanical ventilation.
2. Pregnant patients or those with end-stage cancer.
3. Patients with concurrent pulmonary diseases such as pneumothorax, pulmonary edema, or mediastinal emphysema.
4. Patients with severe cardiac or renal dysfunction.
5. Patients with incomplete clinical data (Patients with incomplete laboratory indicators relevant to this study).
6. Patients with failed image acquisition (Cases with poor image quality or missing key frames during the CT imaging process).
A total of 384 patients were ultimately included in the study. The flowchart for the inclusion and exclusion of patients is shown in Figure 1.
Relevant clinical and laboratory data from the included patients will be collected, including: Baseline Characteristics: Age, sex, BMI, smoking history, and comorbidities. Blood Gas Analysis and PaO2/FiO2: Blood gas analysis results (partial pressure of oxygen, PaO2), oxygen concentration (FiO2), and calculation of the PaO2/FiO2, with the lowest PaO2/FiO2 during hospitalization recorded. Laboratory Indicators on Admission Day: White blood cell count, neutrophil count and percentage, lymphocyte count and percentage, platelet count, C-reactive protein (CRP), D-dimer, lactate dehydrogenase (LDH), interleukin-6 (IL-6), ferritin, liver function indicators (AST, ALT), and cardiac indicators (B-type natriuretic peptide (BNP), troponin). Composite indicators such as the neutrophil-to-lymphocyte ratio (NLR), platelet-to-lymphocyte ratio (PLR), and systemic immune-inflammation index (SII) = platelet count × NLR were also calculated.
Quality control measures for laboratory indicators include: all operators complied with operational procedures, with no human-induced errors. The experimental instruments were all within their calibration periods. Reagents, quality control materials, and calibration standards for each indicator were all within their expiration dates and were properly stored. The laboratory environment’s temperature and humidity were maintained within acceptable ranges. During the experiments, all indicators passed quality control, with no random or systematic errors observed.
Chest non-enhanced CT imaging was performed on the day of admission. All scans were conducted in the supine position with the patient in the inspiratory phase. The CT scans were performed using a Philips Brilliance ICT 256-slice spiral CT scanner, with the scanning range extending from the lung apex to the level of the costophrenic angle. Scanning parameters commonly used in our center included: tube voltage of 120 kV, tube current adjusted automatically, matrix of 512 × 512, pitch of 1, conventional image thickness of 3.0 mm, and thin-slice images with 1.0 mm intervals for 3D reconstruction.
To reduce the interference of extrathoracic factors on the model, we developed a machine learning segmentation algorithm for lung segmentation. The image matrix values were first converted to attenuation values for CT images, and pixels with attenuation values less than −700 were used as a mask. After image erosion, only the largest connected domain was retained, and the mask was then expanded again to determine it as the region of interest (ROI) for the lungs, as shown in Figure 2.
Figure 2. Diagram of lung segmentation. Panels A1–A3 show chest CT cross-sectional images, while panels B1–B3 display the regions of interest (ROI) for the lungs identified by the machine learning model on chest CT. A1–B1 are axial CT images, A2–B2 are sagittal CT images, and A3–B3 are coronal CT images.
Radiomic features were extracted using Python-based Pyradiomics. Prior to feature extraction, the segmented images were preprocessed to minimize the impact of contrast and brightness variations on the radiomic features. A total of 944 radiomic features were generated for each patient, based on first-order (n = 18), shape (n = 14), texture (n = 75), Gaussian Laplacian filters (n = 93), and wavelet filters (n = 744).
In clinical practice, patients with PaO2/FiO2 less than 200 mmHg are considered to have moderate to severe ARDS and usually require mechanical ventilation (Ranieri et al., 2012; Qadir et al., 2024). Therefore, we used PaO2/FiO2 200 mmHg as the grouping criterion, dividing patients into a mechanical ventilation group (PaO2/FiO2 ≤ 200 mmHg) and a non-mechanical ventilation group (PaO2/FiO2 > 200 mmHg). The machine learning models for this study, developed using the Python sklearn library, employed various machine learning methods to predict the aforementioned labels. Model performance was evaluated using the area under the receiver operating characteristic curve (AUC), sensitivity and specificity. Internal validation was used to assess the machine learning models. During model development, the entire dataset was randomly divided into training and validation sets, and five-fold cross-validation was used for model validation.
In this study, we employed the Linear Discriminant Analysis (LDA) algorithm, a form of supervised learning, for dimensionality reduction and essential feature extraction. We extracted over 900 radiomic features for each patient in the study. By utilizing this algorithm, we aimed to reduce the number of features in the input data, enabling the representation of the output affecting labels with a minimal set of features. The fundamental concept is to project the training sample set onto a single line in such a way that the projection points of samples from the same class are as close together as possible, while the centers of the projection points from different classes are as far apart as possible (Xu et al., 2022).
Statistical analysis was performed using IBM SPSS 27.0 software. All data were tested for normality; normally distributed quantitative data were described as mean ± standard deviation, while non-normally distributed quantitative data were described as median (interquartile range). The Kruskal-Wallis test was used for comparing multiple groups, and the Mann–Whitney U test was used for multiple comparisons among groups. Categorical data were described using frequencies (percentages) and compared using the chi-square (χ2) test. A p-value of <0.05 was considered statistically significant. Statistical plots were generated using Python-based matplotlib.
A total of 384 patients were included in this study. Table 1 presents the clinical characteristics of the included patients. The median age of all patients was 71.00 (62.25, 78.00) years, and the median BMI was 24.29 (22.19, 26.88). Among the patients, 227 (59.1%) were male and 157 (40.9%) were female. A total of 105 patients (27.3%) had a history of smoking. The most common comorbidities among the included patients were hypertension, diabetes, and cardiovascular diseases. Based on the Berlin definition guidelines for ARDS (Ranieri et al., 2012), patients were divided into four groups according to their PaO2/FiO2: PaO2/FiO2 > 300 mmHg, PaO2/FiO2 200-300 mmHg, PaO2/FiO2 100-200 mmHg, and PaO2/FiO2 ≤ 100 mmHg.
There were no statistically significant differences in sex, age, BMI, smoking history, or comorbidities among the four patient groups (p > 0.05). See Table 2.
As the PaO2/FiO2 decreases, the range and density of lung lesions in the chest CT images increase. In the PaO2/FiO2 > 300 mmHg group, patients exhibit a few scattered exudative lesions in the lungs (see Figures 3A1–A3). In the PaO2/FiO2 200-300 mmHg group, patients show fewer lung lesions, primarily ground-glass opacities (GGOs) with limited extent (see Figures 3B1–B3). In the PaO2/FiO2 100-200 mmHg group, patients have a larger number of lung lesions, including GGOs and some consolidation, with a more extensive distribution (see Figures 3C1–C3). In the PaO2/FiO2 ≤ 100 mmHg group, patients present with dense lung lesions, including diffuse consolidation, with widespread distribution throughout the lungs (see Figures 3D1–D3).
Figure 3. Chest CT images of patients in different PaO2/FiO2 groups. A1–A3 are chest CT images of patients with PaO2/FiO2 > 300 mmHg; B1–B3 are chest CT images of patients with PaO2/FiO2 200-300 mmHg; C1–C3 are chest CT images of patients with PaO2/FiO2 100-200 mmHg; D1–D3 are chest CT images of patients with PaO2/FiO2 ≤ 100 mmHg. A1–D1 show axial CT; A2–D2 show sagittal CT; A3–D3 show coronal CT. The red thin arrows indicate ground-glass opacities (GGOs) and interlobular septal thickening; the blue thick arrows indicate consolidation.
As shown in Figure 4, we compared the differences in radiomic feature expressions among different groups. There are significant differences in the radiomic features among patients in different PaO2/FiO2 groups (p < 0.05). Specifically, patients in the PaO2/FiO2 ≤ 100 mmHg group show the most pronounced differences in radiomic features compared to the other three groups (Figures 4A–C). As the PaO2/FiO2 increases, the differences in radiomic features gradually decrease (Figures 4D–F).
Figure 4. Comparison of radiomic features between different PaO2/FiO2 groups via volcano plot. There are significant differences in radiomic feature expressions between different PaO2/FiO2 groups (A–F). Patients in the PaO2/FiO2 ≤ 100 mmHg group show the most pronounced differences in radiomic features compared to the other three groups (A–C). The differences in radiomic features between the PaO2/FiO2 100-200 mmHg group and the PaO2/FiO2 200-300 mmHg group, as well as the PaO2/FiO2 ≥ 300 mmHg group, are notable (D,E). There are differences in radiomic features between the PaO2/FiO2 200-300 mmHg group and the PaO2/FiO2 ≥ 300 mmHg group, but the differences are small (F). In the figure, blue points and red points represent significant differences, while gray points indicate no difference. A higher number of points indicates a greater degree of difference.
Comparing laboratory indicators across different PaO2/FiO2 groups, we observed statistical differences in immune-inflammatory indicators, coagulation indicators, and cardiac-related indicators among the four groups (p < 0.05). However, no statistical differences were found in platelet counts and liver-related indicators (AST, ALT) (p > 0.05) (see Table 3).
To clarify the specific differences between groups, we performed pairwise post-hoc comparisons (see Figure 5). We found statistically significant differences in neutrophil percentage, lymphocyte percentage, LDH, NLR, SII, and troponin across different PaO2/FiO2 groups (p < 0.05).
Figure 5. Heatmap of differences in laboratory indicators between pairs of PaO2/FiO2 groups. The x-axis represents laboratory indicators, and the y-axis represents pairwise comparisons between PaO2/FiO2 groups. Differences were analyzed using the Mann–Whitney U test. The bar in the figure indicates the p-value (0–1), with p < 0.05 indicating statistical significance and p > 0.05 indicating no statistical significance.
However, the differences in laboratory indicators such as white blood cells, neutrophils, lymphocytes, CRP, IL-6, ferritin, D-dimer, BNP, and PLR varied inconsistently among the PaO2/FiO2 groups. Specifically, differences in these indicators between the PaO2/FiO2 ≤ 100 mmHg group and the PaO2/FiO2 200-300 mmHg group, as well as the PaO2/FiO2 > 300 mmHg group, were statistically significant (p < 0.05). Conversely, white blood cells, D-dimer, IL-6, and ferritin showed no significant differences between the PaO2/FiO2 200-300 mmHg group and the PaO2/FiO2 > 300 mmHg group, or between the PaO2/FiO2 200-300 mmHg group and the PaO2/FiO2 100-200 mmHg group (p > 0.05). Therefore, the direct correlation between laboratory indicators and PaO2/FiO2 is not clear, and these indicators cannot accurately reflect the PaO2/FiO2 status.
As shown in Figure 6A, correlation analysis between the aforementioned laboratory indicators and radiomic features reveals that changes in laboratory indicators are directly reflected in the patients’ chest CT images and are sharply captured by radiomic features. Among these radiomic features that can capture laboratory indicators, the majority show significant differences between different PaO2/FiO2 groups, regardless of whether the laboratory indicators themselves differ between the PaO2/FiO2 groups, as illustrated in Figure 6B.
Figure 6. Texture feature map showing the correlation between laboratory indicators and radiomic features. In pairwise comparisons of different PaO2/FiO2 groups, different laboratory indicators are directly captured by the intensity of radiomic features (A). Radiomic features that both capture laboratory indicators and show differences between different PaO2/FiO2 groups are illustrated in (B).
In different PaO2/FiO2 groups, there are statistically significant differences in radiomic features. Additionally, regardless of whether laboratory indicators have differences between PaO2/FiO2 groups, they can be captured by radiomic features. Therefore, we selected only radiomic features as variables and established a chest CT radiomic features AI model. To efficiently and accurately predict whether COVID-19 patients require mechanical ventilation due to decreased PaO2/FiO2, we combined patients with PaO2/FiO2 ≤ 100 mmHg and 100-200 mmHg into the mechanical ventilation group, and those with PaO2/FiO2 200-300 mmHg and > 300 mmHg into the non-mechanical ventilation group.
On the training set, the model’s AUC was 0.8137 (95% CI [0.7631–0.8612]), with an accuracy of 0.7249, sensitivity of 0.6626, and specificity of 0.8208. On the validation set, the model’s AUC was 0.8273 (95% CI [0.7475–0.9005]), with an accuracy of 0.7739, sensitivity of 0.7429, and specificity of 0.8222, as shown in Figure 7.
This study is the first to use machine learning methods to segment lung ROIs and extract radiomic features from early chest CT images of over 380 COVID-19 patients. We utilized these radiomic features as intermediate variables to explore the direct and indirect correlations between laboratory indicators and PaO2/FiO2, thereby validating the assessment capability of CT—one of the most commonly used imaging modalities for COVID-19—of the overall physiological and pathological state represented by laboratory indicators. Finally, we developed an AI model based on early chest CT radiomic features of COVID-19 patients to predict whether mechanical ventilation would be required due to a decrease in PaO2/FiO2.
SARS-CoV-2 infection can trigger a robust immune response (Gallais et al., 2021). Early immune response in COVID-19 plays a protective role in viral clearance, whereas an excessive immune response can release an overabundance of pro-inflammatory cytokines and chemokines, leading to cytokine storms and systemic immune cascade reactions, which in turn alter laboratory immune-inflammatory indicators and coagulation indicators (Alzaabi et al., 2021; Chen, R. et al., 2020). Additionally, exacerbated and dysregulated immune responses can cause multi-organ damage, with the lungs being among the earliest and most severely affected organs (Chen N. et al., 2020). Researches by Liu and Fu et al. have demonstrated that laboratory indicators can be used to predict the overall deterioration and adverse outcomes in COVID-19 patients (Fu et al., 2020; Liu J. et al., 2020). However, to date, there have been no studies directly predicting the degree of lung injury and oxygenation function through the analysis of changes in laboratory indicators. To address this, we explored whether multiple laboratory indicators directly correlate with the PaO2/FiO2. Results showed that while there are overall differences in laboratory indicators across four different PaO2/FiO2 groups, the differences are inconsistent when comparing pairwise groups. For example, white blood cell count, CRP, IL-6, and ferritin were statistically significant between the PaO2/FiO2 ≤ 100 mmHg group and the PaO2/FiO2 200-300 mmHg group, but there were no statistical differences between the PaO2/FiO2 200-300 mmHg group and the PaO2/FiO2 > 300 mmHg group. These results suggest that laboratory indicators alone do not fully and accurately assess oxygenation function and the extent of lung damage in COVID-19 patients. Therefore, more appropriate assessment indicators are needed clinically.
We subsequently focused on early chest CT images to analyze their correlation with the PaO2/FiO2. We found significant differences in radiomic features among different PaO2/FiO2 groups, particularly in the patients with PaO2/FiO2 ≤ 100 mmHg, whose radiomic features showed a very significant difference compared to the other three groups. Thus, the radiomic features derived from non-contrast chest CT images may provide a valuable tool for predicting the PaO2/FiO2. Furthermore, we conducted a correlation analysis between radiomic features and laboratory indicators, revealing significant correlations between them. Notably, even laboratory indicators that were not directly related to the PaO2/FiO2 showed a strong association with the radiomic features, indicating that these features may also serve as accurate reflections of the body’s inflammatory response level.
This study is the first to extract radiomic features from early chest CT scans of over 380 COVID-19 patients, using the PaO2/FiO2 as the stratification criterion. We established an AI model based on early chest CT radiomic features, which achieve an accuracy of 0.8 in predicting stratification for the PaO2/FiO2 above and below 200 mmHg. Although AI-driven quantitative analysis of CT scans has shown promise in assessing clinical classifications, predicting disease progression, and evaluating sequelae in COVID-19 patients, the current research often relies on radiologists visually assessing and manually annotating CT images (Salahshour et al., 2021; Tanaka et al., 2023; Wasilewski et al., 2020). This heavy workload limits the ability to evaluate large samples, and reducing human error remains a significant challenge. Furthermore, studies utilizing AI technology for CT imaging primarily focus on identifying and analyzing specific features such as lesion volume, inflammatory area, or lesion density (Pang et al., 2021; Alilou et al., 2023; Chung et al., 2021). This narrow focus may lead to incomplete assessments and, similarly, suffers from issues related to high error margins and low accuracy.
This study departs from traditional visual assessment methods by disruptively applying computer programming languages to extract over 900 radiomic numerical features from CT images, including first-order, shape, texture, Gaussian Laplacian filters, and wavelet filters. Using machine learning for training and validation, we ultimately selected the feature parameter combinations most strongly correlated with the PaO2/FiO2 to construct a CT-AI model for lung assessment, achieving high accuracy and specificity in predicting oxygenation function. Clinically, patients with an PaO2/FiO2 below 200 mmHg generally require mechanical ventilation (Qadir et al., 2024). Santus P and Zhou W have confirmed that an PaO2/FiO2 < 200 mmHg at admission is independently associated with higher mortality, which can help clinicians identify high-risk patients early in their hospital stay (Santus et al., 2020; Zhou et al., 2021). Therefore, we selected PaO2/FiO2 200 mmHg as the threshold value in clinical practice, dividing patients into two groups: the mechanical ventilation group (including the PaO2/FiO2 ≤ 100 mmHg group and the PaO2/FiO2 100–200 mmHg group) and the non-mechanical ventilation group (including the PaO2/FiO2 200–300 mmHg group and the PaO2/FiO2 > 300 mmHg group). The results indicate that this model can predict stratification tasks with an accuracy of 0.8 for determining whether the PaO2/FiO2 is above or below 200 mmHg. This capability can assist clinicians in automatically identifying high-risk patients through early admission CT scans, effectively guiding the monitoring of critically ill patients, the need for increased oxygen supplementation, and decisions regarding mechanical ventilation.
This study does have some limitations. First, it is a single-center study, lacking multi-center data to further validate these conclusions. Second, the study only explored the AI model’s ability to predict the lowest PaO2/FiO2 during hospitalization, lacking comprehensive monitoring throughout the patient’s disease course, the further model can be established for dynamic monitoring and the prediction of the long COVID-19 in the future. Third, we used only the PaO2/FiO2 as the primary parameter for assessing COVID-19 severity, without considering other complications that may arise during the disease course. Finally, given the high heterogeneity of COVID-19, future research will further explore their corresponding mechanism and the impact of genetic susceptibility on the PaO2/FiO2.
This study found that the early chest CT radiomic features of COVID-19 patients show a strong correlation with early laboratory indicators and the lowest PaO2/FiO2. Therefore, we established an AI model based on the early chest CT radiomic characteristics of COVID-19 patients, which can be used to predict the deterioration of oxygenation function in COVID-19 patients, providing a basis for selecting further clinical management and treatment measures.
The original contributions presented in the study are included in the article/Supplementary material, further inquiries can be directed to the corresponding author.
The studies involving humans were approved by the Ethics Committee of Shengjing Hospital Affiliated to China Medical University (Ethics Number: 2021PS621K). The studies were conducted in accordance with the local legislation and institutional requirements. Written informed consent for participation was not required from the participants or the participants’ legal guardians/next of kin in accordance with the national legislation and institutional requirements.
WK: Writing – original draft, Conceptualization, Data curation, Formal analysis, Investigation, Methodology, Validation. YL: Conceptualization, Data curation, Formal analysis, Investigation, Methodology, Validation, Writing – original draft. WL: Data curation, Software, Writing – original draft. KY: Investigation, Validation, Writing – original draft. LY: Investigation, Writing – original draft. GJ: Conceptualization, Funding acquisition, Supervision, Writing – review & editing.
The author(s) declare that financial support was received for the research, authorship, and/or publication of this article. Liaoning University of Traditional Chinese Medicine’s Student Innovation and Entrepreneurship Training Program Project (202310162004x); Applied Basic Research Project of Liaoning Province in 2022 (2022JH2/101500051).
Thanks to the authors of the included studies to provide primary datas and develop the model.
The authors declare that the research was conducted in the absence of any commercial or financial relationships that could be construed as a potential conflict of interest.
All claims expressed in this article are solely those of the authors and do not necessarily represent those of their affiliated organizations, or those of the publisher, the editors and the reviewers. Any product that may be evaluated in this article, or claim that may be made by its manufacturer, is not guaranteed or endorsed by the publisher.
The Supplementary material for this article can be found online at: https://www.frontiersin.org/articles/10.3389/fmicb.2024.1495432/full#supplementary-material
Alilou, S., Zangiabadian, M., Pouramini, A., Jaberinezhad, M., Shobeiri, P., Ghozy, S., et al. (2023). Radiological findings as predictors of COVID-19 lung sequelae: a systematic review and Meta-analysis. Acad. Radiol. 30, 3076–3085. doi: 10.1016/j.acra.2023.06.002
Alzaabi, A. H., Ahmed, L. A., Rabooy, A. E., Zaabi, A. A., Alkaabi, M., AlMahmoud, F., et al. (2021). Longitudinal changes in IgG levels among COVID-19 recovered patients: a prospective cohort study. PLoS One 16:e0251159. doi: 10.1371/journal.pone.0251159
Arian, A., Mehrabi Nejad, M. M., Zoorpaikar, M., Hasanzadeh, N., Sotoudeh-Paima, S., Kolahi, S., et al. (2023). Accuracy of artificial intelligence CT quantification in predicting COVID-19 subjects' prognosis. PLoS One 18:e0294899. doi: 10.1371/journal.pone.0294899
Cai, W., Liu, T., Xue, X., Luo, G., Wang, X., Shen, Y., et al. (2020). CT quantification and machine-learning models for assessment of disease severity and prognosis of COVID-19 patients. Acad. Radiol. 27, 1665–1678. doi: 10.1016/j.acra.2020.09.004
Camporota, L., Cronin, J. N., Busana, M., Gattinoni, L., and Formenti, F. (2022). Pathophysiology of coronavirus-19 disease acute lung injury. Curr. Opin. Crit. Care 28, 9–16. doi: 10.1097/MCC.0000000000000911
Caso, F., Costa, L., Ruscitti, P., Navarini, L., Del Puente, A., Giacomelli, R., et al. (2020). Could Sars-coronavirus-2 trigger autoimmune and/or autoinflammatory mechanisms in genetically predisposed subjects? Autoimmun. Rev. 19:102524. doi: 10.1016/j.autrev.2020.102524
Chen, R., Sang, L., Jiang, M., Yang, Z., Jia, N., Fu, W., et al. (2020). Longitudinal hematologic and immunologic variations associated with the progression of COVID-19 patients in China. J. Allergy Clin. Immunol. 146, 89–100. doi: 10.1016/j.jaci.2020.05.003
Chen, N., Zhou, M., Dong, X., Qu, J., Gong, F., Han, Y., et al. (2020). Epidemiological and clinical characteristics of 99 cases of 2019 novel coronavirus pneumonia in Wuhan, China: a descriptive study. Lancet 395, 507–513. doi: 10.1016/S0140-6736(20)30211-7
Chung, H., Ko, H., Kang, W. S., Kim, K. W., Lee, H., Park, C., et al. (2021). Prediction and feature importance analysis for severity of COVID-19 in South Korea using artificial intelligence: model development and validation. J. Med. Internet Res. 23:e27060. doi: 10.2196/27060
Del Valle, D. M., Kim-Schulze, S., Huang, H. H., Beckmann, N. D., Nirenberg, S., Wang, B., et al. (2020). An inflammatory cytokine signature predicts COVID-19 severity and survival. Nat. Med. 26, 1636–1643. doi: 10.1038/s41591-020-1051-9
Fatima, N., Khokhar, S. A., and Farooq Ur Rehman, R. M. (2023). Correlation between oxygen saturation of patient and severity index of Covid 19 pneumonia on CT. J. Pak. Med. Assoc. 73, 60–63. doi: 10.47391/JPMA.5586
Fu, J., Kong, J., Wang, W., Wu, M., Yao, L., Wang, Z., et al. (2020). The clinical implication of dynamic neutrophil to lymphocyte ratio and D-dimer in COVID-19: a retrospective study in Suzhou China. Thromb. Res. 192, 3–8. doi: 10.1016/j.thromres.2020.05.006
Gallais, F., Velay, A., Nazon, C., Wendling, M. J., Partisani, M., Sibilia, J., et al. (2021). Intrafamilial exposure to SARS-CoV-2 associated with cellular immune response without seroconversion, France. Emerg. Infect. Dis. 27, 113–121. doi: 10.3201/eid2701.203611
Huang, C., Wang, Y., Li, X., Ren, L., Zhao, J., Hu, Y., et al. (2020). Clinical features of patients infected with 2019 novel coronavirus in Wuhan, China. Lancet 395, 497–506. doi: 10.1016/S0140-6736(20)30183-5
Liu, J., Li, S., Liu, J., Liang, B., Wang, X., Wang, H., et al. (2020). Longitudinal characteristics of lymphocyte responses and cytokine profiles in the peripheral blood of SARS-CoV-2 infected patients. EBioMedicine 55:102763. doi: 10.1016/j.ebiom.2020.102763
Liu, F., Zhang, Q., Huang, C., Shi, C., Wang, L., Shi, N., et al. (2020). CT quantification of pneumonia lesions in early days predicts progression to severe illness in a cohort of COVID-19 patients. Theranostics 10, 5613–5622. doi: 10.7150/thno.45985
Matsubara, S., Sudo, K., Kushimoto, K., Yoshii, R., Inoue, K., Kinoshita, M., et al. (2024). Prediction of acute lung injury assessed by chest computed tomography, oxygen saturation/fraction of inspired oxygen ratio, and serum lactate dehydrogenase in patients with COVID-19. J. Infect. Chemother. 30, 406–416. doi: 10.1016/j.jiac.2023.11.013
National Health Commission (2023). NHC of the PRC and National Administration of Traditional Chinese Medicine of the PRC. 10th version of the National Health Commission of China’s guidelines for diagnosis and treatment of novel coronavirus. Beijing: National Health Commission.
Pang, B., Li, H., Liu, Q., Wu, P., Xia, T., Zhang, X., et al. (2021). CT quantification of COVID-19 pneumonia at admission can predict progression to critical illness: a retrospective multicenter cohort study. Front. Med. 8:689568. doi: 10.3389/fmed.2021.689568
Poston, J. T., Patel, B. K., and Davis, A. M. (2020). Management of Critically ill Adults with COVID-19. JAMA 323, 1839–1841. doi: 10.1001/jama.2020.4914
Pu, J., Leader, J. K., Bandos, A., Ke, S., Wang, J., Shi, J., et al. (2021). Automated quantification of COVID-19 severity and progression using chest CT images. Eur. Radiol. 31, 436–446. doi: 10.1007/s00330-020-07156-2
Qadir, N., Sahetya, S., Munshi, L., Summers, C., Abrams, D., Beitler, J., et al. (2024). An update on Management of Adult Patients with acute respiratory distress syndrome: an official American Thoracic Society clinical practice guideline. Am. J. Respir. Crit. Care Med. 209, 24–36. doi: 10.1164/rccm.202311-2011ST
Qin, R., He, L., Yang, Z., Jia, N., Chen, R., Xie, J., et al. (2023). Identification of parameters representative of immune dysfunction in patients with severe and fatal COVID-19 infection: a systematic review and Meta-analysis. Clin. Rev. Allergy Immunol. 64, 33–65. doi: 10.1007/s12016-021-08908-8
Ranieri, V. M., Rubenfeld, G. D., Thompson, B. T., Ferguson, N. D., Caldwell, E., Fan, E., et al. (2012). Acute respiratory distress syndrome: the Berlin Definition. JAMA 307, 2526–2533. doi: 10.1001/jama.2012.5669
Salahshour, F., Mehrabinejad, M. M., Nassiri Toosi, M., Gity, M., Ghanaati, H., Shakiba, M., et al. (2021). Clinical and chest CT features as a predictive tool for COVID-19 clinical progress: introducing a novel semi-quantitative scoring system. Eur. Radiol. 31, 5178–5188. doi: 10.1007/s00330-020-07623-w
Santus, P., Radovanovic, D., Saderi, L., Marino, P., Cogliati, C., De Filippis, G., et al. (2020). Severity of respiratory failure at admission and in-hospital mortality in patients with COVID-19: a prospective observational multicentre study. BMJ Open 10:e043651. doi: 10.1136/bmjopen-2020-043651
Shaikh, F., Andersen, M. B., Sohail, M. R., Mulero, F., Awan, O., Dupont-Roettger, D., et al. (2021). Current landscape of imaging and the potential role for artificial intelligence in the management of COVID-19. Curr. Probl. Diagn. Radiol. 50, 430–435. doi: 10.1067/j.cpradiol.2020.06.009
Sudre, C. H., Murray, B., Varsavsky, T., Graham, M. S., Penfold, R. S., Bowyer, R. C., et al. (2021). Attributes and predictors of long COVID. Nat. Med. 27, 626–631. doi: 10.1038/s41591-021-01292-y
Tanaka, H., Maetani, T., Chubachi, S., Tanabe, N., Shiraishi, Y., Asakura, T., et al. (2023). Clinical utilization of artificial intelligence-based COVID-19 pneumonia quantification using chest computed tomography - a multicenter retrospective cohort study in Japan. Respir. Res. 24:241. doi: 10.1186/s12931-023-02530-2
Tobin, M. J., Laghi, F., and Jubran, A. (2020). Why COVID-19 silent hypoxemia is baffling to physicians. Am. J. Respir. Crit. Care Med. 202, 356–360. doi: 10.1164/rccm.202006-2157CP
Torres-Castro, R., Vasconcello-Castillo, L., Alsina-Restoy, X., Solis-Navarro, L., Burgos, F., Puppo, H., et al. (2021). Respiratory function in patients post-infection by COVID-19: a systematic review and meta-analysis. Pulmonology 27, 328–337. doi: 10.1016/j.pulmoe.2020.10.013
Wang, L., Liu, T., Yue, H., Zhang, J., Sheng, Q., Wu, L., et al. (2023). Clinical characteristics and high risk factors of patients with omicron variant strain infection in Hebei, China. Front. Cell. Infect. Microbiol. 13:1294904. doi: 10.3389/fcimb.2023.1294904
Wasilewski, P. G., Mruk, B., Mazur, S., Półtorak-Szymczak, G., Sklinda, K., and Walecki, J. (2020). COVID-19 severity scoring systems in radiological imaging - a review. Pol. J. Radiol. 85, e361–e368. doi: 10.5114/pjr.2020.98009
Xu, L., Raitoharju, J., Iosifidis, A., and Gabbouj, M. (2022). Saliency-based multilabel linear discriminant analysis. IEEE Trans. Cybern. 52, 10200–10213. doi: 10.1109/TCYB.2021.3069338
Xu, Z., Shi, L., Wang, Y., Zhang, J., Huang, L., Zhang, C., et al. (2020). Pathological findings of COVID-19 associated with acute respiratory distress syndrome. Lancet Respir. Med. 8, 420–422. doi: 10.1016/S2213-2600(20)30076-X
Zhang, L., Chen, H., Zhang, L., and Chen, X. (2021). Analysis of the risk factors for progression from mild to severe cases of COVID-19. Prev. Med. 4, 751–764.
Zhang, K., Liu, X., Shen, J., Li, Z., Sang, Y., Wu, X., et al. (2020). Clinically applicable AI system for accurate diagnosis, quantitative measurements, and prognosis of COVID-19 pneumonia using computed tomography. Cell 181, 1423–1433.e11. doi: 10.1016/j.cell.2020.04.045
Zhao, Q., Meng, M., Kumar, R., Wu, Y., Huang, J., Deng, Y., et al. (2020). Lymphopenia is associated with severe coronavirus disease 2019 (COVID-19) infections: a systemic review and meta-analysis. Int. J. Infect. Dis. 96, 131–135. doi: 10.1016/j.ijid.2020.04.086
Keywords: SARS-CoV-2, COVID-19, artificial intelligence, machine learning, chest CT radiomic features, PaO2/FiO2, laboratory indicators
Citation: Kong W, Liu Y, Li W, Yang K, Yu L and Jiao G (2024) Correlation between oxygenation function and laboratory indicators in COVID-19 patients based on non-enhanced chest CT images and construction of an artificial intelligence prediction model. Front. Microbiol. 15:1495432. doi: 10.3389/fmicb.2024.1495432
Received: 12 September 2024; Accepted: 22 October 2024;
Published: 06 November 2024.
Edited by:
Chen Li, Northeastern University, ChinaReviewed by:
Yongguo Li, First Affiliated Hospital of Chongqing Medical University, ChinaCopyright © 2024 Kong, Liu, Li, Yang, Yu and Jiao. This is an open-access article distributed under the terms of the Creative Commons Attribution License (CC BY). The use, distribution or reproduction in other forums is permitted, provided the original author(s) and the copyright owner(s) are credited and that the original publication in this journal is cited, in accordance with accepted academic practice. No use, distribution or reproduction is permitted which does not comply with these terms.
*Correspondence: Guangyu Jiao, amlhb2d5QHNqLWhvc3BpdGFsLm9yZw==
†These authors have contributed equally to this work and share first authorship
Disclaimer: All claims expressed in this article are solely those of the authors and do not necessarily represent those of their affiliated organizations, or those of the publisher, the editors and the reviewers. Any product that may be evaluated in this article or claim that may be made by its manufacturer is not guaranteed or endorsed by the publisher.
Research integrity at Frontiers
Learn more about the work of our research integrity team to safeguard the quality of each article we publish.