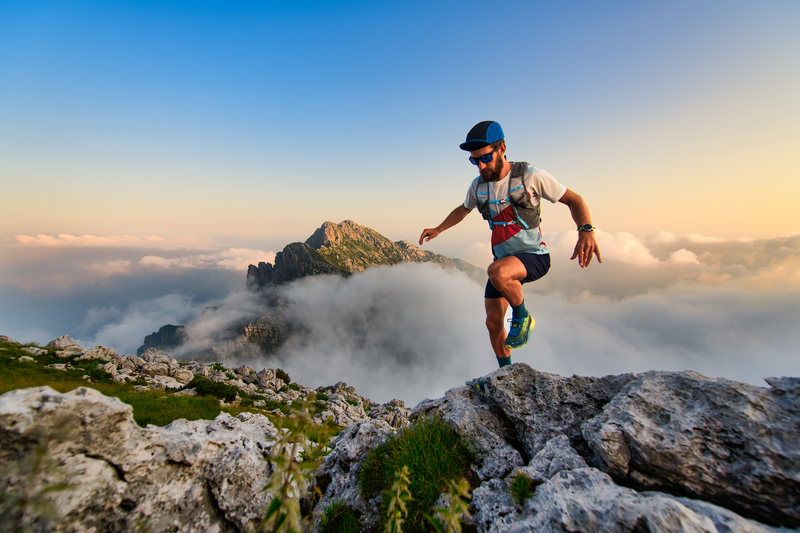
94% of researchers rate our articles as excellent or good
Learn more about the work of our research integrity team to safeguard the quality of each article we publish.
Find out more
ORIGINAL RESEARCH article
Front. Microbiol. , 13 December 2022
Sec. Microbe and Virus Interactions with Plants
Volume 13 - 2022 | https://doi.org/10.3389/fmicb.2022.986519
This article is part of the Research Topic Emerging Frontiers of Microbial Functions in Sustainable Agriculture View all 13 articles
Fungal communities in agricultural soils are assumed to be affected by climate, weather, and anthropogenic activities, and magnitude of their effect depends on the agricultural activities. Therefore, a study was conducted to investigate the impact of the portfolio of management practices on fungal communities and soil physical–chemical properties. The study comprised different climate-smart agriculture (CSA)-based management scenarios (Sc) established on the principles of conservation agriculture (CA), namely, ScI is conventional tillage-based rice–wheat rotation, ScII is partial CA-based rice–wheat–mungbean, ScIII is partial CSA-based rice–wheat–mungbean, ScIV is partial CSA-based maize–wheat–mungbean, and ScV and ScVI are CSA-based scenarios and similar to ScIII and ScIV, respectively, except for fertigation method. All the scenarios were flood irrigated except the ScV and ScVI where water and nitrogen were given through subsurface drip irrigation. Soils of these scenarios were collected from 0 to 15 cm depth and analyzed by Illumina paired-end sequencing of Internal Transcribed Spacer regions (ITS1 and ITS2) for the study of fungal community composition. Analysis of 5 million processed sequences showed a higher Shannon diversity index of 1.47 times and a Simpson index of 1.12 times in maize-based CSA scenarios (ScIV and ScVI) compared with rice-based CSA scenarios (ScIII and ScV). Seven phyla were present in all the scenarios, where Ascomycota was the most abundant phyla and it was followed by Basidiomycota and Zygomycota. Ascomycota was found more abundant in rice-based CSA scenarios as compared to maize-based CSA scenarios. Soil organic carbon and nitrogen were found to be 1.62 and 1.25 times higher in CSA scenarios compared with other scenarios. Bulk density was found highest in farmers' practice (Sc1); however, mean weight diameter and water-stable aggregates were found lowest in ScI. Soil physical, chemical, and biological properties were found better under CSA-based practices, which also increased the wheat grain yield by 12.5% and system yield by 18.8%. These results indicate that bundling/layering of smart agricultural practices over farmers' practices has tremendous effects on soil properties, and hence play an important role in sustaining soil quality/health.
Rice–wheat (RW) system is the dominant cropping system of the Indo-Gangetic Plains of South Asia, spreading over a 13.5 M ha area (Gupta and Seth, 2007). In this system, farmers follow conventional agricultural practices, such as repeated tillage, open field residue burning, indiscreet use of fertilizers, and irrigation waters, which lead to soil health deterioration, groundwater depletion, and environmental pollution (Jat et al., 2021a). In the 21st century, climate change with extreme weather events is likely to be the most serious issue being faced by mankind as per the IPCC predictions (IPCC, 2022). To adapt to extreme events and to manage natural resources, climate-smart agriculture (CSA)-based management practices on the principles of conservation agriculture (CA) (zero tillage, residue retention, and crop rotation) and mitigation co-benefits may prove an excellent option for conventional agriculture to maintain the sustainability of soil and cropping system. In CSA practices, the conventional management practices switch over to resource-conserving management practices by layering with zero/no-tillage, residue retention/incorporation, crop diversification, precise irrigation water, and nutrient management practices to sustain soil and farm productivity and environmental quality (Choudhary et al., 2020). In CSA, residues are managed through retention or incorporation, which are burnt by the farmers under conventional management practices. Crop residues provide an ambient environment for soil fungi, but their density and diversity depend upon the type of residue served. Different types of crop rotations provide different types of root exudates, which influence microbial community compositions (Huang et al., 2014; Jiang et al., 2016). Crop rotation and tillage practices disturb soils and influence the distribution of microbes in the soil layer (Orrù et al., 2021). Fungi play a great role in agricultural practices in nutrient cycling, transformation, and availability.
Soil is the base of all civilizations and mankind, therefore, its functions and structure, directly and indirectly, influence food security. Soil functions are governed by climatic, edaphic, and anthropogenic activities. Soil properties and composition of the soil microbiota are directly interrelated with each other (Wakelin et al., 2008; Bender et al., 2016). Soil microbial communities are essential to biogeochemical cycles, and especially fungi play an important role in the biodegradation of organic matter (Rineau et al., 2012; Qiu et al., 2018). They dominate the microbial biomass in soil habitats (Joergensen and Wichern, 2008) and contribute to nutrient cycling (Stromberger, 2005). Soils have dynamic environments and the microorganisms that live in these soil habitats respond to the changing soil conditions. It has been found that more genera and species of fungi exist in the soil than in any other environment (Nagmani et al., 2006). Fungi are the primary decomposers in soils and secrete various enzymes, such as cellulases, laccases, and xylanases that break down lignocelluloses into simple sugars (Maza et al., 2014; Choudhary et al., 2016). A comprehensive understanding of the fungal community in agricultural soils provides the path to study their roles in the soil ecosystem. The relationship between the biodiversity of soil fungi and ecosystem function is an important issue, particularly in the concern of global climate change and human alteration of ecosystem processes. Fungi play an important role in soil formation, structure, and fertility by contributing to the nutrient cycle and maintenance of the ecosystem (Stromberger, 2005; Hoorman, 2011). Agricultural practices that impact soil conditions can significantly alter soil microbial community composition (Choudhary et al., 2018a,b). The differences in microbial community composition are mainly been attributed to differences in the soil properties (physical and chemical), which are linked to agricultural management practices (IPCC, 2022). Some studies reported the effects of different tillage practices on soil chemical and physical properties and microbial activities, and they reported better soil properties under zero tillage (ZT) and residue retention practices (Choudhary et al., 2018c,d). Agricultural management practices have an impact on different parameters of soil. Under CA practices, better soil physical properties such as lower bulk density (Li et al., 2019), enhanced saturated hydraulic conductivity (Patra et al., 2019), higher infiltration rate (Jat et al., 2018), and increased water-stable aggregates (Bhattacharyya et al., 2012; Jat et al., 2019a) were reported than conventional management practices in cereal-based systems. Increased nutrient availability (Zahid et al., 2020), soil organic carbon (Bera et al., 2017), and nitrogen (Bhattacharyya et al., 2018) were favored under no-tillage/ZT and residue management-based practices. In the western Indo-Gangetic Plains, higher soil biological properties and soil health were reported with CA-based management practices (Choudhary et al., 2018c,d; Sharma et al., 2021).
In any agriculture system, different soil processes depend on microbial community compositions. The diverse nature of fungal communities makes them an important factor for sustainable agriculture in the changing scenarios of climate change (Yadav et al., 2020). The impact of different agricultural management regimes on fungal community composition gained rising interest, although up to date, only a few studies were dedicated to determining the effects of tillage, fertilization, and crop rotation on microbial diversity in cereal systems (Sharma-Poudyal et al., 2017; Choudhary et al., 2018b; Piazza et al., 2019). To date, some studies have applied next-generation sequencing techniques in South Asia to investigate the influence of different tillage regimes and types of crop residue management on soil fungal communities (Choudhary et al., 2018a,b). Our study focused on the changes in the fungal communities under the layering of different management practices, such as soil tillage (conventional vs. zero tillage), residue management (without residue vs. residue retention), crop rotation (rice–wheat vs. maize–wheat), and irrigation (border vs. subsurface drip). The ultimate objective was to identify the impact of layering of management practices on the composition of soil fungal communities.
A 9-year (2009–2018) long-term production scale fixed plot experiment was conducted at the Indian Council of Agricultural Research, Central Soil Salinity Research Institute (29.42°N latitude, 76.57°E longitude, and at an elevation of 243 m.a.s.l.), Karnal, India. The soil of the experimental field is silty loam in texture and falls under the Typic Natrustalf category (Soil Survey Division Staff, 1993). The experiment included six cereal-based scenarios varied with residue, tillage, crop, irrigation, and nutrient supply (Figure 1). The treatments are described as scenarios because they are varied in multiple indicators, namely, tillage, crop establishment, residue management, irrigation management, system intensification, etc. Initially, the experiment started with four scenarios, and later on, in 2016, two more scenarios (ScV and ScVI) were added (Choudhary et al., 2020; Jat et al., 2021b) with minor changes in ScIII and ScIV, and only irrigation management was precise (subsurface drip irrigation was used instead of border irrigation). The details of all the scenarios along with their management practices are presented in Table 1. Scenario III and ScIV were based on the principles of CA practices, so they were described as partial CSA. However, in ScV and ScVI, in addition to ScIII and ScIV, irrigation water and N were precisely managed using subsurface drip irrigation (SDI) and called full CSA. Scenarios were structured in a randomized complete block design and replicated three times. In ScI (farmers' practice or business as usual), both rice and wheat were established with conventional practice. Rice was planted by manual transplanting with 25–30 days old seedlings in puddled fields and wheat was planted by manual broadcasting in tilled soil under ScI. In ScII, manual transplanting for rice in a random geometry (20 × 15 cm) was done. In other scenarios (zero tillage (ZT) conditions), all the crops (rice, wheat, and mungbean) were planted with a row spacing of 22.5 cm using Happy Seeder with an inclined plate seed metering mechanism. However, maize was seeded by Happy Seeder at a row spacing of 67.5 cm. In the farmers' practice (ScI), all the crop residues of rice and wheat were removed from the ground level. However, in other scenarios, it was managed as per the details presented in Table 1. The total amount of crop residue in different scenarios ranged from 100.5 to 119.25 Mg ha−1 in 9 years of study (Table 1). Maize-based scenario received the highest (~119 Mg ha−1) amount of crop residue, while the rice was based on the range of 100–105 Mg ha−1. The NPK dose (nitrogen, phosphorus, and potash) was given as per the recommendation of CCS Haryana Agricultural University for all the crops. In the subsurface drip-irrigated scenario (ScV and ScVI), 80% of the total N as urea (minus N added through DAP and NPK complex) was applied through irrigation (fertigation). In rice, maize, and wheat, irrigation was applied based on soil moisture potential (SMP) using a tensiometer.
Figure 1. Different cereal-based management scenarios at CSSRI-CIMMYT research platform, Karnal, Harayana, India.
The soil samples were collected from all six scenarios (three from each scenario) at 0–15 cm soil depth after harvesting of wheat crop in May 2018. The soil surface was cleaned by removing crop residues and samples were taken randomly aseptically using an auger. Collected soil samples (18 samples) were sieved with 2 mm mesh to eliminate large soil aggregates and plant roots. Samples were divided into three parts, one part was immediately transferred to the laboratory, stored at −20°C until DNA was extracted, and the second part is stored in the refrigerator at 4°C for further analysis of biological properties. The third part was air-dried, ground, and stored in glass containers for chemical and physical analysis.
From the soil samples, DNA was extracted by MO BIO's PowerSoil® DNA Isolation Kit as per the instructions of the manufacturer. The quantity and quality of DNA were measured by Nanodrop spectrophotometer (Thermo Fisher Scientific, USA) and agarose gel electrophoresis, respectively. ITS1 and ITS2 sequencing libraries were constructed by a two-step PCR-based workflow. For round one PCR, using a template of 10–100 ng metagenomic DNA, ITS1 and ITS2 regions were amplified using region-specific proprietary primers developed at Genotypic Technology Pvt. Ltd., Bangalore, India. The protocol also includes overhang adapter sequences that were appended to the primer pair sequences for compatibility with Illumina index and sequencing adapters. PCR was carried out for 26 cycles (hot-start 95°C/3 min, denaturation 95°C/30 s, annealing 55°C/30 s, extension 68°C/1 min, elongation 68°C/5 min, and infinite hold at 4°C) using 0.5 μM primers. The resultant amplicons were analyzed on 1.5% agarose gel whereby the desired amplicons were observed to be of sizes ~350–450 bp for ITS1 and ~450–500 bp for ITS2. For the second round of PCR, 1 μl of 1:2 diluted round one PCR amplicons were taken and amplified for 10 cycles to add Illumina sequencing barcoded adaptors (Nextera XT v2 Index Kit, Illumina, USA). Second round PCR amplicons (sequencing libraries) were analyzed on 1.5% agarose gel, then purified using Ampure XP magnetic beads (Beckman Coulter, USA), and concentrations were measured using Qubit dsDNA HS assay (Thermo Fisher Scientific, USA). The ITS1 and ITS2 amplicons were generated separately by individual PCRs and libraries were constructed off these amplicons individually. The sample libraries were normalized based on the qubit concentrations, then multiplexed on the MiSeq flowcell, and sequenced using a 300PE read length chemistry.
The forward and reverse primers (White et al., 1990; Fujita et al., 2001) were as follows:
ITS1_Forward TCCGTAGGTGAACCTGCGG.
ITS1_Reverse GCTGCGTTCTTCATCGATGC.
ITS2_Forward GCATCGATGAAGAACGCAGC.
ITS2_Reverse TCCTCCGCTTATTGATATGC.
We have adapted our primer design based on fungal rDNA sequencing literature targeting the conserved regions of 5.8S rDNA and 28S rDNA. ITS1 primers were used to amplify the intervening 5.8S rDNA and the adjacent ITS1 region. ITS2 primers were used to amplify a longer region across the 5.8S rDNA through the intervening ITS2 region and the large 28S subunit.
The organic carbon (OC) content of the soils was determined using the wet oxidation method (Walkley and Black, 1934). The available nitrogen (N) in soil was determined by the alkaline permanganate method of Subbiah and Asija (1956), available phosphorus (P) by the ascorbic acid reductant method of Olsen et al. (1954), and available potassium (K) by flame photometer using neutral 1N ammonium acetate extractant as described by Jackson (1973). Mean weight diameter (MWD) and water-stable aggregates (WSA) were determined following the method of Jat et al. (2019b). Soil bulk density (BD) was measured by core sampler method (Blake and Hartge, 1986).
At maturity, crops were harvested manually for grain and straw yields according to residue management protocols. Grain yield was expressed as Mg ha−1 at 14, 12, and 14% grain moisture content for rice, wheat, and maize, respectively. Grain yields of maize, rice, wheat, and mungbean were converted to the rice equivalent yield (REY) by using Equation 1.
where, MSP, Minimum support price of Govt. of India; INR, Indian Rupee.
The Illumina paired-end reads were de-multiplexed using Bc12fastq software v2.20 and FastQ files were generated based on the unique dual barcode sequences. The sequencing quality was assessed using FastQC v0.11.8 software (Andrews, 2010). Raw reads with primer sequence were processed using the Cutadapt tool (Martin, 2011). The adapter sequences were trimmed and bases above Q30 were considered, and low-quality bases were filtered off during read preprocessing and used for downstream analysis. The high-quality R1 and R2 reads were filtered and stitched using Fastq-join (Aronesty, 2013). ITS1 and ITS2 sequences were merged and considered for further analysis using the QIIME pipeline (Caporaso et al., 2010). The query sequences were clustered using the UCLUST (Edgar, 2010) method against the reference UNITE (Abarenkov et al., 2010) database (ver7) with 97% similarity. The reads, which did not hit the reference database, were then clustered using the de novo method where reads are clustered against one another without any external reference sequence collection. Taxonomy was assigned through the BLAST method (Altschul et al., 1990), with an e-value of 0.001. The BIOM file generated was taken ahead for further advanced analysis and visualization. The rarefied biom at a depth of 177,000 for merged ITS sequences/samples was used for the calculation of alpha diversity indices using various metrics. Sequences from all six agricultural management scenarios have been submitted to NCBI with the Bio project: PRJNA563827.
Biplot and principal component analysis (PCA) were done with JMP 14.1 software. The results were submitted to PCA to determine the common relationships between fungi classes, soil physical properties, and SOC, N, P, and K contents of the soil. The crop yield data were analyzed by the analysis of variance (ANOVA) technique for a completely randomized block design using SAS 9.1 software (SAS Institute, Cary, NC). Differences among treatment means were compared using Tukey's HSD test at the 5% probability level.
From the 18 soil samples, three of each from six scenarios, a total of 12,468,774 paired-end reads were obtained after processing of sequences, and a total of 5,033,723 sequences were obtained. Fungal diversity was calculated by different indices, such as Shannon's diversity index, Simpson's diversity index, and Chao1. Diversity indices were found to be significantly affected by agricultural management systems, as Shannon's diversity index, Simpson's diversity index, Chao1, and observed species/OTU were varied in scenarios (Table 2). Shannon diversity index was found to be 1.47 times higher in maize-based scenarios (ScIV and ScVI) as compared to rice-based CSA scenarios (ScIII and ScV). A similar pattern was observed for the Simpson index, as it was 1.12 times higher in maize-based scenarios compared with rice-based CSA scenarios. Shannon's diversity index (6.80 ± 0.551), Simpson's diversity index (0.95 ± 0.016), Chao1 (3,223 ± 256), and observed species/OTU (2,874 ± 229) were found highest in the partial CSA scenario (ScII). Our results showed ahigher Shannon diversity index (1.55 times), Simpson index (1.18 times), Chao1 (1.41 times), and observed species (1.49) in ScII than in ScIII.
A total of seven fungal phyla were present in all six scenarios. Ascomycota is the dominating phyla followed by Basidiomycota and Zygomycota (Figure 2). A higher abundance of Ascomycota was observed in rice-based CSA scenarios (ScIII and ScV) as compared to maize-based scenarios (ScIV and ScVI). In rice-based scenarios (ScI, ScII, ScIII, and ScV), Ascomycota was followed by Basidiomycota but in maize-based scenarios (ScIV and ScVI), it was followed by Zygomycota. Higher Ascomycota (1.31 times) was found in ScIII than ScII, whereas, Glomeromycota was found 7 times higher in ScII than ScIII and 1.66 times higher in ScIV than ScVI. Chytridiomycota was found non-significantly higher in maize-based scenarios as compared to rice-based scenarios. In all six scenarios, a total of 48 classes were observed. Sordariomycetes, an unidentified class of Ascomycota, and Dothideomycetes are among the dominating classes (Table 3). The highest abundance was observed for Sordariomycetes (25.45–43.47%), except for ScV in which an unidentified class of Ascomycota (39.30%) was found in the highest abundance. The abundance of Sordariomycetes was found at par among all the scenarios. The relative abundance of the unidentified class of Ascomycota was found to be 7.8 times higher in CSA-based rice systems compared with maize systems. Although the difference in class Agaricomycetes was non-significant among scenarios, it was two times higher in maize-based scenarios (7.72%) as compared to rice-based scenarios (3.83%). The abundance of Mortierellomycotina_cls_Incertae_sedis class of Zygomycota was recorded 8.4 times higher in maize-based scenarios than in rice-based scenarios. Ascomycota unidentified class was respectively higher in ScIII (2.72 times) than that in ScII and 1.42 times higher in ScV than ScIII. Pezizomycetes class was 6.84 times, Glomeromycetes was 6.97 times, Saccharomycetes was 3.75 times, and Blastocladiomycetes was 8.5 times higher in ScII than that in ScIII. At the level of order, Hypocreales was the dominating order followed by Pleosporales, Pezizales, and Sordariales (Supplementary Table 1).
Figure 2. Abundance of phyla in different crop management-based scenarios. a,b,cMeans followed by similar lowercase letters are not significantly different at 0.05 level of probability using Tukey's HSD test.
Differences among the scenarios were recorded for soil chemical and physical parameters. Soil organic carbon (SOC) and available nitrogen (N) were 1.43 and 1.34 times higher in CSA-based scenarios (ScII, ScIII, ScIV, ScV, and ScVI) compared with farmers' practice (ScI). Available phosphorus and potassium were found to be 1.65 and 1.60 times higher, respectively, in the soil of CSA-based scenarios than that in the CT scenario/farmers' practice. All four SOC (5.7 ± 0.01 g kg−1), N (119.01 ± 0.58 kg ha−1), P (16.1 ± kg ha−1), and K (137 ± kg ha−1) were lowest with farmers' practice (ScI). Bulk density was observed highest in ScI (1.58) followed by ScII (1.52) (Table 4). It was lower in rice-based CSA scenarios (1.36) as compared to maize-based scenarios (1.445). The highest mean weight diameter was found in ScIII (2.95 mm) and ScV (2.70 mm) and the lowest was observed in ScI (1.45 mm). Water-stable aggregate was non-significant in full/CSA scenarios but significantly higher in ScII (68.3%) and farmers' practice (62.7%) than that in other scenarios.
The total residue load in ScI, ScII, ScIII, ScIV, ScV, and ScVI was 105, 101, 119, 101, and 119 Mg ha−1, respectively. During the year 2018, a higher yield of wheat was recorded with all the CSA-based scenarios (ScIII–ScVI) and ranged from 6.58 to 6.79 Mg ha−1. The lowest yield was recorded with farmers' practice, that is, 5.88 Mg ha−1. A similar trend was also observed for the system yield in all the scenarios (Table 4). The higher system yield was recorded with ScVI (16.85 Mg ha−1) followed by ScII (16.19 Mg ha−1) and ScIV (16.04 Mg ha−1). The lowest yield was found with farmers' practice or ScI (13.33 Mg ha−1).
The results from the principal component analysis provided evidence that the different scenarios were diverse regarding the fungi classes, soil physical properties, and SOC, N, P, and K contents of the soil (Figure 3). According to the factor loadings, the first PC, which explains 48.8% of the total variance, had higher positive correlations with Agaricomycetes, Saccharomycetes, Blastocladiomycetes, and bulk density (BD), and a negative correlation with SOC, N, WSA, and MWD, while the second PC, which explains 16.76% of the total variance, was strongly correlated with Chytridiomycetes, Mortierellomycotina_cls_Incertae_sedis, and Pezizomycotina_cls_Incertae_sedis. The third PC explains 12.6% of the total variance and is correlated with Dothideomycetes (Supplementary Table 2). The PCA biplot in Figure 3 shows both PC scores of samples and the loadings of variables.
Figure 3. Biplot obtained from principal components analysis based on the correlation matrix, showing the two first principal components (explaining 48.8 and 28.6%, respectively). Each point represents scenarios (ScI to ScVI), loadings represent fungi classes, soil physical properties, and SOC, N, P, and K contents of the soil.
Significant correlations were observed among the fungi classes, soil physical properties, and SOC, N, P, and K contents of soil irrespective of scenarios (Table 5). An unidentified class of fungi was significantly negatively correlated to Pezizomycetes, Chytridiomycetes, and Pezizomycotina_cls_Incertae_sedis. Dothideomycetes was significantly negatively correlated to WSA and MWD. Agaricomycetes was significantly positively correlated to Pezizomycetes, Saccharomycetes, and Blastocladiomycetes, and negatively correlated to the available N content of the soil. Eurotiomycetes was significantly positively correlated to Pezizomycetes, Tremellomycetes, and Glomeromycetes. Pezizomycetes was significantly positively correlated with Glomeromycetes, Saccharomycetes, and Chytridiomycetes. Glomeromycetes was positively significantly correlated to Chytridiomycetes. Saccharomycetes was positively correlated to Mucoromycotina_cls_Incertae_sedis and Blastocladiomycetes, and negatively correlated to N. Mucoromycotina_cls_Incertae_sedis, which was positively correlated to Chytridiomycota;unidentified. Chytridiomycetes was positively correlated to Mortierellomycotina_cls_Incertae_sedis, Pezizomycotina_cls_Incertae_sedis, and WSA. Mortierellomycotina_cls_Incertae_sedis was significantly positively correlated to Chytridiomycota;unidentified and Pezizomycotina_cls_Incertae_sedis, and WSA. Chytridiomycota;unidentified was significantly positively correlated to Pezizomycotina_cls_Incertae_sedis. Blastocladiomycetes was positively correlated to BD and negatively correlated to the C, N, and P contents of the soil. WSA was significantly positively correlated to MWD and C and K contents. MWD was positively correlated to the C and K contents of the soil. BD was significantly negatively correlated to C, N, P, and K contents of the soil. Soil carbon was positively correlated to the N, P, and K contents of the soil. The available N was significantly positively correlated with available P and K content of the soil. The available P was significantly positively correlated to the available K content of the soil.
Table 5. Correlation between fungal class abundance, soil chemical and physical properties in different management scenarios.
Conservation agriculture-based practices showed higher Shannon and Simpson diversity indices in maize-based scenarios as compared to rice-based scenarios due to the inclusion of maize in the crop rotation system. Although sampling of soil was done in all scenarios after harvesting the common crop among them (wheat), the residue and root system of the previous crop can impact soil properties. Fungal diversity is directly or indirectly affected by plant and soil properties (Yang et al., 2017). Maize has a different root system than rice (tap root instead of fibrous root system); the amount and composition of root exudates vary with crops/plants, which can influence the microbial diversity of soil (Sasse et al., 2018). Moreover, the quantity and quality of residue also play a critical role in the microbial properties of soil (Moore et al., 2000). Maize residue provides higher amounts of lignocellulosic material, which can harbor more types of residue-decomposing fungi, hence showing higher fungal diversity than rice-based CA practices. Similar results were reported in our previous study (Choudhary et al., 2018b) based on 5 years of continuous CA-based practices. This combination of residue retention and incorporation in one calendar year may be the reason for the highest diversity indices in this scenario II instead of only crop residue retention in a similar cropping system. Through the tillage practices, crop residues mix well in the soil and alter the microclimate and distribution of nutrients, which resulted in higher fungal diversity under partial CA practice than that in other practices where only crop residues were retained.
Ascomycota, found as a dominating phylum in all management scenarios, is well known as one of the most abundant fungal phyla of soils globally (Choudhary et al., 2018a,b; Egidi et al., 2019; Maguire et al., 2020). Ascomycota is the largest phylum of fungi and is ubiquitous in soil (Money, 2016; Egidi et al., 2019). Most Ascomycota members are saprophytic and the main decomposers of plant residue in the soil, hence they dominate the fungal community composition in soils. In our study, a higher abundance of Ascomycota was observed in rice-based CA scenarios as compared to maize-based scenarios due to the difference between residues. Since soil samples were taken after the harvesting of the wheat crop (common crop in all scenarios) and by that time ample duration (5 months for maize and rice residue) has been received by the residue to decompose. Initially, the residue decomposes fast due to the presence of water-soluble compounds in the residues, which are easily decomposable (Diochon et al., 2016), but in later stages, decomposition of more recalcitrant compounds such as lignin and cellulose takes place. The composition of residue and decomposition, which varies with crop type, are the main factors in harboring different types of microbial communities. This might also lead to the high abundance of Basidiomycota in rice-based scenarios and Zygomycota in maize-based scenarios. The type of crop, size, and type of residue and tillage practices vary in different management systems leading to variation in soil moisture also. Chytridiomycota are reported in aquatic ecosystems as well as in terrestrial ecosystems (Gleason et al., 2004), but in our study, these were found in different management systems and favored in maize-based scenarios. Not only residue type and decomposition duration but also variation in moisture played an important role in deciding the abundance of different groups of fungi (Miura et al., 2015). Sordariomycetes and Eurotiomycetes were among the dominating classes in all the scenarios, which were previously reported in soils of conservation agricultural practices (Wang et al., 2016; Choudhary et al., 2018b). Sordariomycetes is one of the largest classes of Ascomycota, which includes pathogens, endophytes, and saprobes. Members of the Sordariomycetes are ubiquitous and cosmopolitan. Saprobic Sordariomycetes have the potential to produce cellulolytic enzymes, and its member Chaetomium is a well-known cellulolytic organism responsible for the destruction of paper and fabrics (Zhang et al., 2006). Members of Sordariomycetes class play an important role in the decomposition and nutrient cycling of plant residues. Dothideomycetes also represents one of the largest and dominant classes of the phylum Ascomycota, which is mostly found as endophytes or saprobes (Kirk et al., 2008). Members of this class can cause disease in almost every crop plant including maize and rice (Goodwin, 2013). Members of this class are also known to degrade cellulose and other complex carbohydrates of dead or partially digested plant organic matter (Hyde et al., 2013). One unidentified class of Ascomycota is also among the most dominating classes found in scenarios. Although its identification is not known to class level, these unidentified and unknown fungal groups may be important to community compositions. Many of these previously unknown groups are later found as major components of communities of interest (Rosling et al., 2011). The abundance of some classes found to be high in maize-based scenarios can be correlated with the difference in crop type, root system, and residue depending on plant species, and biochemical characteristics of plant materials differ considerably (Xu et al., 2006). The effect of tillage practices on the abundance of fungi was observed at both phyla and class levels. Some phyla and classes were found higher in ZT, which indicates that the hyphal network in CT practices got disturbed due to intensive tillage practices, which resulted in limited growth. Whereas, the abundance of some phyla/classes was reported higher in CT than ZT, which might be due to the higher growth rate of broken mycelia of these phyla/classes under CT than that in other management practices. Wang et al. (2017) concluded that tillage influenced the distribution of fungal communities with more stable communities under ZT conditions. Irrigation management and soil moisture had a great impact on fungal communities in arid and semi-arid areas where water availability is limited (Vargas-Gastélum et al., 2015; Deng et al., 2022). But in our study, irrigation was given to all the scenarios based on the tensiometer to maintain the soil moisture as per crop requirement to minimize the effect of irrigation management on fungal community composition.
Crop residue retention enriches soils with a significant amount of nutrients (Jat et al., 2018, 2019b; Lohan et al., 2018). It is a major reason behind high SOC, N, P, and K contents in residue retention scenarios. Moreover, under zero tillage practices, the decomposition of residue also does not disturb due to the non-disturbance of soil. Residue not only harbors different types of microfauna but also provides nutrients to them, which in turn release different types of enzymes such as phosphatase, glucosidase, dehydrogenase, etc., responsible for the conversion of unavailable to the available forms of nutrients (Maguire et al., 2020). The activity of different enzymes is reported to be more in CA/CSA-based practices and have a higher soil quality index over farmers' practices (Choudhary et al., 2018c,d). CA practices not only improve nutrient availability but also improve overall soil quality (Jat et al., 2021a). It was observed by many researchers that tillage and residue had a positive effect on bulk density (Gathala et al., 2011). In farmers' practice, the residue is either burnt or removed, which resulted in more compactness in soils. Lower BD in the rice-based system as compared to maize-based CA scenarios might be due to higher conversion of crop residue carbon to SOC leading to good soil structure as evidenced through higher WSA and MWD (Mandal et al., 2007).
The results clearly showed the differential benefits of CSA-based management practices in both rice- and maize-based systems. The highest wheat and systems yield (rice equivalent yield basis) were recorded with all CSA-based scenarios. Tillage and cropping systems, crop residue management, soil type, and climate control the magnitude at which SOC affects crop yields (Blanco-Canqui et al., 2013). About 0.1% increase in SOC concentration enhanced wheat yield by 0.04 Mg ha−1 at 0 kg N ha−1 (Blanco-Canqui et al., 2012). A direct relationship between SOM stocks and crop productivity was observed by Oldfield et al. (2018) through variables for soils and amendments. In a global meta-analysis, zero tillage (ZT) when combined with residue retention and crop rotation can produce equivalent or greater yields than conventional tillage, by minimizing its negative impacts. Higher system productivity in CSA-based scenarios might be due to the integration of mungbean (Gathala et al., 2013), less terminal heat effects on the wheat crop (Gathala et al., 2013; Sharma et al., 2015), higher carbon mineralization (Kirk et al., 2008; Datta et al., 2019) with better nutrient availability (Sasse et al., 2018), and improved soil biological properties (Olsen et al., 1954; Choudhary et al., 2018b,d).
Some classes are negatively correlated while some are positively correlated with each other, and it is due to the ecological relationship between them, which may influence each other's position in the community. The groups, which have similar modes of nutrition, compete for the same biochemical fraction of the crop residues, and this might show a negative correlation. Biochemical qualities of residue are the main drivers in the composition of fungal communities. Specific function during the decomposition of residue linked to specific taxa (Rezgui et al., 2021). In residue decomposition, the end product of one class/group may be acted as food for another group. In such a situation, these may be positively correlated as they do not have competition for food. Due to the preferences of microbes for specific residue compounds, organic matter decomposition and transformation under different agricultural managements influence the abundances of the specific microbial taxa (Zheng et al., 2022). Different type of residue management over the years strongly influences the soil's microbial, chemical, and physical properties. In this 9-year-old experiment, the residue is accumulated over the years in all CSA scenarios, and according to Fontaine et al. (2011), the soil microbes can decompose old recalcitrant soil organic matter by using fresh carbon as a source of energy, which can lead to a different type of distribution of soil fungal taxa in these scenarios. A significant positive correlation between SOC and MWD and WSA was due to good soil structure rendered by higher SOC in CA-based scenarios. Higher MWD and WSA were found under crop residue retention scenarios in cereal systems (Jat et al., 2019a). There is a strong link between microbial communities and SOC (Zheng et al., 2022), which is influenced by the different tillage and crop establishment practices. ZT improved C fractions over CT and these C pools can directly impact the activities of microbes in soils (Rakesh et al., 2021). Soil properties and soil fungal communities also showed different types and magnitudes of relationships because of robust associations between them (Yang et al., 2017). Both the diversity and composition of the fungi community directly correlated with the soil properties and indirectly correlated with management practices (Li and Zhang, 2022).
Agricultural practices in isolation do not prove good for soil and crop sustainability in cereal systems. Crop management practices, such as tillage, residue management, nutrient, water management, and crop diversification have a strong influence on the physical, chemical, and biological properties of soil. Therefore, the combination of different management practices led to differences in fungal community composition and soil chemical properties under climate-smart agriculture (CSA) systems. Maize-based scenarios are more diverse in terms of fungal communities than rice-based CSA scenarios. Rice-based scenarios with CSA practices showed a more abundance of Ascomycota phyla over the maize-based CSA scenarios. Ascomycota is followed by Basidiomycota in rice-based scenarios and Zygomycota in maize-based scenarios. CSA-based scenarios improved the soil chemical properties (organic carbon, nitrogen, and potassium) across the different cereal-based CSA scenarios compared with other scenarios (CT and partial CSA). Soil physical properties such as bulk density, mean weight diameter, and water-stable aggregate were also improved under CSA scenarios. Improved soil properties under CSA-based practices resulted in improved crop and system yield. Results indicate that the bundling/layering of smart agricultural practices not only influences the soil properties but also played an important role in deciding the microbial community composition.
The datasets presented in this study can be found in online repositories. The names of the repository/repositories and accession number(s) can be found at: https://www.ncbi.nlm.nih.gov/, PRJNA563827.
The experiment was conducted after taking proper approval from the Institute Research Committee of ICAR-CSSRI, Karnal. Guidelines of the ICAR-CSSRI were followed for taking data on crops/weeds/plants.
HJ, PS, and MJ conceptualized and designed the experiment. MJ and PS did the funding acquisition. HJ and PS did the management of the experiment. HJ and MC conducted the research. MC wrote the manuscript. HJ provided critical comments on the manuscript. All authors read and approved the final manuscript.
The authors acknowledge the support received from the ICAR-CSSRI, Karnal (CSSRI PME cell reference no. Research Article/48/2021), and CIMMYT and funding from the Bill & Melinda Gates Foundation [OPP1052535] through Cereal System Initiative for South Asia (CSISA) project. The research was financed and supported by CGIAR Research Program (CRPs) on Climate Change, Agriculture and Food Security (CCAFS), and Wheat Agri-Food Systems (WHEAT). We acknowledge the CGIAR Fund Council, Australia (ACIAR), Irish Aid, European Union, and International Fund for Agricultural Development, Netherlands, New Zealand, Switzerland, UK, USAID, and Thailand for funding to the CGIAR Research Program on Climate Change, Agriculture and Food Security (CCAFS).
The authors acknowledged the contribution of Dr. Ashim Datta in the analysis of soil data.
The authors declare that the research was conducted in the absence of any commercial or financial relationships that could be construed as a potential conflict of interest.
All claims expressed in this article are solely those of the authors and do not necessarily represent those of their affiliated organizations, or those of the publisher, the editors and the reviewers. Any product that may be evaluated in this article, or claim that may be made by its manufacturer, is not guaranteed or endorsed by the publisher.
The Supplementary Material for this article can be found online at: https://www.frontiersin.org/articles/10.3389/fmicb.2022.986519/full#supplementary-material
Abarenkov, K., Nilsson, R. H., Larsson, K. H., Alexander, I. J., Eberhardt, U., Erland, S., et al. (2010). The UNITE database for molecular identification of fungi–recent updates and future perspectives. New Phytol. 186, 281–285. doi: 10.1111/j.1469-8137.2009.03160.x
Altschul, S. F., Gish, W., Miller, W., Myers, E. W., and Lipman, D. J. (1990). Basic local alignment search tool. J. Mol. Biol. 215, 403–410. doi: 10.1016/S0022-2836(05)80360-2
Andrews, S. (2010). A Quality Control Tool for High Throughput Sequence Data. Available online at: http://www.bioinformatics.babraham.ac.uk/projects/fastqc (accessed November, 2018).
Aronesty, E. (2013). Comparison of sequencing utility programs. Open Bioinform. J. 7, 1–8. doi: 10.2174/1875036201307010001
Bender, S. F., Wagg, C., and van der Heijden, M. G. (2016). An underground revolution: biodiversity and soil ecological engineering for agricultural sustainability. Trends Ecol. Evol. 31, 440–452. doi: 10.1016/j.tree.2016.02.016
Bera, T., Sharma, S., Thind, H. S., Sidhu, H. S., and Jat, M. L. (2017). Soil biochemical changes at different wheat growth stages in response to conservation agriculture practices in a rice-wheat system of north-western India. Soil Res. 56, 91–104. doi: 10.1071/SR16357
Bhattacharyya, R., Bhatia, A., Das, T. K., Lata, S., Kumar, A., Tomer, R., et al. (2018). Aggregate-associated N and global warming potential of conservation agriculture-based cropping of maize-wheat system in the north-western Indo-Gangetic Plains. Soil Tillage Res. 182, 66–77. doi: 10.1016/j.still.2018.05.002
Bhattacharyya, R., Tuti, M. D., Bisht, J. K., Bhatt, J. C., and Gupta, H. S. (2012). Conservation tillage and fertilization impacts on soil aggregation and carbon pools in the Indian Himalayas under an irrigated rice–wheat rotation. Soil Sci. 177, 218–228. doi: 10.1097/SS.0b013e3182408f1e
Blake, G. R., and Hartge, K. H. (1986). “Bulk density,” in Methods of Soil Analysis, ed A. Klute (Madison, WI: ASA and SSSA), 363–375.
Blanco-Canqui, H., Claassen, M. M., and Presley, D. R. (2012). Summer cover crops fix nitrogen, increase crop yield and improve soil-crop relationships. Agron. J. 104, 137–147. doi: 10.2134/agronj2011.0240
Blanco-Canqui, H., Shapiro, C. A., Wortmann, C. S., Drijber, R. A., Mamo, M., Shaver, T. M., et al. (2013). Soil organic carbon: the value to soil properties. J. Soil Water Conserv. 68, 29A−134A. doi: 10.2489/jswc.68.5.129A
Caporaso, J. G., Kuczynski, J., Stombaugh, J., Bittinger, K., Bushman, F. D., Costello, E. K., et al. (2010). QIIME allows analysis of high-throughput community sequencing data. Nat. Methods 7, 335–336. doi: 10.1038/nmeth.f.303
Choudhary, M., Datta, A., Jat, H. S., Yadav, A. K., Gathala, M. K., Sapkota, T. B., et al. (2018d). Changes in soil biology under conservation agriculture based sustainable intensification of cereal systems in Indo-Gangetic Plains. Geoderma 313, 193–204. doi: 10.1016/j.geoderma.2017.10.041
Choudhary, M., Jat, H. S., Datta, A., Sharma, P. C., Rajashekar, B., and Jat, M. L. (2020). Topsoil bacterial community changes and nutrient dynamics under cereal based climate-smart agri-food systems. Front. Microbiol. 11, 1812. doi: 10.3389/fmicb.2020.01812
Choudhary, M., Jat, H. S., Datta, A., Yadav, A. K., Sapkota, T. B., Mondal, S., et al. (2018c). Sustainable intensification influences soil quality, biota, and productivity in cereal-based agroecosystems. Appl. Soil Ecol. 126, 189–198. doi: 10.1016/j.apsoil.2018.02.027
Choudhary, M., Jat, H. S., Garg, N., and Sharma, P. C. (2018a). Microbial diversity under Conservation Agriculture (CA)-based management scenarios in reclaimed salt-affected soils: metagenomic approach. J. Soil Salinity Water Qual. 10, 87–94.
Choudhary, M., Sharma, P. C., Jat, H. S., McDonald, A., Jat, M. L., Choudhary, S., et al. (2018b). Soil biological properties and fungal diversity under conservation agriculture in Indo-Gangetic Plains of India. J. Soil Sci. Plant Nutr. 18, 1142–1156. doi: 10.4067/S0718-95162018005003201
Choudhary, M., Sharma, P. C., Jat, H. S., Nehra, V., McDonald, A. J., and Garg, N. (2016). Crop residue degradation by fungi isolated from conservation agriculture fields under rice–wheat system of North-West India. Int. J. Recycl. Org. Waste Agric. 5, 349–360. doi: 10.1007/s40093-016-0145-3
Datta, A., Jat, H. S., Yadav, A. K., Choudhary, M., Sharma, P. C., Rai, M., et al. (2019). Carbon mineralization in soil as influenced by crop residue type and placement in an Alfisols of Northwest India. Carbon Mgt. 10, 37–50. doi: 10.1080/17583004.2018.1544830
Deng, Q., Wu, Y., Zhao, X., Qiu, C., Xia, S., Feng, Y., et al. (2022). Influence of different irrigation methods on the alfalfa rhizosphere soil fungal communities in an arid region. PLoS ONE 17, e0268175. doi: 10.1371/journal.pone.0268175
Diochon, A., Gillespie, A. W., Ellert, B. H., Janzen, H. H., and Gregorich, E. G. (2016). Recovery and dynamics of decomposing plant residue in soil: an evaluation of three fractionation methods. Eur. J. Soil Sci. 67, 196–205. doi: 10.1111/ejss.12316
Edgar, R. C. (2010). Search and clustering orders of magnitude faster than BLAST. Bioinformatics 26, 2460–2461. doi: 10.1093/bioinformatics/btq461
Egidi, E., Delgado-Baquerizo, M., Plett, J. M., Wang, J., Eldridge, D. J., Bardgett, R. D., et al. (2019). A few Ascomycota taxa dominate soil fungal communities worldwide. Nat. Commun. 10, 1–9. doi: 10.1038/s41467-019-10373-z
Fontaine, S., Hénault, C., Aamor, A., Bdioui, N., Bloor, J. M. G., Maire, V., et al. (2011). Fungi mediate long term sequestration of carbon and nitrogen in soil through their priming effect. Soil boil. Biochem. 43, 86–96. doi: 10.1016/j.soilbio.2010.09.017
Fujita, S. I., Senda, Y., Nakaguchi, S., and Hashimoto, T. (2001). Multiplex PCR using internal transcribed spacer 1 and 2 regions for rapid detection and identification of yeast strains. J. Clin. Microbiol. 39, 3617–3622. doi: 10.1128/JCM.39.10.3617-3622.2001
Gathala, M. K., Kumar, V., Sharma, P. C., Saharawat, Y. S., Jat, H. S., Singh, M., et al. (2013). Optimizing intensive cereal-based cropping systems addressing current and future drivers of agricultural change in the northwestern Indo-Gangetic Plains of India. Agric. Ecosyst. Environ. 177, 85–97. doi: 10.1016/j.agee.2013.06.002
Gathala, M. K., Ladha, J. K., Kumar, V., Saharawat, Y. S., Kumar, V., Sharma, P. K., et al. (2011). Tillage and crop establishment affects sustainability of South Asian rice–wheat system. Agron. J. 103, 961–971. doi: 10.2134/agronj2010.0394
Gleason, F. H., Letcher, P. M., and McGee, P. A. (2004). Some Chytridiomycota in soil recover from drying and high temperatures. Mycol. Res. 108, 583–589. doi: 10.1017/S0953756204009736
Goodwin, S. B. (2013). Dothideomycetes: plant pathogens, saprobes, and extremophiles. Ecol. Genom. Fungi 20, 117–147. doi: 10.1002/9781118735893.ch6
Gupta, R., and Seth, A. (2007). A review of resource conserving technologies for sustainable management of the rice–wheat cropping systems of the Indo-Gangetic plains (IGP). Crop Prot. 26, 436–447. doi: 10.1016/j.cropro.2006.04.030
Hoorman, J. J. (2011). The Role of Soil Fungus. Factsheet-Agriculture and Natural Resources. Ohio: The Ohio State University Extension.
Huang, X. F., Chaparro, J. M., Reardon, K. F., Zhang, R., Shen, Q., and Vivanco, J. M. (2014). Rhizosphere interactions: root exudates, microbes, and microbialcommunities. Botany 92, 267–275. doi: 10.1139/cjb-2013-0225
Hyde, K. D., Jones, E. B., Liu, J. K., Ariyawansa, H., Boehm, E., Boonmee, S., et al. (2013). Families of dothideomycetes. Fungal Divers 63, 1–313. doi: 10.1007/s13225-013-0263-4
IPCC (2022). “Climate change 2022: impacts, adaptation, and vulnerability,” in Contribution of Working Group II to the Sixth Assessment Report of the Intergovernmental Panel on Climate Change, eds H. O. Pörtner, D. C. Roberts, M. Tignor, E. S. Poloczanska, K. Mintenbeck, A. Alegría, et al. (Cambridge: Cambridge University Press).
Jat, H. S., Datta, A., Choudhary, M., Sharma, P. C., and Jat, M. L. (2021a). Conservation agriculture: factors and drivers of adoption and scalable innovative practices in Indo-Gangetic plains of India: a review. Int. J. Agric. Sustain. 19, 40–55. doi: 10.1080/14735903.2020.1817655
Jat, H. S., Datta, A., Choudhary, M., Sharma, P. C., Yadav, A. K., Choudhary, V., et al. (2019b). Climate Smart Agriculture practices improve soil organic carbon pools, biological properties and crop productivity in cereal-based systems of North-West India. Catena 181, 104059. doi: 10.1016/j.catena.2019.05.005
Jat, H. S., Datta, A., Choudhary, M., Yadav, A. K., Choudhary, V., Sharma, P. C., et al. (2019a). Effects of tillage, crop establishment and diversification on soil organic carbon, aggregation, aggregate associated carbon and productivity in cereal systems of semi-arid Northwest India. Soil Till. Res. 190, 128–138. doi: 10.1016/j.still.2019.03.005
Jat, H. S., Datta, A., Sharma, P. C., Kumar, V., Yadav, A. K., Choudhary, M., et al. (2018). Assessing soil properties and nutrient availability under conservation agriculture practices in a reclaimed sodic soil in cereal-based systems of North-West India. Arch. Agron. Soil Sci. 64, 531–545. doi: 10.1080/03650340.2017.1359415
Jat, H. S., Kumar, V., Kakraliya, S. K., Abdallah, A. M., Datta, A., Choudhary, M., et al. (2021b). Climate-smart agriculture practices influence weed density and diversity in cereal-based agri-food systems of western Indo-Gangetic plains. Sci. Rep. 11, 1–15. doi: 10.1038/s41598-021-95445-1
Jiang, Y., Liang, Y., Li, C., Wang, F., Sui, Y., Suvannang, N., et al. (2016). Crop rotations alter bacterial and fungal diversity in paddy soils across East Asia. Soil Biol. Biochem. 95, 250–261. doi: 10.1016/j.soilbio.2016.01.007
Joergensen, R. G., and Wichern, F. (2008). Quantitative assessment of the fungal contribution to microbial tissue in soil. Soil Biol. Biochem. 40, 2977–2991. doi: 10.1016/j.soilbio.2008.08.017
Kirk, P. M., Cannon, P. F., Minter, D. W., and Stalpers, J. A. (2008). Dictionary of the Fungi, 10th Edn. Wallingford: CABI, 221.
Li, M., and Zhang, X. (2022). Effect of no tillage system on soil fungal community structure of cropland in mollisol: a case study. Front. Microbiol. 13, 2092. doi: 10.3389/fmicb.2022.847691
Li, Y., Li, Z., Cui, S., Jagadamma, S., and Zhang, Q. (2019). Residue retention and minimum tillage improve physical environment of the soil in croplands: a global meta-analysis. Soil Till. Res. 194, 104292. doi: 10.1016/j.still.2019.06.009
Lohan, S. K., Jat, H. S., Yadav, A. K., Sidhu, H. S., Jat, M. L., Choudhary, M., et al. (2018). Burning issues of paddy residue management in north-west states of India. Renew. Sust. Energy Rev. 81, 693–706. doi: 10.1016/j.rser.2017.08.057
Maguire, V. G., Bordenave, C. D., Nieva, A. S., Llames, M. E., Colavolpe, M. B., Gárriz, A., et al. (2020). Soil bacterial and fungal community structure of a rice monoculture and rice-pasture rotation systems. Appl. Soil Ecol. 151, 103535. doi: 10.1016/j.apsoil.2020.103535
Mandal, B., Majumder, B., Bandyopadhyay, P. K., Hazra, G. C., Gangopadhyay, A., Samantaray, R. N., et al. (2007). The potential of cropping systems and soil amendments for carbon sequestration in soils under long-term experiments in subtropical India. Glob. Change Boil 13, 357–369. doi: 10.1111/j.1365-2486.2006.01309.x
Martin, M. (2011). Cutadapt removes adapter sequences from high-throughput sequencing reads. EMBnet. J. 17, 10–12. doi: 10.14806/ej.17.1.200
Maza, M., Pajot, H. F., Amoroso, M. J., and Yasem, M. G. (2014). Post-harvest sugarcane residue degradation by autochthonous fungi. Int. Biodeter. Biodegra. 87, 18–25. doi: 10.1016/j.ibiod.2013.10.020
Miura, T., Niswati, A., Swibawa, I. G., Haryani, S., Gunito, H., Shimano, S., et al. (2015). Diversity of fungi on decomposing leaf litter in a sugarcane plantation and their response to tillage practice and bagasse mulching: implications for management effects on litter decomposition. Microbial. Ecol. 70, 646–658. doi: 10.1007/s00248-015-0620-9
Money, N. P. (2016). “Fungal diversity,” in The Fungi (New York, NY: Academic Press), 1–36. doi: 10.1016/B978-0-12-382034-1.00001-3
Moore, J. M., Klose, S., and Tabatabai, M. A. (2000). Soil microbial biomass carbon and nitrogen as affected by cropping systems. Biol. Fert. Soils 31, 200–210. doi: 10.1007/s003740050646
Nagmani, A., Kunwar, I. K., and Manoharachary, C. (2006). Handbook of Soil Fungi. Published by IK. New Delhi: International Pvt. Ltd., 1–477
Oldfield, E. E., Wood, S. A., and Bradford, M. A. (2018). Direct effects of soil organic matter on productivity mirror those observed with organic amendments. Plant Soil 423, 363–373. doi: 10.1007/s11104-017-3513-5
Olsen, S. R., Cole, C. V., Watenale, F. S., and Dean, L. A. (1954). “Estimation of available phosphorus in soil by extraction with sodium bicarbonate,” in USDA Circulation (Washington, DC), 939.
Orrù, L., Canfora, L., Trinchera, A., Migliore, M., Pennelli, B., Marcucci, A., et al. (2021). How tillage and crop rotation change the distribution pattern of fungi. Front. Microbiol. 12, 634325. doi: 10.3389/fmicb.2021.634325
Patra, S., Julich, S., Feger, K. H., Jat, M. L., Sharma, P. C., and Schwärzel, K. (2019). Effect of conservation agriculture on stratification of soil organic matter under cereal-based cropping systems. Arch. Agron. Soil Sci. 65, 2013–2028. doi: 10.1080/03650340.2019.1588462
Piazza, G., Ercoli, L., Nuti, M., and Pellegrino, E. (2019). Interaction between conservation tillage and nitrogen fertilization shapes prokaryotic and fungal diversity at different soil depths: evidence from a 23-year field experiment in the Mediterranean area. Front. Microbiol. 10, 2047. doi: 10.3389/fmicb.2019.02047
Qiu, H., Ge, T., Liu, J., Chen, X., Hu, Y., Wu, J., et al. (2018). Effects of biotic and abiotic factors on soil organic matter mineralization: experiments and structural modeling analysis. Eur. J. Soil. Biol. 84, 27–34. doi: 10.1016/j.ejsobi.2017.12.003
Rakesh, S., Sarkar, D., Sinha, A. K., Danish, S., Bhattacharya, P. M., Mukhopadhyay, P., et al. (2021). Soil organic carbon and labile and recalcitrant carbon fractions attributed by contrasting tillage and cropping systems in old and recent alluvial soils of subtropical eastern India. PLoS ONE 16, e0259645. doi: 10.1371/journal.pone.0259645
Rezgui, C., Trinsoutrot-Gattin, I., Benoit, M., Laval, K., and Wassila, R. A. (2021). Linking changes in the soil microbial community to C and N dynamics during crop residue decomposition. J. Integr. Agric. 20, 3039–3059. doi: 10.1016/S2095-3119(20)63567-5
Rineau, F., Roth, D., Shah, F., Smits, M., Johansson, T., Canbäck, B., et al. (2012). The ectomycorrhizal fungus Paxillus involutus converts organic matter in plant litter using a trimmed brown-rot mechanism involving Fenton chemistry. Environ. Microbiol. 14, 1477–1487. doi: 10.1111/j.1462-2920.2012.02736.x
Rosling, A., Cox, F., Cruz-Martinez, K., Ihrmark, K., Grelet, G. A., Lindahl, B. D., et al. (2011). Archaeorhizomycetes: unearthing an ancient class of ubiquitous soil fungi. Science 333, 876–879. doi: 10.1126/science.1206958
Sasse, J., Martinoia, E., and Northen, T. (2018). Feed your friends: do plant exudates shape the root microbiome? Trends Plant Sci. 23, 25–41. doi: 10.1016/j.tplants.2017.09.003
Sharma, P. C., Jat, H. S., Kumar, V., Gathala, M. K., Datta, A., Yaduvanshi, N. P. S., et al. (2015). Sustainable Intensification Opportunities Under Current and Future Cereal Systems of North-West India. Karnal: Central Soil Salinity Research Institute, 46.
Sharma, S., Saikia, R., Thind, H. S., Singh, Y., and Jat, M. L. (2021). Tillage, green manure and residue management accelerate soil carbon pools and hydrolytic enzymatic activities for conservation agriculture based rice-wheat systems. Commun. Soil Sci. Plant Analy. 52, 470–486. doi: 10.1080/00103624.2020.1862147
Sharma-Poudyal, D., Schlatter, D., Yin, C., Hulbert, S., and Paulitz, T. (2017). Long-term no-till: a major driver of fungal communities in dryland wheat cropping systems. PLoS ONE 12, e0184611. doi: 10.1371/journal.pone.0184611
Soil Survey Division Staff (1993). Soil Survey Manual. Washington, DC: United States Department of Agriculture, 63–65.
Stromberger, M. E. (2005). “Fungal communities of agroecosystems,” in The Fungal Community: Its Organization and Role in the Ecosystem, 3rd Edn, eds J. Dighton, J. F. White, and P. Oudemans (Boca Raton: CRC Press), 813–832. doi: 10.1201/9781420027891.ch41
Subbiah, B. V., and Asija, G. L. (1956). A rapid procedure for the estimation of available nitrogen in soils. Curr. Sci. India 25, 259–260.
Vargas-Gastélum, L., Romero-Olivares, A. L., Escalante, A. E., Rocha-Olivares, A., Brizuela, C., and Riquelme, M. (2015). Impact of seasonal changes on fungal diversity of a semi-arid ecosystem revealed by 454 pyrosequencing. FEMS Microbiol. Ecol. 91, fiv044. doi: 10.1093/femsec/fiv044
Wakelin, S. A., Macdonald, L. M., Rogers, S. L., Gregg, A. L., Bolger, T. P., and Baldock, J. A. (2008). Habitat selective factors influencing the structural composition and functional capacity of microbial communities in agricultural soils. Soil Biol. Biochem. 40, 803–813. doi: 10.1016/j.soilbio.2007.10.015
Walkley, A., and Black, I. A. (1934). An examination of the Degtjareff method for determining soil organic matter, and a proposed modification of the chromic acid titration method. Soil Sci. 37, 29–38. doi: 10.1097/00010694-193401000-00003
Wang, Z., Chen, Q., Liu, L., Wen, X., and Liao, Y. (2016). Responses of soil fungi to 5-year conservation tillage treatments in the drylands of northern China. Appl. Soil Ecol. 101, 132–140. doi: 10.1016/j.apsoil.2016.02.002
Wang, Z., Li, T., Wen, X., Liu, Y., Han, J., Liao, Y., et al. (2017). Fungal communities in rhizosphere soil under conservation tillage shift in response to plant growth. Front. Microbio. 8, 1301. doi: 10.3389/fmicb.2017.01301
White, T. J., Bruns, T. D., Lee, S. B., and Taylor, J. W. (1990). Amplification and direct sequencing of fungal ribosomal RNA Genes for phylogenetics. PCR Protocols Guide Methods Appl. 18, 315–322. doi: 10.1016/B978-0-12-372180-8.50042-1
Xu, J. M., Tang, C., and Chen, Z. L. (2006). Chemical composition controls residue decomposition in soils differing in initial pH. Soil Biol. Biochem. 38, 544–552. doi: 10.1016/j.soilbio.2005.06.006
Yadav, A.N., Mishra, S., Kour, D., Yadav, N., and Kumar, A., eds. (2020). Agriculturally Important Fungi for Sustainable Agriculture. Cham: Springer. doi: 10.1007/978-3-030-48474-3
Yang, Y., Dou, Y., Huang, Y., and An, S. (2017). Links between soil fungal diversity and plant and soil properties on the Loess Plateau. Front. Microbiol. 8, 2198. doi: 10.3389/fmicb.2017.02198
Zahid, A., Ali, S., Ahmed, M., and Iqbal, N. (2020). Improvement of soil health through residue management and conservation tillage in rice–wheat cropping system of Punjab, Pakistan. Agronomy 10, 1844. doi: 10.3390/agronomy10121844
Zhang, N., Castlebury, L. A., Miller, A. N., Huhndorf, S. M., Schoch, C. L., Seifert, K. A., et al. (2006). An overview of the systematics of the Sordariomycetes based on a four-gene phylogeny. Mycologia 98, 1076–1087. doi: 10.1080/15572536.2006.11832635
Keywords: agriculture management, tillage, fungal community, diversity indices, climate smart agricultural practices, soil organic carbon
Citation: Choudhary M, Jat HS, Jat ML and Sharma PC (2022) Climate-smart agricultural practices influence the fungal communities and soil properties under major agri-food systems. Front. Microbiol. 13:986519. doi: 10.3389/fmicb.2022.986519
Received: 05 July 2022; Accepted: 22 November 2022;
Published: 13 December 2022.
Edited by:
Flávio H. V. Medeiros, Universidade Federal de Lavras, BrazilReviewed by:
Deepranjan Sarkar, Banaras Hindu University, IndiaCopyright © 2022 Choudhary, Jat, Jat and Sharma. This is an open-access article distributed under the terms of the Creative Commons Attribution License (CC BY). The use, distribution or reproduction in other forums is permitted, provided the original author(s) and the copyright owner(s) are credited and that the original publication in this journal is cited, in accordance with accepted academic practice. No use, distribution or reproduction is permitted which does not comply with these terms.
*Correspondence: Hanuman S. Jat, aHNqYXRfYWdyb25AeWFob28uY29t; Mangi L. Jat, bS5qYXRAY2dpYXIub3Jn
Disclaimer: All claims expressed in this article are solely those of the authors and do not necessarily represent those of their affiliated organizations, or those of the publisher, the editors and the reviewers. Any product that may be evaluated in this article or claim that may be made by its manufacturer is not guaranteed or endorsed by the publisher.
Research integrity at Frontiers
Learn more about the work of our research integrity team to safeguard the quality of each article we publish.