- 1Sorbonne Université, CNRS, Institut de Biologie Paris-Seine, UMR 7238, Laboratoire de Biologie Computationnelle et Quantitative, Paris, France
- 2École Normale Supérieure, PSL Research University, CNRS, Inserm U1024, Institut de Biologie de l’École Normale Supérieure, Plateforme Génomique, Paris, France
- 3Sorbonne Université, CNRS, Institut de Biologie Paris-Seine, UMR 7138, Évolution, Paris, France
- 4UMR 9198, Institute for Integrative Biology of the Cell, CEA, CNRS, Université Paris-Sud, UPSay, Gif-sur-Yvette, France
In this work, we used comparative transcriptomics to identify regulatory outliers (ROs) in the human pathogen Candida glabrata. ROs are genes that have very different expression patterns compared to their orthologs in other species. From comparative transcriptome analyses of the response of eight yeast species to toxic doses of selenite, a pleiotropic stress inducer, we identified 38 ROs in C. glabrata. Using transcriptome analyses of C. glabrata response to five different stresses, we pointed out five ROs which were more particularly responsive to iron starvation, a process which is very important for C. glabrata virulence. Global chromatin Immunoprecipitation and gene profiling analyses showed that four of these genes are actually new targets of the iron starvation responsive Aft2 transcription factor in C. glabrata. Two of them (HBS1 and DOM34b) are required for C. glabrata optimal growth in iron limited conditions. In S. cerevisiae, the orthologs of these two genes are involved in ribosome rescue by the NO GO decay (NGD) pathway. Hence, our results suggest a specific contribution of NGD co-factors to the C. glabrata adaptation to iron starvation.
Introduction
Candidemia are systemic infections caused by different Candida yeast species. They are responsible for high mortality rates (40–50%) in immunocompromised patients, despite the existing treatments (Pfaller and Diekema, 2007). These last 20 years, Candida glabrata has become the second leading cause of candidemia, behind the extensively studied Candida albicans (Pfaller et al., 2014). Although they have similar names, C. glabrata and C. albicans are very different species (Brunke and Hube, 2013). C. glabrata, in evolutionary terms, is more closely related to the model yeast Saccharomyces cerevisiae than to C. albicans (Dujon et al., 2004). It actually belongs to the Nakaseomyces clade. In contrast to C. albicans, C. glabrata is a haploid. It is less susceptible to the azole compounds which are commonly used to treat candidemia and can rapidly develop high-level resistance (Pfaller and Diekema, 2007). Moreover, it has evolved distinct invasive strategies and unique transcriptional responses to stress compared to other pathogenic fungi. For instance, Candida glabrata is able to survive and multiply in macrophages by escaping or inhibiting most of the phagolysosome anti-microbial weapons (Kaur et al., 2007; Seider et al., 2011, 2014; Kasper et al., 2015). Identifying the specificities of C. glabrata is therefore a key issue to understand its virulence and eventually find efficient treatments.
One obvious way to find C. glabrata particularities is comparative genomics (Gabaldon and Carrete, 2015). The comparison of C. glabrata and S. cerevisiae genomes indicated that C. glabrata has lost some genes involved in galactose, phosphate, nitrogen, and sulfur metabolisms (Roetzer et al., 2011). These gene losses resulted in auxotrophy for nicotinic acid, pyridoxine, and thiamine (Dujon et al., 2004; Domergue et al., 2005). These features were hypothesized to be related to the pathogenic nature of C. glabrata. However, the sequencing of five more Nakaseomyces species, including two species found in human patients and three, non-pathogenic, environmental species, showed that most of these changes were actually shared by both pathogenic and non-pathogenic Nakaseomyces species (Gabaldon et al., 2013). This study identified the amplification of the EPA genes, which encode for glycosyl-phosphatidylinositol (GPI)-anchored cell wall proteins involved in cell adhesion, stress responses and recognition by the innate immune system (Cormack et al., 1999; De Las Penas et al., 2003, 2015; Domergue et al., 2005; Juarez-Cepeda et al., 2015; Vitenshtein et al., 2016) as the main genomic feature correlating with virulence in this clade (Gabaldon et al., 2013; Gabaldon and Carrete, 2015).
Besides gene gains and losses, phenotypic diversity can also arise from gene regulation divergence (Romero et al., 2012; Roy and Thompson, 2015; Thompson et al., 2015; Johnson, 2017). Hence, numerous cases have been described in which changes in the cis- or trans- regulatory elements of otherwise conserved genes can lead to the emergence of new functions (Romero et al., 2012; Thompson et al., 2015). For instance, the evolution of pregnancy in mammals was associated with transcriptional network rewiring driven by transposable elements (Lynch et al., 2011, 2015). In yeasts, the loss of an AT rich cis-regulatory element in the promoters of oxidative phosphorylation and mitochondrial ribosomal protein genes following a Whole Genome Duplication event (WGD) allowed for the appearance of a respiro-fermentative life style in extent post-WGD species (Ihmels et al., 2005; Habib et al., 2012; Thompson et al., 2013). Comparative transcriptomics (i.e., the comparison of gene expression profiles in different species) has been extensively used in yeasts to identify changes in gene regulation that accompanied the appearance of new physiological properties (Ihmels et al., 2005; Lavoie et al., 2010; Wapinski et al., 2010), to achieve model phylogeny for regulatory evolution (Roy et al., 2013; Thompson et al., 2013) or to predict transcriptional regulatory networks in non-model species (Koch et al., 2017). In the present work, we used comparative transcriptomics to identify regulatory outliers (ROs) in C. glabrata and in seven other yeast species. ROs are genes that have very different expression profiles from their orthologs in the other species. To find them, we designed REGULOUT, a program which automatically identifies genes with unique profiles among their group of orthologs (i.e., orthogroups). We applied REGULOUT to comparative transcriptome analyses of the response of eight yeast species to toxic doses of selenite, a pleiotropic stress inducer. From these data, REGULOUT identified 38 ROs in C. glabrata. Using transcriptome analyses of the C. glabrata response to five different stresses, we pointed out five C. glabrata ROs which were more particularly responsive to iron starvation, a process which is very important for C. glabrata virulence (Nevitt and Thiele, 2011; Srivastava et al., 2014). Global chromatin Immunoprecipitation (ChIP-seq) and gene profiling analyses showed that these five genes were under the control of the iron starvation responsive transcription factor Aft2 and that four of them were actually C. glabrata specific Aft2 targets as compared to S. cerevisiae. Phylogenetic analyses of the promoter sequences of these four genes suggest that their control by Aft2 was fixed after the WGD. Interestingly, the amount of Aft motifs in the promoters of those genes was particularly high in the Nakaseomyces sub-clade including the three potentially pathogenic species sequenced to date (namely C. glabrata, Candida bracarensis and Candida nivariensis), as compared with the non-pathogenic Nakaseomyces sub-clade or with the Saccharomyces genus. Among these four genes, two (HBS1 and DOM34b) were required for optimal growth of C. glabrata in iron limited conditions. In S. cerevisiae, the orthologs of these two genes are involved in ribosome rescue by the NO GO decay (NGD) pathway. Hence, our results demonstrate the power of comparative functional genomics to identify novel regulatory systems in non-model species and suggest a specific contribution of NGD co-factors to the C. glabrata strategy for its adaptation to iron starvation.
Materials and Methods
Strains and Growth Conditions
For comparative transcriptomic analyses, we used the following strains: Saccharomyces cerevisiae S288C, Candida glabrata CBS138, Lachancea kluyveri CBS3082, Lachancea thermotolerans CBS6340, Kluyveromyces lactis CBS2359, Candida albicans SC5315, Debaryomyces hansenii CBS767, Yarrowia lipolytica CLIB122. All strains were grown in rich media at 30°C (YPD: 1% bacto peptone, 1% yeast extract, 2% glucose) on a rotating shaker (150 rpm), except the halophilic yeast D. hansenii which was grown in YPD supplemented with 0.5 M NaCl.
For C. glabrata mutant strain construction, we used the HTL background (his3-, trp1-, leu2-) (Schwarzmuller et al., 2014). The tagging of DOM34a, DOM34b, HBS1, and MAK16b with TAP tag was performed by PCR and homologous recombination as described in Merhej et al. (2015). The tagging cassette was PCR amplified from the pBS1479 plasmid (Puig et al., 2001). The tagging of AFT2 with a myc epitope was performed exactly as described (Merhej et al., 2015) using the myc-His cassette from the Longtine’s collection (Longtine et al., 1998). The AFT2 deleted strain was obtained from the Schwarzmuller et al. (2014) collection. The MAK16b deleted strain was obtained by PCR and homologous recombination as described previously (Merhej et al., 2015). The HBS1, DOM34a, and DOM34b deletion mutants were obtained by a two steps PCR process using large homologous regions as described previously (Schwarzmuller et al., 2014). For all deletions, we used the TRP1 deletion cassette from the Longtine’s collection (Longtine et al., 1998). For all constructs, the positive clones were selected by growth on CSM-TRP media, except for the AFT2-MYC tagged construct which was screened on CSM-HIS media (2% glucose, 0.67% yeast nitrogen base, recommended amounts of CSM-HIS or CSM-TRP from MP Bio). The proper insertion of the cassettes at the targeted genomic loci and the absence of wild type versions of the targeted gene was controlled by PCR. All primers used in this study are available in Supplementary File S1.
Multispecies Transcriptome Analyses: Basic Experimental Set Up and RNA Extractions
For each species, we first determined the dose of selenite required for a 100% decrease of the growth rate in exponential phase. The results were: 0.5 mM for C. albicans, D. hansenii, K. lactis, and L. kluyveri, 1 mM for C. glabrata, 5 mM for L. thermotolerans, 10 mM for S. cerevisiae and Y. lipolytica. Next, we performed kinetic experiments in which the cells were grown in YPD (or YPD + salt for D. hansenii as indicated above) at 30°C until they reach an optical density (OD) at 600 nm of 0.6–0.7. Then, we split the cultures in two: one sub-culture received a mock treatment while the second was treated by the indicated amount of selenite (sodium selenite from sigma, stock solution prepared at 0.2 M in water). This step defines time zero. Every 10 min from time 10 to time 80 min, 20 mL of each culture were collected and flash-frozen in 30 mL of cold (-80°C) absolute ethanol. The cells were centrifuged (5 min, 4,000 rpm), washed with cold (4°C) distilled sterilized water, centrifuged again and cell pellets were stored at -80°C. In parallel to sample collection, the OD of the two cultures was measured every 30 min for 3 h to assess the impact of the selenite treatment on the growth rate. For each species, 6–10 independent kinetic series were generated. Only the four series which were the closest to the 100% decrease of growth rate as compared to the mock treated cultures were used for RNA extraction and microarray analyses. RNAs were extracted using the RNeasy kit (Qiagen) following the protocol provided by the supplier. The quality of the RNA extracts was checked on agarose gels prior to reverse transcription.
Multispecies Transcriptome Analyses: Microarray Design, Microarray Experiments, Data Analyses
Agilent 8x60k custom microarrays were designed for each species (array express accession numbers: A-MEXP-2402, A-MEXP-2365, A-MTAB-642 to 647). For probes design, we used the Teolenn software version 2.0.1 (Jourdren et al., 2010). The genome sequences and ORFs positions used to create probe sequences were downloaded from the Genolevures website. Only the coding sequences were used for probe design, introns and intergenic regions were not considered. The masked genomes (i.e., without repeated sequences) required for Teolenn were created using RepeatMasker version open-3.2.8 with the “-pa 4 -species fungi -xsmall” arguments. The main features of the design are summarized in Supplementary File S2. There were no probe replicates on the arrays but each ORF was covered by eight different probes in average. For microarray experiments, 1 μg of total RNA was used for fluorescent cDNA synthesis according to the amino-allyl protocol (Merhej et al., 2015). The cDNAs were labeled with Cy3 and Cy5 and hybridization was performed as previously described (Merhej et al., 2015). Four biologically independent experiments were performed for each condition, using dye switch. After overnight hybridization and washing, the slides were scanned using a 2-micron Agilent microarray scanner. The images were analyzed using the feature extraction software (Agilent Technologies) and normalized using global LOESS (Lemoine et al., 2006). The mean of the four biological replicates was calculated. A gene was considered as being induced (repressed) if its mean Log2(fold change) value was more than 0.75 (less than -0.75) and if its expression variation was considered as being statistically significant using the LIMMA package with a cut-off p-value of 0.01 (Ritchie et al., 2015) for at least two consecutive time points. The complete microarray data are available at Array express database under the accession numbers: E-MTAB-7022, E-MTAB-7023, E-MTAB-7044 to 7047, E-MTAB-7049 and E-MTAB-7053. The processed dataset is available in Supplementary Table T1.
REGULOUT
REGULOUT is a python program which takes as an input a multispecies expression matrix and the repartition of the genes in orthogroups. There are two parameters which need to be set up by the user: the minimal size of the orthogroups to be used and the Euclidian distance cut-off to be applied. Then REGULOUT calculates, for each orthogroup reaching the defined minimal size, the pairwise distances between the expression profiles of the genes belonging to the orthogroup. In a next step, REGULOUT defines as ROs all the genes which minimal pairwise distance in their orthogroup is equal to or higher than the minimal distance cut-off. As an output, REGULOUT provides a text file with the name of the ROs, their minimal distance in their orthogroup, the ID number and the size of their orthogroup. It also generates automatically PNG files with the expression profiles of the orthogroups in which at least one RO has been identified. REGULOUT can be freely downloaded from www.lcqb.upmc.fr/REGULOUT/, together with a tutorial and the input data sets used in this study. More precisely, the input files used in this article were the expression matrix for all species and the composition of yeast orthogroups taken from the PhylomeDB database (Huerta-Cepas et al., 2014). The distribution of the sizes of the orthogroups is available in Supplementary File S3. The minimal size of the orthogroups to be used was set up to 8 to work mostly with genes which were conserved in all the eight yeast species that were considered. The impact of this filter on the number of expression profiles analyzed by REGULOUT is indicated in Supplementary File S2. The minimal distance cut-off was set up at 3, which corresponded to the 75 percentile of all the possible pairwise distances in the dataset. From the raw output of REGULOUT (440 genes), we filtered out the genes which were defined as ROs based on expression variations that were poorly reproducible (p-value > 0.01). Because the Euclidian distance that we used is very sensitive to stochastic variations for genes with a large amplitude in their expression changes, we also removed from the RO list the genes belonging to orthogroups in which all the members had the same directionality in their expression variations (see Supplementary File S4 for an illustration). The filtered REGULOUT output contained 289 ROs which are listed in Supplementary Table T2.
Multi-Stress Transcriptome Analyses in C. glabrata
All cultures were conducted in a rotating shaker at 30°C in YPD (glucose 2%, yeast extract 1%, bacto peptone 1%). Stress conditions used were: 1 mM for sodium selenite, 1 M for NaCl, 2 mM for cadmium chloride, 5 mM for iron sulfate or 0.5 mM for bathophenanthroline disulfonate (BPS). Stressed and mock-treated cells were collected as described above, 20 min and 40 min after treatment. RNA extractions, microarray hybridization and data normalization were performed as described above. For the aft2Δ versus wild type comparisons, the stress concentrations were the same as above but the cells were collected only 30 min after treatment. The complete microarray data are available at Array express database under the accession numbers: E-MTAB-7023, E-MTAB-7042, and E-MTAB-7043.
Chromatin Immunoprecipitation and High-Throughput Sequencing (ChIP-Seq)
For Aft2 ChIP experiments, myc-tagged strains were grown in YPD until exponential phase (OD = 0.8), they were then treated by 0.5 mM of BPS for 60 min. Cross-linking of the cells and ChIP were performed as described previously (Merhej et al., 2016). The parental HTL (untagged strain) was grown and processed the same way to provide the mock-IP samples. Sequencing of the IPs, Input DNAs and mock IPs samples and primary data analyses (quality controls and mapping of the reads) were performed as described previously (Merhej et al., 2016). Peak calling was performed with the bpeaks software, using both the Input DNA and the mock IP as references (Merhej et al., 2014). For peak calling using the Input DNA as reference, the bpeaks parameters were T1 = 1, T2 = 6, T3 = 1, T4 = 0.7. For peak calling using the Mock IP as reference, the bpeaks parameters were T1 = 1, T2 = 6, T3 = 1, and T4 = 0. Only the peaks that were found in both analyses were kept for further processing. These peaks were then manually checked on a genome browser (Thorvaldsdottir et al., 2013) to discard artifactual peaks (e.g., peaks centered on a tRNA locus, peaks perfectly overlapping a highly expressed ORF) which would have escaped the bpeaks filter. Peaks located outside of a promoter region (i.e., between convergent ORF or inside ORFs) were also discarded from the final list presented in Supplementary Table T3. For DNA motif prediction, DNA sequences of ChIP peaks were retrieved from their genomic locations (BED file) using the “getfasta” function from the BEDTOOLS suite (Quinlan and Hall, 2010). These genomic sequences were used as inputs for the peak-motif tool to search for regulatory motifs (Thomas-Chollier et al., 2012). An additional filtering step was added to the standard peak motif procedure to discard low complexity motifs (e.g., CCCCCCC). The list of Aft targets from S. cerevisiae was obtained from Yeastract (Teixeira et al., 2018) and completed by the “regulation” pages of the SGD1, taking all the documented regulatory interactions using DNA binding or expression evidences and keeping only the targets which were found in relevant growth conditions (i.e., metal stress conditions). The raw ChIP-seq data can be downloaded from the GEO database with the accession number GSE116077.
Bioinformatics Analyses: Gene Ontology, Hierarchical Clustering, Promoter Sequence Analyses
Gene ontology (GO) analyses were performed using the GO term finder tool at the Candida Genome Database2 for C. albicans, C. glabrata, and D. hansenii (with the names of the C. albicans orthologs), at the Saccharomyces Genome Database for S. cerevisiae, the two Lachancea species, K. lactis and Y. lipolytica (using the names of the S. cerevisiae orthologs). The hierarchical clustering of Figure 2 was performed using Mev with Euclidian distance, optimization of gene leaf order and average linkage. For phylogenetic analyses of the promoter sequences, we downloaded the promoter sequences (i.e., 500 base pairs upstream of the ATG) from the Genome Resources for Yeast Chromosomes database (GRYC3), except for the Saccharomyces sequences which were taken from the www.saccharomycessensustricto.org website (Scannell et al., 2011) and for the Kazachstania Africana sequences which were downloaded from the NCBI website. The sequences used can be found in Supplementary Files S5–S8.
Western Blot Analyses
The cells were grown in YPD at 30°C until they reach an OD at 600 nm of 0.6–0.7. Then, we split the cultures in two: one sub-culture received a mock treatment while the second was treated by 0.5 mM BPS. This step defines time zero. At 30, 60, and 90 min, 10 mL of each culture were collected and centrifuged (5 min, 4,000 rpm), washed with cold (4°C) distilled sterilized water, centrifuged again and cell pellets were stored at -80°C. Proteins were separated on 10% SDS-polyacrylamide gel electrophoresis (SDS-PAGE). Proteins were then transferred to Whatman® Protan® BA83 nitrocellulose membrane (GE Healthcare). Immunoblotting of the protein A-tagged proteins was performed with a rabbit IgG-HRP polyclonal antibody (PAP; code Z0113; Dako), which has a high affinity for Protein A. The membrane was stripped by boiling 30 min in 62.5 mM Tris-HCl pH 6.8, SDS 2% and 4 mM DTT, followed by 10 washes in PBS-Tween (0.1%). Then, immunoblotting of the ribosomal protein Rpl3, used as a loading and transfer control, was performed using 1:10000 rabbit IgG Anti-Rpl3 [gift from M. Garcia: refer to (Delaveau et al., 2016)] and 1:15000 anti-rabbit IgG-HRP (Promega) as primary and secondary antibodies, respectively. Detection of the signals was performed using G:BOX Chemi XT4 (Syngene) following incubation with UptiLightTM HRP blot chemiluminescent ECL substrate (Interchim).
Growth Assays
Growth assays were performed in 96 well plates using a TECAN Sunrise machine. Exponential phase growing cultures of the strains to be tested were diluted to OD = 0.1 in a 50 mL sterile Falcon tube. The 96 well plate was filled with 90 μL of the cultures. Then, 10 μL of BPS 0.5 mM stock solution (iron starvation conditions, final concentration 0.05 mM) or 10 μL of sterile water (mock treatment) were added to each well to reach a final culture volume of 100 μL. Cell growth at 30°C was followed for 24 h. The slope of the linear part of the log(OD) = f(t) curve was extracted and used as the growth rate of each culture in exponential phase. Two technical replicates were made in each plate.
Results
Identifying Regulatory Outliers From Comparative Transcriptomics Data
The starting point of this study consisted in analyzing and comparing the response of eight yeast species (S. cerevisiae, C. glabrata, L. kluyveri, L. thermotolerans, K. lactis, C. albicans, D. hansenii, and Y. lipolytica) to the stress caused by detrimental doses of selenite. These eight species were chosen because they span the whole Hemiascomycetes tree and because of their high-quality genome annotation at the time when we started the study (Genolevures et al., 2009). Selenite was chosen because it was shown to induce both iron starvation and oxidative stress responses in S. cerevisiae (Salin et al., 2008; Herrero and Wellinger, 2015), two stress conditions that C. glabrata is facing when invading the human body and being internalized by the cells of the innate immune system (Nevitt and Thiele, 2011; Brunke and Hube, 2013). A key challenge in comparative transcriptomics is to set up the growth conditions to make the physiological state of the different species as similar as possible and to minimize irrelevant differences in gene expression (Kuo et al., 2010; Thompson et al., 2013). To do so, we adjusted the selenite doses used for each species to obtain the same 100% increase in the generation time. Then, RNA samples were collected at eight time points after selenite exposure and compared to the RNAs extracted from a control culture mock-treated for the same duration using microarrays. Four replicates were performed for each species and each time point. Supplementary Table T1 provides the average log2 of the expression ratios between treated and untreated cells for each measured gene in each species for the eight time points, together with a statistical assessment of the significance of the gene expression variations.
As a posteriori validation of our approach, we examined the expression patterns of genes from the general Environmental Stress Response (ESR), which were shown to be highly conserved among the species we studied (Gasch et al., 2000, 2004; Gasch, 2007; Roy et al., 2013; Thompson et al., 2013). We observed that the induction of genes encoding proteasomal subunits and the repression of genes from the Ribosome Biogenesis (Ribi) regulon were consistent between the different species (Supplementary File S9). This was a good indication that the eight species were encountering physiologically similar stress conditions. To identify ROs from these 44,723 expression profiles, we designed a program called REGULOUT (Figure 1, left panel). REGULOUT takes as input files the expression profiles obtained in the different species and a table describing the orthology relationships between genes (i.e., the names of the genes composing each orthogroup). For each orthogroup taken independently, it calculates all the pairwise distances between the expression profiles of all the genes belonging to the orthogroup. Then, it looks for genes which minimal distance value in the orthogroup is higher than a distance cut-off set up by the user. Those genes with special expression profiles are the so-called “ROs.” The key parameters for REGULOUT are the minimal distance that the user accepts to define a RO and the minimal number of genes for an orthogroup to be considered in the analysis. We applied REGULOUT to our data with parameters corresponding to genes conserved in most of our yeast species (i.e., minimal orthogroup size = 8) and to a minimal distance cut-off equal to the 75 percentile of all the calculated pairwise distances (i.e., d = 3). With this criterion, we identified a total of 289 ROs in the eight species, ranging from 6 in L. thermotolerans up to 87 in C. albicans (Figure 1 and Supplementary Table T2). Careful examination of the corresponding expression profiles led us to distinguish three different situations: directional ROs, quantitative ROs and timing-related ROs. Directional ROs are unique in the directionality of their regulation (i.e., up-regulation, down-regulation or unchanged expression). This is exemplified by the DOM34 orthogroup in Figure 1 (right panel). In this example, the expression of one C. glabrata member (CAGL0B04675g) is strongly induced by selenite while its paralog in C. glabrata and its orthologs in the seven other species show unchanged or reduced expression. The quantitative ROs have the same directionality of regulation than other members of the orthogroup, but with much larger amplitude. This is exemplified by the HBS1/SKI7 orthogroup in the right panel of Figure 1. HBS1 is induced by selenite in C. albicans, D. hansenii, L. kluyveri, and C. glabrata, but the induction of the C. glabrata gene CAGL0G02255g is sufficiently higher than its orthologs to be considered as a RO by REGULOUT. The timing-related ROs have the same directionality and range of regulation than some of their orthologs, but with a different timing. This is exemplified by the GCV1 orthogroup in Figure 1 (right panel). GCV1 is induced in several species following selenite exposure but the pattern of early and transient induction is unique to the S. cerevisiae member of this group (YDR019c).
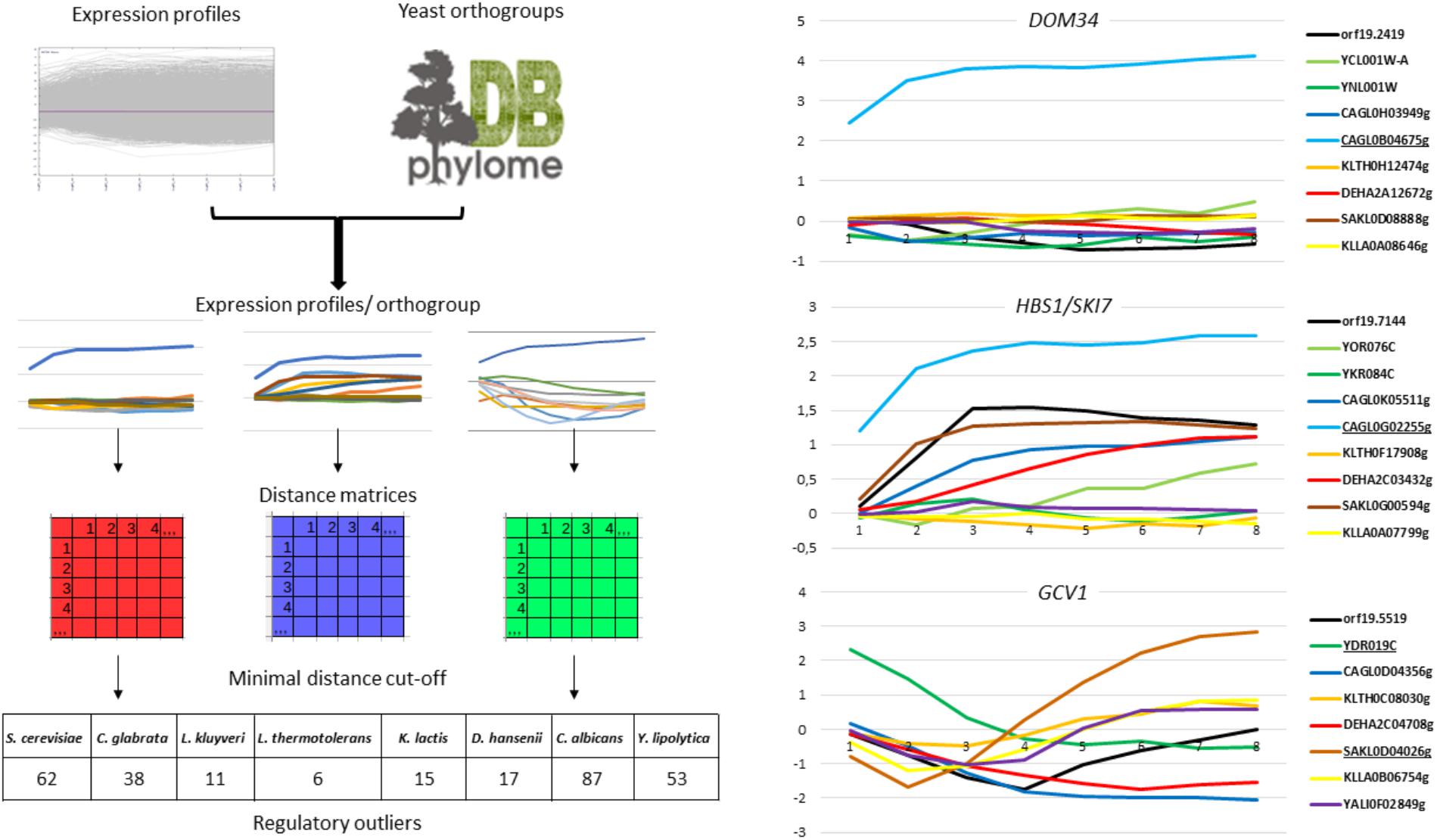
FIGURE 1. Rationale and outputs of REGULOUT. (Left) This scheme summarizes the rationale of REGULOUT. The table at the bottom indicates the number of regulatory outliers identified for each species with the parameters indicated in the material and methods. (Right) Expression profiles [Log2(selenite/mock treatment expression ratio)] as a function of time (1 unit = 10 min) for three orthogroup (DOM34, HBS1/SKI7, and GCV1). The names of the genes in the orthogroup are indicated at the right of each graph. The names of the regulatory outliers are underlined. The colors are: blue for C. glabrata, green for S. cerevisiae, black for C. albicans, orange for L. thermotolerans, red for D. hansenii, brown for L. kluyveri, yellow for K. lactis. The phylome DB logo was taken from the phylome DB database website (Huerta-Cepas et al., 2014).
Gene ontology analyses indicated that only a few GO terms were enriched in the RO lists of the different species (Supplementary File S10). These include genes of the arginine biosynthesis pathway (ARG3, ARG4, ARG5,6, ARG8, CPA1, CPA2), which were much more strongly induced by selenite in C. albicans than in any other species, and orthologs for the two main actors of a ribosome disassembly pathway named NGD (DOM34 and HBS1), which were specifically induced in C. glabrata. Also, several amino-acid metabolism genes (ARG3, HIS1, HIS4, HIS7, CPA1, LYS12, MET6, TRP3) had special expression patterns in C. glabrata. This was already noticed in a previous comparative transcriptomics study analyzing the heat shock stress response in eight yeast species (Roy et al., 2013).
Five ROs in C. glabrata Are Induced in Iron Starvation Conditions
As mentioned above, selenite triggers several stress responses (iron starvation, oxidative stress response, DNA damage…) (Pinson et al., 2000; Salin et al., 2008; Perez-Sampietro et al., 2016) and therefore the ROs identified from the selenite comparative transcriptomics data may actually respond to different physiological signals and pathways. We were more particularly interested in identifying ROs in C. glabrata which would be linked to the iron starvation response. With this goal in mind, we focused on the 38 C. glabrata ROs and took advantage of transcriptome data analyzing the response of C. glabrata to five different stress conditions (osmotic stress, iron excess, cadmium, BPS or selenite treatments), which were obtained in the frame of another project (unpublished data). For the present work, we only extracted the data for the C. glabrata ROs (Supplementary Table T4). We then clustered them according to their expression profiles in these five stress conditions (Figure 2). Doing so, we could point out a group of five ROs which showed early and strong induction in response to the two iron starvation conditions (namely BPS and selenite treatments) and unchanged or slightly increased expression in response to osmotic and iron excess stresses. This group included the C. glabrata orthologs of the S. cerevisiae genes encoding the Grx3 glutaredoxin (CAGL0L11990g), the Mmt2 mitochondrial iron transporter (CAGL0E06006g), the Mak16 ribosome biogenesis factor encoding (CAGL0F00715g) and the Dom34 and Hbs1 translation surveillance factors (CAGL0B04675g and CAGL0G02255g). For the sake of simplicity, we will call the C. glabrata genes by the name of their S. cerevisiae orthologs in the rest of the article. For DOM34 and MAK16, two paralogs exist in C. glabrata. In these cases, the BPS and selenite inducible versions will be called MAK16b and DOM34b and the versions with unchanged or repressed expression patterns will be called MAK16a (CAGL0G06248g) and DOM34a (CAGL0H03949g). Among these five ROs, three were directional ones (GRX3, DOM34b and MAK16b), meaning that they were induced by selenite only in C. glabrata, and two were quantitative ROs (HBS1 and MMT2), meaning that they were also induced in other species, but to a lesser extent than in C. glabrata (Supplementary File S11).
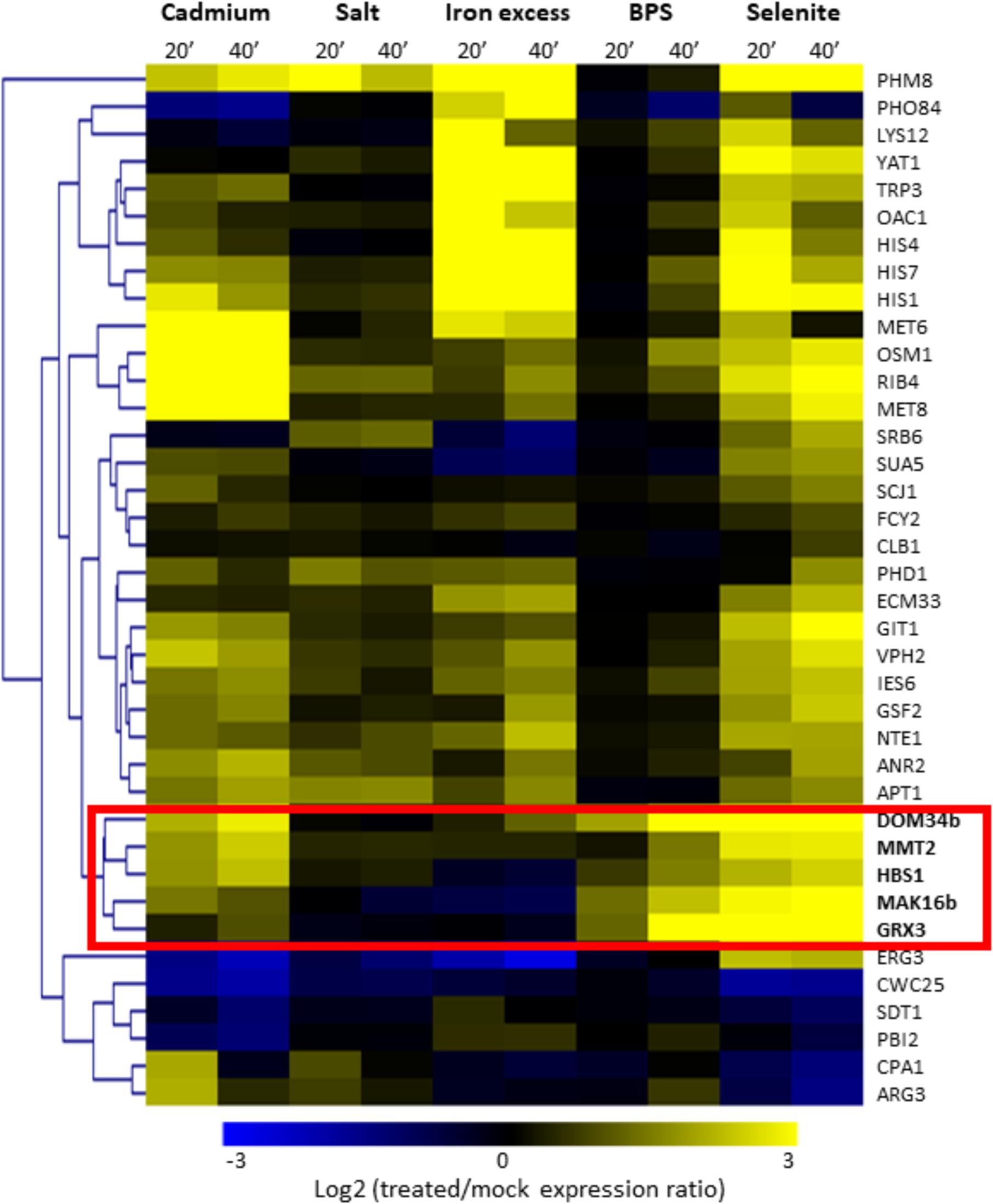
FIGURE 2. Hierarchical clustering of the 38 C. glabrata ROs based on their expression profiles in response to different stress conditions. Expression profiles [Log2(stressed cells/mock treatment expression ratio)] are represented by a color code (scale at the bottom). Two duration times of treatment were used (20 min and 40 min). The names of the genes are based on the names of their closest homologs in S. cerevisiae. A group of genes which is more particularly induced in iron limited conditions (BPS and selenite) has been highlighted by a red box.
GRX3, DOM34b, HBS1, and MAK16b Are New Targets of the Aft2 Transcription Factor in C. glabrata
Interestingly enough, these five genes were also induced by cadmium in C. glabrata (Figure 2). In the model yeast S. cerevisiae, BPS and selenite trigger an iron starvation transcriptional response which is controlled by the two paralogous transcription factors Aft1 and Aft2 (Blaiseau et al., 2001; Rutherford et al., 2001, 2003; Courel et al., 2005; Salin et al., 2008; Perez-Sampietro and Herrero, 2014; Perez-Sampietro et al., 2016). However, only the Aft2 regulon is reproducibly induced by cadmium, while most of the Aft1 regulon remains unchanged or is even repressed (Fauchon et al., 2002; Caetano et al., 2015). The expression profiles of the five ROs identified in Figure 2 hence suggested that their particular stress regulation pattern in C. glabrata could be due to Aft2.
The Aft2 regulon was not previously deciphered in C. glabrata. Then, to test our hypothesis, we conducted genome-wide chromatin immunoprecipitation (ChIP-seq) on a Aft2 myc-tagged strain in iron starvation conditions induced by BPS treatment. ChIP-seq analyses identified 63 promoters bound by Aft2, corresponding to 88 potential gene targets (Supplementary Table T3 and Figure 3, left panel). Sequence analyses of the ChIP peaks identified the ACACCC motif as being the most enriched in the Aft2 bound locus, being present in 80% of the target promoters (Figure 3, left panel). This motif is identical to the consensus previously identified for Aft2 in S. cerevisiae (Courel et al., 2005; Conde e Silva et al., 2009). GO analyses revealed an enrichment in genes involved in iron homeostasis (p-value = 5.15e-08) and oxidative stress response (p-value = 0.00276) (Figure 3, left panel). More specifically, Aft2 targeted genes belonging to the iron homeostasis category were involved in the reductive iron uptake pathway (FRE8, FET4), the siderophore iron uptake pathway (SIT1), the intracellular iron transport (MRS4, SMF3, CCC1, MMT2) and the iron–sulfur cluster biogenesis and assembly pathway (ISU1, ISU2, ISD11, NFS1, YAH1). In connection with redox homeostasis, Aft2 bound the promoters of the genes encoding the reductases HBN1, OSM1, YHB1, RNR1, RNR2, and ERG4, the superoxide dismutases SOD1 and SOD2, the catalase CTA1 and the peroxiredoxin AHP1. Interestingly, Aft2 also targets genes involved in autophagy (ATG8, ATG19, and ATG41), which may be consistent with the recent finding that mitophagy is triggered by iron starvation in C. glabrata (Nagi et al., 2016).
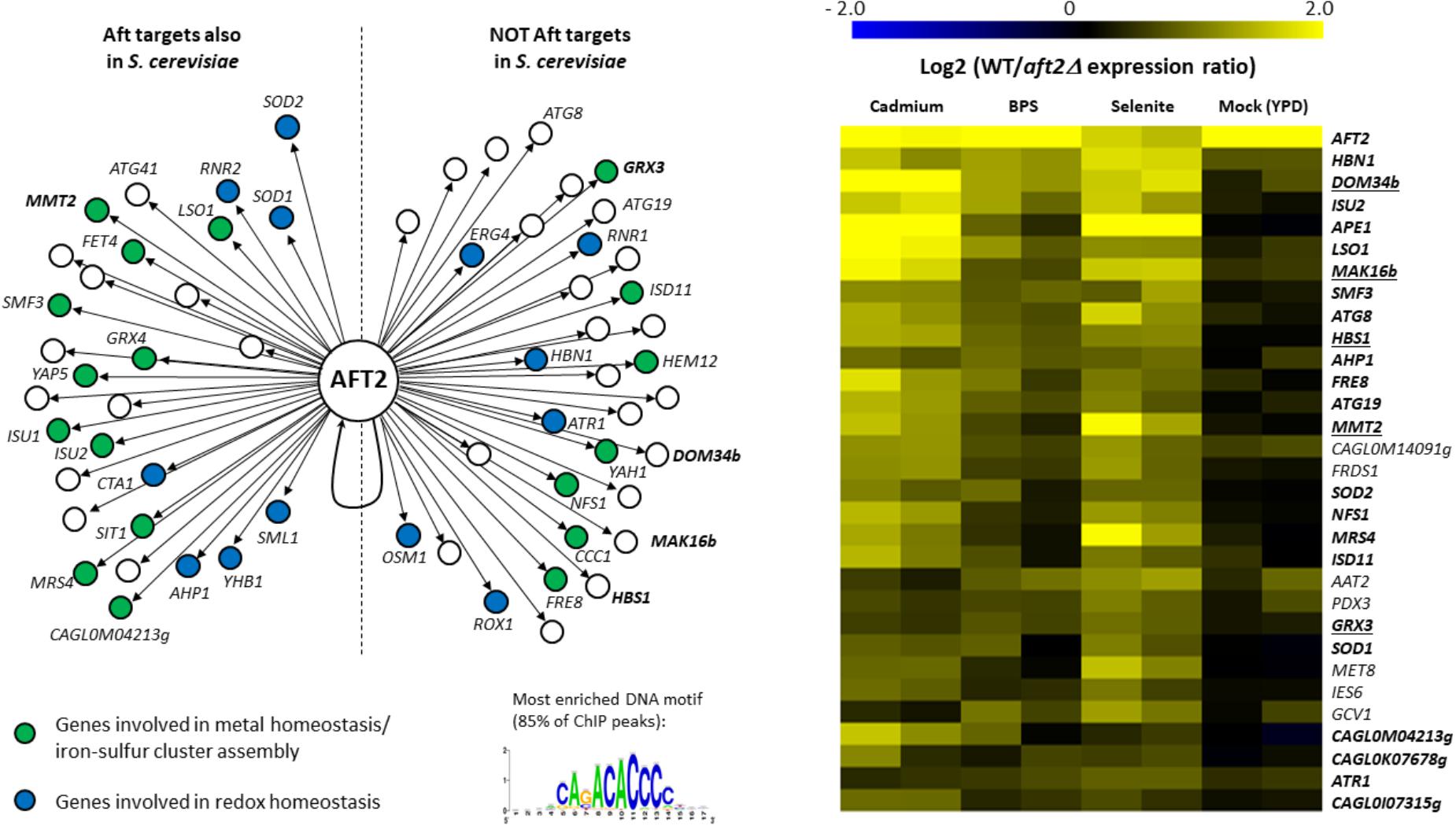
FIGURE 3. The Aft2 regulon in C. glabrata. (Left) The 63 Aft2 target promoters, as defined by ChIP-seq (Supplementary Table T3), were separated in two groups: target promoters which ortholog is also an Aft target in S. cerevisiae and target promoters which ortholog has not been identified as an Aft target in S. cerevisiae. Only the names of the genes which are discussed in the main text are indicated. The names of the five ROs of interest are in bold. The targets are colored according to the two main GO terms enriched among Aft2 targets: blue = redox homeostasis, green = iron metabolism. The most enriched DNA motif among all ChIP-peaks is indicated at the bottom right. (Right) Transcriptomic comparison of gene expression between the wild type and the aft2Δ mutant in four growth conditions. The 30 most affected genes are represented here. The names of genes which were also targeted by Aft2 in our ChIP-seq experiment are in bold. The names of the five ROs of interest have been underlined. The results of two biologically independent replicates are indicated for each growth condition. The complete results are available in Supplementary Table T5.
Then we compared the set of targets identified in C. glabrata with those of Aft2 and Aft1 in S. cerevisiae (Figure 3, left panel). Half of the C. glabrata Aft2 targets were also targets of Aft1 and/or Aft2 in S. cerevisiae (Figure 3, left panel). Among these conserved targets is MMT2, which was consistent with our observation that MMT1 and MMT2 are also induced by selenite in S. cerevisiae (Supplementary File S11). Reciprocally, 50% of the Aft2 targets were specific to C. glabrata (“specific” meaning here as compared to S. cerevisiae). Among these C. glabrata “specific” Aft2 targets were GRX3, DOM34b, HBS1, and MAK16b.
To measure the impact of Aft2 on the expression of its potential targets, we performed transcriptome analyses comparing the aft2Δ and wild type strains grown either in optimal conditions (YPD), iron starvation caused by BPS or by selenite, and cadmium exposure. Twenty-four of the 63 promoters bound by Aft2 (38%) were associated to a gene which showed a significant decrease of expression in the aft2Δ cells in at least one growth condition. Reciprocally, among the 30 genes which were the most reproducibly affected by AFT2 deletion, 23 (77%) were directly targeted by Aft2 according to our ChIP-seq results (Figure 3, right panel). Among the seven genes which showed changed expression but no binding in ChIP-seq, only one had a potential connection with iron homeostasis (MET8, encoding a ferrochelatase) but remarkably six of them (the exception being GCV1) had an Aft2 binding motif in the 500 bp upstream of their ATG (data not shown). These differences in expression were observed only in stress conditions and not in optimal growth conditions (except for AFT2 itself, which was constitutively deleted in the mutant strain). The four new targets of interest (GRX3, DOM34b, HBS1, and MAK16b) showed decreased expression in the aft2Δ strain in all three stress conditions, but with different ranges (Figure 3, right panel). Hence, our results demonstrate that the increase of expression of GRX3, DOM34b, HBS1, and MAK16b in C. glabrata is controlled by Aft2.
DOM34b and HBS1 Are Required for Optimal Growth in Iron Starvation Conditions
This regulation of MAK16b, DOM34b, and HBS1 by Aft2 in C. glabrata was particularly intriguing because none of the processes they are contributing to in the model yeast S. cerevisiae, namely ribosome biogenesis for Mak16, Dom34 and Hbs1 and ribosome rescue pathway for Dom34 and Hbs1, were directly connected to the transcriptional regulation of iron homeostasis.
Therefore, we assessed the physiological impact of these new regulatory interactions. First, we wanted to know if the increase that we observed at the mRNA level for DOM34b, MAK16b, and HBS1 in iron starvation conditions was translated to the protein level. We constructed C. glabrata strains with genomic TAP-tagged versions of DOM34b, MAK16b, and HBS1. A strain tagged for DOM34a was also constructed as a control for a gene which is not induced by stress at the mRNA level. We performed Western blot analyses on these four strains grown in presence or absence of BPS. We observed a clear and fast induction of Dom34b, Mak16b, and Hbs1 in response to stress, which was perfectly mimicking what was observed at the mRNA level (Figure 4, left panel and Supplementary Files S12, S13). In contrast, Dom34a displayed unchanged expression levels, as expected.
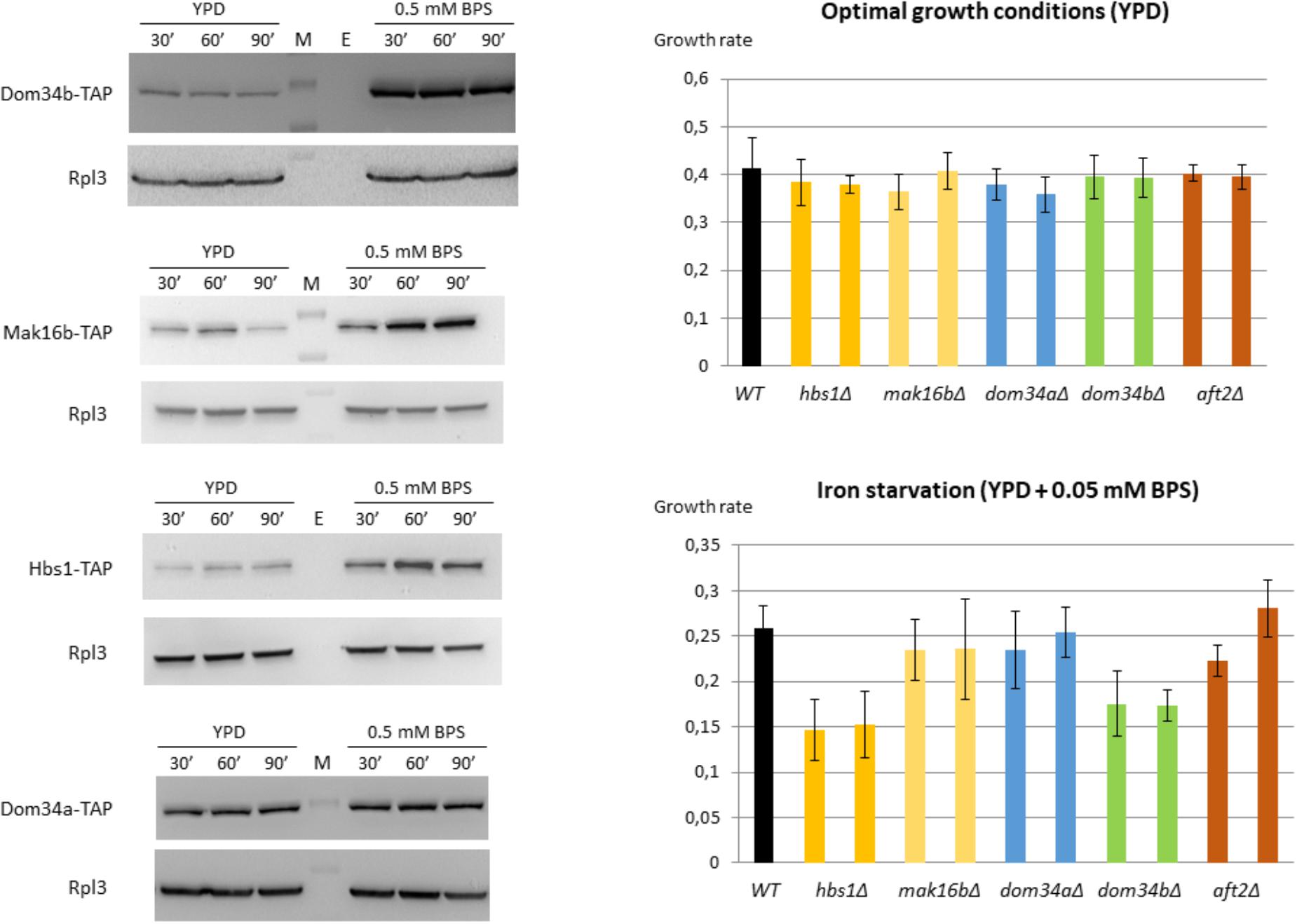
FIGURE 4. Protein expression levels and fitness analyses in iron starvation conditions. (Left) Western blot analyses of the Dom34b-TAP, Hbs1-TAP, Mak16b-TAP, and Dom34a-TAP strains grown in YPD or in YPD + 0.5 mM BPS. Cells were collected 30, 60, and 90 min after the start of BPS or mock treatments. The signal obtained for the Rpl3 ribosomal protein was used as a loading control. Full gel pictures are available in Supplementary File S12. A quantification of the Western blot signals from two biologically independent replicates is available in Supplementary File S13. (Right) Growth rates in exponential phase of different strains in YPD (upper) or in YPD + 0.05 mM BPS (lower). Two independent clones were tested for each mutant strains. The values are the average of six measurements: three independent biological replicates with two technical replicates for each of them.
Second, we assessed the importance of MAK16b, DOM34b, and HBS1 in the adaptation to iron starved conditions. We constructed strains deleted for these three genes. We included in our phenotypic analyses strains deleted for DOM34a and for AFT2. The growth rates of these strains in exponential phase were measured in presence or absence of 0.05 mM BPS and compared to the growth rate of the isogenic wild type strain (Figure 4, right panel). Two independent clones were tested for each strain. All mutant strains had similar growth rates compared to the wild type in standard growth conditions (Figure 4, upper right panel). In presence of BPS, the dom34aΔ, mak16bΔ and aft2Δ strains did not show any growth defect (Figure 4, bottom right panel). In contrast, the dom34bΔ and hbs1Δ mutants had a decreased fitness in iron starvation conditions.
The Regulation of DOM34b and HBS1 by Iron Starvation Probably Arose From the Whole Genome Duplication (WGD)
As mentioned above, in post-WGD species such as S. cerevisiae and C. glabrata, there are two Aft transcription factors: Aft2 having a preference for the ACACCC motif and Aft1 being more likely associated to TGCACCC (Courel et al., 2005; Conde e Silva et al., 2009; Goncalves et al., 2014; Srivastava et al., 2015; Gerwien et al., 2016; this work). Besides post-WGD species, the role of the Aft transcription factors in the control of the iron regulon is apparently conserved in the whole Saccharomycetaceae family, together with their DNA binding preference for PuGACCC motifs (Conde e Silva et al., 2009; Goncalves et al., 2014). To assess the evolution of the regulation of DOM34b and HBS1 by Aft2 and iron starvation that we characterized in C. glabrata, we analyzed the promoter sequences of the orthologs and ohnologs of these two genes in several post- and pre-WGD yeast species spanning the Saccharomycetaceae tree, looking for ACACCC (Aft2-like) and GCACCC (Aft1-like) motifs on both DNA strands (Figure 5 and Supplementary Files S5, S6, S14).
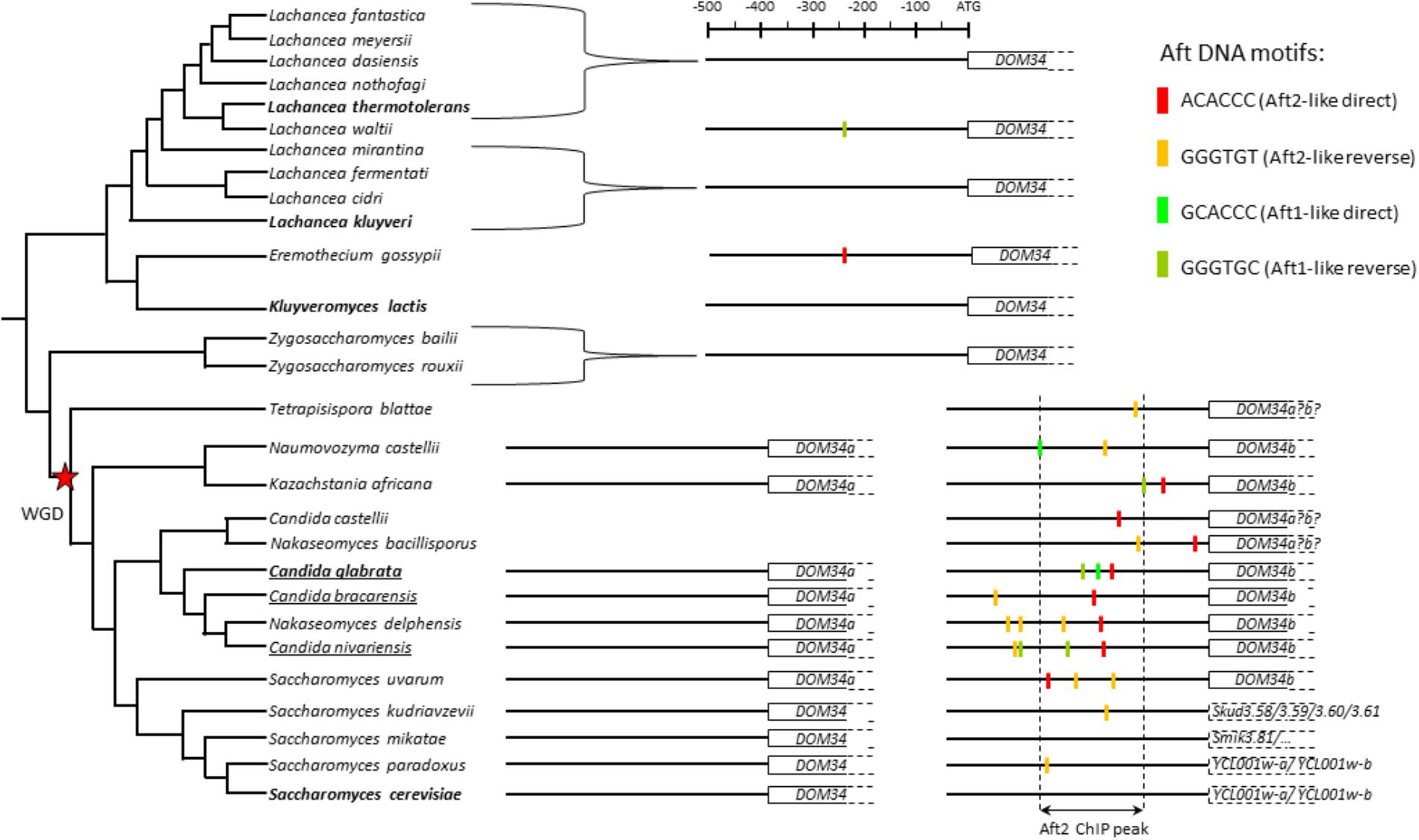
FIGURE 5. Analyses of the promoters of the DOM34 orthogroup in 28 Saccharomycetaceae species. The tree is a schematic representation of the phylogeny of the species inspired from Vakirlis et al. (2016) for the Lachancea species and from Shen et al. (2016) for all the other species. The Whole Genome Duplication (WGD) is indicated by a red star. The positions of the Aft sites in the promoters of the genes are indicated by colored boxes (color code is on the upper right part). The boundaries of the Aft2 ChIP peak in the C. glabrata DOM34b gene are indicated by dashed vertical lines. The ORFs in the DOM34b lineage of the Saccharomyces genus (lower right part of the tree) are interrupted ORFs probably corresponding to pseudogenes. The names of the three pathogenic Nakaseomyces species are underlined. The sequences used for this analysis can be found in Supplementary File S5.
In post-WGD species, the evolution of the DOM34 orthogroup is complex and different situations were observed. In some species (e.g., Candida glabrata and its three most related Nakaseomyces species, but also Naumovozyma castellii, Kazachstania Africana, and Saccharomyces uvarum) the two ohnologs DOM34a and DOM34b were retained. In others (e.g., Tetrapisispora blattae, Candida castellii, Nakaseomyces bacillisporus) only one copy remained and reciprocal BLAST or synteny analyses could not indicate without ambiguity if it corresponded to the DOM34a or DOM34b paralog in the other species. Finally, in most Saccharomyces species, one of the two ohnologs was split in two or more ORFs by non-coding insertions (e.g., YCL001w-a and YCL001w-b in S. cerevisiae) and is probably on its way to pseudogenization. Promoter analyses indicated a clear enrichment for Aft motifs in the DOM34b lineage of the post-WGD species compared to the DOM34a lineage or to the DOM34 orthologs in the pre-WGD species (Figure 5). However, this enrichment is heterogeneous. It is particularly obvious in C. glabrata and its close relatives C. bracarensis, N. delphensis, and C. nivariensis, in which the position of one of the Aft2-like motif is particularly well-conserved while the rest of the promoter sequence largely diverged (Supplementary File S15). In contrast, there is a loss of the Aft motif enrichment in the Saccharomyces species, which seems to be correlated to the pseudogenization of one of the two ohnologs.
A similar pattern was observed for HBS1: there is a clear enrichment for Aft motif in the HBS1 lineage of post-WGD species compared with its SKI7 ohnolog or with the SKI7/HBS1 orthologs in pre-WGD species (Supplementary File S14). Again, this enrichment in post-WGD species was heterogeneous with, for instance, a clear conservation in the C. glabrata sub-clade of the Nakaseomyces genus but no Aft motifs in promoters of the HBS1 versions in the two other Nakaseomyces species C. castellii and N. bacillisporus.
This phylogenetic analysis strongly suggests that the Aft motifs were fixed in the promoters of DOM34b and HBS1 after the whole genome duplication, in a process equivalent to a neo-functionalization event. Then their evolution varied from strong conservation in some species to disappearance in others, possibly reflecting different selection pressures exerted on this new regulation. Yet, it is very important to remember that the presence of an Aft motif does not imply that the motif is active and that the corresponding gene is under Aft regulation. Still, the high conservation of the position of Aft motifs in the promoter of DOM34b in C. glabrata and its three most related species, while the rest of the promoter diverged, strongly suggests that this motif is under positive selection in these species and then that the regulation that we characterized here for C. glabrata is also active in these three species (Supplementary File S15).
Discussion
In this study, we designed a program, called REGULOUT, for the identification of conserved genes with divergent expression profiles from comparative transcriptomics datasets. The inputs of REGULOUT are multispecies gene expression profiles on one hand and the composition of orthogroups on the other. Hence, REGULOUT can be applied to any group of species for which this information is available. The output of REGULOUT is strongly influenced by the distance cut-off chosen and the type of distance used but also by the way the orthogroups were defined and by the stress conditions which were tested. Hence, the label of “ROs” that we introduced in this article is more a technical concept than a straightforward biological feature and REGULOUT should be used as a fast way to sort out lists of potentially interesting cases and not as a tool for gene annotation. We applied REGULOUT to transcriptomics data obtained from eight yeast species responding to the stress caused by detrimental doses of selenite. To assess the power of REGULOUT in highlighting new, biologically meaningful, regulations, we investigated further the 38 ROs identified in the human pathogen C. glabrata and we focused on those which were more particularly induced by stress conditions associated to iron starvation.
Iron acquisition is a critical challenge for most microorganisms and a key virulence factor for many fungal pathogens (reviewed in Bairwa et al., 2017; Gerwien et al., 2018). Iron acquisition genes are required for the survival of C. glabrata in the macrophages, its adhesion to epithelial cells and for its virulence in animal models (Nevitt and Thiele, 2011; Seider et al., 2014; Srivastava et al., 2014; Brunke et al., 2015). In S. cerevisiae, the iron starvation transcriptional response is controlled by the two paralogous transcription factors Aft1 and Aft2. Aft1 activates the expression of genes involved in iron uptake at the plasma membrane (e.g., FET3, FTR1, ATX1, FRE1-3, SIT1, …) and genes involved in the cytoplasmic adaptation to low iron conditions, especially the CTH1 and CTH2 genes encoding RNA binding proteins involved in the selective degradation of mRNAs encoding iron consuming proteins (Puig et al., 2005, 2008). Aft2 shares some targets with Aft1 but also specifically activates the expression of genes encoding, for instance, the vacuolar iron transporter Smf3 and the mitochondrial iron transporter Mrs4 (Rutherford et al., 2003; Courel et al., 2005; Martinez-Pastor et al., 2017).
Previous studies have shown that many aspects of the iron homeostasis regulation in C. glabrata resemble what had been shown in S. cerevisiae. Like S. cerevisiae, C. glabrata strongly depends on the reductive pathway and siderophore uptake for extracellular iron acquisition (Nevitt and Thiele, 2011; Srivastava et al., 2014; Gerwien et al., 2016). Low affinity iron transport mediated by the Fet4 protein also exists in C. glabrata (Srivastava et al., 2014; Gerwien et al., 2016). We showed here that FET4 is targeted by Aft2 but the sole deletion of AFT2 had no impact on its induction by BPS, selenite or cadmium (Supplementary Tables T3, T5). The key genes of the iron regulon in S. cerevisiae are also induced by iron starvation in C. glabrata (Srivastava et al., 2015; Gerwien et al., 2016; Nagi et al., 2016). C. glabrata Aft1 plays a major role in the up-regulation of membrane iron uptake and is itself induced by iron starvation (Srivastava et al., 2015; Gerwien et al., 2016). Moreover, the enrichment of Cth2 motif in the 3′UTR of genes from the C. glabrata iron regulon suggests that the post-transcriptional negative regulation of iron consuming genes is also active in this species (Gerwien et al., 2016). In this work, we showed that the role of Aft2 in the control of intracellular iron trafficking and homeostasis is globally conserved. Especially, the two main specific targets of Aft2 in S. cerevisiae, MRS4 and SMF3 (Courel et al., 2005), were also regulated by this factor in C. glabrata (Figure 3). As in S. cerevisiae (Blaiseau et al., 2001; Rutherford et al., 2001), the aft2Δ mutant exhibited no particular growth defect in response to stress in C. glabrata (Srivastava et al., 2014; Gerwien et al., 2016; Figure 4 of this work).
However, several important differences in iron homeostasis control have also been observed between C. glabrata and other model yeast species. For instance, the deletion of the C. glabrata ferric reductase encoding genes FRE8 and FRE6 does not seem to impact the C. glabrata extracellular iron uptake or extracellular ferric reduction activities, in contrast to what has been shown in S. cerevisiae and C. albicans (Gerwien et al., 2017). Still, these two genes are induced by iron starvation (Nagi et al., 2016; Gerwien et al., 2017) and regulated by Aft2 (this work) and Aft1 (Gerwien et al., 2017), respectively. Moreover, iron starvation was shown to induce the expression of the autophagy genes ATG32, ATG11, and ATG8 (Srivastava et al., 2015; Nagi et al., 2016) and to trigger mitophagy in C. glabrata but not in S. cerevisiae (Nagi et al., 2016; reviewed in Fukuda and Kanki, 2018). We showed here that the iron starvation induction of ATG8 is under the control of Aft2 (Figure 3). Of note, ATG32 and ATG11 are required for C. glabrata dissemination in mice and survival in macrophages, respectively (Roetzer et al., 2010; Nagi et al., 2016).
REGULOUT pointed out GRX3, MAK16b, DOM34b, and HBS1 as being particularly responsive to selenite exposure in C. glabrata. Further analyses showed that in C. glabrata these four genes were also sensitive to BPS and cadmium treatment, but not to iron excess or osmotic stress (Figure 2). We investigated the regulatory mechanisms underlying these expression patterns and found that the stress response of these four genes is under the control of Aft2 in C. glabrata (Figure 3). Our analyses of the evolution of the promoter sequences of the DOM34 and HBS1 orthogroups in 14 post-WGD and 14 pre-WGD yeast species revealed enrichment for Aft-like DNA binding motifs in the DOM34b and HBS1 orthologs but neither in the pre-WGD orthologs nor in the DOM34a and SKI7 ohnologs (Figure 5). This strongly suggests that this regulation appeared after the whole genome duplication and was subsequently lost in Saccharomyces cerevisiae. This is consistent with previous, more global, observations that duplicated genes are often differentially expressed and evolve divergent regulatory patterns (Gu et al., 2004; Conant and Wolfe, 2006; Tirosh and Barkai, 2007; Wapinski et al., 2007; Thompson et al., 2013), with one of the paralogs retaining the ancestral expression profile while the regulation of the other copy evolves more rapidly (Gu et al., 2005). This can be achieved by changes in the transcription factors that regulate the paralogs (Wapinski et al., 2010; Perez et al., 2014; Pougach et al., 2014), by loss and/or gain of different cis-regulatory elements in their promoters (Papp et al., 2003; Zhang et al., 2004; Ihmels et al., 2005) or by a combination of both mechanisms. The latter scenario might be the one at work in our case. Indeed, based on the observation that the Aft transcription factor of the pre-WGD species K. lactis fulfills the Aft1 but not the Aft2 functions, it was proposed that the mitochondrial and vacuolar control of iron homeostasis by Aft2 appeared as a neo-functionalization event after the WGD and the duplication of the Aft ancestral gene (Conde e Silva et al., 2009). Then, the acquisition of Aft2 regulation by DOM34b and HBS1 would be concomitant to the emergence of the specific role of this transcription factor. Among the post-WGD species, the enrichment of Aft motifs in the promoters of the DOM34b and HBS1 was especially clear in the Nakaseomyces sub-lineage which contains C. glabrata and two other potential human pathogens (C. bracarensis and C. nivariensis) (Gabaldon et al., 2013) (Figure 5 and Supplementary File S14), as compared to the other Nakaseomyces sub-clade or to the Saccharomyces genus. A similar pattern was also observed for GRX3/4, which showed a dramatic enrichment of Aft motifs in the promoters of the GRX3 lineage in the C. glabrata sub-clade (Supplementary File S16). C. glabrata, C. bracarensis, C. nivariensis and their non-pathogenic relative N. delphensis were also the only yeast species to have two MAK16 orthologs, with the promoter of the MAK16b paralog being enriched in Aft DNA motifs (Supplementary File S17). Hence, the presence of two DOM34, GRX3/4 and MAK16 orthologs and the co-regulation of DOM34b, HBS1, GRX3, and MAK16b by Aft2 may be a specificity of this Nakaseomyces sub-clade, which is enriched in hosts of the human body (three species out of four). To go further in the characterization of this regulation, it would be interesting to determine the actual contribution of the different Aft motifs in the promoters of the genes by site-directed mutagenesis.
Why were these new regulations fixed in C. glabrata and lost in S. cerevisiae? In other terms, how could these four genes contribute to iron homeostasis in C. glabrata? In S. cerevisiae, the cytosolic monothiol glutaredoxins Grx3 and Grx4 play a central role in communicating the mitochondrial iron status to Aft1 and Aft2 (Ojeda et al., 2006; Pujol-Carrion et al., 2006; Kumanovics et al., 2008). In iron replete cells, the mitochondrial Fe–S clusters biogenesis is very active and Fe–S clusters are exported to the cytosol where Grx3 and Grx4 form Fe–S bridged homodimers which has the capacity to transfer its Fe–S cluster to Aft1 and Aft2, therefore decreasing their DNA binding affinity by favoring the formation of Fe/S bridged Aft homodimers (Kumanovics et al., 2008; Ueta et al., 2012; Poor et al., 2014; Chi et al., 2018). This role of Grx3/4 proteins in the regulation of iron homeostasis is conserved from yeasts to humans (Li et al., 2012; Labbe et al., 2013; Jacques et al., 2014; Banci et al., 2015; Encinar del Dedo et al., 2015). It may seem counter-intuitive that C. glabrata Aft2 induces the expression of a protein which would negatively control its activity. However, besides its role in the regulation of Aft1/2, Grx3 (and its ohnolog Grx4) also makes important contributions to the oxidative stress response and to the cytosolic and nuclear Fe–S cluster protein assembly (Molina et al., 2004; Herrero et al., 2010; Muhlenhoff et al., 2010; Pujol-Carrion and de la Torre-Ruiz, 2010; Vall-Llaura et al., 2016; Pujol-Carrion and Torre-Ruiz, 2017).
In S. cerevisiae, MAK16 was initially identified in a screen for the maintenance of the killer virus double stranded RNA genome (Wickner and Leibowitz, 1979; Icho et al., 1986). Later on, genetic, biochemical and cryoEM analyses showed that Mak16 is actually involved in the biogenesis of the 60S ribosomal particles (Ohtake and Wickner, 1995; Capowski and Tracy, 2003; Pellett and Tracy, 2006; Altvater et al., 2012; Kater et al., 2017; Zhou et al., 2018). MAK16 is an essential gene, conserved from yeast to human (Kaback et al., 1984; Wickner et al., 1987). As mentioned above, C. glabrata and its three most closely related Nakaseomyces species (C. nivariensis, N. delphensis and C. bracarensis) have two MAK16 paralogs, which we named here MAK16a and MAK16b. MAK16b was obviously not essential in C. glabrata since the mak16bΔ mutant cells grew at wild type rates in YPD (Figure 4). As all members of the ribosome biogenesis (RiBi) regulon, MAK16 was repressed by stress in all the species we examined (Supplementary File S11). This was also true for MAK16a in Candida glabrata, which was consistently repressed by selenite and by all the other stresses that we tested (Supplementary File S11, unpublished data). Then, the dramatic induction of MAK16b by selenite, cadmium and BPS, and its regulation by Aft2, is particularly intriguing. The sole link that can be made between Mak16 proteins and stress responses based on the literature is the fact that they were proposed to form an atypical class of glutathione-S transferases (GSTs) (McGoldrick et al., 2005). GSTs are enzymes which assist the cell in the defense against reactive oxygen species (reviewed in Kalinina et al., 2014). Moreover, glutathione plays a key role in iron sensing by Grx3/4 and in iron–sulfur cluster assembly (Muhlenhoff et al., 2010; Kumar et al., 2011; Ueta et al., 2012; Martinez-Pastor et al., 2017; Cardenas-Rodriguez et al., 2018). Still, this annotation of MAK16 orthologs as GSTs only relies on immunological criteria, i.e., the Mak16 protein of the trematode Schistosoma mansoni was probed by an anti-serum against purified S. mansoni GSTs (Milhon et al., 2000), and an actual GST activity was never proven for these proteins. Moreover, the fact that the deletion of MAK16b does not alter the growth of C. glabrata in iron starvation conditions makes its actual contribution to the iron homeostasis in this species questionable.
In contrast to MAK16b, DOM34b, and HBS1 were required for optimal growth in iron starvation conditions, suggesting a role for these genes in the adaptation to iron limitation in C. glabrata (Figure 4). Dom34 and Hbs1 are also highly conserved proteins which are found in all eukaryotes, but they are not essential in yeasts. In S. cerevisiae, Dom34 and Hbs1, together with the general translation termination factor Rli1, were initially described as being responsible for a translation surveillance pathway called NGD, which rescues and recycles the ribosomes that stall before translation is completed (Doma and Parker, 2006; Passos et al., 2009; Simms et al., 2014; see Buskirk and Green, 2017; Simms et al., 2017, for recent reviews). Later on, the NGD co-factors were shown to be involved in ribosome biogenesis (Kispal et al., 2005; Yarunin et al., 2005; Cole et al., 2009; Lebaron et al., 2012; Strunk et al., 2012) and in the re-activation of hibernating ribosomes prior to quiescence exit (van den Elzen et al., 2014). Dom34 and Hbs1 also have a role in preventing ectopic translation of mRNA 3′ untranslated regions (Guydosh and Green, 2014), that is especially important in conditions in which the activity of Rli1 in regular translation termination is impaired (Young et al., 2015). In S. cerevisiae, the expression of DOM34 and HBS1 is not regulated by stress and these proteins have not been connected to the regulation of iron homeostasis. So, what could be the functional meaning of the strong induction of DOM34b and HBS1 by BPS, selenite and cadmium in C. glabrata? Actually, a quite direct connection can be made between iron starvation and the Dom34-Hbs1 co-factor Rli1. Indeed, Rli1 is an essential Fe–S cluster containing protein (Paul et al., 2015). This iron–sulfur cluster is required for most of Rli1 functions and is very sensitive to redox stress (Kispal et al., 2005; Yarunin et al., 2005; Alhebshi et al., 2012). As a consequence, the number of unrecycled ribosomes in 3′UTRs increases upon treatment with oxidizing agents, such as diamide, which targets iron–sulfur cluster proteins (Guydosh and Green, 2014). Some authors even called Rli1 the “Achilles’ heel” of the cells in oxidizing conditions and hypothesized that Rli1 dysfunction is the main cause of cell death in acute oxidative stress (Alhebshi et al., 2012).
Hence, iron starvation is likely to alter the essential Rli1 activity in ribosome rescue at many levels (Figure 6). Indeed, iron starvation has been shown to render yeast cells more sensitive to oxidative stress (Blaiseau et al., 2001; Matsuo et al., 2017). Moreover, the Fe–S cluster biogenesis, which takes place in the mitochondria, obviously depends on the iron supply (reviewed in Outten and Albetel, 2013). Finally, in S. cerevisiae and in C. glabrata, iron starvation provokes the Aft1-mediated overexpression of the RNA binding protein Cth2 which targets the Rli1 mRNA to degradation pathways (Puig et al., 2001, 2005; Gerwien et al., 2016). These effects may be particularly and specifically amplified in C. glabrata due to the active mitophagy triggered by iron limitation conditions (Nagi et al., 2016), which may also alter mitochondrial Fe–S cluster biogenesis. Thus, it is tempting to hypothesize that the transcriptional induction of DOM34b and HBS1 would be a C. glabrata strategy to better cope with the decreased Rli1 activity caused by iron starvation (Figure 6). Interestingly enough, such a transcriptional up-regulation of DOM34 and HBS1 to compensate Rli1’s defects has already been described in human erythroblasts (Mills et al., 2016). When differentiating in red blood cells, erythroblasts face a difficult challenge: they completely get rid of their mitochondria and hence lose the capacity of producing iron–sulfur clusters, while having to maintain an active translation of hemoglobin, and therefore an active ribosome rescue process, in the last differentiation stages. They do so by transiently overexpressing Pelota (the human equivalent for DOM34) and HBS1L (the human ortholog of HBS1), which compensates for the progressive loss of active Rli1 (ABCE1 in Human) (Mills et al., 2016). Then, the hypothetical model that we propose for C. glabrata (Figure 6) would be analog to what was described for human erythroblasts. Of note, the induction of GRX3 by Aft2 can also take place in this model, because Grx3 is involved in the transfer of the Fe–S cluster for the biogenesis of cytosolic and nuclear Fe–S proteins, such as Rli1 (Muhlenhoff et al., 2010) (Figure 6). This model is also consistent with our observation that in C. glabrata Aft2 targets several components of the core iron–sulfur cluster machinery. Obviously, many experiments, which go far beyond the scope of this study, will be required to test this working model. Especially, the actual contribution of Hbs1, Dom34a and Dom34b to ribosome rescue in C. glabrata, the precise role of Grx3 in the C. glabrata iron starvation response and the potential link between the regulation of these genes by Aft2 and the iron starvation-triggered mitophagy in C. glabrata will need to be thoroughly investigated.
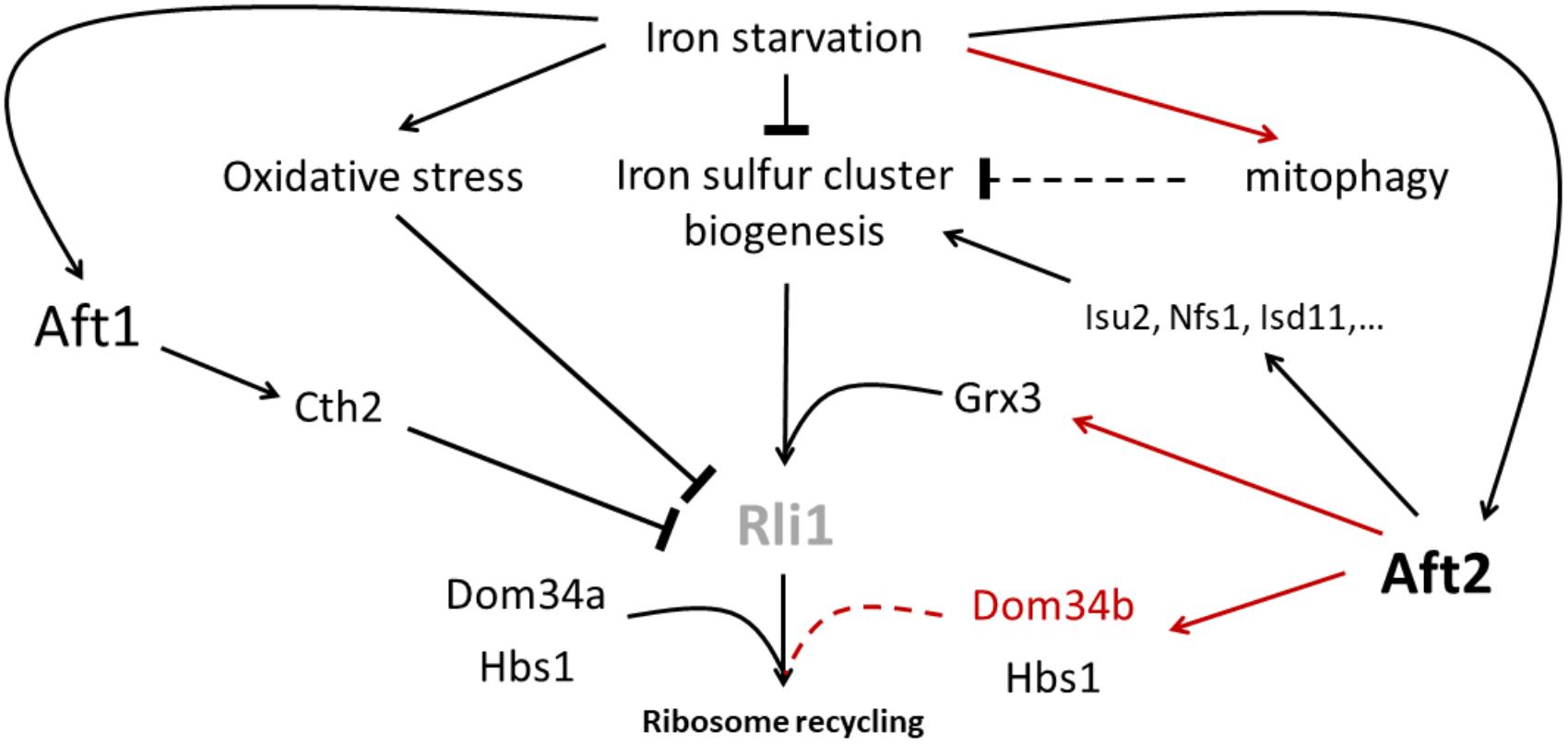
FIGURE 6. Hypothetical working model for the role of Dom34b and Hbs1 in iron starvation adaptation of C. glabrata. Iron starvation alters the functioning of the essential iron–sulfur cluster containing Rli1 in ribosome recycling at many levels: 1- by reducing the iron–sulfur cluster biogenesis potential, 2- by generating oxidative stress which was shown to strongly alter Rli1 activity, 3- by inducing the expression of the Cth2 RNA binding protein which targets part of the Rli1 mRNAs for degradation and 4- specifically in C. glabrata, by inducing mitophagy, which may reduce the mitochondrial biogenesis of iron–sulfur clusters. However, iron starvation also triggers the activation of Aft2 which may compensate the decreased activity of Rli1 by overexpressing 1- components of the mitochondrial core iron–sulfur cluster biogenesis machinery; 2- the glutaredoxin Grx3 which is involved in the delivery of iron–sulfur clusters to Rli1 in the cytosol and 3- the Dom34b and Hbs1 ribosome rescue factors, which, together with the constitutively expressed paralog Dom34a, may contribute to increase the ribosome recycling activity of Rli1. In this scheme, the red lines indicate the regulations which are specific to C. glabrata as compared to S. cerevisiae. The dashed lines indicate hypothetical activities which have not yet been supported by experimental data (namely: an impact of mitophagy on iron–sulfur cluster biogenesis and a role of Dom34b and Dom34a in ribosome rescue in C. glabrata).
Author Contributions
MS, HR, and EC made the REGULOUT script and identified the ROs. LJ and SLC designed the microarrays. GeL, SB, and FD performed the multispecies microarray experiments. GaL, AT, and FD analyzed the microarray experiments. MB, LF, and TD built the strains and performed the Western blot analyzes. MB and LF made the growth assays in 96-well plates. AT and JM performed the multistress C. glabrata microarrays experiments. MB and AT performed the transcriptome analyses of the aft2D mutant strain. AT performed the ChIP experiments, the peak calling and the cis-regulatory motif enrichment analyzes. FD did the phylogenetic analyzes of the promoters, conceived the whole project, interpreted the results and wrote the manuscript.
Funding
This work was funded by the Agence Nationale pour la Recherche (CANDIHUB project, grant number ANR-14-CE14-0018-02 and STRUDYEV project, grant number ANR-10-JCJC-1603) and by the Emergence program of Université Pierre et Marie Curie (2009 session).
Conflict of Interest Statement
The authors declare that the research was conducted in the absence of any commercial or financial relationships that could be construed as a potential conflict of interest.
Acknowledgments
We are grateful to Marc Lemaire, Dominique Sanglard, Christophe D’enfert, Cecile Neuveglise, Cecile Fairhead, and Gilles Fischer for providing strains. We thank Gilles Fischer, Cécile Neuveglise, and Julianna Silva Bernardes for useful discussions. We thank Mathilde Garcia for critical reading of the manuscript. We are grateful to the Array express database staff for their valuable help in the submission of the transcriptome data.
Supplementary Material
The Supplementary Material for this article can be found online at: https://www.frontiersin.org/articles/10.3389/fmicb.2018.02689/full#supplementary-material
Footnotes
References
Alhebshi, A., Sideri, T. C., Holland, S. L., and Avery, S. V. (2012). The essential iron-sulfur protein Rli1 is an important target accounting for inhibition of cell growth by reactive oxygen species. Mol. Biol. Cell 23, 3582–3590. doi: 10.1091/mbc.E12-05-0413
Altvater, M., Chang, Y., Melnik, A., Occhipinti, L., Schutz, S., Rothenbusch, U., et al. (2012). Targeted proteomics reveals compositional dynamics of 60S pre-ribosomes after nuclear export. Mol. Syst. Biol. 8:628. doi: 10.1038/msb.2012.63
Bairwa, G., Hee Jung, W., and Kronstad, J. W. (2017). Iron acquisition in fungal pathogens of humans. Metallomics 9, 215–227. doi: 10.1039/c6mt00301j
Banci, L., Ciofi-Baffoni, S., Gajda, K., Muzzioli, R., Peruzzini, R., and Winkelmann, J. (2015). N-terminal domains mediate [2Fe-2S] cluster transfer from glutaredoxin-3 to anamorsin. Nat. Chem. Biol. 11, 772–778. doi: 10.1038/nchembio.1892
Blaiseau, P. L., Lesuisse, E., and Camadro, J. M. (2001). Aft2p, a novel iron-regulated transcription activator that modulates, with Aft1p, intracellular iron use and resistance to oxidative stress in yeast. J. Biol. Chem. 276, 34221–34226. doi: 10.1074/jbc.M104987200
Brunke, S., and Hube, B. (2013). Two unlike cousins: Candida albicans and C. glabrata infection strategies. Cell. Microbiol. 15, 701–708. doi: 10.1111/cmi.12091
Brunke, S., Quintin, J., Kasper, L., Jacobsen, I. D., Richter, M. E., Hiller, E., et al. (2015). Of mice, flies–and men? Comparing fungal infection models for large-scale screening efforts. Dis. Model. Mech. 8, 473–486. doi: 10.1242/dmm.019901
Buskirk, A. R., and Green, R. (2017). Ribosome pausing, arrest and rescue in bacteria and eukaryotes. Philos. Trans. R. Soc. Lond. B Biol. Sci. 372:20160183. doi: 10.1098/rstb.2016.0183
Caetano, S. M., Menezes, R., Amaral, C., Rodrigues-Pousada, C., and Pimentel, C. (2015). Repression of the low affinity iron transporter Gene FET4: a novel mechanism against cadmium toxicity orchestrated by YAP1 via ROX1. J. Biol. Chem. 290, 18584–18595. doi: 10.1074/jbc.M114.600742
Capowski, E. E., and Tracy, J. W. (2003). Ribosomal RNA processing and the role of SmMAK16 in ribosome biogenesis in Schistosoma mansoni. Mol. Biochem. Parasitol. 132, 67–74. doi: 10.1016/j.molbiopara.2003.08.006
Cardenas-Rodriguez, M., Chatzi, A., and Tokatlidis, K. (2018). Iron-sulfur clusters: from metals through mitochondria biogenesis to disease. J. Biol. Inorg. Chem. 23, 509–520. doi: 10.1007/s00775-018-1548-6
Chi, C. B., Tang, Y., Zhang, J., Dai, Y. N., Abdalla, M., Chen, Y., et al. (2018). Structural and biochemical insights into the multiple functions of yeast Grx3. J. Mol. Biol. 430, 1235–1248. doi: 10.1016/j.jmb.2018.02.024
Cole, S. E., LaRiviere, F. J., Merrikh, C. N., and Moore, M. J. (2009). A convergence of rRNA and mRNA quality control pathways revealed by mechanistic analysis of nonfunctional rRNA decay. Mol. Cell. 34, 440–450. doi: 10.1016/j.molcel.2009.04.017
Conant, G. C., and Wolfe, K. H. (2006). Functional partitioning of yeast co-expression networks after genome duplication. PLoS Biol. 4:e109. doi: 10.1371/journal.pbio.0040109
Conde e Silva, N., Goncalves, I. R., Lemaire, M., Lesuisse, E., Camadro, J. M., and Blaiseau, P. L. (2009). KlAft, the Kluyveromyces lactis ortholog of Aft1 and Aft2, mediates activation of iron-responsive transcription through the PuCACCC Aft-type sequence. Genetics 183, 93–106. doi: 10.1534/genetics.109.104364
Cormack, B. P., Ghori, N., and Falkow, S. (1999). An adhesin of the yeast pathogen Candida glabrata mediating adherence to human epithelial cells. Science 285, 578–582. doi: 10.1126/science.285.5427.578
Courel, M., Lallet, S., Camadro, J. M., and Blaiseau, P. L. (2005). Direct activation of genes involved in intracellular iron use by the yeast iron-responsive transcription factor Aft2 without its paralog Aft1. Mol. Cell. Biol. 25, 6760–6771. doi: 10.1128/MCB.25.15.6760-6771.2005
De Las Penas, A., Pan, S. J., Castano, I., Alder, J., Cregg, R., and Cormack, B. P. (2003). Virulence-related surface glycoproteins in the yeast pathogen Candida glabrata are encoded in subtelomeric clusters and subject to RAP1- and SIR-dependent transcriptional silencing. Genes Dev. 17, 2245–2258. doi: 10.1101/gad.1121003
De Las Penas, A., Juarez-Cepeda, J., Lopez-Fuentes, E., Briones-Martin-Del-Campo, M., Gutierrez-Escobedo, G., and Castano, I. (2015). Local and regional chromatin silencing in Candida glabrata: consequences for adhesion and the response to stress. FEMS Yeast Res. 15:fov056. doi: 10.1093/femsyr/fov056
Delaveau, T., Davoine, D., Jolly, A., Vallot, A., Rouviere, J. O., Gerber, A., et al. (2016). Tma108, a putative M1 aminopeptidase, is a specific nascent chain-associated protein in Saccharomyces cerevisiae. Nucleic Acids Res. 44, 8826–8841. doi: 10.1093/nar/gkw732
Doma, M. K., and Parker, R. (2006). Endonucleolytic cleavage of eukaryotic mRNAs with stalls in translation elongation. Nature 440, 561–564. doi: 10.1038/nature04530
Domergue, R., Castano, I., De Las Penas, A., Zupancic, M., Lockatell, V., Hebel, J. R., et al. (2005). Nicotinic acid limitation regulates silencing of Candida adhesins during UTI. Science 308, 866–870. doi: 10.1126/science.1108640
Dujon, B., Sherman, D., Fischer, G., Durrens, P., Casaregola, S., Lafontaine, I., et al. (2004). Genome evolution in yeasts. Nature 430, 35–44. doi: 10.1038/nature02579
Encinar del Dedo, J., Gabrielli, N., Carmona, M., Ayte, J., and Hidalgo, E. (2015). A cascade of iron-containing proteins governs the genetic iron starvation response to promote iron uptake and inhibit iron storage in fission yeast. PLoS Genet 11:e1005106. doi: 10.1371/journal.pgen.1005106
Fauchon, M., Lagniel, G., Aude, J. C., Lombardia, L., Soularue, P., Petat, C., et al. (2002). Sulfur sparing in the yeast proteome in response to sulfur demand. Mol. Cell. 9, 713–723. doi: 10.1016/S1097-2765(02)00500-2
Fukuda, T., and Kanki, T. (2018). Mechanisms and physiological roles of mitophagy in yeast. Mol. Cells 41, 35–44.
Gabaldon, T., Martin, T., Marcet-Houben, M., Durrens, P., Bolotin-Fukuhara, M., Lespinet, O., et al. (2013). Comparative genomics of emerging pathogens in the Candida glabrata clade. BMC Genomics 14:623. doi: 10.1186/1471-2164-14-623
Gabaldon, T., and Carrete, L. (2015). The birth of a deadly yeast: tracing the evolutionary emergence of virulence traits in Candida glabrata. FEMS Yeast Res. 16:fov110 doi: 10.1093/femsyr/fov110
Gasch, A. P., Spellman, P. T., Kao, C. M., Carmel-Harel, O., Eisen, M. B., Storz, G., et al. (2000). Genomic expression programs in the response of yeast cells to environmental changes. Mol. Biol. Cell 11, 4241–4257. doi: 10.1091/mbc.11.12.4241
Gasch, A. P., Moses, A. M., Chiang, D. Y., Fraser, H. B., Berardini, M., and Eisen, M. B. (2004). Conservation and evolution of cis-regulatory systems in ascomycete fungi. PLoS Biol. 2:e398. doi: 10.1371/journal.pbio.0020398
Gasch, A. P. (2007). Comparative genomics of the environmental stress response in ascomycete fungi. Yeast 24, 961–976. doi: 10.1002/yea.1512
Genolevures, C., Souciet, J. L., Dujon, B., Gaillardin, C., Johnston, M., Baret, P. V., et al. (2009). Comparative genomics of protoploid Saccharomycetaceae. Genome Res. 19, 1696–1709. doi: 10.1101/gr.091546.109
Gerwien, F., Safyan, A., Wisgott, S., Hille, F., Kaemmer, P., Linde, J., et al. (2016). A novel hybrid iron regulation network combines features from pathogenic and nonpathogenic yeasts. mBio 7:e01782-16. doi: 10.1128/mBio.01782-16
Gerwien, F., Safyan, A., Wisgott, S., Brunke, S., Kasper, L., and Hube, B. (2017). The fungal pathogen Candida glabrata does not depend on surface ferric reductases for iron acquisition. Front. Microbiol. 8:1055. doi: 10.3389/fmicb.2017.01055
Gerwien, F., Skrahina, V., Kasper, L., Hube, B., and Brunke, S. (2018). Metals in fungal virulence. FEMS Microbiol. Rev. 42:fux050. doi: 10.1093/femsre/fux050
Goncalves, I. R., Conde e Silva, N., Garay, C. L., Lesuisse, E., Camadro, J. M., and Blaiseau, P. L. (2014). The basis for evolution of DNA-binding specificity of the Aft1 transcription factor in yeasts. Genetics 196, 149–160. doi: 10.1534/genetics.113.157693
Gu, X., Zhang, Z., and Huang, W. (2005). Rapid evolution of expression and regulatory divergences after yeast gene duplication. Proc. Natl. Acad. Sci. U.S.A. 102, 707–712. doi: 10.1073/pnas.0409186102
Gu, Z., Rifkin, S. A., White, K. P., and Li, W. H. (2004). Duplicate genes increase gene expression diversity within and between species. Nat. Genet. 36, 577–579. doi: 10.1038/ng1355
Guydosh, N. R., and Green, R. (2014). Dom34 rescues ribosomes in 3′ untranslated regions. Cell 156, 950–962. doi: 10.1016/j.cell.2014.02.006
Habib, N., Wapinski, I., Margalit, H., Regev, A., and Friedman, N. (2012). A functional selection model explains evolutionary robustness despite plasticity in regulatory networks. Mol. Syst. Biol. 8:619. doi: 10.1038/msb.2012.50
Herrero, E., Belli, G., and Casa, C. (2010). Structural and functional diversity of glutaredoxins in yeast. Curr. Protein Pept. Sci 11, 659–668. doi: 10.2174/138920310794557637
Herrero, E., and Wellinger, R. E. (2015). Yeast as a model system to study metabolic impact of selenium compounds. Microb. Cell 2, 139–149. doi: 10.15698/mic2015.05.200
Huerta-Cepas, J., Capella-Gutierrez, S., Pryszcz, L. P., Marcet-Houben, M., and Gabaldon, T. (2014). PhylomeDB v4: zooming into the plurality of evolutionary histories of a genome. Nucleic Acids Res. 42:D897–D902. doi: 10.1093/nar/gkt1177
Icho, T., Lee, H. S., Sommer, S. S., and Wickner, R. B. (1986). Molecular characterization of chromosomal genes affecting double-stranded RNA replication in Saccharomyces cerevisiae. Basic Life Sci. 40, 165–171. doi: 10.1007/978-1-4684-5251-8_13
Ihmels, J., Bergmann, S., Gerami-Nejad, M., Yanai, I., McClellan, M., Berman, J., et al. (2005). Rewiring of the yeast transcriptional network through the evolution of motif usage. Science 309, 938–940. doi: 10.1126/science.1113833
Jacques, J. F., Mercier, A., Brault, A., Mourer, T., and Labbe, S. (2014). Fra2 is a co-regulator of Fep1 inhibition in response to iron starvation. PLoS One 9:e98959. doi: 10.1371/journal.pone.0098959
Johnson, A. D. (2017). The rewiring of transcription circuits in evolution. Curr. Opin. Genet. Dev. 47, 121–127. doi: 10.1016/j.gde.2017.09.004
Jourdren, L., Duclos, A., Brion, C., Portnoy, T., Mathis, H., Margeot, A., et al. (2010). Teolenn: an efficient and customizable workflow to design high-quality probes for microarray experiments. Nucleic Acids Res. 38:e117. doi: 10.1093/nar/gkq110
Juarez-Cepeda, J., Orta-Zavalza, E., Canas-Villamar, I., Arreola-Gomez, J., Perez-Cornejo, G. P., Hernandez-Carballo, C. Y., et al. (2015). The EPA2 adhesin encoding gene is responsive to oxidative stress in the opportunistic fungal pathogen Candida glabrata. Curr. Genet. 61, 529–544. doi: 10.1007/s00294-015-0473-2
Kaback, D. B., Oeller, P. W., Yde Steensma, H., Hirschman, J., Ruezinsky, D., Coleman, K. G., et al. (1984). Temperature-sensitive lethal mutations on yeast chromosome I appear to define only a small number of genes. Genetics 108, 67–90.
Kalinina, E. V., Chernov, N. N., and Novichkova, M. D. (2014). Role of glutathione, glutathione transferase, and glutaredoxin in regulation of redox-dependent processes. Biochemistry 79, 1562–1583.
Kasper, L., Seider, K., and Hube, B. (2015). Intracellular survival of Candida glabrata in macrophages: immune evasion and persistence. FEMS Yeast Res. 15:fov042. doi: 10.1093/femsyr/fov042
Kater, L., Thoms, M., Barrio-Garcia, C., Cheng, J., Ismail, S., Ahmed, Y. L., et al. (2017). Visualizing the assembly pathway of nucleolar Pre-60S ribosomes. Cell 171: 1599.e14–1610.e14. doi: 10.1016/j.cell.2017.11.039
Kaur, R., Ma, B., and Cormack, B. P. (2007). A family of glycosylphosphatidylinositol-linked aspartyl proteases is required for virulence of Candida glabrata. Proc. Natl. Acad. Sci. U.S.A. 104, 7628–7633. doi: 10.1073/pnas.0611195104
Kispal, G., Sipos, K., Lange, H., Fekete, Z., Bedekovics, T., Janaky, T., et al. (2005). Biogenesis of cytosolic ribosomes requires the essential iron-sulphur protein Rli1p and mitochondria. EMBO J. 24, 589–598. doi: 10.1038/sj.emboj.7600541
Koch, C., Konieczka, J., Delorey, T., Lyons, A., Socha, A., Davis, K., et al. (2017). Inference and evolutionary analysis of genome-scale regulatory networks in large phylogenies. Cell Syst. 4, 543.e8–558.e8. doi: 10.1016/j.cels.2017.04.010
Kumanovics, A., Chen, O. S., Li, L., Bagley, D., Adkins, E. M., Lin, H., et al. (2008). Identification of FRA1 and FRA2 as genes involved in regulating the yeast iron regulon in response to decreased mitochondrial iron-sulfur cluster synthesis. J. Biol. Chem. 283, 10276–10286. doi: 10.1074/jbc.M801160200
Kumar, C., Igbaria, A., D’Autreaux, B., Planson, A. G., Junot, C., Godat, E., et al. (2011). Glutathione revisited: a vital function in iron metabolism and ancillary role in thiol-redox control. EMBO J. 30, 2044–2056. doi: 10.1038/emboj.2011.105
Kuo, D., Tan, K., Zinman, G., Ravasi, T., Bar-Joseph, Z., and Ideker, T. (2010). Evolutionary divergence in the fungal response to fluconazole revealed by soft clustering. Genome Biol. 11:R77. doi: 10.1186/gb-2010-11-7-r77
Labbe, S., Khan, M. G., and Jacques, J. F. (2013). Iron uptake and regulation in Schizosaccharomyces pombe. Curr. Opin. Microbiol. 16, 669–676. doi: 10.1016/j.mib.2013.07.007
Lavoie, H., Hogues, H., Mallick, J., Sellam, A., Nantel, A., and Whiteway, M. (2010). Evolutionary tinkering with conserved components of a transcriptional regulatory network. PLoS Biol. 8:e1000329. doi: 10.1371/journal.pbio.1000329
Lebaron, S., Schneider, C., van Nues, R. W., Swiatkowska, A., Walsh, D., Bottcher, B., et al. (2012). Proofreading of pre-40S ribosome maturation by a translation initiation factor and 60S subunits. Nat. Struct. Mol. Biol. 19, 744–753. doi: 10.1038/nsmb.2308
Lemoine, S., Combes, F., Servant, N., and Le Crom, S. (2006). Goulphar: rapid access and expertise for standard two-color microarray normalization methods. BMC Bioinformatics 7:467. doi: 10.1186/1471-2105-7-467
Li, H., Mapolelo, D. T., Randeniya, S., Johnson, M. K., and Outten, C. E. (2012). Human glutaredoxin 3 forms [2Fe-2S]-bridged complexes with human BolA2. Biochemistry 51, 1687–1696. doi: 10.1021/bi2019089
Longtine, M. S., McKenzie, A. III, Demarini, D. J., Shah, N. G., Wach, A., Brachat, A., et al. (1998). Additional modules for versatile and economical PCR-based gene deletion and modification in Saccharomyces cerevisiae. Yeast 14, 953–961. doi: 10.1002/(SICI)1097-0061(199807)14:10<953::AID-YEA293>3.0.CO;2-U
Lynch, V. J., Leclerc, R. D., May, G., and Wagner, G. P. (2011). Transposon-mediated rewiring of gene regulatory networks contributed to the evolution of pregnancy in mammals. Nat. Genet. 43, 1154–1159. doi: 10.1038/ng.917
Lynch, V. J., Nnamani, M. C., Kapusta, A., Brayer, K., Plaza, S. L., Mazur, E. C., et al. (2015). Ancient transposable elements transformed the uterine regulatory landscape and transcriptome during the evolution of mammalian pregnancy. Cell Rep. 10, 551–561. doi: 10.1016/j.celrep.2014.12.052
Martinez-Pastor, M. T., Perea-Garcia, A., and Puig, S. (2017). Mechanisms of iron sensing and regulation in the yeast Saccharomyces cerevisiae. World J. Microbiol. Biotechnol. 33:75. doi: 10.1007/s11274-017-2215-8
Matsuo, R., Mizobuchi, S., Nakashima, M., Miki, K., Ayusawa, D., and Fujii, M. (2017). Central roles of iron in the regulation of oxidative stress in the yeast Saccharomyces cerevisiae. Curr. Genet. 63, 895–907. doi: 10.1007/s00294-017-0689-4
McGoldrick, S., O’Sullivan, S. M., and Sheehan, D. (2005). Glutathione transferase-like proteins encoded in genomes of yeasts and fungi: insights into evolution of a multifunctional protein superfamily. FEMS Microbiol. Lett. 242, 1–12. doi: 10.1016/j.femsle.2004.10.033
Merhej, J., Frigo, A., Le Crom, S., Camadro, J. M., Devaux, F., and Lelandais, G. (2014). bPeaks: a bioinformatics tool to detect transcription factor binding sites from ChIPseq data in yeasts and other organisms with small genomes. Yeast 31, 375–391. doi: 10.1002/yea.3031
Merhej, J., Delaveau, T., Guitard, J., Palancade, B., Hennequin, C., Garcia, M., et al. (2015). Yap7 is a transcriptional repressor of nitric oxide oxidase in yeasts, which arose from neofunctionalization after whole genome duplication. Mol. Microbiol. 96, 951–972. doi: 10.1111/mmi.12983
Merhej, J., Thiebaut, A., Blugeon, C., Pouch, J., Ali Chaouche Mel, A., Camadro, J. M., et al. (2016). A network of paralogous stress response transcription factors in the human pathogen Candida glabrata. Front. Microbiol. 7:645. doi: 10.3389/fmicb.2016.00645
Milhon, J. L., Albert, T. J., Vande Waa, E. A., O’Leary, K. A., Jackson, R. N., Kessler, M. A., et al. (2000). SmMAK16, the Schistosoma mansoni homologue of MAK16 from yeast, targets protein transport to the nucleolus. Mol. Biochem. Parasitol. 108, 225–236. doi: 10.1016/S0166-6851(00)00221-8
Mills, E. W., Wangen, J., Green, R., and Ingolia, N. T. (2016). Dynamic regulation of a ribosome rescue pathway in erythroid cells and platelets. Cell Rep. 17, 1–10. doi: 10.1016/j.celrep.2016.08.088
Molina, M. M., Belli, G., de la Torre, M. A., Rodriguez-Manzaneque, M. T., and Herrero, E. (2004). Nuclear monothiol glutaredoxins of Saccharomyces cerevisiae can function as mitochondrial glutaredoxins. J. Biol. Chem. 279, 51923–51930. doi: 10.1074/jbc.M410219200
Muhlenhoff, U., Molik, S., Godoy, J. R., Uzarska, M. A., Richter, N., Seubert, A., et al. (2010). Cytosolic monothiol glutaredoxins function in intracellular iron sensing and trafficking via their bound iron-sulfur cluster. Cell Metab. 12, 373–385. doi: 10.1016/j.cmet.2010.08.001
Nagi, M., Tanabe, K., Nakayama, H., Ueno, K., Yamagoe, S., Umeyama, T., et al. (2016). Iron-depletion promotes mitophagy to maintain mitochondrial integrity in pathogenic yeast Candida glabrata. Autophagy 12, 1259–1271. doi: 10.1080/15548627.2016.1183080
Nevitt, T., and Thiele, D. J. (2011). Host iron withholding demands siderophore utilization for Candida glabrata to survive macrophage killing. PLoS Pathog 7:e1001322. doi: 10.1371/journal.ppat.1001322
Ohtake, Y., and Wickner, R. B. (1995). Yeast virus propagation depends critically on free 60S ribosomal subunit concentration. Mol. Cell. Biol. 15, 2772–2781. doi: 10.1128/MCB.15.5.2772
Ojeda, L., Keller, G., Muhlenhoff, U., Rutherford, J. C., Lill, R., and Winge, D. R. (2006). Role of glutaredoxin-3 and glutaredoxin-4 in the iron regulation of the Aft1 transcriptional activator in Saccharomyces cerevisiae. J. Biol. Chem. 281, 17661–17669. doi: 10.1074/jbc.M602165200
Outten, C. E., and Albetel, A. N. (2013). Iron sensing and regulation in Saccharomyces cerevisiae: ironing out the mechanistic details. Curr. Opin. Microbiol. 16, 662–668. doi: 10.1016/j.mib.2013.07.020
Papp, B., Pal, C., and Hurst, L. D. (2003). Evolution of cis-regulatory elements in duplicated genes of yeast. Trends Genet. 19, 417–422. doi: 10.1016/S0168-9525(03)00174-4
Passos, D. O., Doma, M. K., Shoemaker, C. J., Muhlrad, D., Green, R., Weissman, J., et al. (2009). Analysis of Dom34 and its function in no-go decay. Mol. Biol. Cell 20, 3025–3032. doi: 10.1091/mbc.E09-01-0028
Paul, V. D., Muhlenhoff, U., Stumpfig, M., Seebacher, J., Kugler, K. G., Renicke, C., et al. (2015). The deca-GX3 proteins Yae1-Lto1 function as adaptors recruiting the ABC protein Rli1 for iron-sulfur cluster insertion. eLife 4:e08231. doi: 10.7554/eLife.08231
Pellett, S., and Tracy, J. W. (2006). Mak16p is required for the maturation of 25S and 5.8S rRNAs in the yeast Saccharomyces cerevisiae. Yeast 23, 495–506. doi: 10.1002/yea.1368
Perez-Sampietro, M., and Herrero, E. (2014). The PacC-family protein Rim101 prevents selenite toxicity in Saccharomyces cerevisiae by controlling vacuolar acidification. Fungal Genet. Biol. 71, 76–85. doi: 10.1016/j.fgb.2014.09.001
Perez-Sampietro, M., Serra-Cardona, A., Canadell, D., Casas, C., Arino, J., and Herrero, E. (2016). The yeast Aft2 transcription factor determines selenite toxicity by controlling the low affinity phosphate transport system. Sci. Rep. 6:32836. doi: 10.1038/srep32836
Perez, J. C., Fordyce, P. M., Lohse, M. B., Hanson-Smith, V., DeRisi, J. L., and Johnson, A. D. (2014). How duplicated transcription regulators can diversify to govern the expression of nonoverlapping sets of genes. Genes Dev. 28, 1272–1277. doi: 10.1101/gad.242271.114
Pfaller, M. A., and Diekema, D. J. (2007). Epidemiology of invasive candidiasis: a persistent public health problem. Clin. Microbiol. Rev. 20, 133–163. doi: 10.1128/CMR.00029-06
Pfaller, M. A., Andes, D. R., Diekema, D. J., Horn, D. L., Reboli, A. C., Rotstein, C., et al. (2014). Epidemiology and outcomes of invasive candidiasis due to non-albicans species of Candida in 2,496 patients: data from the prospective antifungal therapy (PATH) registry 2004-2008. PLoS One 9:e101510. doi: 10.1371/journal.pone.0101510
Pinson, B., Sagot, I., and Daignan-Fornier, B. (2000). Identification of genes affecting selenite toxicity and resistance in Saccharomyces cerevisiae. Mol. Microbiol. 36, 679–687. doi: 10.1046/j.1365-2958.2000.01890.x
Poor, C. B., Wegner, S. V., Li, H., Dlouhy, A. C., Schuermann, J. P., Sanishvili, R., et al. (2014). Molecular mechanism and structure of the Saccharomyces cerevisiae iron regulator Aft2. Proc. Natl. Acad. Sci. U.S.A. 111, 4043–4048. doi: 10.1073/pnas.1318869111
Pougach, K., Voet, A., Kondrashov, F. A., Voordeckers, K., Christiaens, J. F., Baying, B., et al. (2014). Duplication of a promiscuous transcription factor drives the emergence of a new regulatory network. Nat. Commun. 5:4868. doi: 10.1038/ncomms5868
Puig, O., Caspary, F., Rigaut, G., Rutz, B., Bouveret, E., Bragado-Nilsson, E., et al. (2001). The tandem affinity purification (TAP) method: a general procedure of protein complex purification. Methods 24, 218–229. doi: 10.1006/meth.2001.1183
Puig, S., Askeland, E., and Thiele, D. J. (2005). Coordinated remodeling of cellular metabolism during iron deficiency through targeted mRNA degradation. Cell 120, 99–110. doi: 10.1016/j.cell.2004.11.032
Puig, S., Vergara, S. V., and Thiele, D. J. (2008). Cooperation of two mRNA-binding proteins drives metabolic adaptation to iron deficiency. Cell Metab. 7, 555–564. doi: 10.1016/j.cmet.2008.04.010
Pujol-Carrion, N., Belli, G., Herrero, E., Nogues, A., and de la Torre-Ruiz, M. A. (2006). Glutaredoxins Grx3 and Grx4 regulate nuclear localisation of Aft1 and the oxidative stress response in Saccharomyces cerevisiae. J. Cell Sci. 119, 4554–4564. doi: 10.1242/jcs.03229
Pujol-Carrion, N., and de la Torre-Ruiz, M. A. (2010). Glutaredoxins Grx4 and Grx3 of Saccharomyces cerevisiae play a role in actin dynamics through their Trx domains, which contributes to oxidative stress resistance. Appl. Environ. Microbiol. 76, 7826–7835. doi: 10.1128/AEM.01755-10
Pujol-Carrion, N., and Torre-Ruiz, M. A. (2017). Physical interaction between the MAPK Slt2 of the PKC1-MAPK pathway and Grx3/Grx4 glutaredoxins is required for the oxidative stress response in budding yeast. Free Radic. Biol. Med. 103, 107–120. doi: 10.1016/j.freeradbiomed.2016.12.023
Quinlan, A. R., and Hall, I. M. (2010). BEDTools: a flexible suite of utilities for comparing genomic features. Bioinformatics 26, 841–842. doi: 10.1093/bioinformatics/btq033
Ritchie, M. E., Phipson, B., Wu, D., Hu, Y., Law, C. W., Shi, W., et al. (2015). limma powers differential expression analyses for RNA-sequencing and microarray studies. Nucleic Acids Res. 43:e47. doi: 10.1093/nar/gkv007
Roetzer, A., Gratz, N., Kovarik, P., and Schuller, C. (2010). Autophagy supports Candida glabrata survival during phagocytosis. Cell. Microbiol. 12, 199–216. doi: 10.1111/j.1462-5822.2009.01391.x
Roetzer, A., Gabaldon, T., and Schüller, C. (2011). From Saccharomyces cerevisiae to Candida glabrata in a few easy steps: important adaptations for an opportunistic pathogen. FEMS Microbiol. Lett. 314, 1–9. doi: 10.1111/j.1574-6968.2010.02102.x
Romero, I. G., Ruvinsky, I., and Gilad, Y. (2012). Comparative studies of gene expression and the evolution of gene regulation. Nat. Rev. Genet. 13, 505–516. doi: 10.1038/nrg3229
Roy, S., Wapinski, I., Pfiffner, J., French, C., Socha, A., Konieczka, J., et al. (2013). Arboretum: reconstruction and analysis of the evolutionary history of condition-specific transcriptional modules. Genome Res. 23, 1039–1050. doi: 10.1101/gr.146233.112
Roy, S., and Thompson, D. (2015). Evolution of regulatory networks in Candida glabrata: learning to live with the human host. FEMS Yeast Res. 15:fov087. doi: 10.1093/femsyr/fov087
Rutherford, J. C., Jaron, S., Ray, E., Brown, P. O., and Winge, D. R. (2001). A second iron-regulatory system in yeast independent of Aft1p. Proc. Natl. Acad. Sci. U.S.A. 98, 14322–14327. doi: 10.1073/pnas.261381198
Rutherford, J. C., Jaron, S., and Winge, D. R. (2003). Aft1p and Aft2p mediate iron-responsive gene expression in yeast through related promoter elements. J. Biol. Chem. 278, 27636–27643. doi: 10.1074/jbc.M300076200
Salin, H., Fardeau, V., Piccini, E., Lelandais, G., Tanty, V., Lemoine, S., et al. (2008). Structure and properties of transcriptional networks driving selenite stress response in yeasts. BMC Genomics 9:333. doi: 10.1186/1471-2164-9-333
Scannell, D. R., Zill, O. A., Rokas, A., Payen, C., Dunham, M. J., Eisen, M. B., et al. (2011). The Awesome power of yeast evolutionary genetics: new genome sequences and strain resources for the Saccharomyces sensu stricto Genus. G3 1, 11–25. doi: 10.1534/g3.111.000273
Schwarzmuller, T., Ma, B., Hiller, E., Istel, F., Tscherner, M., Brunke, S., et al. (2014). Systematic phenotyping of a large-scale Candida glabrata deletion collection reveals novel antifungal tolerance genes. PLoS Pathog 10:e1004211. doi: 10.1371/journal.ppat.1004211
Seider, K., Brunke, S., Schild, L., Jablonowski, N., Wilson, D., Majer, O., et al. (2011). The facultative intracellular pathogen Candida glabrata subverts macrophage cytokine production and phagolysosome maturation. J. Immunol. 187, 3072–3086. doi: 10.4049/jimmunol.1003730
Seider, K., Gerwien, F., Kasper, L., Allert, S., Brunke, S., Jablonowski, N., et al. (2014). Immune evasion, stress resistance, and efficient nutrient acquisition are crucial for intracellular survival of Candida glabrata within macrophages. Eukaryot. Cell 13, 170–183. doi: 10.1128/EC.00262-13
Shen, X. X., Zhou, X., Kominek, J., Kurtzman, C. P., Hittinger, C. T., and Rokas, A. (2016). Reconstructing the backbone of the saccharomycotina yeast phylogeny using genome-scale data. G3 6, 3927–3939. doi: 10.1534/g3.116.034744
Simms, C. L., Hudson, B. H., Mosior, J. W., Rangwala, A. S., and Zaher, H. S. (2014). An active role for the ribosome in determining the fate of oxidized mRNA. Cell Rep. 9, 1256–1264. doi: 10.1016/j.celrep.2014.10.042
Simms, C. L., Thomas, E. N., and Zaher, H. S. (2017). Ribosome-based quality control of mRNA and nascent peptides. Wiley Interdiscip. Rev. RNA 8:e1366. doi: 10.1002/wrna.1366
Srivastava, V. K., Suneetha, K. J., and Kaur, R. (2014). A systematic analysis reveals an essential role for high-affinity iron uptake system, haemolysin and CFEM domain-containing protein in iron homoeostasis and virulence in Candida glabrata. Biochem. J. 463, 103–114. doi: 10.1042/BJ20140598
Srivastava, V. K., Suneetha, K. J., and Kaur, R. (2015). The mitogen-activated protein kinase CgHog1 is required for iron homeostasis, adherence and virulence in Candida glabrata. FEBS J. 282, 2142–2166. doi: 10.1111/febs.13264
Strunk, B. S., Novak, M. N., Young, C. L., and Karbstein, K. (2012). A translation-like cycle is a quality control checkpoint for maturing 40S ribosome subunits. Cell 150, 111–121. doi: 10.1016/j.cell.2012.04.044
Teixeira, M. C., Monteiro, P. T., Palma, M., Costa, C., Godinho, C. P., Pais, P., et al. (2018). Yeastract: an upgraded database for the analysis of transcription regulatory networks in Saccharomyces cerevisiae. Nucleic Acids Res. 46:D348–D353. doi: 10.1093/nar/gkx842
Thomas-Chollier, M., Herrmann, C., Defrance, M., Sand, O., Thieffry, D., and van Helden, J. (2012). RSAT peak-motifs: motif analysis in full-size ChIP-seq datasets. Nucleic Acids Res. 40:e31. doi: 10.1093/nar/gkr1104
Thompson, D., Regev, A., and Roy, S. (2015). Comparative analysis of gene regulatory networks: from network reconstruction to evolution. Annu. Rev. Cell Dev. Biol. 31, 399–428. doi: 10.1146/annurev-cellbio-100913-012908
Thompson, D. A., Roy, S., Chan, M., Styczynsky, M. P., Pfiffner, J., French, C., et al. (2013). Evolutionary principles of modular gene regulation in yeasts. eLife 2:e00603. doi: 10.7554/eLife.00603
Thorvaldsdottir, H., Robinson, J. T., and Mesirov, J. P. (2013). Integrative Genomics Viewer (IGV): high-performance genomics data visualization and exploration. Brief. Bioinform. 14, 178–192. doi: 10.1093/bib/bbs017
Tirosh, I., and Barkai, N. (2007). Comparative analysis indicates regulatory neofunctionalization of yeast duplicates. Genome Biol. 8:R50. doi: 10.1186/gb-2007-8-4-r50
Ueta, R., Fujiwara, N., Iwai, K., and Yamaguchi-Iwai, Y. (2012). Iron-induced dissociation of the Aft1p transcriptional regulator from target gene promoters is an initial event in iron-dependent gene suppression. Mol. Cell. Biol. 32, 4998–5008. doi: 10.1128/MCB.00726-12
Vakirlis, N., Sarilar, V., Drillon, G., Fleiss, A., Agier, N., Meyniel, J. P., et al. (2016). Reconstruction of ancestral chromosome architecture and gene repertoire reveals principles of genome evolution in a model yeast genus. Genome Res. 26, 918–932. doi: 10.1101/gr.204420.116
Vall-Llaura, N., Reverter-Branchat, G., Vived, C., Weertman, N., Rodriguez-Colman, M. J., and Cabiscol, E. (2016). Reversible glutathionylation of Sir2 by monothiol glutaredoxins Grx3/4 regulates stress resistance. Free Radic. Biol. Med. 96, 45–56. doi: 10.1016/j.freeradbiomed.2016.04.008
van den Elzen, A. M., Schuller, A., Green, R., and Seraphin, B. (2014). Dom34-Hbs1 mediated dissociation of inactive 80S ribosomes promotes restart of translation after stress. EMBO J. 33, 265–276. doi: 10.1002/embj.201386123
Vitenshtein, A., Charpak-Amikam, Y., Yamin, R., Bauman, Y., Isaacson, B., Stein, N., et al. (2016). NK Cell recognition of Candida glabrata through binding of NKp46 and NCR1 to fungal ligands Epa1, Epa6, and Epa7. Cell Host Microbe 20, 527–534. doi: 10.1016/j.chom.2016.09.008
Wapinski, I., Pfeffer, A., Friedman, N., and Regev, A. (2007). Natural history and evolutionary principles of gene duplication in fungi. Nature 449, 54–61. doi: 10.1038/nature06107
Wapinski, I., Pfiffner, J., French, C., Socha, A., Thompson, D. A., and Regev, A. (2010). Gene duplication and the evolution of ribosomal protein gene regulation in yeast. Proc. Natl. Acad. Sci. U.S.A. 107, 5505–5510. doi: 10.1073/pnas.0911905107
Wickner, R. B., and Leibowitz, M. J. (1979). Mak mutants of yeast: mapping and characterization. J. Bacteriol. 140, 154–160.
Wickner, R. B., Koh, T. J., Crowley, J. C., O’Neil, J., and Kaback, D. B. (1987). Molecular cloning of chromosome I DNA from Saccharomyces cerevisiae: isolation of the MAK16 gene and analysis of an adjacent gene essential for growth at low temperatures. Yeast 3, 51–57. doi: 10.1002/yea.320030108
Yarunin, A., Panse, V. G., Petfalski, E., Dez, C., Tollervey, D., and Hurt, E. C. (2005). Functional link between ribosome formation and biogenesis of iron-sulfur proteins. EMBO J. 24, 580–588. doi: 10.1038/sj.emboj.7600540
Young, D. J., Guydosh, N.R., Zhang, F., Hinnebusch, A.G., and Green, R. (2015). Rli1/ABCE1 recycles terminating ribosomes and controls translation reinitiation in 3′UTRs in vivo. Cell 162, 872–884. doi: 10.1016/j.cell.2015.07.041
Zhang, Z., Gu, J., and Gu, X. (2004). How much expression divergence after yeast gene duplication could be explained by regulatory motif evolution? Trends Genet 20, 403–407.
Keywords: yeast, Aft, ChIP-seq, NO GO decay, evolution
Citation: Benchouaia M, Ripoche H, Sissoko M, Thiébaut A, Merhej J, Delaveau T, Fasseu L, Benaissa S, Lorieux G, Jourdren L, Le Crom S, Lelandais G, Corel E and Devaux F (2018) Comparative Transcriptomics Highlights New Features of the Iron Starvation Response in the Human Pathogen Candida glabrata. Front. Microbiol. 9:2689. doi: 10.3389/fmicb.2018.02689
Received: 13 July 2018; Accepted: 22 October 2018;
Published: 16 November 2018.
Edited by:
John P. Morrissey, University College Cork, IrelandReviewed by:
Cécile Neuvéglise, Institut National de la Recherche Agronomique (INRA), FranceSandra Paiva, University of Minho, Portugal
Gary Moran, Trinity College, Dublin, Ireland
Copyright © 2018 Benchouaia, Ripoche, Sissoko, Thiébaut, Merhej, Delaveau, Fasseu, Benaissa, Lorieux, Jourdren, Le Crom, Lelandais, Corel and Devaux. This is an open-access article distributed under the terms of the Creative Commons Attribution License (CC BY). The use, distribution or reproduction in other forums is permitted, provided the original author(s) and the copyright owner(s) are credited and that the original publication in this journal is cited, in accordance with accepted academic practice. No use, distribution or reproduction is permitted which does not comply with these terms.
*Correspondence: Frédéric Devaux, ZnJlZGVyaWMuZGV2YXV4QHVwbWMuZnI=
†These authors have contributed equally to this work