- 1The Third School of Clinical Medicine, Guangzhou Medical University, Guangzhou, China
- 2Shenzhen Eye Institute, Shenzhen Eye Hospital, Jinan University, Shenzhen, China
Background: The application of Artificial Intelligence (AI) in diagnosing retinal diseases represents a significant advancement in ophthalmological research, with the potential to reshape future practices in the field. This study explores the extensive applications and emerging research frontiers of AI in retinal diseases.
Objective: This study aims to uncover the developments and predict future directions of AI research in retinal disease over the past decade.
Methods: This study analyzes AI utilization in retinal disease research through articles, using citation data sourced from the Web of Science (WOS) Core Collection database, covering the period from January 1, 2014, to December 31, 2023. A combination of WOS analyzer, CiteSpace 6.2 R4, and VOSviewer 1.6.19 was used for a bibliometric analysis focusing on citation frequency, collaborations, and keyword trends from an expert perspective.
Results: A total of 2,861 articles across 93 countries or regions were cataloged, with notable growth in article numbers since 2017. China leads with 926 articles, constituting 32% of the total. The United States has the highest h-index at 66, while England has the most significant network centrality at 0.24. Notably, the University of London is the leading institution with 99 articles and shares the highest h-index (25) with University College London. The National University of Singapore stands out for its central role with a score of 0.16. Research primarily spans ophthalmology and computer science, with “network,” “transfer learning,” and “convolutional neural networks” being prominent burst keywords from 2021 to 2023.
Conclusion: China leads globally in article counts, while the United States has a significant research impact. The University of London and University College London have made significant contributions to the literature. Diabetic retinopathy is the retinal disease with the highest volume of research. AI applications have focused on developing algorithms for diagnosing retinal diseases and investigating abnormal physiological features of the eye. Future research should pivot toward more advanced diagnostic systems for ophthalmic diseases.
1 Introduction
Artificial Intelligence (AI), first introduced in 1956 (1), signifies machines undertaking tasks traditionally aligned with human intelligence. With its ability to process complex data, AI has become a transformative technology in healthcare, offering new solutions for early disease detection and personalized treatment. With AI encompassing Machine Learning (ML) and Deep Learning (DL), the latter advances by employing multilayer artificial neural networks to decode complex patterns from extensive data sets, facilitating the management of intricate diseases (2, 3). By 2015, DL models (4–6) had achieved human-level accuracy in image recognition tasks, underscoring AI’s potential to rival human capability in medicine and science. This prowess, particularly in tasks like image classification through ML algorithms (7–9), has garnered considerable focus. The evolution of digital imaging, image processing, and computer vision notably enhances the role of ML in autonomously identifying retinal maladies from color fundus photographs. Transfer learning (10, 11), as evidenced by prior studies, emerges as a potent strategy, especially under data scarcity, to mitigate overfitting and heighten model precision. Deep transfer learning, sidestepping the need for extensive manual annotations or a vast corpus of labeled training data, presents a more economical and efficient alternative to traditional image recognition methods (12).
Retinal diseases, significant contributors to severe vision impairment, encompass a spectrum from retinopathy to chorioretinopathy (13), with risk factors including age (14), myopia (15), diabetes (16), trauma (17), retinal vascular occlusion (18), hypertension (19), retinitis (20), and genetic disposition (21). The cruciality of early screening and diagnosis stands in preventing irreversible vision loss against the backdrop of widespread blindness and low vision issues amplified by the deficit of medical examination tools (22–24), specialist ophthalmologists (25–27), and effective interventions (28, 29), particularly in under-resourced areas (30). As AI-driven tools gain traction in retinal diagnostics, they support healthcare providers in regions with limited resources by enabling timely detection and intervention.
Recent endeavors have seen researchers leveraging ML and DL for AI-assisted diagnostic systems based on retinal imagery like color fundus photography or Optical Coherence Tomography (OCT), aiming at retinal disease screening. These innovations, rivaling ophthalmologist diagnostics, primarily target conditions such as Diabetic Macular Edema (DME) (31–33), Age-Related Macular Degeneration (AMD) (14, 34, 35), and even non-retinal diseases like glaucoma (36–38) through retinal imaging, despite fewer studies delving into comprehensive disease spectra (39, 40) or delineating normal from abnormal fundus images in AI-supported diagnosis (12, 41).
Our research, using bibliometric tools like CiteSpace, VOSviewer, and bibliometric.com, explores AI’s global impact on retinal disease management. This study aims to elucidate trends, geographical distribution, institutional contributions, and the emerging research focus areas within AI-driven retinal diagnostics. By applying bibliometric and manual screening, this research offers insights into the most influential studies and regions, advancing the understanding of AI’s current and future role in retinal disease management. The results from this analysis aim to guide AI and ophthalmology professionals, marking a significant leap toward integrating AI in retinal disease detection and management.
2 Methods
2.1 Selection of published data
On January 23, 2024, we retrieved citation data published between January 1, 2014, and December 31, 2023, from the Web of Science Core Collection (WoSCC) database. The data retrieval and verification were independently conducted by two authors to ensure accuracy and consistency.
The search formula used in the literature search was:
Topic = (“Artificial Intelligence” or “AI” or “transfer learning” or “neural network” or “Deep Learning” or “Robotic*” or “Supervised Learning” or “Unsupervised Learning” or “Computer Vision System” or “Computational Intelligence” or “Machine Learning” or “Evolutionary Computation” or “Ensemble Learning” or “Reinforcement Learning”) AND Topic = (“Retin*” or “Epiretinal” or “macular” or “Epiretinal Membrane”).
Only articles and review articles in English were included, while early access papers, conference proceedings, book chapters, data papers, and retracted articles were excluded. To refine the results, we manually screened each article title and abstract for relevance, with specific criteria for exclusion:
1. Studies that did not focus on eye diseases.
2. Studies that did not employ AI-based methods.
This approach allowed us to retain only those records directly relevant to AI applications in retinal diseases, enhancing the study’s specificity and accuracy.
For each selected article, we extracted and analyzed essential bibliographic information, including title, publication year, country/region, institution, journal, and keywords. Based on this data, we conducted a thorough bibliometric analysis to explore global research hotspots in AI applications for retinal disease. A detailed search and data processing workflow is illustrated in Figure 1.
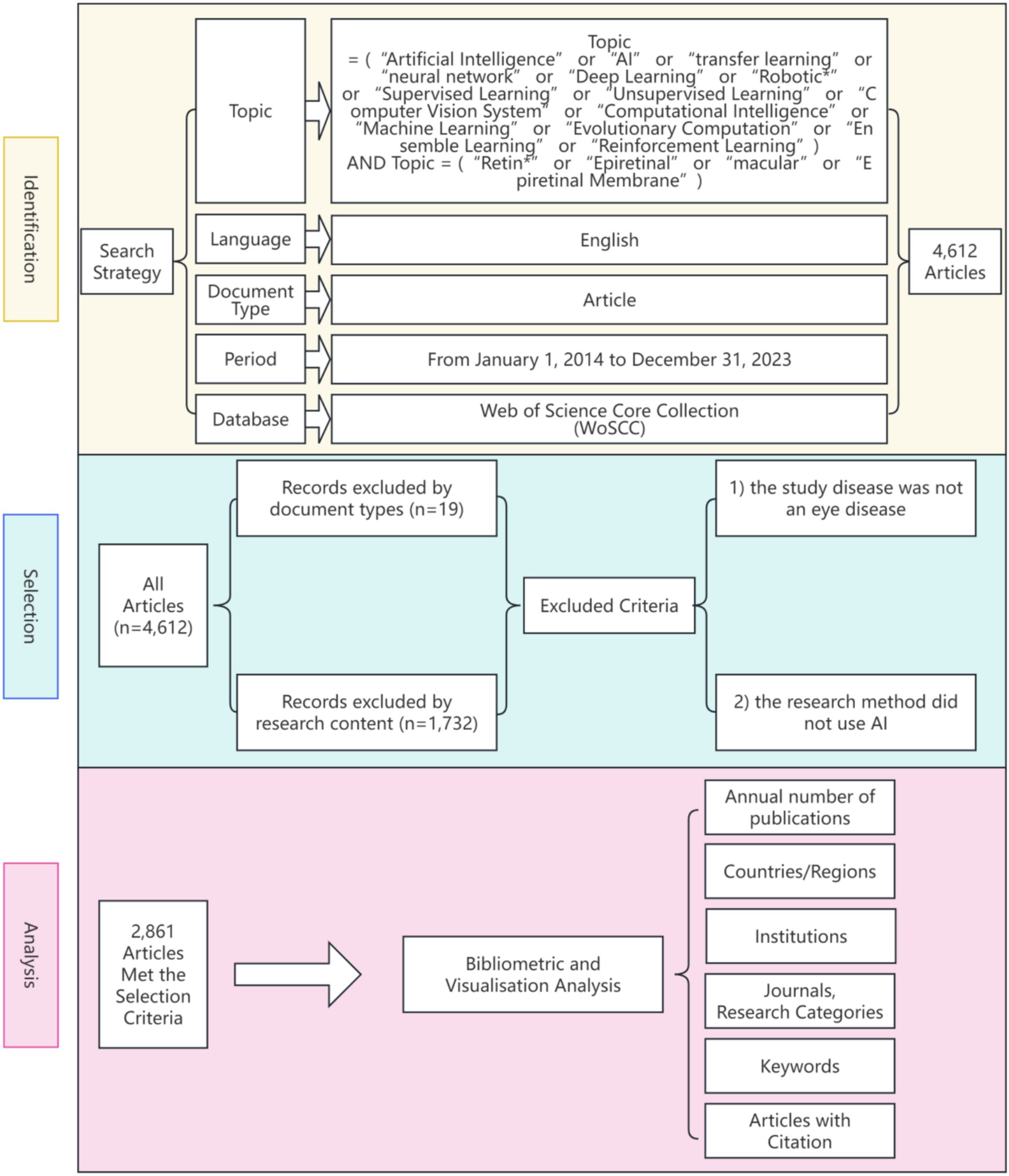
Figure 1. Frame flow diagram showing the detailed selection criteria and bibliometric analysis steps.
2.2 Research and analysis methods
CiteSpace 6.2 was used to perform a cluster analysis of countries or regions, organizations, journals, research categories, and keywords. Centrality was produced using CiteSpace. It represents the degree of cooperation between different regions or institutions. WoSCC can calculate the h-index of published literature. The h-index is defined as the maximum value of h such that the given author/journal has published at least h articles that have each been cited at least h times, which is a new method for evaluating academic achievements (42).
In addition to the functions provided by CiteSpace, we employed WOC Citation Topic Micro to identify and quantify AI applications across various retinal diseases. By counting keyword frequencies in the collected literature, CiteSpace and WOC Citation Topic Micro together facilitated the identification of major research focuses within the field of AI-assisted retinal diagnostics.
Using the bibliometric platform https://bibliometric.com/app, we classified the countries and institutions represented in the literature, generating comprehensive statistics on research distribution. VOSviewer generates heat maps based on keywords, which represent research hotspots. We further conducted an in-depth interpretation and comprehensive analysis of the included articles, especially the high-impact articles.
3 Results
3.1 Distribution of articles by publication year
We carried out an analysis of a total of 2,861 articles published from 2014 to 2023 on the subject of AI in retinal diseases. Employing the citation analyzing features of the WoSCC database, we computed the annual number of citations, with CiteSpace’s deduplication function ensuring the accuracy of these figures. As depicted in Figure 2, the publication frequency was modest before 2017, not exceeding 100 articles per year, and the annual article count’s trend line had a slope of 12.8, indicative of an average annual growth of about 12 articles. Post-2017, the yearly output surpassed 100 articles, showing a marked acceleration in recent years. The trend line’s slope steepened to 120.1, signaling an annual increase that averaged around 120 articles.
3.2 Countries or regions
The citation analyzer of the WoSCC database calculates the article output of various countries or regions, while CiteSpace facilitates the analysis of interdependencies among these entities. A total of 93 countries or regions are represented in the data. Figure 3 visually depicts the article output of each country and region, along with their collaborative engagements. The size of the labels within the circle reflects the number of articles from each entity, with China (926), the United States (637), and India (464) having the most significant shares. The interconnected lines between country labels in Figure 3 symbolize the collaborative efforts, where the density of these lines indicates the extent of literature exchange among countries.
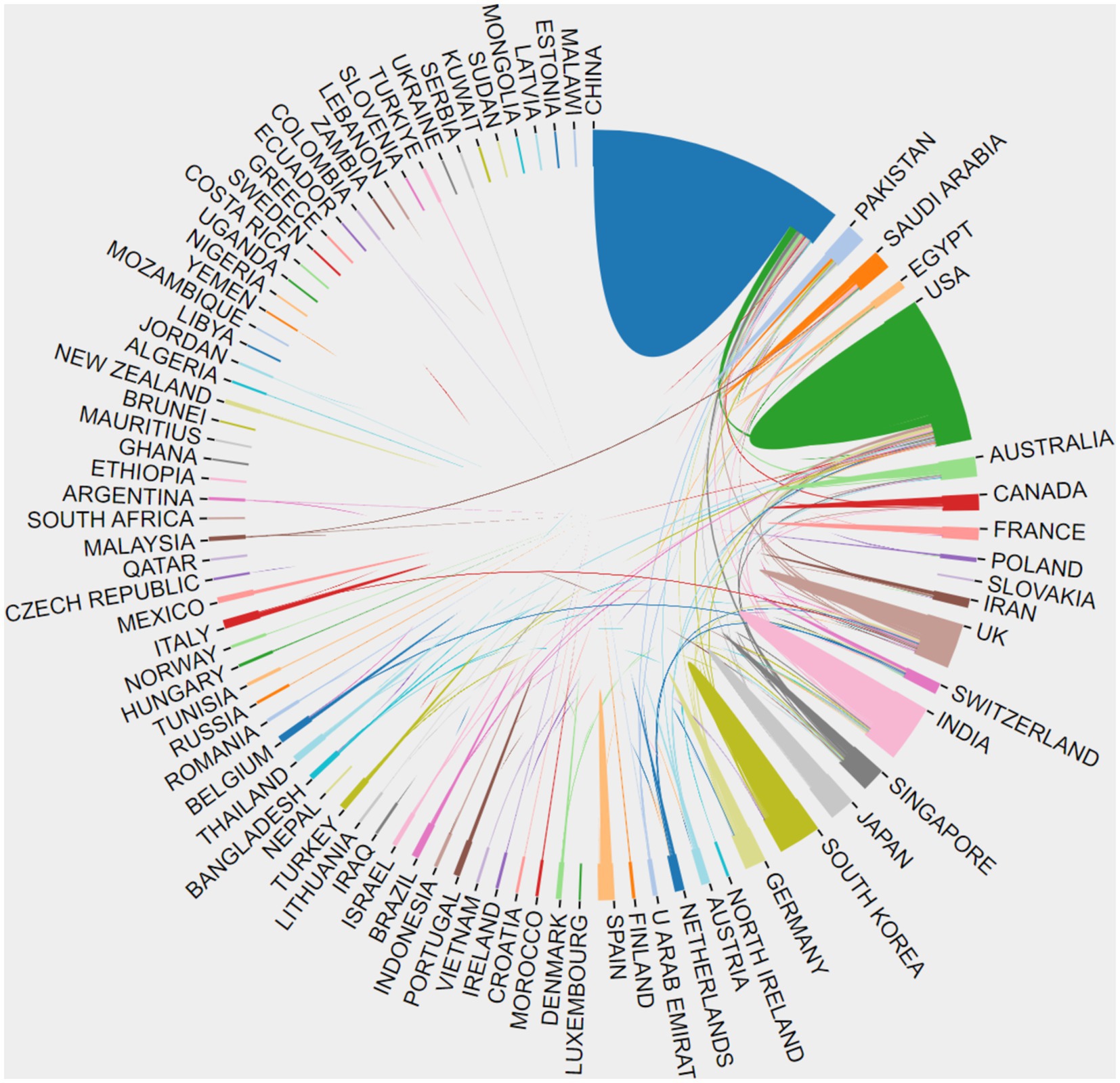
Figure 3. The cooperation of countries or regions that contributed to articles on AI in retinal diseases from 2014 to 2023.
Further analysis reveals trends over the past decade regarding the article counts from the top five contributing countries or regions, as illustrated in Figure 4. Concurrently, Table 1 details the annual article counts on AI applications in retinal disease for these leading contributors.
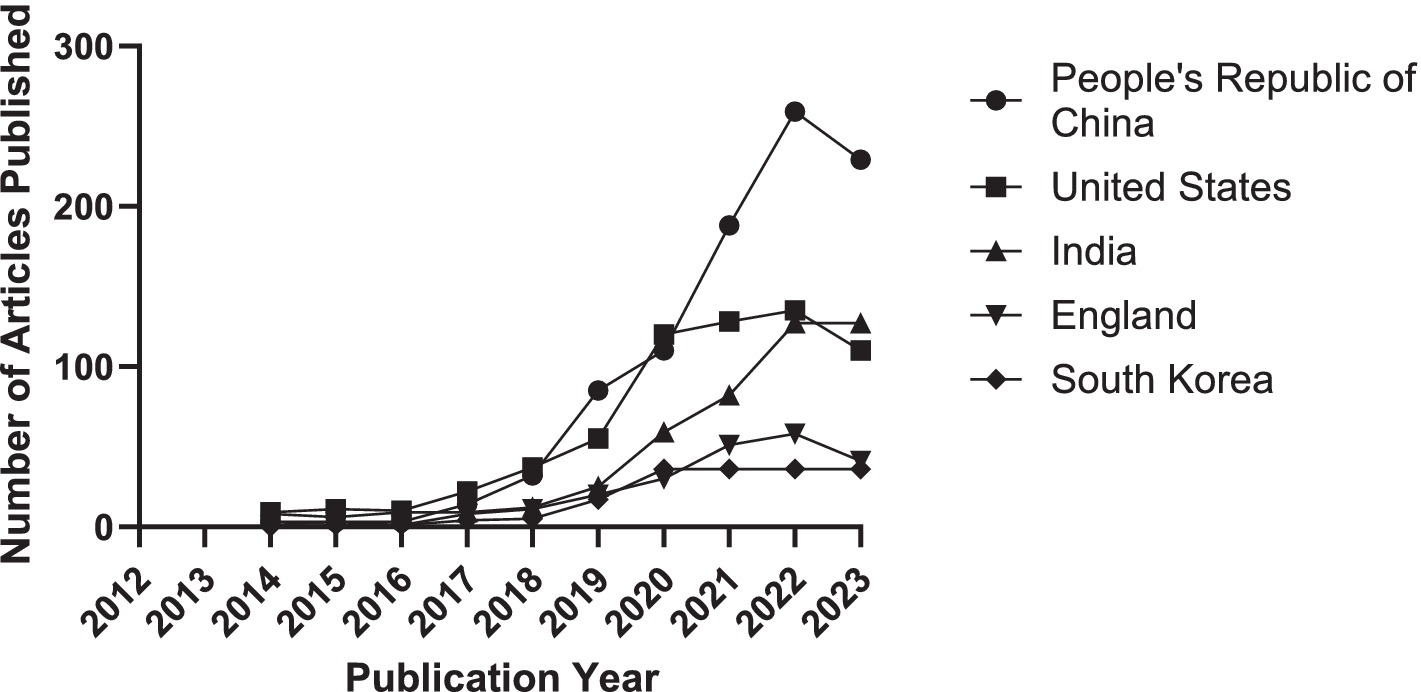
Figure 4. Trends in the number of articles contributed by the top 5 countries or regions from 2014 to 2023.
The visual representation in Figure 5, through varying label sizes and green node areas, highlights the number of articles from each country or region, with connection lines delineating their cooperative relationships. The centrality measure in Table 2, represented by the purple circle’s size, assesses the influence of articles from each country, with England’s purple circle (0.24) being notably the largest, indicating its pivotal role in international collaborations.
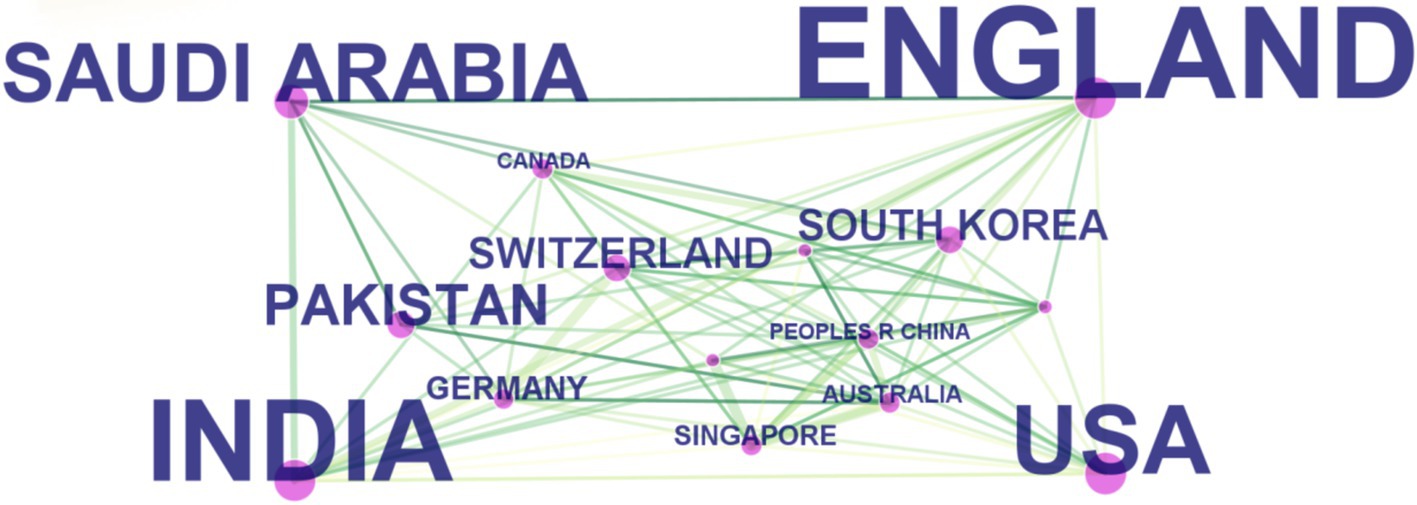
Figure 5. The cooperation of countries or regions that contributed to articles on applying artificial intelligence to the study of retinal diseases from 2014 to 2023.
These findings are grounded in data displayed in Table 2, which also showcases the h-index, an indicator blending academic quality and impact. The United States boasts the highest h-index (66), denoting its leading influence. Summarily, from 2014 to 2023, China emerged as the most prolific publisher, England as the most collaborative, and the United States as having the most substantial influence in AI applications within retinal disease research.
3.3 Institutions
The top 10 institutions with the most articles published are shown in Table 3. The data shown are the results of the CiteSpace software default settings. A total of 291 institutions were counted, constituting 1,375 partnerships. These include three institutions in the United Kingdom, three in China, and one in the United States, Austria, Egypt, and Singapore. Three of the top 5 h-index institutions are from the United Kingdom, and the other two are from China. The links between the labels in Figure 6 show the collaboration between agencies. The node size represents the number of articles sent. According to the centrality, the influence of the National University of Singapore was relatively high (0.13).
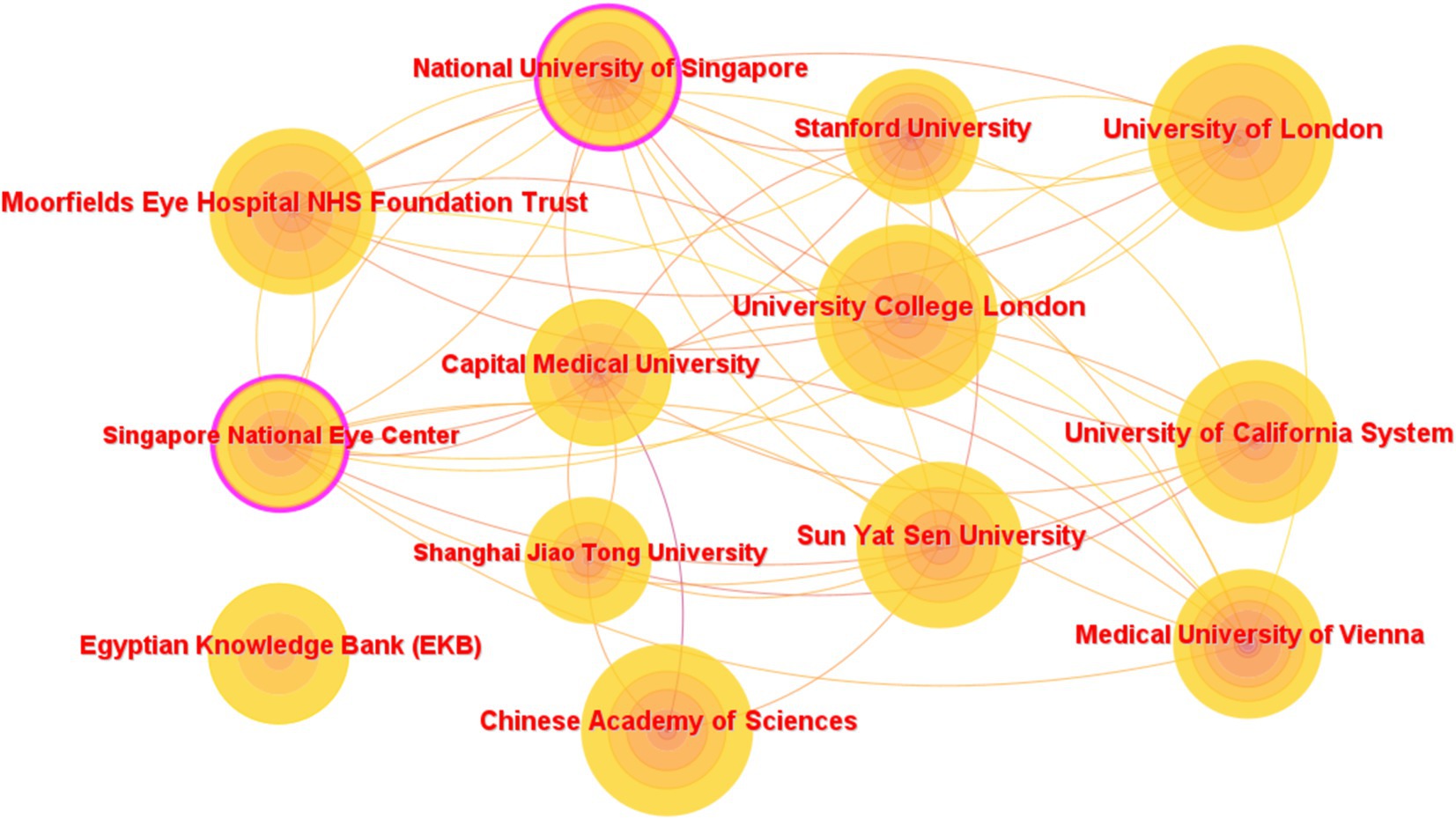
Figure 6. Network map of institutions that contributed to articles on AI in retinal diseases from 2014 to 2023.
3.4 Journals and research categories
The knowledge foundation for the cited articles is the literature found in the cited articles. The research field of highly cited journals is a hotspot of attention. In the past 10 years, the knowledge-based research fields of artificial intelligence applied in ophthalmic diseases have included systems/computing/molecular/biology/genetics/health/nursing/medicine/ophthalmology, which constitute mathematics/systems/mathematics/neurology/hot topics at the forefront of sports/ophthalmology research. Table 4 lists the subject categories of the top 10 cited journals/proceedings. The most common research fields for citing journals include engineering technology/computing. The discipline most involved in the extracted version was classified as medicine/ophthalmology.
3.5 Keywords
According to the keyword co-occurrence and cooperation network analysis chart, we analyzed new keywords used over the past 10 years to understand co-occurrence and cooperation. We modified the default settings of CiteSpace to “year per slice” = 1, “Top N%” = 10.0%, “Top N” = 30, and “minimum duration” = 2. The results are shown in Figure 7. Red indicates the emergence of keywords. The time trend was examined using the hotspot transfer method, which was applied to the first 15 keywords with the highest citation outbreak. From 2014 to 2023, the keywords that were consistently used included segmentation (2014–2018), classification (2014–2017), diagnosis (2014–2016), machine learning (2014–2018), automated detection (2014–2017), blood vessels (2014–2018), images (2014–2017), retinal images (2015–2019), extraction (2018–2020), nerve fiber layer (2019–2021), risk factors (2020–2021), eyes (2020–2021), OCT (2021–2023), attention mechanism (2021–2023), outcome (2021–2023).
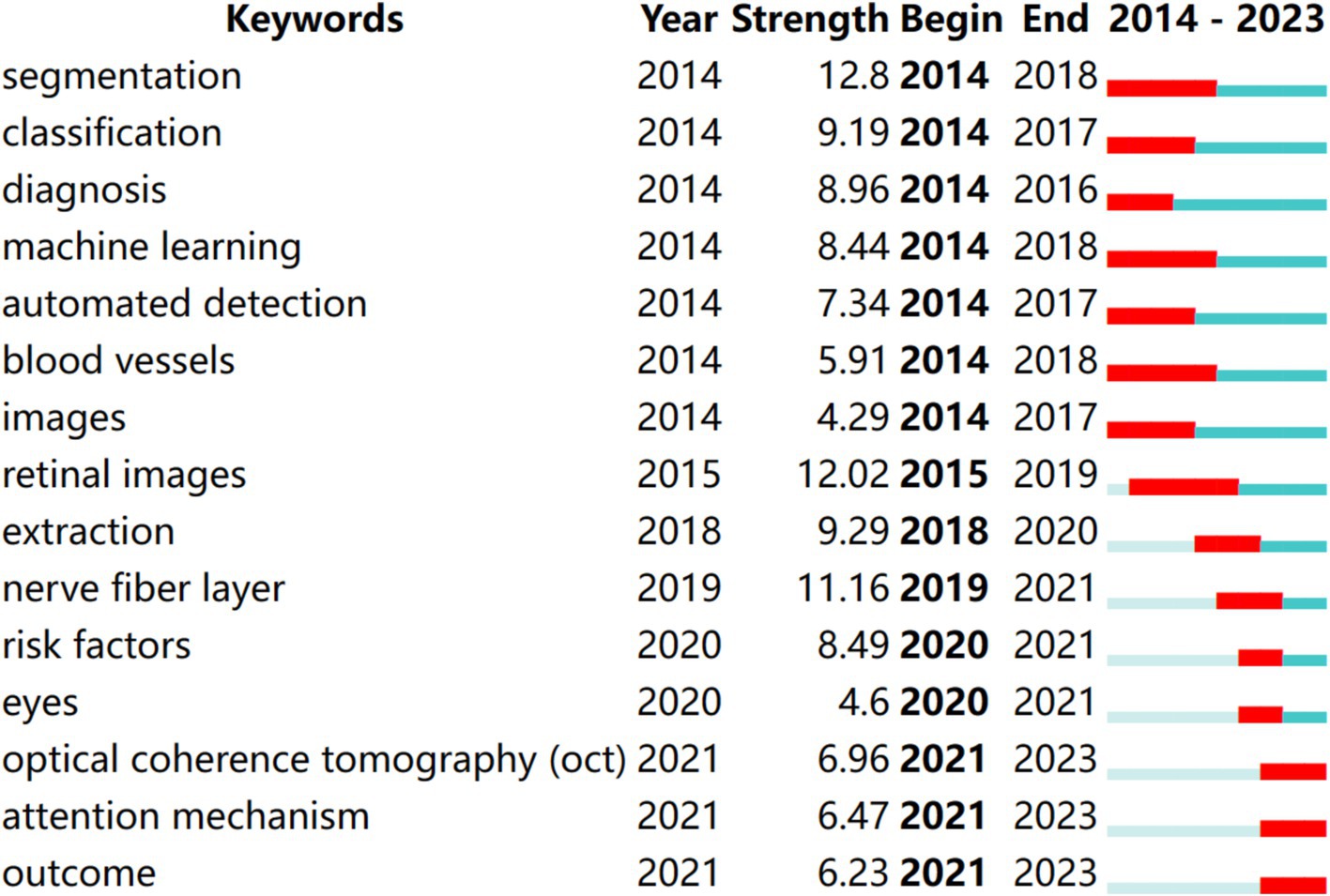
Figure 7. Top 10 keywords with the strongest citation bursts of articles on the application of artificial intelligence in retinal diseases from 2014 to 2023.
These keywords highlight critical areas of research within AI applications in ophthalmology, reflecting advancements in diagnostic capabilities and methodologies. For instance, terms like “segmentation” and “classification” are foundational to developing accurate AI models for analyzing retinal images, while “attention mechanism” signifies a shift toward more sophisticated approaches that enhance model performance in identifying relevant features in complex datasets.
3.6 High-impact articles
The research includes the 10 most cited articles on AI in retinal diseases from 2014 to 2023, as listed in Table 5. These articles indicate that AI research in retinopathy holds promise, yet they also highlight certain limitations in clinical implementation.
3.7 Top 5 retinal diseases researches using AI
We manually screened the keywords from CiteSpace statistics and screened out the keywords for retinal diseases. Furthermore, we refined the search results on using AI in retinal disease on a more granular level using WOS analysis Citation Topics Micro and selected from over 2,500 available micro-level citation topics based on the search results. The “Citation Topic Micro” feature in (WoS) allows researchers to analyze and explore citation patterns at a granular level within a specific topic or field of study. It helps identify the most influential literature, authors, or institutions within a given research area based on their citation impact. This feature facilitates in-depth bibliometric analysis and aids in assessing the influence and significance of scholarly articles within a specific research domain.
The data from the two databases above were statistically analyzed (Table 6). Among the statistical keywords, DR was the most studied disease, followed by Glaucoma. These retinal diseases have mainly been studied and applied to AI.
4 Discussion
4.1 Principal results
From 2014 to 2017, the article counts on the application of AI in retinal diseases showed modest growth. However, a pivotal shift occurred post-2017, sparked by a groundbreaking study by Gulshan et al. from Google Health (43), which demonstrated an automated algorithm capable of accurately interpreting retinal images. This development, characterized by high accuracy and the algorithm’s impressive sensitivity (99% [87.0–97.5%]) and specificity (90.3–98.1%) in diabetic retinopathy screening, fueled advancements in deep learning algorithms for diagnosing and treating retinopathy across diverse populations.
The distribution of publications reveals distinct trends in both output and impact across different countries. China leads in volume, contributing approximately 32% of the global articles. Conversely, the United States boasts the highest h-index at 66, indicating the significant impact of its contributions, followed closely by China with a score of 62. England, with the highest centrality score (0.24) and India following closely (0.22), highlights the importance of international collaborations that strengthen research quality and scope. Notably, joint publications between the United States and China represent 55% of the total output, underscoring the high caliber of collaborative work. Despite this, China’s relatively lower level of international collaboration, especially compared to the active partnerships among the United States, England, and India, suggests an opportunity for China to engage more in transnational research efforts, which could further enhance the global impact of its research.
The global academic landscape features prominent contributions from the UK and China, with these nations housing the majority of the top 10 institutions in this field. China’s recent surge in publications aligns with recent science and technology policy advancements, though its development timeline indicates the need for further consolidation of foundational knowledge. In contrast, the United States’ robust AI ecosystem, supported by research experts and technology firms, plays a crucial role in advancing AI research and applications. This disparity highlights the need for China to enhance its technology development and for the United States to foster greater academic collaborations.
Within Singapore, the National University of Singapore has emerged as a key player in scientific research, demonstrating strong collaborative engagements. On the other hand, the University of London emerges with a broader impact evidenced by its published literature. Alongside, two other UK-based institutions also boast high h-index values. Despite their varied publication frequency and centrality, these institutions collectively mark a significant advancement in academic pursuit, reflecting growth in scholarly output and consistent dissemination of research articles. Analysis of the cooperation network shows that research institutions in this specialty generally have low intermediary centrality, suggesting the need to bolster their influence in the realm. Enhanced collaboration among these institutions is paramount, as insufficient networking can hinder the progression of the domain, potentially impeding academic research.
In the context of AI applications in ophthalmology, an integrative approach combining foundational ophthalmic disease knowledge with innovative computer science methodologies is essential. In Table 6, the top five keywords of retinal disease indicate that DR is the most commonly used AI in fundus diseases, followed by Glaucoma, AMD, and other retinal diseases. These focus areas underscore the importance of AI-driven diagnostic tools for addressing the global burden of retinal diseases and advancing early detection and intervention strategies.
4.2 Research hotspots and emerging trends
The current research frontiers in AI application within retinal disease spotlight three main areas: “OCT,” “Attention Mechanism,” and “Outcome.” These fields have been increasingly active from 2021 to 2023 as per the clustering timeline derived from Figure 5, underscoring evolving research directions and technologies.
4.2.1 Optical coherence tomography
Occupying a pivotal role in ophthalmology, OCT is a non-invasive technique that generates high-resolution, cross-sectional imagery of the retina, optic nerve, and anterior eye segment. It’s essential for the detailed analysis and identification of ocular pathologies through OCT fundus images. OCT’s capability to illuminate microstructural details in the eye facilitates the early identification, diagnosis, and tracking of a variety of eye diseases including age-related macular degeneration (44, 45), diabetic retinopathy (46), glaucoma (47), among others (48).
4.2.1.1 Advancements in AI-enhanced OCT for precise retinal analysis
AI integration with OCT has revolutionized the detection and analysis of retinal structures. OCT uses interference patterns to provide detailed images crucial for identifying eye diseases. AI notably enhances segmentation precision, aiding the delineation of ophthalmic microstructures. For instance, He et al. (49) achieved a Dice score of 91.3% and a mean Intersection over Union (mIoU) of 84.4% for retinal layer segmentation. Other studies, such as those by Lu et al. (50) and Guo et al. (51), have demonstrated significant advancements in efficient segmentation with minimal labeled data and automated segmentation of specific retinal features. Overall, AI’s integration with OCT improves fundus lesion detection capabilities. Zhang et al. (52) introduced X-Net, a weakly supervised DL framework for segmenting paracentral acute middle maculopathy lesions in spectral-domain OCT (SD-OCT) images, achieving 99% accuracy and a mIoU of 0.8. Subsequently, Zhang et al. (53) developed RC-Net for segmenting hyperreflective dots in retinal OCT images, essential for DR diagnosis, with a mean Dice similarity coefficient of 75.29% ± 0.42%, an IoU of 62.27%, recall of 78.36%, and precision of 75.34%. Lastly, Loo et al.’s (54) DL algorithm for classifying and segmenting retinal cavitations in macular telangiectasia type 2 via OCT images reported a sensitivity of 0.94, a specificity of 0.80, and an average Dice similarity coefficient of 0.94. Overall, AI’s integration with OCT improves fundus lesion detection capabilities.
4.2.1.2 Advancements in AI-assisted OCT for detection of retinal pathologies
Advanced deep learning algorithms enhance AI’s ability to analyze OCT imagery, significantly improving the identification of subtle retinal and optic nerve abnormalities. The integration of AI with OCT diagnostic processes boosts efficiency and promotes timely intervention, significantly improving patient outcomes.
4.2.1.3 Advancements in AI-assisted OCT for multiple retinal diseases detection
Prevailing AI applications in retinal disease diagnosis have evolved from targeting single conditions to addressing multiple retinal diseases concurrently within the same patient. This shift is facilitated by deep learning algorithms that benefit from larger and better-quality datasets, feature fusion advancements, and holistic training techniques. This new wave of multi-condition AI detection models has quickly gained traction in academic research. A prime example is Sunija et al. (55), who introduced OctNET, a lightweight CNN for classifying retinal diseases via OCT images, achieving precision, recall, and accuracy rates of 99.69%. Togacar et al. (56) proposed a novel approach combining CNN-derived dominant activations with the slime mold algorithm, resulting in high classification accuracies of 99.60, 99.89, and 97.49% across three distinct datasets. Similarly, Upadhyay et al. (57) presented a CNN framework for four-class retinal disease classification, incorporating batch normalization across five layers to attain a 97.19% accuracy rate. Kayadibi et al.’s (58) Retinal Fine Tuned Convolutional Neural Network (R-FTCNN) demonstrated perfect metrics in one dataset in the Duke dataset and exceptional results in the UCSD dataset, with an accuracy of 99.70%, a sensitivity of 99.70%, and a specificity of 99.90%.
The Hercules model integrates attention mechanisms and uncertainty quantification to enhance classification accuracy, achieving 94.21% in retinal OCT evaluations (59).
Moreover, AI’s potential to revolutionize diagnostic methods in universal healthcare systems, especially in community settings, is increasingly recognized. Bai et al. (60) assessed AI-enabled OCT’s precision in identifying 15 retinal disorders in community environments, demonstrating performance comparable to retina specialists and surpassing both senior and junior ophthalmologists. This suggests AI’s capability to improve accessibility and quality in community-based ophthalmic healthcare.
4.2.1.4 Advancements in AI-assisted OCT for DR
The research community is keenly focused on utilizing AI with OCT to explore DR-related retinopathy, with particular attention to microangiopathy. OCT’s capability in detecting subtle microvascular alterations serves as a cornerstone for early DR detection. Deep learning models have substantively aided clinicians in identifying early-stage DR using OCT. Hua et al. (61) introduced TFA-Net, a DL framework for assessing DR severity through fundus images and wide-field swept-source OCT angiography (SS-OCTA), achieving a Quadratic Weighted Kappa (QWK) of 90.2% in the KHUMC dataset and a mean accuracy of 94.8% with an AUC of 99.4% in the Messidor dataset.
OCT is also vital for detecting and assessing diabetic macular edema (DME), enabling high-resolution imaging that identifies abnormal retinal thickening and specific morphological changes associated with DME. DME is categorized as central involvement (CI-DME) or non-central involvement (NCI-DME) (62). Tang et al. (63) developed DeepDR, a DL framework for diagnosing and staging DME, which achieved AUROC scores of 0.937, 0.958, and 0.937 on the CIRRUS, SPECTRALIS, and Triton OCT datasets, respectively, and 0.965 on an external dataset. In differentiating between CI-DME and NCI-DME, AUROC results were 0.968, 0.951, and 0.975 for the primary dataset, exceeding 0.894 for the external dataset, highlighting DeepDR’s effectiveness in DME assessment.
Additionally, DL techniques enhance OCT’s capability to reveal characteristic changes indicative of DME severity, treatment response, and prognosis, making it a valuable imaging biomarker (64, 65). A study on the automated quantification of central macular fluid volume (CMFV) via OCT involved 215 patients, finding an AUROC of 0.907 for identifying center-involved DME. With a specificity of 95%, CMFV demonstrated a sensitivity of 78.5% for detecting center-involved DME, confirming its role as a diagnostic biomarker (66). Further research has underscored a significant correlation between the extent of the area of avascularity (EAA) measured via OCTA and DME severity. Notably, EAA’s measurements showed no significant correlation with factors like signal strength index and shadow area, affirming its reliability and independence as a biomarker for DME (67).
4.2.1.5 Advancements in AI-assisted OCT for AMD diagnosis and segmentation
Age-related macular degeneration (AMD) has a long developmental cycle and variable symptom progression, necessitating AI algorithms that effectively address disease classification and staging. Accurate training methods are crucial for these applications, as OCT image changes are indicative of disease progression. Thomas et al. (68) introduced a pioneering deep CNN architecture tailored for the early detection of AMD from OCT images, showcasing high accuracy with scores ranging from 0.9666 to 0.9978 across multiple datasets. Moradi et al. (69) explored deep ensemble learning for automated classification of non-advanced AMD, utilizing optimized retinal layer segmentation in spectral-domain OCT (SD-OCT) scans, resulting in an AUC of 99.4% for distinguishing normal eyes from early AMD cases. Sotoudeh-Paima et al. (70) developed a multi-scale CNN for automated AMD classification, implementing gradual learning stages that enhanced accuracy from 87.2 to 93.4%. Liefers et al. (71) developed and validated a deep learning (DL) model for identifying features of neovascular and atrophic AMD in OCT images, achieving a mean Dice score of 0.63 for 11 of 13 features and an intraclass correlation coefficient of 0.66, both comparable to human observers.
4.2.1.6 Advancements in AI-assisted OCT for diagnosis and grading of glaucoma
As can be seen from above, advancements have been achieved in DL methods for assessing changes in cup-to-disk ratio (C/D ratio) and retinal nerve fiber layer (RNFL) thickness. Over recent years, substantial progress has been made in leveraging DL for assisted diagnosis and grading of glaucoma using OCT imaging.
For glaucoma detection, Metha et al. (72) developed a multimodal DL model that combines macular OCT volumes, color fundus photographs, and clinical data for glaucoma detection, achieving an AUC of 0.97 with data from the UK Biobank. Sun et al. (73) introduced a dual-input CNN that analyzes RNFL and ganglion cell-inner plexiform layer (GCIPL) images, demonstrating strong diagnostic performance with an accuracy of 92.793% and an AUC of 0.957. Panda et al. (74) investigated the diagnostic utility of the central retinal vessel trunk and branches’ 3D structure for glaucoma using OCT, employing a DL network for segmentation, achieving a Dice coefficient of 0.81. Utilizing 3D and 3D-to-2D CNN methodologies, they discerned glaucoma from non-glaucoma cases with accuracies of 82.7 and 83.3%, and AUCs of 0.89 and 0.90, respectively. In a study analyzing 130 eyes from healthy subjects and 275 eyes from individuals diagnosed with glaucoma, CNN was utilized to evaluate vessel density and RNFL thickness images, achieving an Area Under the Precision-Recall Curve (AUPRC) of 0.97 (75). Beyond traditional methods, He et al. (76) presented a modality-specific attention network that integrates fundus and OCT images for retinal image classification, highlighting the advantage of multi-modal data integration. For glaucoma grading, Garcia et al. (77) proposed a hybrid network combining hand-crafted features with DL algorithms for analyzing circumpapillary B-scans. This approach, utilizing prototypical networks for few-shot learning, achieved categorical accuracies up to 0.9459.
In addition, AI has the capability to synthesize OCT images to aid in training AI systems for glaucoma diagnosis. Sreejith Kumar et al. (78) evaluated the use of Generative Adversarial Networks (GANs) to train DL networks on synthetic images, achieving an AUC of 0.97 on the internal dataset and 0.90 on the external dataset. These results demonstrate the efficacy of generative models in DL training and the potential for data sharing across institutions.
4.2.2 Attention mechanism
The attention mechanism is a technique in DL that enhances model’s focus on specific input data, thereby optimizing its performance. The articles with the keyword “attention mechanism” show its widespread application in medical image analysis, especially in DR classification. In retinal diseases, attention mechanisms are crucial for lesion detection, diagnosis, and classification. Here are some key points and research directions related to attention mechanisms in the context of the included articles.
4.2.2.1 Retinal structure segmentation based on CFP
In the realm of fundus image segmentation within the domain of AI, the attention mechanism technology integrated within CNNs emerges as a pivotal advancement, directing the model’s focus toward pertinent regions within retinal images. This sophisticated technique has garnered considerable attention and application, particularly in the segmentation of fundamental anatomical features such as blood vessels and optic disks, yielding commendable outcomes.
In vascular segmentation, this method has achieved remarkable achievements. Notable techniques include the AACA-MLA-D-UNet, employing a multi-level attention mechanism and dropout dense blocks for enhanced segmentation accuracy, showing superior performance on DRIVE, STARE, and CHASE_DB1 datasets with reduced complexity (79). Li et al. (80) improved U-Net-based retinal vessel segmentation by incorporating attention mechanisms, yielding better results across multiple datasets. BSEResU-Net utilizes Before-activation Squeeze-and-Excitation blocks (BSE Blocks) with attention mechanisms and drops block regularization to improve performance and generalization. Experimental results on DRIVE, STARE, and HRF datasets with F1-scores of 0.8324, 0.8368, and 0.8237, respectively (81). MSCNN-AM, a multi-scale CNN incorporating attention mechanisms for retinal vessel segmentation, demonstrated notable efficacy, with sensitivities of 0.8342/0.8412/0.8132 and accuracies of 0.9555/0.9658/0.9644 on DRIVE, STARE, and CHASE_DB1, respectively (82). A novel retinal vessel segmentation algorithm, integrating multi-scale attention D-MNet with an enhanced PCNN model, was validated across four databases (DRIVE, STARE, CHASE_DB1, HRF), achieving detection accuracies of 96.83, 97.32, 97.14, and 96.68%, respectively (83). LEA U-Net, a DL framework for retinal vessel segmentation has an evaluation on the DRIVE dataset achieving an accuracy of 0.9563, F1-score of 0.823, TPR of 0.7983, and TNR of 0.9793. The AUC of PRC is 0.9109 and the AUC of ROC is 0.9794 (84).
Besides, the utilization of attention mechanism technology within CNNs has demonstrated remarkable efficacy in segmenting critical ocular structures such as the optic disk and cup, pivotal in glaucoma screening protocols. Guo et al. (85) established FAU-Net, enhancing U-Net with feature fusion and channel-spatial attention, showing superior performance across multiple datasets. RSAP-Net introduces a U-shaped network with a Residual Spatial Attention Path for optic disk (OD) and optic cup (OC) segmentation, achieving F1 scores of 0.9752 (OD) and 0.9012 (OC), and boundary localization errors of 6.33 pixels (OD) and 11.97 pixels (OC) on Drishti-GS1 (86). Wang et al. (87) created a hierarchical CNN with a cascaded two-stage architecture for OD and OC segmentation in fundus images, utilizing an attention mechanism and focal loss for accurate OD identification, followed by multi-task and adversarial learning for OD and OC segmentation. The model exhibited competitive performance on RIM-ONE-r3 and REFUGE datasets.
4.2.2.2 Diagnosis, classification, and staging of DR
DR classification and staging based on DL have seen significant advancements in recent research. According to the American Academy of Ophthalmology’s international clinical classification system for diabetic retinopathy (DR), DR is categorized into various stages, including no DR, mild non-proliferative DR (NPDR), moderate NPDR, severe NPDR, proliferative DR, and diabetic macular edema (DME). Several studies have contributed novel DL models to enhance DR grading accuracy and performance. He et al. (88) introduced CABNet, a CNN with an attention module, achieving enhanced lesion detection and handling of imbalanced data, achieving accuracies of 78.98, 84.08, and 86.18%, with Kappa scores of 0.7863, 0.8723, and 0.8678 on the DDR, Messidor, and EyePACS datasets, respectively. Papadopoulos et al. (89) proposed an interpretable method with an attention mechanism for referable DR detection, with AUCs of 0.961 in Kaggle and 0.976 in Messidor-2, and with valid lesion heatmaps with AUPRC of 0.869 in IDRiD. Li et al. (90) established DACNN, an attentive CNN for imbalanced DR grading in retinal images, incorporating attention mechanisms for improved feature extraction with the results of 88.0% accuracy and 88.6% kappa score for multi-class DR grading on the EyePACS dataset, and 98.5% AUC, 93.8% accuracy, 87.9% kappa, 90.7% recall, 94.6% precision, and 92.6% F1-score for referral and non-referral classification on the Messidor dataset. Jian et al. (91) created Triple-DRNet, a three-stage cascade network with attention-enhanced subnets, achieving 92.08% accuracy and a 93.62% Quadratic Weighted Kappa. This model surpassed previous networks with accuracies of 98.99% for DR-Net, 88.40% for PDR-Net, and 80.20% for NPDR-Net. Murugappan et al. (92) implemented a Few-Shot Learning network with an attention mechanism for DR detection and grading, performing well both in detection (99.73% accuracy, 99.82% sensitivity, 99.63% specificity) and in grading (98.18% accuracy, 97.41% sensitivity, 99.55% specificity). Reddy and Gurrala (93) developed the Joint DR-DME Network (JDD-Net), which utilizes a deep graph correlation learning model. The model combines convolutional block attention module (CBAM) and joint disease attention (JDA) modules to extract disease-specific features, attaining accuracies of 99.53% for individual DR, 99.1% for individual DME, and 99.01% for combined DR-DME grading. In addition to traditional CNNs, Wu et al. (94) employed a Vision Transformer for DR grading, leveraging attention mechanisms for long-range pixel analysis, resulting in 91.4% accuracy, 97.7% specificity, 92.8% precision, 92.6% sensitivity, a QWK of 0.935, and an AUC of 0.986.
However, a common issue persists: these DL systems for DR grading often lack integration with medical knowledge. Consequently, ophthalmologists face challenges in accurately interpreting grading outcomes, limiting the practical applicability of these systems. To address the problem, Tian et al. (95) applied FA + KC-Net to integrate medical knowledge into DR grading, enhancing interpretability for ophthalmologists. The model achieved 84.49% accuracy and 86.17 QWK across datasets.
4.2.3 Outcome
AI has a high reference value for assessing the prognosis of retinal diseases. Accurate prediction will help physicians design treatment plans, improve the quality of care, and improve patient outcomes. Hashimoto et al. (96) trained a CNN with data from 591 eyes to predict visual field (VF) sensitivity using macular layer thickness, achieving low absolute errors (AE of 2.84 dB for the entire VF). This DL model also accurately predicted VF outcomes from the HFA 10–2 test using SD-OCT data. Li et al. (97) analyzed data from 17,497 eyes to develop and validate DL models for glaucoma forecasting, achieving AUROCs of 0.90 for incidence and 0.91 for progression in the validation cohort. External tests showed AUROCs of 0.87 to 0.89 for incidence and consistently 0.88 for progression, demonstrating robust generalizability. Yang et al. (98) utilized an automated algorithm to assess OCTA images from diabetic patients over 4 years, revealing that diabetic microvascular infarctions (DMIs) in both superficial and deep capillary plexuses signal diabetic retinal disease progression. Specifically, DMIs in the deep capillary plexus were significantly associated with DME onset and visual acuity deterioration.
4.2.3.1 Prediction of vision results
A significant advance in the treatment of retinal illnesses is intravitreal anti-vascular endothelial growth factor therapy; however, its effect on some patients is undesirable. AI technology can accurately predict the individual effects of treatment. Ideally, after the initial treatment, the AI model would take baseline photos and clinical data of the patient and provide anticipated follow-up intervals and overall treatment expectations. This could significantly improve the planning of anti-vascular endothelial growth factor therapy, prevent negative effects caused by under-treatment or over-treatment, and reduce treatment costs. Seebock et al. (99) used the random forest AI model to train the prognosis of patients receiving standardized ranibizumab treatment, and the accuracy rate of predicting individual vision results was 71%.
4.2.3.2 Prediction of the future natural course of retinal diseases
Early AMD is a chronic progressive disease with a highly heterogeneous progression rate. Patients with AMD may remain in the early stages without any associated functional impairment and rapidly progress to advanced AMD. Predicting advanced AMD is difficult in clinical practice. Ajana et al. (100) developed a machine-learning model for advanced AMD prediction, incorporating various risk factors and achieving a cross-validated AUC of 0.92 in training and test sets over 5 years, using data from two cohort studies. Moraes et al. (101) introduced a DL algorithm for automated OCT scan quantification in neovascular AMD, utilizing data from the Moorfields Eye Hospital AMD Database. This algorithm segmented features such as neurosensory retina and fluids, noting differences in feature volumes between first and second-treated eyes and among demographic groups, highlighting automated OCT segmentation’s potential in personalizing care and uncovering new structure–function relationships.
4.3 Open challenges and future opportunities
Summarizing the limitations of the research on the application of AI in retinal disease can guide future research. The limitations of the citations can be roughly divided into five aspects: (1) language limitations in the groups included in the studies; (2) Source limitations of the selected articles; (3) limitations of research methods; (4) bias caused by the subjective will of the participants; and (5) limitations of small sample sizes.
To overcome the problem of limitations in the groups included in the studies, researchers should aim to increase the diversity of the study sample by including participants with a wider range of characteristics, such as age, gender, race, ethnicity, socioeconomic status, and health status. This will help to ensure that the results are applicable to a broader population. To address the problem of limitations of research methods, researchers should consider using multiple methods to collect data, verifying their findings by replicating the study, 5 or using complementary methods. To mitigate the bias caused by the subjective will of the participants, researchers can use various strategies, such as ensuring informed consent, using blinding techniques, and avoiding leading questions. To address the limitations of small sample sizes, researchers can try to increase the sample size by recruiting more participants or by using statistical methods to compensate for the smaller sample size. It is also important to report effect sizes and confidence intervals to provide a more accurate estimate of the strength and precision of the results.
From the perspective of hot research directions, there is still room for improvement in future research. The dataset standard is inconsistent. Many retinal diseases show similar manifestations; the diagnosis mainly relies on experienced doctors, and there are variations in diagnosis between doctors. Given this situation, many research and development teams have collected several fundus pictures of different eye diseases, invited senior ophthalmologists to read the pictures, graded and partitioned the pictures, and established diagnostic datasets for some common diseases. However, many diseases are still without officially recognized datasets, and various reliable and unreliable data sets limit current diagnosis and treatment efforts.
Although many scholars have carried out some research work in this field and achieved phased results, the application of AI in retinal diseases mainly focuses on DR, AMD, and glaucoma at present, more data accumulation and data mining is still needed for retinal diseases such as ROP and myopia. In the future, Relevant researchers should develop more systematic prediction models, detect the hidden information in the images according to the guidance of clinicians, incorporate more evaluation factors, and conduct multimodal analysis to predict the treatment progress and success probability of retinal diseases.
4.4 Limitations
Our study encountered several limitations that merit consideration. We confined our literature review to articles dated between 2014 and 2023. Moreover, our search was restricted to English-language literature, thereby excluding potentially valuable data from unpublished or in-progress works in other languages. Additionally, our analysis was confined to the Web of Science Core Collection (WoSCC), a recognized academic database. While incorporating other databases could broaden the dataset, variations in citation metrics and indexing methods across platforms complicate cross-database integration and analysis. Furthermore, limitations in the literature screening process, stemming from both automated tools and manual exclusion, may have introduced minor inaccuracies. Finally, although we rigorously examined 4,386 articles, the study may still reflect a degree of inherent researcher bias that is challenging to eliminate entirely.
5 Conclusion
Through bibliometric analysis of worldwide literature over the past decade, we have traced the historical evolution, pinpointed current research foci, and projected future trends within this field. Despite progress, there remains a pressing need for refinement of DL algorithm models and enhancement of disease process screening methods. China holds the record for the highest volume of literature published, whereas the United States stands out as the most influential contributor to this research area, with Germany leading in international collaborations. The University of London and the National University of Singapore are distinguished by the high citation rates of their articles.
The refinement of AI algorithms and the exploration of abnormal eye physiological features constitute core pursuits in AI-assisted diagnosis of retinal diseases. Looking ahead, research should pivot toward developing sophisticated diagnostic systems for ophthalmic diseases and fostering integration between computer engineering and ophthalmology. Current challenges, such as bringing products to market, the lack of clinical validation, and data standardization issues, all require urgent attention. Furthermore, adapting to the dynamic landscape of medical knowledge is crucial.
Current challenges—including the need for market-ready products, clinical validation, and data standardization—require urgent attention. Adapting to the dynamic landscape of medical knowledge is also crucial. We recommend harnessing AI technology to support mobile healthcare initiatives, the development of intelligent health devices, and the real-time monitoring and evaluation of individual health. These advancements will not only facilitate early disease detection, DR screening, and proactive interventions but will also provide patients suffering from retinal diseases with safer, more convenient, and vastly improved management services.
Data availability statement
Publicly available datasets were analyzed in this study. This data can be found here: https://webofscience.clarivate.cn/wos/woscc/basic-search.
Author contributions
MG: Conceptualization, Formal analysis, Methodology, Software, Visualization, Writing – original draft, Writing – review & editing. DG: Conceptualization, Investigation, Writing – original draft, Writing – review & editing. WY: Funding acquisition, Project administration, Writing – review & editing.
Funding
The author(s) declare that financial support was received for the research, authorship, and/or publication of this article. This work was funded by the SanMing Project of Medicine in Shenzhen (No. SZSM202311012).
Conflict of interest
The authors declare that the research was conducted in the absence of any commercial or financial relationships that could be construed as a potential conflict of interest.
Publisher’s note
All claims expressed in this article are solely those of the authors and do not necessarily represent those of their affiliated organizations, or those of the publisher, the editors and the reviewers. Any product that may be evaluated in this article, or claim that may be made by its manufacturer, is not guaranteed or endorsed by the publisher.
Abbreviations
AI, Artificial intelligence; AMD, Age-related macular degeneration; AUC, Area under the curve; AUROC, Area under the receiver operating characteristic; CFP, Color fundus photograph; CI, Confidence interval; CNN, Convolutional neural network; DL, Deep learning; DME, Diabetic macular edema; DR, Diabetic retinopathy; NPDR, Non-proliferative diabetic retinopathy; OC, Optic cup; OCT, Optical coherence tomography; OCTA, Optical coherence tomography angiography; OD, Optic disc; QWK, Quadratic Weighted Kappa; RNFL, Retinal nerve fiber layer; ROP, Retinopathy of prematurity; SCI, Science Citation Index; WoSCC, Web of science core collection
References
1. Dzobo, K, Adotey, S, Thomford, NE, and Dzobo, W. Integrating artificial and human intelligence: a partnership for responsible innovation in biomedical engineering and medicine. OMICS. (2020) 24:247–63. doi: 10.1089/omi.2019.0038
2. Yang, WH, Shao, Y, and Xu, YW. Guidelines on clinical research evaluation of artificial intelligence in ophthalmology (2023). Int J Ophthalmol. (2023) 16:1361–72. doi: 10.18240/ijo.2023.09.02
3. Bashir, S, Qamar, U, and Khan, FH. WebMAC: a web based clinical expert system. Inform Syst Front. (2018) 20:1135–51. doi: 10.1007/s10796-016-9718-y
4. Somfai, GM, Tátrai, E, Laurik, L, Varga, B, Ölvedy, V, Jiang, H, et al. Automated classifiers for early detection and diagnosis of retinopathy in diabetic eyes. BMC Bioinformatics. (2014) 15:106. doi: 10.1186/1471-2105-15-106
5. Prentašic, P, Heisler, M, Mammo, Z, Lee, S, Merkur, A, Navajas, E, et al. Segmentation of the foveal microvasculature using deep learning networks. J Biomed Opt. (2016) 21:75008. doi: 10.1117/1.JBO.21.7.075008
6. Kassim, YM, Maude, RJ, and Palaniappan, K. Sensitivity of cross-trained deep CNNs for retinal vessel extraction. Annu Int Conf IEEE Eng Med Biol Soc. (2018) 2018:2736–9. doi: 10.1109/EMBC.2018.8512764
7. Benalcázar, ME, Brun, M, and Ballarin, VL. Artificial neural networks applied to statistical design of window operators. Pattern Recogn Lett. (2013) 34:970–9. doi: 10.1016/j.patrec.2013.01.029
8. Acharya, UR, Fujita, H, Bhat, S, Koh, JEW, Adam, M, Ghista, DN, et al. Automated diagnosis of diabetes using entropies and diabetic index. J Mech Med Biol. (2016) 16:1640008. doi: 10.1142/S021951941640008X
9. Hu, W, Zhang, Y, and Li, L. Study of the application of deep convolutional neural networks (CNNs) in processing sensor data and biomedical images. Sensors (Basel). (2019) 19:3584. doi: 10.3390/s19163584
10. Loo, J, Fang, L, Cunefare, D, Jaffe, GJ, and Farsiu, S. Deep longitudinal transfer learning-based automatic segmentation of photoreceptor ellipsoid zone defects on optical coherence tomography images of macular telangiectasia type 2. Biomed Opt Express. (2018) 9:2681–98. doi: 10.1364/BOE.9.002681
11. Burlina, P, Pacheco, KD, Joshi, N, Freund, DE, and Bressler, NM. Comparing humans and deep learning performance for grading AMD: a study in using universal deep features and transfer learning for automated AMD analysis. Comput Biol Med. (2017) 82:80–6. doi: 10.1016/j.compbiomed.2017.01.018
12. Yu, Y, Chen, X, Zhu, X, Zhang, P, Hou, Y, Zhang, R, et al. Performance of deep transfer learning for detecting abnormal fundus images. J Curr Ophthalmol. (2020) 32:368–74. doi: 10.4103/JOCO.JOCO_123_20
13. Al Mouiee, D, Meijering, E, Kalloniatis, M, Nivison-Smith, L, Williams, RA, Nayagam, DAX, et al. Classifying retinal degeneration in histological sections using deep learning. Transl Vis Sci Technol. (2021) 10:9. doi: 10.1167/tvst.10.7.9
14. Bhuiyan, A, Wong, TY, Ting, DSW, Govindaiah, A, Souied, EH, and Smith, RT. Smith: artificial intelligence to stratify severity of age-related macular degeneration (AMD) and predict risk of progression to late AMD. Transl Vis Sci Technol. (2020) 9:25. doi: 10.1167/tvst.9.2.25
15. Li, Q, Zhu, H, Fan, M, Sun, J, Reinach, PS, Wang, Y, et al. Form-deprivation myopia downregulates calcium levels in retinal horizontal cells in mice. Exp Eye Res. (2022) 218:109018. doi: 10.1016/j.exer.2022.109018
16. Dagliati, A, Marini, S, Sacchi, L, Cogni, G, Teliti, M, Tibollo, V, et al. Machine learning methods to predict diabetes complications. J Diabetes Sci Technol. (2018) 12:295–302. doi: 10.1177/1932296817706375
17. Choi, S, Park, J, Park, S, Byon, I, and Choi, HY. Establishment of a prediction tool for ocular trauma patients with machine learning algorithm. Int J Ophthalmol. (2021) 14:1941–9. doi: 10.18240/ijo.2021.12.20
18. Grzybowski, A, Markeviciute, A, and Zemaitiene, R. Treatment of macular edema in vascular retinal diseases: a 2021 update. J Clin Med. (2021) 10:5300. doi: 10.3390/jcm10225300
19. Dai, G, He, W, Xu, L, Pazo, EE, Lin, T, Liu, S, et al. Exploring the effect of hypertension on retinal microvasculature using deep learning on east Asian population. PLoS One. (2020) 15:e0230111. doi: 10.1371/journal.pone.0230111
20. Arsalan, M, Baek, NR, Owais, M, Mahmood, T, and Park, KR. Park: deep learning-based detection of pigment signs for analysis and diagnosis of retinitis Pigmentosa. Sensors (Basel). (2020) 20:3454. doi: 10.3390/s20123454
21. Wood, EH, Korot, E, Storey, PP, Muscat, S, Williams, GA, and Drenser, KA. The retina revolution: signaling pathway therapies, genetic therapies, mitochondrial therapies, artificial intelligence. Curr Opin Ophthalmol. (2020) 31:207–14. doi: 10.1097/ICU.0000000000000656
22. Gu, H, Guo, Y, Gu, L, Wei, A, Xie, S, Ye, Z, et al. Deep learning for identifying corneal diseases from ocular surface slit-lamp photographs. Sci Rep. (2020) 10:17851. doi: 10.1038/s41598-020-75027-3
23. Cavichini, M, An, C, Bartsch, DG, Jhingan, M, Amador-Patarroyo, MJ, Long, CP, et al. Artificial intelligence for automated overlay of fundus camera and scanning laser ophthalmoscope images. Transl Vis Sci Technol. (2020) 9:56. doi: 10.1167/tvst.9.2.56
24. Mun, Y, Kim, J, Noh, KJ, Lee, S, Kim, S, Yi, S, et al. An innovative strategy for standardized, structured, and interoperable results in ophthalmic examinations. BMC Med Inform Decis Mak. (2021) 21:9. doi: 10.1186/s12911-020-01370-0
25. Chen, YQ, Tao, JW, Su, LY, Li, L, Zhao, SX, Yang, Y, et al. Cooperative robot assistant for vitreoretinal microsurgery: development of the RVRMS and feasibility studies in an animal model. Graefes Arch Clin Exp Ophthalmol. (2017) 255:1167–71. doi: 10.1007/s00417-017-3656-3
26. Akbar, S, Sharif, M, Akram, MU, Saba, T, Mahmood, T, and Kolivand, M. Automated techniques for blood vessels segmentation through fundus retinal images: a review. Microsc Res Tech. (2019) 82:153–70. doi: 10.1002/jemt.23172
27. Akyol, K, Şen, B, and Bayır, Ş. Automatic detection of optic disc in retinal image by using Keypoint detection, texture analysis, and visual dictionary techniques. Comput Math Methods Med. (2016) 2016:6814791–10. doi: 10.1155/2016/6814791
28. Al-Bander, B, Al-Nuaimy, W, Williams, BM, and Zheng, Y. Multiscale sequential convolutional neural networks for simultaneous detection of fovea and optic disc. Biomed Signal Proces. (2018) 40:91–101. doi: 10.1016/j.bspc.2017.09.008
29. Azad, R, Gilbert, C, Gangwe, AB, Zhao, P, Wu, WC, Sarbajna, P, et al. Retinopathy of prematurity: how to prevent the third epidemics in developing countries. Asia Pac J Ophthalmol (Phila). (2020) 9:440–8. doi: 10.1097/APO.0000000000000313
30. Coyner, AS, Oh, MA, Shah, PK, Singh, P, Ostmo, S, Valikodath, NG, et al. Campbell: external validation of a retinopathy of prematurity screening model using artificial intelligence in 3 low-and middle-income populations. JAMA Ophthalmol. (2022) 140:791–8. doi: 10.1001/jamaophthalmol.2022.2135
31. Li, F, Wang, Y, Xu, T, Dong, L, Yan, L, Jiang, M, et al. Deep learning-based automated detection for diabetic retinopathy and diabetic macular oedema in retinal fundus photographs. Eye. (2022) 36:1433–41. doi: 10.1038/s41433-021-01552-8
32. Alsaih, K, Lemaitre, G, Rastgoo, M, Massich, J, Sidibé, D, and Meriaudeau, F. Machine learning techniques for diabetic macular edema (DME) classification on SD-OCT images. Biomed Eng Online. (2017) 16:68. doi: 10.1186/s12938-017-0352-9
33. Habra, O, Gallardo, M, Meyer, ZWT, De Zanet, S, Jaggi, D, Zinkernagel, M, et al. Evaluation of an artificial intelligence-based detector of sub-and Intraretinal fluid on a large set of optical coherence tomography volumes in age-related macular degeneration and diabetic macular edema. Ophthalmologica. (2022) 245:516–27. doi: 10.1159/000527345
34. Hao, S, Bai, J, Liu, H, Wang, L, Liu, T, Lin, C, et al. Comparison of machine learning tools for the prediction of AMD based on genetic, age, and diabetes-related variables in the Chinese population. Regen Ther. (2020) 15:180–6. doi: 10.1016/j.reth.2020.09.001
35. Heo, TY, Kim, KM, Min, HK, Gu, SM, Kim, JH, Yun, J, et al. Development of a deep-learning-based artificial intelligence tool for differential diagnosis between dry and Neovascular age-related macular degeneration. Diagnostics (Basel). (2020) 10:261. doi: 10.3390/diagnostics10050261
36. Aamir, M, Irfan, M, Ali, T, Ali, G, Shaf, A, Saeed, AS, et al. An adoptive threshold-based multi-level deep convolutional neural network for Glaucoma eye disease detection and classification. Diagnostics (Basel). (2020) 10:602. doi: 10.3390/diagnostics10080602
37. Abdullah, F, Imtiaz, R, Madni, HA, Khan, HA, Khan, TM, Khan, MAU, et al. A review on Glaucoma disease detection using computerized techniques. IEEE Access. (2021) 9:37311–33. doi: 10.1109/ACCESS.2021.3061451
38. Al-Aswad, LA, Kapoor, R, Chu, CK, Walters, S, Gong, D, Garg, A, et al. Evaluation of a deep learning system for identifying glaucomatous optic neuropathy based on color fundus photographs. J Glaucoma. (2019) 28:1029–34. doi: 10.1097/IJG.0000000000001319
39. Arcadu, F, Benmansour, F, Maunz, A, Michon, J, Haskova, Z, McClintock, D, et al. Deep learning predicts OCT measures of diabetic macular thickening from color fundus photographs. Invest Ophthalmol Vis Sci. (2019) 60:852–7. doi: 10.1167/iovs.18-25634
40. Antal, B, and Hajdu, A. Improving microaneurysm detection in color fundus images by using context-aware approaches. Comput Med Imaging Graph. (2013) 37:403–8. doi: 10.1016/j.compmedimag.2013.05.001
41. Murugan, R, Roy, P, and Singh, U. An abnormality detection of retinal fundus images by deep convolutional neural networks. Multimed Tools Appl. (2020) 79:24949–67. doi: 10.1007/s11042-020-09217-6
42. Hirsch, JE. An index to quantify an individual’s scientific research output. Proc Natl Acad Sci U S A. (2005) 102:16569–72. doi: 10.1073/pnas.0507655102
43. Gulshan, V, Peng, L, Coram, M, Stumpe, MC, Wu, D, Narayanaswamy, A, et al. Development and validation of a deep learning algorithm for detection of diabetic retinopathy in retinal fundus photographs. JAMA. (2016) 316:2402–10. doi: 10.1001/jama.2016.17216
44. Venhuizen, FG, van Ginneken, B, van Asten, F, van Grinsven, MJJP, Fauser, S, Hoyng, CB, et al. Automated staging of age-related macular degeneration using optical coherence tomography. Invest Ophth Vis Sci. (2017) 58:2318–28. doi: 10.1167/iovs.16-20541
45. Bogunović, H, Montuoro, A, Baratsits, M, Karantonis, MG, Waldstein, SM, Schlanitz, F, et al. Machine learning of the progression of intermediate age-related macular degeneration based on OCT imaging. Invest Ophth Vis Sci. (2017) 58:BIO141-BIO150. doi: 10.1167/iovs.17-21789
46. Balakrishnan, U, Venkatachalapathy, K, and Marimuthu, SG. A hybrid PSO-DEFS based feature selection for the identification of diabetic retinopathy. Curr Diabetes Rev. (2015) 11:182–90. doi: 10.2174/1573399811666150330150038
47. Bizios, D, Heijl, A, Hougaard, JL, and Bengtsson, B. Machine learning classifiers for glaucoma diagnosis based on classification of retinal nerve fibre layer thickness parameters measured by stratus OCT. Acta Ophthalmol. (2010) 88:44–52. doi: 10.1111/j.1755-3768.2009.01784.x
48. Legocki, AT, Lee, AY, Ding, L, Moshiri, Y, Zepeda, EM, Gillette, TB, et al. Multivariate models to diagnose early referral-warranted retinopathy of prematurity with handheld optical coherence tomography. Transl Vis Sci Technol. (2023) 12:26. doi: 10.1167/tvst.12.5.26
49. He, X, Wang, Y, Poiesi, F, Song, W, Xu, Q, Feng, Z, et al. Exploiting multi-granularity visual features for retinal layer segmentation in human eyes. Front Bioeng Biotechnol. (2023) 11:1191803. doi: 10.3389/fbioe.2023.1191803
50. Lu, Y, Shen, Y, Xing, X, Ye, C, and Meng, MQ. Boundary-enhanced semi-supervised retinal layer segmentation in optical coherence tomography images using fewer labels. Comput Med Imaging Graph. (2023) 105:102199. doi: 10.1016/j.compmedimag.2023.102199
51. Guo, M, Zhao, M, Cheong, AMY, Corvi, F, Chen, X, Chen, S, et al. Can deep learning improve the automatic segmentation of deep foveal avascular zone in optical coherence tomography angiography? Biomed Signal Proces. (2021) 66:102456. doi: 10.1016/j.bspc.2021.102456
52. Zhang, T, Wei, Q, Li, Z, Meng, W, Zhang, M, and Zhang, Z. Segmentation of paracentral acute middle maculopathy lesions in spectral-domain optical coherence tomography images through weakly supervised deep convolutional networks. Comput Methods Prog Biomed. (2023) 240:107632. doi: 10.1016/j.cmpb.2023.107632
53. Zhang, B, Zhao, H, Si, M, Cui, W, Zhou, Y, Fu, S, et al. RC-net: a region-level context network for hyperreflective dots segmentation in retinal OCT images. Opt Laser Eng. (2024) 172:107872. doi: 10.1016/j.optlaseng.2023.107872
54. Loo, J, Cai, CX, Choong, J, Chew, EY, Friedlander, M, Jaffe, GJ, et al. Deep learning-based classification and segmentation of retinal cavitations on optical coherence tomography images of macular telangiectasia type 2. Brit J Ophthalmol. (2022) 106:396–402. doi: 10.1136/bjophthalmol-2020-317131
55. Sunija, AP, Kar, S, Gayathri, S, Gopi, VP, and Palanisamy, P. OctNET: a lightweight CNN for retinal disease classification from optical coherence tomography images. Comput Meth Prog Biol. (2021) 200:105877. doi: 10.1016/j.cmpb.2020.105877
56. Togacar, M, Ergen, B, and Tumen, V. Use of dominant activations obtained by processing OCT images with the CNNs and slime mold method in retinal disease detection. Biocybern Biomed Eng. (2022) 42:646–66. doi: 10.1016/j.bbe.2022.05.005
57. Upadhyay, PK, Rastogi, S, and Kumar, KV. Coherent convolution neural network based retinal disease detection using optical coherence tomographic images. J King Saud Univ Comput Informat Sci. (2022) 34:9688–95. doi: 10.1016/j.jksuci.2021.12.002
58. Kayadibi, I, Guraksin, GE, and Kose, U. A hybrid R-FTCNN based on principal component analysis for retinal disease detection from OCT images. Expert Syst Appl. (2023) 230:120617. doi: 10.1016/j.eswa.2023.120617
59. Abdar, M, Fahami, MA, Rundo, L, Radeva, P, Frangi, AF, Acharya, UR, et al. Hercules: deep hierarchical attentive multilevel fusion model with uncertainty quantification for medical image classification. IEEE Trans Indust Inform. (2023) 19:274–85. doi: 10.1109/TII.2022.3168887
60. Bai, J, Wan, Z, Li, P, Chen, L, Wang, J, Fan, Y, et al. Accuracy and feasibility with AI-assisted OCT in retinal disorder community screening. Front Cell Dev Biol. (2022) 10:1053483. doi: 10.3389/fcell.2022.1053483
61. Hua, C, Kim, K, Huynh-The, T, You, JI, Yu, S, Le-Tien, T, et al. Convolutional network with twofold feature augmentation for diabetic retinopathy recognition from multi-modal images. IEEE J Biomed Health. (2021) 25:2686–97. doi: 10.1109/JBHI.2020.3041848
62. Flaxel, CJ, Adelman, RA, Bailey, ST, Fawzi, A, Lim, JI, Vemulakonda, GA, et al. Diabetic retinopathy preferred practice pattern®. Ophthalmology. (2020) 127:P66–P145. doi: 10.1016/j.ophtha.2019.09.025
63. Tang, F, Wang, X, Ran, A, Chan, CKM, Ho, M, Yip, W, et al. A multitask deep-learning system to classify diabetic macular edema for different optical coherence tomography devices: a multicenter analysis. Diabetes Care. (2021) 44:2078–88. doi: 10.2337/dc20-3064
64. Goh, JK, Cheung, CY, Sim, SS, Tan, PC, Tan, GS, and Wong, TY. Retinal imaging techniques for diabetic retinopathy screening. J Diabetes Sci Technol. (2016) 10:282–94. doi: 10.1177/1932296816629491
65. Tan, GS, Cheung, N, Simó, R, Cheung, GC, and Wong, TY. Diabetic macular oedema. Lancet Diabetes Endocrinol. (2017) 5:143–55. doi: 10.1016/S2213-8587(16)30052-3
66. You, QS, Tsuboi, K, Guo, Y, Wang, J, Flaxel, CJ, Bailey, ST, et al. Comparison of central macular fluid volume with central subfield thickness in patients with diabetic macular edema using optical coherence tomography angiography. JAMA Ophthalmol. (2021) 139:734–41. doi: 10.1001/jamaophthalmol.2021.1275
67. Xiong, H, You, QS, Guo, Y, Wang, J, Wang, B, Gao, L, et al. Deep learning-based signal-independent assessment of macular avascular area on 6x6 mm optical coherence tomography angiogram in diabetic retinopathy: a comparison to instrument-embedded software. Brit J Ophthalmol. (2023) 107:84–9. doi: 10.1136/bjophthalmol-2020-318646
68. Thomas, A, Harikrishnan, PM, Ramachandran, R, Ramachandran, S, Manoj, R, Palanisamy, P, et al. A novel multiscale and multipath convolutional neural network based age-related macular degeneration detection using OCT images. Comput Meth Prog Biol. (2021) 209:106294. doi: 10.1016/j.cmpb.2021.106294
69. Moradi, M, Chen, Y, Du, X, and Seddon, JM. Deep ensemble learning for automated non-advanced AMD classification using optimized retinal layer segmentation and SD-OCT scans. Comput Biol Med. (2023) 154:106512. doi: 10.1016/j.compbiomed.2022.106512
70. Sotoudeh-Paima, S, Jodeiri, A, Hajizadeh, F, and Soltanian-Zadeh, H. Multi-scale convolutional neural network for automated AMD classification using retinal OCT images. Comput Biol Med. (2022) 144:105368. doi: 10.1016/j.compbiomed.2022.105368
71. Liefers, B, Taylor, P, Alsaedi, A, Bailey, C, Balaskas, K, Dhingra, N, et al. Quantification of key retinal features in early and late age-related macular degeneration using deep learning. Am J Ophthalmol. (2021) 226:1–12. doi: 10.1016/j.ajo.2020.12.034
72. Metha, P, Peterson, CA, Wen, JC, Banitt, MR, Chen, PP, Bojikian, KD, et al. Automated detection of Glaucoma with interpretable machine learning using clinical data and multimodal retinal images. Am J Ophthalmol. (2021) 231:154–69. doi: 10.1016/j.ajo.2021.04.021
73. Sun, S, Ha, A, Kim, YK, Yoo, BW, Kim, HC, and Park, KH. Dual-input convolutional neural network for glaucoma diagnosis using spectral-domain optical coherence tomography. Brit J Ophthalmol. (2021) 105:1555–60. doi: 10.1136/bjophthalmol-2020-316274
74. Panda, SK, Cheong, H, Tun, TA, Chuangsuwanich, T, Kadziauskiene, A, Senthil, V, et al. The three-dimensional structural configuration of the central retinal vessel trunk and branches as a glaucoma biomarker. Am J Ophthalmol. (2022) 240:205–16. doi: 10.1016/j.ajo.2022.02.020
75. Bowd, C, Belghith, A, Zangwill, LM, Christopher, M, Goldbaum, MH, Fan, R, et al. Deep learning image analysis of optical coherence tomography angiography measured vessel density improves classification of healthy and Glaucoma eyes. Am J Ophthalmol. (2022) 236:298–308. doi: 10.1016/j.ajo.2021.11.008
76. He, X, Deng, Y, Fang, L, and Peng, Q. Multi-modal retinal image classification with modality-specific attention network. IEEE Trans Med Imaging. (2021) 40:1591–602. doi: 10.1109/TMI.2021.3059956
77. Garcia, G, Del Amor, R, Colomer, A, Verdu-Monedero, R, Morales-Sanchez, J, and Naranjo, V. Circumpapillary OCT-focused hybrid learning for glaucoma grading using tailored prototypical neural networks. Artif Intell Med. (2021) 118:102132. doi: 10.1016/j.artmed.2021.102132
78. Sreejith Kumar, AJ, Chong, RS, Crowston, JG, Chua, J, Bujor, I, Husain, R, et al. Evaluation of generative adversarial networks for high-resolution synthetic image generation of Circumpapillary optical coherence tomography images for Glaucoma. JAMA Ophthalmol. (2022) 140:974–81. doi: 10.1001/jamaophthalmol.2022.3375
79. Yuan, Y, Zhang, L, Wang, L, and Huang, H. Multi-level attention network for retinal vessel segmentation. IEEE J Biomed Health. (2022) 26:312–23. doi: 10.1109/JBHI.2021.3089201
80. Li, X, Jiang, Y, Li, M, and Yin, S. Lightweight attention convolutional neural network for retinal vessel image segmentation. IEEE Trans Indust Inform. (2021) 17:1958–67. doi: 10.1109/TII.2020.2993842
81. Liu, M, Wang, Z, Li, H, Wu, P, Alsaadi, FE, and Zeng, N. AA-WGAN: attention augmented Wasserstein generative adversarial network with application to fundus retinal vessel segmentation. Comput Biol Med. (2023) 158:106874. doi: 10.1016/j.compbiomed.2023.106874
82. Fu, Q, Li, S, and Wang, X. MSCNN-AM: a multi-scale convolutional neural network with attention mechanisms for retinal vessel segmentation. IEEE Access. (2020) 8:163926–36. doi: 10.1109/ACCESS.2020.3022177
83. Deng, X, and Ye, J. A retinal blood vessel segmentation based on improved D-MNet and pulse-coupled neural network. Biomed Signal Proces. (2022) 73:103467. doi: 10.1016/j.bspc.2021.103467
84. Ouyang, J, Liu, S, Peng, H, Garg, H, and Thanh, DNH. LEA U-net: a U-net-based deep learning framework with local feature enhancement and attention for retinal vessel segmentation. Complex Intell Syst. (2023) 9:6753–66. doi: 10.1007/s40747-023-01095-3
85. Guo, X, Li, J, Lin, Q, Tu, Z, Hu, X, and Che, S. Joint optic disc and cup segmentation using feature fusion and attention. Comput Biol Med. (2022) 150:106094. doi: 10.1016/j.compbiomed.2022.106094
86. Jiang, Y, Ma, Z, Wu, C, Zhang, Z, and Yan, W. RSAP-net: joint optic disc and cup segmentation with a residual spatial attention path module and MSRCR-PT pre-processing algorithm. BMC Bioinformatics. (2022) 23:523. doi: 10.1186/s12859-022-05058-2, Article-Number = {523
87. Wang, Y, Yu, X, and Wu, C. An efficient hierarchical optic disc and cup segmentation network combined with multi-task learning and adversarial learning. J Digit Imaging. (2022) 35:638–53. doi: 10.1007/s10278-021-00579-3
88. He, A, Li, T, Li, N, Wang, K, and Fu, H. CABNet: category attention block for imbalanced diabetic retinopathy grading. IEEE Trans Med Imaging. (2021) 40:143–53. doi: 10.1109/TMI.2020.3023463
89. Papadopoulos, A, Topouzis, F, and Delopoulos, A. An interpretable multiple-instance approach for the detection of referable diabetic retinopathy in fundus images. Sci Rep. (2021) 11:14326. doi: 10.1038/s41598-021-93632-8
90. Li, F, Tang, S, Chen, Y, and Zou, H. Deep attentive convolutional neural network for automatic grading of imbalanced diabetic retinopathy in retinal fundus images. Biomed Opt Express. (2022) 13:5813–35. doi: 10.1364/BOE.472176
91. Jian, M, Chen, H, Tao, C, Li, X, and Wang, G. Triple-DRNet: a triple-cascade convolution neural network for diabetic retinopathy grading using fundus images. Comput Biol Med. (2023) 155:106631. doi: 10.1016/j.compbiomed.2023.106631
92. Murugappan, M, Prakash, NB, Jeya, R, Mohanarathinam, A, Hemalakshmi, GR, and Mahmud, M. A novel few-shot classification framework for diabetic retinopathy detection and grading. Measurement. (2022) 200:111485. doi: 10.1016/j.measurement.2022.111485
93. Reddy, PCV, and Gurrala, KK. Gurrala: joint DR-DME grading classification using optimal feature selection-based deep graph correlation network. Appl Soft Comput. (2023) 149:110981. doi: 10.1016/j.asoc.2023.110981
94. Wu, J, Hu, R, Xiao, Z, Chen, J, and Liu, J. Vision transformer-based recognition of diabetic retinopathy grade. Med Phys. (2021) 48:7850–63. doi: 10.1002/mp.15312
95. Tian, M, Wang, H, Sun, Y, Wu, S, Tang, Q, and Zhang, M. Fine-grained attention & knowledge-based collaborative network for diabetic retinopathy grading. Heliyon. (2023) 9:e17217. doi: 10.1016/j.heliyon.2023.e17217
96. Hashimoto, Y, Asaoka, R, Kiwaki, T, Sugiura, H, Asano, S, Murata, H, et al. Deep learning model to predict visual field in central 10° from optical coherence tomography measurement in glaucoma. Brit J Ophthalmol. (2021) 105:507–13. doi: 10.1136/bjophthalmol-2019-315600
97. Li, F, Su, Y, Lin, F, Li, Z, Song, Y, Nie, S, et al. A deep-learning system predicts glaucoma incidence and progression using retinal photographs. J Clin Invest. (2022) 132:e157968. doi: 10.1172/JCI157968
98. Yang, D, Tang, Z, Ran, A, Nguyen, TXX, Szeto, S, Chan, J, et al. Assessment of Parafoveal diabetic macular ischemia on optical coherence tomography angiography images to predict diabetic retinal disease progression and visual acuity deterioration. JAMA Ophthalmol. (2023) 141:641–9. doi: 10.1001/jamaophthalmol.2023.1821
99. Seeboeck, P, Waldstein, SM, Klimscha, S, Bogunovic, H, Schlegl, T, Gerendas, BS, et al. Unsupervised identification of disease marker candidates in retinal OCT imaging data. IEEE Trans Med Imaging. (2019) 38:1037–47. doi: 10.1109/TMI.2018.2877080
100. S AjanaCougnard-Grégoire, A, Colijn, JM, Merle, BMJ, Verzijden, T, de Jong, PTVM, et al. Predicting progression to advanced age-related macular degeneration from clinical, genetic, and lifestyle factors using machine learning. Ophthalmology. (2021) 128:587–97. doi: 10.1016/j.ophtha.2020.08.031
101. Moraes, G, Fu, DJ, Wilson, M, Khalid, H, Wagner, SK, Korot, E, et al. Quantitative analysis of OCT for Neovascular age-related macular degeneration using deep learning. Ophthalmology. (2021) 128:693–705. doi: 10.1016/j.ophtha.2020.09.025
102. Kermany, DS, Goldbaum, M, Cai, W, Valentim, C, Liang, H, Baxter, SL, et al. Identifying medical diagnoses and treatable diseases by image-based deep learning. Cell. (2018) 172:1122–1131.e9. doi: 10.1016/j.cell.2018.02.010
103. De Fauw, J, Ledsam, JR, Romera-Paredes, B, Nikolov, S, Tomasev, N, Blackwell, S, et al. Clinically applicable deep learning for diagnosis and referral in retinal disease. Nat Med. (2018) 24:1342–50. doi: 10.1038/s41591-018-0107-6
104. Ting, D, Cheung, CY, Lim, G, Tan, G, Quang, ND, Gan, A, et al. Development and validation of a deep learning system for diabetic retinopathy and related eye diseases using retinal images from multiethnic populations with diabetes. JAMA. (2017) 318:2211–23. doi: 10.1001/jama.2017.18152
105. Gu, Z, Cheng, J, Fu, H, Zhou, K, Hao, H, Zhao, Y, et al. CE-net: context encoder network for 2D medical image segmentation. IEEE Trans Med Imaging. (2019) 38:2281–92. doi: 10.1109/TMI.2019.2903562
106. Poplin, R, Varadarajan, AV, Blumer, K, Liu, Y, McConnell, MV, Corrado, GS, et al. Prediction of cardiovascular risk factors from retinal fundus photographs via deep learning. Nat Biomed Eng. (2018) 2:158–64. doi: 10.1038/s41551-018-0195-0
107. Gargeya, R, and Leng, T. Automated identification of diabetic retinopathy using deep learning. Ophthalmology. (2017) 124:962–9. doi: 10.1016/j.ophtha.2017.02.008
108. Abràmoff, MD, Lavin, PT, Birch, M, Shah, N, and Folk, JC. Pivotal trial of an autonomous AI-based diagnostic system for detection of diabetic retinopathy in primary care offices. NPJ Digit Med. (2018) 1:39. doi: 10.1038/s41746-018-0040-6
109. Liskowski, P, and Krawiec, K. Segmenting retinal blood vessels with deep neural networks. IEEE Trans Med Imaging. (2016) 35:2369–80. doi: 10.1109/TMI.2016.2546227
Keywords: artificial intelligence, retinal disease, deep learning, machine learning, hotspot, trend
Citation: Guo M, Gong D and Yang W (2024) In-depth analysis of research hotspots and emerging trends in AI for retinal diseases over the past decade. Front. Med. 11:1489139. doi: 10.3389/fmed.2024.1489139
Edited by:
Yitian Zhao, Chinese Academy of Sciences (CAS), ChinaReviewed by:
Chubin Ou, Macquarie University, AustraliaLi Li, The University of Melbourne, Australia
Copyright © 2024 Guo, Gong and Yang. This is an open-access article distributed under the terms of the Creative Commons Attribution License (CC BY). The use, distribution or reproduction in other forums is permitted, provided the original author(s) and the copyright owner(s) are credited and that the original publication in this journal is cited, in accordance with accepted academic practice. No use, distribution or reproduction is permitted which does not comply with these terms.
*Correspondence: Di Gong, Z29uZ2RpMTk5NTEyQDE2My5jb20=; Weihua Yang, YmVuYmVuMDYwNkAxMzkuY29t