- 1Department of Biology, Institute for General Microbiology, Christian-Albrechts University Kiel, Kiel, Germany
- 2Department of Applied Ecology and Phycology, Institute of Biological Sciences, University of Rostock, Rostock, Germany
- 3Coastal Ecology, Alfred Wegener Institute, List on the island of Sylt, Germany
- 4Department of Evolutionary Genetics, Max Planck Institute for Evolutionary Biology, Plön, Germany
Marine multicellular organisms in composition with their associated microbiota—representing metaorganisms—are confronted with constantly changing environmental conditions. In 2110, the seawater temperature is predicted to be increased by ~5°C, and the atmospheric carbon dioxide partial pressure (pCO2) is expected to reach approximately 1000 ppm. In order to assess the response of marine metaorganisms to global changes, e.g., by effects on host-microbe interactions, we evaluated the response of epibacterial communities associated with Fucus vesiculosus forma mytili (F. mytili) to future climate conditions. During an 11-week lasting mesocosm experiment on the island of Sylt (Germany) in spring 2014, North Sea F. mytili individuals were exposed to elevated pCO2 (1000 ppm) and increased temperature levels (Δ+5°C). Both abiotic factors were tested for single and combined effects on the epibacterial community composition over time, with three replicates per treatment. The respective community structures of bacterial consortia associated to the surface of F. mytili were analyzed by Illumina MiSeq 16S rDNA amplicon sequencing after 0, 4, 8, and 11 weeks of treatment (in total 96 samples). The results demonstrated that the epibacterial community structure was strongly affected by temperature, but only weakly by elevated pCO2. No interaction effect of both factors was observed in the combined treatment. We identified several indicator operational taxonomic units (iOTUs) that were strongly influenced by the respective experimental factors. An OTU association network analysis revealed that relationships between OTUs were mainly governed by habitat. Overall, this study contributes to a better understanding of how epibacterial communities associated with F. mytili may adapt to future changes in seawater acidity and temperature, ultimately with potential consequences for host-microbe interactions.
Introduction
The surfaces of marine macroalgae offer a diverse substrate for attachment and are often colonized by a large variety of bacteria acquired from nearby macroalgae and from the surrounding waters (Lachnit et al., 2011; Egan et al., 2013; Stratil et al., 2013, 2014). It is further known that macroalgae affect and shape the biofilm formation and composition on its surface by either attracting or defending substances (Weinberger, 2007; Lachnit et al., 2010). Both, macroalgal host and microbiota mutually benefit from each other by e.g., nutrient exchange (Hellio et al., 2000; Goecke et al., 2010; Lachnit et al., 2010; Nasrolahi et al., 2012), and microbial biofilms attached to the surface offer a protective layer against e.g., settlement of larvae (Nasrolahi et al., 2012; Egan et al., 2013). Recent studies have shown that bacteria attached to macroalgal surfaces play important roles in the healthy development and life of their host (Egan et al., 2013; Singh and Reddy, 2014; Wichard, 2015). Moreover, Dittami and colleagues hypothesized optimized host-microbe-interactions to be essential for adaption to environmental changes (Dittami et al., 2015). In general, multicellular organisms have been recently recognized as “metaorganisms” comprising the eukaryotic host and its synergistic interdependence with microorganisms associated with the host (Bosch and McFall-Ngai, 2011; McFall-Ngai et al., 2013). Besides, the composition of the microbiota fundamentally depends on many biotic and abiotic factors, as well as numerous conditions (e.g., algal species, age, season, environmental parameters) as reviewed by Martin et al. (2014). Recent examples reported restructuring of marine bacterial communities on macroalgae in response to changing seawater temperature (Stratil et al., 2013), and pH-dependent community shifts on free-living marine bacteria even in response to small changes in pH due to elevated atmospheric carbon dioxide partial pressure (pCO2) levels (Krause et al., 2012). Besides, several other factors shaping microbial communities might be affected by environmental changes and need to be considered: e.g., the complex bacteria-bacteria interactions (cooperation and competition) within epibacterial communities, predatory bacteria like Bdellovibrio and like organisms (BALOs), or organic matter provided by the algal (recently reviewed by Dang and Lovell, 2016).
The atmospheric carbon dioxide (CO2) concentration constantly increases mainly as a result of human activities and dissolves in the oceans thus causing an increase in seawater acidity, designated as “ocean acidification” (Raven et al., 2005). As a second consequence, atmospheric CO2 acts as a greenhouse gas resulting in global warming (Levitus et al., 2000). According to the fifth IPCC report (RCP8.5) in 2110, the pCO2 is expected to reach ~1000 ppm and the seawater temperature is predicted to be increased by ~5°C (Raven et al., 2005; IPCC, 2013, 2014). To date, the impact of elevated pCO2 and increased temperature levels as predicted for the future ocean on marine bacterial communities remains poorly understood, in particular within complex benthic communities. The Sylt outdoor benthic mesocosm facility offers an ideal opportunity to simulate future underwater climate scenarios and thus to study their impacts on complex benthic communities on a large-scale (Stewart et al., 2013; Wahl et al., 2015; Pansch et al., 2016). Thus pCO2 and temperature in a benthic mesocosm experiment was manipulated in accordance to the described future climate predictions to study their single and combined impacts on a typical Wadden Sea benthic community including the key organism Fucus vesiculosus forma mytili (F. mytili).
F. mytili is a marine brown macroalga of the class Fucophyceae (Phaeophyceae) and is discussed to be a hybrid of the world-wide distributed F. vesiculosus and F. spiralis species (Nienburg, 1932; Coyer et al., 2006). However, F. mytili differs in many aspects from these two species (Albrecht, 1998). F. mytili typically grows on Wadden Sea mussel beds as found near the island of Sylt, thus is a key macroalgae for coastal ecosystems (Schories et al., 1997). To date the bacterial communities attached to the surface of North Sea F. mytili remained undescribed, as well as their response to changing environmental conditions. Here, the epibacterial community composition of F. mytili was investigated by 16S rDNA amplicon sequencing during a comprehensive 11-week benthic mesocosm experiment in spring 2014, in which F. mytili individuals were exposed to elevated pCO2 (1000 ppm) and increased temperature (+5°C) levels. Both abiotic stressors were tested for single and combined effects on the bacterial communities attached to the surface of F. mytili at the start and after 4, 8, and 11 weeks of treatment. In addition, the composition of free-living bacteria in the surrounding seawater was analyzed during the experiment. Several indicator operational taxonomic units (Fortunato et al., 2013) were identified for the observed pCO2 and temperature effects. In addition, we performed an OTU association network analysis to specifically address potential effects of the treatments on bacteria-bacteria interactions and thus examine the complex interactions among bacteria and their environment in a more comprehensive way. We further investigated the impacts of increasing seawater acidity and temperature on the F. mytili host individuals by determining relative growth rates and two physiological features, the carbon-to-nitrogen (C:N) ratio and the mannitol content, an important photoassimilate and storage compound in brown algae (Yamaguchi et al., 1966).
Materials and Methods
The Sylt Outdoor Benthic Mesocosms
For a detailed description of the tidal benthic mesocosm facility on the island of Sylt (located at the Wadden Sea Station of the Alfred-Wegener-Institute in List, Germany) see Pansch et al. (2016). In short, the benthic mesocosm facility was constructed to simulate near-natural North Sea underwater climate scenarios. The outdoor system consists of 12 independent experimental units (constructed of black HDPE = high-density polyethylene, Figure S1), each with a seawater capacity of 1800 L and covered with slanted, translucent lids. For seawater sampling, side ports are available at ~40 cm water depth. To mimic Wadden Sea conditions, low/high tide was simulated by moving the gratings up/down and changing direction of seawater flow every 6 h. In order to ensure sufficient nutrient concentrations ~1800 L seawater were added daily to each tank. The non-filtered seawater was provided by a pipeline with its inlet located 50 m offshore. Prior to distribution into the mesocosms, the seawater was transferred into storage tanks inside the institute to remove sediment particles. Seawater overflow was directed back into the sea.
Estimation of pCO2, Measurements of Water Parameters and Nutrients
The actual pCO2 was calculated from weekly measurements of total alkalinity (TA), acidity (total pH; see below), salinity and temperature using the CO2sys EXCEL Macro spreadsheet developed by Pierrot et al. (2006) for describing the marine carbonate system. The Multi Parameter Measurement System continuously measured seawater temperature and pH in the tanks. The pH was measured on NBS scale at 25°C (NBS = National Bureau of Standards; Dickson, 1984). Total pH values were calculated from NBS to total pH scale using the following equation: pH(x) = 8.0939 + ((Es − Ex)/0.05916) with Es = mV Dickson Tris buffer/1000, and Ex = mV sample at 25°C/1000. Additional samples to determine TA and to measure salinity were taken on a weekly basis (n = 3), as well as water samples for the measurement of inorganic nutrient concentrations of silicate (SiO), ammonium (NH), phosphate (PO), total nitrogen oxide (NOx), and nitrite (NO) by spectrophotometry. Respective nitrate (NO) concentrations were calculated as NOx − NO. For a detailed description of sampling and measurement procedures see (Pansch et al., 2016). All data concerning the water parameters and nutrients are provided as Supplementary Material (Figures S2, S3, respectively).
Experimental Setup and Treatments
In early April 2014, individual F. mytili thalli were collected in a Wadden Sea mussel bed (at 55°01′42.2″N 8°25′59.4″E). Several thalli (~15) were bundled with wire rope resulting in voluminous bundles with ~130 g wet weight on average. Eleven of these F. mytili bundles, hereinafter referred to as F. mytili individuals, were fixed on top of the 1.0 m2 grating inside of each tank. In addition, several organisms commonly found in the natural habitat of F. mytili (the blue mussel Mytilus edulis, the Pacific oyster Crassostrea gigas, the periwinkles Littorina littorea and L. mariae, and amphipods of the genus Gammarus spp.) were added consistently in defined biomass to the 12 tanks.
F. mytili individuals were incubated in the benthic mesocosms under four different conditions: (1) increased temperature (+5°C temperature at ambient pCO2), (2) elevated CO2 (1000 ppm pCO2 at ambient temperature), (3) increased temperature and elevated CO2 (+5°C temperature at 1000 ppm pCO2), and (4) ambient control (ambient temperature and ambient pCO2) with three tanks per treatment. As described in detail by Pansch et al. (2016), the seawater temperature in the tanks was monitored by internal sensors and automatically controlled by either heating rods or cooling units, to keep a delta of +5 °C in relation to the ambient control. The seawater pCO2 was manipulated by continuous injections of 1000 ppm pre-mixed CO2 gas (pure CO2 plus compressed air) directly into the water column. The lid ensured similar atmospheric and seawater pCO2levels. The temperature in the tanks was simulated based on two adjustable sinus curves (year and day), checked and adjusted based on field measurements at least once a week. The simulated temperature equals the measured seawater temperature in the tanks due to rapid temperature adjustment.
Sampling of F. mytili Biofilm and Surrounding Waters
Prior to sampling, the gratings carrying the F. mytili individuals were stopped shortly before low tide to keep the F. mytili individuals covered with seawater during the sampling procedure. Seawater and biofilm samples were taken at the beginning (t0) and after 4, 8, and 11 weeks of incubation (2014/04/09, 05/08, 06/05, and 06/25). Planktonic cells of the surrounding waters (1 L) were collected via vacuum filtration of the seawater through 0.2 μm Millipore Express PLUS polyethersulfone membrane filters (Millipore, Billerica, MA, USA) at max. −0.2 bar vacuum. Prior to biofilm sampling, the F. mytili thalli surfaces to be sampled were rinsed with 0.22 μm filtered seawater to remove loosely attached cells and particles. Subsequently, swabs were taken from the surfaces (~15 cm2 by visual estimation) using sterile cotton swabs. Swabs and filters were placed on ice during sampling and stored at −80°C until DNA extraction. Importantly, during the experiment biofilm samples were taken from the same F. mytili individuals (one F. mytili at a defined position on each grating) from the upper, younger parts of several thalli. To prevent resampling of the same region, sampled thalli were labeled with small cable straps.
DNA Extraction and PCR Amplification of Bacterial 16S rDNA for Illumina MiSeq Amplicon Sequencing
Genomic DNA (gDNA) was isolated using the Isol-RNA Lysis Reagent (5 PRIME, Gaithersburg, MD, USA) according to the manual section “Isolation of genomic DNA” with small modifications: Only half the volume of each component was used and the speed of centrifugation steps was generally increased to 12,000 x g. Cotton tips and membrane filters were removed prior to chloroform addition. The hypervariable region V1-V2 of the bacterial 16S rDNA was amplified from gDNA (~100 ng μL−1) using the primer set 27-forward and 338-reverse (Youssef et al., 2010). Beside the target-specific region each primer sequence contained a linker sequence, an 8-base identifier index and the Illumina specific region P5 (forward primer) or P7 (reverse primer), respectively, as recently described by Kozich et al. (2013). The PCR reaction mixture and amplification conditions were performed as described by Löscher et al. (2015). The PCR products were checked for correct size (~350 bp amplicon length) and band intensity, then correct amplicons were purified from 1% agarose gels using the MinElute Gel Extraction Kit (Qiagen, Hilden, Germany). The purified amplicons were quantified using a NanoDrop 1000 spectrophotometer (Thermo Fisher Scientific, Waltham, MA, USA), pooled in equimolar ratio and sequenced according to the manufacturer's protocol on a MiSeq Instrument using the MiSeq reagent Kit V3 chemistry (Illumina, San Diego, CA, USA). Sequences were submitted to the NCBI Sequence Read Archive under accession number SRP069256.
Bioinformatic Processing
Sequence processing was performed using mothur version 1.34.4 (Schloss et al., 2009; Kozich et al., 2013). Raw reads were concatenated to 9,364,598 contiguous sequences (contigs) using the command make.contig. Contigs with ambiguous bases or homopolymers longer than 8 bases as well as contigs longer than 552 bases were removed using screen.seqs. The remaining 8,354,564 contigs were screened for redundant sequences using unique.seqs and clustered into 2,537,511 unique sequences. The sequences were consecutively aligned (with align.seqs) to a modified version of the SILVA database release 102 (Pruesse et al., 2007) containing only the hypervariable regions V1 and V2. Sequences not aligning in the expected region were removed from the dataset with screen.seqs. The alignment was condensed by removing gap-only columns with filter.seqs. The final alignment contained 8,288,816 sequences (2,511,577 unique) of lengths between 253 and 450 bases. Rare and closely related sequences were clustered using unique.seqs and precluster.seqs. The latter was used to include sequences with up to three positional differences compared to larger sequence clusters into the latter. Chimeric sequences were removed using the Uchime algorithm (Edgar et al., 2011) via the command chimera.uchime, followed by remove.seqs. This left 7,934,922 sequences (163,446 unique) in the dataset. Sequence classification was performed using the Wang Method (Wang et al., 2007) on a modified Greengenes database (containing only the hypervariable regions V1 and V2) with a bootstrap threshold of 80%. Sequences belonging to the kingdom archaea, to chloroplasts or mitochondria were removed from the dataset using remove.lineage. OTUs (operational taxonomic units) were formed using the average neighbor clustering method with cluster.split. Parallelization of this step was done taking the taxonomic classification on the order level into account. A sample-by-OTU table containing 55,378 OTUs at the 97% level was generated using make.shared. OTUs were classified taxonomically using the modified Greengenes database mentioned above and the command classify.otu.
Statistics on Bacterial 16S rDNA Amplicon Data
Statistical downstream analysis was performed with custom scripts in R v3.1.3 (R Core Team, 2015). OTUs of very low abundance only increase computation time without contributing useful information. They were thus removed from the dataset as follows: After transformation of counts in the sample-by-OTU table to relative abundances (based on the total number of reads per sample), OTUs were ordered by decreasing mean percentage across samples. The set of ordered OTUs for which the cumulative mean percentage amounted to 95% was retained in the filtered OTU table, resulting in a decrease in the number of OTUs from 55,378 to 4,157.
The extent of change in relative OTU abundance across samples explained by the experimental factors Temperature, pCO2, Time, and Sample Type (see Table S1) was explored by redundancy analysis (RDA) with Hellinger-transformed OTU counts (Stratil et al., 2013, 2014; Langfeldt et al., 2014) using function rda of R package vegan v2.4-0 (Oksanen et al., 2015).
Model selection started with a full RDA model containing all main effects and interactions of experimental factors, using the following model formula:
The Week main effect was omitted from the formula as temporal effects were nested within other levels or their combinations. (Inclusion of a Week main effect would be justified if measures for factor Week were completely independent rather than repeated). A permutation scheme for permutation-based significance tests was chosen with function how of R package permute v0.8-4 (Simpson, 2015) to reflect the repeated-measures design as well as the temporal nature of factor Week. Permutation of samples within a sample unit (a set of repeated measures taken for a particular combination of Type, Temperature and pCO2 at the different time points of Week; see Table S1) was set to “series,” with the same permutation used for each sample unit; clusters of samples belonging to different sample units were allowed to be permuted freely.
The full model was simplified by backward selection with function ordistep. The final RDA model exhibited significant interaction effects CO2:Week and Type:Temp:Week (see Results Section). It was thus necessary to evaluate (i) the Temperature effect within each level of Type and Week and (ii) the pCO2 treatment effect within each level of Week. For (i) the effect of the Temperature:Week interaction was evaluated within each level of factor Type in appropriate submodels of the final RDA model; upon significance, the Temperature effect was further evaluated within each level of factor Week (within the same level of factor Type). For (ii) the pCO2 effect was evaluated within each level of factor Week in appropriate submodels of the final RDA model. p-values for each stage of these hierarchical testing schemes were corrected for multiple testing by Benjamini–Hochberg correction (false discovery rate, FDR; Benjamini and Hochberg, 1995). For each significant submodel, OTUs were determined that were significantly correlated with any axis in the RDA submodel by function envfit with 105 permutations, followed by Benjamini–Hochberg correction. In order to reduce the number of tests in this procedure, OTUs were pre-filtered according to their vector lengths calculated from corresponding RDA scores (scaling 1) by profile likelihood selection (Zhu and Ghodsi, 2006).
OTUs significant at an FDR of 5% were further subject to indicator analysis with function multipatt of the R package indicspecies v1.7.5 (De Cáceres and Legendre, 2009) with 105 permutations. Indicator OTUs (iOTUs)—in analogy to indicator species sensu De Cáceres and Legendre (2009)—are OTUs that prevail in a certain sample group (here: either a level of pCO2 within a certain level of Week, or a level of Temperature within a certain sample Type and level of Week) while being found only irregularly and at low abundance in other sample groups.
3D visualizations of the final RDA model were produced in kinemage format (Richardson and Richardson, 1992) using the R package R2Kinemage developed by S.C.N., and displayed in KiNG v2.21 (Chen et al., 2009). For alpha diversity analysis, effective OTU richness (Shannon numbers equivalent, 1D; Jost, 2006, 2007) was calculated from the filtered OTU table. Multi-panel alpha diversity and iOTU plots were drawn with R package lattice v0.20-33 (Sarkar, 2008).
An OTU association network was inferred with the R package SpiecEasi v0.1 (Kurtz et al., 2015). OTUs selected for network analysis were required to be present in ≥60% of all samples to reduce the number of zeros in the data for a robust calculation, resulting in a set of 97 OTUs. Calculations were performed with the Meinshausen and Bühlmann neighborhood selection framework (MB method; Meinshausen and Bühlmann, 2006). Correlations between associated OTUs were determined from the centered log-ratio-transformed counts. The igraph package v1.0.1 (Csárdi and Nepusz, 2006) was employed for visualizing moderate to strong OTU associations (absolute correlation ≥0.6).
Relative Biomass Growth Rates of F. mytili Macroalgal Hosts
Biomass growth of all F. mytili individuals was measured prior to and after the experiment (n = 11 per treatment) as wet weight. Biomass change (described by the relative growth rate RGR) for wet mass was calculated according to Lüning et al. (1990) using the formula:
where m0 represents the initial wet mass (g) and mt the wet mass (g) after t days (d).
F. mytili Algal Tissue Sampling and Physiological Analysis (C:N, Mannitol)
In order to assess the physiological parameters of F. mytili individuals after growing for 11 weeks under the four different treatments the thallus material was cut, cleaned of epibiota and freeze-dried for further analyses (n = 3 per treatment; same individuals used for biofilm sampling). Prior to the experiment, thallus material without visible epiphytes of six initial F. mytili individuals was freeze-dried to document the initial physiological status of F. mytili in its native habitat. For analyzing carbon (C) and nitrogen (N) contents, freeze-dried algal material was ground to powder using mortar and pistil. Three subsamples of 2 mg from each vegetative apex were loaded and packed into tin cartridges (6 × 6 × 12 mm). These packages were combusted at 950°C and the absolute contents of C and N were automatically quantified in an elemental analyzer (Elementar Vario EL III, Germany) using acetanilide as standard according to Verardo et al. (1990). Mannitol was extracted from three powdered subsamples of 10–20 mg freeze-dried alga material, and quantified by HPLC as described by Karsten et al. (1991).
Statistical Analysis of F. mytili Macroalgal Data
In order to evaluate the interaction effect of temperature and pCO2 on all variables measured (relative biomass growth rates, C:N ratio, C, N and mannitol content) at the end of the experiment, two-way ANOVAs were used with temperature and pCO2 as fixed factors. When the analysis did not show significant interactions, a one-way ANOVA was carried out for each factor separately. When the one-way ANOVA revealed significant differences, a post hoc Tukey's honest significant difference test was applied. Prior to the use of ANOVAs, data were tested for normality with the Kolmogorov-Smirnov and for homogeneity with the Levene's test. Data were analyzed using SPSS Statistics 20 (IBM, Armonk, NY, USA).
Results
Bacterial Community Patterns Depended on the Sample Type and Varied in Time
The bacterial community pattern of F. mytili biofilm and water samples strongly varied from each other (Biofilm F(11, 36) = 3.91, p = 0.003; Water F(11, 36) = 7.06, p = 0.001; Table S2). A distance biplot visualizing the general type effect throughout the experiment is shown in Figure 1, with samples of F. mytili biofilm and water forming separated clusters. Variance partitioning showed that the type effect alone (i.e., with the influence of other variables removed) explained ~36% of the variance in the dataset.
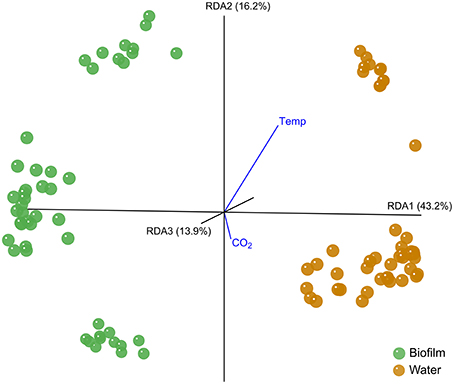
Figure 1. Distance biplot of the type effect. The type effect on Fucus mytili biofilm (green) and water (orange) samples is visualized with KiNG at RDA axes RDA1 43.2%, RDA2 16.2%, and RDA3 13.9% explained variance. Temperature and CO2 treatment effects are visualized as vectors pointing in negative direction of axis RDA3 (away from the observer). The analysis is based on MiSeq V1-V2 bacterial 16S rDNA amplicon sequence data on 97% sequence similarity.
In every week and treatment, the biofilm attached to the F. mytili surface was generally dominated by (in descending order) Proteobacteria (mainly Alpha- and Gamma-, fewer Delta-, and Betaproteobacteria), Bacteroidetes (Flavobacteria, Saprospirae, Cytophagia, BME43 cluster), and Actinobacteria (Acidimicrobiia; Figure 2). Epibacterial community composition was highly variable between F. mytili replicates. At ambient conditions, Alpha- and Gammaproteobacteria together comprised up to ~50% of the epibacterial community on F. mytili. About 25% of the community consisted of Flavobacteriia and Saprospirae.
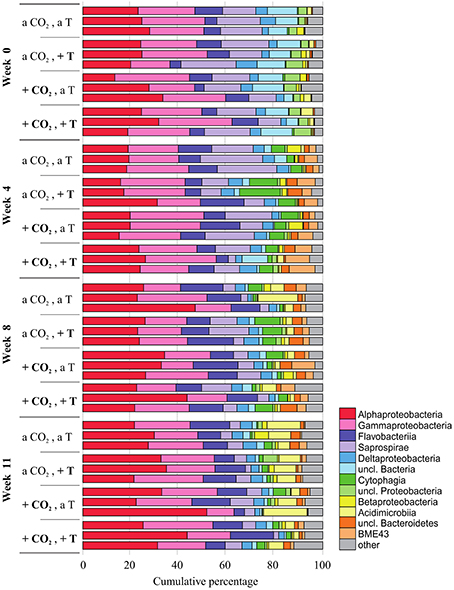
Figure 2. Relative abundances (in cumulative percentage) of the most abundant epibacterial classes of Fucus mytili. Epibacterial community patterns are depicted for each sample (n = 3 per treatment) after 0, 4, 8, and 11 weeks of treatment in the benthic mesocosms at different conditions (see Section “Materials and Methods”; a, ambient; +, increased/elevated). Analysis is based on bacterial 16S rDNA (V1–V2) MiSeq amplicon sequencing. Low abundant classes (≤ 1%) were summarized as “other.”
Alpha-, Gammaproteobacteria, and Flavobacteriia accounted for a major proportion of the free-living bacterial community in the seawater (Figure S4). In contrast to F. mytili biofilm the variation between replicates of water samples was smaller. Concerning biofilm and water samples, respectively, a week-wise evaluation based on OTU level revealed a development over time with weeks 0, 4, 8, and 11, remarkably (F ≥ 2) differing in their community composition (Figure S5).
Alpha Diversity of F. mytili Microbiota was Much Higher than in Seawater
Alpha diversity was expressed as effective OTU richness 1D (also known as Shannon numbers equivalents; Jost, 2006, 2007). In water samples, 1D showed small variation with markedly lower median values in the course of the experiment (max. ~50) compared to the F. mytili biofilm (up to median values of ~300; Figure 3). Unexpectedly, no clear differences in 1D were observed between pCO2 and temperature treatments compared to ambient controls, although biofilm samples showed a tendency of higher 1D in warm treatments (e.g., in week 8 the median value of warm treatments was ~290 vs. 160 under ambient control conditions). In general, diversity of F. mytili biofilm samples showed high variability with a tendency of higher 1D during the experiment compared to the initial time point (median value ~60).
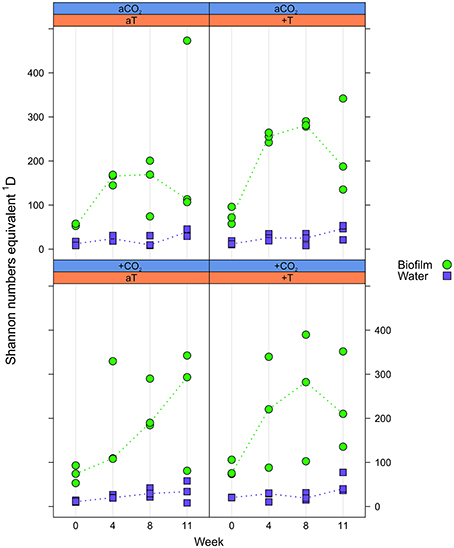
Figure 3. Effective OTU richness. Distribution of Shannon number equivalents 1D at OTU level concerning Fucus mytili biofilm (green circles) and water samples (blue diamonds) after 0, 4, 8, and 11 weeks of incubation in the benthic mesocosms under different conditions (see Section “Materials and Methods”; a, ambient; +, increased/elevated). Dots represent 1D of each single sample and dotted lines connect the medians (n = 3) between weeks.
Elevated pCO2 Affected All Bacterial Communities Similarly
The pCO2 treatment resulted in a constant difference of ~0.2 pH units between ambient and elevated pCO2 levels; resulting in a delta of ~300 μatm pCO2 in the seawater (Figure S2). The inorganic nutrient concentrations were not affected by this pCO2 treatment (Figure S3). A weak time-dependent effect of pCO2 exclusively in week 8 [F(1, 9) = 1.44, p ≈ 0.014] reshaped all bacterial communities in response to elevated pCO2 levels (Table S2). Further no interaction effect of pCO2 with temperature was observed. The pCO2 effect in week 8 explained 1.1% of the total variance. Comparatively few OTUs were affected by pCO2. Indicator OTUs (iOTUs) for elevated pCO2 (+CO2) belonged to the classes Flavobacteriia, BME43, Alpha-, Delta-, and Gammaproteobacteria, whereas iOTUs for ambient pCO2 (aCO2) are members of the Oscillatoriophycideae and Saprospirae (Figure 4). Highest differences in relative abundances due to +CO2 treatment were found among Flavobacteriia and Alphaproteobacteria, with noticeable iOTUs for +CO2 belonging to the genera Pseudoruegeria (OTU #7461, c_Alphaproteobacteria) and Sediminicola (OTU #1326, c_Flavobacteriia) with 0.38 and 2.79%0 in +CO2 compared to 0.01 and 0.38%0 in aCO2, respectively.
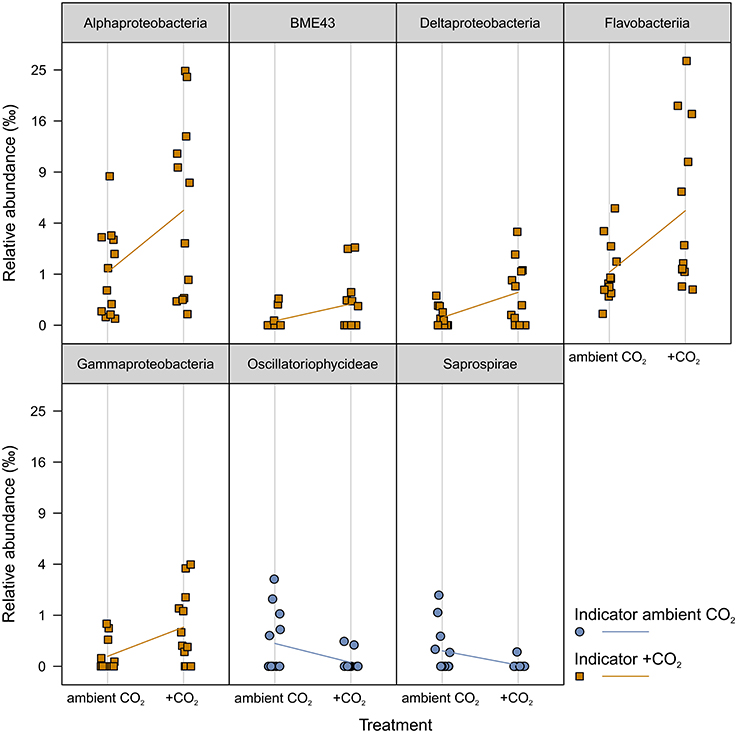
Figure 4. Indicator OTUs (iOTUs) for pCO2 on all samples. Relative abundances in %0 of single iOTUs for ambient (blue circles) and elevated (orange squares) pCO2 in week 8 summarized at class level (q ≤ 0.05) per sample (n = 12). Lines connect the mean relative abundances between both treatment levels. +CO2, elevated pCO2 treatment.
Increased Temperature Affected the Sample Types Differently
The temperature treatment resulted in a constant difference of ~5°C above ambient seawater temperature, with temperature naturally increasing during the course of the experiment (Figure S2). Inorganic nutrients were not affected by the temperature treatment (Figure S3). The microbiota significantly changed due to the temperature effect in weeks 4, 8, and 11, depending on the sample type (Biofilm:Temp:Week interaction F = 1.66, p = 0.006 and Water:Temp:Week interaction F = 1.66, p = 0.015; Table S2).
Epibacterial communities on F. mytili were significantly affected by temperature in weeks 4, 8, and 11 (p = 0.007, p = 0.002, and p = 0.001, respectively). The temperature effect on biofilm in weeks 4, 8, and 11 explained 10, 7, and 10% of total variance, respectively. Bacteria of the surrounding water column were significantly affected by temperature in weeks 4 and 8 (p = 0.004 and p = 0.008, respectively), while in week 11 there was only a trend detectable (p = 0.074). The temperature effect on water communities in weeks 4, 8, and 11 explained 18, 9, and 5 % of total variance, respectively.
iOTUs for temperature effects on F. mytili biofilms in weeks 4, 8, and 11 belonged to 17 bacterial classes: Alpha-, Beta-, Gamma-, Deltaproteobacteria, BME43, Cytophagia, Flavobacteriia, Saprospirae, Acidimicrobiia, Anaerolineae, OM190, Opitutae, Phycisphaerae, Sphingobacteriia, Verrucomicrobiae, Gemm-2, and Oscillatoriophycideae (Figure 5). Most classes comprised iOTUs for both temperature levels, meaning that members of the same class were differently affected by temperature. In particular, iOTUs of Alpha-, Delta- and Gammaproteobacteria were present in each week and showed remarkably high differences in relative abundance between both treatment levels: Within Alphaproteobacteria most iOTUs belonged to the families Rhodobacteraceae and Hyphomonadaceae, and were found in both temperature levels. Among Rhodobacteraceae, Octadecabacter antarcticus (OTU#79) was an iOTU for ambient temperature (aT) with 4.72%0 mean relative abundance in week 11 compared to 0.46%0 at increased temperature (+T).
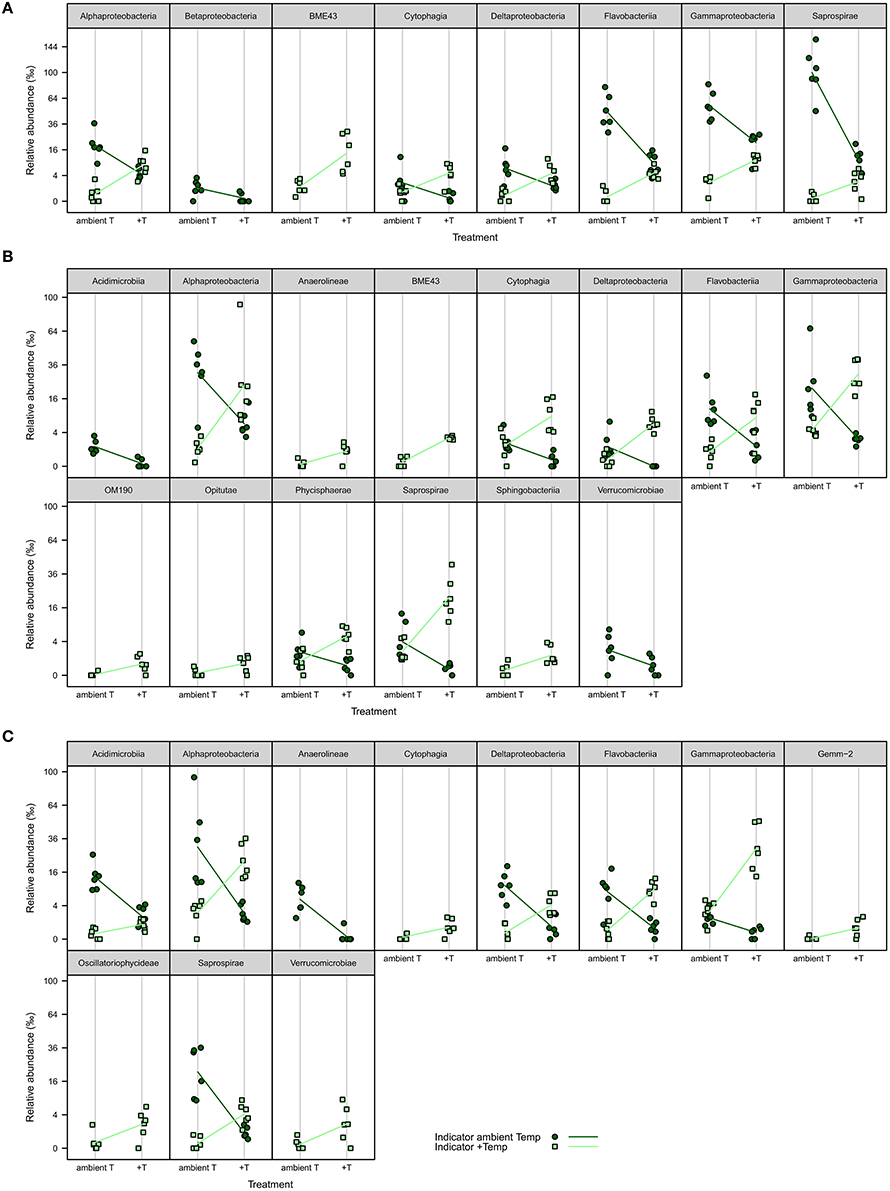
Figure 5. iOTUs for temperature on Fucus mytili. Relative abundances in %0 of single iOTUs for ambient (dark green circles) and increased (light green squares) temperature on F. mytili in (A) week 4, (B) week 8, and (C) week 11 summarized at class level (q ≤ 0.05) per sample (n = 6). Lines connect the mean relative abundances between both treatment levels. +T, increased temperature treatment.
Most iOTUs within Deltaproteobacteria were members of the orders Myxococcales and Bdellovibrionales. Although both orders varied between aT and +T, Myxococcales (family Nannocystaceae) were more common at +T and Bdellovibrionales (family Bacteriovoraceae) more often at aT.
Within Gammaproteobacteria, iOTUs for both temperature levels belonged to the orders Alteromonadales, Thiotrichales, Legionellales and Vibrionales. In week 8, iOTUs for +T were assigned to family Coxiellaceae (OTU#1164/2823, 2.02%0 +T vs. 0.05%0 aT) and for aT to genus Shewanella (OTU#186, 1.70%0 +T vs. 0.00%0 aT). In week 11, iOTUs for +T belonged to family Piscirickettsiaceae (OTU#215/360/525/562/5390, 2.78%0 +T vs. 0.47%0 aT) and genus Vibrio (OTU#204, 3.33%0 +T vs. 0.02%0 aT), whereas an iOTU for aT belonged to Candidatus Endobugula (OTU#2952, 0.19%0 +T vs. 0.81%0 aT). The relative abundance of gammaproteobacterial iOTUs for +T remarkably increased from weeks 4–11, with a concurrent decrease of gammaproteobacterial iOTUs for aT.
iOTUs for temperature effects on the water community in weeks 4 and 8 belonged to five bacterial classes: In week 4, iOTUs for +T belonged to Flavobacteriia, including members of the families Flavobacteriaceae and Cryomorphaceae, whereas iOTUs for aT belonged to the class Saprospirae (Figure 6). In week 8, OTUs of Sphingobacteriia were indicators for +T and OTUs of Alphaproteobacteria for aT. Gammaproteobacteria comprised iOTUs of both temperature levels, including iOTUs for +T of the family Coxiellaceae (OTU#1164, 4.96%0 +T vs. 0.01%0 aT) and the genus Vibrio (OTU#335, 1.34%0 +T vs. 0.00%0 aT), as well as iOTUs for aT of the genus Candidatus Portiera (OTU#6, 23.88%0 +T vs. 75.92%0 aT) and the family Colwelliaceae (OTU#193, 0.34%0 +T vs. 2.66%0 aT).
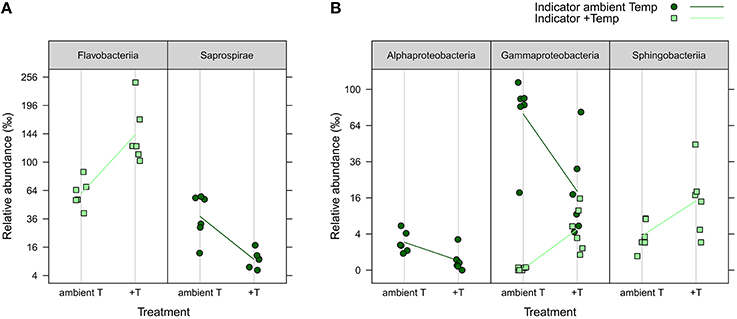
Figure 6. iOTUs for temperature on seawater. Relative abundances in %0 of single iOTUs for ambient (dark green circles) and increased (light green squares) temperature in the water column in (A) week 4 and (B) week 8 summarized at class level (q ≤ 0.05) per sample (n = 6). Lines connect the mean relative abundances between both treatment levels. +T, increased temperature treatment.
Correlations between Associated OTUs within the Bacterial Communities
OTU association network analysis revealed moderate to strong interactions between 25 OTUs (sorted by class-level taxonomy, indicator property and environmental distribution, respectively; see Figure S6 and Table S3). About half of the network-forming OTUs belong to Alphaproteobacteria, complemented by OTUs of Gammaproteobacteria, Flavobacteriia, Betaproteobacteria, Saprospirae, and the BME43 cluster. Both positive and negative correlations between OTUs were detected, the latter between OTUs prevailing in different habitats (F. mytili biofilm vs. seawater) suggesting that relevant (i.e., moderate to strong) correlations between OTUs were mainly influenced by the environment and less by bacterial interactions.
Increased Temperature Reduced Relative Biomass Growth Rates of the Host F. mytili
In order to test the effects of increased temperature and elevated pCO2 levels on the physiological performance of the host-algae itself, the change in biomass of all F. mytili individuals (n = 11 per treatment) was analyzed (Figure 7). At ambient temperature, elevated pCO2 conditions slightly increased the growth rate of F. mytili (1.5 ± 0.3% d−1; mean ± SD; for definition of growth rate see Section“Materials and Methods”) compared to ambient pCO2 (1.4 ± 0.3% d−1), however this tendency was not significant (one-way ANOVA, F = 2.42, dfn = 3, dfd = 8, p = 0.14). Warming reduced growth rate of F. mytili significantly by 20% over the course of the experiment (two-way ANOVA, F = 5.58, dfn = 1, dfd = 10, p < 0.05). Growth rates of F. mytili under elevated pCO2 conditions at increased temperature tended to be higher (1.4 ± 0.4% d−1) compared to growth under warming alone (1.3 ± 0.3% d−1).
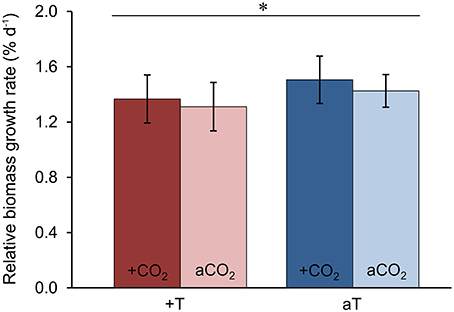
Figure 7. Growth of Fucus mytili. Relative biomass growth rates in % d−1 of F. mytili algae after 11 weeks of incubation in the benthic mesocosms under different conditions (see Section “Materials and Methods”; a, ambient; +, increased/elevated). Mean values ± SD (n = 11) and asterisk (p < 0.05*) indicates significantly different changes of growth rates (two-way ANOVA) driven by temperature effect (p = 0.046*, F = 5.58, dfn = 1, dfd = 10).
Algal Mannitol Contents and C:N Ratios Were Not Affected by Treatments
The carbon-to-nitrogen (C:N) ratio and the mannitol content were analyzed of F. mytili individuals used for biofilm sampling in the course of the entire experiment (n = 3 per treatment; see Table 1). The mannitol and carbon concentrations did not significantly change in the course of the experiment comparing initial and final values (one-way ANOVAs with post hoc Tukey's test, mannitol: F = 1.04, dfn = 4, dfd = 13, C: F = 1.56, dfn = 4, dfd = 13, p > 0.05). However, the C:N ratios obtained from samples at the end of the experiment were significantly higher for samples under all treatments compared to the initial values apparently due to significant decrease of the internal nitrogen concentration over time (one-way ANOVAs with post hoc Tukey's test, CN: F = 11.18, dfn = 4, dfd = 13, N: F = 1.56, dfn = 4, dfd = 13, p < 0.001). After 11 weeks of incubation, C:N ratios and mannitol concentrations did not significantly differ between all treatments (one-way ANOVAs with post hoc Tukey's test, CN: F = 0.53, dfn = 3, dfd = 8, mannitol: F = 0.51, dfn = 3, dfd = 8, p > 0.05).
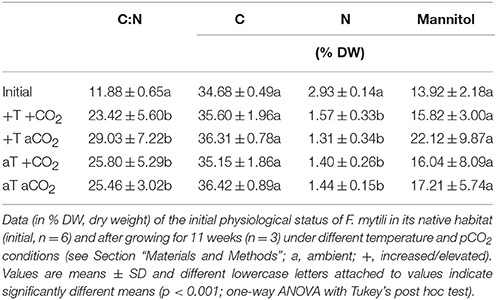
Table 1. Analysis of Fucus mytili tissue: carbon-to-nitrogen (C:N) ratios, C, N, and mannitol contents.
Discussion
Microbiota of Wadden Sea F. mytili Comparable to That of Baltic F. vesiculosus
This first analysis of the epibacterial community composition of Wadden Sea F. vesiculosus forma mytili (F. mytili) demonstrated that the surface of F. mytili was colonized by Proteobacteria (Alpha-, Gamma-, Delta-, Betaproteobacteria), Bacteroidetes (Flavobacteriia, Saprospirae, Cytophagia, BME43 cluster), and Actinobacteria (Acidimicrobiia). Two previous studies on the closely related macroalgal species, Baltic F. vesiculosus, also revealed Proteobacteria (Alpha-, Gamma-, Delta-, Beta-), Bacteroidetes, and Actinobacteria to be the most abundant phyla (Stratil et al., 2013, 2014). The latter was obtained from 16S rDNA V1-V2 amplicon pyrosequencing (454 Roche), hence offering a reliable comparison to our MiSeq amplicon data on F. mytili biofilms, in particular when restricted on the most abundant phyla or classes. The similarities between the epibacterial community compositions of North Sea F. mytili and Baltic F. vesiculosus was an unexpected finding, since the respective habitats highly differ in environmental conditions (e.g., salinity, nutrient concentrations and tidal range conditions). Thus the observed similarity of the epibacterial communities might be due to close genetic relationship of both hosts (Coyer et al., 2006) and might indicate similar host mechanisms to shape surface biofilm establishment. Our findings are in agreement with a comparative 16S rDNA cloning study of six macroalgal species (including F. vesiculosus) occurring in the Baltic and North Sea, respectively, which indicated less differences in epibacterial community composition between habitats than between different host species, moreover, the biofilms were more similar on closely related host species (Lachnit et al., 2009).
Although Planctomycetes are often reported to occur on macroalgae in remarkably high abundances (Bondoso et al., 2014; Lage and Bondoso, 2014), we rarely detected this phylum on F. mytili (e.g., class Phycisphaerae < 0.2% on average per week). However, the respective studies of those reports were mostly based on culture-based analysis (Lage and Bondoso, 2011) and thus do not necessarily reflect natural bacterial abundances. In agreement with our current sequencing results, Stratil and colleagues detected no Planctomycetes on Baltic F. vesiculosus also using a high-throughput sequencing approach (Stratil et al., 2013, 2014). Nonetheless, using 16S rDNA cloning and Sanger sequencing, we previously detected Planctomycetes on Baltic macroalgae, but simultaneously showed high variation in relative abundances of Planctomycetes between seasons/years and different macroalgae (Lachnit et al., 2011). Interestingly, Bengtsson et al. (2012) employed 16S amplicon pyrosequencing and found Planctomycetes to be the most abundant phylum (up to 56% of sequence reads) attached to the kelp Laminaria hyperborea. This finding again is in agreement with the already mentioned more general observation of Lachnit et al. (2009), that rather the host species than the geographic location determines the epibacterial community on macroalgae.
Impact of Elevated pCO2 on Bacterial Communities
Although the pCO2 treatment was successfully applied to the Sylt mesocosms, the effect on the bacterial communities attached to the algal surface and the planktonic ones was rather weak. This might be due to the fact that the majority of all environmental bacteria are known to have broader growth ranges with pH optima between pH 6 and 8 (Clark et al., 2009), thus they might be able to adapt to rapidly changing pH fluctuations. Moreover, it has been hypothesized by Mu and Moreau (2015) that biofilms exhibit larger tolerance to CO2 stress, which might explain the limited impact of the pCO2 treatment on the F. mytili biofilm community.
Nevertheless, the pCO2 effect was significant and we identified indicator OTUs (iOTUs): iOTUs of the genera Pseudoruegeria and Sediminicola showed higher relative abundance at elevated pCO2 levels. The fact that they are usually found at tidal flats (Jung et al., 2010) and deep-sea marine sediments (Hwang et al., 2015) where pCO2 is high due to organic matter decomposition, respectively, let us assume a—albeit slight—shift toward bacterial groups that are more adapted to higher pCO2 levels.
In contrast, in a previous microcosm study the CO2 effect appeared to be much more pronounced (Krause et al., 2012), however, these results are based on laboratory incubation scale and different sequencing techniques were used. This points to the general difficulty that the comparison of datasets obtained by different sequencing approaches are strongly limited due to a large variety of factors: In particular due to the chosen sequencing technology (e.g., Sanger sequencing, 454 Roche pyrosequencing, or Illumina MiSeq/HiSeq sequencing) and the sequenced region (e.g., full-length 16S rDNA or specific hypervariable regions of the 16S rDNA).
Overall our data suggested a pCO2 effect weaker than expected from recent literature. Previous studies with strong pCO2 effects refer to natural CO2-rich sites with long-term exposure of bacteria to high pCO2 (Oppermann et al., 2010), or experiments with overestimated pCO2 values up to 3000 μatm (Endres et al., 2014). Both do not reflect realistic future climate scenarios, as simulated in our mesocosm approach representing a near-natural approach with realistic evaluation of how marine bacterial communities could respond to future changes in seawater temperature and pCO2.
Increased Temperature Affected both Biofilm and Water Bacteria
Although most microorganisms are able to grow within a broad range of temperature (Clark et al., 2009), the temperature effect was markedly stronger than the pCO2 effect. Among epibacterial communities of F. mytili, we identified remarkably more temperature-related iOTUs than among seawater bacterial communities. Attached to the surface of F. mytili, an interesting iOTU for ambient temperature was O. antarcticus, known as cold-adapted species originally isolated from Antarctic Sea ice and water (Gosink et al., 1997). Additionally, the genera Shewanella and C. Endobugula were found to be more abundant at ambient temperature. They are known to be associated with fish tissue (Gram and Melchiorsen, 1996) and described as bacterial symbiont of Bryozoa (Lim and Haygood, 2004), respectively, thus seem to be related to healthy biofilms on a broad range of marine hosts. On the other hand, iOTUs of Nannocystaceae were indicators for increased temperature. Some members of this family were reported to degrade complex macromolecules and to lyse microorganisms (Garcia and Müller, 2014). Interestingly, we found several iOTUs associated with pathogenic features to be more abundant at increased temperature: The families Coxiellaceae (including pathogenic parasites; Lory, 2014) and Piscirickettsiaceae (including fish pathogens; Fryer and Hedrick, 2003), as well as the genus Vibrio. The latter comprises several thermophilic species associated with aquatic animal diseases like V. harveyi which is a pathogen of aquatic animals (Austin and Zhang, 2006). In agreement with our findings, Vezzulli and colleagues reported that temperature promotes Vibrio growth in the aquatic environment, supported by long-term data from the North Sea (Vezzulli et al., 2012, 2013).
In the water column increased temperature also led to significant shifts in community patterns. Remarkably, Gammaproteobacteria include iOTUs for both increased and ambient temperature, respectively. While typical marine members of the orders Oceanospirillales (genus C. Portiera) and Alteromonadales (family Colwelliaceae) were temperature-sensitive, some iOTUs of the orders Legionellales (family Coxiellaceae), and Vibrionales (genus Vibrio) increased in abundance with higher seawater temperature. In the latter case, Coxiellaceae and Vibrio include some parasites and pathogenic species, respectively (Austin and Zhang, 2006; Lory, 2014).
Overall, the increase in seawater temperature appeared to favor potentially pathogenic species over common and widespread bacteria. At increased seawater temperatures, our data showed a correlation between higher abundances of potentially pathogenic bacteria and reduced F. mytili biomass formation. Probably, thermally stressed F. mytili individuals might also exhibit reduced defense mechanisms, thus were more susceptible to infections.
Bacteria-Bacteria Interactions Described by OTU Association Network
Using network analysis, most associations were found between alphaproteobacterial OTUs. Basically, Alphaproteobacteria represented the most abundant class in both F. mytili biofilm and seawater samples, respectively, indicating that abundant bacteria have high impact on microbial structure and function (Lupatini et al., 2014). Positive correlations between OTUs suggest mutualistic interactions, while negative correlations might suggest a competition between bacteria (Steele et al., 2011). However, our network analysis showed negative correlations between OTUs to be due to environmental preferences (F. mytili surface or water column; Figure S6C).
Two typical water-associated OTUs, OTU#2 (Flavobacteriaceae, g_Sediminicola), and OTU#4 (Cryomorphaceae) were found to be strongly positively correlated with OTU#3 (Rhodobacteraceae, g_Octadecabacter), also predominantly found in water. Interestingly, while the former two OTUs were indicators for increased temperature in week 4 in water, OTU#3 was an indicator for ambient temperature in week 4 on F. mytili. This counterintuitive result becomes plausible if one keeps in mind that physiological status and activities can differ between surface-associated and free-living cells of the same taxon (Dang and Lovell, 2016). Thus, as part of the F. mytili biofilm OTU#3 may well prefer higher temperatures than it does as free-living cells.
We found Bdellovibrio to be an indicator for ambient temperature levels on F. mytili (week 11). However, no potential predatory OTUs (e.g., BALOs; Williams and Piñeiro, 2007) were found in the network, because they were not among the OTUs consistently present across the majority of samples. Thus, our dataset does not provide evidence for predatory bacteria to be involved in reshaping the communities as reviewed by Dang and Lovell (2016). However, important impacts of predatory bacteria on bacterial community restructuring might occur during other seasons.
F. mytili Growth Rates But Not Physiological Features Were Affected by Treatments
Our data showed significantly reduced growth rates of F. mytili at increased temperatures often reaching daily maxima of 24°C, suggesting that increased seawater temperature reduced biomass formation of this macroalga. Recently, Graiff et al. (2015a) described an inhibitory temperature effect on biomass growth rates of Baltic F. vesiculosus in most seasons in a comparable experimental design. Here, 24°C was a thermal threshold with biomass production of Baltic F. vesiculosus starting to stagnate, and above 24°C growth rates declined probably because physiological cell processes were affected (Graiff et al., 2015b). Elevated pCO2 appeared to enhance growth of F. mytili even under warmed conditions. The provision of increased amounts of dissolved inorganic carbon (DIC) may have increased—antagonistically to warming—the performance of F. mytili. This finding is consistent with Graiff et al. (2015a), who examined the combined effects of pCO2 and temperature on Baltic F. vesiculosus under similar conditions. Mitigating effects of enhanced pCO2 on growth performance of thermally stressed algae were previously reported, e.g., for the red alga Chondrus crispus (Sarker et al., 2013).
Contrary to the growth rates of F. mytili, C:N ratios and mannitol concentrations were not significantly affected by the applied treatments. This is in agreement with findings on Baltic F. vesiculosus studied under natural conditions (Lehvo et al., 2001) as well as under similar temperature and pCO2 treatments in the Kiel Outdoor Benthocosms (Graiff et al., 2015a). Further evidence is provided by Gutow et al. (2014) detecting no significant pCO2 effect on C:N ratios of North Sea F. vesiculosus. The C:N ratios of North Sea F. mytili were comparable to that found in Baltic F. vesiculosus during spring (Graiff et al., 2015a). Highest differences between initial (at the beginning of April) and final (at the end of June) nitrogen concentrations were typically found in spring for both Fucus species. These results suggest high internal nitrogen storage during winter to spring (before the spring bloom depleted the dissolved inorganic nitrogen of seawater) for stimulating rapid summer growth in nitrogen-depleted conditions (Lehvo et al., 2001). Thus, the increasing C:N ratios of F. mytili during our experiment resulted from decreasing nitrogen concentrations in the seawater (leading to nitrogen limitation due to the spring phytoplankton bloom) and depletion of the internal nitrogen storage of F. mytili due to investment into increased growth.
Mannitol represents a major end product of photosynthesis in brown algae (Phaeophyceae) and serves as energy source for growth and/or survival during the dark winter period (Dunton and Schell, 1986; Dunton and Jodwalis, 1988; Lehvo et al., 2001; Wiencke et al., 2009; Rousvoal et al., 2011; Gómez and Huovinen, 2012). Here, mannitol contents of F. mytili are very similar to those in Baltic F. vesiculosus (Graiff et al., 2015a), suggesting high mannitol accumulation in spring and summer for maintaining growth in winter months (Lehvo et al., 2001). The mannitol concentration in F. mytili did not change significantly over time, neither due to the applied treatments indicating that the photosynthesis was not influenced by increased temperature or elevated pCO2. Interestingly, both Fucus species showed highest variation in mannitol concentrations when exposed to increased temperature (+5°C, single factor treatment) during spring (Graiff et al., 2015a), probably due to individual phenotypic plasticity. Although the Wadden Sea F. mytili appeared to be as well adapted as the Baltic F. vesiculosus to the conditions of northern latitudes (Lehvo et al., 2001), we cannot rule out future F. mytili dying during the summer period when exposed to an increased seawater temperature by +5°C, as recently reported for Baltic F. vesiculosus (Graiff et al., 2015a). In the latter case, F. mytili would become the limiting factor with severe consequences for the marine ecosystem.
Overall we obtained evidence indicating that increasing seawater temperature as predicted for the future ocean might result in significant restructuring of the epibacterial community on F. mytili. Moreover, this might significantly increase the number of potentially pathogenic bacteria attached to the surface of the marine macroalgae, which ultimately will have consequences for the host-microbe interactions.
Author Contributions
BM designed the study, carried out sampling and sample processing, analyzed, and interpreted the data, and wrote the manuscript. SN performed the statistical analyses on the sequencing data. AG analyzed the algal tissue and performed statistics on all algae-related data. AP measured the water parameters and nutrients, and maintained technical support of the mesocosms. MF designed the MiSeq primer and supported the bioinformatical analysis. SK sequenced the amplicon samples. RS designed the experiment, took part in writing the manuscript, founded and supervised the work. All authors gave input to the manuscript.
Conflict of Interest Statement
Conflict of Interest Statement: The authors declare that the research was conducted in the absence of any commercial or financial relationships that could be construed as a potential conflict of interest.
Acknowledgments
We thank Juliane Müller and Julia Regnery for technical support in C:N and mannitol analyses. We are grateful to all colleagues involved in the experimental setup, in particular Vera Winde and Rafael Meichßner, as well as Chris Hoffmann for contribution in sample processing. We acknowledge the AWI for the access to the mesocosm facility. This study was performed in the framework of BIOACID II (Biological Impacts of Ocean Acidification, Phase II, 03F0655M/Schmitz-Streit), financially supported by the Federal Ministry of Education and Research (BMBF, Germany).
Supplementary Material
The Supplementary Material for this article can be found online at: http://journal.frontiersin.org/article/10.3389/fmicb.2016.00434
References
Albrecht, A. S. (1998). Soft bottom versus hard rock: community ecology of macroalgae on intertidal mussel beds in the Wadden Sea. J. Exp. Mar. Biol. Ecol. 229, 85–109. doi: 10.1016/S0022-0981(98)00044-6
Austin, B., and Zhang, X. H. (2006). Vibrio harveyi: a significant pathogen of marine vertebrates and invertebrates. Lett. Appl. Microbiol. 43, 119–124. doi: 10.1111/j.1472-765X.2006.01989.x
Bengtsson, M. M., Sjøtun, K., Lanzén, A., and Ovreås, L. (2012). Bacterial diversity in relation to secondary production and succession on surfaces of the kelp Laminaria hyperborea. ISME J. 6, 2188–2198. doi: 10.1038/ismej.2012.67
Benjamini, Y., and Hochberg, Y. (1995). Controlling the false discovery rate - a practical and powerful approach to multiple testing. J. R. Stat. Soc. B Methodol. 57, 289–300.
Bondoso, J., Balagué, V., Gasol, J. M., and Lage, O. M. (2014). Community composition of the Planctomycetes associated with different macroalgae. FEMS Microbiol. Ecol. 88, 445–456. doi: 10.1111/1574-6941.12258
Bosch, T. C. G., and McFall-Ngai, M. J. (2011). Metaorganisms as the new frontier. Zoology 114, 185–190. doi: 10.1016/j.zool.2011.04.001
Chen, V. B., Davis, I. W., and Richardson, D. C. (2009). KiNG (Kinemage, Next Generation): a versatile interactive molecular and scientific visualization program. Protein Sci. 18, 2403–2409. doi: 10.1002/pro.250
Clark, D. P., Dunlap, P., Madigan, M., and Martinko, J. (2009). Brock Biology of Microorganisms. Beijing: Scientific Publisher.
Coyer, J. A., Hoarau, G., Oudot-Le Secq, M. P., Stam, W. T., and Olsen, J. L. (2006). A mtDNA-based phylogeny of the brown algal genus Fucus (Heterokontophyta; Phaeophyta). Mol. Phylogenet. Evol. 39, 209–222. doi: 10.1016/j.ympev.2006.01.019
Csárdi, G., and Nepusz, T. (2006). The Igraph Software Package for Complex Network Research. Kalamazoo, MI; Budapest: InterJournal Complex Systems, 1695.
Dang, H., and Lovell, C. R. (2016). Microbial surface colonization and biofilm development in marine environments. Microbiol. Mol. Biol. Rev. 80, 91–138. doi: 10.1128/MMBR.00037-15
De Cáceres, M., and Legendre, P. (2009). Associations between species and groups of sites: indices and statistical inference. Ecology 90, 3566–3574. doi: 10.1890/08-1823.1
Dickson, A. G. (1984). Ph scales and proton-transfer reactions in saline media such as sea-water. Geochim. Cosmochim. Acta 48, 2299–2308. doi: 10.1016/0016-7037(84)90225-4
Dittami, S. M., Gobet, A., Duboscq-Bidot, L., Perennou, M., Corre, E., Frioux, C., et al. (2015). Microbiomes impact algal acclimation: the example of a freshwater strain of ectocarpus. Eur. J. Phycol. 50, 44–44.
Dunton, K. H., and Jodwalis, C. M. (1988). Photosynthetic performance of laminaria-solidungula measured insitu in the alaskan high arctic. Mar. Biol. 98, 277–285. doi: 10.1007/BF00391206
Dunton, K. H., and Schell, D. M. (1986). Seasonal carbon budget and growth of laminaria-solidungula in the alaskan high arctic. Mar. Ecol. Prog. Ser. 31, 57–66. doi: 10.3354/meps031057
Edgar, R. C., Haas, B. J., Clemente, J. C., Quince, C., and Knight, R. (2011). UCHIME improves sensitivity and speed of chimera detection. Bioinformatics 27, 2194–2200. doi: 10.1093/bioinformatics/btr381
Egan, S., Harder, T., Burke, C., Steinberg, P., Kjelleberg, S., and Thomas, T. (2013). The seaweed holobiont: understanding seaweed-bacteria interactions. FEMS Microbiol. Rev. 37, 462–476. doi: 10.1111/1574-6976.12011
Endres, S., Galgani, L., Riebesell, U., Schulz, K. G., and Engel, A. (2014). Stimulated bacterial growth under elevated pCO(2): results from an off-shore mesocosm study. PLoS ONE 9:e99228. doi: 10.1371/journal.pone.0099228
Fortunato, C. S., Eiler, A., Herfort, L., Needoba, J. A., Peterson, T. D., and Crump, B. C. (2013). Determining indicator taxa across spatial and seasonal gradients in the Columbia River coastal margin. ISME J. 7, 1899–1911. doi: 10.1038/ismej.2013.79
Fryer, J. L., and Hedrick, R. P. (2003). Piscirickettsia salmonis: a Gram-negative intracellular bacterial pathogen of fish. J. Fish Dis. 26, 251–262. doi: 10.1046/j.1365-2761.2003.00460.x
Garcia, R., and Müller, R. (2014). “The Family Nannocystaceae,” in The Prokaryotes, eds E. Rosenberg, E. Delong, S. Lory, E. Stackebrandt, and F. Thompson (Berlin; Heidelberg: Springer), 213–229.
Goecke, F., Labes, A., Wiese, J., and Imhoff, J. F. (2010). Chemical interactions between marine macroalgae and bacteria. Mar. Ecol. Prog. Ser. 409, 267–299. doi: 10.3354/meps08607
Gómez, I., and Huovinen, P. (2012). “Morpho-functionality of carbon metabolism in seaweeds,” in Seaweed Biology, eds C. Wiencke and K. Bischof (Berlin; Heidelberg: Springer), 25–46.
Gosink, J. J., Herwig, R. P., and Staley, J. T. (1997). Octadecabacter arcticus gen nov, sp nov, and O. antarcticus, sp nov, nonpigmented, psychrophilic gas vacuolate bacteria from polar sea ice and water. Syst. Appl. Microbiol. 20, 356–365. doi: 10.1016/S0723-2020(97)80003-3
Graiff, A., Bartsch, I., Ruth, W., Wahl, M., and Karsten, U. (2015a). Season exerts differential effects of ocean acidification and warming on growth and carbon metabolism of the seaweed Fucus vesiculosus in the western Baltic Sea. Front. Mar. Sci. 2:112. doi: 10.3389/fmars.2015.00112
Graiff, A., Liesner, D., Karsten, U., and Bartsch, I. (2015b). Temperature tolerance of western Baltic Sea Fucus vesiculosus - growth, photosynthesis and survival. J. Exp. Mar. Biol. Ecol. 471, 8–16. doi: 10.1016/j.jembe.2015.05.009
Gram, L., and Melchiorsen, J. (1996). Interaction between fish spoilage bacteria Pseudomonas sp. and Shewanella putrefaciens in fish extracts and on fish tissue. J. Appl. Bacteriol. 80, 589–595. doi: 10.1111/j.1365-2672.1996.tb03262.x
Gutow, L., Rahman, M. M., Bartl, K., Saborowski, R., Bartsch, I., and Wiencke, C. (2014). Ocean acidification affects growth but not nutritional quality of the seaweed Fucus vesiculosus (Phaeophyceae, Fucales). J. Exp. Mar. Biol. Ecol. 453, 84–90. doi: 10.1016/j.jembe.2014.01.005
Hellio, C., Bremer, G., Pons, A. M., Le Gal, Y., and Bourgougnon, N. (2000). Inhibition of the development of microorganisms (bacteria and fungi) by extracts of marine algae from Brittany, France. Appl. Microbiol. Biotechnol. 54, 543–549. doi: 10.1007/s002530000413
Hwang, C. Y., Lee, I., Cho, Y. R., Lee, Y. M., Jung, Y. J., Baek, K., et al. (2015). Sediminicola arcticus sp nov., a psychrophilic bacterium isolated from deep-sea sediment, and emended description of the genus Sediminicola. Int. J. Syst. Evol. Microbiol. 65, 1567–1571. doi: 10.1099/ijs.0.000138
IPCC (2013). Climate Change 2013: The Physical Science Basis. Contribution of Working Group I to the Fifth Assessment Report of the Intergovernmental Panel on Climate Change, eds T. F. Stocker, D. Qin, G.-K. Plattner, M. Tignor, S. K. Allen, J. Boschung, A. Nauels, Y. Xia, V. Bex, and P. M. Midgley (Cambridge, UK; New York, NY: Cambridge University Press), 1535.
IPCC, (2014). Climate Change 2014: Impacts, Adaptation, and Vulnerability. Part A: Global and Sectoral Aspects. Contribution of Working Group II to the Fifth Assessment Report of the Intergovernmental Panel on Climate Change, eds C. B. Field, V. R. Barros, D. J. Dokken, K. J. Mach, M. D. Mastrandrea, T. E. Bilir, M. Chatterjee, K. L. Ebi, Y. O. Estrada, R. C. Genova, B. Girma, E. S. Kissel, A. N. Levy, S. MacCracken, P. R. Mastrandrea, and L. L. White (Cambridge, UK; New York, NY: Cambridge University Press), 1132.
Jost, L. (2007). Partitioning diversity into independent alpha and beta components. Ecology 88, 2427–2439. doi: 10.1890/06-1736.1
Jung, Y. T., Kim, B. H., Oh, T. K., and Yoon, J. H. (2010). Pseudoruegeria lutimaris sp nov., isolated from a tidal flat sediment, and emended description of the genus Pseudoruegeria. Int. J. Syst. Evol. Microbiol. 60, 1177–1181. doi: 10.1099/ijs.0.015073-0
Karsten, U., Thomas, D. N., Weykam, G., Daniel, C., and Kirst, G. O. (1991). A simple and rapid method for extraction and separation of low-molecular-weight carbohydrates from macroalgae using high-performance liquid-chromatography. Plant Physiol. Biochem. 29, 373–378.
Kozich, J. J., Westcott, S. L., Baxter, N. T., Highlander, S. K., and Schloss, P. D. (2013). Development of a dual-index sequencing strategy and curation pipeline for analyzing amplicon sequence data on the miseq illumina sequencing platform. Appl. Environ. Microbiol. 79, 5112–5120. doi: 10.1128/AEM.01043-13
Krause, E., Wichels, A., Giménez, L., Lunau, M., Schilhabel, M. B., and Gerdts, G. (2012). Small changes in pH have direct effects on marine bacterial community composition: a microcosm approach. PLoS ONE 7:e47035. doi: 10.1371/journal.pone.0047035
Kurtz, Z. D., Müller, C. L., Miraldi, E. R., Littman, D. R., Blaser, M. J., and Bonneau, R. A. (2015). Sparse and compositionally robust inference of microbial ecological networks. PLoS Comput. Biol. 11:e1004226. doi: 10.1371/journal.pcbi.1004226
Lachnit, T., Blumel, M., Imhoff, J. F., and Wahl, M. (2009). Specific epibacterial communities on macroalgae: phylogeny matters more than habitat. Aquat. Biol. 5, 181–186. doi: 10.3354/ab00149
Lachnit, T., Meske, D., Wahl, M., Harder, T., and Schmitz, R. (2011). Epibacterial community patterns on marine macroalgae are host-specific but temporally variable. Environ. Microbiol. 13, 655–665. doi: 10.1111/j.1462-2920.2010.02371.x
Lachnit, T., Wahl, M., and Harder, T. (2010). Isolated thallus-associated compounds from the macroalga Fucus vesiculosus mediate bacterial surface colonization in the field similar to that on the natural alga. Biofouling 26, 247–255. doi: 10.1080/08927010903474189
Lage, O. M., and Bondoso, J. (2011). Planctomycetes diversity associated with macroalgae. FEMS Microbiol. Ecol. 78, 366–375. doi: 10.1111/j.1574-6941.2011.01168.x
Lage, O. M., and Bondoso, J. (2014). Planctomycetes and rnacroalgae, a striking association. Front. Microbiol. 5:267. doi: 10.3389/fmicb.2014.00267
Langfeldt, D., Neulinger, S. C., Heuer, W., Staufenbiel, I., Kunzel, S., Baines, J. F., et al. (2014). Composition of microbial oral biofilms during maturation in young healthy adults. PLoS ONE 9:e87499. doi: 10.1371/journal.pone.0087449
Lehvo, A., Back, S., and Kiirikki, M. (2001). Growth of Fucus vesiculosus L. (Phaeophyta) in the northern Baltic proper: energy and nitrogen storage in seasonal environment. Botan. Mar. 44, 345–350. doi: 10.1515/BOT.2001.044
Levitus, S., Antonov, J. I., Boyer, T. P., and Stephens, C. (2000). Warming of the world ocean. Science 287, 2225–2229. doi: 10.1126/science.287.5461.2225
Lim, G. E., and Haygood, M. G. (2004). “Candidatus endobugula glebosa,” a specific bacterial symbiont of the marine bryozoan Bugula simplex. Appl. Environ. Microbiol. 70, 4921–4929. doi: 10.1128/AEM.70.8.4921-4929.2004
Lory, S. (2014). “The Family Coxiellaceae,” in The Prokaryotes, eds E. Rosenberg, E. Delong, S. Lory, E. Stackebrandt, and F. Thompson (Berlin; Heidelberg: Springer), 197–198.
Löscher, C. R., Fischer, M. A., Neulinger, S. C., Fiedler, B., Philippi, M., Schutte, F., et al. (2015). Hidden biosphere in an oxygen-deficient Atlantic open-ocean eddy: future implications of ocean deoxygenation on primary production in the eastern tropical North Atlantic. Biogeosciences 12, 7467–7482. doi: 10.5194/bg-12-7467-2015
Lüning, K., Yarish, C., and Kirkman, H. (1990). Seaweeds: Their Environment, Biogeography, and Ecophysiology. New York, NY: John Wiley & Sons.
Lupatini, M., Suleiman, A., Jacques, R., Antoniolli, Z., Ferreira, A., Kuramae, E. E., et al. (2014). Network topology reveal high connectance levels and few key microbial genera within soils. Front. Environ. Sci. 2:10. doi: 10.3389/fenvs.2014.00010
Martin, M., Portetelle, D., Michel, G., and Vandenbol, M. (2014). Microorganisms living on macroalgae: diversity, interactions, and biotechnological applications. Appl. Microbiol. Biotechnol. 98, 2917–2935. doi: 10.1007/s00253-014-5557-2
McFall-Ngai, M., Hadfield, M. G., Bosch, T. C. G., Carey, H. V., Domazet-Lošo, T., Douglas, A. E., et al. (2013). Animals in a bacterial world, a new imperative for the life sciences. Proc. Natl. Acad. Sci. U.S.A. 110, 3229–3236. doi: 10.1073/pnas.1218525110
Meinshausen, N., and Bühlmann, P. (2006). High-dimensional graphs and variable selection with the lasso. Ann. Stat. 34, 1436–1462. doi: 10.1214/009053606000000281
Mu, A., and Moreau, J. W. (2015). The geomicrobiology of CO2 geosequestration: a focused review on prokaryotic community responses to field-scale CO2 injection. Front. Microbiol. 6:263. doi: 10.3389/fmicb.2015.00263
Nasrolahi, A., Stratil, S. B., Jacob, K. J., and Wahl, M. (2012). A protective coat of microorganisms on macroalgae: inhibitory effects of bacterial biofilms and epibiotic microbial assemblages on barnacle attachment. FEMS Microbiol. Ecol. 81, 583–595. doi: 10.1111/j.1574-6941.2012.01384.x
Oksanen, J., Blanchet, F. G., Kindt, R., Legendre, P., Minchin, P. R., O'hara, R. B., et al. (2015). Vegan: Community Ecology Package. R Package Version 2.4-0. Available online at: https://github.com/vegandevs/vegan
Oppermann, B. I., Michaelis, W., Blumenberg, M., Frerichs, J., Schulz, H. M., Schippers, A., et al. (2010). Soil microbial community changes as a result of long-term exposure to a natural CO2 vent. Geochim. Cosmochim. Acta 74, 2697–2716. doi: 10.1016/j.gca.2010.02.006
Pansch, A., Winde, V., Asmus, R., and Asmus, H. (2016). Tidal benthic mesocosms simulating future climate change scenarios in the field of marine ecology. Limnol. Oceanogr. doi: 10.1002/lom3.10086. [Epub ahead of print].
Pierrot, D., Lewis, E., and Wallace, D. (2006). MS Excel Program Developed for CO2 System Calculations. ORNL/CDIAC-105a. Oak Ridge, TN: Carbon Dioxide Information Analysis Center, Oak Ridge National Laboratory, US Department of Energy.
Pruesse, E., Quast, C., Knittel, K., Fuchs, B. M., Ludwig, W. G., Peplies, J., et al. (2007). SILVA: a comprehensive online resource for quality checked and aligned ribosomal RNA sequence data compatible with ARB. Nucleic Acids Res. 35, 7188–7196. doi: 10.1093/nar/gkm864
Raven, J., Caldeira, K., Elderfield, H., Hoegh-Guldberg, O., Liss, P., Riebesell, U., et al. (2005). Ocean Acidification Due to Increasing Atmospheric Carbon Dioxide. The Royal Society.
R Core Team (2015). R: A Language and Environment for Statistical Computing, Vienna. Available online at: URL http://www.R-project.org
Richardson, D. C., and Richardson, J. S. (1992). The kinemage: a tool for scientific communication. Protein Sci. 1, 3–9. doi: 10.1002/pro.5560010102
Rousvoal, S., Groisillier, A., Dittami, S. M., Michel, G., Boyen, C., and Tonon, T. (2011). Mannitol-1-phosphate dehydrogenase activity in Ectocarpus siliculosus, a key role for mannitol synthesis in brown algae. Planta 233, 261–273. doi: 10.1007/s00425-010-1295-6
Sarkar, D. (2008). Lattice: Multivariate Data Visualization with R. New York, NY: Springer Science & Business Media.
Sarker, M. Y., Bartsch, I., Olischläger, M., Gutow, L., and Wiencke, C. (2013). Combined effects of CO2, temperature, irradiance and time on the physiological performance of Chondrus crispus (Rhodophyta). Botan. Mar. 56, 63–74. doi: 10.1515/bot-2012-0143
Schloss, P. D., Westcott, S. L., Ryabin, T., Hall, J. R., Hartmann, M., Hollister, E. B., et al. (2009). Introducing mothur: open-source, platform-independent, community-supported software for describing and comparing microbial communities. Appl. Environ. Microbiol. 75, 7537–7541. doi: 10.1128/AEM.01541-09
Schories, D., Albrecht, A., and Lotze, H. (1997). Historical changes and inventory of macroalgae from Konigshafen Bay in the northern Wadden Sea. Helgolander Meeresuntersuchungen 51, 321–341. doi: 10.1007/BF02908718
Simpson, G. L. (2015). Permute: Functions for Generating Restricted Permutations of Data. R Package Version 0.8-4. Available onlne at: http://CRAN.R-project.org/package=permute
Singh, R. P., and Reddy, C. R. K. (2014). Seaweed-microbial interactions: key functions of seaweed-associated bacteria. FEMS Microbiol. Ecol. 88, 213–230. doi: 10.1111/1574-6941.12297
Steele, J. A., Countway, P. D., Xia, L., Vigil, P. D., Beman, J. M., Kim, D. Y., et al. (2011). Marine bacterial, archaeal and protistan association networks reveal ecological linkages. ISME J. 5, 1414–1425. doi: 10.1038/ismej.2011.24
Stewart, R. I. A., Dossena, M., Bohan, D. A., Jeppesen, E., Kordas, R. L., Ledger, M. E., et al. (2013). Mesocosm experiments as a tool for ecological climate-change research. Adv. Ecol. Res. 48, 71–181. doi: 10.1016/b978-0-12-417199-2.00002-1
Stratil, S. B., Neulinger, S. C., Knecht, H., Friedrichs, A. K., and Wahl, M. (2013). Temperature-driven shifts in the epibiotic bacterial community composition of the brown macroalga Fucus vesiculosus. Microbiologyopen 2, 338–349. doi: 10.1002/mbo3.79
Stratil, S. B., Neulinger, S. C., Knecht, H., Friedrichs, A. K., and Wahl, M. (2014). Salinity affects compositional traits of epibacterial communities on the brown macroalga Fucus vesiculosus. FEMS Microbiol. Ecol. 88, 272–279. doi: 10.1111/1574-6941.12292
Verardo, D. J., Froelich, P. N., and McIntyre, A. (1990). Determination of organic-carbon and nitrogen in marine-sediments using the Carlo-Erba-Na-1500 analyzer. Deep Sea Res. A Oceanogr. Res. Papers 37, 157–165. doi: 10.1016/0198-0149(90)90034-S
Vezzulli, L., Brettar, I., Pezzati, E., Reid, P. C., Colwell, R. R., Hofle, M. G., et al. (2012). Long-term effects of ocean warming on the prokaryotic community: evidence from the vibrios. ISME J. 6, 21–30. doi: 10.1038/ismej.2011.89
Vezzulli, L., Colwell, R. R., and Pruzzo, C. (2013). Ocean warming and spread of pathogenic vibrios in the aquatic environment. Microb. Ecol. 65, 817–825. doi: 10.1007/s00248-012-0163-2
Wahl, M., Buchholz, B., Winde, V., Golomb, D., Guy-Haim, T., Müller, J., et al. (2015). A mesocosm concept for the simulation of near-natural shallow underwater climates: the Kiel Outdoor Benthocosms (KOB). Limnol. Oceanogr. 13, 651–663. doi: 10.1002/lom3.10055
Wang, Q., Garrity, G. M., Tiedje, J. M., and Cole, J. R. (2007). Naive Bayesian classifier for rapid assignment of rRNA sequences into the new bacterial taxonomy. Appl. Environ. Microbiol. 73, 5261–5267. doi: 10.1128/AEM.00062-07
Weinberger, F. (2007). Pathogen-induced defense and innate immunity in macroalgae. Biol. Bull. 213, 290–302. doi: 10.2307/25066646
Wichard, T. (2015). Exploring bacteria-induced growth and morphogenesis in the green macroalga order Ulvales (Chlorophyta). Front. Plant Sci. 6:86. doi: 10.3389/fpls.2015.00086
Wiencke, C., Gomez, I., and Dunton, K. (2009). Phenology and seasonal physiological performance of polar seaweeds. Botan. Mar. 52, 585–592. doi: 10.1515/BOT.2009.078
Williams, H. N., and Piñeiro, S. (2007). “Ecology of the predatory bdellovibrio and like organisms,” in Predatory Prokaryotes: Biology, Ecology and Evolution, ed E. Jurkevitch. (Berlin; Heidelberg: Springer), 213–248.
Yamaguchi, T., Ikawa, T., and Nisizawa, K. (1966). Incorporation of radioactive carbon from H14CO3− into sugar constituents by a brown alga Eisenia bicyclis during photosynthesis and its fate in dark. Plant Cell Physiol. 7, 217–229.
Youssef, N. H., Couger, M. B., and Elshahed, M. S. (2010). Fine-scale bacterial beta diversity within a complex ecosystem (Zodletone Spring, OK, USA): the role of the rare biosphere. PLoS ONE 5:e12414. doi: 10.1371/journal.pone.0012414
Keywords: ocean acidification, pCO2, global warming, metaorganism, epibacteria, Fucus vesiculosus forma mytili, 16S rDNA, mesocosm
Citation: Mensch B, Neulinger SC, Graiff A, Pansch A, Künzel S, Fischer MA and Schmitz RA (2016) Restructuring of Epibacterial Communities on Fucus vesiculosus forma mytili in Response to Elevated pCO2 and Increased Temperature Levels Front. Microbiol. 7:434. doi: 10.3389/fmicb.2016.00434
Received: 01 December 2015; Accepted: 17 March 2016;
Published: 31 March 2016.
Edited by:
Olga Lage, University of Porto, PortugalCopyright © 2016 Mensch, Neulinger, Graiff, Pansch, Künzel, Fischer and Schmitz. This is an open-access article distributed under the terms of the Creative Commons Attribution License (CC BY). The use, distribution or reproduction in other forums is permitted, provided the original author(s) or licensor are credited and that the original publication in this journal is cited, in accordance with accepted academic practice. No use, distribution or reproduction is permitted which does not comply with these terms.
*Correspondence: Ruth A. Schmitz, cnNjaG1pdHpAaWZhbS51bmkta2llbC5kZQ==
†Present Address: Sven C. Neulinger, omics2view.consulting GbR, Kiel, Germany