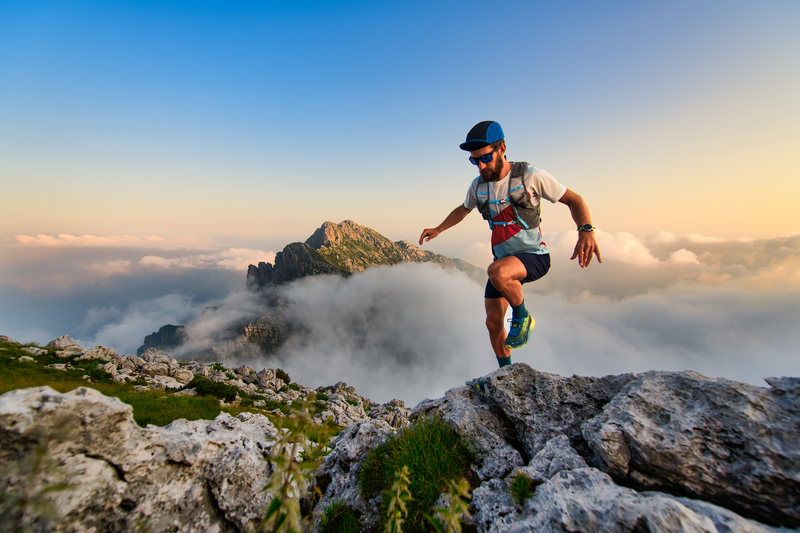
94% of researchers rate our articles as excellent or good
Learn more about the work of our research integrity team to safeguard the quality of each article we publish.
Find out more
ORIGINAL RESEARCH article
Front. Microbiol. , 05 October 2022
Sec. Antimicrobials, Resistance and Chemotherapy
Volume 13 - 2022 | https://doi.org/10.3389/fmicb.2022.939919
This article is part of the Research Topic Novel Approaches towards Antimicrobial Drug Design and Target Discovery View all 5 articles
Gram-positive Streptomyces bacteria can produce valuable secondary metabolites. Streptomyces genomes include huge unknown silent natural product (NP) biosynthetic gene clusters (BGCs), making them a potential drug discovery repository. To collect antibiotic-producing bacteria from unexplored areas, we identified Streptomyces sp. CS-7 from mountain soil samples in Changsha, P.R. China, which showed strong antibacterial activity. Complete genome sequencing and prediction in silico revealed that its 8.4 Mbp genome contains a total of 36 BGCs for NPs. We purified two important antibiotics from this strain, which were structurally elucidated to be mayamycin and mayamycin B active against Staphylococcus aureus. We identified functionally a BGC for the biosynthesis of these two compounds by BGC direct cloning and heterologous expression in Streptomyces albus. The data here supported this Streptomyces species, especially from unexplored habitats, having a high potential for new NPs.
Antibiotic resistance is a serious problem in medicine and agriculture due to the prevalence of drug-resistant bacteria and fungi (O’Neill, 2014; Toner et al., 2015). Antibiotic-resistant illnesses are responsible for more than 35,000 fatalities in the United States. Antibiotic microbial resistance (AMR) is on the rise across all major antibiotic classes, therefore the search for new antibacterial chemicals is becoming more urgent (Genilloud, 2014). Following the World Health Organization (WHO) and the center for disease control and prevention (CDC) crisis reports in 2015, 40 antibiotics have been approved, 75% of which are reformulations of older antibiotics. Researchers are now looking at previously ignored or extreme environments for new producers that exhibit antibiotic action, hoping to find new compounds with new structures (Chevrette et al., 2019).
Natural products make up more than 75% of antibiotics (Katz and Baltz, 2016; Hutchings et al., 2019). Many natural antibiotics have been isolated from plants, fungi, and bacteria as the primary sources (Firn and Jones, 1996; Luo et al., 2014; Katz and Baltz, 2016; Baltz, 2019). It is well known that bacteria in the genus Streptomyces can produce more than two-thirds of all therapeutically effective antibiotics (Hopwood, 2006; Tchize Ndejouong et al., 2010; Tiwari and Gupta, 2012; Lee et al., 2019). For example, daptomycin as the last line against drug-resistant pathogens was isolated from Streptomyces (Li et al., 2013).
Streptomyces could produce not only antibiotics but also antifungal, antiviral, antiparasitic, antitumoral, and immunosuppressive analogs, as well as other important secondary metabolites (Jones and Elliot, 2017). These features enable Streptomyces to outcompete other microbes and overcome environmental stresses in harsh conditions (Lo Giudice et al., 2007; Fajardo and Martínez, 2008; Gulder and Moore, 2009; Núñez-Montero et al., 2019). These bioactive compounds from Streptomyces are classified into several major groups according to their core skeletons and biosynthetic mechanisms, including PKs (polyketides), NRPs (non-ribosomal peptides), RiPPs (ribosomally synthesized and post-translationally modified peptides), aminoglycoside, terpenes, and so on. Notably, these common types of secondary metabolites are produced in modular modes using large molecular assembly lines encoded by biosynthetic gene clusters (BGCs) (Newman and Cragg, 2020).
Besides terrestrial habitats, numerous Streptomyces individuals with antimicrobial properties have been identified in some extreme or unexplored environments, such as marine sponges (Kennedy et al., 2009), hot springs (Nakaew et al., 2019), salty and alkalic lakes (Terra et al., 2018), insect guts (Chevrette et al., 2019), polar areas, and so on (Chevrette et al., 2019). Streptomyces strains from these special habitats might have more potential to produce compounds with novel structures (Masand et al., 2018; Sivalingam et al., 2019).
Historically, drug development from Streptomyces has relied on bioactivity screening coupled with mass spectrometry and NMR-based molecular identification (Ziemert et al., 2016). A variety of techniques and machineries were established throughout the years for the detection of NPs. “One Strain Many Compounds” (OSMAC) technique is a simple and effective method for activating quiet BGCs (Pan et al., 2019). This method may be carried out by modifying the medium components, the culture conditions, or co-cultivating with different strains.
With advances in biosynthesis, bioinformatics, and whole genome sequencing (Galanie et al., 2020), natural product discovery has had a revival in the last decade which is featured as referenced genome mining approach: whole genome sequencing is the first step, followed by computer mining for BGCs, selecting specific BGCs, and activating BGC in situ or cloning and expressing the particular BGCs in a model heterologous chassis. This approach reduces the requirement for dereplication and speeds up NP discovery. Computing methods and BGC databases like antiSMASH, PRISM, and MIBiG have made computational genome mining a feasible tool for identifying novel NPs in recent years (Alam et al., 2021).
Currently, the NCBI (National Center for Biotechnology Information) genome datasets have 4,919 different Streptomyces genome assemblies. Streptomyces species have a larger capability to produce secondary metabolites than previously thought, which was proved by a significant number of BGCs, occupying more than 15% of the Streptomyces genomes (Nett et al., 2009; Ikeda et al., 2014; Chevrette and Currie, 2019), with high-quality sequencing of genomes (Reva and Tümmler, 2008; Li et al., 2018; Hwang et al., 2019). Generally, a single genome of Streptomyces possesses 20–50 different BGCs.
However, BGCs’ expression is strictly controlled and many of them are still dormant (Rodríguez et al., 2013; Hoshino et al., 2019) and more than 90% of BGCs are not expressed under standard laboratory conditions (Nett et al., 2009; Katz and Baltz, 2016), explaining that though Streptomyces have been projected to create around 100,000 antimicrobial metabolites, only a tiny percentage of them have been discovered (Watve et al., 2001; Kumar et al., 2014; Stulberg et al., 2016).
But activation of in situ or heterologous expression of these BGCs will be an alternative way to solve this problem (Ômura et al., 2001; Rebets et al., 2014). Scleric acid, a novel chemical active against Mycobacterium TB, was discovered recently when its BGC from Streptomyces sclerotialus NRLP ISP-5269 was activated through genetic engineering (Alberti et al., 2019).
This study aimed to explore the potential of a Streptomyces strain CS-7 obtained from an unexplored mountain. This strain’s genome was sequenced and mined to its secondary metabolites. It was found that Streptomyces sp. CS-7 is highly antibacterial against Staphylococcus aureus and Bacillus cereus, which is tested and possesses a substantial number of BGCs on its genome, indicating that the strain is capable of producing new chemicals with biological activity. Moreover, directed by antibacterial activity, we isolated two compounds from it and confirmed their BGC using heterologous expression.
Escherichia coli GB05-dir was used for linear plus linear homologous recombination (LLHR) to construct plasmids while E. coli GB08-red for linear plus circular homologous recombination (LCHR) (Fu et al., 2012). E. coli ET12567/pUZ8002 was used for plasmid conjugation. Streptomyces albus (S. albus) J1074 was a commonly used host to express the predicted gene cluster.
All E. coli strains were grown on a Luria broth (LB) medium for propagation at 37°C. The antibiotic concentrations used for resistance selection of E. coli strains on LB agar plates or liquid medium were as follows: chloramphenicol (15 or 10 μg/ml), kanamycin (15 or 10 μg/ml), and apramycin (20 or 10 μg/ml). The S. albus J1074 was grown on Mannitol soya flour medium (MS) for sporulation and conjugation at 30°C. Tryptic soy broth (TSB) and R5MS liquid medium was used as seed medium and fermentation medium. The concentrations of antibiotics used for resistance selection of S. albus J1074 strains on MS agar plates were as follows: apramycin (40 μg/ml) and nalidixic acid (25 μg/ml).
The PCR amplification was performed with PrimeSTAR HS DNA polymerase (Takara, cat. -no. R044A) and further purification of dsDNA was conducted with agarose gel DNA recovery kit (TIANGEN, cat. -no. DP219-03) according to the manufacturer’s instructions. The restriction enzymes were purchased from New England Biolabs. Antibiotics were purchased from Sangon (Shanghai) and Sigma.
Soil from a small unexplored habitat (Tiesi Gang, a mountain area not explored) in Zoushi Town, Changde City, Hunan, China was sampled with a sterilized spoon, then placed in a plastic bag and sent to the lab for additional processing. Soil samples were immersed and serially diluted in buffer solutions, and spread on an actinomycetes isolation agar (AIA) medium. The plates were incubated at 28°C for 5–7 days. Then, particular actinomycetes colonies were subcultured on ISP-2 medium and kept at 28°C for 5–7 days.
Morphological observation of the CS-7 was conducted under Scanning Electron Microscope (SEM), including sampling, fixation, dehydration, drying, and observing steps in the following: (i) CS-7 was inoculated into 50 mL of liquid culture medium TSB in a 250 ml flask and shaken for 48 h. After liquid seed cultures of CS7 were centrifuged for 15 min at 4,000 × g, the resultant pellets were suspended in 1 × PBS (pH 6.8 ∼ 7.4) and then centrifuged for 15 min to discard the supernatants. The pellets were added quickly with 25 mL 2.5% glutaraldehyde (configured with 1 × PBS) and then fixed for 3 h in a 4°C refrigerator. After centrifugation, the fixed pellets were washed with 1 × PBS 3 times (15 min each time, and remove the supernatants by centrifugation). (ii) Next, samples were dehydrated with ethanol aqueous solution at the concentration gradient of 30, 50, 70, 80, and 90%, and centrifuged for 15 min to discard the supernatants. Finally, samples were dehydrated again in 100% ethanol and centrifuged for 15 min to remove the supernatants (repeat this step two times). (iii) Samples were resuspended in 100% ethanol and 5 μL of resultant bacterial suspension was dripped on the Φ12 mm cover glass. After volatilizing the alcohol to a semi-dry state, the cover glasses containing the samples were placed in a critical point dryer for drying. (iv) After the samples were fully dried, they adhered to the sample table with conductive tape, then an ultrathin coating of electrically conducting material was added onto the surface of the samples by using Sputter Coater. (v) The samples were observed under SEM (FEI Quanta 250F field emission environmental scan, US) for the spore structure and mycelium appearance.
The seed culture was made in Trypticase soy broth (TSB) and inoculated into a 1 L Erlenmeyer flask containing liquid R5MS fermentation medium (Composition mentioned in Supplementary File). The flask was placed in a rotary shaker for 5–7 days at 28°C and added with the 1% resin again placed into the rotary shaker for 2 days. After centrifugation, an equal amount of methanol was added to the pellets and shaken for 24 h at 28°C, then concentrated to dry in a rotary evaporator (Liu et al., 2011).
The cross-streak method was used to screen potential actinomycetes isolates for antibacterial activity on Mueller Hinton Agar (MHA) medium.
Kirby–Bauer disk diffusion method was used for bioactivity-based screening of bacterial strains (Hudzicki, 2009): 1 ml methanolic crude extracts were obtained from 50 mL culture broth of CS-7, then used for disk diffusion assay. Gram-positive Staphylococcus aureus ATCC 29213 and Bacillus subtilis ATCC6633, and Gram-negative Escherichia coli ATCC35218 and Pseudomonas aeruginosa ATCC 27853 were used as test organisms and planted on MHA medium, and filter paper disks were placed on the top of the agar surface. The methanolic extracts of the prospective isolates were put into disks (10 μl for a Φ0.6 cm filter paper disk) and incubated for 24 h at 37°C. Following inoculation, the plates were examined for the presence of a distinct zone of inhibition.
Streptomyces strain CS-7 was inoculated into 50 mL of liquid culture medium TSB with 0.16 g mL–1 glass beads (3 ± 0.3 mm diameter) in a 250-mL baffled flask and cultured for 2 days at 30°C in a 200-rpm orbital shaker. To extract gDNA, 50 mL cultivated cells were collected during the exponential growth phase and washed two times with the same amount of 10 mM EDTA followed by 45-min lysis at 37°C with lysozyme (10 mg mL–1). gDNA for Gram-positive bacteria was extracted according to the lab protocol offered by the kit manufacturer (Bioteke Corporation, Beijing). The quality and concentration of extracted gDNA samples were determined using 1% agarose gel electrophoresis and Nanodrop (Thermo Fisher Scientific, Waltham, MA, USA).
The gDNA was submitted to the company (Genewiz, China) for Next Generation Sequencing, including DNA quality control, library construction, sequencing, assembly, and annotation:
(i) Library construction: DNAs were fragmented to <500 bp by sonication (Covaris S220), treated with End Prep Enzyme Mix for end repairing, 5′ Phosphorylation, and dA-tailing, followed by a T-A ligation to add adaptors to both ends. Size selection of adaptor-ligated DNA was then performed, and fragments of ∼470 bp were recovered. Each sample was then amplified by PCR. The PCR products were cleaned and checked using an Agilent 2100 Bioanalyzer (Agilent Technologies, Palo Alto, CA, USA) before being quantified with a Qubit 3.0 Fluorometer (Invitrogen, Carlsbad, CA, USA). The libraries were then multiplexed and put on an Illumina HiSeq instrument in accordance with the manufacturer’s instructions (Illumina, San Diego, CA, USA).
(ii) Sequencing: It was carried out using a 2 × 150 paired-end (PE) configuration; image analysis and base calling were conducted by the HiSeq Control Software (HCS) + OLB + GAPipeline-1.6 (Illumina) on the HiSeq instrument image analysis and base calling were conducted by the NovaSeq Control Software (NCS) + OLB + GAPipeline-1.6 (Illumina) on the NovaSeq instrument. Image analysis and base calling were also conducted by the Zebeacall on the MGI2000 instrument.
(iii) Assembly: The reads that passed QC were assembled using velvet, gap filled with SSPACE and GapFiller (Zerbino and Birney, 2008; Zerbino et al., 2009; Boetzer et al., 2011; Boetzer and Pirovano, 2012; Hunt et al., 2014). The Prodigal (for prokaryotes) (Delcher et al., 2007) or Augustus (for eukaryon) (Stanke et al., 2006) gene finding application has been utilized for identifying coding genes in bacteria. The software tRNAscan-SE (Lowe and Eddy, 1997) was used to identify tRNAs in the genome. RNAmmer was used to find the rRNA (Lagesen et al., 2007).
(iv) Annotation: BLAST was used to annotate the coding genes using the National Center for Biotechnology Information (NCBI) nr database. The GO (Gene Ontology) database (Gene Ontology Consortium, 2004) and the KEGG (Kyoto Encyclopedia of Genes and Genomes) database (Kanehisa and Goto, 2000) were used to annotate gene functions and pathways. Based on the predicted protein sequence of the coding gene, protein sequences in the database were aligned using BLAST software (version 2.2.31+). The E-value of the sequence alignment was set to 1e–5, and the selected best matching result is taken as the annotation result of the gene. The proteins encoded by genes were classified on a phylogenetic classification by the database of COG (Clusters of Orthologous Groups).
The TrueBac™ ID technology, a cloud-based service for bacterial identification utilizing whole genome sequences, was used in this experiment (Ha et al., 2019). Its aim is to reveal the genuine identification of bacterial isolates using a multitude of methods. TrueBac™ ID Genome depends entirely on genome sequence data, differing from other bacterial identification methods. Given that modern bacterial taxonomy uses genome sequence data to identify taxa, using genome sequence data to identify a bacterial species is always correct and persuasive.
Phylogenetic trees were constructed based on the 16S rRNA gene sequence and whole genome sequence of the Streptomyces strain CS-7. First, a set of related reference sequences were extracted from the list of hits from the EzBioCloud 16S database (Yoon et al., 2017a) to make the evolutionary tree using neighbor-joining methods (Saitou and Nei, 1987) and maximum-likelihood methods (Felsenstein, 1981) in MEGA X package (Kumar et al., 2018). The confidence of the tree topologies was assessed by 100 bootstrap replicates.
Moreover, the Type (Strain) Genome Server (TYGS) webserver was used to generate a 16S rRNA gene sequence and a complete genome-based phylogenetic tree. TYGS, a free bioinformatics platform, is accessible at https://tygs.dsmz.de (Meier-Kolthoff and Göker, 2019). TYGS webpage was used to upload the entire genome sequences. TYGS used RNAmmer (Lagesen et al., 2007) to extract 16S rRNA gene sequences from query genomes, followed by NCBI BLAST+ searches against the TYGS database. To calculate the Genome BLAST Distance Phylogeny (GBDP) (Hahnke et al., 2016) values, the top 50 BLAST bitscore genomes were considered. The closest relatives were identified as the genomes having the lowest 16S rRNA gene GBDP distances between each query genome and type strain under the algorithm “coverage” and distance formula d5 (Meier-Kolthoff et al., 2013a). After that, FastME 2.1.6.1 was used to build a 16S rRNA-gene-sequence-based phylogenetic tree combining subtree pruning and regrafting (SPR) (Lefort et al., 2015). Using MEGA X, the tree was depicted (Kumar et al., 2018). In the same way, GBDP was used for a whole genome-based taxonomic analysis, using TYGS (accessed December 28, 2021).
Digital DNA: DNA hybridization (dDDH) values for Streptomyces sp. CS-7 genome and its neighbors were calculated using the TYGS analysis tool’s Genome-to-Genome Distance Calculator (GGDC 2.1) (Meier-Kolthoff et al., 2013a; Kumar et al., 2018). The average nucleotide identity (ANI) values between the CS-7 genome and its nearest neighbors were computed using the Kostas lab’s ANI calculator1. ANI is the mean identity of BLASTn matches (Goris et al., 2007). DNA-DNA hybridization (DDH) is the “gold standard” for species delineation and is still commonly employed to evaluate the genetic relatedness between closely related organisms (Ramasamy et al., 2014). The systematic community has universally adopted Wayne et al. (1987) 70% DDH recommendation for bacterial species boundaries.
EZBIOCLOUD was used to determine the average nucleotide identity (ANI) of the Streptomyces nucleotide files, by comparing them to the strains’ whole-genome sequences 16S rRNA sequence (Yoon et al., 2017b). This approach uses pairwise sequence alignment to calculate nucleotide identity, offering an average genome similarity.
The CGView2 (Grant and Stothard, 2008) was used to generate a graphical representation of the BLAST results by comparing the available genomes to the genome of CS-7.
For the possible discovery of BGCs involved in the production of secondary metabolites, genome mining prediction platforms using a combination of antiSMASH 6 (Blin et al., 2021) with Known ClusterBlast, ActiveSiteFinder, ClusterBlast, Cluster PFam analysis, and SubClusterBlast. PRISM 4 (Skinnider et al., 2020) and BAGEL 4 (van Heel et al., 2018) with default settings computational programs were implemented. AntiSMASH 6 makes finding, annotating, and researching secondary metabolite BGCs across the genome. BAGEL 4 is designed to mine RiPPs and bacteriocin, whereas PRISM 4 is designed to analyze secondary metabolite structure and biological activity in a comprehensive manner. These sophisticated computer model services provide accurate predictions of microbial secondary metabolite encoding potential and putative structures (Machado et al., 2015). For BGC annotation from genomic sequences, these programs use several database systems, including the principles of hidden Markov model (HMM) (Churchill, 1989), BLAST algorithm (Altschul et al., 1990), PFAM (Finn et al., 2014), GenBank (Benson et al., 2013), UniprotKB (UniProt Consortium, 2015), bactibase (Hammami et al., 2010), CAMPR3 (Waghu et al., 2016), and the MiBiG data repository (Medema et al., 2015). NaPDoS was also used (Ziemert et al., 2012) to look for KS (ketosynthase) and C (condensation) domains in these genomic sequences.
The seed cultures were made in trypticase soy broth (TSB) and incubated at 30°C on a 200-rpm shaker. Then, seed cultures were diluted at the ratio of 1:50 into 50 mL of R5MS broth in 250 mL flasks. The 2% resin XAD-16 was added into the fermentation broth after 4-day cultivation and cultivated at 30°C, 200 rpm for another day. The resin XAD-16 was collected and extracted with methanol. The MeOH crude extract was separated by silica gel column chromatography (CH2Cl2-MeOH, 20:1 to 1:1) to yield five fractions (Frs. 1-5). Fr. 4 was further purified by semi-preparative HPLC (B: ACN and A:H2O with 0.1% TFA (trifluoroacetic acid), 0–4 min 25% B, 4–29 min 50% B, 29–40 min 100% B, 40–45 min 25% B, 2 mL/min) to give 1 (5 mg, tR = 28 min) and 2 (8 mg, tR = 29 min) (Supplementary File).
1D NMR spectrum for compound 1 or 2 was obtained using TMS as an internal standard on a Bruker AVNEO 600 MHz. HRESIMS spectra were obtained using the standard ESI source on a Bruker Impact HD microTOF Q III mass spectrometer (Bruker Daltonics, Bremen, Germany). Semi-preparative HPLC was performed using an ODS column (Agilent ZORBAX SB-C18, 9.4 mm × 250 mm, 5 μm, 2 mL/min).
Two pairs of primers were used to clone the putative BGC (BGC8.1, also named as cluster may) from CS-7 genomic DNA via two-step recombination using two pairs of primers: Forward primer 1 (5′-gtgagtgaacactcaccctcccgtcaaatgcctggcgcgacccgg tgcggAGATCCGAAAACCCCAAG)/reverse primer 1 (5′-gggaggcgggtgagtagaatag ggaaagagatgtcaaggaacggggggttAAGCTTTTAATTAAAGATCCTTTCTCCTCTTT) and Forward primer 2 (atcagtgatagagaaaagaattcaaaagat ctaaagaggagaaaggatctTGCCGGGCGTCGGCGCAG)/reverse primer 2 (gggaggcgggtgagtagaataggg aaagagatgtcaaggaacggggggttAACCATGCGTCCAGTAGT). The lowercase parts in primer sequences match the target sequences of the BGC while the uppercase parts are homologous to the plasmid vector and the introduced restriction site is underlined.
gDNA of CS-7 was digested with HpaI and SpeI to obtain the may-1 fragment (BGC). Using the p15A-cm-tetR-tetO-hyg-ccdB plasmid as a template, the vector fragment p15A-cm-tetR-tetO containing the may-1 homology arms was obtained by PCR amplification with primer pair 1. The vector fragment was recovered and recombined with may-1 in Escherichia coli GB05-dir by the Red/ET recombineering technique. The correct recombinant p15A-cm-may-1 was screened by enzyme digestion, then confirmed further by sequencing.
Plasmid p15A-cm-may-1 digested with HindIII and PacI was recombined in E. coli GB05-dir with the may-2 fragment amplified by primer pair 2 using gDNA of CS7 as a template. Enzyme digestion and sequencing were used to verify the correct recombinant p15A-cm-may.
After being inserted with the oriT-attP-phiC31-apra cassette, the plasmid p15A-phiC31-apra-may was electroporated into E. coli ET12567/pUZ8002, then further transferred into the host S. albus J1074 (the conjugant strain was named S. albus J1074/may). P15A-phiC31-apra-may was integrated into the specific landing point (attB loci for phiC31) of the chromosome of S. albus J1074 via site-specific integration.
Seed cultures of S. albus J1074/may on TSB medium were incubated at 30°C on a 220 rpm shaker for 36 h. Then, the seed liquid broth was inoculated into a 250-ml shaking flask containing 50 ml of R5MS fermentation medium at an inoculation amount of 2%, and the cultivation continued according to the above conditions. After the cultures were incubated for 4 days, 1 mL of resin Amberlite XAD16 was added, and the mixture was incubated for another 24 h continually.
Cultures were centrifuged at 8,000 × g for 15 min, and the supernatant was discarded. The XAD16 and sedimented mycelium were extracted with 40 mL MeOH incubated at 30°C with 200 rpm shaking for 3 h. Then, the organic layer was evaporated to dryness after filtering. After the crude extract was dissolved in 1 mL MeOH, it was centrifuged for 20 min, and the supernatant was taken and filtered through a 0.22-μm filter for subsequent high-performance liquid chromatography-mass spectrometry (HPLC-MS) analysis.
The HPLC-MS analysis was carried out on a Bruker amazon SL Ion Trap mass spectrometer coupled with an Ultimate 3000 UHPLC-DAD system (Thermo Scientific). The HPLC conditions were as follows: reversed-phase C18 column (2.2 μm, 2.1 mm × 100 mm, Thermo) at a flow rate of 0.3 mL/min using a mobile phase with a linear gradient of A: H2O with 0.1% FA (formic acid) and B: acetonitrile (ACN) with 0.1% FA, 0–3 min: 5% B; 3–19 min: 5–95% B; 19–22 min: 95% B; 23–25 min: 5% B.
A total of 17 putative colonies were isolated from sediment samples of a mountain area, based on Actinomycetes-specific morphological traits such as sluggish development, colony sporulation, and filamentous appearance (Figure 1A). One of them, strain CS-7, stood out from the others due to its antimicrobial activity against Gram-positive and Gram-negative bacteria. In ISP-2 medium, the mycelia were, respectively, yellowish brown. The Streptomyces strain CS-7 colonies on ISP-2 medium and the microscopic observation of the cells were recorded (Figures 1B,C).
Figure 1. Isolation and morphological observation of Streptomyces spp. (A) Isolated samples from mountain soils in China cultivated on International Streptomyces Project agar number 2 (ISP2) media at 28°C for 15 days (plate’s number indicates the soil site and letter indicates a phenotype). (B) Streptomyces strain CS-7 colonies on ISP-2 medium. (C) Scanning electron micrographs of the CS-7 isolate grown on TSB broth at 28°C for 2 days.
Among all the isolates subjected to preliminary and secondary screening against various bacteria, interestingly, the isolate CS-7 exhibited selective strongest action against pathogenic Staphylococcus aureus ATCC 29213 (Figure 2).
Figure 2. Antimicrobial activity test of Streptomyces isolated from soil samples. Methanolic extracts of Streptomyces bacteria were analyzed by disk diffusion methods. The appearance of inhibition zones indicated the antimicrobial activity against the indicator Staphylococcus aureus.
This activity test pushed us to isolate antibacterial compounds from CS-7. We purified two compounds (1 and 2) from its fermentation broth and elucidated their structures:
Compound 1 (Figure 3), isolated as a brown amorphous powder (MeOH), gave a molecular formula of C25H23NO7, as deduced from the quasi-molecular ion at m/z [M + H]+ 450.1543 (calcd 450.1547) by HRESIMS. Further analysis of the 1D NMR data (Table 1) of 1 revealed that the structure of 1 was the same as that of mayamycin B, which is an angucycline-type polyketide with a C-glycosidically bound amino sugar moiety (Bo et al., 2018). Compound 2 (Figure 3) was also obtained as a brown amorphous powder (MeOH). Its molecular formula, C26H25NO7, was established from a quasi-molecular ion peak at m/z [M + H]+ 464.1707 (calcd 464.1704) by HRESIMS. The 1D NMR data of 2 was similar to that of 1, except for the presence of a methyl group [δH 1.45, d, J = 6.6 Hz; δC 18.4]. Further elucidation of the HRESIMS spectra indicated that compound 2 has the same anguacycline aglycone as that of 1. The structure of compound 2 was determined to be mayamycin by comparison with reported spectroscopic data (Schneemann et al., 2010).
Figure 3. Structures of compounds 1 and 2 isolated from Streptomyces CS-7. The compounds (1: mayamycin B and 2: mayamycin) were isolated in this research and elucidated structurally using HRESIMS and NMR techniques.
In whole-genome sequencing (single-molecule real-time sequencing), the complete genome sequence of Streptomyces strain CS-7 was composed of 35 contigs with a total length of 8,404,904 bps. The contig length of N50 was 773,247 bp. The partial 16S rDNA gene sequence of the CS-7 strain, 1,390 bps in length, was deposited in the GenBank nucleotide database with an accession number OM009281.
The complete genome sequence of Streptomyces strain CS-7 is 8,404,904 bps in length, with an average G + C content of 71.51%. Totally 7,593 genes are identified in its genome, including 7,466 annotated protein-coding genes, 67 tRNA, and 6 rRNA genes (Tables 2, 3).
Phylogenetic analyses indicated that CS-7 belongs to the genus Streptomyces and shared the highest gene identity of 16S rDNA (99.93%) with the type strain Streptomyces mediolani and Streptomyces pratensis (NCBI Blastn).
The phylogenetic tree constructed from the EzBioCloud 16S database by maximum-likelihood and neighbor-joining methods by Mega X application with 100 bootstrap values was depicted in Figures 4A,B. According to the maximum likelihood method, CS-7 is close to the Streptomyces parvus NBRC 3388, Streptomyces badius NRRL B-2567, Streptomyces globisporus NBRC 12867, Streptomyces sindenensis NBRC 3399, Streptomyces pluricolorescens NBRC 12808, and Streptomyces rubiginosohelvolus NBRC 12912.
Figure 4. Analysis of phylogenetic links among the close taxa. The phylogenetic lineage was inferred using (A) the Maximum Likelihood method and (B) the Neighbor-Joining method. The numbers above branches are GBDP pseudo-bootstrap support values from 100 replicates and only values above 50% are shown.
On the other hand, CS-7 is most similar to S. pluricolorescens NBRC 12808, S. globisporus NBRC 12867, and S. rubiginosohelvolus NBRC 12912 according to the neighbor-joining phylogeny approach.
On the contrary, the phylogeny of Streptomyces strain CS-7 was derived by means of the GBDP method depicted in Figures 5A,B. FastME was used to estimate the tree using GBDP intergenomic distances derived from complete proteomes. Both the genome and 16S rRNA gene GBDP trees were made by the tree builder service. S. globisporus JCM 4378 and S. rubiginosohelvolus JCM 4415 are closest to CS-7 according to 16S rRNA while S. rubiginosohelvolus JCM 4415 and S. pluricolorescens JCM 4602 are most related to CS-7 genome sequence phylogeny build by GBDP method.
Figure 5. Genome BLAST Distance Phylogeny (GBDP) computed taxonomy for Streptomyces CS-7. GBDP intergenomic distances were derived from complete proteomes, and the trees were inferred using FastME. (A) Phylogenetic constructed from 16S rRNA and (B) phylogenetic tree constructed from whole genome trimming.
According to the TrueBac™ ID system (Ha et al., 2019) for bacterial identification using whole genome sequences, the strain CS-7 was identified as Streptomyces sp. by 16S rDNA evidence (Table 3).
A comparative genomics technique was used to generate the circular chromosome. The map of the linear chromosome of Streptomyces strain CS-7 was created by the CG View server (see Text Footnote 2) and illustrated in Figure 6. The CGView Server is a web-based tool for doing comparative genomics on circular genomes (Grant and Stothard, 2008). The sequence of the Streptomyces strain CS-7 genome has been deposited at GenBank under the GenBank with accession number JAJUKK000000000.
Figure 6. Schematic representation of the linear chromosome of Streptomyces strain CS-7, created by CG View server (http://cgview.ca/). Circles 1, 2, and 3 display the ORFs. Circle 4 displays the CDS. Circle 6 displays the contigs. Circle 5 displays the tRNA genes. Circle 6 displays the GC percentage plot. Circle 7 displays the GC skew.
The pairwise comparison of Streptomyces strain CS-7 was recorded from TYGR (Meier-Kolthoff and Göker, 2019) in Supplementary Table 1. TYGS Genomics-based taxonomy is a fast-increasing discipline of genome-based taxonomy descriptions of new genera, species, and subspecies. dDDH values derived from 15 closely similar-type strain genomes revealed that they fall lower than the 70% threshold (Wayne et al., 1987) except for S. rubiginosohelvolus JCM 4415, S. globisporus JCM 4378, and S. mediolani NRRL WC-3934, which were above 70% threshold.
According to genome-wide alignment using the TrueBac™ ID (Ha et al., 2019), Streptomyces strain CS-7 has the highest similarity to S. badius, S. globisporus, S. pluricolorescens, and S. parvus (Supplementary Table 2).
The strain CS-7 demonstrated antimicrobial activity against pathogenic microbes, indicating the potential to produce NPs having antimicrobial activity. To find new compounds, here we predicted NP BGCs on its genome.
In silico genome analyses with antiSMASH 6.0 (Blin et al., 2021), BAGEL 4 (van Heel et al., 2018), and PRISM 4 (Skinnider et al., 2020) on the Streptomyces CS-7 genome revealed its potential to produce several types of different secondary metabolites, spanning polyketides (by PKS/polyketide synthases), non-ribosomal peptides (NRPS/non-ribosomal peptide synthases), and bacteriocins (Table 5).
By the antiSMASH database, 36 BGCs were found in the Streptomyces sp. CS-7 genome, among which 25 clusters showed different similarities to gene clusters with a known function (Table 5).
A total of 36 BGCs were identified by antiSMASH, in which contig 1, 3, 9, 11, 12, 20, 22, 23, 25, 29, 30, 31, 34, and 28 have only 1 (One) BGC, contig 4, 6, and 16 contains 2 (Two) BGCs, contig 8 contains 3 BGCs, contig 2 and 5 contain 4 BGCs, contig 7 contains 5 BGCs, and no secondary metabolite regions exist in scaffold 10, 13, 14, 15, 17, 18, 19, 21, 24, 26, 27, and 33 (Figure 7).
Table 5 and Figure 7 support that Streptomyces sp. CS-7 has a better possibility of producing new antibiotics. BGCs typical for the Streptomyces genus include those for the synthesis of desferrioxamine B, a siderophore involved in iron chelation, and ectoine helps survive extreme osmotic stress.
Next, we used BAGEL to analyze the genome sequence of CS-7 and found a total of 8 BGCs for different bacteriocins and RiPPs (Table 6).
Using the PRISM algorithm, a total of 29 clusters were identified. Among them, 12 NRPS, 1 PKS, 4 hybrid clusters, 1 lassopeptide, 1 ectoine, 1 thiopeptide, 2 butyrolactone, 1 melanin, 1 class III/IV lantipeptide, 1 terpene, 2 NRPS-independent siderophore synthase, 1 class I lantipeptide, and 1 class II lantipeptide/class III/IV lantipeptide.
Based on the BGCs identified using three approaches, we made a structural prediction of proposed compounds by these BGCs as shown in Figure 8.
Analysis of the CS7 genome sequence by antiSMASH revealed a putative gene cluster (BGC8.1) for mayamycin biosynthesis (Figure 9). Besides PKS-II genes (composed of six genes encoding ketosynthase alpha, ketosynthase chain-length factor, ketoreductase, cyclase, and aromatase), this cluster contains some genes for tailoring modification, regulation, and transportation. It was highly featured with six genes predicted for the biosynthesis of the rare angolasamine moiety and a gene for rare C-glycosyl transfer.
To investigate if it is responsible for the biosynthesis of mayamycins, a genome fragment (∼25 kb) covering BGC8.1 (named as may cluster) was cloned into the p15A-cm-oriT vector in E. coli using the Red/ET recombineering technique (Wang et al., 2018). The resultant plasmid p15A-may with the oriT-attP-phiC31 cassette was transferred and integrated into the target chromosome of S. albus J1074 to obtain S. albus J1074/may. The correct transformants were cultivated in the R5MS liquid medium for metabolic analysis.
Using crude extracts of wild-type S. albus J1074 and blank medium as negative controls, and purified mayamycin and mayamycin B as the positive control, LC-MS analysis revealed that introduction of BGC8.1 into S. albus J1074 led to the accumulation of mayamycin and mayamycin B at 11 and 10.9 min, respectively (Figure 10).
Due to the capability of producing therapeutically active chemicals, members of the genus Streptomyces have been given a lot of attention (Nguyen et al., 2020). Exploring Streptomyces species in new environments has resulted in the discovery of novel species and new secondary metabolites. The majority of Streptomyces were able to produce newly identified active metabolites originating from marine habitats and harsh environments (Riahi et al., 2019). The identification of novel microbial compounds has been dependent on the quantity and variety of isolated and screened strains. In our work here, the isolate CS-7 found in an unexplored mountain habitat showed a high level of inhibitory activity against a variety of Gram-positive and Gram-negative pathogens in this investigation.
Bacteria of Streptomyces might synthesize more than 100,000 antimicrobial metabolites, although only a few are characterized (Watve et al., 2001). Uncovering novel natural product biosynthesis pathways using genomic-based bottom-up techniques has become a popular research topic (Winter et al., 2011).
Here, bioinformatic analyses of the whole genome sequence of Streptomyces strain CS-7 exposed numerous biosynthetic pathways. However, bioinformatics analysis uncovered numerous novel gene clusters in CS-7 that are not related to recognized clusters (Table 5). Then, more research in future will be needed to improve, isolate, and identify the new bio-active molecules from CS-7.
The genome sequence of CS-7 gives us a way to look at and study new natural products and we found that CS-7 produced potent mayamycin B and mayamycin. Mayamycin B and mayamycin as a member in rare C-glycosylated polyketides were previously reported from the Streptomyces sp. 120454 and marine Streptomyces sp. strain HB202 which were isolated from the marine sponge Halichondria panicea (Schneemann et al., 2010; Bo et al., 2018). But no genetic evidence were reported to support their production in these two strains. In our work here, we examined the genome of the Streptomyces strain CS-7, which has extraordinary biotechnological potential, with the goal of elucidating its functional characteristics. We confirmed a BGC (cluster 20, region 8.1) in the CS-7 genome for the production of mayamycins.
C-Glycosylation is a unique phenomenon in nature and is involved in the bioactivity of natural products. Only a small number of biologically important natural products are C-glycosides (Cai et al., 2021). In the mayamycin gene cluster, we predicted the presence of genes for a rare C-glycosyltransferase and biosynthesis of a rare deoxy-amino sugar (angolosamine), which will be confirmed functionally and used for combinatorial biosynthesis of glycosides, to enrich the structural and bioactivity diversification of these therapeutically important compounds.
For bacterial identification and classification, we employed both whole-genome sequences of Streptomyces sp. CS-7 and 16S rRNA-based phylogenetic trees, which sometimes are of too low resolution to discriminate between related taxa (Nouioui et al., 2018). The dDDH approaches using whole genome sequence comparisons produce superior quality data than experimental methods that are known to be costly, labor-intensive, and prone to experimental error, and are now well established in the scientific community (Stackebrandt et al., 2005; Rossello-Mora et al., 2011; Meier-Kolthoff et al., 2013b). Although both ways gave different results, we assume that the whole genome-based phylogeny should have more real evolutionary with its relatives.
The rise of drug-resistant bacterial infections has become a major worldwide issue, rendering therapy ineffective on a global scale. It is critical to develop efficient control mechanisms for the management and treatment of antibiotic resistance at the moment. Various Streptomyces strains have been shown to generate a variety of secondary metabolites that are active against a variety of microbiological diseases. However, only a handful of these compounds are known to engage in anti-S. aureus activity. Screening the crude extract of CS-7 (disk diffusion technique) revealed a substantial zone of inhibition against tested S. aureus bacteria, indicating that this isolate generates bioactive compounds. Genome mining and metabolite analyses suggest that the isolated CS-7 strain has considerable potential for the synthesis of secondary metabolites. Numerous genes implicated in antibiotic production had a high degree of similarity with previously identified genes, suggesting that CS-7 and related strains may be sources of economically viable secondary metabolites. AntiSMASH has been extensively utilized to discover biosynthetic gene clusters in a variety of organisms, including Streptomyces (Amin et al., 2019), but antiSMASH may have missed several BGCs. Further mass spectrometry-based genome mining may identify new metabolites and combinations of several genome mining approaches are expected to detect more BGCs in the genome of a certain microbial strain.
The data presented in this study are deposited in the Genbank repository, accession number JAJUKK000000000.
AL conceived the concept and funds, supervised the work, and validated the results. KA and JH conducted all experiments, analyzed the data, and wrote the original draft of the manuscript. KA, MI, and LZ conducted software. SI, GF, and QO conducted validation. HS conducted formal analysis. YZ and RL visualized and wrote and data analysis. All authors read and approved the manuscript.
This study was supported by the National Key R&D Program of China (2019YFA0905700), the National Natural Science Foundation of China (32170038 and 32270088), the Open Project Program of the State Key Laboratory of Bio-based Material and Green Papermaking (KF201825), and the 111 Project (B16030).
We thank Jingyao Qu, Zhifeng Li, and Jing Zhu from the Analysis and Testing Center of SKLMT (State Key Laboratory of Microbial Technology, Shandong University) for assistance with HRESIMS and Haiyan Sui and Xiaoju Li from Shandong University Core Facilities for Life and Environmental Sciences for their help with the NMR.
The authors declare that the research was conducted in the absence of any commercial or financial relationships that could be construed as a potential conflict of interest.
All claims expressed in this article are solely those of the authors and do not necessarily represent those of their affiliated organizations, or those of the publisher, the editors and the reviewers. Any product that may be evaluated in this article, or claim that may be made by its manufacturer, is not guaranteed or endorsed by the publisher.
The Supplementary Material for this article can be found online at: https://www.frontiersin.org/articles/10.3389/fmicb.2022.939919/full#supplementary-material
Alam, K., Hao, J., Zhang, Y., and Li, A. (2021). Synthetic biology-inspired strategies and tools for engineering of microbial natural product biosynthetic pathways. Biotechnol. Adv. 49:107759. doi: 10.1016/j.biotechadv.2021.107759
Alberti, F., Leng, D. J., Wilkening, I., Song, L., Tosin, M., and Corre, C. (2019). Triggering the expression of a silent gene cluster from genetically intractable bacteria results in scleric acid discovery. Chem. Sci. 10, 453–463. doi: 10.1039/c8sc03814g
Altschul, S. F., Gish, W., Miller, W., Myers, E. W., and Lipman, D. J. (1990). Basic local alignment search tool. J. Mol. Biol. 215, 403–410.
Amin, D. H., Abolmaaty, A., Borsetto, C., Tolba, S., Abdallah, N. A., and Wellington, E. M. H. (2019). In silico genomic mining reveals unexplored bioactive potential of rare actinobacteria isolated from Egyptian soil. Bull. Natl. Res. Cent. 43, 1–9.
Baltz, R. H. (2019). Natural product drug discovery in the genomic era: Realities, conjectures, misconceptions, and opportunities. J. Ind. Microbiol. Biotechnol. 46, 281–299. doi: 10.1007/s10295-018-2115-4
Benson, D. A., Cavanaugh, M., Clark, K., Karsch-Mizrachi, I., Lipman, D. J., Ostell, J., et al. (2013). GenBank. Nucleic Acids Res. 41, D36–D42.
Blin, K., Shaw, S., Kloosterman, A. M., Charlop-Powers, Z., van Wezel, G. P., Medema, M. H., et al. (2021). antiSMASH 6.0: Improving cluster detection and comparison capabilities. Nucleic Acids Res. 49, W29–W35. doi: 10.1093/nar/gkab335
Bo, S. T., Xu, Z. F., Yang, L., Cheng, P., Tan, R. X., Jiao, R. H., et al. (2018). Structure and biosynthesis of mayamycin B, a new polyketide with antibacterial activity from Streptomyces sp. 120454. J. Antibiot. 71, 601–605. doi: 10.1038/s41429-018-0039-x
Boetzer, M., Henkel, C. V., Jansen, H. J., Butler, D., and Pirovano, W. (2011). Scaffolding pre-assembled contigs using SSPACE. Bioinformatics 27, 578–579.
Boetzer, M., and Pirovano, W. (2012). Toward almost closed genomes with GapFiller. Genome Biol. 13:R56. doi: 10.1186/gb-2012-13-6-r56
Cai, X., Taguchi, T., Wang, H., Yuki, M., Tanaka, M., Gong, K., et al. (2021). Identification of a C-glycosyltransferase involved in medermycin biosynthesis. ACS Chem. Biol. 16, 1059–1069. doi: 10.1021/acschembio.1c00227
Chevrette, M. G., Carlson, C. M., Ortega, H. E., Thomas, C., Ananiev, G. E., Barns, K. J., et al. (2019). The antimicrobial potential of Streptomyces from insect microbiomes. Nat. Commun. 10:516. doi: 10.1038/s41467-019-08438-0
Chevrette, M. G., and Currie, C. R. (2019). Emerging evolutionary paradigms in antibiotic discovery. J. Ind. Microbiol. Biotechnol. 46, 257–271.
Churchill, G. A. (1989). Stochastic models for heterogeneous DNA sequences. Bull. Math. Biol. 51, 79–94.
Delcher, A. L., Bratke, K. A., Powers, E. C., and Salzberg, S. L. (2007). Identifying bacterial genes and endosymbiont DNA with glimmer. Bioinformatics 23, 673–679. doi: 10.1093/bioinformatics/btm009
Fajardo, A., and Martínez, J. L. (2008). Antibiotics as signals that trigger specific bacterial responses. Curr. Opin. Microbiol. 11, 161–167.
Felsenstein, J. (1981). Evolutionary trees from DNA sequences: A maximum likelihood approach. J. Mol. Evol. 17, 368–376.
Finn, R. D., Bateman, A., Clements, J., Coggill, P., Eberhardt, R. Y., Eddy, S. R., et al. (2014). Pfam: The protein families database. Nucleic Acids Res. 42, D222–D230.
Firn, R. D., and Jones, C. G. (1996). “An explanation of secondary product ‘redundancy’,” in Phytochemical diversity and redundancy in ecological interactions, eds J. T. Romeo, J. A. Saunders, and P. Barbosa (Boston, MA: Springer), 295–312. doi: 10.1128/JVI.63.12.5006-5012.1989
Fu, J., Bian, X., Hu, S., Wang, H., Huang, F., Seibert, P. M., et al. (2012). Full-length RecE enhances linear-linear homologous recombination and facilitates direct cloning for bioprospecting. Nat. Biotechnol. 30, 440–446. doi: 10.1038/nbt.2183
Galanie, S., Entwistle, D., and Lalonde, J. (2020). Engineering biosynthetic enzymes for industrial natural product synthesis. Nat. Prod. Rep. 37, 1122–1143.
Gene Ontology Consortium (2004). The Gene Ontology (GO) database and informatics resource. Nucleic Acids Res. 32, D258–D261.
Genilloud, O. (2014). The re-emerging role of microbial natural products in antibiotic discovery. Antonie Van Leeuwenhoek 106, 173–188. doi: 10.1007/s10482-014-0204-6
Goris, J., Konstantinidis, K. T., Klappenbach, J. A., Coenye, T., Vandamme, P., and Tiedje, J. M. (2007). DNA–DNA hybridization values and their relationship to whole-genome sequence similarities. Int. J. Syst. Evol. Microbiol. 57, 81–91.
Grant, J. R., and Stothard, P. (2008). The CGView server: A comparative genomics tool for circular genomes. Nucleic Acids Res. 36, W181–W184. doi: 10.1093/nar/gkn179
Gulder, T. A. M., and Moore, B. S. (2009). Chasing the treasures of the sea—bacterial marine natural products. Curr. Opin. Microbiol. 12, 252–260. doi: 10.1016/j.mib.2009.05.002
Ha, S.-M., Kim, C. K., Roh, J., Byun, J.-H., Yang, S.-J., Choi, S.-B., et al. (2019). Application of the whole genome-based bacterial identification system, TrueBac ID, using clinical isolates that were not identified with three matrix-assisted laser desorption/ionization time-of-flight mass spectrometry (MALDI-TOF MS) systems. Ann. Lab. Med. 39, 530–536. doi: 10.3343/alm.2019.39.6.530
Hahnke, R. L., Meier-Kolthoff, J. P., García-López, M., Mukherjee, S., Huntemann, M., Ivanova, N. N., et al. (2016). Genome-based taxonomic classification of Bacteroidetes. Front. Microbiol. 7:2003. doi: 10.3389/fmicb.2016.02003
Hammami, R., Zouhir, A., Le Lay, C., Ben Hamida, J., and Fliss, I. (2010). BACTIBASE second release: A database and tool platform for bacteriocin characterization. BMC Microbiol. 10:22. doi: 10.1186/1471-2180-10-22
Hoshino, S., Onaka, H., and Abe, I. (2019). Activation of silent biosynthetic pathways and discovery of novel secondary metabolites in actinomycetes by co-culture with mycolic acid-containing bacteria. J. Ind. Microbiol. Biotechnol. 46, 363–374.
Hudzicki, J. (2009). Kirby-Bauer disk diffusion susceptibility test protocol. Am. Soc. Microbiol. 15, 55–63.
Hunt, M., Newbold, C., Berriman, M., and Otto, T. D. (2014). A comprehensive evaluation of assembly scaffolding tools. Genome Biol. 15:R42.
Hutchings, M. I., Truman, A. W., and Wilkinson, B. (2019). Antibiotics: Past, present and future. Curr. Opin. Microbiol. 51, 72–80.
Hwang, S., Lee, N., Jeong, Y., Lee, Y., Kim, W., Cho, S., et al. (2019). Primary transcriptome and translatome analysis determines transcriptional and translational regulatory elements encoded in the Streptomyces clavuligerus genome. Nucleic Acids Res. 47, 6114–6129. doi: 10.1093/nar/gkz471
Ikeda, H., Shin-ya, K., and Omura, S. (2014). Genome mining of the Streptomyces avermitilis genome and development of genome-minimized hosts for heterologous expression of biosynthetic gene clusters. J. Ind. Microbiol. Biotechnol. 41, 233–250. doi: 10.1007/s10295-013-1327-x
Jones, S. E., and Elliot, M. A. (2017). Streptomyces exploration: Competition, volatile communication and new bacterial behaviours. Trends Microbiol. 25, 522–531. doi: 10.1016/j.tim.2017.02.001
Kanehisa, M., and Goto, S. (2000). KEGG: Kyoto encyclopedia of genes and genomes. Nucleic Acids Res. 28, 27–30.
Katz, L., and Baltz, R. H. (2016). Natural product discovery: Past, present, and future. J. Ind. Microbiol. Biotechnol. 43, 155–176.
Kennedy, J., Baker, P., Piper, C., Cotter, P. D., Walsh, M., Mooij, M. J., et al. (2009). Isolation and analysis of bacteria with antimicrobial activities from the marine sponge Haliclona simulans collected from Irish waters. Mar. Biotechnol. 11, 384–396. doi: 10.1007/s10126-008-9154-1
Kumar, P. S., Al-Dhabi, N. A., Duraipandiyan, V., Balachandran, C., Kumar, P. P., and Ignacimuthu, S. (2014). In vitro antimicrobial, antioxidant and cytotoxic properties of Streptomyces lavendulae strain SCA5. BMC Microbiol. 14:291. doi: 10.1186/s12866-014-0291-6
Kumar, S., Stecher, G., Li, M., Knyaz, C., and Tamura, K. (2018). MEGA X: Molecular evolutionary genetics analysis across computing platforms. Mol. Biol. Evol. 35, 1547–1549. doi: 10.1093/molbev/msy096
Lagesen, K., Hallin, P., Rødland, E. A., Stærfeldt, H.-H., Rognes, T., and Ussery, D. W. (2007). RNAmmer: Consistent and rapid annotation of ribosomal RNA genes. Nucleic Acids Res. 35, 3100–3108. doi: 10.1093/nar/gkm160
Lee, N., Hwang, S., Lee, Y., Cho, S., Palsson, B., and Cho, B.-K. (2019). Synthetic biology tools for novel secondary metabolite discovery in Streptomyces. J. Microbiol. Biotechnol. 29, 667–686. doi: 10.4014/jmb.1904.04015
Lefort, V., Desper, R., and Gascuel, O. (2015). FastME 2.0: A comprehensive, accurate, and fast distance-based phylogeny inference program. Mol. Biol. Evol. 32, 2798–2800. doi: 10.1093/molbev/msv150
Li, L., Ma, T., Liu, Q., Huang, Y., Hu, C., and Liao, G. (2013). Improvement of daptomycin production in Streptomyces roseosporus through the acquisition of pleuromutilin resistance. Biomed Res. Int. 2013:479742. doi: 10.1155/2013/479742
Li, Y., Zhang, C., Liu, C., Ju, J., and Ma, J. (2018). Genome sequencing of Streptomyces atratus SCSIOZH16 and activation production of nocardamine via metabolic engineering. Front. Microbiol. 9:1269. doi: 10.3389/fmicb.2018.01269
Liu, C., Bayer, A., Cosgrove, S. E., Daum, R. S., Fridkin, S. K., Gorwitz, R. J., et al. (2011). Clinical practice guidelines by the Infectious Diseases Society of America for the treatment of methicillin-resistant Staphylococcus aureus infections in adults and children. Clin. Infect. Dis. 52, e18–e55.
Lo Giudice, A., Bruni, V., and Michaud, L. (2007). Characterization of Antarctic psychrotrophic bacteria with antibacterial activities against terrestrial microorganisms. J. Basic Microbiol. 47, 496–505. doi: 10.1002/jobm.200700227
Lowe, T. M., and Eddy, S. R. (1997). tRNAscan-SE: A program for improved detection of transfer RNA genes in genomic sequence. Nucleic Acids Res. 25, 955–964.
Luo, Y., Cobb, R. E., and Zhao, H. (2014). Recent advances in natural product discovery. Curr. Opin. Biotechnol. 30, 230–237.
Machado, H., Sonnenschein, E. C., Melchiorsen, J., and Gram, L. (2015). Genome mining reveals unlocked bioactive potential of marine Gram-negative bacteria. BMC Genomics 16:158. doi: 10.1186/s12864-015-1365-z
Masand, M., Sivakala, K. K., Menghani, E., Thinesh, T., Anandham, R., Sharma, G., et al. (2018). Biosynthetic potential of bioactive streptomycetes isolated from arid region of the Thar desert, Rajasthan (India). Front. Microbiol. 9:687. doi: 10.3389/fmicb.2018.00687
Medema, M. H., Kottmann, R., Yilmaz, P., Cummings, M., Biggins, J. B., Blin, K., et al. (2015). Minimum information about a biosynthetic gene cluster. Nat. Chem. Biol. 11, 625–631.
Meier-Kolthoff, J. P., and Göker, M. (2019). TYGS is an automated high-throughput platform for state-of-the-art genome-based taxonomy. Nat. Commun. 10:2182. doi: 10.1038/s41467-019-10210-3
Meier-Kolthoff, J. P., Auch, A. F., Klenk, H.-P., and Göker, M. (2013a). Genome sequence-based species delimitation with confidence intervals and improved distance functions. BMC Bioinformatics 14:60. doi: 10.1186/1471-2105-14-60
Meier-Kolthoff, J. P., Göker, M., Spröer, C., and Klenk, H.-P. (2013b). When should a DDH experiment be mandatory in microbial taxonomy? Arch. Microbiol. 195, 413–418. doi: 10.1007/s00203-013-0888-4
Nakaew, N., Lumyong, S., Sloan, W. T., and Sungthong, R. (2019). Bioactivities and genome insights of a thermotolerant antibiotics-producing Streptomyces sp. TM32 reveal its potentials for novel drug discovery. Microbiologyopen 8, e842. doi: 10.1002/mbo3.842
Nett, M., Ikeda, H., and Moore, B. S. (2009). Genomic basis for natural product biosynthetic diversity in the actinomycetes. Nat. Prod. Rep. 26, 1362–1384.
Newman, D. J., and Cragg, G. M. (2020). Natural products as sources of new drugs over the nearly four decades from 01/1981 to 09/2019. J. Nat. Prod. 83, 770–803. doi: 10.1021/acs.jnatprod.9b01285
Nguyen, C. T., Dhakal, D., Pham, V. T. T., Nguyen, H. T., and Sohng, J.-K. (2020). Recent advances in strategies for activation and discovery/characterization of cryptic biosynthetic gene clusters in Streptomyces. Microorganisms 8:616. doi: 10.3390/microorganisms8040616
Nouioui, I., Carro, L., García-López, M., Meier-Kolthoff, J. P., Woyke, T., Kyrpides, N. C., et al. (2018). Genome-based taxonomic classification of the phylum Actinobacteria. Front. Microbiol. 9:2007. doi: 10.3389/fmicb.2018.02007
Núñez-Montero, K., Lamilla, C., Abanto, M., Maruyama, F., Jorquera, M. A., Santos, A., et al. (2019). Antarctic Streptomyces fildesensis So13.3 strain as a promising source for antimicrobials discovery. Sci. Rep. 9:7488. doi: 10.1038/s41598-019-43960-7
Ômura, S., Ikeda, H., Ishikawa, J., Hanamoto, A., Takahashi, C., Shinose, M., et al. (2001). Genome sequence of an industrial microorganism Streptomyces avermitilis: Deducing the ability of producing secondary metabolites. Proc. Natl. Acad. Sci. U.S.A. 98, 12215–12220. doi: 10.1073/pnas.211433198
O’Neill, J. (2014). Antimicrobial resistance: Tackling a crisis for the health and wealth of nations. The review on antimicrobial resistance, Vol. 20. London: Wellcome Trust, 1–16.
Pan, R., Bai, X., Chen, J., Zhang, H., and Wang, H. (2019). Exploring structural diversity of microbe secondary metabolites using OSMAC strategy: A literature review. Front. Microbiol. 10:294. doi: 10.3389/fmicb.2019.00294
Ramasamy, D., Mishra, A. K., Lagier, J.-C., Padhmanabhan, R., Rossi, M., Sentausa, E., et al. (2014). A polyphasic strategy incorporating genomic data for the taxonomic description of novel bacterial species. Int. J. Syst. Evol. Microbiol. 64, 384–391.
Rebets, Y., Brötz, E., Tokovenko, B., and Luzhetskyy, A. (2014). Actinomycetes biosynthetic potential: How to bridge in silico and in vivo? J. Ind. Microbiol. Biotechnol. 41, 387–402. doi: 10.1007/s10295-013-1352-9
Reva, O., and Tümmler, B. (2008). Think big–giant genes in bacteria. Environ. Microbiol. 10, 768–777. doi: 10.1111/j.1462-2920.2007.01500.x
Riahi, K., Hosni, K., Raies, A., and Oliveira, R. (2019). Unique secondary metabolites of a Streptomyces strain isolated from extreme salty wetland show antioxidant and antibacterial activities. J. Appl. Microbiol. 127, 1727–1740. doi: 10.1111/jam.14428
Rodríguez, H., Rico, S., Díaz, M., and Santamaría, R. I. (2013). Two-component systems in Streptomyces: Key regulators of antibiotic complex pathways. Microb. Cell Fact. 12:127. doi: 10.1186/1475-2859-12-127
Rossello-Mora, R., Urdiain, M., and Lopez-Lopez, A. (2011). “DNA–DNA hybridization,” in Methods in microbiology, eds F. Rainey and A. Oren (Amsterdam: Elsevier), 325–347.
Saitou, N., and Nei, M. (1987). The neighbor-joining method: A new method for reconstructing phylogenetic trees. Mol. Biol. Evol. 4, 406–425.
Schneemann, I., Kajahn, I., Ohlendorf, B., Zinecker, H., Erhard, A., Nagel, K., et al. (2010). Mayamycin, a cytotoxic polyketide from a Streptomyces strain isolated from the marine sponge Halichondria panicea. J. Nat. Prod. 73, 1309–1312. doi: 10.1021/np100135b
Sivalingam, P., Hong, K., Pote, J., and Prabakar, K. (2019). Extreme environment Streptomyces: Potential sources for new antibacterial and anticancer drug leads? Int. J. Microbiol. 2019:5283948. doi: 10.1155/2019/5283948
Skinnider, M. A., Johnston, C. W., Gunabalasingam, M., Merwin, N. J., Kieliszek, A. M., MacLellan, R. J., et al. (2020). Comprehensive prediction of secondary metabolite structure and biological activity from microbial genome sequences. Nat. Commun. 11:6058. doi: 10.1038/s41467-020-19986-1
Stackebrandt, E., Van de Peer, Y., Vandamme, P., Thompson, F. L., and Swings, J. (2005). Re-evaluating prokaryotic species. Nat. Rev. Microbiol. 3, 733–739. doi: 10.1038/nrmicro1236
Stanke, M., Schöffmann, O., Morgenstern, B., and Waack, S. (2006). Gene prediction in eukaryotes with a generalized hidden Markov model that uses hints from external sources. BMC Bioinformatics 7:62. doi: 10.1186/1471-2105-7-62
Stulberg, E. R., Lozano, G. L., Morin, J. B., Park, H., Baraban, E. G., Mlot, C., et al. (2016). Genomic and secondary metabolite analyses of Streptomyces sp. 2AW provide insight into the evolution of the cycloheximide pathway. Front. Microbiol. 7:573. doi: 10.3389/fmicb.2016.00573
Tchize Ndejouong, B. L. S., Sattler, I., Maier, A., Kelter, G., Menzel, K.-D., Fiebig, H.-H., et al. (2010). Hygrobafilomycin, a cytotoxic and antifungal macrolide bearing a unique monoalkylmaleic anhydride moiety, from Streptomyces varsoviensis. J. Antibiot. 63, 359–363. doi: 10.1038/ja.2010.52
Terra, L., Dyson, P. J., Hitchings, M. D., Thomas, L., Abdelhameed, A., Banat, I. M., et al. (2018). A novel alkaliphilic Streptomyces inhibits ESKAPE pathogens. Front. Microbiol. 9:2458. doi: 10.3389/fmicb.2018.02458
Tiwari, K., and Gupta, R. K. (2012). Rare actinomycetes: A potential storehouse for novel antibiotics. Crit. Rev. Biotechnol. 32, 108–132. doi: 10.3109/07388551.2011.562482
Toner, E., Adalja, A., Gronvall, G. K., Cicero, A., and Inglesby, T. V. (2015). Antimicrobial resistance is a global health emergency. Heal. Secur. 13, 153–155.
UniProt Consortium (2015). UniProt: A hub for protein information. Nucleic Acids Res. 43, D204–D212.
van Heel, A. J., de Jong, A., Song, C., Viel, J. H., Kok, J., and Kuipers, O. P. (2018). BAGEL4: A user-friendly web server to thoroughly mine RiPPs and bacteriocins. Nucleic Acids Res. 46, W278–W281. doi: 10.1093/nar/gky383
Waghu, F. H., Barai, R. S., Gurung, P., and Idicula-Thomas, S. (2016). CAMPR3: A database on sequences, structures and signatures of antimicrobial peptides. Nucleic Acids Res. 44, D1094–D1097.
Wang, H., Li, Z., Jia, R., Yin, J., Li, A., Xia, L., et al. (2018). ExoCET?: Exonuclease in vitro assembly combined with RecET recombination for highly efficient direct DNA cloning from complex genomes. Nucleic Acids Res. 46:2697. doi: 10.1093/nar/gkx1296
Watve, M. G., Tickoo, R., Jog, M. M., and Bhole, B. D. (2001). How many antibiotics are produced by the genus Streptomyces? Arch. Microbiol. 176, 386–390.
Wayne, L. G., Brenner, D. J., Colwell, R. R., Grimont, P. A. D., Kandler, O., Krichevsky, M. I., et al. (1987). Report of the ad hoc committee on reconciliation of approaches to bacterial systematics. Int. J. Syst. Evol. Microbiol. 37, 463–464.
Winter, J. M., Behnken, S., and Hertweck, C. (2011). Genomics-inspired discovery of natural products. Curr. Opin. Chem. Biol. 15, 22–31. doi: 10.1016/j.cbpa.2010.10.020
Yoon, S.-H., Ha, S.-M., Kwon, S., Lim, J., Kim, Y., Seo, H., et al. (2017a). Introducing EzBioCloud: A taxonomically united database of 16S rRNA gene sequences and whole-genome assemblies. Int. J. Syst. Evol. Microbiol. 67, 1613–1617. doi: 10.1099/ijsem.0.001755
Yoon, S.-H., Ha, S.-M., Lim, J., Kwon, S., and Chun, J. (2017b). A large-scale evaluation of algorithms to calculate average nucleotide identity. Antonie Van Leeuwenhoek 110, 1281–1286.
Zerbino, D. R., and Birney, E. (2008). Velvet: Algorithms for de novo short read assembly using de Bruijn graphs. Genome Res. 18, 821–829. doi: 10.1101/gr.074492.107
Zerbino, D. R., McEwen, G. K., Margulies, E. H., and Birney, E. (2009). Pebble and rock band: Heuristic resolution of repeats and scaffolding in the velvet short-read de novo assembler. PLoS One 4:e8407. doi: 10.1371/journal.pone.0008407
Ziemert, N., Alanjary, M., and Weber, T. (2016). The evolution of genome mining in microbes–a review. Nat. Prod. Rep. 33, 988–1005. doi: 10.1039/c6np00025h
Keywords: Streptomyces, natural products, biosynthetic gene cluster, genome mining, mayamycin
Citation: Alam K, Hao J, Zhong L, Fan G, Ouyang Q, Islam MM, Islam S, Sun H, Zhang Y, Li R and Li A (2022) Complete genome sequencing and in silico genome mining reveal the promising metabolic potential in Streptomyces strain CS-7. Front. Microbiol. 13:939919. doi: 10.3389/fmicb.2022.939919
Received: 09 May 2022; Accepted: 05 September 2022;
Published: 05 October 2022.
Edited by:
Marko Jukic, University of Maribor, SloveniaReviewed by:
Hua Yuan, Shanghai Normal University, ChinaCopyright © 2022 Alam, Hao, Zhong, Fan, Ouyang, Islam, Islam, Sun, Zhang, Li and Li. This is an open-access article distributed under the terms of the Creative Commons Attribution License (CC BY). The use, distribution or reproduction in other forums is permitted, provided the original author(s) and the copyright owner(s) are credited and that the original publication in this journal is cited, in accordance with accepted academic practice. No use, distribution or reproduction is permitted which does not comply with these terms.
*Correspondence: Aiying Li, YXlsaUBzZHUuZWR1LmNu; Ruijuan Li, bGlydWlqdWFuQHNkdS5lZHUuY24=
†These authors have contributed equally to this work
Disclaimer: All claims expressed in this article are solely those of the authors and do not necessarily represent those of their affiliated organizations, or those of the publisher, the editors and the reviewers. Any product that may be evaluated in this article or claim that may be made by its manufacturer is not guaranteed or endorsed by the publisher.
Research integrity at Frontiers
Learn more about the work of our research integrity team to safeguard the quality of each article we publish.