- 1Division of Colorectal Surgery, Changzheng Hospital, Navy Medical University, Shanghai, China
- 2UM-SJTU Joint Institute, Shanghai Jiao Tong University, Shanghai, China
- 3Department of Pathology, Changzheng Hospital, Navy Medical University, Shanghai, China
- 4Division of Breast and Thyroid Surgery, Changzheng Hospital, Navy Medical University, Shanghai, China
This study aims to evaluate the feasibility of large language model (LLM) in answering pathology questions based on pathology reports (PRs) of colorectal cancer (CRC). Four common questions (CQs) and corresponding answers about pathology were retrieved from public webpages. These questions were input as prompts for Chat Generative Pretrained Transformer (ChatGPT) (gpt-3.5-turbo). The quality indicators (understanding, scientificity, satisfaction) of all answers were evaluated by gastroenterologists. Standard PRs from 5 CRC patients who received radical surgeries in Shanghai Changzheng Hospital were selected. Six report questions (RQs) and corresponding answers were generated by a gastroenterologist and a pathologist. We developed an interactive PRs interpretation system which allows users to upload standard PRs as JPG images. Then the ChatGPT's responses to the RQs were generated. The quality indicators of all answers were evaluated by gastroenterologists and out-patients. As for CQs, gastroenterologists rated AI answers similarly to non-AI answers in understanding, scientificity, and satisfaction. As for RQ1-3, gastroenterologists and patients rated the AI mean scores higher than non-AI scores among the quality indicators. However, as for RQ4-6, gastroenterologists rated the AI mean scores lower than non-AI scores in understanding and satisfaction. In RQ4, gastroenterologists rated the AI scores lower than non-AI scores in scientificity (P = 0.011); patients rated the AI scores lower than non-AI scores in understanding (P = 0.004) and satisfaction (P = 0.011). In conclusion, LLM could generate credible answers to common pathology questions and conceptual questions on the PRs. It holds great potential in improving doctor-patient communication.
Introduction
Large language model (LLM) combines the power of deep learning with transformer architectures to understand and generate human language, showing potential in answering medical questions (1, 2). The current LLM has been found to be prone to errors in the specialty, leading to limited efficacy in clinical practice (3). Nonetheless, several studies showed LLM can effectively convey health information and generate answers of higher quality and greater empathy compared to those produced by doctors (4, 5). Pathology reports (PRs) which contain information for diagnostic evaluation and clinical decision making are often hard to understand for patients (6–8). Incorporating these cost-effective technological solutions into clinical practice has the potential to mitigate disparities and provide particular benefits to patients from socioeconomically disadvantaged backgrounds, who typically exhibit lower levels of health literacy (9). In this study, we evaluated the ability of LLM to answer pathology questions based on PRs of colorectal cancer (CRC). These efforts take a patient-centric approach and aim to holistically evaluate LLM-based systems' ability to explain complex medical reports to patients, which distinguishes our study from previous works.
Materials and methods
LLM answers common pathology questions
Four common questions (CQs) and corresponding answers (1 question matches 2 answers) about pathology were retrieved based on their frequency and relevance from publicly available medical resources including reputable medical websites, online health forums, and pathology textbooks. These questions were input as prompts for Chat Generative Pretrained Transformer (ChatGPT) (gpt-3.5-turbo) twice on the same day, and the answers were recorded as artificial intelligence (AI1 and AI2), respectively. All the answers were anonymized to prevent any identification of the generation time or sequence, ensuring an unbiased evaluation. The quality indicators (understanding, scientificity, satisfaction) of 16 answers were evaluated by six gastroenterologists (three senior gastroenterologists and three fellows) on a 7-point Likert scale (10). The answers were randomized before being presented to the gastroenterologists to avoid potential order effects. The text similarity among all answers were compared using Jaccard Similarity (11). The raters were also asked to determine whether the answers were generated by AI or not.
LLM answers pathology questions based on PRs
Standard PRs from 5 CRC patients who received radical surgeries in Shanghai Changzheng Hospital between January 1, 2022, and December 31, 2022 were selected. These patients were selected based on the criteria of being free from distant metastasis. The selection of these reports aimed to ensure a diverse yet representative sample of standard PRs in CRC. Six report questions (RQs) were developed for each PR, focus on pathological type, pathological stage, immunohistochemical result, adjuvant therapy, prognosis, and follow-up. The corresponding answers (1 question matches 1 answer) were generated by a gastroenterologist and a pathologist based on National Comprehensive Cancer Network Guidelines (Version 3. 2023). We developed an interactive PRs interpretation system which allows users to upload standard PRs of CRC as JPG images (http://pathology.doctorhealthx.com). We used optical character recognition to digitalize the uploaded reports and convert image data into text format and then prompt ChatGPT questions based on the reports (12, 13). ChatGPT's responses to these questions were generated, recorded, and carefully reviewed to ensure accurate representation of the original reports. The quality of 60 answers were evaluated by six gastroenterologists and seven out-patients using the 7-point Likert scale.
Statistical analysis
Data was shown as mean (standard deviation). We used Mann-Whitney U test to compare the quality indicator and readability of answers. We used the readability metric from a text analysis package “cntext” to calculate the text complexity of Chinese, measured by the average number of characters in each clause and the proportion of verbs and conjunctions in each sentence (14). Sensitivity, specificity, positive predictive value, negative predictive value, and accuracy were calculated to evaluate raters' performance in detecting AI-generated answers. All analyses were accomplished using software SPSS (version 25), and 2-sided P < 0.05 was considered statistically significant.
Results
Performance of answering CQs
As for CQs, the AI answers had low text similarity (10%-23%) compared to non-AI answers, and the text similarity ranged from 30% to 42% between the 2 AI answers (Table 1). The gastroenterologists demonstrated 56.9% accuracy in identifying AI answers, with 45.8% sensitivity and 62.5% specificity. Fellows showed 50.0% accuracy and 33.3% sensitivity (Table 2). Gastroenterologists rated AI answers similarly to non-AI answers in understanding (AI, 4.5–5.7 vs. non-AI, 4.7–5.3, nonsignificant), with the AI mean scores higher than non-AI scores in the CQ2 and 4. Scientific adequacy scores were also similar (AI, 4.8–5.7 vs. non-AI, 4.7–5.3, nonsignificant), with the AI mean scores higher than non-AI scores, except for the CQ3. AI and non-AI received similar scores regarding satisfaction with the answers (AI, 5.2–5.7 vs. non-AI, 4.4–5.1, nonsignificant), with the AI mean scores higher than non-AI scores (Table 3).
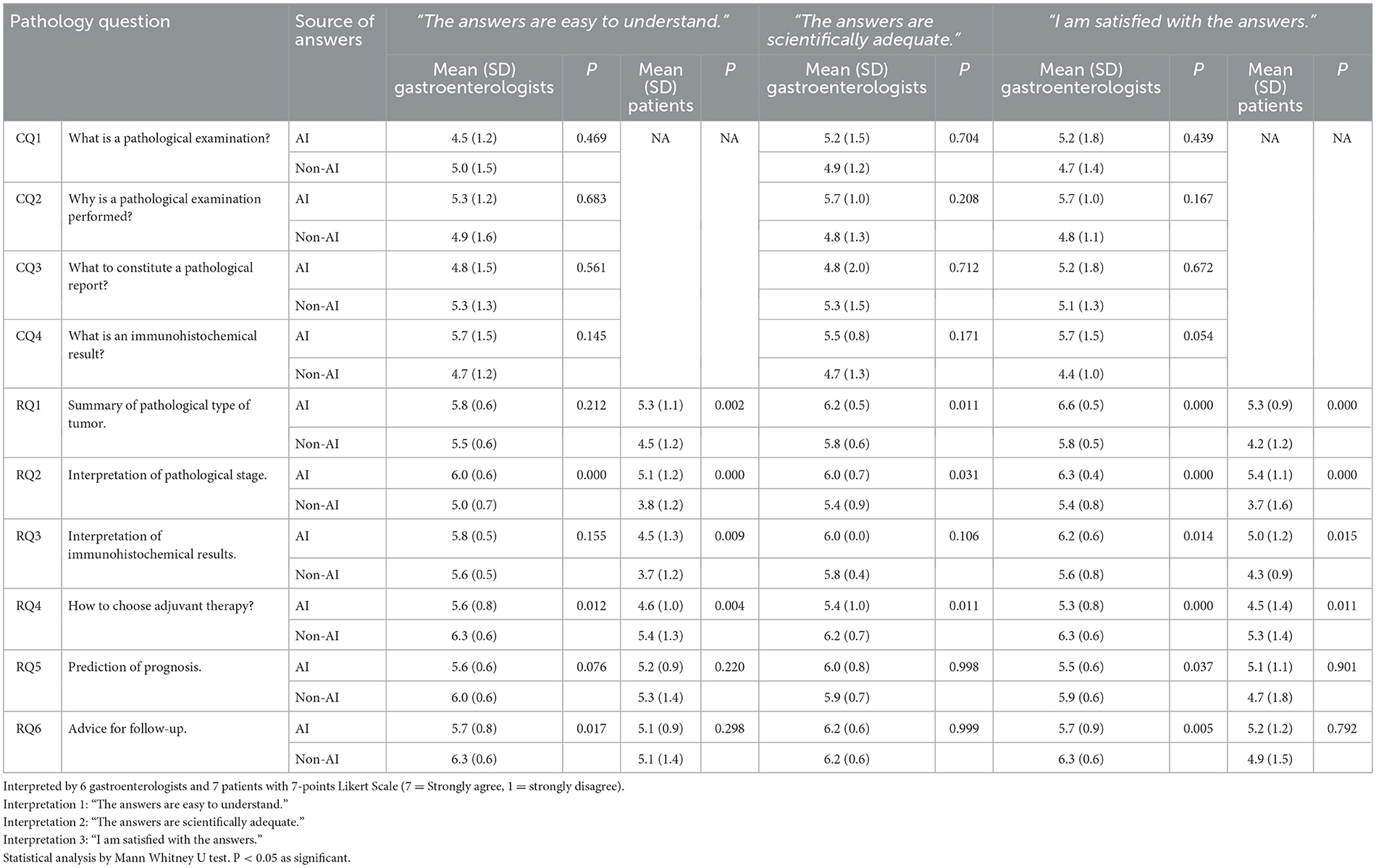
Table 3. Quality indicators for answers to common question (CQ) and report question (RQ) from AI and from non-AI sources.
Performance of answering questions based on PRs
As for RQ1-3, gastroenterologists rated the AI mean scores higher than non-AI scores in understanding (AI, 5.8–6.0 vs. non-AI, 5.0–5.6, significant in RQ2), scientific adequacy (AI, 6.0–6.2 vs. non-AI, 5.4–5.8, significant in RQ1 and 2), and satisfaction (AI, 6.2–6.6 vs. non-AI, 5.4–5.8, significant in RQ1-3). Similarly, patients rated the AI mean scores higher than non-AI scores in understanding (AI, 4.5–5.3 vs. non-AI, 3.7–4.5, significant in RQ1-3) and satisfaction (AI, 5.0–5.4 vs. non-AI, 3.7–4.3, significant in RQ1-3). However, as for RQ4-6, gastroenterologists rated the AI mean scores lower than non-AI scores in understanding (AI, 5.6–5.7 vs. non-AI, 6.0–6.3, significant in RQ4 and 6) and satisfaction (AI, 5.3–5.7 vs. non-AI, 5.9–6.3, significant in RQ4-6). In RQ4, gastroenterologists rated the AI mean scores lower than non-AI scores in scientific adequacy (AI, 5.4 vs. non-AI, 6.2, P = 0.011); patients rated the AI mean scores lower than non-AI scores in understanding (AI, 4.6 vs. non-AI, 5.4, P = 0.004) and satisfaction (AI, 4.5 vs. non-AI, 5.3, P = 0.011) (Table 3).
Performance of readability
Overall, AI answers had more words than non-AI answers (AI, 338.1 vs. non-AI, 179.7). The complexity levels were a little higher for AI answers, with readability scores lower than non-AI answers (AI, 18.4 vs. non-AI, 21.5), although no statistical significance was found. In answering CQs, AI answers had lower readability scores than non-AI answers (AI, 18.1 vs. non-AI, 29.7, P = 0.005). In answering RQs, AI had lower readability scores in RQ2, 3, 4, and 6, but no significant statistical differences were found except in RQ3 (P = 0.008); AI had higher readability scores in RQ1 and 5, with significant statistical differences (P = 0.021 and 0.008). This could be attributed to the overall long lengths of the AI answers (Table 4).
Discussion
The text similarity results suggested the inherent anti-plagiarism design in LLM and the ability of LLM to create unique answers. Though raters performed low sensitivity in identifying AI-generated answers, an out-performing gastroenterologist considered that AI-generated answer was more like a structured paragraph. In contrast, answers from public webpages were more like colloquial responses. Our evaluation revealed that AI matched human performance in answering CQs and excelled in conceptual matters on the PRs (RQ1-3). However, AI struggled with questions requiring current domain-specific knowledge, such as treatment plans, prognostic prediction, and follow-up advice (RQ4-6). Despite AI's propensity for generating comprehensible responses, it may contain fabricated or outdated information—a phenomenon known as “hallucination” (15).
We conducted this study as a proof-of-concept and performed thorough quantitative and qualitative analyses to ensure that our findings are statistically rigorous. While the small sample size and specific type of standard PRs in the present study are limitations that may impact the universality of our results, our findings nonetheless reflect the potential of LLMs for improving doctor-patient communication and patients' understanding of complex medical reports. In future works, we plan to expand the sample size to include multi-center data in order to more systematically verify our findings.
As LLMs continue to advance, we have found that more recent models, such as Anthropic's Claude 3 Opus, demonstrate improved readability (Supplementary Table 3) and hold great potential for driving continuous enhancements in the clarity of explanations. In future work, we plan to further develop more advanced LLM-based PRs interpretation systems by integrating external medical data, such as cancer knowledge graphs and clinical guidelines (16). By applying techniques like Retrieval Augmented Generation and tool learning agents, we aim to further enhance the accuracy and response verifiability of LLM-based systems in answering pathology questions (17, 18).
In conclusion, LLMs have shown potential to generate credible answers to common pathology questions. With further enhancements, LLMs hold great promise in improving doctor-patient communication regarding professional PRs.
Data availability statement
The raw data supporting the conclusions of this article will be made available by the authors, without undue reservation.
Ethics statement
The studies involving humans were approved by the Ethics Committee of Changzheng Hospital. The studies were conducted in accordance with the local legislation and institutional requirements. Written informed consent for participation was not required from the participants or the participants' legal guardians/next of kin in accordance with the national legislation and institutional requirements.
Author contributions
AW: Data curation, Formal analysis, Methodology, Project administration, Software, Writing – original draft. JZ: Investigation, Software, Supervision, Validation, Writing – original draft. PZ: Software, Writing – original draft. HC: Data curation, Software, Writing – original draft. HX: Conceptualization, Formal analysis, Project administration, Supervision, Writing – review & editing. XX: Formal analysis, Resources, Supervision, Validation, Visualization, Writing – review & editing. HZ: Conceptualization, Data curation, Funding acquisition, Project administration, Resources, Supervision, Validation, Visualization, Writing – review & editing.
Funding
The author(s) declare financial support was received for the research, authorship, and/or publication of this article. This work was supported by the National Key R&D Program of China (2019YFA0110601).
Acknowledgments
We selected Chat Generative Pretrained Transformer (gpt-3.5-turbo) from OpenAI as the large language model at the time of this study.
Conflict of interest
The authors declare that the research was conducted in the absence of any commercial or financial relationships that could be construed as a potential conflict of interest.
Publisher's note
All claims expressed in this article are solely those of the authors and do not necessarily represent those of their affiliated organizations, or those of the publisher, the editors and the reviewers. Any product that may be evaluated in this article, or claim that may be made by its manufacturer, is not guaranteed or endorsed by the publisher.
Supplementary material
The Supplementary Material for this article can be found online at: https://www.frontiersin.org/articles/10.3389/fmed.2024.1402457/full#supplementary-material
References
1. Gilson A, Safranek CW, Huang T, Socrates V, Chi L, Taylor RA, et al. How does ChatGPT perform on the United States Medical Licensing Examination (USMLE)? The implications of large language models for medical education and knowledge assessment. JMIR Med Educ. (2023) 9:e45312. doi: 10.2196/45312
2. Johnson D, Goodman R, Patrinely J, Stone C, Zimmerman E, Donald R, et al. Assessing the accuracy and reliability of AI-generated medical responses: an evaluation of the Chat-GPT model. Res Sq. (2023). rs.3.rs-2566942. doi: 10.21203/rs.3.rs-2566942/v1
3. Thirunavukarasu AJ, Ting DSJ, Elangovan K, Gutierrez L, Tan TF, Ting DSW. Large language models in medicine. Nat Med. (2023) 29:1930–40. doi: 10.1038/s41591-023-02448-8
4. Ayers JW, Poliak A, Dredze M, Leas EC, Zhu Z, Kelley JB, et al. Comparing physician and artificial intelligence Chatbot responses to patient questions posted to a public social media forum. JAMA Intern Med. (2023) 183:589–96. doi: 10.1001/jamainternmed.2023.1838
5. Ali SR, Dobbs TD, Hutchings HA, Whitaker IS. Using ChatGPT to write patient clinic letters. Lancet Digit Health. (2023) 5:e179–81. doi: 10.1016/S2589-7500(23)00048-1
6. Koetsier S, Jones GR, Badrick T. Safe reading of chemical pathology reports: the RCPAQAP Report Assessment Survey. Pathology. (2016) 48:357–62. doi: 10.1016/j.pathol.2016.02.018
7. Mossanen M, Calvert JK, Wright JL, True LD, Lin DW, Gore JL. Readability of urologic pathology reports: the need for patient-centered approaches. Urol Oncol. (2014) 32:1091–4. doi: 10.1016/j.urolonc.2014.04.011
8. Daniel C, Booker D, Beckwith B, Mea VD, García-Rojo M, Havener L, et al. Standards and specifications in pathology: image management, report management and terminology. Stud Health Technol Inform. (2012) 179:105–22. doi: 10.3233/978-1-61499-086-4-105
9. Stormacq C, Van den Broucke S, Wosinski J. Does health literacy mediate the relationship between socioeconomic status and health disparities? Integrative review. Health Promot Int. (2019) 34:e1–17. doi: 10.1093/heapro/day062
10. Lee TC, Staller K, Botoman V, Pathipati MP, Varma S, Kuo B. ChatGPT answers common patient questions about colonoscopy. Gastroenterology. (2023) 165:509–11.e7. doi: 10.1053/j.gastro.2023.04.033
11. Chung NC, Miasojedow B, Startek M, Gambin A. Jaccard/Tanimoto similarity test and estimation methods for biological presence-absence data. BMC Bioinform. (2019) 20:644. doi: 10.1186/s12859-019-3118-5
12. Beutel G, Geerits E, Kielstein JT. Artificial hallucination: GPT on LSD? Crit Care. (2023) 27:148. doi: 10.1186/s13054-023-04425-6
13. Balas M, Herman J, Bhambra NS, Longwell J, Popovic MM, Melo IM, et al. OCTess: an optical character recognition algorithm for automated data extraction of spectral domain optical coherence tomography reports. Retina. (2024) 44:558–64. doi: 10.1097/IAE.0000000000003990
14. Hasan SMS, Rivera D, Wu XC, Durbin EB, Christian JB, Tourassi G. Knowledge graph-enabled cancer data analytics. IEEE J Biomed Health Inform. (2020) 24:1952–67. doi: 10.1109/JBHI.2020.2990797
15. Eysenbach G. The role of ChatGPT, Generative language models, and artificial intelligence in medical education: a conversation with ChatGPT and a call for papers. JMIR Med Educ. (2023) 9:e46885. doi: 10.2196/46885
16. Fei H, Ren Y, Zhang Y, Ji D, Liang X. Enriching contextualized language model from knowledge graph for biomedical information extraction. Brief Bioinform. (2021) 22:bbaa110. doi: 10.1093/bib/bbaa110
17. Zakka C, Shad R, Chaurasia A, Dalal AR, Kim JL, Moor M, et al. Almanac - retrieval-augmented language models for clinical medicine. NEJM AI. (2024) 1:10.1056/aioa2300068. doi: 10.1056/aioa2300068
Keywords: large language model, medical question, pathology report, colorectal cancer, Generative Pretrained Transformer
Citation: Wang A, Zhou J, Zhang P, Cao H, Xin H, Xu X and Zhou H (2024) Large language model answers medical questions about standard pathology reports. Front. Med. 11:1402457. doi: 10.3389/fmed.2024.1402457
Received: 17 March 2024; Accepted: 28 August 2024;
Published: 18 September 2024.
Edited by:
Fuyong Xing, University of Colorado Anschutz Medical Campus, United StatesReviewed by:
Dingxi Liu, Wuhan University of Science and Technology, ChinaTian Bai, Jilin University, China
Copyright © 2024 Wang, Zhou, Zhang, Cao, Xin, Xu and Zhou. This is an open-access article distributed under the terms of the Creative Commons Attribution License (CC BY). The use, distribution or reproduction in other forums is permitted, provided the original author(s) and the copyright owner(s) are credited and that the original publication in this journal is cited, in accordance with accepted academic practice. No use, distribution or reproduction is permitted which does not comply with these terms.
*Correspondence: Haiyang Zhou, aGFpeWFuZzE5ODVfMSYjeDAwMDQwO2FsaXl1bi5jb20=; Xinyun Xu, eHh5MTIyODExMTcmI3gwMDA0MDsxNjMuY29t
†These authors have contributed equally to this work