- 1Department of Rheumatology, The Second Hospital of Shanxi Medical University, Taiyuan, Shanxi, China
- 2Shanxi Provincial Key Laboratory of Rheumatism Immune Microecology, Taiyuan, Shanxi, China
- 3Key Laboratory of Cellular Physiology at Shanxi Medical University, Ministry of Education, Taiyuan, Shanxi, China
- 4SXMU-Tsinghua Collaborative Innovation Center for Frontier Medicine, Shanxi medical university, Taiyuan, Shanxi, China
Introduction: Observational studies suggest associations between gut microbiota and polymyositis (PM) and dermatomyositis (DM), but causal relationships are unclear. We investigate the causal effects of gut microbiota on PM and DM, providing insights hoping to provide insights for future treatment and prevention.
Methods: Summary statistics of gut microbiota were obtained from a multi-ethnic Genome Wide Association Studies (GWAS) meta-analysis, including 119 taxa from 18,340 Europeans. PM/DM statistics were extracted from GWAS analyses. Mendelian randomization (MR) with IVW, MR-Egger, and weighted median methods was performed. Sensitivity analyses addressed heterogeneity and pleiotropy. Of the 119 bacterial genera studied, six showed causal links.
Results: Alloprevotella (OR: 3.075, 95% CI: 1.127–8.386, p = 0.028), Ruminococcaceae UCG003 (OR: 4.219, 95% CI: 1.227–14.511, p = 0.022), Dialister (OR: 0.273, 95% CI: 0.077–0.974, p = 0.045) were associated with PM. Anaerotruncus (OR: 0.314, 95% CI: 0.112–0.882, p = 0.028), Ruminococcaceae UCG002 (OR: 2.439, 95% CI: 1.173–5.071, p = 0.017), Sutterella (OR: 3.392, 95% CI: 1.302–8.839, p = 0.012) were related to DM. Sensitivity analyses validated these associations
Discussion: We establish causal relationships between Ruminococcaceae, Sutterella, Anaerotruncus with DM, Alloprevotella, Ruminococcaceae UCG003, and Dialister with PM. Common microbiota, like Ruminococcaceae, have significant clinical implications. These findings open up greater possibilities for the gut microbiota to contribute to the development of PM/DM and for future monitoring of the gut microbiota in patients with PM/DM.
Introduction
Polymyositis (PM) and dermatomyositis (DM) are autoimmune muscle diseases characterized by an infiltration of inflammatory cells in the muscle tissue (Ricroch and Hénard-Damave, 2016), and both belong to the category of idiopathic inflammatory myopathies (IIM) (Findlay et al., 2015). The incidence rates for these diseases range from 4.27 and 7.89 per 100,000 person-years, with prevalence rates ranging from 9.54 to 32.74 cases per 100,000 individuals (Bernatsky et al., 2009; Furst et al., 2012; Smoyer-Tomic et al., 2012). PM is more common in women and African Americans, while DM primarily affects children and adults (Amato and Barohn, 2009). Recent studies have implicated the potential role of the gut microbiota in the development of various autoimmune diseases, including PM and DM (Findlay et al., 2015). Understanding the underlying mechanisms is critical for future treatment and prevention of PM/DM (Li et al., 2021).
The gut microflora has always been a more popular topic (De Luca and Shoenfeld, 2019). Compared to other parts of the body, the intestinal flora has the largest number and variety of bacteria (Zhufeng et al., 2022), with about 35,000 species. Human gut microbes are determined by a combination of genetic, epigenetic, and dietary factors. Interestingly, several studies have shown that gut microbiota plays a key role in autoimmune diseases by altering the abundance of microbial metabolites for immunomodulatory functions (Findlay et al., 2015). In particular, the Firmicutes (FC) and Bacteroidetes (BT) phyla play important roles in nutrient metabolism, antimicrobial protection, and immunomodulation (Geuking et al., 2011; Chung et al., 2012; Jandhyala et al., 2015). Previous studies have shown that the human gut microbiota is determined by a combination of genetic, epigenetic, and dietary factors. Recent studies have revealed the potential role of gut microbiota dysbiosis in the pathogenesis of various autoimmune diseases, including PM and DM (Findlay et al., 2015). Moreover, the dysregulation of gut microbiota has been associated with several human diseases, such as inflammatory bowel disease (IBD), metabolic disorders like diabetes and obesity, allergic diseases, and neurodevelopmental disorders (Bisgaard et al., 2011; Karlsson et al., 2013; Ferreira et al., 2014). However, the specific causal relationship between different gut microorganisms and the development of PM/DM remains uncertain and requires further research (Liu et al., 2023).
To address the need for causal inference and identify the primary influencing factors within the gut microbiome, we conducted a Mendelian randomization (MR) analysis. MR analysis was employed as a driving force for further research to meet the need for causal inference and to identify key factors influencing the gut microbiome. By utilizing genetic instruments to minimize confounding factors, MR analysis enables the assessment of causal effects and aims to uncover the potential relationship between gut microbiota and polymyositis (PM) and dermatomyositis (DM). This analysis furthers understanding of the role of gut flora in PM/DM by identifying relevant gut microbes to facilitate early disease screening, implementation of interventions, and personalized healthcare (Bowden and Holmes, 2019).
Previous observational studies have emphasized the link between reduced microbial diversity and DM, but a causal relationship has not been established (Zhufeng et al., 2022). In addition, there is a dearth of research on the key gut microbiota that may impact the development of PM. This study aims to elucidate the specific gut microflora that potentially contribute to the onset of PM/DM and assess their potential as novel targets for treatment. Notably, as muscle biopsy is invasive and required for definitive PM/DM diagnosis (Findlay et al., 2015), the non-invasive, convenient, and rapid fecal testing for gut flora offers promising opportunities. It is anticipated that the use of fecal testing of intestinal flora will enable early identification of patients with PM/DM and provide personalized therapeutic interventions.
Materials and methods
Study design
This study employs a two-sample Mendelian randomization (MR) analysis, utilizing summary statistics from Genome Wide Association Studies (GWAS), to explore the potential causal effect of gut microbiota on PM and DM. This MR analysis was conducted following the Strengthening the Reporting of Observational Studies in Epidemiology using Mendelian Randomization (STROBE-MR) Statement (Skrivankova et al., 2021a,b). Single nucleotide polymorphisms (SNPs) significantly associated with the gut microbiota were selected as instrumental variables (IVs), and three assumptions were made to ensure accuracy. First, the genetic variants selected as IVs should be significantly associated with gut microbiota. Second, IVs should not be associated with any confounding factors. Third, IVs should affect the risk of PM and DM only through gut microbiota. A series of sensitivity analyses were then conducted for significant associations. The overall flowchart of the study is presented in Figure 1.
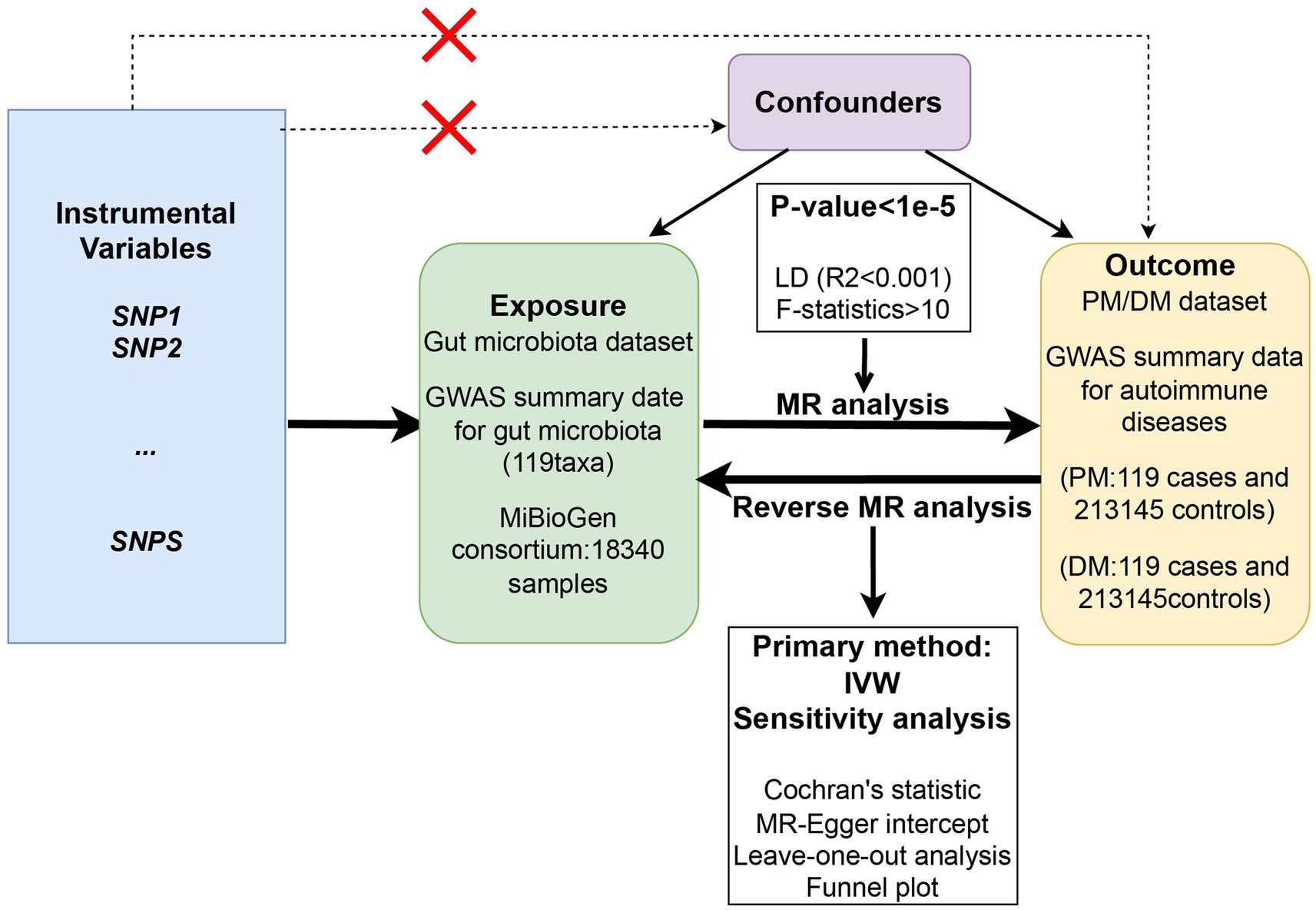
Figure 1. The overall flowchart of the study design of the two-sample Mendelian randomization for the effect of genetically predicted gut microbiome on PM/DM.
Genetic datasets
Summary statistics of the gut microbiota were obtained from a large-scale multi-ethnic GWAS meta-analysis, involving 18,340 individuals from 24 cohorts, most of which were of European ancestry (Kurilshikov et al., 2021). Genus-level taxa were analyzed, with a total of 131 genera showing an average abundance greater than 1%. However, 12 of these genera were unknown, leaving 119 genus-level taxa included in this study. Covariate adjustments were made for gender, age, technical covariates, and genetic principal components using Spearman correlation analysis.
The outcome datasets were extracted from the large publicly available GWAS analysis. Summary data for DM were obtained from a GWAS study of European ancestry, including 208 cases and 213,145 controls. For polymyositis (PM), the study included 119 cases and 213,145 controls.
Selection of IVs
Prior to the MR analysis, a rigorous screening process was conducted to ensure the reliability of the SNPs and meet the assumptions of MR analysis. The IVs were selected based on SNPs associated with gut microbiota at a genome-wide significance level (p < 1 × 10−5). A linkage disequilibrium (LD) aggregation threshold of r2 < 0.001 was applied to remove correlated and dependent SNPs to ensure independence and validity (Li et al., 2023). To assess potential confounding effects, the selected SNPs were evaluated for associations with other phenotypes using the publicly available PhenoScanner V21 (Kamat et al., 2019). SNPs related to any potential confounders were removed at genome-wide significance. Additionally, only SNPs with consistent effect alleles between the exposure and outcome GWAS datasets were included, while those absent in the outcome GWAS were removed. In addition, to eliminate the bias of weak IVs, we calculated the F-statistic for SNPs using the following formula: F = r2(n − k − 1)/[k(1 − r2)]. the value of the F-statistic indicates the strength of the IV, and F-statistic >10 is a strong IV (Ni et al., 2021).
Statistical analysis
MR analysis
To assess the causal relationship between gut microflora and outcomes, MR analysis was conducted using three methods: inverse variance weighting (IVW), MR-Egger regression, and weighted median (WME). These methods have been extensively described in previous studies (Didelez and Sheehan, 2007; Burgess et al., 2017). IVW is essentially a meta-analytic approach that translates into a weighted regression of the outcome effects of instrumental variables on exposure effects to obtain an overall estimate of the impact of the gut microbiome on PM/DM risk, where the intercept is restricted to zero (Choi et al., 2019). When there is no horizontal pleiotropy, IVW avoids the effects of confounders and obtains unbiased estimates. Besides, IVW analysis was considered the most reliable method for estimating the causal relationship between exposure and outcome while being sensitive to pleiotropy. MR-Egger regression and WME were further applied as complementary analyses (Burgess et al., 2017). For MR-egger, the traditional Mendelian approach to randomization analysis assumes that all genetic variants satisfy the instrumental variable assumptions (Burgess and Thompson, 2017). However, MR-Egger regression, which has the property of detecting and adjusting for pleiotropy in MR analysis and obtaining causal effect estimates (Bowden et al., 2016), and checking whether the results are driven by directional horizontal pleiotropy (Burgess and Thompson, 2017). Even if under an assumption that is weaker than standard instrumental variable assumptions, the slope coefficient from the Egger regression method provides an estimate of the causal effect that is consistent asymptotically even if all the genetic variants have pleiotropic effects on the outcome (Bowden et al., 2015, 2016). This is the assumption that the pleiotropic effect of a genetic variant (i.e., the direct effect of the genetic variant on the outcome without acting through risk factors) is independent of instrument strength (known as the InSIDE assumption Instrument Strength Is Independent of Direct Effect). At the same time, the MR-Egger regression provides a useful additional sensitivity analysis to the standard inverse variance weighting (IVW) method (Burgess et al., 2017). Weighted medians can provide consistent estimates of causal effects, even if up to 50 per cent of the information in the analysis comes from the change of interest being an invalid instrumental variable. The weighted median approach has some important advantages over MR-Egger because it improves the accuracy of the results. When most instrumental variables with similar causal estimates are valid, the weighted model approach remains valid even if other instrumental variables do not satisfy the MR method’s requirements for causal inference (Bowden et al., 2016; Ooi et al., 2019; Chen et al., 2022).
Sensitivity analysis
To ensure the robustness of the results, a series of sensitivity analyses were performed. Cochran’s Q statistics were used to assess heterogeneity among IVs, concluding no heterogeneity when p > 0.05 (Huedo-Medina et al., 2006). Horizontal polytropic was tested using MR-Egger regression, examining the intercept term to evaluate its impact on the MR analysis results (Burgess and Thompson, 2017). Leave-one-out analysis was used to determine if any of the SNPs were driving the causal estimates. Finally, the MR Pleiotropy Residual Sum and Outlier (MR-PRESSO) test was employed to identify and correct for outliers in the IVW linear regression, eliminating SNPs associated with heterogeneity (Frazier and Price, 1998; Wu et al., 2020).
Results
Causal effects of gut microbiota on PM and DM
As shown in Table 1 and Figure 2, we observed a certain genus-level taxa of gut microbiota have causal effects on the risk of PM and DM. Specifically, a higher genetically predicted level of Alloprevotella (OR: 2.626, 95% CI: 1.095–6.298, p = 0.031) and Ruminococcaceae UCG003 (OR: 4.219, 95% CI: 1.227–14.511, p = 0.022) was associated with an increased risk of PM, while Dialister was found to be related to a lower risk of PM (OR:0.273, 95% CI:0.077–0.974, p = 0.045). For DM, genus Anaerotruncus showed a negative correlation with DM (OR: 0.314, 95%CI: 0.112–0.882, p = 0.028), indicating a lower risk of DM, while Ruminococcaceae UCG002 (OR:2.439, 95% CI:1.173–5.071, p = 0.017) and Sutterella (OR:3.392, 95% CI:1.302–8.839, p = 0.012) were associated with an increased risk of DM. Detailed information on the specific SNPs used in the analysis is provided in the Supplementary materials.
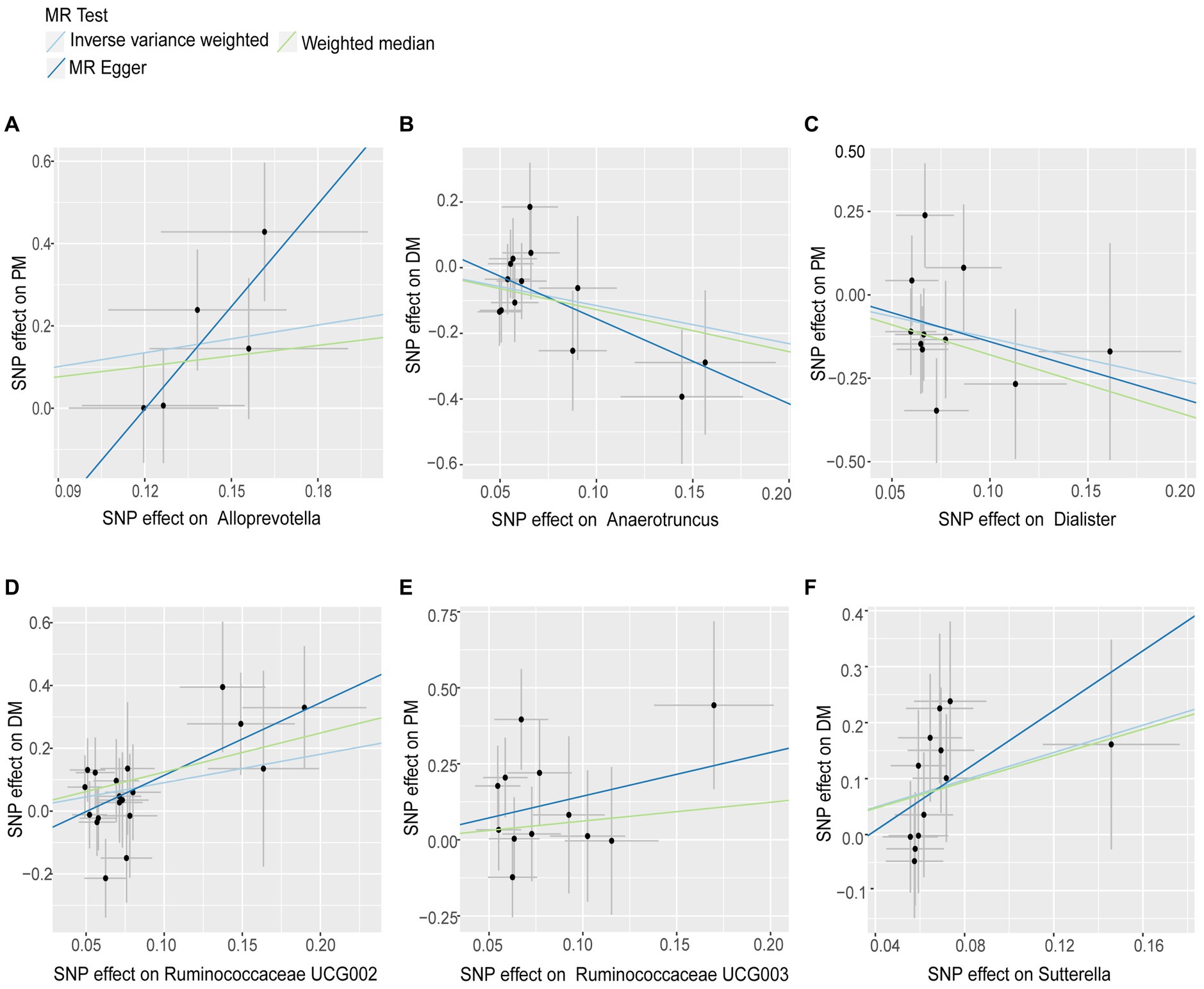
Figure 2. Scatter plots for Gut Microbiota on Polymyositis and Dermatomyositis. The pleiotropic effect was detected by testing whether the y-intercept from the MR-ER analysis was zero. Causal effects of (A) Alloprevotella, (C) Dialister and (E) Ruminococcaceae UCG003 on the risk of Polymyositis; Causal effects of (B) Anaerotruncus, (D) Ruminococcaceae UCG002 and (F) Sutterella on the risk of Dermatomyositis.
Reverse Mendelian randomization analysis
In order to explore whether PM/DM had any causal effect on the identified important bacterial genera, we also performed a reverse MR analysis using SNPs associated with PM/DM as IVs (i.e., PM/DM as the exposure and the identified pathogenic bacterial genera as the outcome), and we did not find statistically significant associations between PM/DM and the identified pathogenic bacterial genera, as shown in Table 2. We performed a series of sensitivity analyses (same as MR analyses) to ensure the robustness of the results.
Sensitivity analysis
None of the MR-Egger regression intercepts deviated from zero, indicating no evidence of horizontal pleiotropy (all intercepts p > 0.05). Additionally, the leave-one-out analysis demonstrated consistent causal estimations, suggesting that none of the identified causal associations were heavily driven by any single IV. The results of the sensitivity analysis are presented in Table 3 and Figure 3.
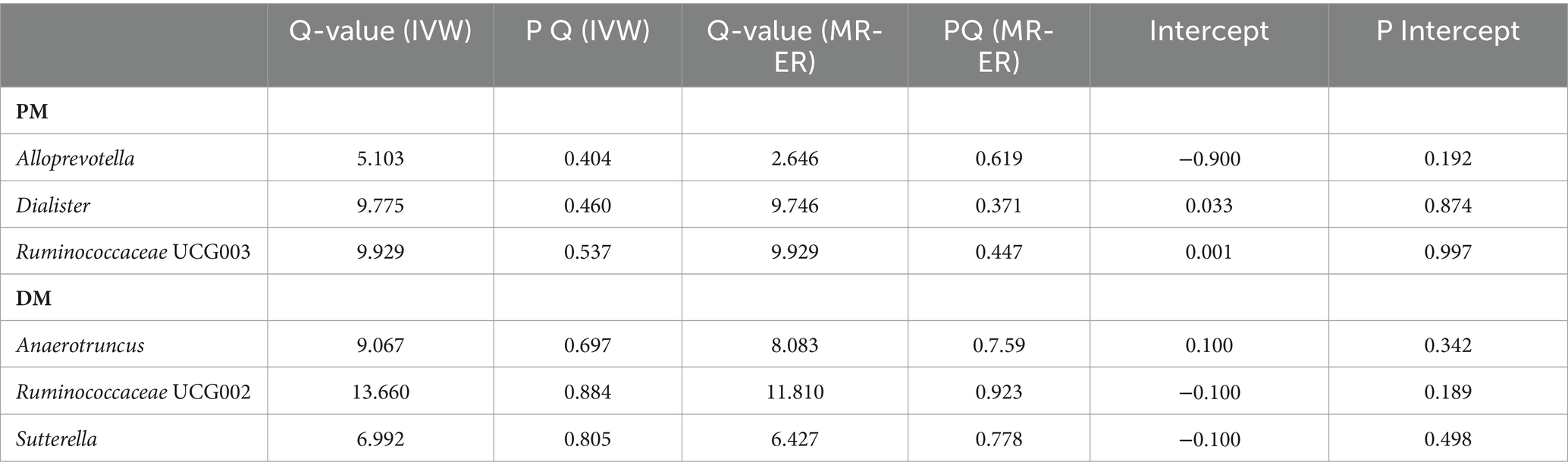
Table 3. Correlation of heterogeneity and pleiotropy tests for polymyositis and dermatomyositis with genetic predictors of gut microbiota.
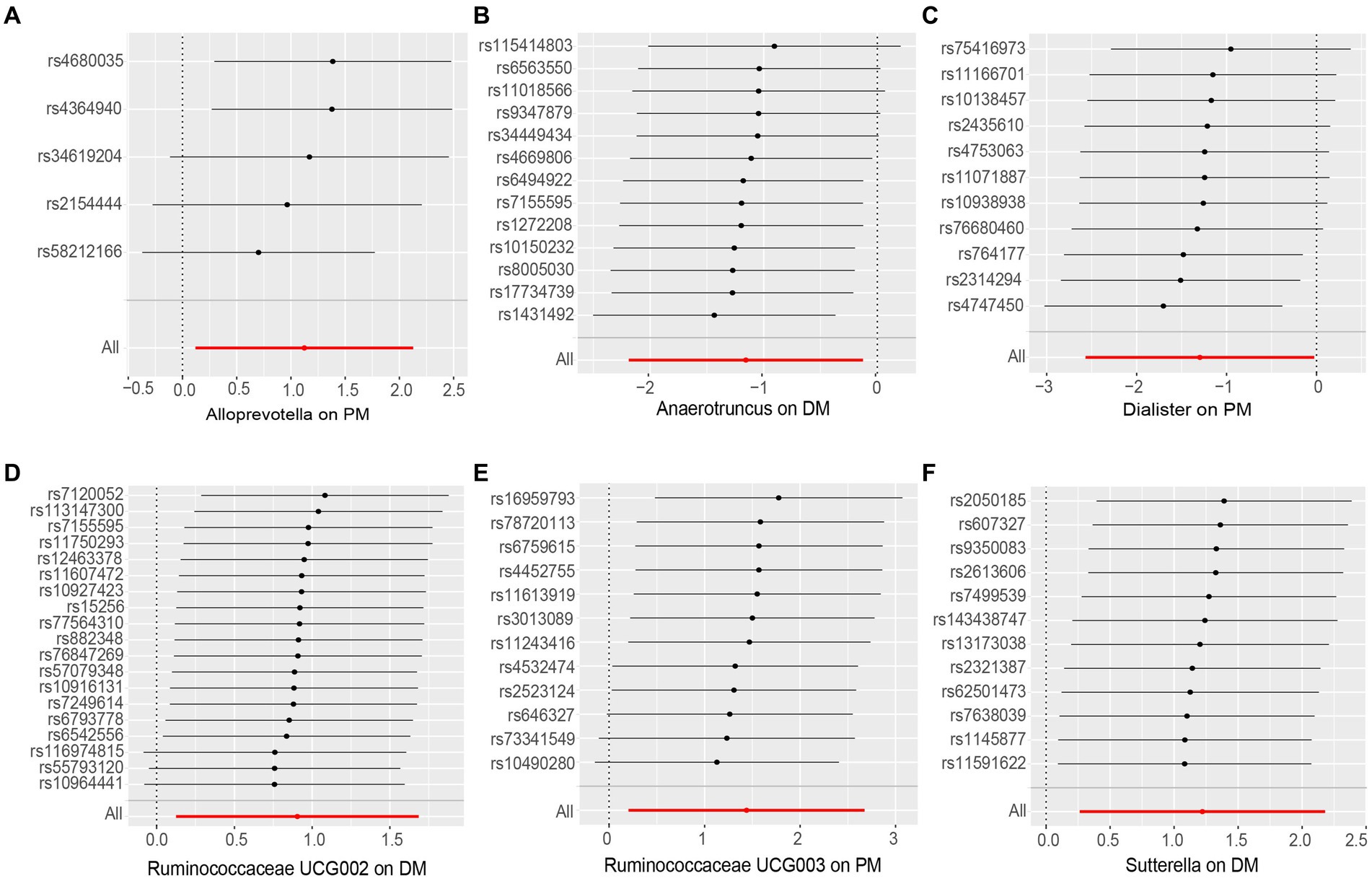
Figure 3. Leave-one-out analysis of the causal effect of Gut Microbiota on PM and DM. (A) Alloprevotella, (B) Anaerotruncus, (C) Dialister, (D) Ruminococcaceae UCG002, (E) Ruminococcaceae UCG003 and (F) Sutterella. Red lines represent estimations from the IVW test.
Discussion
This study represents the first attempt to investigate the causal relationship between gut microbiota and PM/DM using a two-sample MR analysis. Our findings reveal five different bacterial genera (Alloprevotella, Ruminococcaceae, Anaerotruncus, Sutterella, and Dialister) that are associated with PM and DM. Notably, the genus Ruminococcaceae was found to be causally involved in both conditions, which may allow us to early prevention strategies rather than just treating the disease after it has progressed (Xi et al., 2023). It is also worth noting that muscle biopsy is invasive and requires a definitive diagnosis of PM/DM (Findlay et al., 2015), and that non-invasive, convenient, and rapid fecal testing for intestinal flora offers excellent opportunities.
Gut microbes associated with PM and DM play an important role in prevention and treatment. One particular bacterium we studied, Ruminococcaceae (De Weirdt and Van de Wiele, 2015), belonging to the Clostridium sp. Blautia genus in the Lachnospiraceae family (Azzouz et al., 2019). It is a strictly anaerobic bacterium known for its ability to promote intestinal health by producing butyrate and other short-chain fatty acids (SCFA) (Gu et al., 2022). Ruminococcaceae is naturally present in the colonic mucosa of healthy individuals and has been found to play an important role in the development of various autoimmune diseases such as ankylosing spondylitis, lupus, and inflammatory bowel disease (Azzouz et al., 2019; Zhang et al., 2021). Recent reports have shown that Ruminococcaceae is present in high abundance in patients with these conditions.
Through our research, we discovered that the relative abundance of Ruminococcaceae may be related to disease activity in PM as well as DM. Although Ruminococcaceae are commonly found in low levels in the feces of healthy populations (Liu et al., 2008), even patients with mild disease activity showed higher-than-normal levels of Ruminococcaceae. We observed that patients with high disease activity had even higher levels of Ruminococcaceae, leading us to hypothesize that increased activity of this bacterium could raise the risk of developing PM/DM. Considering this association, monitoring the abundance of Rumenococcaceae flora at an early stage can provide targeted recommendations for preventive and therapeutic approaches.
Several studies have indicated that butyrate-producing bacteria promote the development of regulatory T cells (Tregs) in the gut-associated lymphatic system, which helps restore immune homeostasis and reduce the risk of autoimmune disease pathogenesis (Chen et al., 2020; Zhufeng et al., 2022). Additionally, butyrate production by Ruminococcaceae is known to enhance gut health, support epithelial cell function and morphology, and regulate the balance of intestinal flora (Zhufeng et al., 2022).
However, it is important to note that butyrate production by Ruminococcaceae inhibits histone deacetylase (HDAC), which can affect the accumulation of immune-related molecules and CD8+ T cells. The increase in CD8+ T cells has been associated with muscle fiber destruction observed in PM, suggesting a potential mechanism linking Ruminococcaceae and an increased risk of PM. Furthermore, evidence from observational studies, MR analyses, and clinical trials suggests that the effects of Ruminococcaceae may vary depending on the specific species and strain. Genomic analysis has revealed that certain Ruminococcaceae strains associated with autoimmune diseases are distinct from those found in healthy individuals (Azzouz et al., 2019). However, further investigation at a specialized genomic level is necessary to fully understand this new finding.
Overall, the interactions between Ruminococcaceae and autoimmune diseases like PM involve butyrate production, modulation of Tregs, influence on immune-related molecules and CD8+ T cells, and strain-specific determinants that require in-depth genomic analysis for better insights.
The strength of this study lies in the early monitoring of risk factors and the availability of effective treatment strategies that can minimize the burden of healthcare costs and disease suffering. Our study utilized MR to use genetic letters as IVs, thereby predicting the relationship between gut flora and PM/DM at the genetic level (Xi et al., 2023). The strengths of our study compared with previous studies are threefold: first, it is the first attempt of its kind, giving us more possibilities to study this type of disease; second, it lies in our rigorous selection of IVs and thorough sensitivity analyses to ensure the validity of our causal estimates. During the IV selection process, we employed stringent quality control measures, including the use of independent GW AS SNPs and the assessment of horizontal pleiotropy; finally, the large sample size of this study and the European origin of the participants further enhanced the reliability of our results. These advantages help to deepen our understanding of the relationship between gut flora and PM/DM and facilitate the development of therapeutic strategies for PM/DM.
Indeed, certain limitations in our study should be acknowledged. Firstly, our analysis of the intestinal flora was conducted at the genus level, and a more detailed analysis at the species or strain level could provide deeper insights and improve the accuracy of the findings. By examining specific bacterial species or strains, we could potentially identify more precise associations with PM and DM. Additionally, it is important to consider the age differences in the occurrence of PM and DM. DM shows a bimodal pattern of incidence, with peaks in childhood and later between the ages of 50 and 70 years. On the other hand, PM is rare in childhood and typically occurs after the second decade of life. Furthermore, both conditions are more common in females (Dalakas and Hohlfeld, 2003; Khan and Christopher-Stine, 2011). Gender differences are known to exist in autoimmune disorders, but our study did not explore the gender-specific effects of the gut microbiota on PM and DM. Future research should aim to investigate these potential differences and explore the specific associations between gut microbiota, gender, and PM/DM. Another aspect to be considered is the threshold used for statistical significance. In our study, we employed a p-value threshold of p < 1 × 10−5, which may have resulted in some significant associations being missed. Future studies aiming to establish a causal relationship between gut bacteria and PM/DM should consider using a more stringent threshold to ensure robust findings.
Moving forward, it is important for future studies to address these limitations. More detailed analysis at the species or strain level, consideration of age and gender differences, and the use of appropriate statistical thresholds will enable a more comprehensive understanding of the relationship between gut microbiota and PM/DM and provide more reliable strategies for early monitoring and treatment.
In Summary, our study reveals the causal impact of specific gut microbiota on PM/DM. This finding opens up new avenues for future studies of these diseases. In particular, our finding of a consistent association between Ruminococcaceae and PM and DM could provide a promising direction for early prevention of the disease. These findings contribute to a better understanding of the intricate relationship between the gut microbiota and PM/DM. In addition, more in-depth investigations at a finer taxonomic level and consideration of the influence of gender will enhance our knowledge and potentially lead to the study of therapeutic interventions targeting the gut microbiota for the effective management of these diseases. It is important to note that these findings are based on the current scientific evidence and the interpretation of the study results. For these individuals with abnormal intestinal flora, it is important to consider early and aggressive correction of intestinal dysbiosis, which can be done through dietary changes, probiotic supplementation, and increased physical activity. Especially for patients with dysbiosis of the Ruminococcaceae, direct oral administration of probiotics may be considered. Precise personalized treatments, such as individually testing the individual gut microbiota, designing probiotic strains that are relevant to a particular individual person, dietary changes, and administration of specific probiotic preparations may help to improve muscle inflammatory responses and symptoms and improve the quality of life of patients with PM/DM in the early stages of the disease, leading to secondary prevention of the disease. As research progresses, new findings and insights may further shape our understanding of these diseases and their relationship with gut microbiota. Consulting with medical professionals and experts in the field can provide personalized guidance and recommendations for individuals experiencing or at risk of developing polymyositis and dermatomyositis.
Data availability statement
The datasets presented in this study can be found in online repositories. The names of the repository/repositories and accession number(s) can be found in the article/Supplementary material.
Ethics statement
The studies involving humans were approved by the Ethics Committee of the Second Hospital of Shanxi Medical University (2019-YX-107). The studies were conducted in accordance with the local legislation and institutional requirements. Written informed consent for participation was not required from the participants or the participants’ legal guardians/next of kin because This study used the GWSA database and did not involve informed consent from patients.
Author contributions
YN: Conceptualization, Data curation, Formal analysis, Methodology, Writing – original draft. YZ: Formal analysis, Methodology, Visualization, Writing – original draft. KF: Resources, Visualization, Writing – original draft. JH: Writing – original draft. LL: Data curation, Writing – original draft. HZ: Visualization, Writing – original draft. XG: Data curation, Formal analysis, Writing – original draft. XM: Writing – original draft. SL: Visualization, Writing – original draft. MG: Writing – original draft. XL: Writing – review & editing. SZ: Funding acquisition, Resources, Writing – review & editing.
Funding
The author(s) declare that financial support was received for the research, authorship, and/or publication of this article. This project was supported by grants from the National Natural Science Foundation of China (No. 82001740) and the Natural Science Foundation of Shanxi Province (No. 202203021221269).
Acknowledgments
We thank all participants and staffs in the participating studies for their contribution to the study. We want to acknowledge the participants and investigators of the GWAS and MiBioGen consortium for sharing the genetic data.
Conflict of interest
The authors declare that the research was conducted in the absence of any commercial or financial relationships that could be construed as a potential conflict of interest.
Publisher’s note
All claims expressed in this article are solely those of the authors and do not necessarily represent those of their affiliated organizations, or those of the publisher, the editors and the reviewers. Any product that may be evaluated in this article, or claim that may be made by its manufacturer, is not guaranteed or endorsed by the publisher.
Supplementary material
The Supplementary material for this article can be found online at: https://www.frontiersin.org/articles/10.3389/fmicb.2024.1409497/full#supplementary-material
Footnotes
References
Amato, A. A., and Barohn, R. J. (2009). Inclusion body myositis: old and new concepts. J. Neurol. Neurosurg. Psychiatry 80, 1186–1193. doi: 10.1136/jnnp.2009.173823
Azzouz, D., Omarbekova, A., Heguy, A., Schwudke, D., Gisch, N., Rovin, B. H., et al. (2019). Lupus nephritis is linked to disease-activity associated expansions and immunity to a gut commensal. Ann. Rheum. Dis. 78, 947–956. doi: 10.1136/annrheumdis-2018-214856
Bernatsky, S., Joseph, L., Pineau, C. A., Bélisle, P., Boivin, J. F., Banerjee, D., et al. (2009). Estimating the prevalence of polymyositis and dermatomyositis from administrative data: age, sex and regional differences. Ann. Rheum. Dis. 68, 1192–1196. doi: 10.1136/ard.2008.093161
Bisgaard, H., Li, N., Bonnelykke, K., Chawes, B. L., Skov, T., Paludan-Müller, G., et al. (2011). Reduced diversity of the intestinal microbiota during infancy is associated with increased risk of allergic disease at school age. J. Allergy Clin. Immunol. 128:646-52.e1-5. doi: 10.1016/j.jaci.2011.04.060
Bowden, J., Davey Smith, G., and Burgess, S. (2015). Mendelian randomization with invalid instruments: effect estimation and bias detection through Egger regression. Int. J. Epidemiol. 44, 512–525. doi: 10.1093/ije/dyv080
Bowden, J., Davey Smith, G., Haycock, P. C., and Burgess, S. (2016). Consistent estimation in mendelian randomization with some invalid instruments using a weighted median estimator. Genet. Epidemiol. 40, 304–314. doi: 10.1002/gepi.21965
Bowden, J., Del Greco, M. F., Minelli, C., Davey Smith, G., Sheehan, N. A., and Thompson, J. R. (2016). Assessing the suitability of summary data for two-sample mendelian randomization analyses using MR-Egger regression: the role of the I2 statistic. Int. J. Epidemiol. 45, 1961–1974. doi: 10.1093/ije/dyw220
Bowden, J., and Holmes, M. V. (2019). Meta-analysis and mendelian randomization: a review. Res. Synth. Methods 10, 486–496. doi: 10.1002/jrsm.1346
Burgess, S., Bowden, J., Fall, T., Ingelsson, E., and Thompson, S. G. (2017). Sensitivity analyses for robust causal inference from mendelian randomization analyses with multiple genetic variants. Epidemiology 28, 30–42. doi: 10.1097/EDE.0000000000000559
Burgess, S., and Thompson, S. G. (2017). Interpreting findings from mendelian randomization using the MR-Egger method. Eur. J. Epidemiol. 32, 377–389. doi: 10.1007/s10654-017-0255-x
Chen, X., Hong, X., Gao, W., Luo, S., Cai, J., Liu, G., et al. (2022). Causal relationship between physical activity, leisure sedentary behaviors and COVID-19 risk: a mendelian randomization study. J. Transl. Med. 20:216. doi: 10.1186/s12967-022-03407-6
Chen, D., Jin, D., Huang, S., Wu, J., Xu, M., Liu, T., et al. (2020). Clostridium butyricum, a butyrate-producing probiotic, inhibits intestinal tumor development through modulating Wnt signaling and gut microbiota. Cancer Lett. 469, 456–467. doi: 10.1016/j.canlet.2019.11.019
Choi, K. W., Chen, C. Y., Stein, M. B., Klimentidis, Y. C., Wang, M. J., Koenen, K. C., et al. (2019). Assessment of bidirectional relationships between physical activity and depression among adults: a 2-sample mendelian randomization study. JAMA Psychiatry 76, 399–408. doi: 10.1001/jamapsychiatry.2018.4175
Chung, H., Pamp, S. J., Hill, J. A., Surana, N. K., Edelman, S. M., Troy, E. B., et al. (2012). Gut immune maturation depends on colonization with a host-specific microbiota. Cell 149, 1578–1593. doi: 10.1016/j.cell.2012.04.037
Dalakas, M. C., and Hohlfeld, R. (2003). Polymyositis and dermatomyositis. Lancet 362, 971–982. doi: 10.1016/S0140-6736(03)14368-1
De Luca, F., and Shoenfeld, Y. (2019). The microbiome in autoimmune diseases. Clin. Exp. Immunol. 195, 74–85. doi: 10.1111/cei.13158
De Weirdt, R., and Van de Wiele, T. (2015). Micromanagement in the gut: microenvironmental factors govern colon mucosal biofilm structure and functionality. NPJ Biofilms Microbiomes 1:15026. doi: 10.1038/npjbiofilms.2015.26
Didelez, V., and Sheehan, N. (2007). Mendelian randomization as an instrumental variable approach to causal inference. Stat. Methods Med. Res. 16, 309–330. doi: 10.1177/0962280206077743
Ferreira, C. M., Vieira, A. T., Vinolo, M. A., Oliveira, F. A., Curi, R., and Martins, F. S. (2014). The central role of the gut microbiota in chronic inflammatory diseases. J. Immunol. Res. 2014:689492. doi: 10.1155/2014/689492
Findlay, A. R., Goyal, N. A., and Mozaffar, T. (2015). An overview of polymyositis and dermatomyositis. Muscle Nerve 51, 638–656. doi: 10.1002/mus.24566
Frazier, D. L., and Price, G. S. (1998). Use of body surface area to calculate chemotherapeutic drug dose in dogs: II. Limitations imposed by pharmacokinetic factors. J. Vet. Intern. Med. 12, 272–278. doi: 10.1111/j.1939-1676.1998.tb02122.x
Furst, D. E., Amato, A. A., Iorga, Ş. R., Gajria, K., and Fernandes, A. W. (2012). Epidemiology of adult idiopathic inflammatory myopathies in a U.S. managed care plan. Muscle Nerve 45, 676–683. doi: 10.1002/mus.23302
Geuking, M. B., Cahenzli, J., Lawson, M. A., Ng, D. C., Slack, E., Hapfelmeier, S., et al. (2011). Intestinal bacterial colonization induces mutualistic regulatory T cell responses. Immunity 34, 794–806. doi: 10.1016/j.immuni.2011.03.021
Gu, X., Sim, J. X. Y., Lee, W. L., Cui, L., Chan, Y. F. Z., Chang, E. D., et al. (2022). Gut Ruminococcaceae levels at baseline correlate with risk of antibiotic-associated diarrhea. iScience. 25:103644. doi: 10.1016/j.isci.2021.103644
Huedo-Medina, T. B., Sánchez-Meca, J., Marín-Martínez, F., and Botella, J. (2006). Assessing heterogeneity in meta-analysis: Q statistic or I2 index? Psychol. Methods 11, 193–206. doi: 10.1037/1082-989X.11.2.193
Jandhyala, S. M., Talukdar, R., Subramanyam, C., Vuyyuru, H., Sasikala, M., and Nageshwar Reddy, D. (2015). Role of the normal gut microbiota. World J. Gastroenterol. 21, 8787–8803. doi: 10.3748/wjg.v21.i29.8787
Kamat, M. A., Blackshaw, J. A., Young, R., Surendran, P., Burgess, S., Danesh, J., et al. (2019). PhenoScanner V2: an expanded tool for searching human genotype-phenotype associations. Bioinformatics 35, 4851–4853. doi: 10.1093/bioinformatics/btz469
Karlsson, F., Tremaroli, V., Nielsen, J., and Bäckhed, F. (2013). Assessing the human gut microbiota in metabolic diseases. Diabetes 62, 3341–3349. doi: 10.2337/db13-0844
Khan, S., and Christopher-Stine, L. (2011). Polymyositis, dermatomyositis, and autoimmune necrotizing myopathy: clinical features. Rheum. Dis. Clin. N. Am. 37, 143–158. doi: 10.1016/j.rdc.2011.01.001
Kurilshikov, A., Medina-Gomez, C., Bacigalupe, R., Radjabzadeh, D., Wang, J., Demirkan, A., et al. (2021). Large-scale association analyses identify host factors influencing human gut microbiome composition. Nat. Genet. 53, 156–165. doi: 10.1038/s41588-020-00763-1
Li, W., Ren, A., Qin, Q., Zhao, L., Peng, Q., Ma, R., et al. (2023). Causal associations between human gut microbiota and cholelithiasis: a mendelian randomization study. Front. Cell. Infect. Microbiol. 13:1169119. doi: 10.3389/fcimb.2023.1169119
Li, B., Wang, X., Yang, C., Wen, S., Li, J., Li, N., et al. (2021). Human growth hormone proteoform pattern changes in pituitary adenomas: potential biomarkers for 3P medical approaches. EPMA J. 12, 67–89. doi: 10.1007/s13167-021-00232-7
Liu, K., Cai, Y., Song, K., Yuan, R., and Zou, J. (2023). Clarifying the effect of gut microbiota on allergic conjunctivitis risk is instrumental for predictive, preventive, and personalized medicine: a mendelian randomization analysis. EPMA J. 14, 235–248. doi: 10.1007/s13167-023-00321-9
Liu, C., Finegold, S. M., Song, Y., and Lawson, P. A. (2008). Reclassification of Clostridium coccoides, Ruminococcus hansenii, Ruminococcus hydrogenotrophicus, Ruminococcus luti, Ruminococcus productus and Ruminococcus schinkii as Blautia coccoides gen. nov., comb. nov., Blautia hansenii comb. nov., Blautia hydrogenotrophica comb. nov., Blautia luti comb. nov., Blautia producta comb. nov., Blautia schinkii comb. nov. and description of Blautia wexlerae sp. nov., isolated from human faeces. Int. J. Syst. Evol. Microbiol. 58, 1896–1902. doi: 10.1099/ijs.0.65208-0
Ni, J. J., Xu, Q., Yan, S. S., Han, B. X., Zhang, H., Wei, X. T., et al. (2021). Gut microbiota and psychiatric disorders: a two-sample mendelian randomization study. Front. Microbiol. 12:737197. doi: 10.3389/fmicb.2021.737197
Ooi, B. N. S., Loh, H., Ho, P. J., Milne, R. L., Giles, G., Gao, C., et al. (2019). The genetic interplay between body mass index, breast size and breast cancer risk: a mendelian randomization analysis. Int. J. Epidemiol. 48, 781–794. doi: 10.1093/ije/dyz124
Ricroch, A. E., and Hénard-Damave, M. C. (2016). Next biotech plants: new traits, crops, developers and technologies for addressing global challenges. Crit. Rev. Biotechnol. 36, 675–690. doi: 10.3109/07388551.2015.1004521
Skrivankova, V. W., Richmond, R. C., Woolf, B. A. R., Davies, N. M., Swanson, S. A., VanderWeele, T. J., et al. (2021a). Strengthening the reporting of observational studies in epidemiology using mendelian randomisation (STROBE-MR): explanation and elaboration. BMJ 375:n2233. doi: 10.1136/bmj.n2233
Skrivankova, V. W., Richmond, R. C., Woolf, B. A. R., Yarmolinsky, J., Davies, N. M., Swanson, S. A., et al. (2021b). Strengthening the reporting of observational studies in epidemiology using mendelian randomization: the STROBE-MR statement. JAMA 326, 1614–1621. doi: 10.1001/jama.2021.18236
Smoyer-Tomic, K. E., Amato, A. A., and Fernandes, A. W. (2012). Incidence and prevalence of idiopathic inflammatory myopathies among commercially insured, Medicare supplemental insured, and Medicaid enrolled populations: an administrative claims analysis. BMC Musculoskelet. Disord. 13:103. doi: 10.1186/1471-2474-13-103
Wu, F., Huang, Y., Hu, J., and Shao, Z. (2020). Mendelian randomization study of inflammatory bowel disease and bone mineral density. BMC Med. 18:312. doi: 10.1186/s12916-020-01778-5
Xi, Y., Zhang, C., Feng, Y., Zhao, S., Zhang, Y., Duan, G., et al. (2023). Genetically predicted the causal relationship between gut microbiota and infertility: bidirectional mendelian randomization analysis in the framework of predictive, preventive, and personalized medicine. EPMA J. 14, 405–416. doi: 10.1007/s13167-023-00332-6
Zhang, S. X., Wang, J., Chen, J. W., Zhang, M. X., Zhang, Y. F., Hu, F. Y., et al. (2021). The level of peripheral regulatory T cells is linked to changes in gut commensal microflora in patients with systemic lupus erythematosus. Ann. Rheum. Dis. 80:e177. doi: 10.1136/annrheumdis-2019-216504
Zhufeng, Y., Xu, J., Miao, M., Wang, Y., Li, Y., Huang, B., et al. (2022). Modification of intestinal microbiota dysbiosis by low-dose interleukin-2 in dermatomyositis: a post hoc analysis from a clinical trial study. Front. Cell. Infect. Microbiol. 12:757099. doi: 10.3389/fcimb.2022.757099
Glossary
Keywords: causal relationship, dermatomyositis, gut microbiota, Mendelian randomization, polymyositis
Citation: Niu Y, Zhang Y, Fan K, Hou J, Liu L, Zhang H, Geng X, Ma X, Lin S, Guo M, Li X and Zhang S (2024) Genetically predicted the causal relationship between gut microbiota and the risk of polymyositis/dermatomyositis: a Mendelian randomization analysis. Front. Microbiol. 15:1409497. doi: 10.3389/fmicb.2024.1409497
Edited by:
Georgia Damoraki, National and Kapodistrian University of Athens, GreeceReviewed by:
Le Liu, Southern Medical University, ChinaVasiliki Bourika, National and Kapodistrian University of Athens, Greece
Copyright © 2024 Niu, Zhang, Fan, Hou, Liu, Zhang, Geng, Ma, Lin, Guo, Li and Zhang. This is an open-access article distributed under the terms of the Creative Commons Attribution License (CC BY). The use, distribution or reproduction in other forums is permitted, provided the original author(s) and the copyright owner(s) are credited and that the original publication in this journal is cited, in accordance with accepted academic practice. No use, distribution or reproduction is permitted which does not comply with these terms.
*Correspondence: Shengxiao Zhang, zhangshengxiao1@sxmu.edu.cn