- 1Centre Oceanogràfic de les Balears (COB-IEO), CSIC, Palma, Spain
- 2Centro Oceanográfico de Cádiz (COCAD-IEO), CSIC, Cádiz, Spain
- 3Directorate D – Sustainable Resources, Joint Research Centre (JRC), European Commission, Ispra, Italy
Climate change is affecting marine ecosystems altering the distribution and abundance of organisms, with implications for fisheries and food security. This warming-induced reshuffle in species abundance could bring threats and opportunities to the fisheries, but needs to be assessed to promote effective actions and to foster resilience. We analyzed the density and distribution patterns of deep-sea rose shrimp (Parapenaeus longirostris), as well as identified the main environmental have identified the environmental drivers shaping its habitat along the western Mediterranean (Iberian Peninsula) during the period 2001–2020. Using spatial distribution models developed concurrently with an ensemble of four Regional Climate Models (RCMs), we have projected the density of this species during the next century under two climate scenarios (RCP4.5 and RCP8.5). Bathymetry and sea bottom temperature drove the density of the species, leading to a marked northward expansion during the last two decades. Our results projected an increase in its distribution and especially in density throughout the area along the 21st century, mirroring the effect of global warming. Consequently, the most distant period (i.e. 2100s) and the warmest scenario (RCP8.5) presented also the highest densities and low internal variability of the ensemble. We discussed the power of assessing uncertainties using a RCM ensemble, particularly under complex oceanographic features, to bring robust information for an effective scientific advice to fisheries management.
1 Introduction
Marine species are spatially distributed based on their habitat preferences, with a pervasive influence of oceanographic processes and environmental conditions on the abundance and distribution of their populations (Orio et al., 2019). Expanding the knowledge on habitat preferences is especially crucial in the current context of global change, as water warming, pollution and overfishing are altering the structure and function of marine ecosystems (Barange et al., 2018; Issifu et al., 2022). Species distribution models (SDMs) combined with climate change projections is a powerful tool to assess future species response (Townhill et al., 2023). To develop reliable projections is crucial, thus derived uncertainties should be addressed and considered to provide robust and useful information to plan for the protection of marine ecosystems and the sustainable use of their living resources (Davies et al., 2023). Uncertainties derived from physical modelling could not be overlooked, particularly in marginal seas with intense atmospheric forcing and complex oceanographic dynamics such as the Mediterranean basin (Ruti et al., 2016). To address these issues, we propose here a widely used approach in climate science, an ensemble of simulations gathering similar models outputs that followed a standardized methodology.
Comprising all the raised issues, our target species here was the deep-water rose shrimp (Parapenaeus longirostris), a decapod crustacean with a wide geographic distribution along the Atlantic Ocean. Its easternmost distribution ranges from northern Iberian Peninsula to southern Angola, including the Mediterranean Sea (Sobrino et al., 2005). It is a fast-growing demersal species that inhabits muddy and sandy bottoms mainly of the deep shelf and upper slope between 100 and 400 m depth (Tom et al., 1988; Fortibuoni et al., 2010), although its general bathymetric range can encompass from 20 to 750 m (Sobrino et al., 2005). Several factors have been suggested as drivers of the abundance and distribution of this species: sea temperature and salinity (Benchoucha et al., 2008; Mingote et al., 2024), interactions between wind and marine currents (Ligas et al., 2011), biomass of primary producers (Guijarro et al., 2009) and fishing effort (Abelló et al., 2002; D’Onghia et al., 2012).
Deep-water rose shrimp is a key fisheries resource in the Mediterranean, due to its high economic value (Sbrana et al., 2006; Politou et al., 2008; Guijarro et al., 2009), being more abundant in the eastern and central basins (Ligas et al., 2011; Colloca et al., 2014). This species is included as target species in the Regulation (EU) 2019/1022 of the European Parliament and of the Council, of 20 June 2019, establishing a multiannual plan for the fisheries exploiting demersal stocks in the western Mediterranean Sea which aims to sustainably manage demersal stocks in the western Mediterranean. Its current catches are provided by bottom trawl fisheries and represent more than a quarter of total decapods crustaceans landings in the area (FAO Fishery and Aquaculture Global Statistics, 2023). Over the past few decades, landings have consistently increased, highlighting its importance in the fisheries sector. This increment can be attributed to shifts in fishing strategies (Mingote et al., 2024), with fleets targeting higher-value decapod crustaceans due to declining of fish stocks, as well as for the increase of abundance of the species in the area.
In western Mediterranean, the deep-water rose shrimp is also one of the most economically important species, with a marked role of the Alboran Sea (Figure 1) in terms of landings. Total landings of the species along the Spanish Mediterranean ranged from 65 to 600 tons per year from 2001 to 2015. However, since 2016 a noticeable rising landings trend was registered, reaching minimum of 620 tons per year and maxima of 1,750 tons in 2021 and becoming one of the most important resources for the bottom trawl fleet.
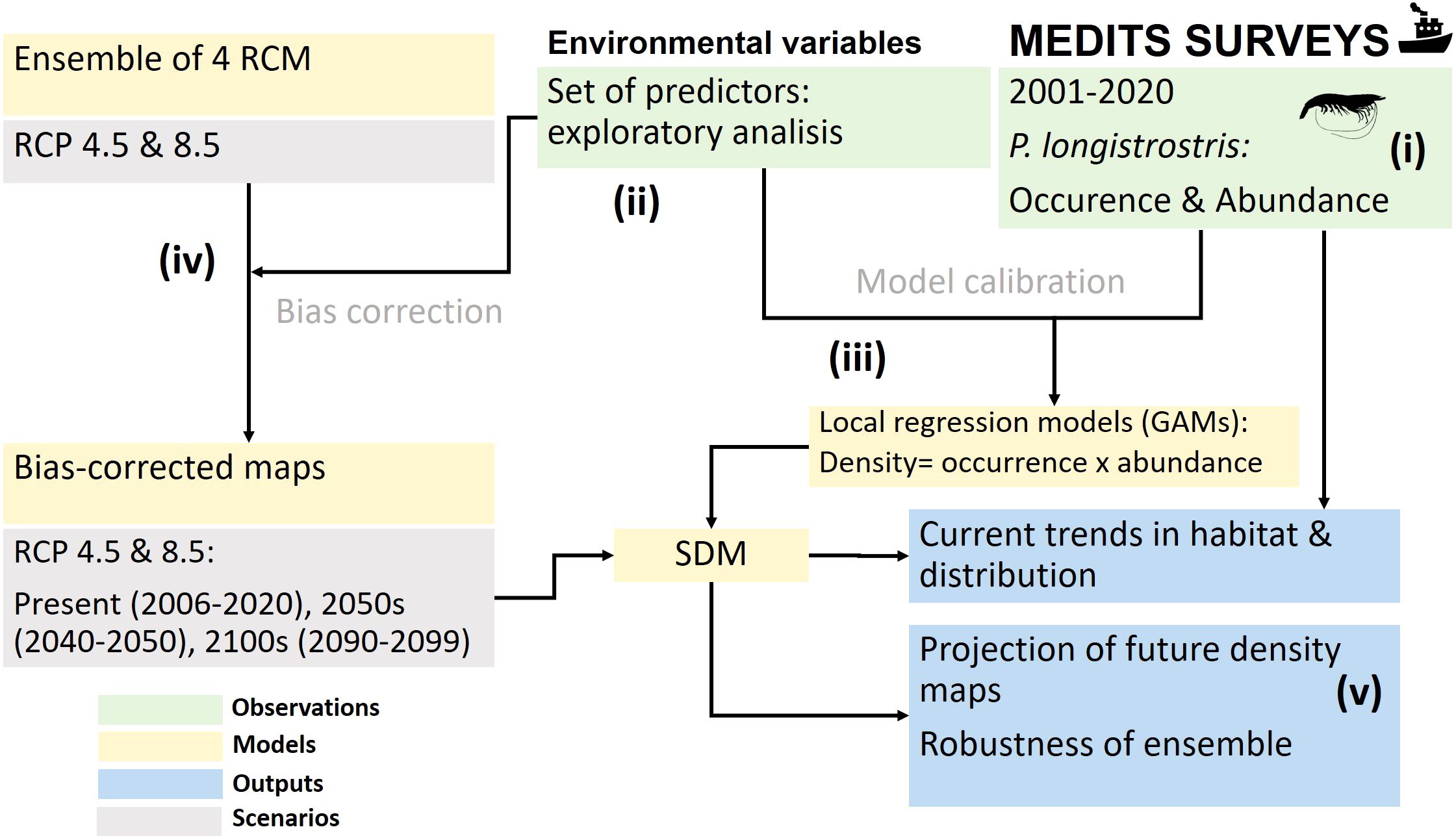
Figure 1. Procedure to derive the Spatial Distribution Models (SDM) from MEDITS surveys, local regression models: Generalized Additive Models (GAM) and projections based on future climate change scenarios using an ensemble of Regional Climate Models (RCMs). Representative Concentration Pathway (RCP) are the emissions scenarios proposed by the Intergovernmental Panel on Climate Change (IPCC): RCP4.5 and RCP8.5.
In this study we aim to analyze the recent trends in the distribution of deep-water rose shrimp along the western Mediterranean to project its future potential habitat and density during the 21st century. We started from the hypothesis that this species is strongly controlled by its habitat availability and environmental drivers here, responding to recent environmental trends. Therefore, first we disentangle the environmental drivers of the occurrence and abundance of the species, using regression models of the habitat parametrized with in situ observations, combined with oceanographic data, to explain recent trends in fisheries-independent and dependent data. Then habitat models were coupled with an ensemble of regional climate model projections for the middle and final decades of the present century, to project the distribution and density of the species and to assess the robustness of the predictions and uncertainties derived from the projection process.
2 Materials and Methods
We followed a step-by-step procedure with the following milestones (Figure 1): (i) exploration of the recent trends in the distribution range and abundance of the species, with fisheries-independent (oceanographic research surveys) and fisheries-dependent data (bottom trawl fleet landings); (ii) assessment the habitat preferences of the species, using an aprioristic set of environmental variables as potential predictors; (iii) modelling the influence of environment on its occurrence and abundance; (iv) projection of its distribution and density for the middle and final decades of the 21st century, using concurrently the main explanatory drivers and an ensemble of simulations with different greenhouse gas concentration scenarios adopted by the Intergovernmental Panel on Climate Change (IPCC); and (v) computation of the robustness of the forecasts.
2.1 Biological data: Abundance and distribution
Fisheries-independent data come from MEDITS research surveys, carried out annually during May-September along the northern Mediterranean, using an experimental bottom trawl to assess demersal fishing resources (Bertrand et al., 2002; Spedicato et al., 2019). The sampling strategy of these research surveys follows standard protocols (MEDITS group, 2017). Data used in the present study covered the period 2001–2020, the depth range 40-800 m and the Geographic Sub-Areas (GSAs) 1, 2, 5 and 6 (Figure 2A), established by the General Fisheries Commission for the Mediterranean (GFCM), which encompass the westernmost range of the Mediterranean basin (Iberian Peninsula and Balearic Islands). More information about MEDITS sampling methodology is included in Supplementary Information.
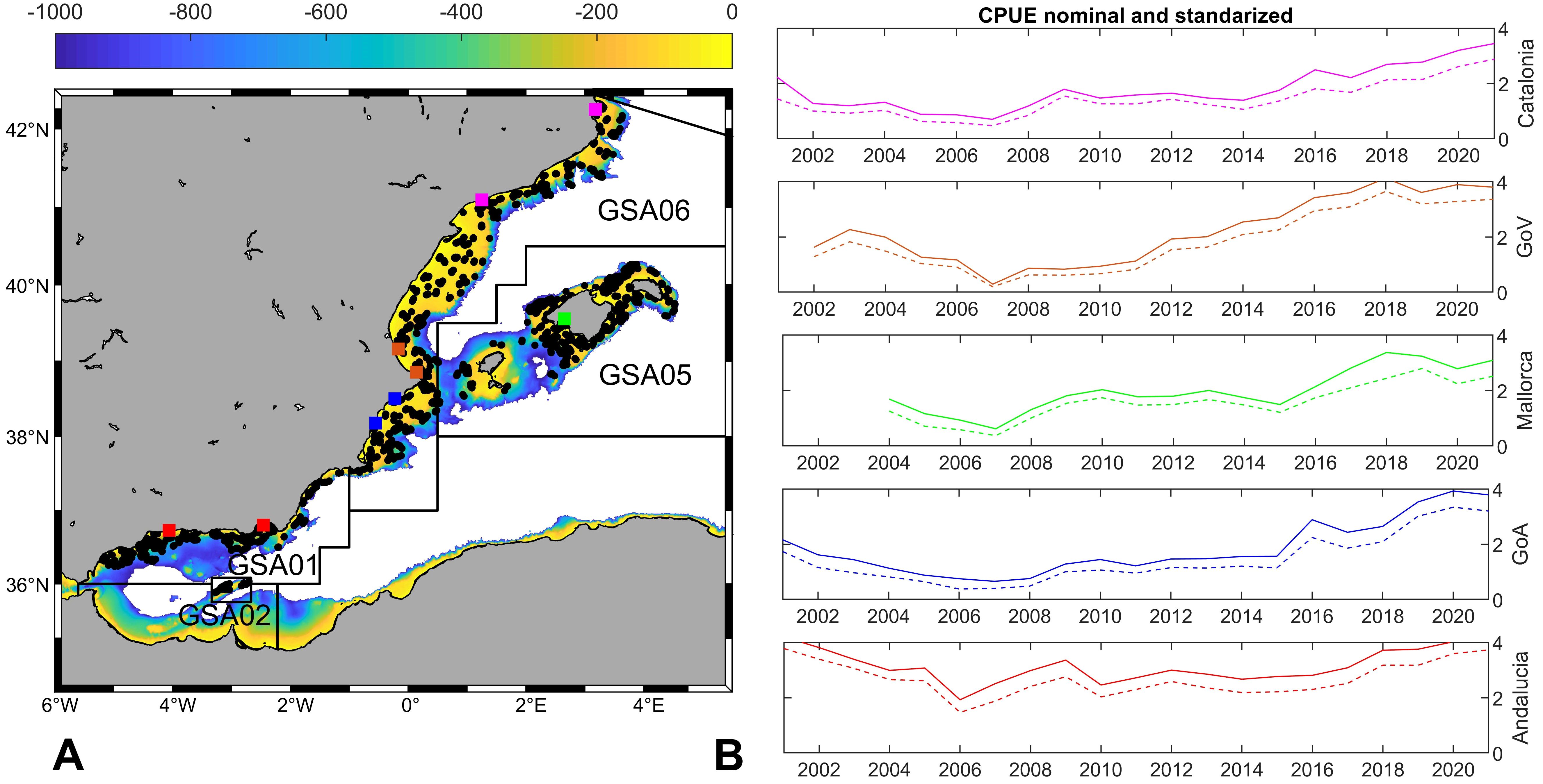
Figure 2. (A) MEDITS hauls (2001–2020) and GSAs included in this study. The colours map indicates the depth of the seabed (0-1000m). (B) CPUE nominal and standardized of landings for the main fishing harbours grouped by subregions, line colours match the location mark in (A): Catalonia (pink dots), Gulf of Valencia (brown dots), Gulf of Alicante (blue dots), Andalucia (red dots) and Mallorca (green dots).
Data on standardized density (individuals/km2) of deep-water rose shrimp were compiled from a total of 3,822 sampling hauls (1,025 species presences and 2,797 absences; Supplementary Table S1). These samples were aggregated in a 0.1x0.1° grid (ca. 100 km2) and the proportion of presence and abundance of the species per each cell and year was calculated, to avoid bias derived from varying sampling effort over time. Subsequently linear regression with time (year) was computed per each cell and only significant positive slopes (p-value<0.05) were retrieved. The calculations were done using MATLAB v.R2010b and plotted with “M Map: A mapping package for MATLAB”, v.1.4m (www.eoas.ubc.ca/~rich/map.html).
As fisheries data, landings of deep-water rose shrimp along the period 2001–2021 were used to estimate nominal and standardized Catch Per Unit of Effort (CPUE), following the methodology by Soto et al. (2002) as a proxy of its abundance. More detailed information about the methodology applied to estimate CPUE is included in Supplementary Information. The nominal CPUE has variations, mainly associated with changes in the characteristics and composition of the fishing fleet (factors related to catchability; Hilborn and Walters, 1992) and environmental factors (factors related to abundance; Sund et al., 1981). To avoid biases related to these aforementioned factors, catch indices were standardized (Beverton and Holt, 1959) by means of Generalized Linear Models (GLM; Punt et al., 2000). The GLM included the nominal CPUE as a response variable, being the explanatory variables factors associated with abundance, catchability and random error.
Fishing landings were analysed for the most important fishing harbours along the whole Mediterranean coast of Iberian Peninsula, grouped by sub-regions (Figure 2A): Catalonia (Roses and Tarragona), Gulf of Valencia (GoV: Cullera and Denia), Gulf of Alicante (GoA: Santa Pola and Villajoyosa) and Andalucía (Almería and Velez-Málaga). Data from the Fish Auction of Palma (Balearic Islands), where all the fishing landings from Mallorca harbours are commercialized, were also used. Nominal CPUE was fitted using a gamma distribution with a logistic link and explained deviations of 46.3%. Finally, the nominal CPUE was replaced by the CPUE estimated by the model, obtaining the standardized CPUE.
2.2 Environmental data
Nine environmental variables were considered as potential predictors (Table 1), including main previously suggested drivers of habitat preference for deep-water rose shrimp: temperature and salinity (Sbrana et al., 2006; Fortibuoni et al., 2010) and surface chlorophyll, as proxy of biomass of primary producers in the water column (Guijarro et al., 2009). More information about these variables is included in Supplementary Information.
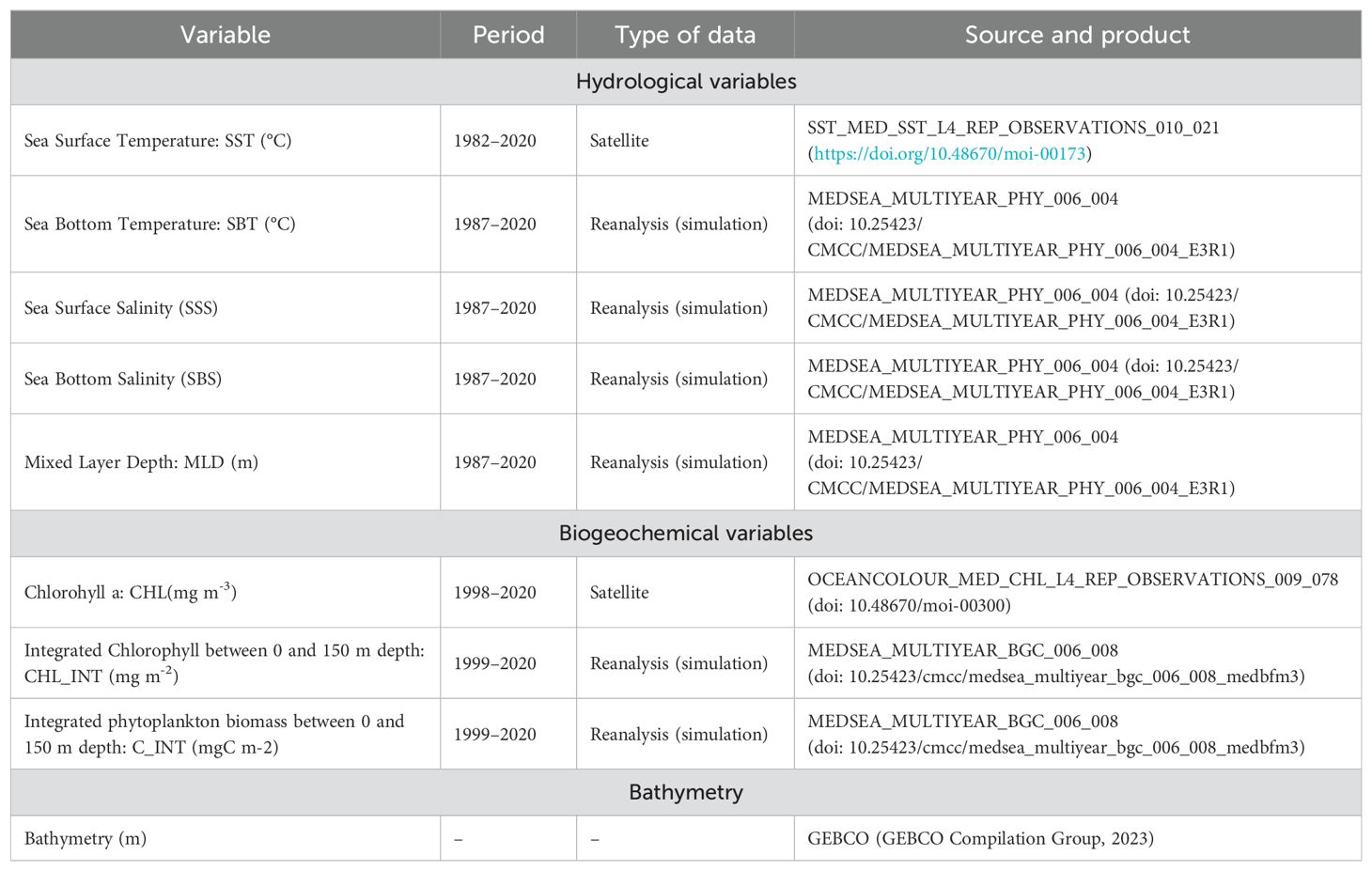
Table 1. Summary of the environmental variables considered as potential predictors to develop species distribution models of deep-water rose shrimp (Parapenaeus longirostris) in the western Mediterranean.
Data were mostly extracted from Copernicus Marine Environment Monitoring Services (CMEMS; https://marine.copernicus.eu/), which use remote sensing observations and include reanalysis products specifics for the Mediterranean (Table 1). Monthly data were averaged for the spring-summer season, matching the timing of MEDITS surveys (May-September) and all variables were reprocessed to match the same grid of 0.1x0.1° spatial resolution used for biological data (see Section 2.1). All environmental variables were explored for collinearity, outliers and missing data, before being used in the analysis and modelling (Zuur et al., 2009). Finally, values of the selected environmental variables were extracted from all MEDITS hauls locations where the species was captured, using extract function from “raster” R package (Hijmans, 2023).
2.3 Climate change projections
A set of simulations (ensemble) were gathered from four downscaled Regional Climate Models (RCMs) (Supplementary Table S2), to describe potential future conditions in the western Mediterranean, under the frame of the Coupled Model Intercomparison Project Phase 5 (CMIP5). This sub-basin presents complex oceanographic features (e.g. fronts, deep water formation) that need to be properly captured in the models, through using high-resolution simulations (Ruti et al., 2016; Schoeman et al., 2023).
We followed previous similar efforts (e.g. CERES project; Maynou et al., 2020) for the Representative Concentration Pathways (RCPs) 4.5 and 8.5 greenhouse gas concentration scenarios. RCP4.5 and RCP8.5 represent moderate and high greenhouse gas concentration, that increase radiative forcing on Earth by 4.5 and 8.5 Wm−2, respectively, at the end of the 21st century. All the simulations outputs were interpolated to the same grid (0.1x0.1°) and bathymetry. The climatological values corresponding to May-September were extracted and averaged for 2006–2020 (present, representing the current conditions), middle 21st century (2040–2050, coded as 2050s) and the end of the 21st century (2090–2100, coded as 2100s). We followed similar widely implemented approaches for projecting shifting spatial distribution of marine species (Assis et al., 2018).
Bias corrected maps were computed subtracting delta values per cell from original projection maps. Delta values are the difference between the original projection of 2006–2020 (“present”) and the same overlapping period from the physical reanalysis of the Mediterranean (Table 1 and Supplementary Table S2). This reanalysis included data assimilation and properly captured the main hydrological and biogeochemical features of this area (Ramirez-Romero et al., 2020). Furthermore, the performance of each ensemble member (Supplementary Table S2) was assessed using the physical reanalysis as reference and collected in a Taylor diagram (Taylor, 2001) (Supplementary Figure S4). All the model details and validation could be found in supplementary information.
2.4 Statistical analysis
To avoid overfitting and to reduce the correlation and collinearity between variables, we filtered the initial set of predictors based on two metrics. First, all predictor covariates were examined using Pearson’s rank correlation (Wood, 2006). Pairs of variables with high correlation values (Pearson correlation; r>0.7) were identified and only one of the correlated pairs was included in the modelling process (Lezama-Ochoa et al., 2017). Subsequently, multicollinearity between predictor variables was tested by calculating the variance inflation factor (VIF) with a cutoff value of 5 (Hahlbeck et al., 2017; Lopez et al., 2020) and using the corvif function from the “AED” package in R (Zuur et al., 2009). As a result, highly correlated and multicollinearity variables were tested separately during the modelling, and only the one with the highest total explained variance was included in the final models.
For the spatial distribution modelling we used a two-stage approach to predict habitat suitability in density of deep-water rose shrimp, as the survey data was inflated to zero (Barry and Welsh, 2002). Generalized Additive Models (GAM; Hastie and Tibshirani, 1990; Wood, 2006) were used to build two models: (i) a binomial model (presence/absence) to predict the occurrence of the species, which was fitted through a binomial distribution with a logistic link function; and (ii) an abundance model (truncated; all zeros excluded), which was fitted through a Gamma distribution with a logarithmic link function.
The GAM models were fitted in the R software using the “mgcv” package (Wood and Wood, 2023), where the degrees of freedom of the smoothing functions were limited to 10 for the latitude and longitude interaction spline (spatial component) in the binomial model and for the abundance model, achieving the best fits. For the explanatory variables, the degrees of freedom of the smoothing functions were limited to 5 in bathymetry and 4 in sea bottom temperature. A forward step-wise variable selection procedure and the best model was selected based on Akaike Information Criterion (AIC), the unbiased risk estimator (UBRE) (Burnham and Anderson, 2004), significant explanatory variables and the highest explained deviance. These two models were combined by multiplying the predictions from both steps to obtain the final predicted value or preferred habitat model.
The evaluation of the predictive model of density was carried out in two phases: (i) for the model of occurrence and abundance, correlating the occurrence and the abundance predicted and observed by using the Pearson correlation coefficient (r); and (ii) for the binomial model by using the area under the receiver operating curve (AUC) (Fielding and Bell, 1997; Elith et al., 2006), and specificity, sensitivity, and True Skill Statistic (TSS) (Allouche et al., 2006). This model validation was performed using the “PresenceAbsence” R package (Freeman and Moisen, 2008).
The spatial structure of the forecasts, derived from the occurrence models in the three time windows (present, 2050s and 2100s) and taking into account the two different warming scenarios (RCP4.5 and RCP8.5), were compared using Schoener and similarity statistics (Warren et al., 2008). These analyses were performed using the “NicheOverlap” function of the “dismo” package (Hijmans et al., 2011) within the R software. In addition, we compared abundance and density maps using Pearson’s r spatial correlations with the “corLocal” function (“R raster” package; Hijmans, 2023). The spatial distribution model, evaluation current predictions and validation projections details could be found in Supplementary Information.
3 Results
3.1 Recent distribution and density trends
The analysis of MEDITS data for the period 2001–2021 showed that the initial presence of the deep-water rose shrimp was mainly restricted to the southernmost part of the study area (GSAs 1, 2 and southern part of GSA 6; Figure 3A). However, density increasing trends were registered along the two-decades period at the central and northernmost areas (GSA 6) and noticeably around the Balearic Islands (GSA 5; Figure 3B). The CPUEs of this species shared the same patterns. Initially, the highest catches were found in the southernmost basin and since 2007 a rising trend was registered in central and northernmost areas (GSAs 5 and 6), reaching similar levels in the whole area during the recent years (Figure 2B).
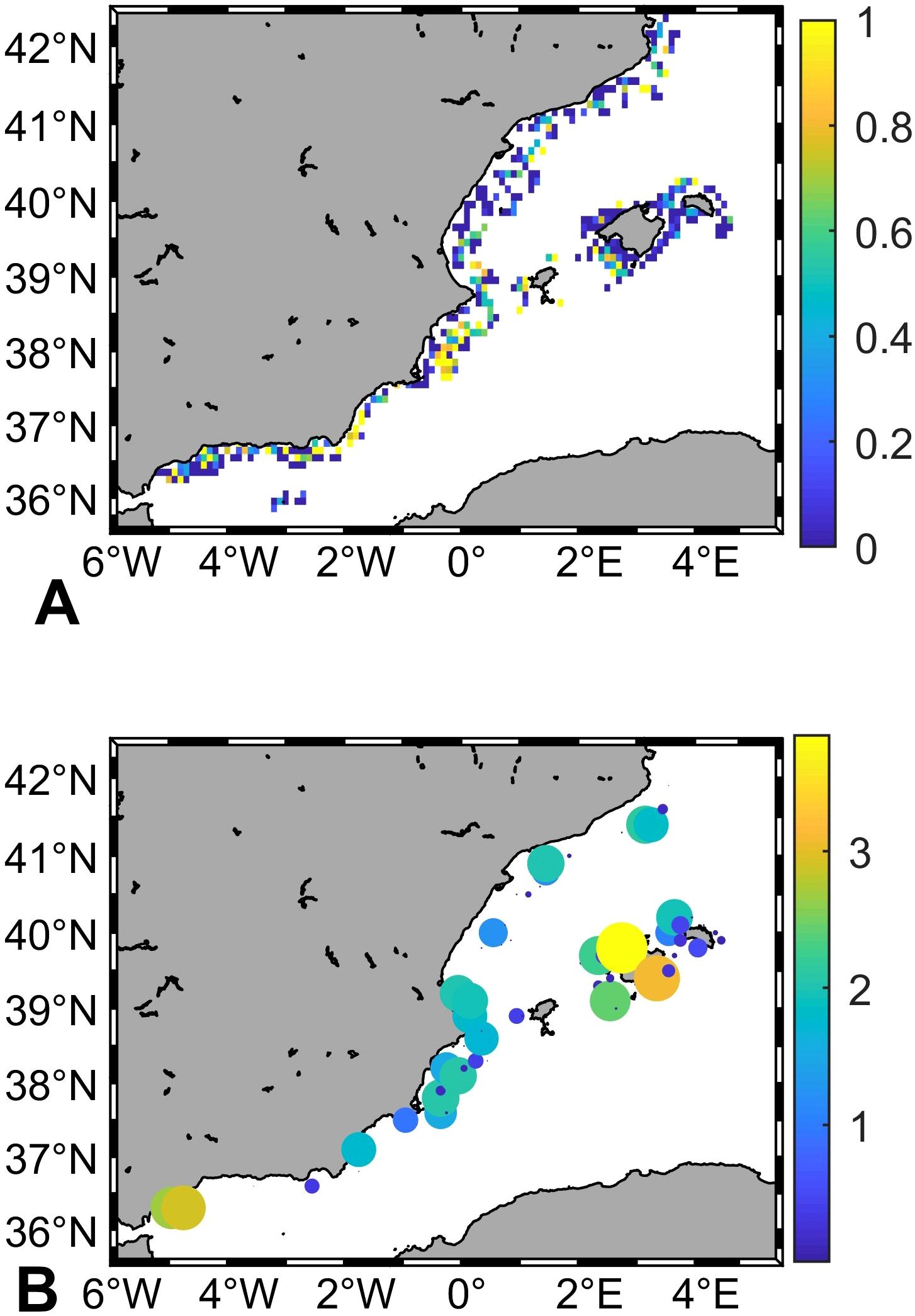
Figure 3. (A) Average presence of deep-water rose shrimp (Parapenaeus longirostris) in the sampling stations from the MEDITS bottoms trawl between 2001 and 2020; and (B) Significant slope values (log10; p-value<0.05) from the linear regression of density over time for each grid cell.
3.2 Environmental drivers and habitat distribution models
According to the cross-correlation matrix of the environmental variables included in the models and their variance inflation factors: Sea Bottom Temperature (SBT) was highly correlated with Sea Bottom Salinity (SBS), Integrated Phytoplankton Carbon Biomass and Integrated Chlorophyll were also highly correlated, bathymetry was highly correlated with SBS (Supplementary Figure S1A) and VIF values indicated multicollinearity between them (Supplementary Figure S1B).
Bathymetry, SBT and spatial component (latitude-longitude interaction) were the main drivers of the occurrence and abundance of the deep-water rose shrimp, with the greatest explained deviations (46.3 and 50%, respectively; Table 2). The most important covariate to explain the occurrence and abundance of the species was latitude-longitude interaction, with an explained deviation of 32.7 and 47.9%, respectively, followed by bathymetry with 29.8 and 19.3%, respectively and finally SBT with 12.8 and 17.2%, respectively.
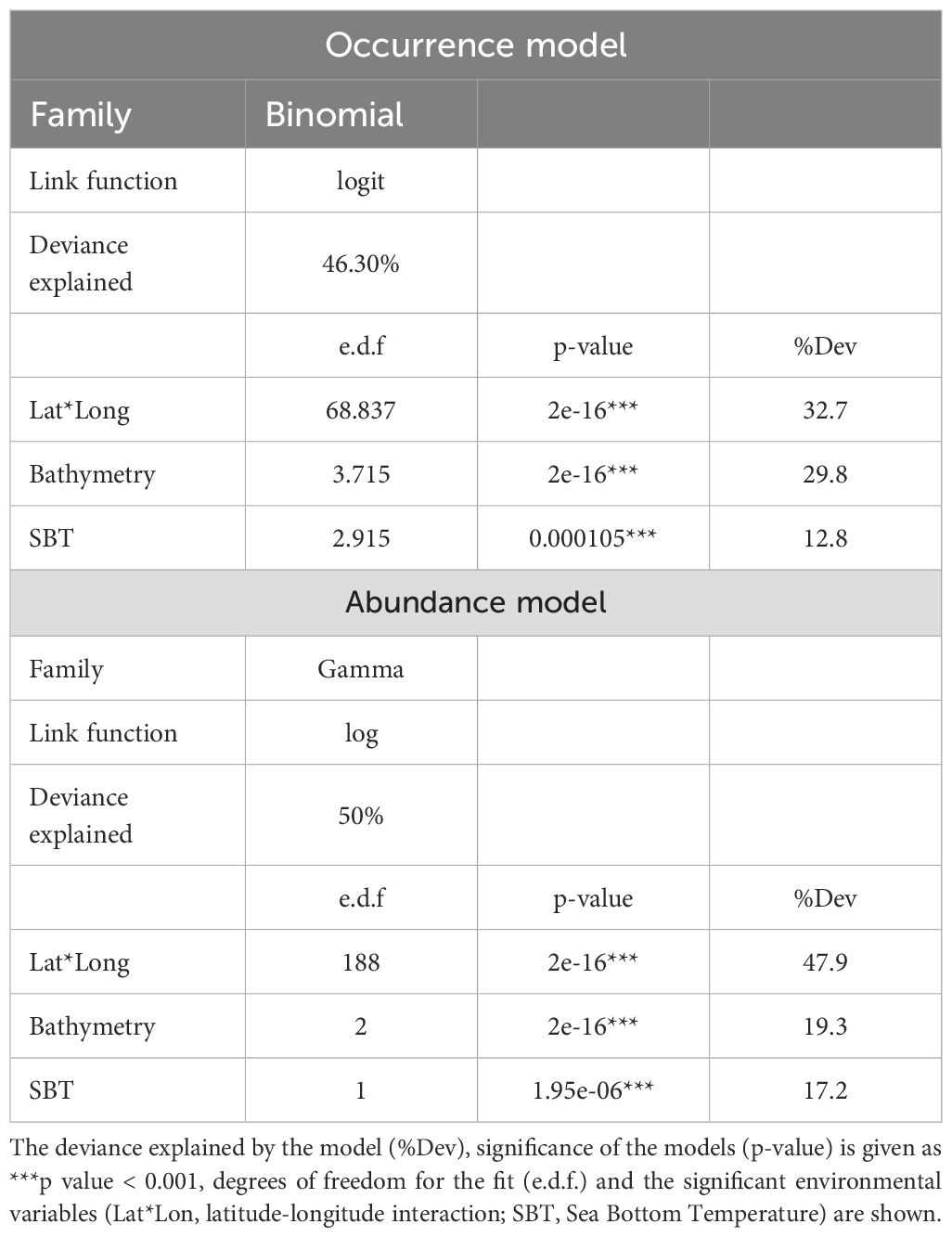
Table 2. Summary results for the Generalized Additive Models (GAM) selected for modelling the occurrence and abundance of deep-water rose shrimp (Parapenaeus longirostris).
For the predictive performance of species occurrence, the Pearson correlation coefficient (r) ranged from 0.58 to 0.59 and for abundance it was 0.40-0.41 (Supplementary Table S1). In addition, the occurrence model indicates a good degree of discrimination between presences and absences (AUC>0.5), good ability to fit true positive and negative predictions (SPEC and SENS ranged between 0.5 and 0.8) and good degree of similarity between the occurrence of the species and the available evidence (TSS ranging between 0.2 and 0.55). The spatial autocorrelation of the residuals of the final models indicates the absence of significant spatial autocorrelation in the residuals of the models of presence (Moran’s I= 0.11; p-value= 0.45) and abundance (Moran’s I= 0.69; p-value= 0.24). The species had occurrence and abundance maxima between 200 and 500 m depth (Figure 4). Regarding the SBT, both GAM models showed positive linear responses, increasing probabilities with higher SBT, particularly from ca. 15.5°C. Predictive maps showed an increasing northward gradient along the north-western Mediterranean, mainly due to SBT. All predictive maps for the density of the species are shown in Supplementary Figure S2.
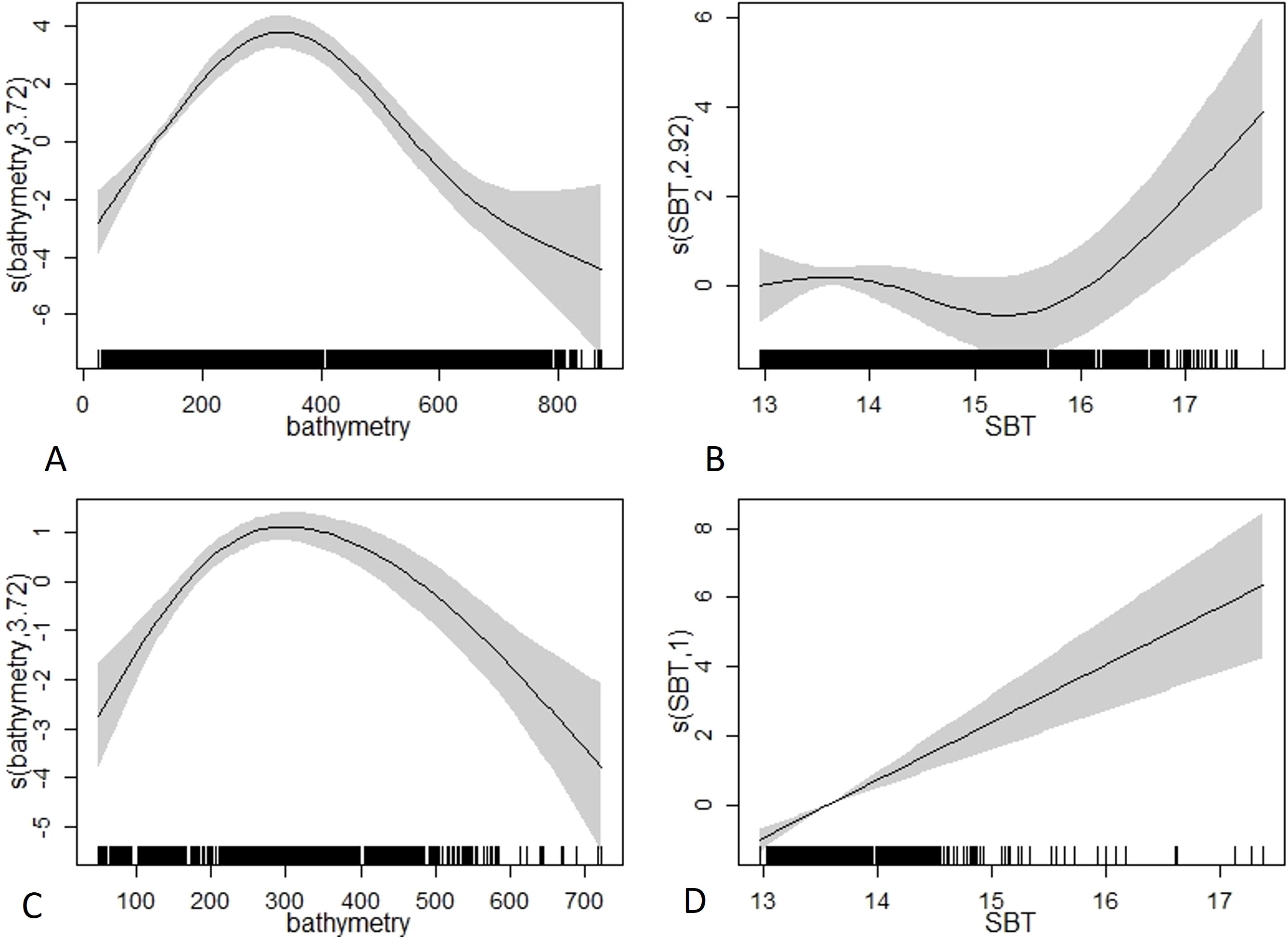
Figure 4. GAM partial plots for the best occurrence (A, B) and abundance (C, D) models developed for the deep-water rose shrimp (Parapenaeus longirostris). The Y axis (logit scale) represents the probability of occurrence and abundance of the species and the X axis represents the ranges of the significant environmental variables Bathymetry (m) and Sea Bottom Temperature (SBT; °C). The grey shaded areas represent the 95% confidence intervals around the response curve.
SBT patterns matched the deep-water rose shrimp distribution shift (Figure 3; Supplementary Figure S3). During the last decades (2001–2020), the southernmost area (GSA 1) was the warmest, but the central and northern areas (GSAs 5 and 6) presented the highest linear warming trends (Supplementary Figure S2).
3.3 Projections
Overall, the ensemble members (four RCMs) reproduced the observed patterns at present conditions (2006–2020) reasonably well, particularly for spatial correlations (Supplementary Figure S4). The ensemble averaged maps (bias-corrected) showed progressive warming of SBT along all the study area in both scenarios (Supplementary Figures S5, S6), more marked in the most extreme scenario (RCP8.5; Supplementary Figure S6). The internal variability in the ensemble (captured by the standard deviation) was generally larger in the 2050s compared to the 2100s (Supplementary Figures S5, S6).
Deep-water rose shrimp are expected to slightly increase its occupied area, with an expansion from 50 to 60% of the occupied area. Furthermore, projected densities showed a noticeably increase for both RCPs, rising from current estimated densities (104 individuals Km-2) to 105 individuals Km-2 (2050s) and reaching 106 individuals Km-2 at the end of the 21st century (2100s, Supplementary Figures S5, S6). This density raise was projected in almost the entire study area, but it was more marked in the central and northernmost parts (GSAs 5 and 6). Figure 5
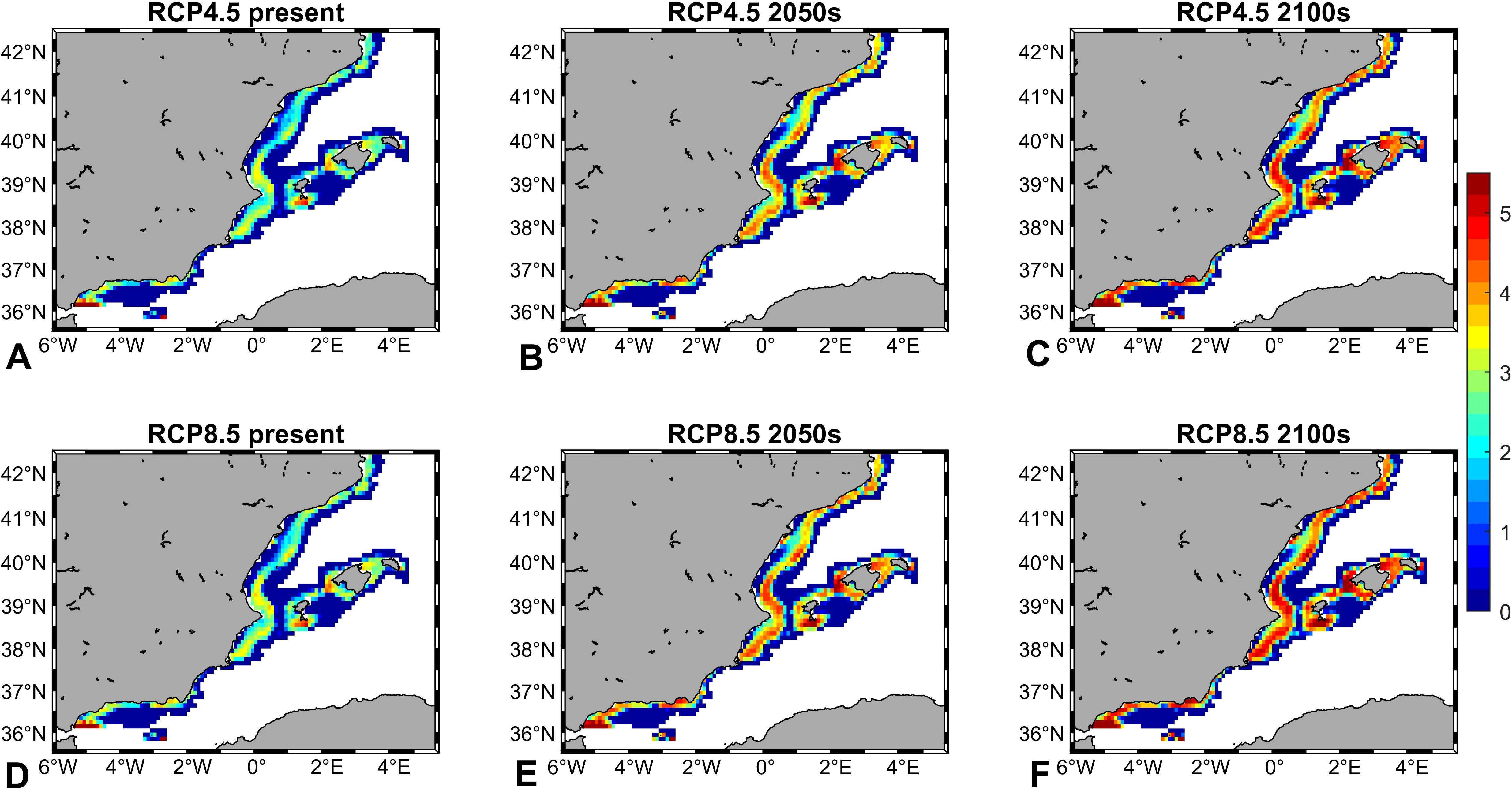
Figure 5. Projections of deep-water rose shrimp (Parapenaeus longirostris) density (individuals Km-2) during the present (2006–2020) and the future (2050s: 2040–205; 2100s: 2090–2100), under the different Representative Concentration Pathway (RCP) greenhouse gas concentration scenarios adopted by the Intergovernmental Panel on Climate Change (IPCC): RCP4.5 (A-C) and RCP8.5 (D-F).
Simulations registered a good level of agreement regarding the internal coherence of the ensemble, excepting a more divergent response under RCP4.5 in 2050s. Although being the most temporally remote period, in 2100s both RCP presented low internal variability within the ensemble (Figure 6).
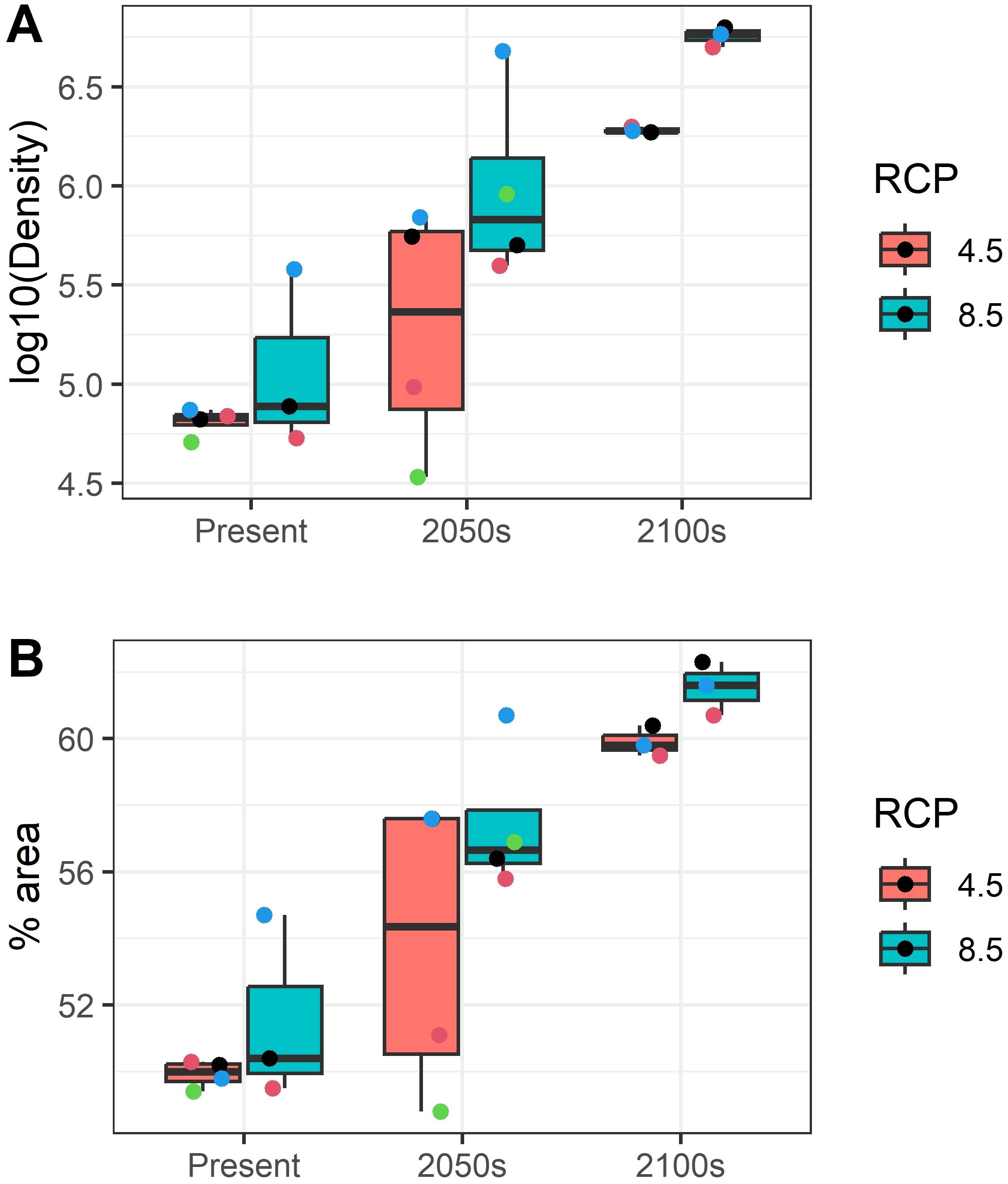
Figure 6. (A) Deep-water rose shrimp (Parapenaeus longirostris) density in individuals Km-2 and (B) percentage of occupied area forecasted for each member of the ensemble of projections during: present and the future (2050s: 2040–2050; 2100s: 2090–2100), under different Representative Concentration Pathway (RCP) greenhouse gas concentration scenarios adopted by the Intergovernmental Panel on Climate Change (IPCC): RCP4.5 and RCP8.5. The points represent each one of the ensemble members (Regional Climate Models CMIP5, Supplementary Table S2).
Regarding the robustness of these forecasts, in general the similarity statistics highlighted a good level of overlap in the occurrence predictions of the two data sets. In fact, Schoener’s D and Warren’s I ranged from approximately 0.87 to 0.99 for the RCP4.5 and RCP8.5 scenarios (Supplementary Table S3). For the abundance and density maps, the spatial correlations also indicated a high similarity between the predictions of different models in of present, 2050s and 2100s for the RCP4.5 and RCP8.5 scenarios (Supplementary Figures S7, S8).
Ensemble of simulations presented a high degree of match regarding the spatial forecast of the occupied area and density of the species (Supplementary Figure S9). In addition, the increase in density is also spatially forecasted with a reasonably internal coherence, particularly for the furthest future conditions and for the RCP8.5 scenario (Supplementary Figure S10).
4 Discussion
4.1 Monitoring climate change impacts in the Mediterranean
The Mediterranean Sea, a climate change hot spot (Shaltout and Omstedt, 2014; Tuel and Eltahir, 2020), presents unique oceanographic processes typical from marginal seas, such as the thermohaline circulation and deep-convective water formation, tightly coupled to intense atmospheric processes (Millot and Taupier-Letage, 2005). Because of this, a high sensitivity to climate forcing is expected in this basin, as small changes in the water masses hydrology can induce large variations in the thermohaline circulation and energy or mass budgets. In addition, complex mesoscale dynamics (e.g. fronts and filaments) are broadly present over the basin (Mason et al., 2023).
Despite this complexity, RCMs could properly capture these main features and coordinated multi-model and multi-scenario studies were carried out in an initiative as Med-CORDEX, specifically for the Mediterranean (https://www.medcordex.eu/; Ruti et al., 2016). According to previous RCM ensembles, which provide information on the range of projection outcomes, the water temperature in the intermediate layers (150-600 m depth) of the western Mediterranean, where many of its key demersal fishing resources inhabit, are expected to increase in 1.32 ± 0.16 and 2.23 ± 0.42°C for RCP4.5 and RCP8.5, respectively (Soto-Navarro et al., 2020). Recent works even forecasted a collapse of the deep-water formation in the Gulf of Lions by 2040–2050, attributed to an increasing stratification potentially leading on pervasive modifications of the basin circulation (Parras-Berrocal et al., 2022).
4.2 Habitat shifts in demersal resources: insights from spatial distribution models
The positive linear response of the deep-water rose shrimp to SBT (Figures 4B, D), modeled in this study from research survey data, confirmed the fisheries-data presented here (Figure 2) and the results by Mingote et al. (2024) in the northernmost area using landings. This species has been favored in terms of abundance and density, due to the water warming affecting the Mediterranean during recent decades (Colloca et al., 2014) and, therefore, can be considered a sentinel of climate change (Hazen et al., 2019). Given that the species is an important component of demersal crustacean communities (e.g. Abelló et al., 2002) and fishing resources (Bertrand et al., 2002; Spedicato et al., 2019), this finding is particularly useful for early detecting warming hotspots in deep ecosystems, where tracking changes in communities is challenging.
Although water temperature above seabed has been validated as a good descriptor of habitat suitability for this species in the western Mediterranean (Abelló et al., 2002; Colloca et al., 2014; Mingote et al., 2024), our forecasting results could be considered in the future for their improvement. The spatial distribution of demersal species can be projected more accurately and precisely by incorporating mechanistic/physiological or density-dependent effects, among other factors. Moreover, the predictions were carried out without taking into account other factors such as fishing effort, biotic interactions (e.g. competition and predation, reproduction/fecundity rates) and ecological processes, such as dispersal limitations or habitat connectivity. This simplification may limit the accuracy of predictions, especially for ecologically complex systems.
4.3 Impact of global warming and overexploitation on Mediterranean fisheries and the need for adaptive management strategies
The warming progression along the Mediterranean Sea can extensively alter the availability of fishing resources (e.g. Ben Lamine et al., 2022), emerging as an essential factor for the assessment and management of fisheries. It becomes especially relevant in areas like the Mediterranean, where most demersal stocks have been overexploited for long time (Vasilakopoulos et al., 2014), generally impaired by a high fishing pressure, combined with the low selectivity of bottom trawling (Colloca et al., 2013). As a consequence, most commercial species are currently overexploited (FAO Fishery and Aquaculture Global Statistics, 2023) and show truncated size- and age-structures of their populations, which can cause then the loss of resilience in the face of climate change (Hilborn and Walters, 1992; Pauly and Zeller, 2016; Barange et al., 2018). In fact, the synergistic effects of fishing-induced demographic changes and climate variation on Mediterranean fish population dynamics have been already described (Hidalgo et al., 2011).
Understanding medium and long-term changes in marine ecosystems allows fishing stakeholders to anticipate potential changes in exploited resources in the coming decades. This enables them to propose adaptation strategies to minimize climate change impacts and regulate fishing efforts. These strategies are crucial, as climate-driven changes in the distribution, abundance and/or migration of species might not align with fishing efforts, due to the complex response of the socio-economic system (Barange et al., 2018). This adaptation, defined as a “process of adjustment in ecological, social or economic systems to actual or expected climate and its effects” (IPCC, 2014), has been insufficiently considered for Mediterranean fisheries. In fact, National Adaptation Plans regarding climate change generally show limited references to adaptation measures for fisheries (Hidalgo et al., 2018).
The predictions and projections on the impact of climate on fisheries must be made at regional and local scales, where historical data series can contribute to improving complex models. These scales are particularly suitable for adapting fisheries industries and fisheries management, since global models are of limited value (Brander et al., 2013). By focusing on regional and local scales, it is possible to tailor strategies to the specific conditions and challenges of different areas. This allows for more precise and effective adaptation measures that take into account local ecosystem dynamics, socio-economic factors and community needs. Adapting at these scales ensures that fishing sector can respond more accurately to the impacts of climate change at different regions, leading to more sustainable and resilient fisheries. In this context, the present results are even more crucial considering that the deep-water rose shrimp is one of the six target species under Regulation (EU) 2019/1022 of the European Parliament and the Council, which aims to sustainably manage demersal stocks in the western Mediterranean. The implementation of this multi-annual plan is leading to a reduction up to 40% in the fishing days of the bottom trawl fleet during the recent years. According to our results, this reduction would not be fully justified in the case of this species.
In conclusion, this study improves the understanding of the habitat preferences and current and future distribution of one of the most relevant demersal species in the western Mediterranean. Our results indicate a northward expansion and an increase in the density of the species, mainly driven by warming trends of water above seafloor. These findings are fundamental to develop effective fisheries management strategies in the face of global warming, ensuring the sustainability of this key fishing resource. The use of a RCM ensemble has proven to be advantageous in providing robust and ecologically interpretable projections, ultimately contributing to better scientific advice for fisheries planning adapted to environmental changes caused by global warming.
Data Availability Statement
Climate data supporting this study are openly available mainly from CMEMS and MedCordex initiatives. Biological data are available on reasonable request.
Author contributions
CG: Data curation, Formal analysis, Investigation, Methodology, Visualization, Writing – original draft, Writing – review & editing. ER: Data curation, Formal analysis, Investigation, Methodology, Visualization, Writing – original draft, Writing – review & editing. BG: Conceptualization, Funding acquisition, Investigation, Resources, Writing – original draft, Writing – review & editing. MF: Data curation, Investigation, Writing – original draft, Writing – review & editing. DM: Conceptualization, Data curation, Funding acquisition, Project administration, Resources, Supervision, Writing – original draft, Writing – review & editing. EM: Conceptualization, Funding acquisition, Project administration, Resources, Supervision, Writing – original draft, Writing – review & editing.
Funding
The author(s) declare financial support was received for the research, authorship, and/or publication of this article. This study has been developed within the FishClim project “Scientific knowledge for adaptation to climate change in the Spanish fishing sector” (MAPA-04-03), financed by the European Union-NextGenerationEU through the Axis 4 of the “Agreement between the Ministry of Agriculture, Fisheries and Food and the State Agency Superior Council for Scientific Research, M.P. –through the Spanish Institute of Oceanography– to promote fishing research as a basis for sustainable fisheries management”. It has also received support from SosMed Project (MAPA-01-01), included in Axis 1 of the same agreement. MEDITS surveys are co-financed by the General Secretariat for Fisheries, the Spanish Institute of Oceanography and the European Union through the European Maritime and Fisheries Fund (FEMP), to support the Common Fisheries Policy.
Acknowledgments
We would like to thank the General Secretariat of Fisheries, the Spanish Institute of Oceanography (IEO-CSIC) and the Institute of Marine Sciences of Andalucia (ICMAN-CSIC) for their help in the creation of the FishClim project.
Conflict of interest
The authors declare that the research was conducted in the absence of any commercial or financial relationships that could be construed as a potential conflict of interest.
Publisher’s note
All claims expressed in this article are solely those of the authors and do not necessarily represent those of their affiliated organizations, or those of the publisher, the editors and the reviewers. Any product that may be evaluated in this article, or claim that may be made by its manufacturer, is not guaranteed or endorsed by the publisher.
Supplementary material
The Supplementary Material for this article can be found online at: https://www.frontiersin.org/articles/10.3389/fmars.2024.1494177/full#supplementary-material
References
Abelló P., Abella Á., Adamidou A., Jukic-Peladic S., Maiorano P., Spedicato M. T. (2002). Geographical patterns in abundance and population structure of Nephrops norvegicus and Parapenaeus longirostris (Crustacea: Decapoda) along the European Mediterranean coasts. Sci. Mar. 66, 125. doi: 10.3989/scimar.2002.66s2125
Allouche O., Tsoar A., Kadmon R. (2006). Assessing the accuracy of species distribution models: prevalence, kappa and the true skill statistic (TSS). J. Appl. Ecol. 43, 1223–1232. doi: 10.1111/j.1365-2664.2006.01214.x
Assis J., Tyberghein L., Bosch S., Verbruggen H., Serrão E. A., De Clerck O., et al. (2018). Bio-ORACLE v2.0: Extending marine data layers for bioclimatic modelling. Global Ecol. Biogeography 27, 277–284. doi: 10.1111/geb.12693
Barange M., Bahri T., Beveridge M. C. M., Cochrane K. L., Funge-Smith S., Poulain F. (2018).Impacts of climate change on fisheries and aquaculture: synthesis of current knowledge, adaptation and mitigation options. In: FAO fisheries and aquaculture technical paper 627 (Rome). Available online at: https://openknowledge.fao.org/server/api/core/bitstreams/9aeb8ade-a623-4954-8adf-204daae3b5de/content (Accessed September 6, 2024).
Barry S. C., Welsh A. H. (2002). Generalized additive modelling and zero inflated count data. Ecol. Modell 157, 179–188. doi: 10.1016/S0304-3800(02)00194-1
Benchoucha S., Berraho A., Bazairi H., Katara I., Benchrifi S., Valavanis V. D. (2008). “Salinity and temperature as factors controlling the spawning and catch of Parapenaeus longirostris along the Moroccan Atlantic Ocean,” in Essential fish habitat mapping in the mediterranean (Springer Netherlands, Dordrecht), 109–123. doi: 10.1007/978-1-4020-9141-4_9
Ben Lamine E., Schickele A., Goberville E., Beaugrand G., Allemand D., Raybaud V. (2022). Expected contraction in the distribution ranges of demersal fish of high economic value in the Mediterranean and European Seas. Sci. Rep. 12, 10150. doi: 10.1038/s41598-022-14151-8
Bertrand J. A., Gil de Sola L., Papaconstantinou C., Relini G., Souplet A. (2002). The general specifications of the MEDITS surveys. Sci. Mar. 66, 9. doi: 10.3989/scimar.2002.66s29
Beverton R. J., Holt S. J. (1959). On the dynamics of exploited fish populations. Fisheries Investigations 19, 1–533. doi: 10.1007/978-94-011-2106-4
Brander K., Neuheimer A., Andersen K. H., Hartvig M. (2013). Overconfidence in model projections. ICES J. Mar. Sci. 70, 1065–1068. doi: 10.1093/icesjms/fst055
Burnham K. P., Anderson D. R. (2004). Multimodel inference. Sociol Methods Res. 33, 261–304. doi: 10.1177/0049124104268644
Colloca F., Cardinale M., Maynou F., Giannoulaki M., Scarcella G., Jenko K., et al. (2013). Rebuilding Mediterranean fisheries: a new paradigm for ecological sustainability. Fish Fisheries 14, 89–109. doi: 10.1111/j.1467-2979.2011.00453.x
Colloca F., Mastrantonio G., Lasinio G. J., Ligas A., Sartor P. (2014). Parapenaeus longirostris (Lucas 1846) an early warning indicator species of global warming in the central Mediterranean Sea. J. Mar. Syst. 138, 29–39. doi: 10.1016/j.jmarsys.2013.10.007
D’Onghia G., Giove A., Maiorano P., Carlucci R., Minerva M., Capezzuto F., et al. (2012). Exploring relationships between demersal resources and environmental factors in the ionian sea (Central mediterranean). J. Mar. Biol. 2012, 1–12. doi: 10.1155/2012/279406
Davies S. C., Thompson P. L., Gomez C., Nephin J., Knudby A., Park A. E., et al. (2023). Addressing uncertainty when projecting marine species’ distributions under climate change. Ecography. 2023 (11 1-18), e06731. doi: 10.1111/ecog.06731
Elith J. ,. H., Graham C. ,. P., Anderson R., Dudík M., Ferrier S., Guisan A., et al. (2006). Novel methods improve prediction of species’ distributions from occurrence data. Ecography 29, 129–151. doi: 10.1111/j.2006.0906-7590.04596.x
FAO Fishery and Aquaculture Global Statistics (2023). Fishstat. Available online at: http://www.fao.org/fishery/statistics/software/fishstatj/en (Accessed October 3, 2023).
Fielding A. H., Bell J. F. (1997). A review of methods for the assessment of prediction errors in conservation presence/absence models. Environ. Conserv. 24, 38–49. doi: 10.1017/S0376892997000088
Fortibuoni T., Bahri T., Camilleri M., Gristina M., Garofalo G., Fiorentino F. (2010). Nursery and spawning areas of deep-water rose shrimp, Parapenaeus longirostris (Decapoda: penaeidae), in the strait of sicily (Central mediterranean sea). J. Crustacean Biol. 30, 167–174. doi: 10.1651/09-3167.1
Freeman E. A., Moisen G. (2008). PresenceAbsence : an R package for presence absence analysis. J. Stat. Softw 23, 1–31. doi: 10.18637/jss.v023.i11
Guijarro B., Massutí E., Moranta J., Cartes J. E. (2009). Short spatio-temporal variations in the population dynamics and biology of the deep-water rose shrimp Parapenaeus longirostris (Decapoda: Crustacea) in the western Mediterranean. Sci. Mar. 73, 183–197. doi: 10.3989/scimar.2009.73n1183
Hahlbeck N., Scales K. L., Dewar H., Maxwell S. M., Bograd S. J., Hazen E. L. (2017). Oceanographic determinants of ocean sunfish (Mola mola) and bluefin tuna (Thunnus orientalis) bycatch patterns in the California large mesh drift gillnet fishery. Fish Res. 191, 154–163. doi: 10.1016/j.fishres.2017.03.011
Hastie T., Tibshirani R. (1990). Generalized additive models. Eds. Cox R., Hinkley, Silverman (Chapman & Hall/CRC), 352.
Hazen E. L., Abrahms B., Brodie S., Carroll G., Jacox M. G., Savoca M. S., et al. (2019). Marine top predators as climate and ecosystem sentinels. Front. Ecol. Environ. 17, 565–574. doi: 10.1002/fee.2125
Hidalgo M., Mihneva V., Vasconcellos M., Bernal M. (2018). “Chapter 7: Climate change impacts, vulnerabilities and adaptations: Mediterranean Sea and the Black Sea marine fisheries,” in Impacts of climate change on fisheries and aquaculture: synthesis of current knowledge, adaptation and mitigation options. FAO Fisheries and Aquaculture Technical Paper No. 627 (Rome; FAO). Available at: https://www.researchgate.net/publication/326302661.
Hidalgo M., Rouyer T., Molinero J. C., Massutí E., Moranta J., Guijarro B., et al. (2011). Synergistic effects of fishing-induced demographic changes and climate variation on fish population dynamics. Mar. Ecol. Prog. Ser. 426, 1–12. doi: 10.3354/meps09077
Hijmans R. J. (2023).raster: geographic data analysis and modeling. In: R package version 3. Available online at: https://CRAN.R-project.org/package=raster (Accessed May 15, 2023).
Hijmans R. J., Phillips S., Leathwick J., Elith J. (2011). Package ‘dismo.’. Available online at: http://cran.r-project.org/web/packages/dismo/index.html (Accessed September 21, 2023).
Hilborn R., Walters C. J. (1992). Quantitative fisheries stock assessment (Boston, MA: Springer US). doi: 10.1007/978-1-4615-3598-0
IPCC (2014). “Annex II: glossary,” in Climate change 2014: synthesis report. Contribution of working groups I, II and III to the fifth assessment report of the intergovernmental panel on climate change. (Geneva, Switzerland: IPCC).
Issifu I., Alava J. J., Lam V. W. Y., Sumaila U. R. (2022). Impact of ocean warming, overfishing and mercury on european fisheries: A risk assessment and policy solution framework. Front. Mar. Sci. 8. doi: 10.3389/fmars.2021.770805
Lezama-Ochoa N., Murua H., Hall M., Román M., Ruiz J., Vogel N., et al. (2017). Biodiversity and habitat characteristics of the bycatch assemblages in fish aggregating devices (FADs) and school sets in the eastern pacific ocean. Front. Mar. Sci. 4. doi: 10.3389/fmars.2017.00265
Ligas A., Sartor P., Colloca F. (2011). Trends in population dynamics and fishery of Parapenaeus longirostris and Nephrops norvegicus in the Tyrrhenian Sea (NW Mediterranean): the relative importance of fishery and environmental variables. Mar. Ecol. 32, 25–35. doi: 10.1111/j.1439-0485.2011.00440.x
Lopez J., Alvarez-Berastegui D., Soto M., Murua H. (2020). Using fisheries data to model the oceanic habitats of juvenile silky shark (Carcharhinus falciformis) in the tropical eastern Atlantic Ocean. Biodivers Conserv. 29, 2377–2397. doi: 10.1007/s10531-020-01979-7
Mason E., Barceló-Llull B., Sánchez-Román A., Rodríguez-Tarry D., Cutolo E., Delepoulle A., et al. (2023). “Fronts, eddies and mesoscale circulation in the Mediterranean Sea,” in Oceanography of the mediterranean sea (Elsevier), 263–287. doi: 10.1016/B978-0-12-823692-5.00003-0
Maynou F., Sabatés A., Raya V. (2020). Changes in the spawning habitat of two small pelagic fish in the Northwestern Mediterranean. Fish Oceanogr 29, 202–214. doi: 10.1111/fog.12464
MEDITS group (2017). MEDITS Handbook v9 (MEDITS Working Group). Available online at: http://www.sibm.it/MEDITS%202011/principaledownload.htm (Accessed September 20, 2023).
Millot C., Taupier-Letage I. (2005). Circulation in the mediterranean sea (Springer-Verlag Berlin Heidelberg). 29–66. doi: 10.1007/b107143
Mingote M. G., Galimany E., Sala-Coromina J., Bahamon N., Ribera-Altimir J., Santos-Bethencourt R., et al. (2024). Warming and salinization effects on the deep-water rose shrimp, Parapenaeus longirostris, distribution along the NW Mediterranean Sea: Implications for bottom trawl fisheries. Mar. pollut. Bull. 198, 115838. doi: 10.1016/J.MARPOLBUL.2023.115838
Orio A., Bergström U., Florin A. B., Lehmann A., Šics I., Casini M. (2019). Spatial contraction of demersal fish populations in a large marine ecosystem. J. Biogeogr 46, 633–645. doi: 10.1111/jbi.2019.46.issue-3
Parras-Berrocal I. M., Vázquez R., Cabos W., Sein D. V., Álvarez O., Bruno M., et al. (2022). Surface and intermediate water changes triggering the future collapse of deep water formation in the north western mediterranean. Geophys Res. Lett. 49. doi: 10.1029/2021GL095404
Pauly D., Zeller D. (2016). Catch reconstructions reveal that global marine fisheries catches are higher than reported and declining. Nat. Commun. 7, 10244. doi: 10.1038/ncomms10244
Politou C.-Y., Tserpes G., Dokos J. (2008). “Identification of deep-water pink shrimp abundance distribution patterns and nursery grounds in the eastern Mediterranean by means of generalized additive modelling,” in Essential fish habitat mapping in the mediterranean (Springer Netherlands, Dordrecht), 99–107. doi: 10.1007/978-1-4020-9141-4_8
Punt A., Walker T., Taylor B., Pribac F. (2000). Standardization of catch and effort data in a spatially-structured shark fishery. Fish Res. 45, 129–145. doi: 10.1016/S0165-7836(99)00106-X
Ramirez-Romero E., Jordà G., Amores A., Kay S., Segura-Noguera M., Macias D. M., et al. (2020). Assessment of the skill of coupled physical–biogeochemical models in the NW mediterranean. Front. Mar. Sci. 7. doi: 10.3389/fmars.2020.00497
Ruti P. M., Somot S., Giorgi F., Dubois C., Flaounas E., Obermann A., et al. (2016). Med-CORDEX initiative for mediterranean climate studies. Bull. Am. Meteorol Soc. 97, 1187–1208. doi: 10.1175/BAMS-D-14-00176.1
Sbrana M., Viva C., Belcari P. (2006). Fishery of the deep-water rose shrimp Parapenaeus longirostris (Lucas 1846) (Crustacea: decapoda) in the northern tyrrhenian sea (Western mediterranean). Hydrobiologia 557, 135–144. doi: 10.1007/s10750-005-1316-9
Schoeman D. S., Gupta A., Sen Harrison C. S., Everett J. D., Brito-Morales I., Hannah L., et al. (2023). Demystifying global climate models for use in the life sciences. Trends Ecol. Evol. 38 (9), 843–858. doi: 10.1016/j.tree.2023.04.005
Shaltout M., Omstedt A. (2014). Recent sea surface temperature trends and future scenarios for the Mediterranean Sea. Oceanologia 56, 411–443. doi: 10.5697/oc.56-3.411
Sobrino I., Silva C., Sbrana M., Kapiris K. (2005). A review of the biology and fisheries of the deep water rose shrimp, Parapenaeus longirostris, in European Atlantic and Mediterranean waters. Crustaceana 78, 1153–1184. doi: 10.1163/156854005775903564
Soto M., Pallarés P., Gaertner D., Delgado de Molina A., Fonteneau A., Ariz J. (2002). Standardization of tropical purse seine fishing effort by generalized linear model (GLM). IOTC Proceedings IOTC Proceedings 5, 388–397. Available online at: https://openknowledge.fao.org/server/api/core/bitstreams/4cee94b6-b114-48bd-a56a-e2c59586a69e/content.
Soto-Navarro J., Jordá G., Amores A., Cabos W., Somot S., Sevault F., et al. (2020). Evolution of Mediterranean Sea water properties under climate change scenarios in the Med-CORDEX ensemble. Clim Dyn 54, 2135–2165. doi: 10.1007/s00382-019-05105-4
Spedicato M. T., Massutí E., Mérigot B., Tserpes G., Jadaud A., Relini G. (2019). The MEDITS trawl survey specifications in an ecosystem approach to fishery management. Sci. Mar. 83, 9–20. doi: 10.3989/scimar.04915.11X
Sund P. N., Blackburn M., Williams F. (1981). Tuna and their environment in the pacific ocean: A review. Oceanography Mar. Biol. Annu. Rev. 19, 443–512.
Taylor K. E. (2001). Summarizing multiple aspects of model performance in a single diagram. J. Geophysical Research: Atmospheres 106, 7183–7192. doi: 10.1029/2000JD900719
Tom M., Goren M., Ovadia M. (1988). The benthic phase of the life cycle of Parapenaeus longirostris (Crustacea, Decapoda, Penaeidae) along the Mediterranean coast of Israel. Hydrobiologia 169, 339–352. doi: 10.1007/BF00007557
Townhill B. L., Couce E., Tinker J., Kay S., Pinnegar J. K. (2023). Climate change projections of commercial fish distribution and suitable habitat around north western Europe. Fish Fisheries 24, 848–862. doi: 10.1111/faf.12773
Tuel A., Eltahir E. A. B. (2020). Why is the mediterranean a climate change hot spot. J. Clim 33, 5829–5843. doi: 10.1175/JCLI-D-19-0910.1
Vasilakopoulos P., Maravelias C. D., Tserpes G. (2014). The alarming decline of mediterranean fish stocks. Curr. Biol. 24, 1643–1648. doi: 10.1016/j.cub.2014.05.070
Warren D. L., Glor R. E., Turelli M. (2008). Environmental niche equivalency versus conservatism: Quantitative approaches to niche evolution. Evol. (N Y) 62, 2868–2883. doi: 10.1111/j.1558-5646.2008.00482.x
Wood S. N. (2006). On confidence intervals for generalized additive models based on penalized regression splines. Aust. N Z J. Stat. 48, 445–464. doi: 10.1111/j.1467-842X.2006.00450.x
Keywords: spatial distribution model, regional climate mode, demersal fishing resources, climate change, fisheries resilience, Parapenaeus longirostris
Citation: González-Andrés C, Ramírez-Romero E, Guijarro B, Farré M, Macias D and Massutí E (2024) Warming promotes expansion of a key demersal fishing resource of the western Mediterranean. Front. Mar. Sci. 11:1494177. doi: 10.3389/fmars.2024.1494177
Received: 10 September 2024; Accepted: 15 October 2024;
Published: 08 November 2024.
Edited by:
Gualtiero Basilone, National Research Council (CNR), ItalyReviewed by:
Gleyci A. O. Moser, Universidade do Estado do Rio de Janeiro, BrazilBarbara Zorica, Institute of Oceanography and Fisheries (IZOR), Croatia
Copyright © 2024 González-Andrés, Ramírez-Romero, Guijarro, Farré, Macias and Massutí. This is an open-access article distributed under the terms of the Creative Commons Attribution License (CC BY). The use, distribution or reproduction in other forums is permitted, provided the original author(s) and the copyright owner(s) are credited and that the original publication in this journal is cited, in accordance with accepted academic practice. No use, distribution or reproduction is permitted which does not comply with these terms.
*Correspondence: Eduardo Ramírez-Romero, ZS5yYW1pcmV6LnJvbWVyb0BpZW8uY3NpYy5lcw==
†These authors contributed equally to this work and share first authorship