- 1Department of Respiratory and Critical Care Medicine, The First Affiliated Hospital of Chongqing Medical University, Chongqing, China
- 2Dianei Technology, Shanghai, China
- 3University of California, San Diego, San Diego, CA, United States
- 4Department of Radiology, The First Affiliated Hospital of Chongqing Medical University, Chongqing, China
- 5School of Computer Science, Wuhan University, Wuhan, China
- 6Shanghai Jiao Tong University, Shanghai, China
- 7École Polytechnique Fédérale de Lausanne, Lausanne, Switzerland
In the clinic, it is difficult to distinguish the malignancy and aggressiveness of solid pulmonary nodules (PNs). Incorrect assessments may lead to delayed diagnosis and an increased risk of complications. We developed and validated a deep learning-based model for the prediction of malignancy as well as local or distant metastasis in solid PNs based on CT images of primary lesions during initial diagnosis. In this study, we reviewed the data from multiple patients with solid PNs at our institution from 1 January 2019 to 30 April 2022. The patients were divided into three groups: benign, Ia-stage lung cancer, and T1-stage lung cancer with metastasis. Each cohort was further split into training and testing groups. The deep learning system predicted the malignancy and metastasis status of solid PNs based on CT images, and then we compared the malignancy prediction results among four different levels of clinicians. Experiments confirmed that human–computer collaboration can further enhance diagnostic accuracy. We made a held-out testing set of 134 cases, with 689 cases in total. Our convolutional neural network model reached an area under the ROC (AUC) of 80.37% for malignancy prediction and an AUC of 86.44% for metastasis prediction. In observer studies involving four clinicians, the proposed deep learning method outperformed a junior respiratory clinician and a 5-year respiratory clinician by considerable margins; it was on par with a senior respiratory clinician and was only slightly inferior to a senior radiologist. Our human–computer collaboration experiment showed that by simply adding binary human diagnosis into model prediction probabilities, model AUC scores improved to 81.80–88.70% when combined with three out of four clinicians. In summary, the deep learning method can accurately diagnose the malignancy of solid PNs, improve its performance when collaborating with human experts, predict local or distant metastasis in patients with T1-stage lung cancer, and facilitate the application of precision medicine.
1. Introduction
Lung cancer is the leading cause of cancer-related death worldwide (1–3). Pulmonary nodules (PNs) are an early and potentially curable form of lung cancer (4). In screening for lung cancer, the average detection rate of PNs has increased to 22.00%−59.70%, of which < 5% are malignant nodules (5–7). Early diagnosis of malignant solid nodules is especially important to improve the prognosis of lung cancer due to its indeterminate aggressive characteristics (8–11). Although several studies have shown that the rate of malignancy in solid nodules is lower than that in ground-glass nodules, distinguishing benign and malignant solid PNs is even more difficult than distinguishing ground-glass nodules due to their overlapping characteristics with lung cancer in CT imaging (12–16). It was reported that among excision PNs, the proportion of benign lesions can be as high as 51.67%, and most of them were solid nodules (17, 18). Meanwhile, metastasis accounts for a vast majority of lung cancer-related deaths (19). Early screening for lung cancer has shown an increased detection rate of early-stage lung cancer, with some small nodules having been found to have metastases at preliminary diagnosis (10, 11, 20–22). Accurate TNM staging is an important prerequisite for the treatment of lung cancer. At least 20% of patients who undergo curative lung surgery relapse with undiagnosed metastatic disease, indicating that the current approach, which mainly includes positron-emission tomography (PET-CT), CT, MRI, or invasive pathologic assessment of cancer staging, has its limitations (23–25). There is still a clinical need for new, robust, cost-effective, and convenient, non-invasive imaging parameters to better predict the malignancy and metastasis status of solid PNs.
In recent years, deep learning has shown vast potential in medical applications and has also made great progress in pulmonary nodule diagnosis (26–34). Moreover, some researchers have predicted lymph node invasion using deep learning, radiomics models, and other methods (35–40), but predicting malignancy and M-stage metastasis for solid PNs remains inadequate. Therefore, the purpose of this study is to predict the malignancy and local or distant metastasis of solid PNs with deep learning based on chest CT images of the primary lesions. The hope is to increase the potential for the timely and reliable treatment of these highly aggressive lung nodules.
2. Materials and methods
2.1. Patients
This study was approved by the Ethics Committee of the First Affiliated Hospital of Chongqing Medical University (2022-K139), and patient confidentiality was maintained. We retrospectively reviewed the data from 1,571 consecutive patients with solid PNs who joined the management database of PNs and lung cancer in the First Affiliated Hospital of Chongqing Medical University from 1 January 2019 to 30 April 2022. The patients were divided into three groups: the benign group, the Ia-stage lung cancer group, and the T1-stage lung cancer metastasis group. The benign group can be further divided into the pathological benign group and the follow-up benign group.
The inclusion criteria were as follows: dominant nodules with a size of ≤ 30.00 mm on preoperative CT images; nodule density of solid nodules; and availability of pathological report in malignant nodule patients diagnosed by non-surgical biopsy (CT-guided transthoracic biopsy and bronchoscopy non-surgical biopsy) or surgical resection. The T1-stage lung cancer metastasis patients were confirmed by PET-CT or CT combined with ultrasound and radionuclide imaging at diagnosis, imaging follow-up within 3 months, and clinicians. Pathological benign refers to getting the confirmed pathological result while excluding non-diagnostic results such as inflammation and fibroplasia that lacked follow-up data. Follow-up benign means that the solid nodules were completely absorbed, shrunk, or unchanged within 2 years of the follow-up period. The exclusion criteria were as follows: lack of thin CT images in DICOM format, metastatic cancer, and recurrence within 2 years post-operation in the Ia-stage lung cancer group. Finally, 689 patients were enrolled and divided into the training group and the testing group randomized for 8:2, which included the benign group (n = 333), the Ia-stage lung cancer group (n = 196), and the T1-stage lung cancer with metastasis group (n = 160) (Figure 1).
2.2. Data collection
The clinical characteristics included age, gender, smoking status, history of cancer, family history of cancer, images of PNs contained size and location, histological type, lung cancer staging, distribution of metastases, and confirmed diagnosis method collected retrospectively (Table 1 and Figure 2).
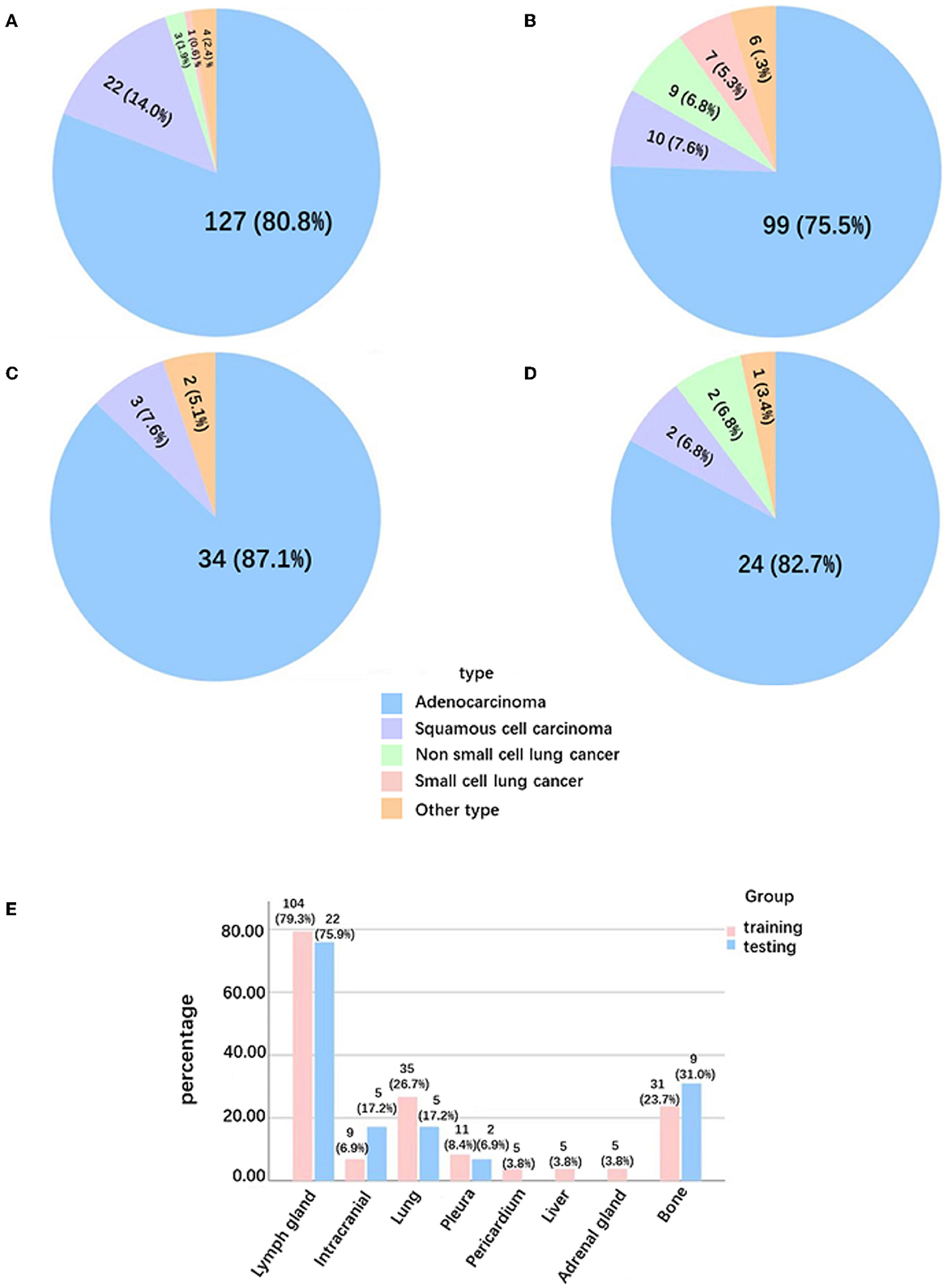
Figure 2. Pathological type and distribution of metastases in solid lung cancer nodules. (A) Pathological type of Ia-stage lung cancer patients in the training group. (B) Pathological type of T1-stage lung cancer patients with metastasis in the training group. (C) Pathological type of Ia-stage lung cancer patients in the testing group. (D) Pathological type of T1-stage lung cancer patients with metastasis in the testing group. (E) Distribution of metastases in T1-stage lung cancer patients with metastasis.
2.3. CT scanning parameters
All patients underwent chest CT scanning in our Department of Radiology before receiving a confirmed diagnosis using the following scanners: SOMATOM Perspective (Siemens Healthineers, Erlangen, Germany), SOMATOM Definition Flash (Siemens Healthineers, Erlangen, Germany), or Discovery CT750 HD (GE Healthcare, Milwaukee, WI, USA) with the following parameters: 120 kVp; 80 mAs; pitch 1.0; and collimation 0.6 mm, respectively. All imaging data were reconstructed using a medium sharp reconstruction algorithm with a thickness of ≤ 1 mm. CT scans were obtained from all patients in the supine position at full inspiration.
2.4. Development of the deep learning system
Gives a visualization of the proposed deep learning system. This section is organized into two parts: data preprocessing and classification network (Figure 3).
2.5. Data preprocessing
Before being fed into the neural network, each data sample is preprocessed using the following steps:
1. Resample the CT volume Xvol into the spacing of 1 mm * 1 mm * 1 mm using trilinear interpolation and obtain the normalized volume Xnorm<uscore> vol;
2. Crop a 64 mm * 64 mm * 64 mm patch Xnorm<uscore>patch around the center of each nodule from the resampled CT volume;
3. Clip HU values of Xnorm<uscore>patch into [−1,000, 400] (equivalent to torch.clamp(x_norm_patch, −1,000, 400) or numpy.clip [x_norm_patch, −1,000, 400)];
4. Apply HU value min–max normalization, normalize HU values into [0, 1], and obtain the final output of data preprocessing .
The resampling step ensures isotropy along each dimension of the 3D nodule patch, which facilitates training of the 3D convolutional neural network. HU clipping and normalization filter out irrelevant noises in the CT patch and stabilize the training of the deep learning model.
2.6. Classification network
We used a 3D ResNet18 (41, 42) as the classification network in our experiments. The input of the model is a preprocessed 3D patch, together with the nodule segmentation obtained from the segmentation system developed by Dianei Technology, Shanghai in a previous study (27). The model outputs the classification probabilities of the three following probabilities: nodules with metastasis, phase 1A nodules, and benign nodules.
We train the deep learning model for 100 epochs using the AdamW (43) optimizer with a batch size of 64. The learning rate is adjusted following a cosine learning rate decay schedule (44) from 10−3 to 10−6. Hyperparameters are selected according to the network performances of 3-fold cross-validation on the training and validation datasets. The split of cross-validation is done randomly and stratified using the nodule classification labels. To alleviate overfitting caused by the limited dataset size and to improve the generalization performance of the model various data augmentation techniques were adopted during training. A full list of data augmentation is as follows:
1. Random Gaussian noise;
2. Random crop near the center;
3. Random flipping and transposing;
4. Mixup (45).
With a single forward pass and an input 3D patch of 64 mm * 64 mm * 64 mm from the CT scan, the trained network can predict the three-class probability together with the nodule mask. The nodule is classified as the category with highest probability.
2.7. Testing the performance of deep learning in the diagnosis of solid PNs
To test the effectiveness of our proposed method in predicting malignancy and metastasis of solid PNs, we evaluated its performances using three-class accuracy and AUC scores on predictions of nodule malignancy (benign vs. malignant+metastasis) and metastasis (benign+malignant vs. metastasis). Furthermore, we performed subgroup analysis in the following settings:
1. Total: In this setting, we evaluated our model on the entire dataset;
2. Follow-up benign: In this setting, we performed evaluations on all malignant nodules and progress-free benign nodules during follow-up visits. This setting is considered easier since the diagnosis evidence is more obvious, where we expect higher performances;
3. Pathological benign: In this setting, we included only benign nodules confirmed by pathological results and also all malignant nodules. Compared with the follow-up benign setting, this setting can be regarded as a differential diagnosis, which is more challenging for both deep learning models and human experts.
2.8. Observer studies
To compare the performance of the deep learning system with that of humans, an observer study of four clinicians was conducted. The specialization and years of experience of these clinicians are given in Table 2. All 134 cases in the test dataset were included in the observer studies. We evaluated the performances of both the deep learning model and clinicians using the F1 score to balance both precision and sensitivity. Meanwhile, we analyzed the inter-rater consistency among human experts in diagnosing solid nodules using Cohen's kappa scores.
2.9. Diagnosis accuracy of human–computer collaboration
In the observer studies, we conducted experiments to investigate whether human–computer collaboration can further enhance diagnostic accuracy. In the task of malignancy diagnosis, we combined human expert opinions with deep learning model predictions by a simple strategy, adding binary clinician diagnoses into model prediction probabilities with different weights:
Where pHC is the human–computer collaboration probability, wH ∈ [0, 1] is the weight of human diagnosis, 1H is the binary malignancy prediction from clinicians, and pC ∈ [0, 1] is the malignancy probability given by the deep learning model.
2.10. Statistical analysis
SPSS 25.0 software was used for statistics, and the sorted data were imported into SPSS for weighted data analysis. The independent sample t-test in the software analysis list was used for the P-value analysis of age and diameter data, and Pearson's χ2 test in the software analysis list was used for the P-value analysis of other data. A P-value of < 0.5 was defined as statistically significant.
3. Results
3.1. Clinical and pathological characteristics
A total of 333 benign nodule patients, 196 Ia-stage lung cancer patients, and 160 T1-stage lung cancer metastasis patients were enrolled. The average age was 54.82 ± 12.13 years, 65.26 ± 9.78 years, and 64.59 ± 9.48 years, respectively. The diameter of nodules was 12.53 ± 6.36 mm, 16.64 ± 5.62 mm, and 19.83 ± 5.84 mm in the benign nodules group, Ia stage group, and T1-stage metastasis groups, respectively (Appendix 1). The three groups were further divided into a training and testing group, randomized for 8:2. There was no significant difference in the clinical data between the training set and the testing set, as shown in Table 1 (p > 0.05). The metastases sites of T1-stage lung cancer in the training group and testing group were mainly distributed in the lymph nodes (79.3%,75.9%), lung (26.7%, 17.2%), bone (23.7%, 31%), pleura (8.4%, 6.9%), adrenal gland (3.8%, 0%), and brain (6.9%, 17.2%) (Figure 2).
3.2. Performance of deep learning in the diagnosis of solid PNs
Since our dataset includes both benign nodules confirmed by pathological results and those diagnosed as benign via non-progression during follow-up visits, we evaluated our deep learning model accordingly. Specifically, we reported model performances on the following three settings:
1. Total: In this setting, we evaluated our model on the entire dataset.
2. Follow-up benign: In this setting, we performed evaluations on all malignant nodules and progress-free benign nodules during follow-up visits. This setting is considered easier since the diagnostic evidence is more obvious, and we expect higher performance.
3. Pathological benign: In this setting, we included only benign nodules confirmed by pathological results and all malignant nodules. Compared with the follow-up benign setting, this setting is more challenging for both deep learning models and human experts.
Overall, our deep learning model achieved a three-class accuracy of 64.93% and AUC scores of 80.37% and 86.44% in malignancy and metastasis prediction, respectively. For the follow-up benign subset, our model reached an even higher three-class accuracy of 72.53%, a malignancy prediction AUC of 93.48%, and a metastasis prediction AUC of 87.93%. In terms of the pathological benign group, which is considered difficult to diagnose, our model achieved a decent three-class accuracy of 59.46% and scored 73.36% and 83.18% on the malignancy and metastasis prediction AUCs, respectively (Table 3).
3.3. Benchmarking deep learning against clinicians for malignancy prediction performance
The deep learning method outperformed the junior respiratory clinician (Clinician A) and the respiratory clinician with 5 years of experience (Clinician B) in the overall evaluation and both subgroups. Our proposed model was on par with the senior respiratory clinician (Clinician C), with slightly inferior performance on the entire dataset (77.11% vs. 78.08%) and better performances in both subgroups (93.43% vs. 89.76% in the follow-up group and 79.50% vs. 79.17% in the pathological group). Nevertheless, our proposed model fell short when compared with the senior radiologist (Clinician D), but not by a large margin. Such performances show that the deep learning model is promising when it comes to facilitating decisions similar to human clinicians in the complex task of solid nodule diagnosis (Table 4 and Figure 4). In contrast, human clinicians behave inconsistently, with a highest Cohen's kappa score of 0.4306 (Table 5). The low inter-rater consistency shows that our proposed deep learning model has better diagnostic stability in such scenarios.
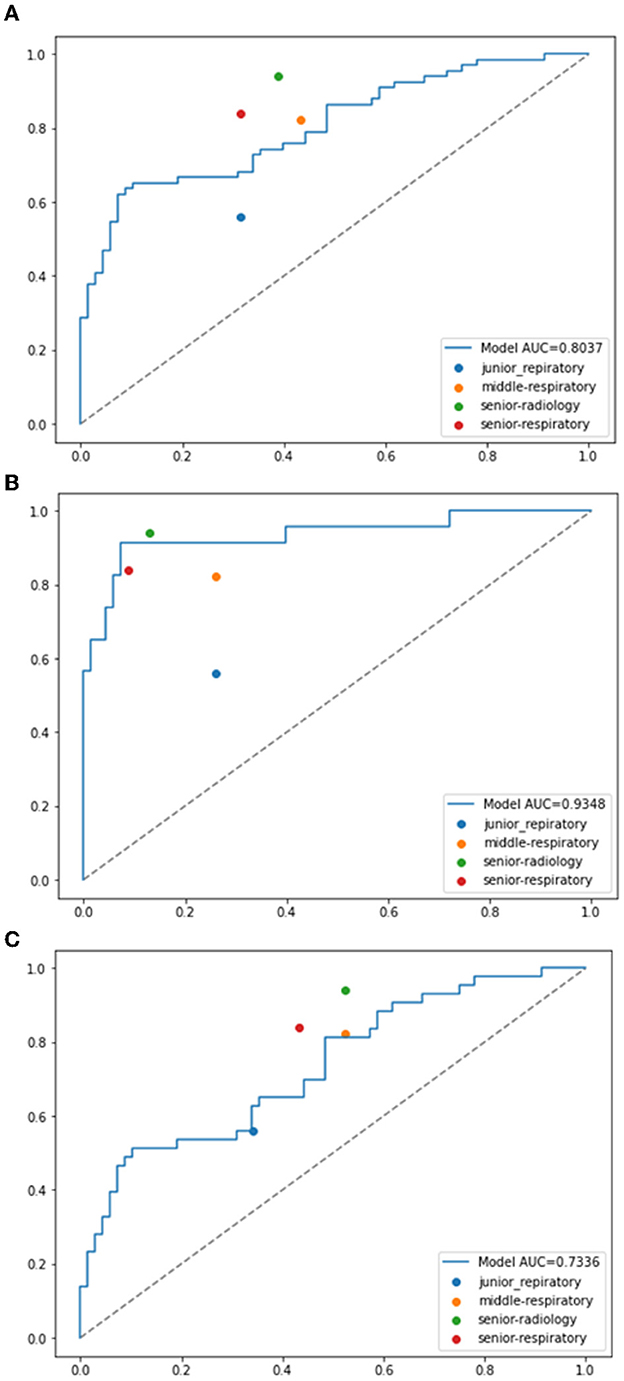
Figure 4. ROC curves of the proposed model compared with the performances of clinicians. (A) Malignancy prediction performances compared with total benign nodules data. (B) Malignancy prediction performances compared with the follow-up benign nodules data. (C) Malignancy prediction performances compared with the pathological benign nodules data.
3.4. Human–computer collaboration
The results of combining human and computer diagnoses with different wH ∈ [0, 1] with steps of 0.01, where wH controls the weight of the human experts in collaboration. We found that Clinicians B, C, and D improved the AUC score of the deep learning model regardless of the value of wH. Clinician D increased the model AUC from 80.37% to 88.73% at most. Empirically, we observed that wH = 0.22 improved the average AUC score the most. Under this hyperparameter setting, the model AUC is increased to 82.60%, 84.83%, 85.54%, and 88.00% when combined with Clinicians A, B, C, and D, respectively. Our human–computer collaboration experiments show that the proposed model becomes more accurate when working with humans, demonstrating its great potential in clinical practice (Figure 5).
4. Discussion
The best clinical management of PNs requires the evaluation of the probability of malignancy, which determines the most cost-effective diagnostic and therapeutic strategies. Previous studies on the diagnosis of solid PNs mainly focused on using radiomics models or nomograms or included only pathologically benign nodules (14, 15, 29) and did not take advantage of the deep learning technique. Heuvelmans et al. trained and validated a lung cancer prediction convolutional neural network on an independent dataset of small-to-intermediate nodules sized 5–15 mm and demonstrated its excellent performance in identifying benign nodules (46). In their research, benign nodules were determined by screenings and follow-ups until 7 years after baseline in the National Lung Screening Trial as well as solid nodules (46). Moreover, larger benign solid PNs are usually characterized by overlapping imaging features and are easily misdiagnosed and overtreated. Our study provided evidence that deep learning methods based on CT images of the primary lesions can be used to predict the malignancy of solid PNs (size ≤ 30 mm) and performed better than two junior or middle-level clinicians, only slightly inferior to the senior radiologist. In the follow-up benign subset, our model reached an even higher three-class accuracy of 72.53% and a malignancy prediction AUC of 93.48%. In terms of the pathological benign group, which is considered difficult to diagnose, our model achieved a decent three-class accuracy of 59.46% and scored 73.36% on the malignancy prediction AUC. Our human–computer collaboration experiments show that the proposed model becomes more accurate when working with humans, demonstrating its great potential when used in clinical practice. Therefore, the proposed deep learning method can accurately diagnose solid PNs, even if they are indeterminate solid lung nodules, and has demonstrated improved performance upon working in tandem with human experts.
At present, it is difficult to detect and predict the metastasis of T1-stage lung cancer until it has already developed to a certain stage (25), but it is critical to match patients with appropriate individualized therapy strategies and predicting prognoses. Numerous studies have reported using radiomics features, deep learning, or other methods to predict lymph node metastasis, but not the M staging of lung cancer. Beck et al. reported that the deep cubical nodule transfer learning (CUBIT) algorithm, using transfer learning and a 3D convolutional neural network (CNN) based on CT scan images, can accurately predict LVI or nodal involvement in primary non-small cell lung cancer (NSCLC) (36). Nie et al. reported that a radiomics nomogram incorporating the Rad-score and clinical and PET/CT parameters shows favorable predictive efficacy for lymph vascular invasion status in lung adenocarcinoma (47). Zhang et al. established a PET/CT nomogram based on the metabolic information (SUVmax) and structural information (radiomics features) of lymph nodes for preoperative quantitative estimation of lymph node metastasis (48). Tau et al. used convolutional neural networks to predict the nodal and distant metastatic potential of newly diagnosed NSCLC on FDG PET images (49), but the authors did not specifically identify solid PNs. Tian et al. reported that the radiomics features of pretherapy CT images may be used as predictors of distant metastasis, but there were only 43 cases of solid lung cancer nodules, and only three patients had metastases in their study (25). In this research, we collected a cohort of 689 patients with solid PNs and trained a 3D CNN to predict the local or distant metastasis of nodules. On a held-out testing set of 134 cases, the deep learning approach achieved an AUC score of 86.44% for metastasis prediction. The method employed in this study can be used to predict or diagnose the metastasis of T1-stage lung cancer nodules based on CT imaging. When we are able to better evaluate the characteristics of these nodules, clinicians will have a greater chance of identifying highly aggressive lung cancer at its earliest stages, making treatment planning and patient stratification viable for everyone.
5. Conclusion
Although this proposed model shows great promise and is able to compete with senior clinicians in the solid nodule diagnosis task, there are limitations worth mentioning. First, not all patients in the metastasis group had pathological results for the metastatic sites. Because most of them were local or late-stage lung cancer patients, metastasis was mainly confirmed by non-invasive systemic screening, clinician experience, or follow-up in ethics. Second, this was a single-center retrospective study with a relatively small sample size. However, because of the difficulty of medical data collection, it is the largest sample size reported in T1-stage solid lung cancer patients with metastases, according to the literature. Multicenter studies with larger datasets can be validated in the future. In addition, only one single CT scan is included for each patient in our experiments, while in practice clinicians usually take multiple follow-up CT scans into account. The next step is to design a prospective study in which follow-up CT sequences can be added to make the best use of information from multiple time points and to improve diagnostic accuracy (50, 51). Additionally, our human–computer collaboration experiment settings are not close enough to real-world clinical settings. This is due to the labor intensiveness of having all four clinicians carry out the diagnosis once again. In our future research, we will experiment with human experts diagnosing with computer assistance in real-world scenarios. However, we argue that our human–computer collaboration method has its own benefit since it draws a frontier of possible collaboration results, demonstrating that human–computer collaboration is a bonus under various levels of human trust. Our approach is also robust against human variance, which is high, as shown in our inter-rater consistency analysis. Finally, the current method focuses on modeling only the CT modality, whereas ideally, clinicians use a variety of information, such as smoking history and multiomics information (52, 53) to better estimate the metastasis and malignancy of solid PNs. Aggregating such information in our modeling may further boost its diagnostic performance.
In summary, this study provided evidence that the proposed deep learning method extracted from CT images of primary lesions can accurately diagnose the malignancy of solid PNs and its performance improves when collaborating with human experts. To the best of our knowledge, this is the first study to use deep learning with pretherapy CT images of primary tumors to judge N and M staging in T1 solid lung cancer nodules, which could help to provide optimal care for these patients. The prediction of metastasis in T1-stage lung cancer using CT images has become simple yet accurate through deep learning methods.
Data availability statement
The datasets presented in this article are not readily available because of ethical and copyright restrictions. Requests to access the datasets should be directed to the corresponding author.
Ethics statement
The studies involving human participants were reviewed and approved by the Ethics Committee of the First Affiliated Hospital of Chongqing Medical University. Written informed consent to participate in this study was provided by the participants' legal guardian/next of kin.
Author contributions
LY, JY, and SG designed this study. JM, MA, WL, ZO, and JH collected the data. LY and HD performed the statistical analysis. JY, KK, and JL developed the deep learning model. LY, KK, JL, JY, and JM wrote the manuscript. LY, MA, and HD performed the procedures. All authors read and approved the final version of the manuscript.
Funding
The Program for National Natural Science Foundation of China (82203181), the Chongqing Science and Technology Commission, the Chongqing People's Municipal Government (cstc2019jscxmsxmX0184), the Senior Medical Talents of Chongqing for Young and Middle-aged (0202czzx2108: 2020GDRC029), the Youth Innovation in Future Medicine, the Chongqing Medical University (W0102), and the Discipline Innovation Fund of Discipline Cultivation Project from the First Affiliated Hospital of Chongqing Medical University (XKST134) supported the conduct of the study but had no involvement in the study design, implementation, or manuscript writing.
Acknowledgments
We appreciate the work of all healthcare workers involved in the diagnosis and treatment of patients in the First Affiliated Hospital of Chongqing Medical University. We are grateful to all patients involved in the study.
Conflict of interest
KK, JL, and JY were employed by company Dianei Technology.
The remaining authors declare that the research was conducted in the absence of any commercial or financial relationships that could be construed as a potential conflict of interest.
Publisher's note
All claims expressed in this article are solely those of the authors and do not necessarily represent those of their affiliated organizations, or those of the publisher, the editors and the reviewers. Any product that may be evaluated in this article, or claim that may be made by its manufacturer, is not guaranteed or endorsed by the publisher.
References
1. Siegel RL, Miller KD, Fuchs HE, Jemal A. Cancer statistics, 2022. CA Cancer J Clin. (2022) 72:7–33. doi: 10.3322/caac.21708
2. Giaquinto AN, Miller KD, Tossas KY, Winn RA, Jemal A, Siegel RL. Cancer statistics for African American/Black people 2022. CA Cancer J Clin. (2022) 72:202–29. doi: 10.3322/caac.21718
3. Xia C, Dong X, Li H, Cao M, Sun D, He S, et al. Cancer statistics in China and United States, 2022: profiles, trends, and determinants. Chin Med J (Engl). (2022) 135:584–90. doi: 10.1097/CM9.0000000000002108
4. Lackey A, Donington JS. Surgical management of lung cancer. Semin Intervent Radiol. (2013) 30:133–40. doi: 10.1055/s-0033-1342954
5. Oudkerk M, Liu S, Heuvelmans MA, Walter JE, Field JK. Lung cancer LDCT screening and mortality reduction - evidence, pitfalls and future perspectives. Nat Rev Clin Oncol. (2021) 18:135–51. doi: 10.1038/s41571-020-00432-6
6. McWilliams A, Tammemagi MC, Mayo JR, Roberts H, Liu G, Soghrati K, et al. Probability of cancer in pulmonary nodules detected on first screening CT. N Engl J Med. (2013) 369:910–9. doi: 10.1056/NEJMoa1214726
7. Mazzone PJ, Lam L. Evaluating the Patient With a Pulmonary Nodule: A Review. Jama. (2022) 327:264–73. doi: 10.1001/jama.2021.24287
8. Ye T, Deng L, Wang S, Xiang J, Zhang Y, Hu H, et al. Lung adenocarcinomas manifesting as radiological part-solid nodules define a special clinical subtype. J Thorac Oncol. (2019) 14:617–27. doi: 10.1016/j.jtho.2018.12.030
9. Berry MF, Gao R, Kunder CA, Backhus L, Khuong A, Kadoch M, et al. Presence of even a small ground-glass component in lung adenocarcinoma predicts better survival. Clin Lung Cancer. (2018) 19:e47–51. doi: 10.1016/j.cllc.2017.06.020
10. Ujiie H, Kadota K, Chaft JE, Buitrago D, Sima CS, Lee MC, et al. Solid predominant histologic subtype in resected stage i lung adenocarcinoma is an independent predictor of early, extrathoracic, multisite recurrence and of poor postrecurrence survival. J Clin Oncol. (2015) 33:2877–84. doi: 10.1200/JCO.2015.60.9818
11. Rami-Porta R, Bolejack V, Crowley J, Ball D, Kim J, Lyons G, et al. The IASLC lung cancer staging project: proposals for the revisions of the T descriptors in the forthcoming eighth edition of the TNM classification for lung cancer. J Thorac Oncol. (2015) 10:990–1003. doi: 10.1097/JTO.0000000000000559
12. Diederich S, Wormanns D, Semik M, Thomas M, Lenzen H, Roos N, et al. Screening for early lung cancer with low-dose spiral CT: prevalence in 817 asymptomatic smokers. Radiology. (2002) 222:773–81. doi: 10.1148/radiol.2223010490
13. Truong MT, Ko JP, Rossi SE, Rossi I, Viswanathan C, Bruzzi JF, et al. Update in the evaluation of the solitary pulmonary nodule. Radiographics. (2014) 34:1658–79. doi: 10.1148/rg.346130092
14. She Y, Zhao L, Dai C, Ren Y, Jiang G, Xie H, et al. Development and validation of a nomogram to estimate the pretest probability of cancer in Chinese patients with solid solitary pulmonary nodules: a multi-institutional study. J Surg Oncol. (2017) 116:756–62. doi: 10.1002/jso.24704
15. Liu J, Xu H, Qing H, Li Y, Yang X, He C, et al. Comparison of radiomic models based on low-dose and standard-dose CT for prediction of adenocarcinomas and benign lesions in solid pulmonary nodules. Front Oncol. (2020) 10:634298. doi: 10.3389/fonc.2020.634298
16. Aberle DR, Adams AM, Berg CD, Black WC, Clapp JD, Fagerstrom RM, et al. Reduced lung-cancer mortality with low-dose computed tomographic screening. N Engl J Med. (2011) 365:395–409. doi: 10.1056/NEJMoa1102873
17. Zhihua Kong CM. Value of thoracoscopic surgery in the treatment of solitary pulmonary nodules with a history of extrapulmonary malignancy. Ji Lin Medical. (2020) 6:1425–6.
18. Liu Y, Chen M, Guo C, Zhong W, Ye Q, Zhao J, et al. Clinical-radiological-pathological characteristics of 297 cases of surgical pathology confirmed benign pulmonary lesions in which malignancy could not be excluded in preoperative assessment: a retrospective cohort analysis in a single chinese hospital. Zhongguo Fei Ai Za Zhi. (2020) 23:792–9.
19. Bai Q, Yang X, Li Q, Chen W, Tian H, Lian R, et al. Metastatic tumor cell-specific FABP7 promotes NSCLC metastasis via inhibiting β-catenin degradation. Cells. (2022) 11:805. doi: 10.3390/cells11050805
20. Hanahan D. Hallmarks of cancer: new dimensions. Cancer Discov. (2022) 12:31–46. doi: 10.1158/2159-8290.CD-21-1059
21. DuComb EA, Tonelli BA, Tuo Y, Cole BF, Mori V, Bates JHT, et al. Evidence for expanding invasive mediastinal staging for peripheral T1 lung tumors. Chest. (2020) 158:2192–9. doi: 10.1016/j.chest.2020.05.607
22. Dickson JL, Horst C, Nair A, Tisi S, Prendecki R, Janes SM. Hesitancy around low-dose CT screening for lung cancer. Ann Oncol. (2022) 33:34–41. doi: 10.1016/j.annonc.2021.09.008
23. Taylor SA, Mallett S, Ball S, Beare S, Bhatnagar G, Bhowmik A, et al. Diagnostic accuracy of whole-body MRI versus standard imaging pathways for metastatic disease in newly diagnosed non-small-cell lung cancer: the prospective Streamline L trial. Lancet Respir Med. (2019) 7:523–32. doi: 10.1016/S2213-2600(19)30090-6
24. Hwang KE, Oh SJ, Park C, Jeon SJ, Lee JM, Cha BK, et al. Computed tomography morphologic features of pulmonary adenocarcinoma with brain/bone metastasis. Korean J Intern Med. (2018) 33:340–6. doi: 10.3904/kjim.2016.134
25. Zhou H, Dong D, Chen B, Fang M, Cheng Y, Gan Y, et al. Diagnosis of distant metastasis of lung cancer: based on clinical and radiomic features. Transl Oncol. (2018) 11:31–6. doi: 10.1016/j.tranon.2017.10.010
26. Bi WL, Hosny A, Schabath MB, Giger ML, Birkbak NJ, Mehrtash A, et al. Artificial intelligence in cancer imaging: clinical challenges and applications. CA Cancer J Clin. (2019) 69:127–57. doi: 10.3322/caac.21552
27. Zhao W, Yang J, Sun Y, Li C, Wu W, Jin L, et al. 3D deep learning from CT scans predicts tumor invasiveness of subcentimeter pulmonary adenocarcinomas. Cancer Res. (2018) 78:6881–9. doi: 10.1158/0008-5472.CAN-18-0696
28. Xie Y, Xia Y, Zhang J, Song Y, Feng D, Fulham M, et al. Knowledge-based collaborative deep learning for benign-malignant lung nodule classification on chest CT. IEEE Trans Med Imaging. (2019) 38:991–1004. doi: 10.1109/TMI.2018.2876510
29. Duan XQ, Wang XL, Zhang LF, Liu XZ, Zhang WW, Liu YH, et al. Establishment and validation of a prediction model for the probability of malignancy in solid solitary pulmonary nodules in northwest China. J Surg Oncol. (2021) 123:1134–43. doi: 10.1002/jso.26356
30. Zhang J, Li C, Yin Y, Zhang J, Grzegorzek M. Applications of artificial neural networks in microorganism image analysis: a comprehensive review from conventional multilayer perceptron to popular convolutional neural network and potential visual transformer. Artif Intell Rev. (2023) 56:1013–70. doi: 10.1007/s10462-022-10192-7
31. Zou W, Qi X, Zhou W, Sun M, Sun Z, Shan C. Graph flow: cross-layer graph flow distillation for dual efficient medical image segmentation. IEEE Trans Med Imaging. (2022) 3:4459. doi: 10.1109/TMI.2022.3224459
32. Yamashita R, Nishio M, Do RKG, Togashi K. Convolutional neural networks: an overview and application in radiology. Insights Imaging. (2018) 9:611–29. doi: 10.1007/s13244-018-0639-9
33. Liu W, Li C, Xu N, Jiang T, Rahaman M, Sun H, et al. CVM-cervix: a hybrid cervical pap-smear image classification framework using CNN, visual transformer and multilayer perceptron. Pattern Recognit. (2022) 130:108829. doi: 10.1016/j.patcog.2022.108829
34. Zhang J, Li C, Kosov S, Grzegorzek M, Shirahama K, Jiang T, et al. LCU-net: a novel low-cost U-net for environmental microorganism image segmentation. Pattern Recognit. (2021) 115:107885. doi: 10.1016/j.patcog.2021.107885
35. Zhao X, Wang X, Xia W, Li Q, Zhou L, Li Q, et al. A cross-modal 3D deep learning for accurate lymph node metastasis prediction in clinical stage T1 lung adenocarcinoma. Lung Cancer. (2020) 145:10–7. doi: 10.1016/j.lungcan.2020.04.014
36. Beck KS, Gil B, Na SJ, Hong JH, Chun SH, An HJ, et al. DeepCUBIT: predicting lymphovascular invasion or pathological lymph node involvement of clinical T1 stage non-small cell lung cancer on chest CT scan using deep cubical nodule transfer learning algorithm. Front Oncol. (2021) 11:661244. doi: 10.3389/fonc.2021.661244
37. Ran J, Cao R, Cai J, Yu T, Zhao D, Wang Z. Development and validation of a nomogram for preoperative prediction of lymph node metastasis in lung adenocarcinoma based on radiomics signature and deep learning signature. Front Oncol. (2021) 11:585942. doi: 10.3389/fonc.2021.585942
38. Cong M, Feng H, Ren JL, Xu Q, Cong L, Hou Z, et al. Development of a predictive radiomics model for lymph node metastases in pre-surgical CT-based stage IA non-small cell lung cancer. Lung Cancer. (2020) 139:73–9. doi: 10.1016/j.lungcan.2019.11.003
39. Hu D, Li S, Zhang H, Wu N, Lu X. Using natural language processing and machine learning to preoperatively predict lymph node metastasis for non-small cell lung cancer with electronic medical records: development and validation study. JMIR Med Inform. (2022) 10:e35475. doi: 10.2196/35475
40. Tian Y, He Y, Li X, Liu X. Novel nomograms to predict lymph node metastasis and distant metastasis in resected patients with early-stage non-small cell lung cancer. Ann Palliat Med. (2021) 10:2548–66. doi: 10.21037/apm-20-1756
41. Niethammer M, Kwitt R, Vialard FX. Metric learning for image registration. Proc IEEE Comput Soc Conf Comput Vis Pattern Recognit. (2019) 2019:8455–64. doi: 10.1109/CVPR.2019.00866
42. Tran D, Wang H, Torresani L, Ray J, LeCun Y, Paluri M, editor. A closer look at spatiotemporal convolutions for action recognition. 2018 IEEE/CVF Conference on Computer Vision and Pattern Recognition. (2018). doi: 10.1109/CVPR.2018.00675
43. Loshchilov I, Hutter F. Decoupled Weight Decay Regularization. ICLR. (2019). doi: 10.48550/arXiv.1711.05101
44. Loshchilov I, Hutter F. SGDR: Stochastic Gradient Descent with Warm Restarts. ICLR. (2017). doi: 10.48550/arXiv.1608.03983
45. Zhang H, Cissé M, Dauphin Y, Lopez-Paz D. Mixup: Beyond Empirical Risk Minimization. ICLR. (2018).
46. Heuvelmans MA, van Ooijen PMA, Ather S, Silva CF, Han D, Heussel CP, et al. Lung cancer prediction by Deep Learning to identify benign lung nodules. Lung Cancer. (2021) 154:1–4. doi: 10.1016/j.lungcan.2021.01.027
47. Nie P, Yang G, Wang N, Yan L, Miao W, Duan Y, et al. Additional value of metabolic parameters to PET/CT-based radiomics nomogram in predicting lymphovascular invasion and outcome in lung adenocarcinoma. Eur J Nucl Med Mol Imaging. (2021) 48:217–30. doi: 10.1007/s00259-020-04747-5
48. Xie Y, Zhao H, Guo Y, Meng F, Liu X, Zhang Y, et al. A PET/CT nomogram incorporating SUVmax and CT radiomics for preoperative nodal staging in non-small cell lung cancer. Eur Radiol. (2021) 31:6030–8. doi: 10.1007/s00330-020-07624-9
49. Tau N, Stundzia A, Yasufuku K, Hussey D, Metser U. Convolutional neural networks in predicting nodal and distant metastatic potential of newly diagnosed non-small cell lung cancer on FDG PET images. AJR Am J Roentgenol. (2020) 215:192–7. doi: 10.2214/AJR.19.22346
50. Xu Y, Hosny A, Zeleznik R, Parmar C, Coroller T, Franco I, et al. deep learning predicts lung cancer treatment response from serial medical imaging. Clin Cancer Res. (2019) 25:3266–75. doi: 10.1158/1078-0432.CCR-18-2495
51. Zhao W, Sun Y, Kuang K, Yang J, Li G, Ni B, et al. ViSTA: a novel network improving lung adenocarcinoma invasiveness prediction from follow-up CT series. Cancers. (2022) 14:3675. doi: 10.3390/cancers14153675
52. Yang Y, Yang J, Shen L, Chen J, Xia L, Ni B, et al. A multi-omics-based serial deep learning approach to predict clinical outcomes of single-agent anti-PD-1/PD-L1 immunotherapy in advanced stage non-small-cell lung cancer. Am J Transl Res. (2021) 13:743–56.
53. Zhao W, Chen W, Li G, Lei D, Yang J, Chen Y, et al. GMILT: a novel transformer network that can noninvasively predict EGFR mutation status. IEEE Trans Neural Netw Learn Syst. (2022) 3:671. doi: 10.1109/TNNLS.2022.3190671
Appendix
Keywords: deep learning, malignancy, metastasis, solid pulmonary nodule, CT
Citation: Mu J, Kuang K, Ao M, Li W, Dai H, Ouyang Z, Li J, Huang J, Guo S, Yang J and Yang L (2023) Deep learning predicts malignancy and metastasis of solid pulmonary nodules from CT scans. Front. Med. 10:1145846. doi: 10.3389/fmed.2023.1145846
Received: 16 January 2023; Accepted: 10 April 2023;
Published: 19 May 2023.
Edited by:
Mizuho Nishio, Kyoto University, JapanCopyright © 2023 Mu, Kuang, Ao, Li, Dai, Ouyang, Li, Huang, Guo, Yang and Yang. This is an open-access article distributed under the terms of the Creative Commons Attribution License (CC BY). The use, distribution or reproduction in other forums is permitted, provided the original author(s) and the copyright owner(s) are credited and that the original publication in this journal is cited, in accordance with accepted academic practice. No use, distribution or reproduction is permitted which does not comply with these terms.
*Correspondence: Jiancheng Yang, amlhbmNoZW5nLnlhbmdAZXBmbC5jaA==; Li Yang, MjA0NTM0QGhvc3BpdGFsLmNxbXUuZWR1LmNu
†These authors have contributed equally to this work