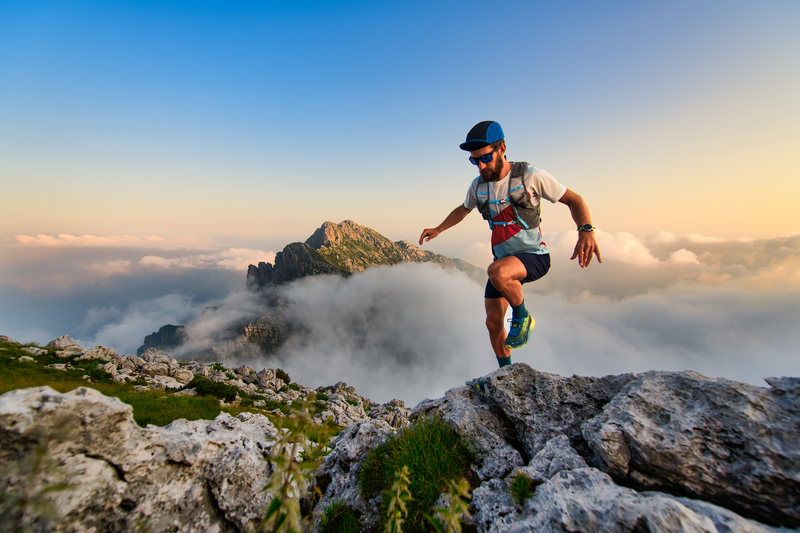
95% of researchers rate our articles as excellent or good
Learn more about the work of our research integrity team to safeguard the quality of each article we publish.
Find out more
ORIGINAL RESEARCH article
Front. Mar. Sci. , 14 November 2024
Sec. Ocean Solutions
Volume 11 - 2024 | https://doi.org/10.3389/fmars.2024.1485161
This article is part of the Research Topic Data-Driven Ocean Environmental Perception with its Applications View all 10 articles
The withdrawal of the United Kingdom from the European Union will likely result in reduced fishing grounds for the Belgian fishing fleet. This fleet now targets demersal fish, but there used to be a tradition of catching Atlantic herring (Clupea harengus). After the stock collapse of Atlantic herring in the 1970s, fishing on herring by the Belgian fleet did not recover and herring quotas are now exchanged with the Netherlands and Germany. To assess the feasibility of reintroducing herring fisheries for the Belgian fishing fleet, our study created spatiotemporal species distribution models for Atlantic herring in the Northeast Atlantic Ocean, focusing results on the Belgian Part of the North Sea. In total 30078 occurrence records were derived and processed to fit species-environmental relationships with temperature, salinity, seabed characteristics and plankton concentration using Maximum entropy (Maxent) models. The Area Under the Curve of the Receiver Operating Characteristic plot (AUC) and the True Skill Statistic (TSS) were used to assess model fit. Models performed well (AUC > 0.7 and TSS > 0.6). While a broad spatiotemporal distribution of Atlantic herring in the Northeast Atlantic Ocean was inferred, regional differences show that herring habitat is most suitable during winter months in the Belgian Part of the North Sea for both adult and larval herring (habitat suitability index > 75%). This regional trend in the Belgian Part of the North Sea was negatively correlated (R = -0.8) with the North Atlantic Oscillation (NAO). We anticipate that these findings will provide valuable insights for policymakers to implement sustainable fisheries management practices.
Since the start of the Common fisheries policy in 1970, European Union (EU) members have been allowed equal access to fish in their shared waters, including those of the United Kingdom (UK). With the withdrawal of the UK from the EU on the 1st of January 2021, it was decided that this will change (Regulation 1380/2013, 2013). As a transition period, EU vessels are allowed to access UK waters until 30 June 2026 and afterwards, access will require annual negotiation. Furthermore, fishing quotas with a value of 25% of the EU landings in UK waters will be gradually transferred from the EU to the UK from 2021 to 2025 (Popescu and Scholaert, 2022).
For the Belgian fishing fleet, the loss by transfer of fishing quota to the UK is estimated at 3.7 million euros in 2023 (Popescu and Scholaert, 2022) and estimated to increase up to 6.8 million euros in 2026 (Coudyser, 2021). Recently, the Belgian fishing fleet has experienced a steady decline in catches in the southern North Sea and a decrease in the number of vessels (data up to 2021, Maertens, 2022). The Belgian fleet targets demersal fish, mainly sole (Solea solea) and plaice (Pleuronectes platessa) using beam trawlers (Regulation No 1380/2013). Historically, pelagic fish species, particularly Atlantic herring (Clupea harengus), were also targeted with annual yields reaching up to 58 000 tons in 1943 (Lescrauwaet et al., 2010). One of the initiatives to overcome the loss of fishing grounds and quota following Brexit is to provide information about alternative fishing grounds and niche fisheries (European Commission, 2020). One of those alternatives for the Belgian fishing fleet could be the restoration of pelagic fisheries in Belgian waters. Because the current Belgian fishing fleet mainly targets bottom-dwelling fish (Regulation No 1380/2013, n.d), the whereabouts of pelagic fish are often unknown, anecdotical, or expert-based.
Atlantic herring is a valuable pelagic fish species in the North Sea in terms of both economy but also in terms of ecology. It plays a key role in the ecosystem as a regulator for seabird abundance (through bottom-up control; Fauchald et al., 2011) and zooplankton (through top-down control; Fauchald et al., 2011). During early larval stages, Atlantic herring feeds on phytoplankton and unicellular organisms (Marshall et al., 1937; Joly et al., 2021), subsequently larval diet shifts towards zooplankton (Van Ginderdeuren et al., 2014). For adults, the main prey is copepods, but feeding on other zooplankton and fish larvae is also common (Van Ginderdeuren et al., 2014). Atlantic herring can tolerate a wide range of temperatures (1 – 19°C; de Groot, 1980; Whitehead, 1985) and salinities (2 – 35 PSU; Brevé et al., 2007; de Groot, 1980), which allows them to migrate between feeding, spawning, and nursing grounds. Typical for this species is their natal homing behavior or the return to the same area where they hatched for spawning (Geffen, 2009).
In the Northeast Atlantic, Atlantic herring populations are divided into several distinct stocks, based primarily on their spawning grounds and migration behaviors. These stocks include the North Sea autumn spawners, the West of Scotland stock, the Irish and Celtic Sea stocks, and the Downs stock. Each stock shows unique migration and spawning behaviors. Based on the spawning period, Atlantic herring populations are divided into two groups in the Northeast Atlantic: spring and autumn spawners (Heath, 1993). Herring in the Belgian Part of the North Sea (BPNS) belong to the Downs stock which are autumn spawners.
Currently, the stock of autumn-spawning Atlantic herring is stable in the North Sea (ICES, 2023a). For the Irish and Celtic Sea, the International Council for the Exploration of the Sea (ICES) advises zero catches of herring to sustain maximum sustainable yield (ICES, 2023b, ICES, 2023c, ICES, 2023d). Atlantic herring stocks are prone to collapse due to natural fluctuations in abundance, aggravated by poorly managed fishing pressure with slow recovery rates (Stephenson et al., 2001). In addition to maintaining the overall stock biomass, effective management also requires the preservation of their spatial and temporal spawning distribution (Frost and Diele, 2022; Stephenson et al., 2001). During spawning, herring lay their eggs on the seabed or aquatic vegetation creating dense egg carpets that are vulnerable to bottom trawling (Morrison et al., 1991; Watling and Norse, 1998). Since visual observations of spawning grounds in situ are scarce, spawning areas have been allocated using the position of young larvae (Frost and Diele, 2022). Following a stock collapse of the Down’s stock of Atlantic herring in 1955, which saw recovery only after a fishing ban was implemented from 1977 to 1980, commercial pelagic fisheries disappeared completely in the Belgian fishing fleet (Cushing, 1992; Lescrauwaet et al., 2010). Currently, Belgium exchanges its Atlantic herring fishing quota with the Netherlands and Germany in return for quotas on sole and Atlantic cod (Gadus morhua) (Departement Landbouw en Visserij, 2021).
Species distribution models use species field observations and environmental information to create species-environment relationships and infer the spatial distribution and ecological niche of a species. Outcomes quantify the habitat suitability for the species at each location, given the local environmental conditions. Previous studies by Turner et al. (2016) and Wang et al. (2018) modeled the distribution of adult Atlantic herring, while Aires et al. (2014) and Maravelias et al. (2000) modeled their spawning distribution. Of these studies, they either looked at different areas (Shetland Islands, Maravelias et al., 2000; Northeast US continental shelf, Turner et al., 2016 and Northwest Atlantic Shelf, Wang et al., 2018) or did not consider the monthly variation of their distribution (Aires et al., 2014; Maravelias et al., 2000). In addition to studying the monthly distributional variation (Turner et al., 2016), the current abundance of available data enables analysis of distributional variation across different years (Wang et al., 2018). Notably, comparable models for both adults and larvae are lacking. Generating two models with identical settings for these two life stages of herring facilitates the comparison of their seasonal distribution and their ecological tolerance to various environmental gradients within the research area.
The objective of this study is to model the spatiotemporal distribution of Atlantic herring in the Northeast Atlantic towards informing sustainable fisheries. Due to our focus on the Belgian fishing fleet, outcomes for the BPNS will be highlighted throughout the study. Furthermore, since Atlantic herring is prone to stock collapse, we aim to model their spawning distribution as well and compare the ecological needs of two life stages: adults and larvae. It is expected that this study will contribute to sustainable fisheries management by providing the time and location of Atlantic herring occurrences and by giving insight into the ecological needs of Atlantic herring. In the Northeast Atlantic, we hypothesize that both adults and larvae will have recurring annual distributional patterns due to their natal homing behavior (Geffen, 2009). Furthermore, we expect that larvae and adults will occur in the BPNS during November – January since they are known to spawn in the English Channel during this period (Limborg et al., 2012).
A total of 22176 occurrences of adult Atlantic herring were retrieved from the Database of Trawl Surveys (DATRAS) for the Northeast Atlantic (http://marineregions.org/mrgid/5664), restricted to 48°N – 62°N and 12°W – 10°E for all months for the years 2000 to 2020 (Figure 1, Supplementary Table 1; ICES, 2023e). This region spans about 1 367 600 km² of ocean and includes the English Channel, the North Sea, the Scottish Sea, the Irish Sea, the Celtic Sea and part of the North Atlantic Ocean. Following a general overview of the Northeast Atlantic, we specifically looked at the model outcomes for the BPNS, situated between 51°N – 52°N and 2°E – 4°E and spanning 3454 km² or 0.25% of the total area of the study area (https://marineregions.org/gazetteer.php?p=details&id=3293, Figure 1). Herring observations with a length below 20 cm, the approximate length when Atlantic herring become mature (Brevé et al., 2007), were discarded from the adult set. This way, in total 13112 occurrences of adult herring were available for the Northeast Atlantic in all months excluding April, May and June and this for a time frame of 21 years (i.e. years 2000 – 2020; Figure 1). Larval occurrence data of Atlantic herring was retrieved from the International Herring Larvae Surveys (IHLS) within the same spatiotemporal frame as adult occurrences (ICES, 2023f). In total 7902 larval observations were available for the North Sea and the English Channel in September, October, December, and January from 2000 to 2020 inclusive (Figure 1) with larval size ranging from 5 – 24 mm in length. Since these are the months when spawning occurs in the North Sea and the English Channel (Geffen, 2009), larval model outcomes were restricted to these months.
Figure 1. Study area with occurrences in green and background points in red of adult (A; ICES, 2023e) and larval herring (B; ICES, 2023f). Occurrences were compiled from the period 2000 – 2020 inclusive. In this period, larvae were present in September, October, December and January only, while adults were present in all months excluding April, May and June. Background points were restricted to the International Council for the Exploration of the Sea (ICES) statistical areas where occurrences are present. The location of geographic features that are used throughout the text are indicated in panel (C). Here, names of water bodies are denoted in blue and names of terrestrial areas in black or white. Projection: EPSG 4326/WGS 84.
Four steps were taken to transform the raw occurrence data into the final pre-processed occurrence dataset ready for analysis, being 1) conducting outlier analysis, 2) eliminating duplicated occurrences, 3) applying geographical filtering to address spatial bias and 4) applying environmental filtering to address spatial autocorrelation (SAC).
Outliers were defined as being farther away from other observations than 1.5 times the interquartile range of geographical distances (Yang et al., 2019) and were flagged using the cc_outl function from R-package CoordinateCleaner (Zizka et al., 2019). For the response variable, no outliers were identified, and all observations were kept. Next, duplicated occurrences per location (grid cell, 10 x 10 NM, see section 2.2) and time steps (months) were removed (Phillips, 2021).
To account for sampling bias in the compiled datasets of DATRAS and IHLS (Loiselle et al., 2008; Merow et al., 2013), we applied a filtering technique in geographical space as per Vollering et al. (2019). Occurrences were removed randomly until a dataset was retained where each pair of occurrences had a minimum distance of 10 nautical miles (NM, or 18.5 km), which is the recommended distance between valid hauls in the DATRAS trawl surveys (ICES, 2020). This filtering was done on the projected datum ETRS89-extended/LCC Europe (EPSG 3034), which covers the entire study area, using the R-package spThin (Aiello-Lammens et al., 2015).
Spatial autocorrelation (SAC), where locations close to each other are more similar than locations further apart, is common in spatial data. Spatial autocorrelation in model residuals violates the assumption that model residuals are independent and identically distributed (Legendre, 1993). Since initial results revealed SAC in the model residuals (tested using Moran’s I statistic from the ape package; Paradis and Schliep, 2019), SAC was reduced by an additional filtering technique in environmental space (de Oliveira et al., 2014). First, the environmental Mahalanobis distance was calculated between all observations using eight environmental variables (see section 2.2). Based on these environmental distances, the two most distant observations were selected and added to a new dataset. Subsequently, we iteratively added the observation that is most distant to this new dataset until we retained a new dataset of 400 occurrences. The objectively selected 400 occurrences minimized SAC while keeping enough observations to construct robust models. We applied this procedure to both adult and larval datasets, resulting in a total of 800 occurrences for calibrating and validating the two species distribution models (Figure 1, section 0).
Relevant environmental variables were selected through a literature review on the ecology of Atlantic herring and include bathymetry, sea surface temperature (SST) (Turner et al., 2016; Wang et al., 2018), sea surface salinity (SSS) (Aires et al., 2014), seabed substrate and energy (Brevé et al., 2007; Maravelias et al., 2000), sea surface phytoplankton concentration (Marshall et al., 1937), zooplankton concentration in the epipelagic layer (Maravelias et al., 2000; Van Ginderdeuren et al., 2014), and windfarm presence to include a measure of artificial coarse substrate (Frost and Diele, 2022). Substrate energy is a measure of the average hydrodynamics of the seabed by European Marine Observation and Data Network (EMODnet Seabed Habitats product). It has been found that a highly energetic seabed is important for Atlantic herring egg development (Haegele and Schweigert, 1985). Of all eight variables, bathymetry, seabed substrate and energy were considered static variables over time for each location, while the remaining variables were dynamic and derived monthly for 2000 – 2020. All environmental variables used were obtained from the European Marine Observation and Data Network (EMODnet) and the Copernicus Marine Service (CMEMS, Table 1). Zooplankton concentration was derived from CMEMS, with the units g C/m², representing the average biomass (expressed in carbon content) over the depth of the epipelagic layer (see also Global ocean low and mid trophic levels biomass content hindcast | Copernicus Marine Service).
Table 1. Selected environmental variables for modeling with the corresponding source, value range, data type, uni, original resolution, and DOI/URL.
Preprocessing of the environmental variables involved aggregation by averaging to match the coarser spatiotemporal resolution of the occurrence data (10 NM x 10 NM, monthly for 2000 – 2020 inclusive) (Sillero and Barbosa, 2021). Additionally, we calculated a measure of windfarm presence using a buffer of 200 m around active windfarms to indicate nearby windfarm presence since Atlantic herring are known to spawn on coarse substrate (Frost and Diele, 2022). To avoid adding highly correlated variables in the models, the Variance Inflation Factor (VIF) was calculated for each combination of variable pairs and a VIF larger than 10 was considered as a threshold for collinearity (Zuur et al., 2010). No correlated variable pairs were found and hence all variables were kept.
Since Atlantic herring is a mobile and migratory species, the absence of the fish at a location during a sampling event does not necessarily indicate that environmental conditions are not suitable at this location and time (Lobo et al., 2010). For this reason, we based our models on presence-background data instead of presence-absence data (Fernandez et al., 2022). Background points are defined as a general sample of the environmental conditions of the entire study area or a sample of all sites that are available for the species to occupy (Phillips et al., 2009). We developed Maximum entropy (Maxent) presence-background models, a machine learning model that is commonly used in species distribution modeling because it is flexible, simple to use and performs well (using R-package dismo; Hijmans et al., 2023) (Barber et al., 2022; Phillips et al., 2006; Valavi et al., 2023). Background points were sampled randomly in the study area, restricted to the ICES areas of the occurrences (Figure 1). The number of background points was set at 10 times the number of presences (Hysen et al., 2022). Maxent can be tailored by employing combinations of feature classes and regularization multipliers (Phillips et al., 2006). Feature classes are transformations that can be applied to each predictor variable by the model, for example, linear and quadratic transformations, while the regularization multiplier is a penalty to avoid overfitting (Merow et al., 2013). Fifteen combinations were tested using the corrected Akaike’s Information Criterion (AICc) as a selection criterion (R-package ENMeval; Kass et al., 2021) (Table 2A; Zeng et al., 2016). Following the recommendations of Merow et al. (2013), we included all combinations of the feature classes L, LQ and LQH (with L linear, Q quadratic and H hinge) and the regularization multipliers 1, 2, 4, 8 and 32. Finally, one model was retained for adult herring and one model for larvae. Next, habitat suitability maps were calculated per month and year in 2000 - 2020, by applying the models to each monthly map of the environmental variables in 2000 - 2020. Average and standard deviation maps were calculated per month. Model outcomes are shown in terms of habitat suitability indices (HSI). To enhance clarity, habitat suitability indices above 50% will be addressed as suitable and indices below 50% as unsuitable (Manel et al., 1999).
Table 2. Model evaluation using the area under the curve (AUC), true skill statistic (TSS), sensitivity and specificity.
The importance of each environmental variable in the model was evaluated using a bootstrapping method adopted by Thuiller et al. (2009). Hereby, the correlation was calculated between the original model prediction and a model prediction where one variable was randomly permuted. Following the approach of Thuiller et al. (2009), this calculation was repeated 50 times for each variable. The variable importance score was calculated as the mean correlation coefficient for each variable, normalized across all variables to collectively contribute to a total variable importance of 100%. Response plots were created that depict the modeled relationship between each environmental variable and the HSI. For each plot, HSI was simulated across 100 values over the range of the environmental variable, with other variables constant at their mean value (response.plot function from R-package Maxnet, Phillips, 2021).
Model performance was evaluated using the Area Under the Curve of the Receiver Operating Characteristic plot (AUC) and the True Skill Statistic (TSS) metrics (Báez et al., 2020; Liu et al., 2013). The AUC ranges from 0 to 1, whereby an AUC of 0.5 or lower indicates that the model is no better than random and 1 indicates perfect model performance; the TSS ranges from -1 to 1, whereby a random model would have a TSS of 0 or less, and a perfect model a TSS of 1. To have a full view of the various aspects of the model performance (Grimmett et al., 2020), the model sensitivity (ability to accurately predict presences) and specificity (ability to accurately predict background points) were also included as performance metrics. All four metrics (AUC, TSS, sensitivity and specificity) were calculated using k-fold cross-validation as follows: (1) the complete dataset was divided randomly into a training and a test set (training-test ratio of 80-20%), (2) a model was built on the training set and (3) the model performance was tested based on its ability to predict the test set. This process was repeated ten times.
The North Atlantic Oscillation (NAO) is an important climate process in the North Atlantic and affects ocean dynamics (Hurrell and Deser, 2010). Variations in the NAO index can have direct effects on the biology in the ocean (e.g., Alheit et al., 2005). For example, Corten (1999) found a link between the NAO and the occurrence of Atlantic herring in the Norwegian trench and Gröger et al. (2010) between the NAO and the number of Atlantic herring recruits in the North Sea. We correlated the seasonal and inter-annual variability of our models’ spatiotemporal HSI with NAO indices. The impact of the NAO varies across regions of the Northeast Atlantic (van der Molen and Pätsch, 2022). Therefore, a regional assessment was made, particularly for the focus area of this study: the BPNS.
To analyze the effect of the NAO on the HSI in the BPNS, the following method was adapted from the one used by Corten (1999): Monthly NAO indices from 2000 – 2020 were retrieved from the National Oceanic and Atmospheric Administration (NOAA, https://www.ncei.noaa.gov/access/monitoring/nao/) and seasonal averages were calculated. For each season, a separate time series was created. For example, for the winter, NAO indices of January, February and March were averaged for each year from 2000 until 2020. Next, based on our model outcomes, an average HSI was calculated for adults and larvae in the BPNS for each year in 2000 – 2020, using the months during which habitat was calculated to be most suitable in the BPNS (winter, specifically January – March and December – January for adults and larvae respectively, see section 3.4). As per Corten (1999), these NAO and HSI time series were smoothed using a running average. We used three smoothing windows: (1) non-smoothed indices, (2) a three-year average window and (3) a five-year average window. Finally, the autocorrelation was calculated by crossing NAO and HSI time series using the base R function ccf (R Core Team, 2023). This autocorrelation was calculated for each of the three smoothing windows and for each of the four seasonal NAO time series. Besides calculating autocorrelation, the ccf function was also used to detect if there is a lag between two correlated time series. The significance of the correlation coefficients was tested by calculating 99% confidence intervals using Fisher’s Z transformation for correlation coefficients (CorCI function from R-package DescTools; Signorell, 2024) (Zou, 2007).
After filtering, 400 adult and 400 larval Atlantic herring occurrences were retained for modeling. Of these, two adult occurrences and four larval occurrences were located in the BPNS. After filtering in geographical and environmental space, adult occurrences were present at a broad range of bathymetry (8 – 700 m), sea surface temperature (3 – 20°C) and sea surface salinity (29 – 35 PSU) and at zooplankton concentrations in the epipelagic layer of 0 – 12 g C m-2, sea surface phytoplankton concentrations of 0 – 11 mmol C m-3, all seabed energy classes (low, moderate and high) and above multiple seabed substrate classes (including both coarse and muddy classes and several categories in between). Larval occurrences for modeling were present at a narrower range of bathymetry (12 – 134 m), sea surface temperature (5 – 17°C), sea surface salinity (31 – 35 PSU), zooplankton concentrations in the epipelagic layer (1 – 10 g C m-2) and sea surface phytoplankton concentrations (0 – 8 mmol C m-3). Additionally, these occurrences were situated above all seabed energy levels and sandy and coarse substrate types.
The larval model performed best with an AUC of 0.9 and a TSS of 0.7 (Table 2). The adult model had lower performance metrics with an AUC of 0.7 and a TSS of 0.6. The adult model scored better at accurately predicting presences (model sensitivity) than background points (model specificity). Optimal Maxent model settings (minimal AICc) were obtained using feature classes linear, quadratic and hinge and a regularization multiplier of one for both adult and larval models. Filtering in environmental space was successful in reducing spatial autocorrelation but did not remove it completely (from I = 0.18 to I = 0.09 and from I = 0.16 to I = 0.14 for the adult and larval models, respectively). However, bootstrapping methods for variable importance ensured that selected variables were not selected due to type I errors derived from SAC in the residuals of the models.
Bathymetry was the most influential variables for both adults (63%) and larvae (37%) (Table 3). The dynamic variables SST, SSS, phyto- and zooplankton concentrations were important in both models but have a higher summed importance in the larval model (46%) compared to the adult model (35% in total). Seabed characteristics were important (18% in total) in the larval model only, while windfarm presence did not influence any model.
Response curves displayed distinct patterns for the adult and larval life stages (Figure 2). In general, adult Atlantic herring were inferred to have a capacity to withstand a broader range of environmental conditions than their larvae. For both larvae and adults, the habitat was most suitable at shallow depths with a decreasing HSI towards deeper depths. Adults were able to tolerate a wider bathymetrical range compared to larvae (HSI drops to 25% at 660 and 81 m respectively). Sea surface temperature was optimal at 5 and 7°C for adults and larvae respectively, situated at the lower end of the temperature range observed in the study area (Northeast Atlantic 2000 – 2020, 3 – 20°C). Adult herring tolerate a wide temperature range (3 – 15°C, HSI > 50%), while larvae favor a narrower temperature range (5 – 8°C). The response of suitability to salinity is low in the study area. The highest HSI (38%) were reached for adults at high salinity values around 35 PSU and at both ends of the salinity range for larvae (HSI > 50% at 29 – 35 PSU). Adult herring was more likely to be found in low concentrations of zooplankton in the pelagic layer (< 2.5 g C m-²), although other concentrations in the study area were not restrictive (HSI around 50%). For larvae, optimal zooplankton concentrations were quantified at 4.5 – 6.5 g C m-². On top of these environmental variables that were important in both adult and larval models, larval models were also influenced by seabed substrate and sea surface phytoplankton concentration (Table 3). Models suggested that larvae are more likely to be found above coarse substrate, sandy mud, and sand (HSI > 45%) than the unclassified group ‘seabed’ (unclassified in EMODnet Predictive Habitat Map for Europe), fine mud, and rock or other hard substrata (HSI < 20%). Finally, larvae were simulated to be found in the highest concentrations of phytoplankton in the study area (8 mmol C m-³).
Figure 2. Response curves relating the modeled habitat suitability index for Atlantic herring to the environmental variables for adult and larval life stages. Only environmental variables with a variable importance larger than 5% are shown. The range of the environmental values shown was restricted to the range of values of the variable where occurrences were present.
On average, adult Atlantic herring was projected to have a wide spatial distribution across the Northeast Atlantic throughout the entire year (Figure 3, left; see Supplementary Figure 1 for distribution maps for all months). Early in the year, habitat was suitable in the North Sea and around the Faroe Islands (HSI > 50%, Figure 3). No observations were present around the Faroe Islands, so this is an extrapolation of the model and should be interpreted with caution (Elith et al., 2010). In July, waters surrounding Ireland become suitable and in October, the English Channel was included as suitable waters for adult herring (HSI > 50%). Year-to-year variability of the habitat suitability was highest in the Celtic Sea in July and October (Figure 3, right). For example, the standard deviation peaked at 18% in October in the Celtic Sea.
Figure 3. Average HSI (left) and average year-to-year variability (given as standard deviation, right) of the habitat suitability for adult Atlantic herring for January, April, July and October (representing the four seasons) in 2000 – 2020. High values are indicated in red, and low values in blue. Areas where the model extrapolated, i.e., where no occurrences were present, are hatched. Projection: EPSG 4326/WGS 84. Distribution maps for all months are shown in Supplementary Figure 1.
Zooming in on the BPNS specifically, habitat was calculated to be suitable for adult Atlantic herring throughout the entire year with increasing values through autumn and peaking in winter (January, February, and March, Figure 4). More specifically, monthly averages never dropped below 25% HSI and the highest average HSI was 75% in February. The variability of the HSI was highest in March and April with HSI varying between 21 and 85% and 12 and 64%, respectively.
Figure 4. Monthly variability of the habitat suitability index for adult Atlantic herring in the Belgian Part of the North Sea, averaged over 2000 – 2020. For each month, the horizontal lines in the rectangular part of the boxplot represent, from low to high respectively, the 25th percentile, the 50th percentile and the 75th percentile. Points that fall outside of these ranges are shown by whiskers (vertical lines) that extend up to 1.5 times the interquartile range. Points falling outside of 1.5 times the interquartile range are shown as dots.
For larvae of Atlantic herring, a gradual southward movement of suitable areas (HSI > 50%) can be seen in the distribution maps from September to January in the Northeast Atlantic (Figure 5). In September and October, high habitat suitability was calculated surrounding Ireland and the United Kingdom. Later, in December and January, areas with high habitat suitability occurred in the Celtic Sea and the English Channel (including the BPNS, HSI up to 85%, Figure 5). Year-to-year variability was higher in larval HSI than adult HSI (Figure 3 right vs. Figure 5 right) and reached up to a standard deviation of 30% in all months (Figure 5, right). In the BPNS, this variation showed an oscillating pattern, as visualized using boxplots in Figure 6.
Figure 5. Average HSI (left) and average year-to-year variability (given as standard deviation, right) of the habitat suitability for larvae of Atlantic herring for September, October, December and January (months where data was available) in 2000 – 2020. High values are indicated in red, and low values in blue. Areas where the model extrapolated, i.e., where no occurrences were present, are hatched. Projection: EPSG 4326/WGS 84.
Figure 6. Yearly variability of habitat suitability for larvae of Atlantic herring in January in the Belgian Part of the North Sea. For each month, the horizontal lines in the rectangular part of the boxplot represent, from low to high respectively, the 25th percentile, the 50th percentile and the 75th percentile. Points that fall outside of these ranges are shown by whiskers (vertical lines) that extend up to 1.5 times the interquartile range. Points falling outside of 1.5 times the interquartile range are shown as dots.
The presence of Atlantic herring did not only show seasonal patterns (section 3.4) but also patterns across years. The HSI in the BPNS was negatively correlated with the NAO. More specifically and using a confidence interval of 99%, a significant correlation coefficient was found between winter NAO and winter HSI of adults (correlation of -0.57) at a moving average window of one year. At a moving average window of three years, significant correlation coefficients were found between autumn NAO and the winter HSI (-0.83 and -0.63 for adults and larvae respectively) and winter NAO and winter HSI (-0.83 and -0.76 for adults and larvae respectively). At a moving average of five years, both the autumn NAO and winter HSI (-0.86 and -0.63 for adults and larvae respectively) and the winter NAO and winter HSI were significantly correlated (-0.88 and -0.78 for adults and larvae respectively). For all correlations, the time lag was either zero (winter NAO – winter HSI) or one season (autumn NAO – winter HSI), but no autocorrelations with a time lag of (over) a year were seen. For example, the effect of the winter NAO on the winter HSI for adults using a three-year averaging window is shown in Figure 7. In the winter of 2010 in the BPNS, the HSI for adults was simulated high (0.8) coinciding with low average NAO indices (-0.7). In contrast in the winter of 2015, the HSI was simulated relatively low (0.6) during high average NAO indices (0.8). The same pattern can be seen for the other significant combinations from above between autumn/winter NAO and modeled HSI for adults/larvae (Supplementary Figures 5–7).
Figure 7. Effect of the North Atlantic Oscillation (NAO) on modeled habitat suitability indices (HSI) of adults in the Belgian Part of the North Sea during winter. (A) time series of 3-year averaged winter HSI (left y-axis) and winter NAO (in red, right y-axis). (B) Correlation plot between three-year averaged winter NAO and winter HSI.
This is one of the first studies making dynamic species distribution models for both adults and larvae of Atlantic herring in the Northeast Atlantic. Model outcomes suggest that suitable habitat for adult herring is widely spread over the Northeast Atlantic throughout the entire year (Figure 3). Suitable habitat for larvae occurs first in the North of the UK in September and moves gradually southward towards the English Channel throughout the spawning season (Figure 5). Focusing on the BPNS provided valuable insights for the development of spatiotemporally specific management strategies for fisheries on Atlantic herring by the Belgian fishing fleet. In this area, both adults and larvae are most likely to occur during winter months (adults: January – March; larvae: December – January).
Bathymetry was the main explanatory variable in the adult model, followed by sea surface temperature and salinity (variable importance of 63, 13 and 11% respectively, Table 3). Response curves showed that habitat was suitable at depths shallower than 200 m, aligning with the region of the European continental shelf of which the edge is situated at approximately 200 m depth (Figure 2, Ricker and Stanev, 2020). The importance of bathymetry is assumed to be related to the Atlantic herring’s adaptability to a wide range of temperatures and salinities (de Groot, 1980; Whitehead, 1985). This was also reflected in the response curves for sea surface temperature and salinity, being able to tolerate the entire range of values over 21 years in the study area (Figure 2).
To model the distribution of larvae, apart from bathymetry, sea surface temperature and salinity, also seabed substrate, zooplankton and phytoplankton concentration were important variables (37, 15, 5, 14, 13 and 13% variable importance respectively, Table 3). In terms of abiotic factors, response curves matched well with known characteristics of Atlantic herring spawning sites for the bathymetric range (7 – 150 m, Figure 2; Brevé et al., 2007; Dickey-Collas et al., 2004; Frost and Diele, 2022), substrate (sandy and course; de Groot, 1980) and salinity (both marine and brackish; Frost and Diele, 2022). Modeled temperature ranges (optimum at 7°C) also agree well with historical catch data from the North Sea (8°C; Hay et al., 2000). The inferred inclination of Atlantic herring towards colder water temperatures suggests that global warming may disrupt their current migration patterns, potentially leading to a shift towards more northern spawning grounds. However, the visual feeding behavior of herring, dependent on light for hunting (Blaxter, 1968), may hinder a northward shift during the short daylengths in northern winters, as suggested by Hufnagl and Peck (2011). Spawning sites are often classified as high-energy environments, by wave or tidal movement, which is important for egg development (Haegele and Schweigert, 1985). Seabed energy was included for modeling, but no effect was found.
A remarkable difference between the adult and the larval model was that prey concentration was important to model the distribution of larvae (26% variable importance in total including both phyto- and zooplankton concentration) while being less important to model the distribution of adults (11% variable importance in total, Table 3). An explanation could be that the adult model includes data on the complete cycle of their migration, including feeding and spawning grounds (de Groot, 1980; Coull et al., 1998). Therefore, the effect of feeding might be partly obscured. Furthermore, even though adult herring may be drawn to areas with high concentrations of zooplankton, their top-down influence could lead to lower concentrations of phyto- and zooplankton in those specific locations. Supporting both scenarios, Atlantic herring has previously been reported directly inside or at the edges of plankton patches (Maravelias, 2001).
For larvae, habitat suitability increased with increasing phytoplankton concentration and was optimal at zooplankton concentration in the epipelagic layer between 4.5 and 6.5 g C m-². This aligns with the fundamental physiological need of early life stages, as young herring prioritize somatic growth. Phytoplankton serves as the base of the food web, and the availability of zooplankton, which feeds on phytoplankton, becomes crucial for larval development. A lack of sufficient prey during these early stages can hinder growth and survival, as larvae rely on abundant food sources to sustain their rapid growth during early life (Fletcher et al., 2019). For example, for an epipelagic layer depth of 81 m (the average epipelagic layer depth in the Northeast Atlantic at noon, 01/01/2020, https://doi.org/10.48670/moi-00020), this would correspond to 55.6 – 80.2 mg C m-3. Spawning grounds of Atlantic herring are chosen to promote larval retention (Sinclair and Power, 2015) and longer retention times near spawning grounds can lead to higher recruitment of Down’s herring (Dickey-Collas et al., 2009). On top of this, Hufnagl and Peck (2011) reported that the duration of the hatching period is influenced by minimum prey concentration and prey size. Integrating these findings with our modeling results suggests that optimal spawning occurs in specific spawning grounds that promote retention and where an ample supply of prey is available. Our modeled outcomes suggest optimal prey concentrations for larval herring in the Northeast Atlantic.
The inclusion of nearby wind farm presence as a predictive variable to model the distribution of Atlantic herring is novel. During the construction of offshore wind farms, no spatial deterrence was found for free-ranging pelagic fish (Hubert et al., 2024). Offshore wind farms with scour protection introduce artificial hard substrates on the seabed and can attract demersal benthopelagic fish species by providing shelter, food sources and spawning sites (Degraer et al., 2018). To date, the spawning of Atlantic herring on windfarm substrate has not been observed, nor has our model detected any effect of nearby windfarm presence on the occurrence of Atlantic herring. However, the potential for wind farms to serve as artificial reefs could offer some benefits, particularly for larvae and early life stages. Artificial reefs have been shown to enhance local biodiversity by providing shelter, feeding opportunities, and protection from predators (Higgins et al., 2022). For herring larvae, the structural complexity of wind farms could increase prey availability and offer protection from predation, supporting their early development. However, the effect might be obscured by (1) inadequate sampling near windfarms due to fishing restrictions (Bonsu et al., 2024) or (2) the dispersal of larvae away from spawning sites following hatching (Sinclair and Power, 2015).
Adding on to the specific spawning requirements, larval habitat suitability indices had a larger year-to-year variability than adult HSI (up to 30 and 18% standard deviation respectively). This variability was found to be correlated with the NAO index. More specifically, positive autumn and winter NAO indices had a negative effect on adult and larval occurrence in the BPNS during winter. The NAO index represents an atmospheric sea level pressure difference between the Azores, Portugal and Iceland (Rogers, 1984). These pressure differences result in temporal variation of storms, precipitation, temperature, salinity, mixed-layer depth and circulation patterns (Hurrell and Deser, 2010). From the 1960s until the early 1990s a general positive trend of winter NAO indices has been observed and afterwards, the trend was less positive or even negative (Gulev et al., 2021). Climate models forecast a slight increase in the winter NAO in the future, with large natural variations (Lee et al., 2021).
Positive NAO indices and associated westerly winds lead to increased inflow of Atlantic waters in the North Sea which can increase nutrient concentrations and temperatures (van der Molen and Pätsch, 2022). Additionally, a stronger outflow of Baltic waters under positive NAO indices leads to a reduced exchange between the northern and southern North Sea (Salt et al., 2013). Considering the modeled response curves for temperature for both life stages of herring, the increase in temperature during positive NAO indices might have a direct impact on their physiology. Furthermore, the reduced exchange will affect the plankton distribution, which might affect herring. Investigating a correlation between temperature and Atlantic herring spawning stock biomass, Akimova et al. (2016) employed comparable reasoning. They suggested that the correlation was more likely attributed to fluctuations in the zooplankton composition than a direct impact of water temperature on larval growth rates. However, they were not able to find a match between the time series of the spawning stock biomass of Atlantic herring and zooplankton species in the North Sea. Finally, the impact of large-scale climate processes, including the NAO, on North Sea herring stock was modeled by Gröger et al. (2010). Gröger et al. (2010) did not find any correlation between the NAO and herring spawning stock biomass but did find a correlation with the number of recruits at a time lag of 5 years. Here they defined the number of recruits as the number of fish at 1 year of age (about 10 cm in length, Brevé et al., 2007), which is a different age group from the larvae used for modeling in our study (0.5 – 2.4 cm length). The age difference can partly explain the time lag seen in the study of Gröger et al. (2010), a phenomenon we did not observe in our study. On top of this, Gröger et al. (2010) looked at a different spatial scale than we did (North Sea vs. BPNS) and the effect of the NAO differs regionally (van der Molen and Pätsch, 2022). Since Atlantic herring is a key species in the North Sea food web (Fauchald et al., 2011) and is impacted by both top-down and bottom-up processes (Lynam et al., 2017), the net effect of NAO on the larval occurrence of herring in the BPNS is probably a combination of different effects. We recommend future ecological modeling work to focus on integrated approaches that include environmental variables, food web interactions, and climate processes and consider both the effects of space and time.
Models performed well in terms of AUC and TSS values. Performance of the larval model was higher (AUC of 0.89 and TSS of 0.71) compared to the adult model (AUC of 0.73 and TSS of 0.61). To get a comprehensive understanding of the model’s strengths and weaknesses, Grimmett et al. (2020) emphasize the importance of using multiple performance statistics alongside commonly used metrics like AUC. Specifically, the sensitivity and the specificity give information on the model’s capability of predicting presences and background points, respectively. The adult model showed a better performance in predicting suitable habitat (sensitivity of 0.78), compared to unsuitable habitat (specificity of 0.68). The lower specificity suggests that the actual species range might be more confined than what the models projected.
The higher performance of the larval model compared to the adult model indicates that larvae exhibit more characteristics of habitat specialists than the generalist adults. Habitat specialists, characterized by narrow environmental tolerances, are often more straightforward to model compared to habitat generalists (Brotons et al., 2004; Elith et al., 2006). In modeling terms, this can be explained by the selection of background points, which are more likely to be true absences for a species with a narrow spatial distribution (habitat specialist) compared to a widely distributed species (habitat generalist; Grimmett et al., 2020; Lobo et al., 2008). Fernandez et al. (2022) suggest adopting the non-observation of highly mobile species in dynamic environments (such as the ocean) as part of the study area background, rather than treating it as an absence. Building upon this recommendation, we opted for Maxent models, which integrate non-observations as background points.
One of the limitations of this study is the reliance on occurrence data rather than abundance data to model Atlantic herring distribution. While occurrence-based models such as Maxent are valuable for capturing species-environment relationships, they do not account for the density or biomass of herring, which is essential for effective fisheries management. Species that are found in high numbers in specific locations can play a significant role in ecosystem dynamics, and focusing solely on occurrence data may miss capturing these ecological interactions. On the other hand, incorporating abundance data poses challenges of its own. Abundance data can be influenced by biases stemming from differences in sampling methods, spatial and temporal coverage, and variable sampling efforts, leading to difficulties in interpreting true species-environment relationships (Bonar et al., 2011). Factors like fishing gear types and tactics can strongly impact the perceived abundance of species in a particular area, introducing inaccuracies in data analysis (Mehdi et al., 2021; Moriarty et al., 2020). Additionally, the migratory nature and aggregative behavior of Atlantic herring makes it challenging to gather consistent and comprehensive abundance data.
While abundance data was available for adult herring, we opted not to use it. The ICES surveys are compiled of different surveys of different countries (Supplementary Table 1). On top of this, no abundance data was available for larvae. Using the same form of input data (occurrences data) for both life stages allowed for comparison of the ecological needs for the two life stages. While this approach may not fully capture the ecological dynamics of Atlantic herring, it provides a reliable and expansive dataset for evaluating habitat suitability over large spatial extents. Occurrence data is also noted to enhance the performance of species distribution models, particularly for migratory species or those with extensive ranges. Despite the advantages of using occurrence data, it is essential to recognize the limitations of this approach for fisheries management and consider integrating abundance data where feasible to gain a more nuanced insight into herring distribution and its implications for fisheries.
We acknowledge that the outcomes of our model may be partly biased due to the use of demersal input data to model pelagic adult herring (Brevé et al., 2007; ICES, 2023e). Demersal sampling is likely to miss some occurrences of herring when they are swimming in the upper water column. Since the Maxent model does not consider non-observations as true absences, but rather as a part of the background, this model could be more robust against such biases (Fernandez et al., 2022). Additionally, the DATRAS trawl surveys, which are compiled from different surveys organized by multiple countries, involve varying sampling depth ranges (Supplementary Table 1). This variation can introduce bias into the model output, leading to instances such as the unsuitability of deeper waters in the Norwegian Trench (Figure 3). The absence of occurrences at these depths is reflected in the model as being unsuitable, despite evidence that spring-spawning Atlantic herring are present in this region during winter (Corten, 2000). To address these biases, we have highlighted areas where the model extrapolates beyond the spatial range of observations in Figure 3 and Figure 5. Moreover, to the best of our knowledge, we have used the most reliable dataset currently available for our study area. Fisheries independent datasets, such as the DATRAS trawl surveys, are often preferred over fishery dependent data because they follow a recurring sampling scheme with sufficient spatiotemporal coverage (Hilborn and Walters, 2013). Finally, other studies such as Turner et al. (2016) and Wang et al. (2018) have also employed demersal surveys to develop species distribution models for Atlantic herring with good model prediction accuracies (AUC values > 0.75).
A visual comparison shows a good match of our spatiotemporal habitat suitability maps of larval herring with the location and timing of known spawning grounds (Figure 5 and Supplementary Figure 8). Spawning time is used to distinguish different autumn-spawning stocks (Heath et al., 1997). In the North Sea, spawning starts in August around the Shetland Islands, Orkney Islands and west of Scotland and ends in January in the southern North Sea (Figure 7; Coull et al., 1998; Gröger et al., 2010). On the east of the UK, model outcomes (Figure 5) correspond accurately with the Shetland stock in September, the Buchan stock in September – October, the Banks stock in October – December and the Downs stocks in December – January (Figure 7). No observations were found to include in the model from the west side of the UK, however, the model was able to extrapolate larval habitat preferences to these areas as well. These extrapolations accurately show the spawning grounds west of Scotland and Ireland during September and October. Spawning grounds in the east of Ireland (October – December – January) and in the English Channel (December – January) were predicted wider than the findings from Coull et al. (1998) (Figures 5 , 7). These authors stress that the location of spawning grounds should be under continuous revision. The precise location might be blurred in our model due to the lack of direct spawning ground observations since larvae can show some degree of dispersal from spawning grounds through local currents (Bauer et al., 2014; Funk et al., 2001; Sinclair and Power, 2015).
The adult model forecasts a wide distribution of Atlantic herring across the Northeast Atlantic throughout the entire year (Figure 3). Over different seasons, some regional differences could be seen. The most suitable areas were centered in the North Sea and around the Faroe Islands in the first half of the year to an even wider area including the east of the UK during the second half of the year.
The Belgian fishing fleet has witnessed a steady decline in number of catches and number of fishing vessels in the southern North Sea (Maertens, 2022) and this decline might be aggravated when the effects of Brexit come fully into force (Popescu and Scholaert, 2022). This study aimed to provide information on the location of Atlantic herring as a first assessment towards restoring pelagic fishing for the Belgian fishing fleet. Note that our models predict environmentally suitable areas where herring can be found during different seasons, but they do not provide any information on their biomass at those locations. The outcomes of the adult model show that Atlantic herring is likely to occur in the Greater North Sea throughout the entire year. For the Belgian fishing fleet, fishing directly in the BPNS would incur the lowest cost for ship operation. Here, areas of high habitat suitability for Atlantic herring were simulated in December – January for larvae and later, in January – February, for adults. The habitat in the BPNS being suitable for larvae first, before adults, could indicate that early spawning takes place outside of the BPNS in November – December and that, following hatching, larvae could be transported towards the BPNS through eastward local currents (Turrell, 1992). Sinclair and Power (2015) found that Atlantic herring choose their spawning sites to limit larval transport and hence spawning might occur nearby, likely in the Downs and Banks stocks. Later, in December – January adults arrive in the BPNS and spawning might occur on the sandbanks and gravel grounds of the BPNS itself.
Given these outcomes, pelagic fishing on adult Atlantic herring in the BPNS would be most suitable during winter months. However, due to the potential presence of spawning nearby and herring’s susceptibility to collapse (Stephenson et al., 2001), fisheries must be managed effectively. Bottom trawling can have a direct negative impact on deposited eggs (Watling and Norse, 1998). Atlantic herring are caught using different types of gear including purse seine, mid-water trawl, pair trawl and otter trawl (ICES, 2005). Therefore, if fishing near spawning areas would be permitted, fisheries should at least consider employing non-bottom-stirring techniques.
Our study showed the widespread spatiotemporal distribution of Atlantic herring in the Northeast Atlantic, using species distribution models (AUC of 0.7). Models based on larval data were effective in deriving the Atlantic herring spawning distribution (AUC of 0.9). For the BPNS, outcomes show that Atlantic herring is likely to be present during winter months, both as adults and larvae. The year-to-year variability of habitat suitability during these months in the BPNS was negatively correlated (up to - 0.88) with the autumn and winter NAO indices. Positive NAO events might negatively impact spawning success through increased temperature and changes in prey composition.
Publicly available datasets were analyzed in this study. This data can be found here: https://datras.ices.dk/home/descriptions.aspx#FRA; https://www.ices.dk/data/data-portals/Pages/DATRAS.aspx; https://emodnet.ec.europa.eu/en/bathymetry; https://emodnet.ec.europa.eu/en/seabed-habitats; https://emodnet.ec.europa.eu/en/human-activities; https://doi.org/10.48670/moi-00021; https://doi.org/10.48670/moi-00021; https://doi.org/10.48670/moi-00058; https://doi.org/10.48670/moi-00020. All datasets and scripts of this study can be found in the Marine Data Archive (MDA), https://doi.org/10.14284/657 (under Creative Commons Attribution 4.0 International License).
WS: Writing – review & editing, Writing – original draft, Visualization, Validation, Software, Methodology, Investigation, Formal analysis, Data curation, Conceptualization. RM: Writing – review & editing, Methodology, Investigation, Formal analysis, Data curation, Conceptualization. MS: Writing – review & editing, Conceptualization. JG: Writing – review & editing, Resources, Data curation. CM: Writing – review & editing, Project administration, Funding acquisition. ED: Writing – review & editing, Project administration, Funding acquisition. SP: Writing – review & editing, Conceptualization. GE: Writing – review & editing, Supervision, Project administration, Methodology, Investigation, Formal analysis, Conceptualization.
The author(s) declare financial support was received for the research, authorship, and/or publication of this article. This work was supported by the European Commission’s Brexit Adjustment Reserve (BAR) – Veerkracht voor de kustvloot en klein vlootsegment post-Brexit (project No 1654) and the EU Public Infrastructure for the Digital Twin of the Ocean (EDITO-Infra) (project No 101101473).
The authors would like to thank Damian Villagra Villanueva (Flanders Research Institute for Agriculture, Fisheries and Food) and Ruben Perez Perez (Flanders Marine Institute) for their vital help with the data retrieval of the DATRAS and OBIS occurrence data. Furthermore, we would like to thank Dr. Leandro Ponsoni (Flanders Marine Institute) for his input on the decomposition of the NAO effect. This study has been conducted using E.U. Copernicus Marine Service Information; https://doi.org/10.48670/moi-00021, https://doi.org/10.48670/moi-00058, https://doi.org/10.48670/moi-00020. During the preparation of this work, the authors used ChatGPT 3.5 and Grammarly to improve readability and language. After using this tool/service, the authors reviewed and edited the content as needed and take full responsibility for the content of the publication.
The authors declare that the research was conducted in the absence of any commercial or financial relationships that could be construed as a potential conflict of interest.
All claims expressed in this article are solely those of the authors and do not necessarily represent those of their affiliated organizations, or those of the publisher, the editors and the reviewers. Any product that may be evaluated in this article, or claim that may be made by its manufacturer, is not guaranteed or endorsed by the publisher.
The Supplementary Material for this article can be found online at: https://www.frontiersin.org/articles/10.3389/fmars.2024.1485161/full#supplementary-material
Aiello-Lammens M. E., Boria R. A., Radosavljevic A., Vilela B., Anderson R. P. (2015). spThin: an R package for spatial thinning of species occurrence records for use in ecological niche models. Ecography 38, 541–545. doi: 10.1111/ecog.01132
Aires C., Manuel González-Irusta J., Watret R. (2014). Updating fisheries sensitivity maps in British waters. UK: Scottish Marine and Freshwater Science.
Akimova A., Núñez-Riboni I., Kempf A., Taylor M. H. (2016). Spatially-resolved influence of temperature and salinity on stock and recruitment variability of commercially important fishes in the North Sea. PloS One 11. doi: 10.1371/journal.pone.0161917
Alheit J., Möllmann C., Dutz J., Kornilovs G., Loewe P., Mohrholz V., et al. (2005). Synchronous ecological regime shifts in the central Baltic and the North Sea in the late 1980s., in. ICES J. Mar. Sci. 62, 1205–1215. doi: 10.1016/j.icesjms.2005.04.024
Báez J. C., Barbosa A. M., Pascual P., Ramos M. L., Abascal F. (2020). Ensemble modeling of the potential distribution of the whale shark in the Atlantic Ocean. Ecol. Evol. 10, 175–184. doi: 10.1002/ece3.5884
Barber R. A., Ball S. G., Morris R. K. A., Gilbert F. (2022). Target-group backgrounds prove effective at correcting sampling bias in Maxent models. Divers. Distrib 28, 128–141. doi: 10.1111/ddi.13442
Bauer R. K., Gräwe U., Stepputtis D., Zimmermann C., Hammer C. (2014). Identifying the location and importance of spawning sites of Western Baltic herring using a particle backtracking model. ICES J. Mar. Sci. 71, 499–509. doi: 10.1093/icesjms/fst163
Blaxter J. H. S. (1968). Visual thresholds and spectral sensitivity of herring larvae. J. Exp. Biol. 48, 39–53. doi: 10.1242/jeb.48.1.39
Bonar S., Fehmi J., Mercado-Silva N. (2011). “An onverview of sampling issues in species diversity and abundance surveys,” in Biological Diversity Frontiers in Measirement and Assessment. (UK: Oxford University Press Oxford), 11–27.
Bonsu P. O., Letschert J., Yates K. L., Svendsen J. C., Berkenhagen J., Rozemeijer M. J. C., et al. (2024). Co-location of fisheries and offshore wind farms: Current practices and enabling conditions in the North Sea. Mar. Policy 159, 105941. doi: 10.1016/j.marpol.2023.105941
Brevé N. P. W., van Emmerik W. A. M., van Beek G. C. W. (2007). Kennisdocument Atlantische haring. Available online at: http://www.clupea.net (Accessed May 19, 2023).
Brotons L., Thuiller W., Araújo M. B., Hirzel A. H. (2004). Presence-absence versus presence-only modelling methods for predicting bird habitat suitability. Ecography 27, 437–448. doi: 10.1111/j.0906-7590.2004.03764.x
Corten A. (1999). A proposed mechanism for the Bohuslän herring periods. ICES J. Mar. Sci. 56, 207–2020. doi: 10.1006/jmsc.1998.0429
Corten A. (2000). A possible adaptation of herring feeding migrations to a change in timing of the Calanus finmarchicus season in the eastern North Sea. ICES J. Mar. Sci. 57, 1261–1270. doi: 10.1006/jmsc.2000.0812
Coudyser C. (2021). Eerste gevolgen van Brexit voor Vlaamse visserijsector worden duidelijk. Available online at: https://www.cathycoudyser.be/nieuws/eerste-gevolgen-van-brexit-voor-de-vlaamse-visserijsector-worden-duidelijk (Accessed May 22, 2024).
Coull K. A., Johnstone R., Rogers S. I. (1998). Fishery sensitivity maps in british waters. (UK: UKOOA Ltd).
Cushing D. H. C. (1992). A short history of the Downs stock of herring. Available online at: https://academic.oup.com/icesjms/article/49/4/437/653339 (Accessed January 10, 2024).
Degraer S., Brabant R., Rumes B., Vigin L. (2018). Environmental impacts of offshore wind farms in the belgian part of the north sea: assessing and managing effect spheres of influence. Available online at: https://www.researchgate.net/publication/328095905 (Accessed June 1, 2023).
de Groot S. J. (1980). The consequences of marine gravel extraction on the spawning of herring, Clupea harengus. J. Fish Biol. 16, 605–611. doi: 10.1111/j.1095-8649.1980.tb03739.x
de Oliveira G., Rangel T. F., Lima-Ribeiro M. S., Terribile L. C., Diniz-Filho J. (2014). Evaluating, partitioning, and mapping the spatial autocorrelation component in ecological niche modeling: A new approach based on environmentally equidistant records. A. F.Ecography 37, 637–647. doi: 10.1111/j.1600-0587.2013.00564.x
Departement Landbouw en Visserij (2021). Visserijrapport 2020 (Brussels: Departement Landbouw en Visserij).
Dickey-Collas M., Bolle L. J., Van Beek J. K. L., Erftemeijer P. L. A. (2009). Variability in transport of fish eggs and larvae. II. effects of hydrodynamics on the transport of Downs herring larvae. Mar. Ecol. Prog. Ser. 390, 183–194. doi: 10.3354/meps08172
Dickey-Collas M., Van Beek D. F. A., Wot H., Voor Visserijonderzoek C. (2004). The current state of knowledge on the ecology and interactions of North Sea Herring within the North Sea ecosystem. (Ijmuiden, Netherlands: Stichting DLO, Centre for Fishery Research).
Elith J., Graham H., C. P., Anderson R., Dudík M., Ferrier S., et al. (2006). Novel methods improve prediction of species' distributions from occurrence data. Ecography 29, 129–151. doi: 10.1111/j.2006.0906-7590.04596.x
Elith J., Kearney M., Phillips S. (2010). The art of modelling range-shifting species. Methods Ecol. Evol. 1, 330–342. doi: 10.1111/j.2041-210x.2010.00036.x
European Commission (2020). Proposal for a regulation of the European Parliament and of the Council establishing the Brexit Adjustment Reserve. Available online at: https://commission.europa.eu/publications/brexit-adjustment-reserve_enfiles (Accessed February 14, 2024).
Fauchald P., Skov H., Skern-Mauritzen M., Johns D., Tveraa T. (2011). Wasp-Waist interactions in the North Sea ecosystem. PloS One 6. doi: 10.1371/journal.pone.0022729
Fernandez M., Sillero N., Yesson C. (2022). To be or not to be: the role of absences in niche modelling for highly mobile species in dynamic marine environments. Ecol. Modell 471. doi: 10.1016/j.ecolmodel.2022.110040
Fletcher C., Collins S., Nannini M., Wahl D. (2019). Competition during early ontogeny: Effects of native and invasive planktivores on the growth, survival, and habitat use of bluegill. Freshw. Biol. 64, 697–707. doi: 10.1111/FWB.13255
Frost M., Diele K. (2022). Essential spawning grounds of Scottish herring: current knowledge and future challenges. Rev. Fish Biol. Fish 32, 721–744. doi: 10.1007/s11160-022-09703-0
Funk F., Blackburn J., Hay D., Paul A. J., Stephenson R., Toresen R., et al. (2001). Herring: expectations for a new millennium (Anchorage, Alaska, USA: University of Alaska Sea Grant).
Geffen A. J. (2009). Advances in herring biology: from simple to complex, coping with plasticity and adaptability. Available online at: https://academic.oup.com/icesjms/article/66/8/1688/672963 (Accessed June 1, 2023).
Grimmett L., Whitsed R., Horta A. (2020). Presence-only species distribution models are sensitive to sample prevalence: Evaluating models using spatial prediction stability and accuracy metrics. Ecol. Modell 431. doi: 10.1016/j.ecolmodel.2020.109194
Gröger J. P., Kruse G. H., Rohlf Gröger N. (2010). Slave to the rhythm: how large-scale climate cycles trigger herring (Clupea harengus) regeneration in the North Sea. Available online at: https://academic.oup.com/icesjms/article/67/3/454/732742 (Accessed February 1, 2024).
Gulev S. K., Thorne P. W., Ahn J., Dentener F. J., Domingues C. M., Gerland S., et al. (2021). “Changing state of the climate system,” in Climate change 2021 – the physical science basis. Eds. Masson-Delmotte V., Zhai P., Pirani A., Connors S. L., Péan C., Berger S. (Cambridge, UK: Cambridge University Press), 287–422. doi: 10.1017/9781009157896.004
Haegele C. W., Schweigert F. (1985). Distribution and characteristics of herring spawning grounds and description of spawning behavior. Can. J. Fisheries Aquat. Sci. 42, s39–s55. Available at: www.nrcresearchpress.com.
Hay D. E., Toresen R., Stephenson R., Thompson M., Claytor R., Funk F., et al. (2000). Herring: expectations for a new millennium taking stock: an inventory and review of world herring stocks in 2000. (USA: University of Alaska Sea Grant College Program).
Heath M. (1993). An evaluation and review of the ICES Herring Larval Surveys in the North Sea and adjacent waters. Bull. Mar. Sci. 53, 993.
Heath M., Scott B., Bryant A. D. (1997). Modelling the growth of herring from four different stocks in the North Sea. J. Sea Res. 38, 413–436. doi: 10.1016/S1385-1101(97)00045-2
Higgins E., Metaxas A., Scheibling R. E. (2022). A systematic review of artificial reefs as platforms for coral reef research and conservation. PloS One 17, e0261964. doi: 10.1371/journal.pone.0261964
Hijmans R. J., Phillips S., Leathwick J., Elith J. (2023). dismo: species distribution modeling. Available online at: https://CRAN.R-project.org/package=dismo (Accessed October 4, 2023).
Hilborn R., Walters C. J. (2013). Quantitative fisheries stock assessment: choice, dynamics and uncertainty (Berlin/Heidelberg, Germany: Springer Science & Business Media).
Hubert J., Demuynck J. M., Remmelzwaal M. R., Muñiz C., Debusschere E., Berges B., et al. (2024). An experimental sound exposure study at sea: No spatial deterrence of free-ranging pelagic fish. J. Acoust Soc. Am. 155, 1151–1161. doi: 10.1121/10.0024720
Hufnagl M., Peck M. A. (2011). Physiological individual-based modelling of larval Atlantic herring (Clupea harengus) foraging and growth: Insights on climate-driven life-history scheduling. ICES J. Mar. Sci. 68, 1170–1188. doi: 10.1093/icesjms/fsr078
Hurrell J. W., Deser C. (2010). North Atlantic climate variability: The role of the North Atlantic Oscillation. J. Mar. Syst. 79, 231–244. doi: 10.1016/j.marsys.2008.11.026
Hysen L., Nayeri D., Cushman S., Wan H. Y. (2022). Background sampling for multi-scale ensemble habitat selection modeling: Does the number of points matter? Ecol. Inform 72. doi: 10.1016/j.ecoinf.2022.101914
ICES (2005). ICES FishMap species factsheet: Herring. In: ICES-fishMap. Available online at: https://www.ices.dk/about-ICES/projects/EU-RFP/EU%20Repository/ICES%20FIshMap/ICES%20FishMap%20species%20factsheet-herring.pdf (Accessed January 23, 2024).
ICES (2020). “Manual for the north sea international bottom trawl surveys,” in Series of ICES survey protocols, vol. 10. . doi: 10.17895/ices.pub.7562
ICES (2023a). “Herring (Clupea harengus) in division 7.a north of 52°30’N (Irish sea),” in Report of the ICES advisory comitte. ICES advice 2023, her.27.nirs. doi: 10.17895/ices.advice.23608098
ICES (2023b). “Herring (Clupea harengus) in divisions 6.a South of 56°00’N and West of 07°00’W and 7.b-c (Northwest and West of Ireland),” in Report of the ICES advisory comitte. ICES advice 2023, her.27.6aS7bc. doi: 10.17895/ices.advice.21907953
ICES (2023c). “Herring (Clupea harengus) in divisions 7.a South of 52°30’N, 7.g-h, and 7.j-k (Irish Sea, Celtic Sea, and Southwest of Ireland),” in Report of the ICES advisory comitte. ICES advice 2023, her.27.irls. doi: 10.17895/ices.advice.21907962
ICES (2023d). “Herring (Clupea harengus) in Subarea 4 and divisions 3.a and 7.d, autumn spawners (North Sea, Skagerrak and Kattegat, eastern English Channel),” in Report of the ICES advisory committe. ICES advice 2023, her.27.3a47d. doi: 10.17895/ices.advice.21907947
ICES (2023e). ICES database on trawl surveys (DATRAS). Available online at: https://datras.ices.dk (Accessed October 2, 2023).
ICES (2023f). The international herring larvae surveys. Available online at: http://eggsandlarvae.ices.dk/ (Accessed November 23, 2023).
Joly L. J., Loots C., Meunier C. L., Boersma M., Collet S., Lefebvre V., et al. (2021). Maturation of the digestive system of Downs herring larvae (Clupea harengus, Linnaeu): identification of critical periods through ontogeny. Mar. Biol. 168. doi: 10.1007/s00227-021-03894-z
Kass J. M., Muscarella R., Galante P. J., Bohl C. L., Pinilla-Buitrago G. E., Boria R. A., et al. (2021). ENMeval 2.0: Redesigned for customizable and reproducible modeling of species’ niches and distributions. Methods Ecol. Evol. 12, 1602–1608. doi: 10.1111/2041-210X.13628
Lee J. Y., Marotzke J., Bala G., Cao L., Corti S., Dunne J. P., et al. (2021). “Future global climate: scenario-based projections and near-term information,” in Climate change 2021 – the physical science basis. Eds. Masson-Delmotte V. ,. P., Zhai A., Pirani S. L., Connors C., Péan S., Berger, et al (Cambridge, UK: Cambridge University Press), 553–672. doi: 10.1017/9781009157896.006
Legendre P. (1993). Spatial autocorrelation trouble or new paradigm? Ecology 74, 1659–1673. doi: 10.2307/1939924
Lescrauwaet A. K., Debergh H., Vincx M., Mees J. (2010). Fishing in the past: Historical data on sea fisheries landings in Belgium. Mar. Policy 34, 1279–1289. doi: 10.1016/j.marpol.2010.05.006
Limborg M. T., Helyar S. J., De Bruyn M., Taylor M. I., Nielsen E. E., Ogden R., et al. (2012). Environmental selection on transcriptome-derived SNPs in a high gene flow marine fish, the Atlantic herring (Clupea harengus). Mol. Ecol. 21, 3686–3703. doi: 10.1111/j.1365-294X.2012.05639.x
Liu C., White M., Newell G. (2013). Selecting thresholds for the prediction of species occurrence with presence-only data. J. Biogeogr 40, 778–789. doi: 10.1111/jbi.12058
Lobo J. M., Jiménez-Valverde A., Hortal J. (2010). The uncertain nature of absences and their importance in species distribution modelling. Ecography 33, 103–114. doi: 10.1111/j.1600-0587.2009.06039.x
Lobo J. M., Jiménez-Valverde A., Real R. (2008). AUC: a misleading measure of the performance of predictive distribution models. Global Ecol. Biogeography 17, 145–151. doi: 10.1111/J.1466-8238.2007.00358.X
Loiselle B. A., Jørgensen P. M., Consiglio T., Jiménez I., Blake J. G., Lohmann L. G., et al. (2008). Predicting species distributions from herbarium collections: Does climate bias in collection sampling influence model outcomes? J. Biogeogr 35, 105–116. doi: 10.1111/j.1365-2699.2007.01779.x
Lynam C. P., Llope M., Möllmann C., Helaouët P., Bayliss-Brown G. A., Stenseth N (2017). Interaction between top-down and bottom-up control in marine food webs. C.Proc. Natl. Acad. Sci. U.S.A. 114, 1952–1957. doi: 10.1073/pnas.1621037114
Manel S., Dias J. M., Ormerod S. J. (1999). Comparing discriminant analysis, neural networks and logistic regression for predicting species distributions: a case study with a Himalayan river bird. Ecol. Modell 120, 337–347. doi: 10.1016/S0304-3800(99)00113-1
Maravelias C. D. (2001). Habitat associations of Atlantic herring in the Shetland area: Influence of spatial scale and geographic segmentation. Fish Oceanogr 10, 259–267. doi: 10.1046/j.1365-2419.2001.00172.x
Maravelias C. D., Reid D. G., Swartzman G. (2000). Seabed substrate, water depth and zooplankton as determinants of the prespawning spatial aggregation of North Atlantic herring. Mar. Ecol. Prog. Ser. 195, 249–259. doi: 10.3354/meps195249
Marshall S. M., Nicholls A. G., Orr A. P. (1937). On the growth and feeding of the larval and post-larval stages of the Clyde herring. J. Mar. Biol. Assoc. United Kingdom 22, 245–267. doi: 10.1017/S002531540001198X
Mehdi H., Lau S. C., Synyshyn C., Salena M. G., Morphet M. E., Hamilton J., et al. (2021). A comparison of passive and active gear in fish community assessments in summer versus winter. Fisheries Res. 242, 106016. doi: 10.1016/j.fishres.2021.106016
Merow C., Smith M. J., Silander J. A. (2013). A practical guide to MaxEnt for modeling species’ distributions: What it does, and why inputs and settings matter. Ecography 36, 1058–1069. doi: 10.1111/j.1600-0587.2013.07872.x
Moriarty M., Sethi S. A., Pedreschi D., Smeltz T. S., McGonigle C., Harris B. P., et al. (2020). Combining fisheries surveys to inform marine species distribution modelling. ICES J. Mar. Sci. 77, 539–552. doi: 10.1093/icesjms/fsz254
Morrison J. A., Napier I. R., Gamble Morrison J. C., Napier ,. J. A. (1991).Mass mortality of herring eggs associated with a sedimenting diatom bloom. Available online at: https://academic.oup.com/icesjms/article/48/2/237/644514 (Accessed January 12, 2024).
Paradis E., Schliep K. (2019). ape 5.0: an environment for modern phylogenetics and evolutionary analyses in R. Bioinformatics 35, 526–528. doi: 10.1093/bioinformatics/bty633
Phillips S. (2021). maxnet: Fitting “Maxent” Species Distribution Models with “glmnet”. R package version 0.1.4. Available online at: https://CRAN.R-project.org/package=maxnet (Accessed February 6, 2024).
Phillips S. B., Aneja V. P., Kang D., Arya S. P. (2006). “Modelling and analysis of the atmospheric nitrogen deposition in North Carolina,” in International journal of global environmental issues (Geneva, Switzerland: Inderscience Publishers), 231–252. doi: 10.1016/j.ecolmodel.2005.03.026
Phillips S. J., Dudík M., Elith J., Graham C. H., Lehmann A., Leathwick J., et al. (2009). Sample selection bias and presence-only distribution models: implications for background and pseudo-absence data. Ecol. Appl. 19, pp. 181 197. doi: 10.1890/07-2153.1
Popescu I., Scholaert F. (2022). Brexit and the reduction in EU fishing quota share to 2023. Eur. Parliamentary Res. Service.
R Core Team (2023).R: A language and environment for statistical computing. In: R foundation for statistical computing. Available online at: https://www.R-project.org/ (Accessed August 11, 2023).
Regulation 1380/2013 (2013). Regulation (EU) No 1380/2013 Of The European Parliament And Of The Council of 11 December 2013 on the Common Fisheries Policy, amending Council Regulations (EC) No 1954/2003 and (EC) No 1224/2009 and repealing Council Regulations (EC) No 2371/2002 and (EC) No 639/2004 and Council Decision 2004/585/EC. Strasbourg: Official Journal of the European Union.
Ricker M., Stanev E. V. (2020). Circulation of the European northwest shelf: A Lagrangian perspective. Ocean Sci. 16, 637–655. doi: 10.5194/os-16-637-2020
Rogers J. C. (1984). The association between the North Atlantic Oscillation and the Southern Oscillation in the northern hemisphere. Mon Weather Rev. 112, 1999–2015. doi: 10.1175/1520-0493(1984)112<1999:TABTNA>2.0.CO;2
Salt L. A., Thomas H., Prowe A. E. F., Borges A. V., Bozec Y., De Baar H. J. W. (2013). Variability of North Sea pH and CO2 in response to North Atlantic Oscillation forcing. J. Geophys Res. Biogeosci 118, 1584–1592. doi: 10.1002/2013JG002306
Signorell A. (2024). DescTools tools for descriptive statistics. Available online at: https://CRAN.R-project.orgpackage=DescTools (Accessed March 18, 2024).
Sillero N., Barbosa A. M. (2021). Common mistakes in ecological niche models. Int. J. Geographical Inf. Sci. 35, 213–226. doi: 10.1080/13658816.2020.1798968
Sinclair M., Power M. (2015). The role of “larval retention” in life-cycle closure of Atlantic herring (Clupea harengus) populations. Fish Res. 172, 401–414. doi: 10.1016/j.fishres.2015.07.026
Stephenson R., Clark K. J., Power M. J., Fife F. J., Melvin G. D. (2001). “Herring stock structure, stock discreteness, and biodiversity,” in Herring: expectations for a new millennium (Alaska, US: University of Alaska Sea Grant College Program), 559–571.
Thuiller W., Lafourcade B., Engler R., Araújo M. B. (2009). BIOMOD - A platform for ensemble forecasting of species distributions. Ecography 32, 369–373. doi: 10.1111/j.1600-0587.2008.05742.x
Turner S. M., Manderson J. P., Richardson D. E., Hoey J. J., Hare J. A. (2016). Using habitat association models to predict Alewife and Blueback Herring marine distributions and overlap with Atlantic Herring and Atlantic Mackerel: Can incidental catches be reduced? ICES J. Mar. Sci. 73, 1912–1924. doi: 10.1093/icesjms/fsv166
Turrell W. R. (1992). New hypotheses concerning the circulation of the northern North Sea and its relation to North Sea fish stock recruitment. ICES J. Mar. Sci. 49, 107–123. doi: 10.1093/icesjms/49.1.107
Valavi R., Elith J., Lahoz-Monfort J. J., Guillera-Arroita G. (2023). Flexible species distribution modelling methods perform well on spatially separated testing data. Global Ecol. Biogeography 32, 369–383. doi: 10.1111/geb.13639
van der Molen J., Pätsch J. (2022). An overview of Atlantic forcing of the North Sea with focus on oceanography and biogeochemistry. J. Sea Res. 189. doi: 10.1016/j.seares.2022.102281
Van Ginderdeuren K., Vandendriessche S., Prössler Y., Matola H., Vincx M., Hostens K. (2014). Selective feeding by pelagic fish in the Belgian part of the North Sea. ICES J. Mar. Sci. 71, 808–820. doi: 10.1093/icesjms/fst183
Vollering J., Halvorsen R., Auestad I., Rydgren K. (2019). Bunching up the background betters bias in species distribution models. Ecography 42, 1717–1727. doi: 10.1111/ecog.04503
Wang L., Kerr L. A., Record N. R., Bridger E., Tupper B., Mills K. E., et al. (2018). Modeling marine pelagic fish species spatiotemporal distributions utilizing a maximum entropy approach. Fish Oceanogr 27, 571–586. doi: 10.1111/fog.12279
Watling L., Norse E. A. (1998). Disturbance of the seabed by mobile fishing gear: A comparison with forest clear-cutting. Conserv. Biol. 12, 1180–1197. doi: 10.1046/j.1523-1739.1998.0120061180.x
Whitehead P. J. P. (1985). “Clupeoid fishes of the world. An annotated and illustrated catalogue of the herrings, sardines, pilchards, sprats, shads, anchovies and wolf-herrings,” in Part 1 chirocentridae, clupeidae and pristigasteridae. (FAO fisheries synopsis).
Yang J., Rahardja S., Fränti P. (2019). “Outlier detection: How to threshold outlier scores?,” in ACM International Conference Proceeding Series (Sanya, China: Association for Computing Machinery). doi: 10.1145/3371425.3371427
Zeng Y., Low B. W., Yeo D. C. J. (2016). Novel methods to select environmental variables in MaxEnt: A case study using invasive crayfish. Ecol. Modell 341, 5–13. doi: 10.1016/j.ecolmodel.2016.09.019
Zizka A., Silvestro D., Andermann T., Azevedo J., Duarte Ritter C., Edler D., et al. (2019). CoordinateCleaner: Standardized cleaning of occurrence records from biological collection databases. Methods Ecol. Evol. 10, 744–751. doi: 10.1111/2041-210X.13152
Zou G. Y. (2007). Toward using confidence intervals to compare correlations. Psychol. Methods 12, 399–413. doi: 10.1037/1082-989X.12.4.399
Keywords: Atlantic herring, species distribution modeling, machine learning, fishery, sustainable blue economy, Northeast Atlantic, North Atlantic Oscillation, Belgian Part of the North Sea
Citation: Standaert W, Musimwa R, Stevens M, Guerra JA, Muñiz C, Debusschere E, Pint S and Everaert G (2024) Modeling Atlantic herring distribution in the Northeast Atlantic for informed decision-making towards sustainable fisheries. Front. Mar. Sci. 11:1485161. doi: 10.3389/fmars.2024.1485161
Received: 23 August 2024; Accepted: 22 October 2024;
Published: 14 November 2024.
Edited by:
Jianchuan Yin, Guangdong Ocean University, ChinaCopyright © 2024 Standaert, Musimwa, Stevens, Guerra, Muñiz, Debusschere, Pint and Everaert. This is an open-access article distributed under the terms of the Creative Commons Attribution License (CC BY). The use, distribution or reproduction in other forums is permitted, provided the original author(s) and the copyright owner(s) are credited and that the original publication in this journal is cited, in accordance with accepted academic practice. No use, distribution or reproduction is permitted which does not comply with these terms.
*Correspondence: Gert Everaert, Z2VydC5ldmVyYWVydEB2bGl6LmJl
Disclaimer: All claims expressed in this article are solely those of the authors and do not necessarily represent those of their affiliated organizations, or those of the publisher, the editors and the reviewers. Any product that may be evaluated in this article or claim that may be made by its manufacturer is not guaranteed or endorsed by the publisher.
Research integrity at Frontiers
Learn more about the work of our research integrity team to safeguard the quality of each article we publish.