- 1Department of Pharmacy, Guangdong Provincial People's Hospital, Guangdong Academy of Medical Sciences, Southern Medical University, Guangzhou, Guangdong, China
- 2Department of Neurosurgery, Huizhou Third People's Hospital, Guangzhou Medical University, Huizhou, Guangdong, China
- 3School of Medicine, South China University of Technology, Guangzhou, Guangdong, China
- 4Guangdong Cardiovascular Institute, Guangdong Provincial People's Hospital (Guangdong Academy of Medical Sciences), Southern Medical University, Guangzhou, China
Background: Inflammation serves as a key pathologic mediator in the progression of infections and various diseases, involving significant alterations in the gut microbiome and metabolism. This study aims to probe into the potential causal relationships between gut microbial taxa and human blood metabolites with various serum inflammatory markers (CRP, SAA1, IL-6, TNF-α, WBC, and GlycA) and the risks of seven common infections (gastrointestinal infections, dysentery, pneumonia, bacterial pneumonia, bronchopneumonia and lung abscess, pneumococcal pneumonia, and urinary tract infections).
Methods: Two-sample Mendelian randomization (MR) analysis was performed using inverse variance weighted (IVW), maximum likelihood, MR-Egger, weighted median, and MR-PRESSO.
Results: After adding other MR models and sensitivity analyses, genus Roseburia was simultaneously associated adversely with CRP (Beta IVW = −0.040) and SAA1 (Beta IVW = −0.280), and family Bifidobacteriaceae was negatively associated with both CRP (Beta IVW = −0.034) and pneumonia risk (Beta IVW = −0.391). After correction by FDR, only glutaroyl carnitine remained significantly associated with elevated CRP levels (Beta IVW = 0.112). Additionally, threonine (Beta IVW = 0.200) and 1-heptadecanoylglycerophosphocholine (Beta IVW = −0.246) were found to be significantly associated with WBC levels. Three metabolites showed similar causal effects on different inflammatory markers or infectious phenotypes, stearidonate (18:4n3) was negatively related to SAA1 and urinary tract infections, and 5-oxoproline contributed to elevated IL-6 and SAA1 levels. In addition, 7-methylguanine showed a positive correlation with dysentery and bacterial pneumonia.
Conclusion: This study provides novel evidence confirming the causal effects of the gut microbiome and the plasma metabolite profile on inflammation and the risk of infection. These potential molecular alterations may aid in the development of new targets for the intervention and management of disorders associated with inflammation and infections.
Introduction
The inflammatory response is a vital component of immunity and acts as a critical mechanism against damage and infection. However, inflammation will become harmful when it loses control, spreads becomes systemic, or lasts for extended periods and becomes chronic. Inflammation can cause cellular injury, tissue destruction, cancer, organ failure, and death (Wang and Ma, 2008; Greten and Grivennikov, 2019), playing a key role in the pathology of various diseases. Infection represents a local tissue and systemic inflammatory response caused by pathogens invading the human body. Gastrointestinal infections (GI), pneumonia, and urinary tract infections (UTI) are common infections causing hospitalization and death (Collaborators, 2017). Therefore, it is essential to prevent and manage inflammation and infectious disorders (including their subtypes) with appropriate intervention strategies.
Systemic inflammation and infection are characterized by an increased release of cytokines and acute-phase proteins (APPs), as well as changes in the components of the blood (Slaats et al., 2016; Liu et al., 2017). Some of the most routinely used markers for inflammation and infection in clinical practice (Dos Anjos and Grotto, 2010; Menzel et al., 2021; Yin and Mo, 2022) include cytokines (e.g., interleukin-6 [IL-6] and tumor necrosis factor-alpha [TNF-α]), APPs (e.g., C-reactive protein [CRP] and serum amyloid a [SAA]), and blood cell counts (e.g., white blood cell count [WBC]), representing several components of the inflammatory process. Glycoprotein acetylation (GlycA) is a novel biomarker of systemic inflammation and cardiovascular disease (Connelly et al., 2017), reflecting both increased glycan complexity and circulating APPs. Elevated levels of these markers in the blood can be early signs of health effects (Qu et al., 2015; Zacho et al., 2016; Yin and Mo, 2022) and are clinically useful in tracing and detecting inflammatory severity and infectious risks, diagnosing, and following-up on diseases (Ponti et al., 2020; Menzel et al., 2021). However, most of the molecular mechanisms underlying inter-individual variation in systemic inflammation and the infectious risks remain to be illustrated.
It has been revealed that certain environmental and lifestyle factors can promote systemic inflammation, thereby contributing to disease development, severe disability, and mortality (Furman et al., 2019). The metabolome defines metabolic perturbations resulting from the interplay between the genome and environmental factors, representing an immediate host response to environmental exposures and pathological processes (Kaddurah-Daouk and Krishnan, 2009). Prior metabolomic studies have pinpointed specific metabolic changes associated with the urea cycle and oxidative stress, which correlate with inflammatory markers in healthy individuals (Pietzner et al., 2017) and rheumatoid arthritis patients (Jutley et al., 2021). Importantly, metabolomics was also widely applied to identify infection biomarkers (Araújo et al., 2022). Moreover, microbiota balance has a powerful regulatory effect on the human immune and metabolic systems (Samuelson et al., 2015). The gut microbiota, including its composition and metabolites such as lipopolysaccharides, bile acids, and short-chain fatty acids, also play important roles in inflammation development, significantly affecting host health (Al Bander et al., 2020; Tilg et al., 2020). At present, probiotics and micronutrients have been demonstrated to modulate some immune and inflammatory biomarkers and reduce the risk and severity of gastrointestinal and respiratory infections (Calder et al., 2022). Thus, the gut microbiota and metabolic signature are closely related to the host's immune-inflammatory response, potentially influencing the susceptibility to infections.
While research on the gut microbiome and blood metabolites is growing, the causal relationships between these factors and inflammation as well as the risk of infection remain poorly understood. Mendelian randomization (MR) is a powerful tool for inferring causal effects between exposures and outcomes (Davies et al., 2018). By leveraging genetic variants associated with the exposure, MR helps overcome the limitations of traditional observational studies, such as confounding and reverse causation. Therefore, we employed a two-sample MR approach to investigate the causal effects of 195 gut bacterial traits and 529 (290 annotated) human blood metabolites on inflammation and the risk of infections, aiming to gain deeper molecular insights. Our findings shed new light on the intricate relationships between the gut microbiome, blood metabolites, and inflammation and the risk of infections, which may have significant implications for preventing and treating inflammatory symptoms and infectious diseases.
Materials and methods
The study flow is depicted in Figure 1. A two-sample MR analysis was employed to assess the potential causal relationship between gut microbiota composition, blood metabolite levels, and nine outcomes. The MR analysis is based on the following three assumptions to avoid the causal estimates from being biased: (1) instrumental variables (IVs) are strongly associated with the exposure; (2) IVs are not correlated with the confounders; and (3) IVs can only affect the outcome through the exposure.
Data source
The genetic predictors of human gut microbiome composition were obtained from a large-scale, multi-ethnic meta-analysis of microbiome-based GWAS, the MiBioGen study (Kurilshikov et al., 2021). This meta-analysis included 18,340 participants from 24 cohorts. Microbial abundance was based on 16S rRNA gene data, and the use of the direct taxonomic classification of reads, along with an up-to-date reference database, allowed for good concordance of taxonomic composition across domains and a higher mapping rate. After quality control (including duplication of the family Bifidobacteriaceae with the order Bifidobacteriales in the results of the GWAS, and deletion of larger taxonomic units of bacteria), a total of 195 microbial genera (119 genera, 32 families, 19 orders, 16 classes, and 9 phyla) were retained. Supplementary Table S1 provides a comprehensive list of all datasets used.
The metabolite GWAS dataset used in this study includes the largest meta-GWAS data to date, as published in Nature Genetics in 2014 by Shin et al. (2014). The dataset consists of a meta-analysis of seven European populations, comprising a total of 7,824 individuals of European ancestry. Following rigorous quality control procedures, 290 annotated blood metabolites (Supplementary Table S2) were included in the genome-wide association analysis.
CRP: The GWAS result of serum CRP levels was conducted within a large, population-based cohort of half a million individuals in the United Kingdom, aged between 40 and 69 years (Bycroft et al., 2018). Han et al. (2020) accessed serum CRP levels for 469,881 individuals from the 2019 serum biochemistry data release. The researchers calculated the mean serum CRP levels for individuals who had undergone two assessments. Prior to the GWAS analysis, a rank-based inverse normal transformation was applied to serum CRP levels to ensure a normal data distribution.
SAA1: A study integrates large-scale genomic and aptamer-based plasma proteomic data from 10,708 individuals prior to any SARS-CoV-2 infection or COVID-19 (Pietzner et al., 2020).
IL-6: The GWAS data for IL-6 is obtained from a study encompassing 90 cardiovascular proteins, involving more than 30,000 individuals from 15 distinct studies (Folkersen et al., 2020).
TNF-α: The TNF-α GWAS results were obtained from a GWAS meta-analysis that investigated the circulating concentrations of 41 cytokines, involving up to 8,293 Finnish individuals from three independent population cohorts (Ahola-Olli et al., 2017).
WBC: The WBC data are sourced from an extensive study providing genetic insights into blood cells across diverse populations, including 184,535 individuals of non-European ancestry, from a total of 746,667 individuals across five global populations (Chen et al., 2020).
GlycA: The GlycA GWAS meta-analysis was based on data from 249 metabolites quantified using nuclear magnetic resonance spectroscopy (NMR) in the UKB by Nightingale Health in 2020. Data can be accessed at https://gwas.mrcieu.ac.uk/datasets/met-d-GlycA/.
GWAS meta-analyses for GI (Phecode:008), pneumonia (Phecode:480), bacterial pneumonia (BP) (Phecode:480.1), bronchopneumonia, and lung abscess (BLA) (Phecode:480.5), pneumococcal pneumonia (PP) (Phecode:480.11), and UTI (Phecode:591) were derived using the GLMM-based GWA tool, fastGWA-GLMM. Application to UKB data included 456,348 individuals, 11,842,647 variants, and 2,989 binary traits (Jiang et al., 2021a). The meta-GWAS data for dysentery (Phecode:008.5) comes from meta-analysis across populations in three cohorts, namely, UKB, BioBank Japan, and FinnGen (Sakaue et al., 2021). All patients' diseases are systematically defined using the phecode framework; each phenotype (“phecode”) has defined case, control, and exclusion criteria (Denny et al., 2013).
Selection of IVs
Additional quality control steps were implemented to select candidate IVs. Candidate IVs from the GWAS meta-results of gut microbiota and metabolites were included using a relatively relaxed standard of P < 1e−5 (Sanna et al., 2019). Considering that single nucleotide polymorphism (SNP) variations may affect several metabolites in the same pathway, thus violating the assumptions of the MR, a restrictive SNP selection is applied to metabolite research, excluding those that are significantly associated with more than two metabolites (Hwang et al., 2019). Genomic samples from the 1,000 Genomes Project (EUR) served as a linkage disequilibrium (LD) reference panel, and independent SNPs for gut bacteria and metabolites were retained using standards of R2 < 0.001, window size = 10,000 kb and R2 < 0.01, window size = 500 kb, respectively. The F-statistic, typically used to assess the strength of the correlation between IV and exposure, was calculated using the formula 1: F = R2 (n-k-1)/k(1-R2), where R2 is the variance of exposure explained by the selected SNP, n is the sample size, and k is the number of IVs included. IVs with an F-statistic of < 10 were considered weak and were excluded (Pierce et al., 2011). For metabolites, we used formula 2: F = (PVE(n-k-1))/(1-PVE) k, where PVE is the proportion of exposure variance for the selected IV. By setting k equal to 1, the PVE for each IV was calculated using the formula 3:
PVE = (2*β2*MAF*(1-MAF))/[(2*β2*MAF*(1-MAF)+2*Se2* n*MAF*(1-MAF)].
Since the gut microbiota SNPs did not provide minor allele frequencies, R2 was estimated directly using the “get_r_from_pn” function from the “TwoSampleMR” package (Hemani et al., 2017, 2018) and then using formula 1 to calculate the F-statistic. Finally, horizontal pleiotropic effects, i.e., confounding effects caused by other diseases, may violate the second assumption in MR analysis (SNP is unrelated to outcome). Once these were detected, the associated IVs were removed. The MR-PRESSO test was used to detect potential horizontal pleiotropy (Verbanck et al., 2018). We mitigated the influence of pleiotropy by removing outliers, specifically SNPs with a global test P-value of < 0.05 in the MR-PRESSO test. After removing these outliers, we performed a re-analysis to ensure the accuracy of our results.
Removing confounders
In adherence to the independence assumption of MR, we utilized PhenoScanner V2 to identify potential confounders for each outcome (Kamat et al., 2019). Our search parameters were the following. CRP (Luan and Yao, 2018): coronary heart disease, diabetes, Alzheimer's, Parkinson's, stroke, and macular degeneration. SAA1 (Sack, 2018): lipid profiles, atherosclerosis, cholesterol, and cancer. IL-6 (Tanaka et al., 2014): autoimmunity, chronic inflammatory diseases, inflammatory myopathies, juvenile idiopathic arthritis, rheumatoid arthritis, systemic sclerosis, and Castleman disease. TNF-α (Locksley et al., 2001; Brynskov et al., 2002; Swardfager et al., 2010): Alzheimer's, diabetes mellitus, inflammatory bowel disease, major depression, obesity, and psoriasis. WBC (Hasegawa et al., 2002): CAD and leukemia. GlycA (Connelly et al., 2017): body mass index, colorectal cancer, coronary artery disease, psoriasis, and rheumatoid arthritis. GI, dysentery (Lamps, 2009): salmonella, E. coli, giardiasis, cryptosporidiosis, viruses. Pneumonia, BP, BLA, PP (https://www.nhlbi.nih.gov/health/pneumonia/causes, accessed August 30, 2023): COPD, dementia, diabetes, heart failure, HIV, kidney diseases, Parkinson's, pregnancy, smoking, and stroke. UTI (Medina and Castillo-Pino, 2019): diabetes mellitus and viral infections. Potential confounders were subsequently excluded from the main MR analysis.
MR analysis
The focus was on two relationships: between gut bacteria and serum inflammatory factors and the risks of infections and between blood metabolites and serum inflammatory factors and the risks of infections. This study is reported following the Strengthening the Reporting of Observational Studies in Epidemiology Using Mendelian Randomization guidelines (STROBE-MR, S1 Checklist) (Supplementary material 1). First, following the criteria mentioned earlier, we sequentially excluded the IVs with LD and confounders. Second, IVs with F-statistics < 10 were excluded. Third, after rigorously screening quality IVs, we explored potential causal relationships using two-sample MR with at least three IVs. Fourth, the following five methods were used to assess these associations: the inverse variance weighted (IVW) test (Burgess et al., 2013), the weighted median estimator (WME) (Pierce and Burgess, 2013), the maximum likelihood estimator (MLE) (Pierce and Burgess, 2013), the weighted mode-based estimator (Hartwig et al., 2017), and MR-Egger regression (Bowden et al., 2015). For multiple hypothesis testing, the false discovery rate (FDR) calculation for gut microbiota was performed at different taxonomic levels, while for metabolites, it was performed among the 290 annotated metabolites. A relationship was considered potentially causal if it was statistically significant in at least three of these methods, including the IVW method (Liu et al., 2022; Guo et al., 2023). Special attention was given to the IVW method because of its robustness to MR analysis. Fifth, a series of sensitivity analyses were used to ensure the robustness of our results. Finally, we performed bidirectional MR analysis on significant results to ensure the validity of the results and to avoid confusion in the causal interpretation.
Sensitivity analysis
We used sensitivity analysis to ensure the robustness of our results and to identify potential biases such as pleiotropy and data heterogeneity. We also tested whether a particular instrumental variable significantly influenced the outcome variable. Our sensitivity analysis included a pleiotropy test, a heterogeneity test, and a leave-one-out method. For the pleiotropy test, we used the MR-PRESSO method and assumed that the horizontal pleiotropy of the IVs would not significantly affect the causal inference if the absolute value of the intercept was < 0.1 and the corresponding P-value was > 0.05. The heterogeneity test was used to identify differences between different IVs. P-value = 0.05 is the threshold value. The leave-one-out method allowed us to assess whether the MR estimate was driven or biased by a single SNP with a particularly large pleiotropic effect. This effect was re-estimated by sequentially removing one SNP at a time.
Software
For data cleaning and structuring, we used Jupyter Notebook in Python (version 3.0). To perform the MR analysis, we used R (version 4.2.1) and the “TwoSampleMR” package.
Results
Selection of IVs
The characteristics of the selected IVs for each gut microbiota are listed in Supplementary Table S3. After conducting LD analyses and eliminating confounding variables, the ultimate counts of IVs for CRP, SAA1, IL-6, TNF-α, WBC, GlycA, GI, dysentery, pneumonia, BP, BLA, PP, and UTI are 1,594, 1,959, 1,021, 1,911, 1,597, 1,871, 1,870, 1,965, 1,876, 1,875, 1,864, 1,877, and 1,888, respectively. These IVs will be employed for subsequent MR analyses. Bacterial traits with fewer than three IVs were excluded.
Supplementary Table S4 provides the details of the selected IVs for each metabolite. Of the 56,147 SNPs significantly correlated with 290 blood metabolites (P < 1 × 10−5), 23,198 SNPs linked to at least two metabolites were excluded. After performing LD analyses and accounting for confounding factors, the instrumental variable counts for CRP, SAA1, IL-6, TNF-α, WBC, GlycA, GI, dysentery, pneumonia, BP, BLA, PP, and UTI are 2,879, 3,517, 2,619, 4,416, 3,031, 4,857, 5,095, 5,125, 5,066, 5,042, 5,068, 5,056, and 5,025, respectively. These instruments will be used in subsequent MR analyses, with the exclusion of metabolites having < 3 IVs.
In all analyses of serum inflammatory markers, the F-statistics of the IVs were >10, indicating less possibility of weak instrument bias.
Two-sample MR analysis of gut microbiota on inflammation and risk of infections
We first used the IVW method as the primary analysis to evaluate the causal relationships between gut microbiota, serum inflammatory markers, and seven risks of infection (Supplementary Table S5). A total of 23 (including 14 genera), 9 (6 genera), 3 (2 genera), 8 (2 genera), 27 (16 genera), and 9 (6 genera) bacterial traits were suggested as significant for CRP, SAA1, IL-6, TNF-α, WBC, and GlycA (PIVW < 0.05). Besides, 9 (3 genera), 6 (3 genera), 7 (2 genera), 9 (2 genera), 12 (6 genera), 5 (4 genera), and 8 (3 genera) bacterial traits were associated with risks of GI, dysentery, pneumonia, BP, BLA, PP, and UTI. Then, the other four MR methods, namely, MR-Egger, maximum likelihood, weighted mode-based estimator, and weighted median-based estimator, were added to further evaluate the causal estimates. The full results of all MR methods are presented in Supplementary Table S6. There are 7, 3, 1, 2, 9, and 1 suggestive causal associations detected for CRP, SAA1, IL-6, TNF-α, WBC, and GlycA across at least three MR methods (including IVW, P < 0.05). Additionally, 3, 2, 1, 4, 0, 1, and 1 associations were confirmed for the risks of GI, dysentery, pneumonia, BP, BLA, PP, and UTI using at least three MR methods. We next performed a heterogeneity and horizontal pleiotropy test to assess the robustness of the above suggestive associations (Supplementary Table S7). The above suggestive associations, where the intercept of the MR-Egger regression approached 0 and the p-values of both the MR-Egger and MR-PRESSO global tests were >0.05, indicating no evidence of horizontal pleiotropy, were retained.
Overall, after multiple-testing correction, nine associations exceeded the strict threshold ([FDR] < 0.05, Figure 2). A genetically predicted increase in family Bifidobacteriaceae (Beta[95%CI] IVW = −0.034 [−0.055, −0.012]) and Christensenellaceae (Beta[95%CI] IVW = −0.038 [−0.062, −0.013]) demonstrated a suggestive inverse association with CRP levels. Genus Lachnospiraceae (Beta[95%CI] IVW = −0.033 [−0.052, −0.015]) and order Bacillales (Beta[95%CI] IVW = −0.016 [−0.027, −0.006]) were related to decreased WBC; genera Eggerthella (Beta[95%CI] IVW = −0.024 [0.009, 0.038]) and Sutterella (Beta[95%CI] IVW = 0.098 [0.052, 0.143]) were related to increased WBC and GlycA levels, respectively. As for the infectious risks, family Bifidobacteriaceae (Beta[95%CI] IVW = −0.391 [−0.621, −0.161]) and order Lactobacillales (Beta[95%CI] IVW = −0.374 [−0.597, −0.151]) were respectively linked to reduced pneumonia and gastrointestinal infection risk, while order Burkholderiales (Beta[95%CI] IVW = 0.533 [0.216, 0.850]) were linked to elevated GI risk. Importantly, we also observed three bacterial traits were common to two different inflammatory markers or infectious risks, such as family Bifidobacteriaceae, which was negatively associated with both CRP and pneumonia risk, and genus Roseburia, which was simultaneously associated adversely with CRP [Beta (95%CI) IVW = −0.040 (−0.066, −0.013), PIVW = 0.004] and SAA1 [Beta (95%CI) IVW = −0.280 (−0.465, −0.095), PIVW = 0.003] levels. Interestingly, the Bifidobacteriaceae family was associated with an increased risk of pneumonia subtype BP [Beta (95%CI) IVW = 0.718 (0.160, 1.276), PIVW = 0.117]. The scatterplots of the SNP effect sizes for the MR results are displayed in Figure 3 and Supplementary Figure 2. The sensitivity estimators were relatively consistent across different MR tests, with similar directions and comparable magnitudes of effect.
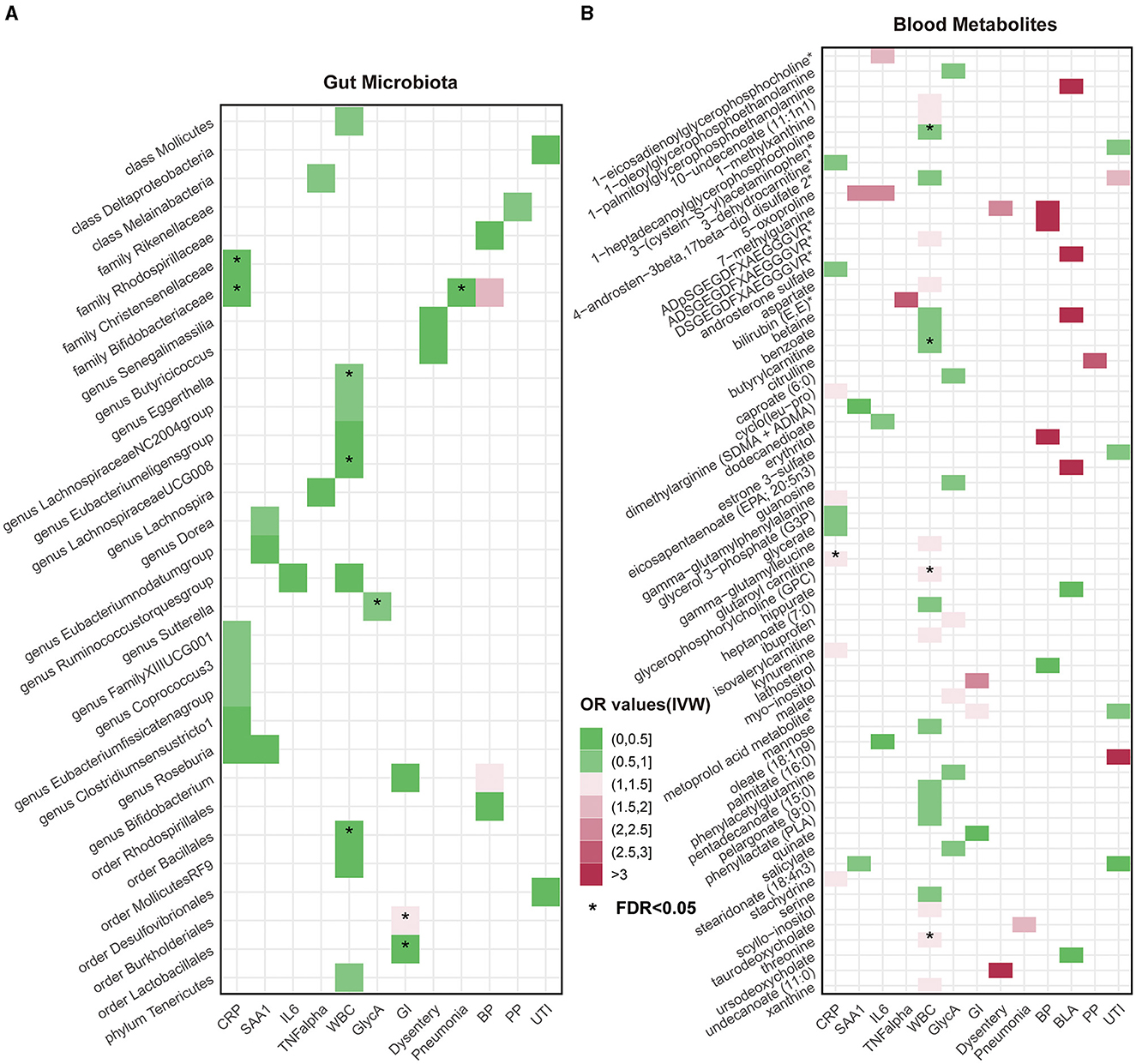
Figure 2. Heatmaps of significant potential causal associations in at least three MR methods including IVW. (A) Heatmap of Odds Ratios (ORs) between gut microbiota and all inflammatory factors and risks of seven infections under IVW analysis. The color intensity represents the magnitude of ORs. An asterisk (*) indicates cases where the false discovery rate (FDR) value for the IVW method is less than 0.05. (B) Heatmap of causal relationship ORs between metabolites and all inflammatory factors and risks of seven infections under the IVW method. CRP, C-reactive protein; SAA1, serum amyloid A1; IL6, interleukin 6; TNF-α, tumor necrosis factor-alpha; WBC, white blood cells; GlycA, glycoprotein acetylation; GI, gastrointestinal infections; BP, bacterial pneumonia; BLA, bronchopneumonia and lung abscess; PP, pneumococcal pneumonia; UTI, urinary tract infections.
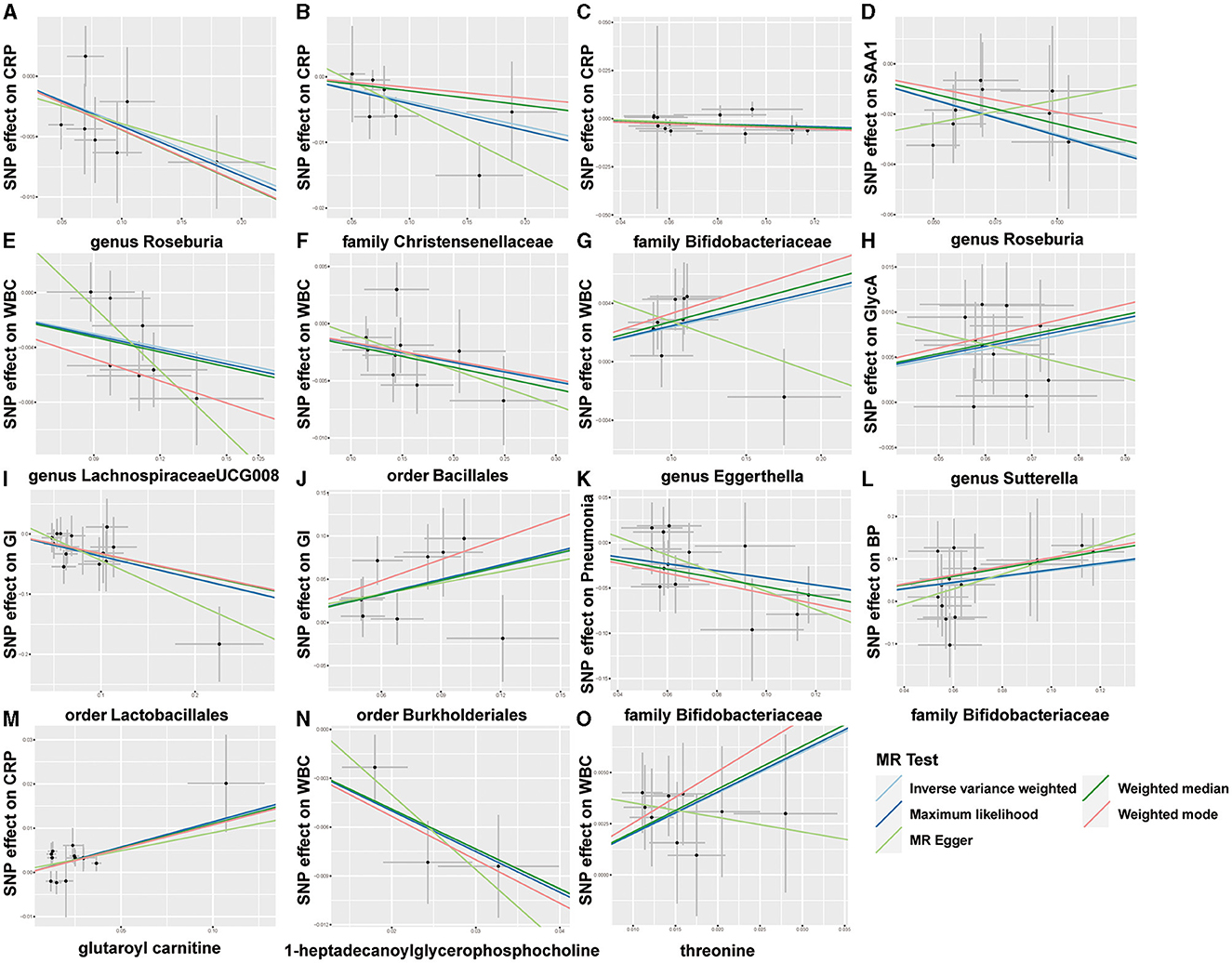
Figure 3. Scatterplots of five MR results. (A–L) Scatterplots of the five MR models for 9 gut microbes on CRP, SAA1, WBC, GlycA, GI, pneumonia, and bacterial pneumonia (BP). (M–O) Scatterplots of the five MR models for three metabolites on CRP and WBC.
Finally, a leave-one-out sensitivity analysis was further employed to confirm the reliability and stability of these results (Supplementary Figures 1, 2). The identified causal associations (Figure 4) include four taxa (genus Roseburia and family XIII UCG001, families Bifidobacteriaceae, and Christensenellaceae) for CRP, genus Roseburia for SAA1, genus Lachnospira for TNF-α, four taxa (genera Eggerthella, Lachnospiraceae, and Ruminococcustorques, order Bacillales) for WBC, and genus Sutterella for GlycA remains displayed no sensitivity with any single IVs, indicating robust causal links from the identified taxa to the corresponding outcomes. In addition, orders Burkholderiales and Lactobacillales for GI, family Bifidobacteriaceae for pneumonia, family Bifidobacteriaceae and genus Bifidobacterium both for BP, and family Rikenellaceae for PP also passed the leave-one-out analysis.
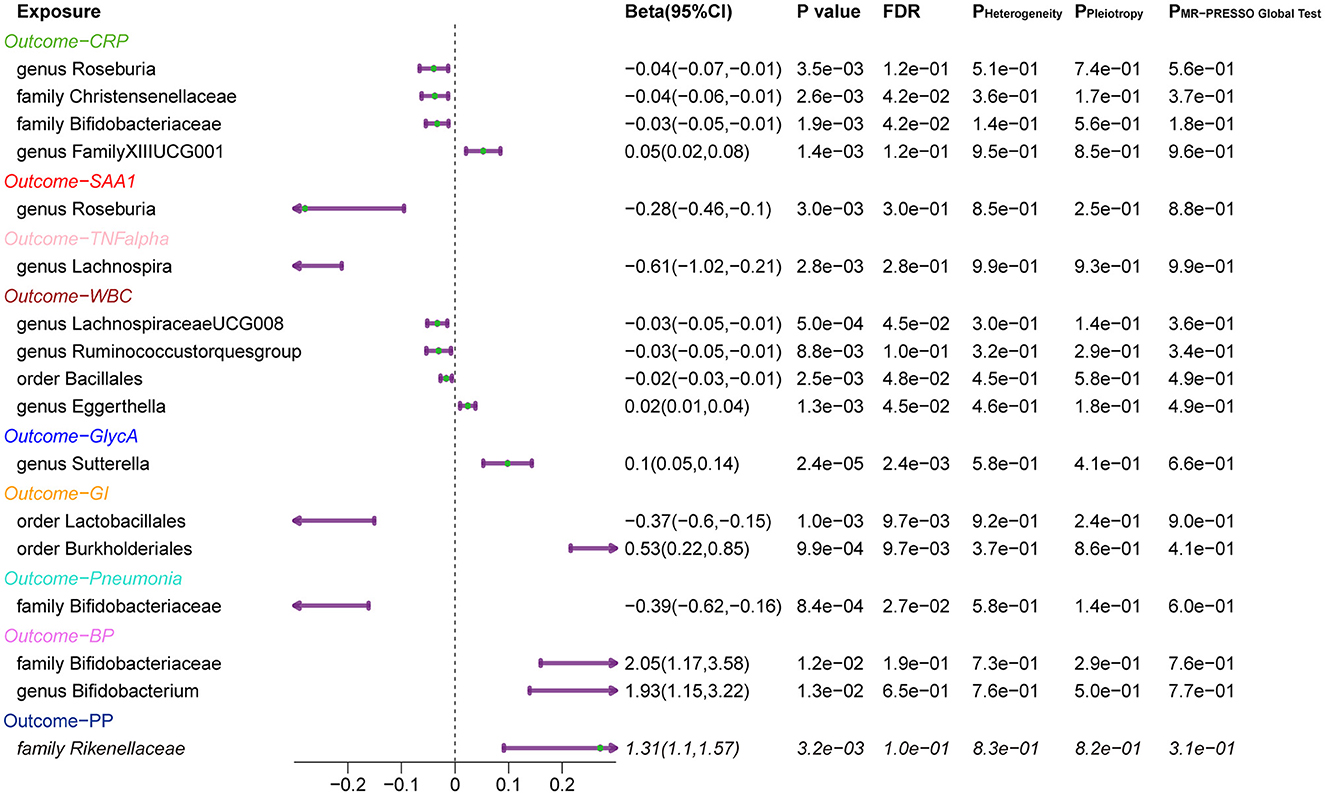
Figure 4. Forest Plots of MR Results for the Identified Causal Relationship between Gut Microbiota and Inflammation and Risks of Infection. 95% CI: 95% confidence interval; P Heterogeneity: P-value of heterogeneity test; P Pleiotropy: P-value of the intercept of MR Egger; P MR-PRESSO Global test: P-value of the MR-PRESSO global test. CRP, C-reactive protein; SAA1, serum amyloid A1; TNF-α, tumor necrosis factor-alpha; WBC, white blood cells; GlycA, glycoprotein acetylation; GI, gastrointestinal infections; BP, bacterial pneumonia; PP, pneumococcal pneumonia.
Two-sample MR analysis of blood metabolites on inflammation and risk of infections
Utilizing the IVW method, we identified 27, 10, 11, 13, 41, and 18 significant annotated metabolites for CRP, SAA1, IL-6, TNF-α, WBC, and GlycA (PIVW < 0.05, Supplementary Table S8). There are 11, 11, 16, 14, 22, 10, and 20 significant annotated metabolites for risk of GI, dysentery, pneumonia, BP, BLA, PP, and UTI. The above associations were further validated by MR-Egger, MR-PRESSO, maximum likelihood, and weighted median methods. The results of all MR methods are shown in Supplementary Table S9. Then, 9, 3, 4, 1, 21, and 7 annotated metabolites displayed significant associations with CRP, SAA1, IL-6, TNF-α, WBC, and GlycA, and 3, 2, 1, 4, 6, 2, and 6 metabolites were significant for the risk of GI, dysentery, pneumonia, BP, BLA, PP, and UTI in at least three MR methods (P < 0.05) and demonstrated no heterogeneity or pleiotropy effects (Supplementary Table S10).
As shown in Figure 2, only five associations remained significant after correction for FDR ([FDR] < 0.05). Glutaroyl carnitine is correlated with elevated CRP levels (Beta [95%CI] IVW = 0.112 [0.057, 0.166]), threonine (Beta [95%CI] IVW = 0.200 [0.093, −0.307]) is correlated with increased WBC, and 1-heptadecanoylglycerophosphocholine (Beta [95%CI] IVW = −0.246 [−0.363, −0.128]) is correlated with decreased WBC. Notably, we observed that two metabolites that were common to two different inflammatory markers or infectious risk, such as 5-oxoproline positively associated with both IL-6 (Beta[95%CI] IVW = 0.697 [0.065, 1.329], PIVW = 0.031) and SAA1 (Beta [95%CI] IVW = 0.912 [0.254, 1.569], PIVW = 0.007), 7-methylguanine positively related to dysentery (Beta [95%CI] IVW = 0.813 [0.012, 1.614], PIVW = 0.047) and BP (Beta [95%CI] IVW= 2.099 [0.051, 4.147], PIVW= 0.045), and stearidonate (18:4n3) negatively related to SAA1 (Beta [95%CI] IVW = −0.468 [−0.930, −0.007], PIVW = 0.047) and UTI (Beta [95%CI] IVW = −1.025 [−1.666, −0.385], PIVW = 0.002). The scatterplots of the SNP effect sizes for the above associations are shown in Figure 3 and Supplementary Figure 3, demonstrating relatively consistent effect direction and magnitude across methods.
Subsequently, the leave-one-out sensitivity analysis confirmed these identified causal associations (Figure 5), including six metabolites [glycerate, glycerol 3-phosphate, glutaroyl carnitine, kynurenine, cyclo (leu-pro), and 3-dehydrocarnitine] to CRP, oleate (18:1n9) to IL-6, nine metabolites [threonine, pentadecanoate (15:0), benzoate, gamma-glutamylleucine, phenyllactate, ADSGEGDFXAEGGGVR, isovalerylcarnitine, 4-androsten-3beta, 17beta-diol disulfate 2, and 1-heptadecanoylglycerophosphocholine] to WBC, and phenylacetylglutamine to GlycA, with no individual SNPs significantly affecting these associations (Supplementary Figures 1, 4). Besides, quinate to GI, undecanoate (11:0) to dysentery, taurodeoxycholate to pneumonia, lathosterol to BP, two metabolites (hippurate and ursodeoxycholate) to BLA, citrulline to PP, 3-(cysteine-S-yl) acetaminophen, stearidonate (18:4n3), and metoprolol acid metabolite to UTI showed no sensitivity to any single IVs.
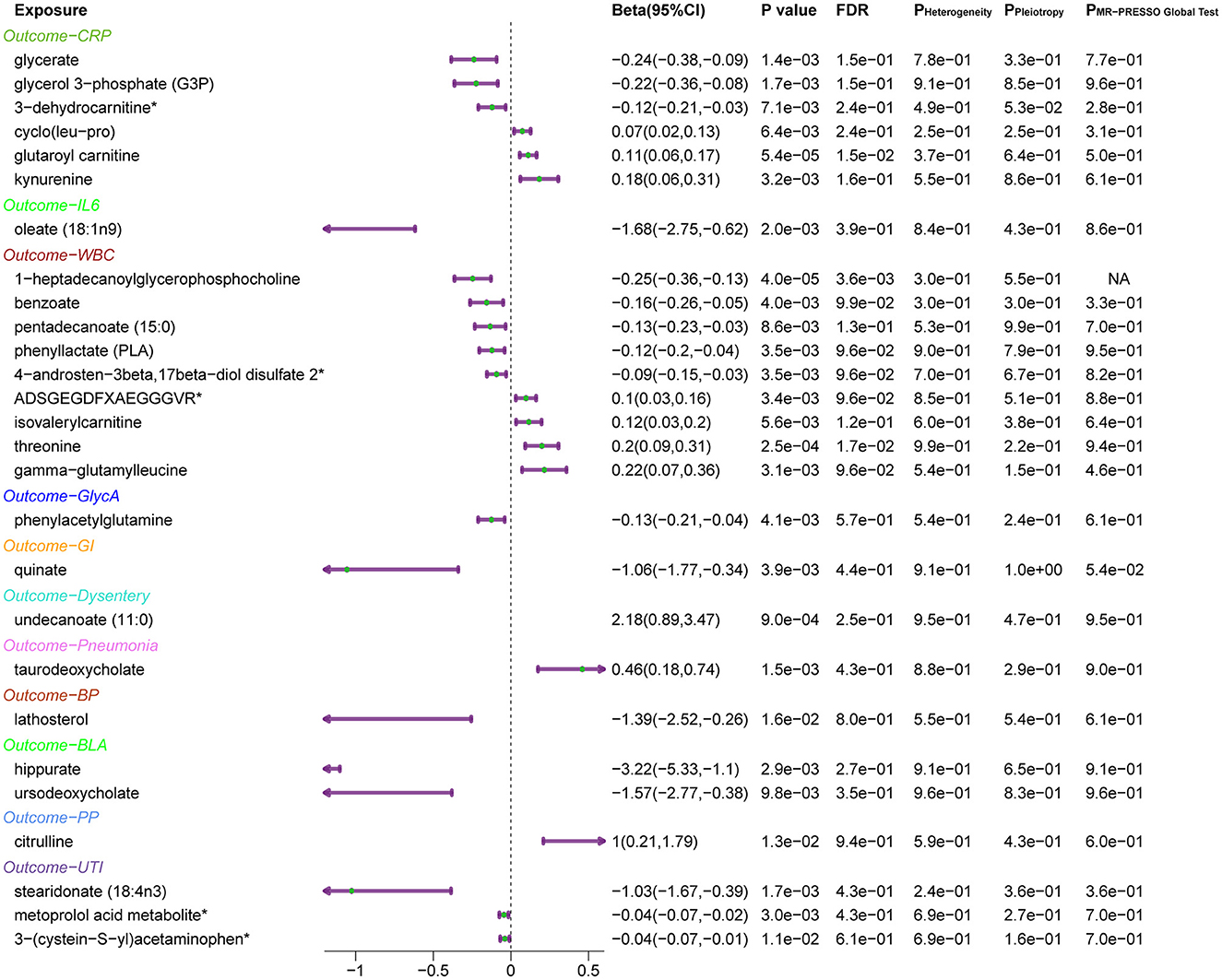
Figure 5. Forest plots of MR results for the identified causal relationship between metabolites and inflammation and risks of infection. The beta value for undecanoate (11:0) exceeds the range of the plot. CRP, C-reactive protein; IL6, interleukin 6; WBC, white blood cells; GlycA, glycoprotein acetylation; GI, gastrointestinal infections; BP, bacterial pneumonia; BLA, bronchopneumonia and lung abscess; PP, pneumococcal pneumonia; UTI, urinary tract infections.
Reverse MR
The results of the reverse MR analysis are shown in Supplementary Tables S11, S12. Causal associations involving fewer than three IVs were not analyzed, using a threshold of P-value < 5e-8 for IV selection. Here, WBC has causal effects on order Bacillales abundance. Except for this, no significant causal estimates were detected by the five MR methods, suggesting a lack of evidence for a causal effect from the changes in inflammatory markers or infectious risks to identified gut bacterial taxa or blood metabolites.
Discussion
Systemic inflammation is often accompanied by metabolic alterations and gut microbiota dysbiosis; hence, a comprehensive study to investigate the causal effects of blood metabolomics and the gut microbiome on inflammation and infection risk is of considerable scientific interest. This MR study suggested a potential role of increasing genus Roseburia and family Bifidobacteriaceae abundance, as well as stearidonate (18:4n3), in mitigating inflammation or infectious risks, which could reduce CRP, SAA1, or GlycA levels, pneumonia, or UTI risk. Conversely, elevating 5-oxoproline levels may enhance inflammatory markers of IL-6 and SAA1 levels. Collectively, these results underscore the importance of elucidating the causal effects between gut microbiota, blood metabolites, inflammatory markers, and infection risk in understanding the biological mechanisms underlying systemic inflammation, which might provide promising targets to ease the monitoring and therapy of inflammation-associated diseases and infectious risks.
The gut microbiota can modulate the host's immune-inflammatory process, thus affecting the development of inflammatory disorders and even infectious risks. This MR study suggested that the genus Roseburia may help mitigate CRP and GlycA-indicated inflammation, and the family Bifidobacteriaceae may help control CRP levels and pneumonia risk. Consistent with this study, previous studies have also noted an inverse correlation between Roseburia and Bifidobacteriaceae abundance and CRP levels (Malaguarnera et al., 2012; Groeger et al., 2013; Xu et al., 2021; Bao et al., 2022), and their absence is commonly observed in diverse diseases (Tamanai-Shacoori et al., 2017). Roseburia, a major butyrate producer, is one of the most abundant species in the gut microbiota of healthy humans (Aminov et al., 2006). Both Roseburia and Bifidobacteria are vital for maintaining gut homeostasis and anti-inflammatory effects by promoting butyrate production (Kasahara et al., 2018; Seo et al., 2020). Notably, Bifidobacteria show butyrogenic effects through cross-feeding interactions with other butyrate-producing bacteria like Roseburia (Riviere et al., 2016). Butyrate has anti-inflammatory properties, can induce Treg cell differentiation, limits pro-inflammatory cytokines by inhibiting the nuclear factor kappa-B (NF-kB) pathway, releases anti-inflammatory molecules, and maintains gut homeostasis by secreting antimicrobial peptides (Tamanai-Shacoori et al., 2017; Singh et al., 2022). Interestingly, Roseburia intestinalis interacts with dietary plant polysaccharides and can further alleviate systemic inflammation and atherosclerotic lesions via butyrate production (Kasahara et al., 2018). Therefore, our results support the prospect of shifting the interest from simply increasing Bifidobacterial concentrations to stimulating or supplementing butyrate-producing bacteria like Roseburia as next-generation candidate probiotics for alleviating inflammation magnitude (Zhang et al., 2022).
Bifidobacteria have been shown to interact with human immune cells, involving innate and adaptive immune processes (Ruiz et al., 2017). Bifidobacteriaceae, the sole family within Bifidobacteriales, encompasses many species with demonstrated multifaceted probiotic effects (Hidalgo-Cantabrana et al., 2017). The typical genus, Bifidobacterium, has been widely used as a probiotic to maintain gut flora balance and address gastrointestinal disorders (Chen et al., 2021). Extensive research has confirmed that some strains of Bifidobacteria could impart anti-inflammatory benefits by inhibiting NF-κB activation and lipopolysaccharide production, reducing IL-1β levels, regulating immune balance and inflammatory response, and countering neutrophil migration (Chen et al., 2021). Decreased Bifidobacteriaceae abundance was also observed in children with mycoplasma pneumoniae (Shi et al., 2022) and respiratory tract infection (Li et al., 2019). Existing studies have revealed that intestinal probiotics enhance the host's resistance to pneumonia, and novel therapeutic strategies could exploit the gut-lung axis in bacterial infections (Schuijt et al., 2016). As known, multiple studies have demonstrated that long-term use of probiotics such as Lactobacillus or Bifidobacterium could significantly reduce the risk of infections, including respiratory and GI (Hojsak et al., 2010; Wolvers et al., 2010; Ozen et al., 2015). Similarly, our results also supported that order Lactobacillales were linked to reduced GI risk.
In addition, several potentially harmful bacteria were found to enhance inflammation or infectious risks. We found that order Burkholderiales were linked to elevated GI risk. Burkholderia are known as mammalian pathogens and consist mainly of pathogenic bacteria (e.g., Bordetella, Ralstonia, and Oxalobacter). It was already identified as an important pathogen for chronic infections (Lewis and Torres, 2016), including GI (Sanchez-Villamil et al., 2022). Recent studies revealed that type 6 secretion system-dependent blockage of TNF-α signaling and BicA as a Burkholderia pseudomallei pathogenesis during GI (Sanchez-Villamil et al., 2022). Besides, the genera Eggerthella and Sutterella were related to increased WBC and GlycA levels after multiple-testing correction, respectively. It was reported that both genera Eggerthella (Chang and Choi, 2023) and Sutterella (Hiippala et al., 2016; Kaakoush, 2020) have pro-inflammatory properties. An increasing number of studies have shown that Eggerthella lenta could be an important pathogen for humans, even causing life-threatening infection under certain conditions (Jiang et al., 2021b), which can drive Th17 activation in immune-related diseases (Alexander et al., 2022). Although Sutterella may have an immunomodulatory role and has been frequently identified as being associated with autism and inflammatory bowel disease (Hiippala et al., 2016), Sutterella is a controversial bacterium; whether Sutterella species represents the cause or consequence of inflammation and infection remains unclear.
Furthermore, metabolic balance is closely related to immune-inflammatory processes. Specifically, glutaroyl carnitine (C5DC) was the only metabolite that remained significant on elevated CRP levels after FDR correction in the IVW analysis. In line with us, C5DC levels were positively correlated with the inflammatory marker IL-1β (Guerreiro et al., 2021). Increased C5DC levels are linked to cardiovascular risks (Zhao et al., 2020) and aging (Carlsson et al., 2021), both of which are commonly accompanied by prolonged chronic inflammation (Shi et al., 2021). Notably, C5DC elevations caused by glutaryl-CoA dehydrogenase deficiency are generally seen in the inborn glutaric aciduria type I disorder, leading to neurological dysfunction and high inflammatory states (Zhao et al., 2014). In addition, after FDR correction, 1-heptadecanoyglycerophosphocholine decreased WBC levels, and threonine increased WBC levels, still statistically significant. The results in aged mice showed that ingestion of α-glycerophosphocholine (GPC) decreased the expression levels of aging-related long-term enhancement genes associated with long-term enhancement of gene expression levels (Narukawa et al., 2020). There is growing evidence that there is a strong association between leukocyte levels of telomeres and aging (Aviv, 2004). Threonine is used by lymphocytes to increase antibody secretion to maintain immune function (Tang et al., 2021). The other three metabolites are also worth mentioning, which showed similar causal effects on two inflammatory makers or infectious phenotypes. 5-oxoproline is a well-known mediator inducing inflammation and oxidative stress (Pederzolli et al., 2010; Van Der Pol et al., 2018), which could be biomarkers for early diagnosis of sepsis, reflecting an imbalance in glutamine and glutathione metabolism (Lu et al., 2022). 7-methylguanine increases the risk of both dysentery and hypertension. Stearidonate (18:4n3) is a specific omega-3 polyunsaturated fatty acid (PUFA) that is abundant in seafood and is related to reduced SAA1 levels and the risk of urinary tract infection. In the past few decades, many epidemiological studies have been reported on the myriad health benefits of omega-3 PUFAs (Shahidi and Ambigaipalan, 2018), including preventing and resolving inflammation disorders (Yates et al., 2014). Collectively, these findings indicate the potential for interventions modulating the levels of these metabolites to impact the body's immune inflammatory response, further highlighting the therapeutic potential of metabolic modulation.
This study used the largest publicly available GWAS statistics on the gut microbiome and blood metabolome to conduct MR analysis, which can minimize the effects of potential confounders and enhance the causal inference in the associations (Davies et al., 2018). Strict quality control procedures and sensitivity analysis approaches were used to ensure the robustness of the MR estimates. Hence, the associations identified in this study could help guide further exploration into the mechanisms linking the gut microbiome, metabolic signature, and inflammatory and infectious phenotypes. Importantly, our results provide new insights into the potential of supplementing probiotics like Roseburia and Bifidobacteriaceae and metabolic reprogramming strategies for alleviating inflammation and infectious risks. Furthermore, we could extend the potential benefits of gut microbiota to systemic inflammatory diseases beyond the gut. Although current research is still limited, this study could help us improve our knowledge on the molecular mechanism behind systemic inflammation, suggesting potential therapeutic targets. Nonetheless, this study has certain limitations. First, though MR methods enable causal inference from exposure to the outcome, the magnitude of the effects is hard to estimate accurately. Further large-scale clinical trials and mechanistic research are required. Second, this study focused on populations of European descent without extrapolating the results to other ethnic groups. Thirdly, data on bacterial taxa at the species level was unavailable; further study is needed to illustrate the causal links between the specific species or strains and inflammatory phenotypes. Additionally, it is important to note that the GWAS data on gut microbiota did not take into account the potential impact of medication treatments, such as antibiotics, on an individual's gut microbiota balance. This highlights the necessity for further experimental validation. Furthermore, our study does not account for the duration of inflammation or the timing of blood sample collection, which could significantly affect biomarker levels.
Conclusion
This study provides novel evidence for a causal association between metabolites, gut microbiota, inflammation, and the risk of infection. Our MR study suggests a potentially protective effect of the genus Roseburia, family Bifidobacteriaceae, and stearidonate (18:4n3) against inflammation or infectious risks. In contrast, 5-oxoproline showed a possible pro-inflammatory role. These findings shed light on new insights into metabolic and microbially mediated alterations in inflammatory extent, suggesting their potential implications in the prevention and treatment of inflammation and infections.
Data availability statement
Publicly available datasets were analyzed in this study. The methodology section of this article details the data sources.
Author contributions
YL: Writing—review & editing, Writing—original draft, Visualization, Software, Methodology, Data curation, Conceptualization. QZ: Conceptualization, Software, Writing—original draft, Writing—review & editing. GG: Writing—review & editing, Software, Data curation. ZX: Formal analysis, Investigation, Writing—review & editing. SL: Writing—review & editing, Software, Data curation, Conceptualization. CL: Writing—review & editing, Software, Data curation. YW: Methodology, Software, Writing—review & editing. LW: Software, Writing—review & editing, Methodology. SZ: Writing—review & editing.
Funding
The author(s) declare financial support was received for the research, authorship, and/or publication of this article. This research was funded by the National Natural Science Foundation of China (grant number: 82274016 and 81872934) and the Science and Technology Program of Guangzhou (grant number: 2023B03J1251). The funders were not involved in designing the study; collecting, analyzing, or interpreting the data; or in writing or submitting the manuscript for publication.
Acknowledgments
We would like to thank all the authors who shared these GWAS data.
Conflict of interest
The authors declare that the research was conducted in the absence of any commercial or financial relationships that could be construed as a potential conflict of interest.
Publisher's note
All claims expressed in this article are solely those of the authors and do not necessarily represent those of their affiliated organizations, or those of the publisher, the editors and the reviewers. Any product that may be evaluated in this article, or claim that may be made by its manufacturer, is not guaranteed or endorsed by the publisher.
Supplementary material
The Supplementary Material for this article can be found online at: https://www.frontiersin.org/articles/10.3389/fmicb.2024.1342653/full#supplementary-material
References
Ahola-Olli, A. V., Würtz, P., Havulinna, A. S., Aalto, K., Pitkänen, N., Lehtimäki, T., et al. (2017). Genome-wide association study identifies 27 loci influencing concentrations of circulating cytokines and growth factors. Am. J. Hum. Genet. 100, 40–50. doi: 10.1016/j.ajhg.2016.11.007
Al Bander, Z., Nitert, M. D., Mousa, A., and Naderpoor, N. (2020). The gut microbiota and inflammation: an overview. Int J. Environ. Res. Public Health 17:7618. doi: 10.3390/ijerph17207618
Alexander, M., Ang, Q. Y., Nayak, R. R., Bustion, A. E., Sandy, M., Zhang, B., et al. (2022). Human gut bacterial metabolism drives Th17 activation and colitis. Cell Host Microbe 30, 17–30.e19. doi: 10.1016/j.chom.2021.11.001
Aminov, R. I., Walker, A. W., Duncan, S. H., Harmsen, H. J., Welling, G. W., and Flint, H. J. (2006). Molecular diversity, cultivation, and improved detection by fluorescent in situ hybridization of a dominant group of human gut bacteria related to Roseburia spp. or Eubacterium rectale. Appl. Environ. Microbiol. 72, 6371–6376. doi: 10.1128/AEM.00701-06
Araújo, R., Bento, L. F. N., Fonseca, T. a.H , Von Rekowski, C. P., Da Cunha, B. R., et al. (2022). Infection biomarkers based on metabolomics. Metabolites 12:92. doi: 10.3390/metabo12020092
Aviv, A. (2004). Telomeres and human aging: facts and fibs. Sci. Aging Knowl. Environ. 2004, pe43. doi: 10.1126/sageke.2004.51.pe43
Bao, C., Wu, L., Wang, D., Chen, L., Jin, X., Shi, Y., et al. (2022). Acupuncture improves the symptoms, intestinal microbiota, and inflammation of patients with mild to moderate Crohn's disease: a randomized controlled trial. EClinicalMedicine 45:101300. doi: 10.1016/j.eclinm.2022.101300
Bowden, J., Davey Smith, G., and Burgess, S. (2015). Mendelian randomization with invalid instruments: effect estimation and bias detection through Egger regression. Int. J. Epidemiol. 44, 512–525. doi: 10.1093/ije/dyv080
Brynskov, J., Foegh, P., Pedersen, G., Ellervik, C., Kirkegaard, T., Bingham, A., et al. (2002). Tumour necrosis factor alpha converting enzyme (TACE) activity in the colonic mucosa of patients with inflammatory bowel disease. Gut 51, 37–43. doi: 10.1136/gut.51.1.37
Burgess, S., Butterworth, A., and Thompson, S. G. (2013). Mendelian randomization analysis with multiple genetic variants using summarized data. Genet. Epidemiol. 37, 658–665. doi: 10.1002/gepi.21758
Bycroft, C., Freeman, C., Petkova, D., Band, G., Elliott, L. T., Sharp, K., et al. (2018). The UK Biobank resource with deep phenotyping and genomic data. Nature 562, 203–209. doi: 10.1038/s41586-018-0579-z
Calder, P. C., Ortega, E. F., Meydani, S. N., Adkins, Y., Stephensen, C. B., Thompson, B., et al. (2022). Nutrition, immunosenescence, and infectious disease: an overview of the scientific evidence on micronutrients and on modulation of the gut microbiota. Adv. Nutr. 13, S1–S26. doi: 10.1093/advances/nmac052
Carlsson, H., Rollborn, N., Herman, S., Freyhult, E., Svenningsson, A., Burman, J., et al. (2021). Metabolomics of cerebrospinal fluid from healthy subjects reveal metabolites associated with ageing. Metabolites 11:126. doi: 10.3390/metabo11020126
Chang, S. H., and Choi, Y. (2023). Gut dysbiosis in autoimmune diseases: association with mortality. Front. Cell Infect. Microbiol. 13:1157918. doi: 10.3389/fcimb.2023.1157918
Chen, J., Chen, X., and Ho, C. L. (2021). Recent development of probiotic bifidobacteria for treating human diseases. Front. Bioeng. Biotechnol. 9:770248. doi: 10.3389/fbioe.2021.770248
Chen, M. H., Raffield, L. M., Mousas, A., Sakaue, S., Huffman, J. E., Moscati, A., et al. (2020). Trans-ethnic and ancestry-specific blood-cell genetics in 746,667 individuals from 5 global populations. Cell 182, 1198–1213.e1114. doi: 10.1016/j.cell.2020.06.045
Collaborators, G. B. D. C. O. D. (2017). Global, regional, and national age-sex specific mortality for 264 causes of death, 1980-2016: a systematic analysis for the Global Burden of Disease Study 2016. Lancet 390, 1151–1210. doi: 10.1016/S0140-6736(17)32152-9
Connelly, M. A., Otvos, J. D., Shalaurova, I., Playford, M. P., and Mehta, N. N. (2017). GlycA, a novel biomarker of systemic inflammation and cardiovascular disease risk. J. Transl. Med. 15:219. doi: 10.1186/s12967-017-1321-6
Davies, N. M., Holmes, M. V., and Davey Smith, G. (2018). Reading Mendelian randomisation studies: a guide, glossary, and checklist for clinicians. BMJ 362:k601. doi: 10.1136/bmj.k601
Denny, J. C., Bastarache, L., Ritchie, M. D., Carroll, R. J., Zink, R., Mosley, J. D., et al. (2013). Systematic comparison of phenome-wide association study of electronic medical record data and genome-wide association study data. Nat. Biotechnol. 31, 1102–1110. doi: 10.1038/nbt.2749
Dos Anjos, B. L., and Grotto, H. Z. (2010). Evaluation of C-reactive protein and serum amyloid A in the detection of inflammatory and infectious diseases in children. Clin. Chem. Lab. Med. 48, 493–499. doi: 10.1515/CCLM.2010.110
Folkersen, L., Gustafsson, S., Wang, Q., Hansen, D. H., Hedman, Å. K., et al. (2020). Genomic and drug target evaluation of 90 cardiovascular proteins in 30,931 individuals. Nat. Metab. 2, 1135–1148. doi: 10.1038/s42255-020-00287-2
Furman, D., Campisi, J., Verdin, E., Carrera-Bastos, P., Targ, S., Franceschi, C., et al. (2019). Chronic inflammation in the etiology of disease across the life span. Nat. Med. 25, 1822–1832. doi: 10.1038/s41591-019-0675-0
Greten, F. R., and Grivennikov, S. I. (2019). Inflammation and cancer: triggers, mechanisms, and consequences. Immunity 51, 27–41. doi: 10.1016/j.immuni.2019.06.025
Groeger, D., O'mahony, L., Murphy, E. F., Bourke, J. F., Dinan, T. G., Kiely, B., et al. (2013). Bifidobacterium infantis 35624 modulates host inflammatory processes beyond the gut. Gut Micr. 4, 325–339. doi: 10.4161/gmic.25487
Guerreiro, G., Faverzani, J., Moura, A. P., Volfart, V., Gome Dos Reis, B., Sitta, A., et al. (2021). Protective effects of L-carnitine on behavioral alterations and neuroinflammation in striatum of glutaryl-COA dehydrogenase deficient mice. Arch. Biochem. Biophys. 709:108970. doi: 10.1016/j.abb.2021.108970
Guo, G., Wu, Y., Liu, Y., Wang, Z., Xu, G., Wang, X., et al. (2023). Exploring the causal effects of the gut microbiome on serum lipid levels: a two-sample Mendelian randomization analysis. Front. Microbiol. 14:1113334. doi: 10.3389/fmicb.2023.1113334
Han, X., Ong, J. S., An, J., Hewitt, A. W., Gharahkhani, P., and Macgregor, S. (2020). Using Mendelian randomization to evaluate the causal relationship between serum C-reactive protein levels and age-related macular degeneration. Eur. J. Epidemiol. 35, 139–146. doi: 10.1007/s10654-019-00598-z
Hartwig, F. P., Davey Smith, G., and Bowden, J. (2017). Robust inference in summary data Mendelian randomization via the zero modal pleiotropy assumption. Int. J. Epidemiol. 46, 1985–1998. doi: 10.1093/ije/dyx102
Hasegawa, T., Negishi, T., and Deguchi, M. (2002). WBC count, atherosclerosis and coronary risk factors. J. Atheroscler Thromb. 9, 219–223. doi: 10.5551/jat.9.219
Hemani, G., Tilling, K., and Davey Smith, G. (2017). Orienting the causal relationship between imprecisely measured traits using GWAS summary data. PLoS Genet. 13:e1007081. doi: 10.1371/journal.pgen.1007081
Hemani, G., Zheng, J., Elsworth, B., Wade, K. H., Haberland, V., Baird, D., et al. (2018). The MR-Base platform supports systematic causal inference across the human phenome. Elife 7:e34408. doi: 10.7554/eLife.34408
Hidalgo-Cantabrana, C., Delgado, S., Ruiz, L., Ruas-Madiedo, P., Sanchez, B., and Margolles, A. (2017). Bifidobacteria and their health-promoting effects. Microbiol. Spectr. 5:73–98. doi: 10.1128/9781555819705.ch3
Hiippala, K., Kainulainen, V., Kalliomäki, M., Arkkila, P., and Satokari, R. (2016). Mucosal prevalence and interactions with the epithelium indicate commensalism of Sutterella spp. Front. Microbiol. 7:1706. doi: 10.3389/fmicb.2016.01706
Hojsak, I., Snovak, N., Abdovi,ć, S., Szajewska, H., Misak, Z., and Kolacek, S. (2010). Lactobacillus GG in the prevention of gastrointestinal and respiratory tract infections in children who attend day care centers: a randomized, double-blind, placebo-controlled trial. Clin. Nutr. 29, 312–316. doi: 10.1016/j.clnu.2009.09.008
Hwang, L. D., Lawlor, D. A., Freathy, R. M., Evans, D. M., and Warrington, N. M. (2019). Using a two-sample Mendelian randomization design to investigate a possible causal effect of maternal lipid concentrations on offspring birth weight. Int. J. Epidemiol. 48, 1457–1467. doi: 10.1093/ije/dyz160
Jiang, L., Zheng, Z., Fang, H., and Yang, J. (2021a). A generalized linear mixed model association tool for biobank-scale data. Nat. Genet. 53, 1616–1621. doi: 10.1038/s41588-021-00954-4
Jiang, S., E, J., Wang, D., Zou, Y., Liu, X., Xiao, H., et al. (2021b). Eggerthella lenta bacteremia successfully treated with ceftizoxime: case report and review of the literature. Eur. J. Med. Res. 26:111. doi: 10.1186/s40001-021-00582-y
Jutley, G. S., Sahota, K., Sahbudin, I., Filer, A., Arayssi, T., Young, S. P., et al. (2021). Relationship between inflammation and metabolism in patients with newly presenting rheumatoid arthritis. Front. Immunol. 12:676105. doi: 10.3389/fimmu.2021.676105
Kaakoush, N. O. (2020). Sutterella species, IgA-degrading bacteria in ulcerative colitis. Trends Microbiol. 28, 519–522. doi: 10.1016/j.tim.2020.02.018
Kaddurah-Daouk, R., and Krishnan, K. R. (2009). Metabolomics: a global biochemical approach to the study of central nervous system diseases. Neuropsychopharmacology 34, 173–186. doi: 10.1038/npp.2008.174
Kamat, M. A., Blackshaw, J. A., Young, R., Surendran, P., Burgess, S., Danesh, J., et al. (2019). PhenoScanner V2: an expanded tool for searching human genotype-phenotype associations. Bioinformatics 35, 4851–4853. doi: 10.1093/bioinformatics/btz469
Kasahara, K., Krautkramer, K. A., Org, E., Romano, K. A., Kerby, R. L., Vivas, E. I., et al. (2018). Interactions between Roseburia intestinalis and diet modulate atherogenesis in a murine model. Nat. Microbiol. 3, 1461–1471. doi: 10.1038/s41564-018-0272-x
Kurilshikov, A., Medina-Gomez, C., Bacigalupe, R., Radjabzadeh, D., Wang, J., Demirkan, A., et al. (2021). Large-scale association analyses identify host factors influencing human gut microbiome composition. Nat. Genet. 53, 156–165. doi: 10.1038/s41588-020-00763-1
Lamps, L. W. (2009). “Infectious disorders of the GI tract,” in Surgical Pathology of the GI Tract, Liver, Biliary Tract, and Pancreas 51–79. doi: 10.1016/B978-141604059-0.50007-2
Lewis, E. R., and Torres, A. G. (2016). The art of persistence-the secrets to Burkholderia chronic infections. Pathog. Dis. 74:ftw070. doi: 10.1093/femspd/ftw070
Li, K. L., Wang, B. Z., Li, Z. P., Li, Y. L., and Liang, J. J. (2019). Alterations of intestinal flora and the effects of probiotics in children with recurrent respiratory tract infection. World J. Pediatr. 15, 255–261. doi: 10.1007/s12519-019-00248-0
Liu, C. H., Abrams, N. D., Carrick, D. M., Chander, P., Dwyer, J., Hamlet, M. R. J., et al. (2017). Biomarkers of chronic inflammation in disease development and prevention: challenges and opportunities. Nat. Immunol. 18, 1175–1180. doi: 10.1038/ni.3828
Liu, K., Zou, J., Fan, H., Hu, H., and You, Z. (2022). Causal effects of gut microbiota on diabetic retinopathy: a Mendelian randomization study. Front. Immunol. 13:930318. doi: 10.3389/fimmu.2022.930318
Locksley, R. M., Killeen, N., and Lenardo, M. J. (2001). The TNF and TNF receptor superfamilies: integrating mammalian biology. Cell 104, 487–501. doi: 10.1016/S0092-8674(01)00237-9
Lu, G., Zhou, J., Yang, T., Li, J., Jiang, X., Zhang, W., et al. (2022). Landscape of metabolic fingerprinting for diagnosis and risk stratification of sepsis. Front. Immunol. 13:883628. doi: 10.3389/fimmu.2022.883628
Luan, Y. Y., and Yao, Y. M. (2018). The clinical significance and potential role of c-reactive protein in chronic inflammatory and neurodegenerative diseases. Front. Immunol. 9:1302. doi: 10.3389/fimmu.2018.01302
Malaguarnera, M., Vacante, M., Antic, T., Giordano, M., Chisari, G., Acquaviva, R., et al. (2012). Bifidobacterium longum with fructo-oligosaccharides in patients with non alcoholic steatohepatitis. Dig. Dis. Sci. 57, 545–553. doi: 10.1007/s10620-011-1887-4
Medina, M., and Castillo-Pino, E. (2019). An introduction to the epidemiology and burden of urinary tract infections. Ther. Adv. Urol. 11:1756287219832172. doi: 10.1177/1756287219832172
Menzel, A., Samouda, H., Dohet, F., Loap, S., Ellulu, M. S., and Bohn, T. (2021). Common and novel markers for measuring inflammation and oxidative stress ex vivo in research and clinical practice-which to use regarding disease outcomes? Antioxidants (Basel) 10:414. doi: 10.3390/antiox10030414
Narukawa, M., Kamiyoshihara, A., Izu, H., Fujii, T., Matsubara, K., and Misaka, T. (2020). Efficacy of long-term feeding of alpha-glycerophosphocholine for aging-related phenomena in old mice. Gerontology 66, 275–285. doi: 10.1159/000504962
Ozen, M., Kocabas Sandal, G., and Dinleyici, E. C. (2015). Probiotics for the prevention of pediatric upper respiratory tract infections: a systematic review. Expert. Opin. Biol. Ther. 15, 9–20. doi: 10.1517/14712598.2015.980233
Pederzolli, C. D., Mescka, C. P., Zandon,á, B. R., De Moura Coelho, D., Sgaravatti, A. M., Sgarbi, M. B., et al. (2010). Acute administration of 5-oxoproline induces oxidative damage to lipids and proteins and impairs antioxidant defenses in cerebral cortex and cerebellum of young rats. Metab. Brain Dis. 25, 145–154. doi: 10.1007/s11011-010-9190-1
Pierce, B. L., Ahsan, H., and Vanderweele, T. J. (2011). Power and instrument strength requirements for Mendelian randomization studies using multiple genetic variants. Int. J. Epidemiol. 40, 740–752. doi: 10.1093/ije/dyq151
Pierce, B. L., and Burgess, S. (2013). Efficient design for Mendelian randomization studies: subsample and 2-sample instrumental variable estimators. Am. J. Epidemiol. 178, 1177–1184. doi: 10.1093/aje/kwt084
Pietzner, M., Kaul, A., Henning, A. K., Kastenmuller, G., Artati, A., Lerch, M. M., et al. (2017). Comprehensive metabolic profiling of chronic low-grade inflammation among generally healthy individuals. BMC Med. 15:210. doi: 10.1186/s12916-017-0974-6
Pietzner, M., Wheeler, E., Carrasco-Zanini, J., Raffler, J., Kerrison, N. D., Oerton, E., et al. (2020). Genetic architecture of host proteins involved in SARS-CoV-2 infection. Nat. Commun. 11:6397. doi: 10.1038/s41467-020-19996-z
Ponti, G., Maccaferri, M., Ruini, C., Tomasi, A., and Ozben, T. (2020). Biomarkers associated with COVID-19 disease progression. Crit. Rev. Clin. Lab. Sci. 57, 389–399. doi: 10.1080/10408363.2020.1770685
Qu, J., L, X., Liu, Y., and Wang, X. (2015). Evaluation of procalcitonin, C-reactive protein, interleukin-6 and serum amyloid A as diagnostic biomarkers of bacterial infection in febrile patients. Indian J. Med. Res. 141, 315–321. doi: 10.4103/0971-5916.156617
Riviere, A., Selak, M., Lantin, D., Leroy, F., and De Vuyst, L. (2016). Bifidobacteria and butyrate-producing colon bacteria: importance and strategies for their stimulation in the human gut. Front. Microbiol. 7:979. doi: 10.3389/fmicb.2016.00979
Ruiz, L., Delgado, S., Ruas-Madiedo, P., Sánchez, B., and Margolles, A. (2017). Bifidobacteria and their molecular communication with the immune system. Front. Microbiol. 8:2345. doi: 10.3389/fmicb.2017.02345
Sakaue, S., Kanai, M., Tanigawa, Y., Karjalainen, J., Kurki, M., Koshiba, S., et al. (2021). A cross-population atlas of genetic associations for 220 human phenotypes. Nat. Genet. 53, 1415–1424. doi: 10.1038/s41588-021-00931-x
Samuelson, D. R., Welsh, D. A., and Shellito, J. E. (2015). Regulation of lung immunity and host defense by the intestinal microbiota. Front. Microbiol. 6:1085. doi: 10.3389/fmicb.2015.01085
Sanchez-Villamil, J. I., Tapia, D., Khakhum, N., Widen, S. G., and Torres, A. G. (2022). Dual RNA-seq reveals a type 6 secretion system-dependent blockage of TNF-α signaling and BicA as a Burkholderia pseudomallei virulence factor important during gastrointestinal infection. Gut Microbes 14:2111950. doi: 10.1080/19490976.2022.2111950
Sanna, S., Van Zuydam, N. R., Mahajan, A., Kurilshikov, A., Vich Vila, A., Vosa, U., et al. (2019). Causal relationships among the gut microbiome, short-chain fatty acids and metabolic diseases. Nat. Genet. 51, 600–605. doi: 10.1038/s41588-019-0350-x
Schuijt, T. J., Lankelma, J. M., Scicluna, B. P., De Sousa E Melo, F., Roelofs, J. J., De Boer, J. D., et al. (2016). The gut microbiota plays a protective role in the host defence against pneumococcal pneumonia. Gut 65, 575–583. doi: 10.1136/gutjnl-2015-309728
Seo, B., Jeon, K., Moon, S., Lee, K., Kim, W. K., Jeong, H., et al. (2020). Roseburia spp. abundance associates with alcohol consumption in humans and its administration ameliorates alcoholic fatty liver in mice. Cell Host Microbe 27, 25–40.e26. doi: 10.1016/j.chom.2019.11.001
Shahidi, F., and Ambigaipalan, P. (2018). Omega-3 polyunsaturated fatty acids and their health benefits. Annu. Rev. Food Sci. Technol. 9, 345–381. doi: 10.1146/annurev-food-111317-095850
Shi, D., Tan, Q., Ruan, J., Tian, Z., Wang, X., Liu, J., et al. (2021). Aging-related markers in rat urine revealed by dynamic metabolic profiling using machine learning. Aging (Albany NY) 13, 14322–14341. doi: 10.18632/aging.203046
Shi, D. W., Wang, D. M., Ning, L. H., Li, J., Dong, Y., Zhang, Z. K., et al. (2022). Using 16S rDNA sequencing technology to preliminarily analyze intestinal flora in children with mycoplasma pneumoniae pneumonia. Biomed. Environ. Sci. 35, 528–537. doi: 10.3967/bes2022.070
Shin, S. Y., Fauman, E. B., Petersen, A. K., Krumsiek, J., Santos, R., Huang, J., et al. (2014). An atlas of genetic influences on human blood metabolites. Nat. Genet. 46, 543–550. doi: 10.1038/ng.2982
Singh, V., Lee, G., Son, H., Koh, H., Kim, E. S., Unno, T., et al. (2022). Butyrate producers, “The Sentinel of Gut”: Their intestinal significance with and beyond butyrate, and prospective use as microbial therapeutics. Front. Microbiol. 13:1103836. doi: 10.3389/fmicb.2022.1103836
Slaats, J., Ten Oever, J., Van De Veerdonk, F. L., and Netea, M. G. (2016). IL-1beta/IL-6/CRP and IL-18/ferritin: distinct inflammatory programs in infections. PLoS Pathog. 12:e1005973. doi: 10.1371/journal.ppat.1005973
Swardfager, W., Lanctôt, K., Rothenburg, L., Wong, A., Cappell, J., and Herrmann, N. (2010). A meta-analysis of cytokines in Alzheimer's disease. Biol. Psychiatry 68, 930–941. doi: 10.1016/j.biopsych.2010.06.012
Tamanai-Shacoori, Z., Smida, I., Bousarghin, L., Loreal, O., Meuric, V., Fong, S. B., et al. (2017). Roseburia spp.: a marker of health? Future Microbiol. 12, 157–170. doi: 10.2217/fmb-2016-0130
Tanaka, T., Narazaki, M., and Kishimoto, T. (2014). IL-6 in inflammation, immunity, and disease. Cold Spring Harb. Perspect Biol. 6:a016295. doi: 10.1101/cshperspect.a016295
Tang, Q., Tan, P., Ma, N., and Ma, X. (2021). Physiological functions of threonine in animals: beyond nutrition metabolism. Nutrients 13:2592. doi: 10.3390/nu13082592
Tilg, H., Zmora, N., Adolph, T. E., and Elinav, E. (2020). The intestinal microbiota fuelling metabolic inflammation. Nat. Rev. Immunol. 20, 40–54. doi: 10.1038/s41577-019-0198-4
Van Der Pol, A., Gil, A., Tromp, J., Sillj,é, H. H. W., Van Veldhuisen, D. J., Voors, A. A., et al. (2018). OPLAH ablation leads to accumulation of 5-oxoproline, oxidative stress, fibrosis, and elevated fillings pressures: a murine model for heart failure with a preserved ejection fraction. Cardiovasc. Res. 114, 1871–1882. doi: 10.1093/cvr/cvy187
Verbanck, M., Chen, C. Y., Neale, B., and Do, R. (2018). Detection of widespread horizontal pleiotropy in causal relationships inferred from Mendelian randomization between complex traits and diseases. Nat. Genet. 50, 693–698. doi: 10.1038/s41588-018-0099-7
Wang, H., and Ma, S. (2008). The cytokine storm and factors determining the sequence and severity of organ dysfunction in multiple organ dysfunction syndrome. Am. J. Emerg. Med. 26, 711–715. doi: 10.1016/j.ajem.2007.10.031
Wolvers, D., Antoine, J. M., Myllyluoma, E., Schrezenmeir, J., Szajewska, H., and Rijkers, G. T. (2010). Guidance for substantiating the evidence for beneficial effects of probiotics: prevention and management of infections by probiotics. J. Nutr. 14, 698S−712S. doi: 10.3945/jn.109.113753
Xu, Y., Wang, Y., Li, H., Dai, Y., Chen, D., Wang, M., et al. (2021). Altered fecal microbiota composition in older adults with frailty. Front. Cell Infect. Microbiol. 11:696186. doi: 10.3389/fcimb.2021.696186
Yates, C. M., Calder, P. C., and Ed Rainger, G. (2014). Pharmacology and therapeutics of omega-3 polyunsaturated fatty acids in chronic inflammatory disease. Pharmacol. Ther. 141, 272–282. doi: 10.1016/j.pharmthera.2013.10.010
Yin, H., and Mo, S. (2022). Value of combined detection of serum amyloid A, C-reactive protein and procalcitonin in differential diagnosis of respiratory tract infection in children of China. Ann. Med. 54, 1732–1737. doi: 10.1080/07853890.2022.2064542
Zacho, J., Benfield, T., Tybjærg-Hansen, A., and Nordestgaard, B. G. (2016). Increased baseline c-reactive protein concentrations are associated with increased risk of infections: results from 2 large danish population cohorts. Clin. Chem. 62, 335–342. doi: 10.1373/clinchem.2015.249680
Zhang, H., Duan, Y., Cai, F., Cao, D., Wang, L., Qiao, Z., et al. (2022). Next-generation probiotics: microflora intervention to human diseases. Biomed. Res. Int. 2022:5633403. doi: 10.1155/2022/5633403
Zhao, D., Han, L., He, Z., Zhang, J., and Zhang, Y. (2014). Identification of the plasma metabolomics as early diagnostic markers between biliary atresia and neonatal hepatitis syndrome. PLoS ONE 9:e85694. doi: 10.1371/journal.pone.0085694
Keywords: gut microbiome, infection, inflammation, Mendelian randomization analysis, metabolome
Citation: Liu Y, Zhu Q, Guo G, Xie Z, Li S, Lai C, Wu Y, Wang L and Zhong S (2024) Causal associations of genetically predicted gut microbiota and blood metabolites with inflammatory states and risk of infections: a Mendelian randomization analysis. Front. Microbiol. 15:1342653. doi: 10.3389/fmicb.2024.1342653
Received: 23 November 2023; Accepted: 28 February 2024;
Published: 22 March 2024.
Edited by:
Chen Li, Northeastern University, ChinaReviewed by:
Marcos Edgar Herkenhoff, University of São Paulo, BrazilGeorgia Damoraki, National and Kapodistrian University of Athens, Greece
Copyright © 2024 Liu, Zhu, Guo, Xie, Li, Lai, Wu, Wang and Zhong. This is an open-access article distributed under the terms of the Creative Commons Attribution License (CC BY). The use, distribution or reproduction in other forums is permitted, provided the original author(s) and the copyright owner(s) are credited and that the original publication in this journal is cited, in accordance with accepted academic practice. No use, distribution or reproduction is permitted which does not comply with these terms.
*Correspondence: Shilong Zhong, c2h6MjAyMEBxcS5jb20=
†These authors share first authorship