- Department of Veterinary Pathobiology, College of Veterinary Medicine and Biomedical Sciences, Texas A&M University, College Station, TX, United States
Infections lacking precise diagnosis are often caused by a rare or uncharacterized pathogen, a combination of pathogens, or a known pathogen carrying undocumented or newly acquired genes. Despite medical advances in infectious disease diagnostics, many patients still experience mortality or long-term consequences due to undiagnosed or misdiagnosed infections. Thus, there is a need for an exhaustive and universal diagnostic strategy to reduce the fraction of undocumented infections. Compared to conventional diagnostics, metagenomic next-generation sequencing (mNGS) is a promising, culture-independent sequencing technology that is sensitive to detecting rare, novel, and unexpected pathogens with no preconception. Despite the fact that several studies and case reports have identified the effectiveness of mNGS in improving clinical diagnosis, there are obvious shortcomings in terms of sensitivity, specificity, costs, standardization of bioinformatic pipelines, and interpretation of findings that limit the integration of mNGS into clinical practice. Therefore, physicians must understand the potential benefits and drawbacks of mNGS when applying it to clinical practice. In this review, we will examine the current accomplishments, efficacy, and restrictions of mNGS in relation to conventional diagnostic methods. Furthermore, we will suggest potential approaches to enhance mNGS to its maximum capacity as a clinical diagnostic tool for identifying severe infections.
1. Introduction
1.1. Overview of the limitations of routine diagnostics for pathogen detection
Conventionally, the clinical detection of pathogens is based on the isolation and cultivation of organisms (Fournier et al., 2014). Once cultivated, these organisms are typically characterized using biochemical tests, mass spectrometry, nuclear magnetic resonance (NMR) spectrometry, nucleic acid amplification, or immunological testing (Carroll et al., 2019). Culture-dependent methods are considered the “gold standard” for diagnosis of infectious diseases in clinics but it may take several days to weeks to cultivate slow-growing organisms. Also, prior exposure to antibiotics can impair the sensitivity of culturing, thus missing cases of treatable diseases (Govender et al., 2021).
Polymerase chain reaction (PCR) is a widely used molecular diagnostic method in clinical laboratories that can rapidly detect the presence or absence of DNA and RNA from a clinical specimen without the need for microbial cultivation (Carroll et al., 2019). PCR-based tests have been further developed into real-time PCR, allowing the amplification, quantification of expression, and thus identification of specific pathogen genetic content with high sensitivity and specificity. However, PCR-based methodologies typically detect the presence or absence of a single gene at a time, offering low sensitivity, and potentially providing false negatives in cases containing low gene copy numbers (Huanyu Wang, 2021). To enhance the diagnostic capacity of PCR, multiplex PCR was developed to allow the simultaneous detection of multiple targets in a single PCR reaction (Huanyu Wang, 2021), although, it requires prior knowledge about pathogens of interest in order to identify them (Gu et al., 2021). Broad-range PCR is another effective method for hypothesis-independent detection of bacterial or fungal species, but has limitations. It has lower sensitivity than species-specific PCRs, cannot detect viral or parasitic infections, is only suitable for sterile bodily fluids and tissues, and can be more expensive than traditional methods (Rampini et al., 2011; Tkadlec et al., 2019; Aggarwal et al., 2020). Different PCR tests have varying diagnostic accuracy. In-house PCR tests are cheaper but require more time and training, while commercial PCR tests are automated and faster with higher sensitivity (Venter et al., 2019).
Alternatively, although antigen-detection is inexpensive and can be used in point-of-care setting due to promptness of the assay, diagnosis based on immunological tests are inherently less sensitive and may not provide accurate information (Govender et al., 2021). Furthermore, since it may take 1–2 weeks for antibodies to develop, antibody testing is not recommended for the diagnosis of acute disease (Govender et al., 2021).
In recent years, matrix-assisted laser desorption/ionization time-of-flight mass spectrometry (MALDI-TOF MS) has become a tool of choice for bacterial and fungal identification in clinical laboratories (Dingle and Butler-Wu, 2013). Although identification can be provided in minutes, MALDI-TOF requires bacterial cultivation prior to analysis. Furthermore, it is not a quantitative approach and presents low specificity (Roux-Dalvai et al., 2019).
Peptide nucleic acid fluorescent in situ hybridization (PNA-FISH) is a recently introduced rapid and reliable method for the detection of bacteria and fungi responsible for blood stream infections. It provides more timely results compared to traditional culturing-based methods (Calderaro et al., 2014). PNA-FISH is validated by the U.S. Food and Drug Administration (FDA) for diagnosis of blood samples, however, it is not available to use at the tissue level (Weaver et al., 2019). A summary of the current technologies used in routine diagnostics in the clinical setting is provided in Table 1.
1.2. Overview of clinical need and advantages of metagenomics
Clinical metagenomics using next-generation sequencing (mNGS) has the potential to surpass the limitations of conventional diagnostics and make a seismic shift in the care of patients suffering from various infections (Simner et al., 2018). Unlike other diagnostic methods, mNGS does not require background knowledge of a suspected pathogen (Duan et al., 2021). mNGS can capture millions to billions of nucleic acids sequences at once and detect multiple organisms including novel pathogens that may be present in a clinical specimen (John et al., 2021). The time required for sample preparation, sequencing, and preliminary bioinformatic analysis depends on the nature of sequencing platform being used (Morsli et al., 2021a,b, 2022a,b). For example, newly available long-read sequencing platforms, such as Oxford Nanopore sequencing, provide real time pathogen detection within minutes and additional information regarding genotyping and bacterial profiling in less than 6 h (Morsli et al., 2021a,b, 2022a,b). Also, Oxford Nanopore Technologies is currently the most prevalent and cost-effective mNGS platform in low- and middle-income countries (Yek et al., 2022). Clinical NGS includes two sequencing strategies: targeted amplicon sequencing and untargeted shotgun metagenomic sequencing. The targeted amplicon sequencing targets the universally conserved regions among bacteria (16S or 23S rRNA gene) or fungi and parasites (internal transcribed spacer (ITS), 18S rRNA, 28S rRNA gene) for pathogen detection (Salipante et al., 2013; Wagner et al., 2018). As an example, PCR-amplified 16S rRNA gene sequencing targets and amplifies one or more selected hypervariable regions (V1–V9) of the 16S rRNA gene. However, the choice of a particular hypervariable region targeted in 16S rRNA gene sequencing appears to be one of the biggest factors underlying technical variation in microbiome composition (Hiergeist et al., 2015; Tremblay et al., 2015; Gohl et al., 2016).
Unlike targeted amplicon sequencing, which only targets specific genes or gene regions, shotgun metagenomic sequencing targets the entire genetic content of a clinical sample, thus permitting the detection of all potential pathogens (Chiu and Miller, 2019). The capability to simultaneously identify viruses, bacteria, fungi, and parasites in a sample makes it broadly appealing for co-infection cases (Chen et al., 2021a). Furthermore, the amount of information derived from shotgun mNGS sequencing can potentially be used for additional analyses, such as antibiotic resistance profiling, virulence gene information, metabolic function profiling, and analyses of human host responses via transcriptome profiling (Chiu and Miller, 2019).
1.3. Commercially available clinical metagenomics platforms
Recently mNGS testing and analyses have become commercially available. For example, Charles Chiu and colleagues from the University of California, San Francisco (UCSF) are pioneers in the development of mNGS testing for the diagnosis of central nervous system (CNS) infections (Wilson et al., 2014). In 2014, the first use of mNGS was reported for the diagnosis of neuro-leptospirosis on CSF from a 14-year-old boy presenting the signs of meningoencephalitis (Wilson et al., 2014). This was the first report demonstrating the use of mNGS with medically actionable information and successful clinical diagnosis that led to the appropriate treatment of the patient (Wilson et al., 2014). Since then, UCSF provides validated mNGS DNA and RNA testing for patients with meningitis and/or encephalitis. The UCSF diagnostic lab also offers mNGS DNA testing for patients with sepsis and disseminated infections (Chiu and Miller, n.d.). UCSF software analyzes sequence reads, identifies those reads which align to pathogens in the GenBank database, and issues a report showing the presence of pathogens in a clinical sample, along with clinical interpretation. At least 66.7% of pathogens detected from CSF were true positives, and only 5.6% were found to be false positives (Miller et al., 2019). The mNGS test at USCF for pathogen detection from CSF specimens showed a sensitivity of 86.1% and a specificity of 97.9%. The mNGS test for pathogen detection from plasma samples showed a sensitivity of 77% and specificity of 86%. The turnaround time from shipping samples to delivery of a report is generally 1–2 weeks (Chiu and Miller, n.d.).
The Karius test (Karius, California, United States) is another example of how mNGS is useful for the diagnosis of bloodstream infections (BSIs) and sepsis (Blauwkamp et al., 2019). The Karius test involves extraction of cell-free DNA (cfDNA) from plasma, then a sequencing library is created and sequenced using Illumina technology. The sequence data is compared to an internal reference database encompassing a number of microbial genomes (Blauwkamp et al., 2019). A published study by Thair et al. confirmed that the Karius test detected approximately three times more positive cases than culture-based detection (Thair et al., 2017). However, the limitation is that the test can give false positive results. The Arizona-based Fry Laboratories also provides DNA sequencing diagnostic services for cutaneous, gastrointestinal, hematologic, musculoskeletal, and pulmonary infections (Fry, n.d.).
The Beijing Genomic Institute (BGI Genomics), a China-based company, is one of the largest companies that provide clinical mNGS services for the detection of pathogens causing respiratory infections such as Coronavirus and other pathogenic microorganisms (Jiang, n.d.). The sequencing services by the Zhejiang, China-based IngeniGen XunMinKang Biotechnology company also provide the detection of undiagnosed pathogens in patients with respiratory diseases (Wang et al., 2019; Li et al., 2022).
2. Detection of pathogens via clinical metagenomics
mNGS is an unbiased culture-independent and hypothesis-free sequencing technology that has shown tremendous clinical application particularly in the diagnosis of CNS infections, bloodstream infections, and respiratory tract infections (Blauwkamp et al., 2019; Miller et al., 2019; Wilson et al., 2019; Chen H. et al., 2020; Hasan et al., 2020; Haston et al., 2020; Li et al., 2020; Chen et al., 2021b; Hogan et al., 2021; Jing et al., 2021; Liu et al., 2021; Mu et al., 2021; Pollock et al., 2021; Zhou et al., 2021; Deng et al., 2022; Fu et al., 2022; Guo et al., 2022; Li et al., 2022; Wang et al., 2022; Zhang et al., 2022; Morsli et al., 2022b). Examples of recent applications of mNGS in the diagnosis of these infections are provided in Table 2. Below is a brief overview of the areas where mNGS has made considerable impact and the implications.
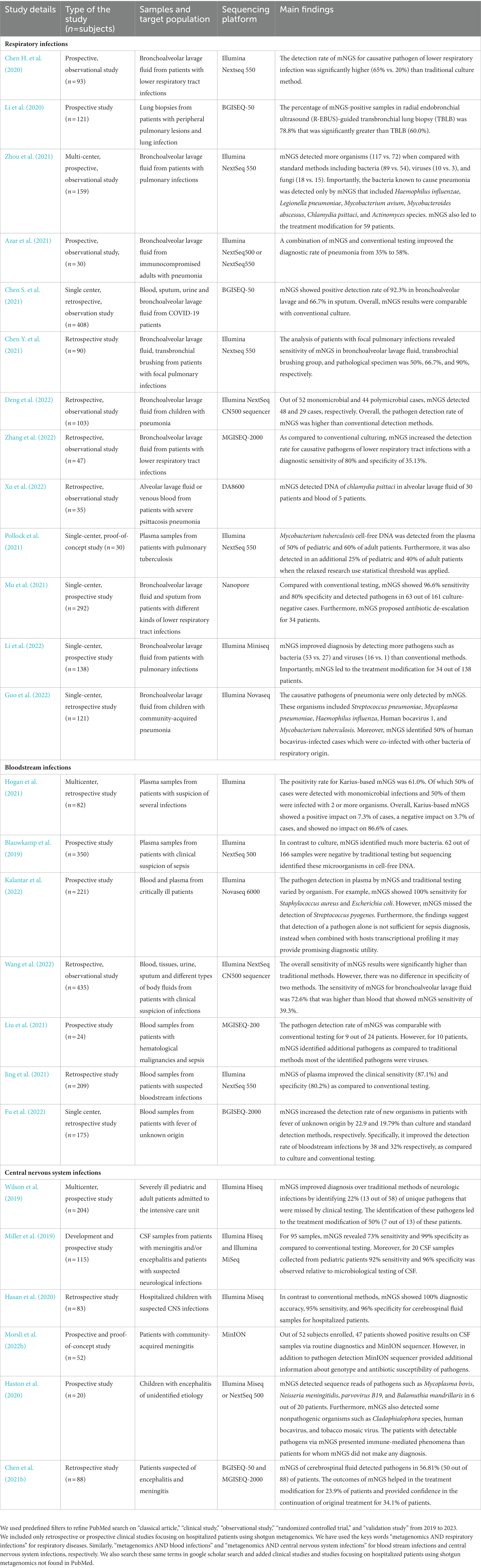
Table 2. Examples of the potential impact of clinical shotgun metagenomics (2019–2022) on infectious disease diagnosis.
2.1. Respiratory infections
Pneumonia is considered among the top 10 causes of death in the United States, especially among immunocompromised patients such as those with hematologic malignancy or undergoing hematopoietic stem cell transplant (Lippert et al., 2022). The identification of the causative agent of pneumonia is difficult and often inaccurate due to the pathogen diversity, heterogeneity of sampling, and limited detection methods (Buchan et al., 2022). Traditional molecular diagnosis for pneumonia is pathogen-specific but unreliable for novel or unexpected pathogens (Diao et al., 2022). The ability of mNGS to provide a comprehensive view of pathogens makes it useful in the diagnosis of unexplained pneumonia and disease of unknown etiology (Ramesh et al., 2019; Diao et al., 2022). Recently, mNGS has improved the diagnosis of pulmonary infections over traditional methods by detecting a broad range of organisms including bacteria, viruses and fungi in a number of recent investigations (Chen H. et al., 2020; Li et al., 2020; Mu et al., 2021; Deng et al., 2022; Li et al., 2022; Zhang et al., 2022). Remarkably, the causative agent was identified only by mNGS in two recent studies (Zhou et al., 2021; Guo et al., 2022). Importantly, mNGS led to the treatment modifications and guided treatment decisions for 127 patients with pulmonary infections (Mu et al., 2021; Zhou et al., 2021; Li et al., 2022). Moreover, mNGS detected 50% of cases coinfected with bacteria of different respiratory origin in another study (Guo et al., 2022).
A mNGS approach can be superior to traditional methods for pathogen detection and confirmation of respiratory infections, particularly for Mycobacterium tuberculosis (Jin et al., 2022). Mycobacterium tuberculosis, can be quite challenging to detect, however, it has been shown in the last few years that mNGS could potentially be used as the first-line diagnostic test for tuberculosis. Karius-based mNGS testing of plasma samples detected suspected tuberculosis in 60% of adults and 50% of pediatric patients (Pollock et al., 2021).
Lastly, RNA viruses are also considered one of the primary causes of respiratory infections (Miller and Chiu, 2022). mNGS can detect a number of viruses that are usually not screened for in respiratory infections using routine diagnostic assays (Prachayangprecha et al., 2014; Bohl et al., 2022). It has shown good sensitivity and specificity compared to conventional testing and can identify viruses such as Influenza, Rhinovirus, and HIV (Jia et al., 2021). An additional advantage to mNGS is the potential to document and describe emerging, and re-emerging viral infections associated with outbreaks (Quer et al., 2022). For example, RNA-based viral metagenomics has detected the presence of novel human coronavirus variants from patients with respiratory symptoms (Wu et al., 2020; Castañeda-Mogollón et al., 2021).
2.2. Bloodstream infections
In 2017, it was estimated that 48.9 million cases and 11 million deaths were related to sepsis globally (Rudd et al., 2020). Thus, the early and accurate diagnosis of BSI is critical to initiate appropriate antibiotic therapy and for patient survival. Recent findings indicate sequencing microbial cfDNA using mNGS is a valuable approach for the detection of BSI pathogens when the conventional diagnostics fail to detect the etiological agent (Hogan et al., 2021; Eichenberger et al., 2022). A retrospective multi-center study utilizing the cfDNA and RNA showed that mNGS had a positive impact in 7.3% of cases, a negative impact in 3.7% of cases, and no impact in 86.6% of cases in patients with suspicion of multiple infections (Hogan et al., 2021). Another study applied mNGS on cfDNA in septic and non-septic intensive care unit (ICU) patients and was able to diagnose sepsis and predicted mortality as soon as the first day (Jing et al., 2022). Similarly, cfDNA of relevant pathogens was detected in the blood plasma of cystic fibrosis patients (Barrett et al., 2020). mNGS testing improved the detection rate of BSI in patients having fever of unknown origin or patients with suspected BSI from 38% to 87.1% when compared to conventional methods (Jing et al., 2021; Fu et al., 2022; Wang et al., 2022). However, no difference was observed in specificity between two methods for patients with clinical suspicion of infections (Wang et al., 2022). In one report, mNGS pathogen detection rate was comparable with routine diagnostics in 37% of cases (Liu et al., 2021). In some scenarios, the pathogen detection rate for mNGS varied by organism. For instance, mNGS was 100% sensitive for the detection of Staphylococcus aureus and Escherichia coli. However, mNGS test missed the presence of Streptococcus pyogenes (Kalantar et al., 2022). In contrast, 37% of BSI cases were found to be positive by only mNGS test in patients with clinical suspicion of sepsis in another study (Blauwkamp et al., 2019).
2.3. Central nervous system infections
Neuroinflammatory diseases such as meningitis and encephalitis can be diagnostically challenging due to the requirement of invasive procedures for CSF collection, limited availability/low volume of CNS samples, and difficulty of detection by traditional culture (Vetter et al., 2020; Heming et al., 2022; Mokhtari et al., 2022). Furthermore, meningoencephalitis is related with increased risk of morbidity and mortality and thus needs prompt diagnosis and disease management (Ramachandran and Wilson, 2020). CSF culture is considered the gold-standard method for the diagnosis of meningitis. However, prior antibiotic therapy may reduce the sensitivity of CSF cultures, increasing the possibility of false-negatives (Greenberg and Herrera, 2019). mNGS has potential to detect pathogens of unknown etiology as evidenced by clinical series demonstrating the success of mNGS in the detection of hard-to-diagnose CNS infection cases (Miller et al., 2019; Wilson et al., 2019; Hasan et al., 2020; Haston et al., 2020; Chen et al., 2021b; Morsli et al., 2022b). Recently published studies have confirmed the diagnostic sensitivity of mNGS ranging 22% to 95% in patients with CNS infections (Miller et al., 2019; Wilson et al., 2019; Hasan et al., 2020). mNGS testing guided treatment decisions and clinical actionable management for 34.1%–53% of patients in these reports (Wilson et al., 2019; Chen et al., 2021b). However, contaminants from skin flora can lead to false positive bacterial sequences in CSF specimens obtained by lumbar puncture.
3. Limitations, knowledge gaps, and potential solutions of clinical metagenomics
As demonstrated in the previous sections, mNGS is a promising diagnostic technology. However, in the present state of knowledge, metagenomics is not always well-positioned to assist clinicians in rapid clinical decision-making due to expertise required for sample preparation, sequencing, bioinformatic analysis, and the high variability in methodologies and interpretation.
Pathogen detection by mNGS depends on the proportion of pathogen sequences in the total sequencing library. Essentially, the diagnostic performance of mNGS is optimal when the sequencing library contains a nominal fraction of host DNA or there is an enrichment of pathogenic sequences (Olausson et al., 2022). While mNGS tends to be more sensitive than traditional methods as evidenced by several studies (Duan et al., 2021; Guo et al., 2022; Li et al., 2022; Zhu et al., 2022; Wang et al., 2023), one of the caveats to being more sensitive is that mNGS can pick up microbial contamination derived from the environment, containers, reagents, and colonizing microorganisms in the human body, thus giving false-positive results. The use of negative controls is recommended for reagents and containers (López-Labrador et al., 2021). Moreover, depending on the sample source and bacterial load, in most cases the majority of reads in a mNGS data set can be derived from human DNA, while the proportion of pathogens tends to be very low (Gu et al., 2019). In order to overcome this challenge, depletion of host background DNA or targeted sequencing approaches such as depletion of abundant sequences by hybridization (DASH), and finding low-abundance sequences by hybridization (FLASH) in a combination of mNGS could be used (Gu et al., 2016; Hasan et al., 2016; Gu et al., 2019; Quan et al., 2019). For example, a positive selection probe-based system called virome capture sequencing platform for vertebrate viruses (VirCapSeq-VERT) has been established by a research group from the United States to increase the sensitivity of detecting viral sequences in clinical samples (Briese et al., 2015). Compact aggregation of targets for comprehensive hybridization (CATCH) is another available method to capture diverse targets from diverse patient samples (Viral Hemorrhagic Fever Consortium et al., 2019). The sequencing depth required to detect and characterize the genome of interest is a major influencer of metagenomics sensitivity. However, there is no consensus for how sequencing depth should be reported. For now, the choice of sequencing depth is dependent on budget and desired outcomes (Greninger, 2018). Retrospective experiments testing different human specimens with known infection at various sequencing depths are required to determine the ideal sequencing coverage for the diagnosis of different human specimens and infectious agents.
On that note, NGS tests do not equally detect all pathogens. For example, the detection rate of intracellular pathogens in clinical samples, such as a Mycobacterium, is relatively low as the amount of cell-free DNA of intracellular pathogen released into extracellular body fluids is relatively minute (Chen P. et al., 2020). The use of higher sequencing depth may help in identifying the presence of less abundant pathogens in a clinical sample. However, as a result of higher sequencing depth, a large amount of data would be generated, and thus, require more time for analysis. To avoid this challenge, rapid and more advanced bioinformatic tools must be developed (Chiu and Miller, 2019; Miller et al., 2020).
The human is a host to several commensal organisms, thus separating organisms associated with true bloodstream infection from transient gastrointestinal or oral flora in blood/plasma samples is an obstacle to the interpretation of mNGS results (Chiu and Miller, 2019; Chen et al., 2022). Retrospective studies using different threshold levels (such as a cut-off value for the abundance of pathogens, number of sequencing reads to detect a specific pathogen, and sequencing read normalization) are required to differentiate potential pathogens from commensal organisms. Moreover, the clinical significance of identified organisms needs to be further confirmed by conventional testing and the condition of the host (Chen et al., 2022). If a patient presents with an infection and receives appropriate curative therapy, mNGS can still detect lingering DNA from dead pathogens. It is unknown how long the detectable half-life of a pathogen is once the patient receives appropriate treatment and is conceivably circumstantial. A potential solution is the detection of RNA, whose abundance is directly correlated with the degree of gene transcription activity, thus, it can distinguish dead and live organisms in a clinical sample (d’Humières et al., 2021). Compared with DNA sequencing, a combination of DNA and RNA sequencing may have additional benefits (Arroyo Mühr et al., 2021). However, the detection of RNA through mNGS still has its challenges because of the higher abundance of human-derived RNA in a clinical sample as well as the labile nature of RNA. To overcome this challenge, depletion of the host background is needed (Zheng et al., 2021).
Another limitation of the technology is the actual determination of what is detected (López-Labrador et al., 2021). For example, incomplete databases, mis-annotated sequences, databases containing contaminating organisms, bias in databases, and misclassification of organisms all affect the actual determination of the pathogen after sequencing (López-Labrador et al., 2021; Diao et al., 2022). Moreover, differences in pipelines, reproducibility, quality control, and workflow may lead to different and inaccurate pathogen identification between hospitals and commercially available mNGS tools (López-Labrador et al., 2021). Other logistical challenges include patient privacy, bioinformatic data storage, and lack of standardization (Diao et al., 2022). Continuous effort is needed to improve academically and commercially available tools and make them more accessible to the public (Chen et al., 2022). For example, efforts for improving crowdsourcing of bioinformatics pipelines, software, and taxonomic metagenome profilers could be made. Furthermore, stringent quality controls in the laboratory such as unidirectional workflow, strict decontamination methods during nucleic acid processing, and use of negative controls can help in reducing the detection of exogenous DNA contamination derived from reagents and laboratory environment (Chen et al., 2022).
Currently, the role of mNGS is mostly derived from case reports and small cohort studies (Zhang et al., 2020). Large-scale clinical and cross-institutional studies are required to validate the clinical efficacy of mNGS and to provide a better understanding of how metagenomic approaches could help us to improve patient outcomes over the current standard of care (Zhang et al., 2023). While mNGS may be more expensive than routine diagnostics, the incremental cost is minimum when compared to the cost of invasive diagnostic procedures, a series of diagnostic tests, and cost of intensive care units in hospital; thus, it may help in reducing the overall health care resources (Miller et al., 2020).
4. Conclusions and future perspectives
In conclusion, the diversity in clinical metagenomics methods, although allowing flexibility, translates to variability in application and performance relative to conventional diagnostics. Given sensitivity and specificity of mNGS is influenced by a number of factors including the sample type, quantity of host DNA, the sequencing platform used, number of reads generated, selected reference database, and data analysis tools, as well as issues surrounding the current cost and expertise limitations, it is unlikely clinical metagenomics will be utilized as a first-line approach unless these issues are resolved. Thus, an important question in clinical metagenomics remains: Should mNGS only be used as a last resort when gold-standard culture-based or current molecular diagnostic methods fail? Or are there benefits to using it at earlier stages in certain populations?
At this juncture, clinical settings where copious resources are already expended or where patients are at risk for mortality from rare or difficult to treat pathogens, such as in critically ill or immunosuppressed patients (transplant, cancer etc.), could exemplify practical target populations for implementing clinical metagenomics as a standard of care. Despite unresolved application questions, mNGS has undeniable benefits over traditional testing and potentially provides a more complete picture of the state of any clinical infection. Thus, the combination of mNGS and conventional diagnostic methods could be a superior diagnostic strategy to improve overall public health and healthcare associated costs. Moreover, mNGS may vital for time-sensitive diagnostics in life-threatening infections. With advancements in sequencing technology, clinical metagenomic sequencing can not only identify the pathogen but also predict antimicrobial resistance and virulence, enabling prompt and effective treatment. However, to fully utilize mNGS as a clinical diagnostic tool, it is essential to standardize the methods, bioinformatics, and databases used in practice.
Author contributions
JG-P conceived and supervised the review topic. All authors contributed to the article and approved the submitted version.
Funding
This work was partially supported by NIH 1K01AI143881 to JG-P.
Conflict of interest
The authors declare that the research was conducted in the absence of any commercial or financial relationships that could be construed as a potential conflict of interest.
Publisher’s note
All claims expressed in this article are solely those of the authors and do not necessarily represent those of their affiliated organizations, or those of the publisher, the editors and the reviewers. Any product that may be evaluated in this article, or claim that may be made by its manufacturer, is not guaranteed or endorsed by the publisher.
References
Aggarwal, D., Kanitkar, T., Narouz, M., Azadian, B. S., Moore, L. S. P., and Mughal, N. (2020). Clinical utility and cost-effectiveness of bacterial 16S rRNA and targeted PCR based diagnostic testing in a UK microbiology laboratory network. Sci. Rep. 10:7965. doi: 10.1038/s41598-020-64739-1
Arroyo Mühr, L. S., Dillner, J., Ure, A. E., Sundström, K., and Hultin, E. (2021). Comparison of DNA and RNA sequencing of total nucleic acids from human cervix for metagenomics. Sci. Rep. 11:18852. doi: 10.1038/s41598-021-98452-4
Azar, M. M., Schlaberg, R., Malinis, M. F., Bermejo, S., Schwarz, T., Xie, H., et al. (2021). Added diagnostic utility of clinical metagenomics for the diagnosis of pneumonia in immunocompromised adults. Chest 159, 1356–1371. doi: 10.1016/j.chest.2020.11.008
Barrett, S. L. R., Holmes, E. A., Long, D. R., Shean, R. C., Bautista, G. E., Ravishankar, S., et al. (2020). Cell free DNA from respiratory pathogens is detectable in the blood plasma of cystic fibrosis patients. Sci. Rep. 10, 1–6. doi: 10.1038/s41598-020-63970-0
Blauwkamp, T. A., Thair, S., Rosen, M. J., Blair, L., Lindner, M. S., Vilfan, I. D., et al. (2019). Analytical and clinical validation of a microbial cell-free DNA sequencing test for infectious disease. Nat. Microbiol. 4, 663–674. doi: 10.1038/s41564-018-0349-6
Bohl, J. A., Lay, S., Chea, S., Ahyong, V., Parker, D. M., Gallagher, S., et al. (2022). Discovering disease-causing pathogens in resource-scarce Southeast Asia using a global metagenomic pathogen monitoring system. Proc. Natl. Acad. Sci. 119:e2115285119. doi: 10.1073/pnas.2115285119
Briese, T., Kapoor, A., Mishra, N., Jain, K., Kumar, A., Jabado, O. J., et al. (2015). Virome capture sequencing enables sensitive viral diagnosis and comprehensive virome analysis. MBio 6, e01491–e01415. doi: 10.1128/mBio.01491-15
Buchan, B. W., Armand-Lefevre, L., and Anderson, N. (2022). Molecular diagnosis of pneumonia (including multiplex panels). Clin. Chem. 68, 59–68. doi: 10.1093/clinchem/hvab143
Calderaro, A., Martinelli, M., Motta, F., Larini, S., Arcangeletti, M. C., Medici, M. C., et al. (2014). Comparison of peptide nucleic acid fluorescence in situ hybridization assays with culture-based matrix-assisted laser desorption/ionization-time of flight mass spectrometry for the identification of bacteria and yeasts from blood cultures and cerebrospinal fluid cultures. Clin. Microbiol. Infect. 20, O468–O475. doi: 10.1111/1469-0691.12490
Carroll, K. C., Hobden, J. A., Miller, S., Morse, S. A., Mietzner, T. A., Detrick, B., et al. (eds). (2019). “Principles of diagnostic medical microbiology” in Jawetz, Melnick, & Adelberg’s medical microbiology, 27e (New York, NY: McGraw-Hill Education)
Castañeda-Mogollón, D., Kamaliddin, C., Oberding, L., Liu, Y., Mohon, A. N., Faridi, R. M., et al. (2021). A metagenomics workflow for SARS-CoV-2 identification, co-pathogen detection, and overall diversity. J. Clin. Virol. 145:105025. doi: 10.1016/j.jcv.2021.105025
Chen, Y., Fan, L. C., Chai, Y. H., and Xu, J. F. (2022). Advantages and challenges of metagenomic sequencing for the diagnosis of pulmonary infectious diseases. Clin. Respir. J. 16, 646–656. doi: 10.1111/crj.13538
Chen, P., Sun, W., and He, Y. (2020). Comparison of metagenomic next-generation sequencing technology, culture and GeneXpert MTB/RIF assay in the diagnosis of tuberculosis. J. Thorac. Dis. 12, 4014–4024. doi: 10.21037/jtd-20-1232
Chen, Y., Yan, X., Li, N., Zhang, Q., Lv, Y., and Ruan, T. (2021). Metagenomic next-generation sequencing of radial ultrasound bronchoscopy-guided" cocktail" specimens as an efficient method for the diagnosis of focal pulmonary infections: a randomised study. Annals Palliat Med 10, 2080–2088. doi: 10.21037/apm-20-2578
Chen, H., Yin, Y., Gao, H., Guo, Y., Dong, Z., Wang, X., et al. (2020). Clinical utility of in-house metagenomic next-generation sequencing for the diagnosis of lower respiratory tract infections and analysis of the host immune response. Clin. Infect. Dis. 71, S416–S426. doi: 10.1093/cid/ciaa1516
Chen, J., Zhang, R., Liu, L., Qi, T., Wang, Z., Song, W., et al. (2021b). Clinical usefulness of metagenomic next-generation sequencing for the diagnosis of central nervous system infection in people living with HIV. Int. J. Infect. Dis. 107, 139–144. doi: 10.1016/j.ijid.2021.04.057
Chen, J., Zhao, Y., Shang, Y., Lin, Z., Xu, G., Bai, B., et al. (2021a). The clinical significance of simultaneous detection of pathogens from bronchoalveolar lavage fluid and blood samples by metagenomic next-generation sequencing in patients with severe pneumonia. J. Med. Microbiol. 70:001259. doi: 10.1099/jmm.0.001259
Chen, S., Zhu, Q., Xiao, Y., Wu, C., Jiang, Z., Liu, L., et al. (2021). Clinical and etiological analysis of co-infections and secondary infections in COVID-19 patients: an observational study. Clin. Respir. J. 15, 815–825. doi: 10.1111/crj.13369
Chiu, C. Y., and Miller, S. A. (2019). Clinical metagenomics. Nat. Rev. Genet. 20, 341–355. doi: 10.1038/s41576-019-0113-7
Chiu, C. Y., and Miller, S. A.. (n.d.). Univeristy of California San Francisco: The next generation of diagnostic tests. Available at: https://nextgendiagnostics.ucsf.edu/our-diagnostic-lab/.
d’Humières, C., Salmona, M., Dellière, S., Leo, S., Rodriguez, C., Angebault, C., et al. (2021). The potential role of clinical metagenomics in infectious diseases: therapeutic perspectives. Drugs 81, 1453–1466. doi: 10.1007/s40265-021-01572-4
Deng, W., Xu, H., Wu, Y., and Li, J. (2022). Diagnostic value of bronchoalveolar lavage fluid metagenomic next-generation sequencing in pediatric pneumonia. Front. Cell. Infect. Microbiol. 12:1536. doi: 10.3389/fcimb.2022.950531
Diao, Z., Han, D., Zhang, R., and Li, J. (2022). Metagenomics next-generation sequencing tests take the stage in the diagnosis of lower respiratory tract infections. J. Adv. Res. 38, 201–212. doi: 10.1016/j.jare.2021.09.012
Dingle, T. C., and Butler-Wu, S. M. (2013). MALDI-TOF mass spectrometry for microorganism identification. Clin. Lab. Med. 33, 589–609. doi: 10.1016/j.cll.2013.03.001
Duan, H., Li, X., Mei, A., Li, P., Liu, Y., Li, X., et al. (2021). The diagnostic value of metagenomic next⁃ generation sequencing in infectious diseases. BMC Infect. Dis. 21, 1–13. doi: 10.1186/s12879-020-05746-5
Eichenberger, E. M., de Vries, C. R., Ruffin, F., Sharma-Kuinkel, B., Park, L., Hong, D., et al. (2022). Microbial cell-free DNA identifies etiology of bloodstream infections, persists longer than conventional blood cultures, and its duration of detection is associated with metastatic infection in patients with staphylococcus aureus and gram-negative bacteremia. Clin. Infect. Dis. 74, 2020–2027. doi: 10.1093/cid/ciab742
Fournier, P.-E., Dubourg, G., and Raoult, D. (2014). Clinical detection and characterization of bacterial pathogens in the genomics era. Genome Med. 6, 1–15. doi: 10.1186/s13073-014-0114-2
Fry, S. E. (n.d.). Fry laboratories: Next-generation sequencing for infectious diseases Available at: https://frylabs.com/dna-sequencing/.
Fu, Z.-F., Zhang, H. C., Zhang, Y., Cui, P., Zhou, Y., Wang, H. Y., et al. (2022). Evaluations of clinical utilization of metagenomic next-generation sequencing in adults with fever of unknown origin. Front. Cell. Infect. Microbiol. 11:1250. doi: 10.3389/fcimb.2021.745156
Gohl, D. M., Vangay, P., Garbe, J., MacLean, A., Hauge, A., Becker, A., et al. (2016). Systematic improvement of amplicon marker gene methods for increased accuracy in microbiome studies. Nat. Biotechnol. 34, 942–949. doi: 10.1038/nbt.3601
Govender, K. N., Street, T. L., Sanderson, N. D., and Eyre, D. W. (2021). Metagenomic sequencing as a pathogen-agnostic clinical diagnostic tool for infectious diseases: a systematic review and meta-analysis of diagnostic test accuracy studies. J. Clin. Microbiol. 59, e02916–e02920. doi: 10.1128/JCM.02916-20
Greenberg, R. G., and Herrera, T. I. (2019). “Chapter 8—when to perform lumbar puncture in infants at risk for meningitis in the neonatal intensive care unit” in Infectious disease and pharmacology. eds. W. E. Benitz and P. B. Smith (Philadelphia: Elsevier), 87–102.
Greninger, A. L. (2018). The challenge of diagnostic metagenomics. Expert Rev. Mol. Diagn. 18, 605–615. doi: 10.1080/14737159.2018.1487292
Gu, W., Crawford, E. D., O’Donovan, B. D., Wilson, M. R., Chow, E. D., Retallack, H., et al. (2016). Depletion of abundant sequences by hybridization (DASH): using Cas9 to remove unwanted high-abundance species in sequencing libraries and molecular counting applications. Genome Biol. 17, 1–13. doi: 10.1186/s13059-016-0904-5
Gu, W., Deng, X., Lee, M., Sucu, Y. D., Arevalo, S., Stryke, D., et al. (2021). Rapid pathogen detection by metagenomic next-generation sequencing of infected body fluids. Nat. Med. 27, 115–124. doi: 10.1038/s41591-020-1105-z
Gu, W., Miller, S., and Chiu, C. Y. (2019). Clinical metagenomic next-generation sequencing for pathogen detection. Annu. Rev. Pathol. 14, 319–338. doi: 10.1146/annurev-pathmechdis-012418-012751
Guo, W., Cui, X., Wang, Q., Wei, Y., Guo, Y., Zhang, T., et al. (2022). Clinical evaluation of metagenomic next-generation sequencing for detecting pathogens in bronchoalveolar lavage fluid collected from children with community-acquired pneumonia. Front. Med. 9:9. doi: 10.3389/fmed.2022.952636
Hasan, M. R., Rawat, A., Tang, P., Jithesh, P. V., Thomas, E., Tan, R., et al. (2016). Depletion of human DNA in spiked clinical specimens for improvement of sensitivity of pathogen detection by next-generation sequencing. J. Clin. Microbiol. 54, 919–927. doi: 10.1128/JCM.03050-15
Hasan, M. R., Sundararaju, S., Tang, P., Tsui, K. M., Lopez, A. P., Janahi, M., et al. (2020). A metagenomics-based diagnostic approach for central nervous system infections in hospital acute care setting. Sci. Rep. 10:11194. doi: 10.1038/s41598-020-68159-z
Haston, J. C., Rostad, C. A., Jerris, R. C., Milla, S. S., McCracken, C., Pratt, C., et al. (2020). Prospective cohort study of next-generation sequencing as a diagnostic modality for unexplained encephalitis in children. J Pediatr Infect Diseases Soc 9, 326–333. doi: 10.1093/jpids/piz032
Heming, M., Börsch, A. L., Wiendl, H., and Meyer zu Hörste, G. (2022). High-dimensional investigation of the cerebrospinal fluid to explore and monitor CNS immune responses. Genome Med. 14, 1–12. doi: 10.1186/s13073-022-01097-9
Hiergeist, A., Gläsner, J., Reischl, U., and Gessner, A. (2015). Analyses of intestinal microbiota: culture versus sequencing. ILAR J. 56, 228–240. doi: 10.1093/ilar/ilv017
Hogan, C. A., Yang, S., Garner, O. B., Green, D. A., Gomez, C. A., Dien Bard, J., et al. (2021). Clinical impact of metagenomic next-generation sequencing of plasma cell-free DNA for the diagnosis of infectious diseases: a multicenter retrospective cohort study. Clin. Infect. Dis. 72, 239–245. doi: 10.1093/cid/ciaa035
Huanyu Wang, S. J. (2021). Next-generation sequencing for infectious diseases diagnostics—Is it worth the hype? Washington, DC: American Association for clinical chemistry.
Jia, X., Hu, L., Wu, M., Ling, Y., Wang, W., Lu, H., et al. (2021). A streamlined clinical metagenomic sequencing protocol for rapid pathogen identification. Sci. Rep. 11, 1–10. doi: 10.1038/s41598-021-83812-x
Jiang, W. (n.d.). Beijing genomic institute (BGI genomics): Metagenomic sequencing. Available at: https://www.bgi.com/global/science-detail/metagenomic-sequencing.
Jin, X., Li, J., Shao, M., Lv, X., Ji, N., Zhu, Y., et al. (2022). Improving suspected pulmonary infection diagnosis by Bronchoalveolar lavage fluid metagenomic next-generation sequencing: a multicenter retrospective study. Microbiol Spectr 10, e02473–e02421. doi: 10.1128/spectrum.02473-21
Jing, C., Chen, H., Liang, Y., Zhong, Y., Wang, Q., Li, L., et al. (2021). Clinical evaluation of an improved metagenomic next-generation sequencing test for the diagnosis of bloodstream infections. Clin. Chem. 67, 1133–1143. doi: 10.1093/clinchem/hvab061
Jing, Q., Leung, C. H. C., and Wu, A. R. (2022). Cell-free DNA as biomarker for sepsis by integration of microbial and host information. Clin. Chem. 68, 1184–1195. doi: 10.1093/clinchem/hvac097
John, G., Sahajpal, N. S., Mondal, A. K., Ananth, S., Williams, C., Chaubey, A., et al. (2021). Next-generation sequencing (NGS) in COVID-19: a tool for SARS-CoV-2 diagnosis, monitoring new strains and phylodynamic modeling in molecular epidemiology. Curr. Issues Mol. Biol. 43, 845–867. doi: 10.3390/cimb43020061
Kalantar, K. L., Neyton, L., Abdelghany, M., Mick, E., Jauregui, A., Caldera, S., et al. (2022). Integrated host-microbe plasma metagenomics for sepsis diagnosis in a prospective cohort of critically ill adults. Nat. Microbiol. 7, 1–12. doi: 10.1038/s41564-022-01237-2
Li, G., Huang, J., Li, Y., and Feng, J. (2020). The value of combined radial endobronchial ultrasound-guided transbronchial lung biopsy and metagenomic next-generation sequencing for peripheral pulmonary infectious lesions. Can. Respir. J. 2020, 1–9. doi: 10.1155/2020/2367505
Li, N., Ma, X., Zhou, J., Deng, J., Gu, C., Fei, C., et al. (2022). Clinical application of metagenomic next-generation sequencing technology in the diagnosis and treatment of pulmonary infection pathogens: a prospective single-center study of 138 patients. J. Clin. Lab. Anal. 36:e24498. doi: 10.1002/jcla.24498
Lippert, J. F., Buscemi, J., Saiyed, N., Silva, A., and Benjamins, M. R. (2022). Influenza and pneumonia mortality across the 30 biggest US cities: assessment of overall trends and racial inequities. J. Racial Ethn. Health Disparities 9, 1152–1160. doi: 10.1007/s40615-021-01056-x
Liu, W.-D., Yen, T. Y., Liu, P. Y., Wu, U. I., Bhan, P., Li, Y. C., et al. (2021). Clinical application of metagenomic next-generation sequencing in patients with hematologic malignancies suffering from sepsis. Microorganisms 9:2309. doi: 10.3390/microorganisms9112309
López-Labrador, F. X., Brown, J. R., Fischer, N., Harvala, H., van Boheemen, S., Cinek, O., et al. (2021). Recommendations for the introduction of metagenomic high-throughput sequencing in clinical virology, part I: wet lab procedure. J. Clin. Virol. 134:104691. doi: 10.1016/j.jcv.2020.104691
Miller, S., and Chiu, C. (2022). The role of metagenomics and next-generation sequencing in infectious disease diagnosis. Clin. Chem. 68, 115–124. doi: 10.1093/clinchem/hvab173
Miller, S., Chiu, C., Rodino, K. G., and Miller, M. B. (2020). Point-counterpoint: should we be performing metagenomic next-generation sequencing for infectious disease diagnosis in the clinical laboratory? J. Clin. Microbiol. 58, e01739–e01719. doi: 10.1128/JCM.01739-19
Miller, S., Naccache, S. N., Samayoa, E., Messacar, K., Arevalo, S., Federman, S., et al. (2019). Laboratory validation of a clinical metagenomic sequencing assay for pathogen detection in cerebrospinal fluid. Genome Res. 29, 831–842. doi: 10.1101/gr.238170.118
Mokhtari, M., Alizadeh, A., Monabati, A., and Safaei, A. (2022). Comparison of flowcytometry and conventional cytology for diagnosis of CNS involvement in hematologic malignancies. Pediatr Hematol Oncol J 7, 146–154. doi: 10.1016/j.phoj.2022.08.005
Morsli, M., Boudet, A., Kerharo, Q., Stephan, R., Salipante, F., Dunyach-Remy, C., et al. (2022b). Real-time metagenomics-based diagnosis of community-acquired meningitis: a prospective series, southern France. EBioMedicine 84:104247. doi: 10.1016/j.ebiom.2022.104247
Morsli, M., Kerharo, Q., Delerce, J., Roche, P. H., Troude, L., and Drancourt, M. (2021a). Haemophilus influenzae meningitis direct diagnosis by metagenomic next-generation sequencing: a case report. Pathogens 10:461. doi: 10.3390/pathogens10040461
Morsli, M., Lavigne, J. P., and Drancourt, M. (2022a). Direct metagenomic diagnosis of community-acquired meningitis: state of the art. Front. Microbiol. 13:13. doi: 10.3389/fmicb.2022.926240
Morsli, M., Vincent, J. J., Milliere, L., Colson, P., and Drancourt, M. (2021b). Direct next-generation sequencing diagnosis of echovirus 9 meningitis, France. Eur. J. Clin. Microbiol. Infect. Dis. 40, 2037–2039. doi: 10.1007/s10096-021-04205-6
Mu, S., Hu, L., Zhang, Y., Liu, Y., Cui, X., Zou, X., et al. (2021). Prospective evaluation of a rapid clinical metagenomics test for bacterial pneumonia. Front. Cell. Infect. Microbiol. 11:953. doi: 10.3389/fcimb.2021.684965
Olausson, J., Brunet, S., Vracar, D., Tian, Y., Abrahamsson, S., Meghadri, S. H., et al. (2022). Optimization of cerebrospinal fluid microbial DNA metagenomic sequencing diagnostics. Sci. Rep. 12:3378. doi: 10.1038/s41598-022-07260-x
Pollock, N., MacIntyre, A., Blauwkamp, T. A., Blair, L., Ho, C., Calderon, R., et al. (2021). Detection of Mycobacterium tuberculosis cell-free DNA to diagnose TB in pediatric and adult patients. Int. J. Tuberc. Lung Dis. 25, 403–405. doi: 10.5588/ijtld.21.0055
Prachayangprecha, S., Schapendonk, C. M. E., Koopmans, M. P., Osterhaus, A. D. M. E., Schürch, A. C., Pas, S. D., et al. (2014). Exploring the potential of next-generation sequencing in detection of respiratory viruses. J. Clin. Microbiol. 52, 3722–3730. doi: 10.1128/JCM.01641-14
Quan, J., Langelier, C., Kuchta, A., Batson, J., Teyssier, N., Lyden, A., et al. (2019). FLASH: a next-generation CRISPR diagnostic for multiplexed detection of antimicrobial resistance sequences. Nucleic Acids Res. 47:e83. doi: 10.1093/nar/gkz418
Quer, J., Colomer-Castell, S., Campos, C., Andrés, C., Piñana, M., Cortese, M. F., et al. (2022). Next-generation sequencing for confronting virus pandemics. Viruses 14:600. doi: 10.3390/v14030600
Ramachandran, P. S., and Wilson, M. R. (2020). Metagenomics for neurological infections—expanding our imagination. Nat. Rev. Neurol. 16, 547–556. doi: 10.1038/s41582-020-0374-y
Ramesh, A., Nakielny, S., Hsu, J., Kyohere, M., Byaruhanga, O., de Bourcy, C., et al. (2019). Metagenomic next-generation sequencing of samples from pediatric febrile illness in Tororo, Uganda. PLoS One 14:e0218318. doi: 10.1371/journal.pone.0218318
Rampini, S. K., Bloemberg, G. V., Keller, P. M., Büchler, A. C., Dollenmaier, G., Speck, R. F., et al. (2011). Broad-range 16S rRNA gene polymerase chain reaction for diagnosis of culture-negative bacterial infections. Clin. Infect. Dis. 53, 1245–1251. doi: 10.1093/cid/cir692
Roux-Dalvai, F., Gotti, C., Leclercq, M., Hélie, M. C., Boissinot, M., Arrey, T. N., et al. (2019). Fast and accurate bacterial species identification in urine specimens using LC-MS/MS mass spectrometry and machine learning*[S]. Mol. Cell. Proteomics 18, 2492–2505. doi: 10.1074/mcp.TIR119.001559
Rudd, K. E., Johnson, S. C., Agesa, K. M., Shackelford, K. A., Tsoi, D., Kievlan, D. R., et al. (2020). Global, regional, and national sepsis incidence and mortality, 1990–2017: analysis for the global burden of disease study. Lancet 395, 200–211. doi: 10.1016/S0140-6736(19)32989-7
Salipante, S. J., Sengupta, D. J., Rosenthal, C., Costa, G., Spangler, J., Sims, E. H., et al. (2013). Rapid 16S rRNA next-generation sequencing of polymicrobial clinical samples for diagnosis of complex bacterial infections. PLoS One 8:e65226. doi: 10.1371/journal.pone.0065226
Simner, P. J., Miller, S., and Carroll, K. C. (2018). Understanding the promises and hurdles of metagenomic next-generation sequencing as a diagnostic tool for infectious diseases. Clin. Infect. Dis. 66, 778–788. doi: 10.1093/cid/cix881
Thair, S., Seng, H., Hollemon, D., Hong, D., Blauwkamp, T., Kertesz, M., et al. (2017). “The SEP-SEQ trial: clinical validation of the Karius plasma next-generation sequencing test for pathogen detection in sepsis” in Open forum infectious diseases (Oxford University Press), 4.
Tkadlec, J., Peckova, M., Sramkova, L., Rohn, V., Jahoda, D., Raszka, D., et al. (2019). The use of broad-range bacterial PCR in the diagnosis of infectious diseases: a prospective cohort study. Clin. Microbiol. Infect. 25, 747–752. doi: 10.1016/j.cmi.2018.10.001
Tremblay, J., Singh, K., Fern, A., Kirton, E. S., He, S., Woyke, T., et al. (2015). Primer and platform effects on 16S rRNA tag sequencing. Front. Microbiol. 6:771. doi: 10.3389/fmicb.2015.00771
Venter, J. M., Mahlangu, P. M., Müller, E. E., Lewis, D. A., Rebe, K., Struthers, H., et al. (2019). Comparison of an in-house real-time duplex PCR assay with commercial HOLOGIC® APTIMA assays for the detection of Neisseria gonorrhoeae and Chlamydia trachomatis in urine and extra-genital specimens. BMC Infect. Dis. 19, 6–7. doi: 10.1186/s12879-018-3629-0
Vetter, P., Schibler, M., Herrmann, J. L., and Boutolleau, D. (2020). Diagnostic challenges of central nervous system infection: extensive multiplex panels versus stepwise guided approach. Clin. Microbiol. Infect. 26, 706–712. doi: 10.1016/j.cmi.2019.12.013
Viral Hemorrhagic Fever ConsortiumMetsky, H. C., Siddle, K. J., Gladden-Young, A., Qu, J., Yang, D. K., et al. (2019). Capturing sequence diversity in metagenomes with comprehensive and scalable probe design. Nat. Biotechnol. 37, 160–168. doi: 10.1038/s41587-018-0006-x
Wagner, K., Springer, B., Pires, V. P., and Keller, P. M. (2018). Molecular detection of fungal pathogens in clinical specimens by 18S rDNA high-throughput screening in comparison to ITS PCR and culture. Sci. Rep. 8, 1–7. doi: 10.1038/s41598-018-25129-w
Wang, L., Li, S., Qin, J., Tang, T., Hong, J., Tung, T. H., et al. (2023). Clinical diagnosis application of metagenomic next-generation sequencing of plasma in suspected Sepsis. Infect Drug Resist 16, 891–901. doi: 10.2147/IDR.S395700
Wang, G., Xu, N., Yang, L., Zheng, F., Sai, L., Zhou, J., et al. (2019). Community acquired Stenotrophomonas maltophilia discitis: diagnosis aided by shotgun metagenomic sequencing. Int. J. Infect. Dis. 81, 1–3. doi: 10.1016/j.ijid.2019.01.032
Wang, C., Yan, D., Huang, J., Yang, N., Shi, J., Pan, S., et al. (2022). The clinical application of metagenomic next-generation sequencing in infectious diseases at a tertiary hospital in China. Front. Cell. Infect. Microbiol. 12:1853. doi: 10.3389/fcimb.2022.957073
Weaver, A. J., Brandenburg, K. S., Sanjar, F., Wells, A. R., Peacock, T. J., and Leung, K. P. (2019). Clinical utility of PNA-FISH for burn wound diagnostics: a noninvasive, culture-independent technique for rapid identification of pathogenic organisms in burn wounds. J. Burn Care Res. 40, 464–470. doi: 10.1093/jbcr/irz047
Wilson, M. R., Naccache, S. N., Samayoa, E., Biagtan, M., Bashir, H., Yu, G., et al. (2014). Actionable diagnosis of neuroleptospirosis by next-generation sequencing. N. Engl. J. Med. 370, 2408–2417. doi: 10.1056/NEJMoa1401268
Wilson, M. R., Sample, H. A., Zorn, K. C., Arevalo, S., Yu, G., Neuhaus, J., et al. (2019). Clinical metagenomic sequencing for diagnosis of meningitis and encephalitis. N. Engl. J. Med. 380, 2327–2340. doi: 10.1056/NEJMoa1803396
Wu, F., Zhao, S., Yu, B., Chen, Y. M., Wang, W., Song, Z. G., et al. (2020). A new coronavirus associated with human respiratory disease in China. Nature 579, 265–269. doi: 10.1038/s41586-020-2008-3
Xu, L., Zhao, Z., Mai, H., Tan, X., du, Y., and Fang, C. (2022). Clinical and chest computed tomography features associated with severe Chlamydia psittaci pneumonia diagnosed by metagenomic next-generation sequencing: a multicenter, retrospective, observational study. Medicine 101:e32117. doi: 10.1097/MD.0000000000032117
Yek, C., Pacheco, A. R., Vanaerschot, M., Bohl, J. A., Fahsbender, E., Aranda-Díaz, A., et al. (2022). Metagenomic pathogen sequencing in resource-scarce settings: lessons learned and the road ahead. Front Epidemiol 2:2. doi: 10.3389/fepid.2022.926695
Zhang, X., Chen, H., Han, D., and Wu, W. (2023). Clinical usefulness of metagenomic next-generation sequencing for Rickettsia and Coxiella burnetii diagnosis. Eur. J. Clin. Microbiol. Infect. Dis. 42, 681–689. doi: 10.1007/s10096-023-04586-w
Zhang, P., Chen, Y., Li, S., Li, C., Zhang, S., Zheng, W., et al. (2020). Metagenomic next-generation sequencing for the clinical diagnosis and prognosis of acute respiratory distress syndrome caused by severe pneumonia: a retrospective study. PeerJ 8:e9623. doi: 10.7717/peerj.9623
Zhang, D., Yang, X., Wang, J., Xu, J., and Wang, M. (2022). Application of metagenomic next-generation sequencing for bronchoalveolar lavage diagnostics in patients with lower respiratory tract infections. J. Int. Med. Res. 50:030006052210897. doi: 10.1177/03000605221089795
Zheng, Y., Qiu, X., Wang, T., and Zhang, J. (2021). The diagnostic value of metagenomic next–generation sequencing in lower respiratory tract infection. Front. Cell. Infect. Microbiol. 11:694756. doi: 10.3389/fcimb.2021.694756
Zhou, H., Larkin, P. M. K., Zhao, D., Ma, Q., Yao, Y., Wu, X., et al. (2021). Clinical impact of metagenomic next-generation sequencing of bronchoalveolar lavage in the diagnosis and management of pneumonia: a multicenter prospective observational study. J. Mol. Diagn. 23, 1259–1268. doi: 10.1016/j.jmoldx.2021.06.007
Keywords: metagenomics, sepsis, diagnostics, meningitis, next-generation sequencing
Citation: Batool M and Galloway-Peña J (2023) Clinical metagenomics—challenges and future prospects. Front. Microbiol. 14:1186424. doi: 10.3389/fmicb.2023.1186424
Edited by:
Axel Cloeckaert, Institut National de recherche pour l’agriculture, l’alimentation et l’environnement (INRAE), FranceReviewed by:
Madjid Morsli, Aix Marseille Université, FranceVladimir Lazarevic, Hôpitaux Universitaires de Genève (HUG), Switzerland
Jessica E. Manning, National Institute of Allergy and Infectious Diseases (NIH), United States
Copyright © 2023 Batool and Galloway-Peña. This is an open-access article distributed under the terms of the Creative Commons Attribution License (CC BY). The use, distribution or reproduction in other forums is permitted, provided the original author(s) and the copyright owner(s) are credited and that the original publication in this journal is cited, in accordance with accepted academic practice. No use, distribution or reproduction is permitted which does not comply with these terms.
*Correspondence: Jessica Galloway-Peña, jgallowaypena@cvm.tamu.edu