- 1Département de Biologie, Université Laval, Québec, QC, Canada
- 2Institut de Biologie Intégrative et des Systèmes (IBIS), Université Laval, Québec, QC, Canada
Phytoplankton and other protists in the 3 µm to 50 µm size fraction are grazed on by zooplankton and form the base of Arctic marine food webs essential for local indigenous communities. Anthropogenic climate change is increasing stratification over much of the Arctic Ocean and surrounding seas, but the influence of stratification on protist communities in more coastal regions along Eastern Hudson Bay, Hudson Strait and Ungava Bay is little known. We used 18S rRNA and rDNA amplicon sequencing during two consecutive summers (2017 and 2018) and detailed water column properties to compare the 3 µm to 50 µm protist communities under contrasting stratification regimes in the Eastern Hudson Bay Complex. We found that the surface mixed layer in Eastern Hudson Bay, which is under the influence of river runoff, was strongly stratified and dominated by mixotrophic and bacterivorous taxa, mostly the dinoflagellates Heterocapsa rotundata and Gymnodiniales spp., and a more diatom-dominated community at the Subsurface Chlorophyll Maximum (SCM), which persisted in deeper colder and more saline water. The massive sequencing effort retrieved seven putative toxic algae from the upper warmer waters of eastern Hudson Bay. These included Pseudo-nitzschia spp. and potentially harmful dinoflagellates, most notably Alexandrium sp. The persistent weaker stratification conditions in Hudson Strait and Ungava Bay in summer favored a different diatom community, dominated by Chaetoceros spp. and Thalassiosira spp., and small photosynthetic flagellates including Phaeocystis pouchetii and Micromonas polaris. As freshwater input increases and stratification intensifies in the Arctic, our findings suggest the summer dinoflagellate-based community seen in coastal Hudson Bay may also be favored in other regions receiving increased river runoff. These conditions could also favor harmful algal events. The Hudson Strait and Ungava Bay protist communities were found nearer the surface and consisted of diverse species able to profit from ongoing nutrient input due to tidal mixing. These results suggest greater resilience in this and other tidally influenced coastal Arctic Bays lacking larger rivers inputs.
Introduction
Biogeography and phenology of phytoplankton and other planktonic microbial eukaryote species are intimately associated with local nutrient and light conditions determined by mixing and stratification dynamics of the sunlit water column. In recent decades, global warming has fundamentally altered oceanic temperature and salinity, resulting in increasing stratification of the world upper ocean (Li et al., 2020). By inhibiting vertical mixing of the water column, stratification slows the exchange of nutrients and gases, which ultimately decreases marine productivity (Matear and Hirst, 2003; Behrenfeld et al., 2006; Breitburg et al., 2018). While low latitude oceans are mainly stratified by temperature, for Arctic and sub-Arctic seas, by far the most significant determinant of stratification is salinity (Carmack, 2007). Upper water column stratification is particularly pronounced in high latitude regions where rivers discharge, freshening the surface waters and increasing stratification. The strong water column stratification prevents vertical transfer of nutrient-rich deeper water into the euphotic zone (Arrigo et al., 1999; Tremblay and Gagnon, 2009; Ferland et al., 2011). Because of anthropogenic warming, river discharge is increasing along with an accelerated hydrological cycle, impacting Arctic regions including the large Arctic-sub-Arctic Hudson Bay Complex (Stadnyk et al., 2020). An additional concern is that warming conditions could also increase the probability of harmful algal events with impacts on human health and local food chains (Anderson et al., 2021).
Hudson Bay (HB) is the core of the Hudson Bay Complex that connects Arctic modified Pacific origin waters to the Labrador Sea through Hudson Strait (HS) and Ungava Bay (UB). The Hudson Bay catchment covers one third of Canada’s land mass and is dominated by large riverine inputs (Déry and Wood, 2005; Macdonald and Kuzyk, 2011). In the greater Hudson Bay a low nutrient, warmer and fresher summer mixed layer sits atop colder saltier waters that are more nutrient rich (Granskog et al., 2011). This surface layer ranges from 30-60 m depth over most of the HB and contains most of the freshwater load from the annual accumulated river runoff of the catchment as well as seasonal sea-ice melt. These large volumes of freshwater feed an anti-clockwise north flowing boundary current along the eastern coastal region of HB (Granskog et al., 2009). The warmer, less-saline and strongly stratified waters limit phytoplankton production of HB in late summer (Harvey et al., 1997; Ferland et al., 2011).
HS+UB regions are influenced by different hydrodynamic processes compared to HB. The HS+UB region drains a much smaller catchment basin and is characterized by strong tidal and meteorological mixing that weakens stratification and increases biological productivity (Drinkwater and Jones, 1987; Straneo and Saucier, 2008). However, although net annual productivity differences between HB and HS+UB are reported, other than historic species lists of mostly phytoplankton from microscopy (Poulin et al., 2011), little is known about the composition of other microbial eukaryotic communities in the two hydrologically contrasting systems. To our knowledge, there have been no direct comparisons of the summer protist communities that occur when stratification is greatest in HB, but nearly absent in the HS+UB region. Here, we addressed this data gap using molecular techniques to identify the protist taxa and in combination with physical oceanographic data sought to gain understanding for the role of stratification in species selection in this high latitude region. We focused our analysis on protist communities in the 3 µm to 50 µm size range, which includes nanoplankton (2-20 µm) and the slightly larger diatoms, dinoflagellates and ciliates, which are prey for zooplankton and commonly reported using light microscopy. As this size fraction would be biased towards taxa that are reported from microscopy, our results should facilitate transition to community-based monitoring by local indigenous communities that live along the coastal Ungava Bay Peninsula. However, as filtration is not perfect, typically some smaller and larger taxa are also retained on the filters, due to filter blockage and cell breakage, respectively, material captured on the filters would provide catalog of taxa suitable for comparison between different systems.
To address which organisms were likely more active as opposed those that persist under the different conditions (Morency et al., 2022), we used amplicon sequencing targeting both 18S rRNA genes (rDNA) and its transcriptional products (rRNA). Although rRNA cannot be directly used to assess the growth and activity of microbes, the variability of rRNA within a species may provide information on the potential of organisms to synthesize proteins in a particular environment (Blazewicz et al., 2013). In addition, given that harmful algal groups are prone to bloom under summer conditions, and climate change scenarios are of concern to local communities (McKenzie et al., 2020), we paid particular attention to the potential presence of harmful algal species in eastern coastal HB and HS+UB under their respective summer stratification regimes.
Materials and methods
The study was carried out in eastern Hudson Bay, the southern reaches of Hudson Strait and along the coast of Ungava Bay (Nunavik, Québec, Canada) (Table 1). Sampling was realized onboard the Canadian research icebreaker CCGS Amundsen during two cruises in early July 2017 and 2018. Seawater was collected directly from 12-L Niskin-type bottles mounted on a rosette system equipped with a relative nitrate (In-Situ Ultraviolet Spectrophotometer, ISUS, Satlantic), dissolved oxygen (Seabird SBE-43), chlorophyll fluorescence (Seapoint) and a fluorescent colored dissolved organic matter sensor (fCDOM; Wetlabs ECO). We targeted the surface layer (1 or 2 m) and the subsurface chlorophyll maximum (SCM). The depth of the subsurface chlorophyll maxima (SCM) was determined on the downward cast. For each depth, 6 L of seawater was first prefiltered through a 50 µm mesh to minimize microzooplankton and then filtered onto a 3 µm pore size polycarbonate filter (Millipore, Canada) so the nominal community collected would be in the 3 to 50 µm range. The polycarbonate filters were placed in 2 ml microtubes containing 1.8 ml of RNAlater (Thermo Fisher Scientific, USA). After 30 min at room temperature, filters were transferred to –80°C until nucleic acid extraction. Water samples for nitrate (NO3-), nitrite (NO2-), phosphate (PO43-) and silicate (Si(OH)4) measurements were collected into acid rinsed, then sample rinsed, 15-ml Falcon™ tubes (Corning, USA) after filtration through a 0.22 µm syringe filter. The nutrient samples were analyzed on board the ship using a Bran-Luebbe 3 autoanalyzer (Grasshoff et al., 1999). The stratification parameter was defined with the Brunt-Väisalä (or buoyancy) maximum frequency N2 calculated for each station in Ocean Data View (v 5.2.1; Schlitzer, 2002). The more stratified the water column, the higher the N2 value.
DNA and RNA were co-extracted from the filters using AllPrep DNA/RNA Mini kit (Qiagen, Germany) following the manufacturer protocol and V4 region of 18S rRNA gene (rDNA) and 18S rRNA (rRNA) was amplified and sequenced using a combination of universal forward E572F (5’-CYG CGG TAA TTC CAG CTC-3’) and reverse primers E1009R (5’-CRA AGA YGA TYA GAT ACC RT-3’) using the cycling conditions as described in Comeau et al. (2011). 26 Samples from 11 sampling events were sequenced at the Institut de Biologie Intégrative et des Systèmes, Université Laval, Sequencing Plateform (Table 1). Overlapping paired end reads from the fastq files were processed within the qiime2 environment (Caporaso et al., 2010) using dada2 v2019.10 (Callahan et al., 2016). The quality of the forward and reverse reads was estimated using fastqc v0.12.0 (Andrews, 2010). For each run, forward and reverse reads were demultiplexed. Removing of primers, trimming of forward and reverse reads, denoising of low-quality reads, merging and removing of chimeras was performed using the denoise-paired command in dada2 (trim-left-f = 5, trim-left-r = 5, trunc-len-f = 285, trunc-len-r = 260, maxEE = 1). The two denoised runs were merged and taxonomy was assigned to each ASV in mothur using the PR2 database v4.12 (Guillou et al., 2013). Sequences affiliated to Metazoa and chloroplasts were removed from the analysis as well as sequences at the unclassified Phylum level using the R package Phyloseq v1.32.0 (McMurdie and Holmes, 2013). For dinoflagellate taxa detected as potentially harmful, taxonomy was refined by BLASTn against the NCBI nr database (Table S2).
To correct for differential sequencing depth of the samples, data were transformed to relative abundance and ASVs below the threshold of 1 × 10-5 total relative abundance were removed from the resulting matrix table. The rDNA and rRNA ASVs and reads were analysed on the same pipeline in Phyloseq, resulting in a relative abundance table of 856 ASVs. All the subsequent clustering analysis were run in the R environment. A square-root transformation was applied to the relative abundance table and a Bray-Curtis (BC) matrix was calculated. Hierarchical clustering was performed using the “ward.D2” method on the BC matrix. Distance-based redundancy analysis (db-RDA) of microbial communities was performed with standardized environmental variables using the capscale() function in vegan v2.5.6. We adjusted the R2 results using Ezekiel’s formula with the RsquareAdj() function. The adjusted R2 measures the unbiased amount of explained variation and was used to reduce the number of significant variables using a forward selection and 999 ANOVA permutations. Co-varying variables were removed from the final db-RDA calculation. Pearson correlation between environmental variables and relative abundance of taxa at the “Genus” classification level was calculated with the stats R package and visualized using ggcorrplot v0.1.4 (Kassambara, 2019). Taxa with significant correlation (P < 0.001) with at least one environmental parameter in the rDNA and rRNA dataset were retained for specific correlation analysis. Linear regression models were used on these selected taxa to further test the influence of environmental parameters on the distribution of rDNA and rRNA reads. To assess the distribution of the most abundant taxa, the relative abundance of ASVs were summed at the species level and zscore-transformed ((x-mean) sd-1). When no known species were identified for the group, taxa were annotated at the highest taxonomic level. DNA and RNA sourced datasets were treated separately. To assess significant differences in mean relative abundance between the HB and HS+UB clusters, Student’s t-tests were performed with a P-value threshold of 0.05.
Results
Environmental parameters
Temperature and salinity profiles in 2017 and 2018 both show warmer and fresher surface waters in eastern HB compared to surface waters in HS+UB (Figures 1A, B). The warmest temperature was recorded at the surface of station st732 in 2017 (9.49°C) and freshest surface waters were at st730 (salinity = 23.27; Table 1). The upper warmer layer in eastern HB was constrained to the top 20 meters of the water column and contrasted with colder and saltier waters underneath (Figure 1B), for example with a salinity of 31.62 and a temperature of -0.62°C for deeper water of st732. The stratification was associated with the marked SCM with a maximum fluorescence detected at st736 in 2017 (7.67 µg chl a L-1). The SCM in eastern Hudson Bay was more developed during the sampling in 2017 than in 2018 with higher chl a concentrations as estimated by fluorescence. Surface waters were depleted in nutrients all along the cruise track for both years, contrasting with nutrient rich water in the deepest layers. In the HS+UB, the nitracline was closer to the surface and associated with higher surface chl a concentration compared to the HB. Temperature and salinity profiles in the HS+UB indicate a mixed water column contrasting with the stratified water column in the HB. The Brunt-Väisälä frequency maximum (higher values indicate stronger stratification) through the pynocline ranged from 9.88 to 16.89 in HS+UB, and 19.78 to 45.47 in the HB with the highest value at st730 in 2018 (Table 1).
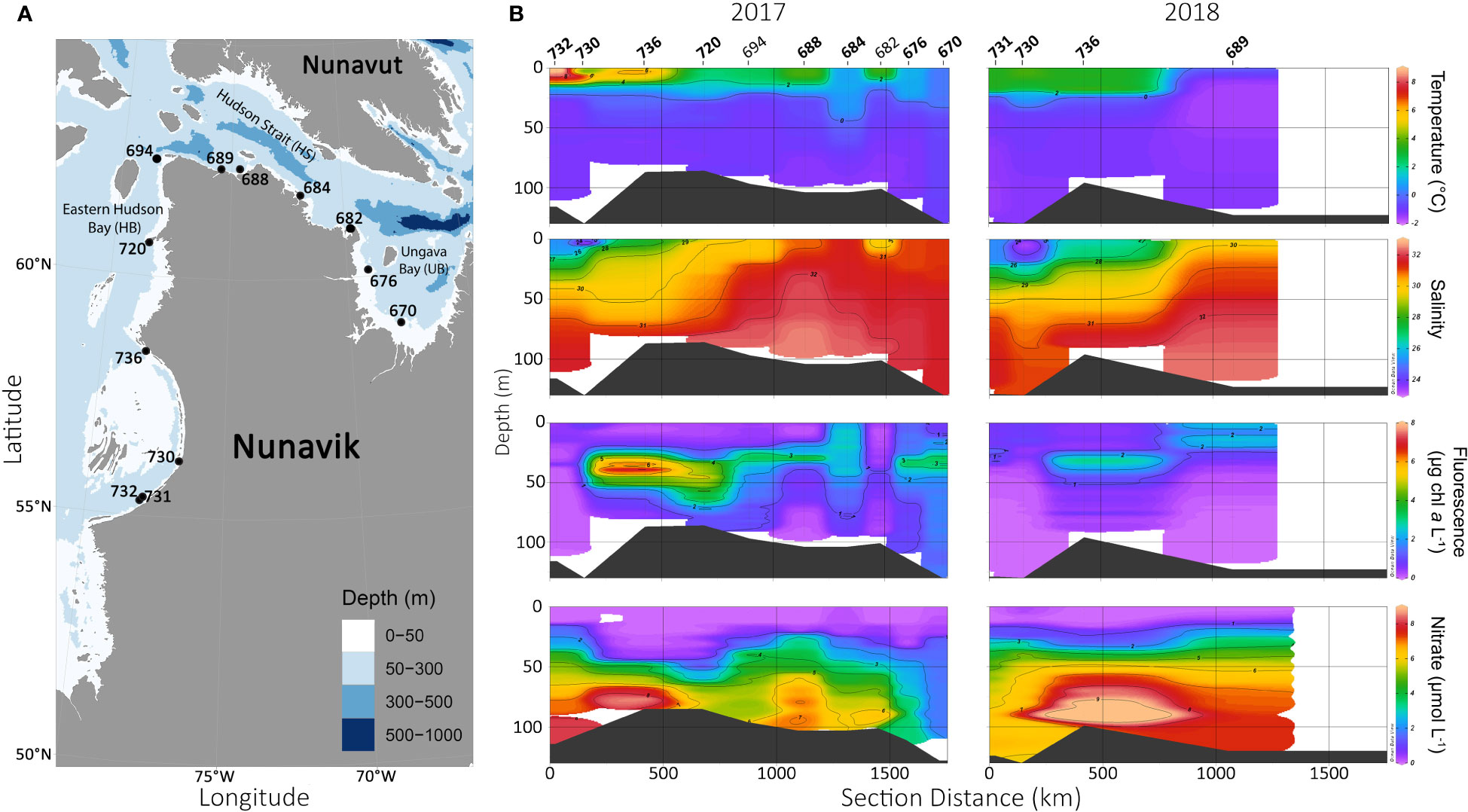
Figure 1 Sampling sites and water column properties in Eastern Hudson Bay, Hudson Strait and Ungava Bay. (A) Station locations during summer 2017 and 2018. (B) Vertical water temperature, salinity, fluorescence and nitrate concentrations from individual stations, the continuum is extrapolated from CTD profiles using Ocean Data View v5.2.1.
Structure of protist communities
The hierarchical clustering based on the Bray-Curtis distance separated the community sequences into 4 distinct clusters (Figure 2B). The most distant cluster consisted of mostly the rDNA collected from the HB surface in both 2017 and 2018. The vast majority of HB rRNA surface communities formed a separate cluster. The HB SCM rDNA and rRNA samples and st688 in Hudson Strait clustered together. The remaining stations that were collected along Hudson Strait and the Ungava Bay formed a separate cluster consisting of rRNA and rDNA reads from both surface and SCM of the Hudson Strait and Ungava Bay (collectively referred to as HS+UB). The samples from the same location and depth category tended to cluster together independent of collection year, except for st688_2017, where rDNA clustered within HS+UB while rRNA clustered within HB.
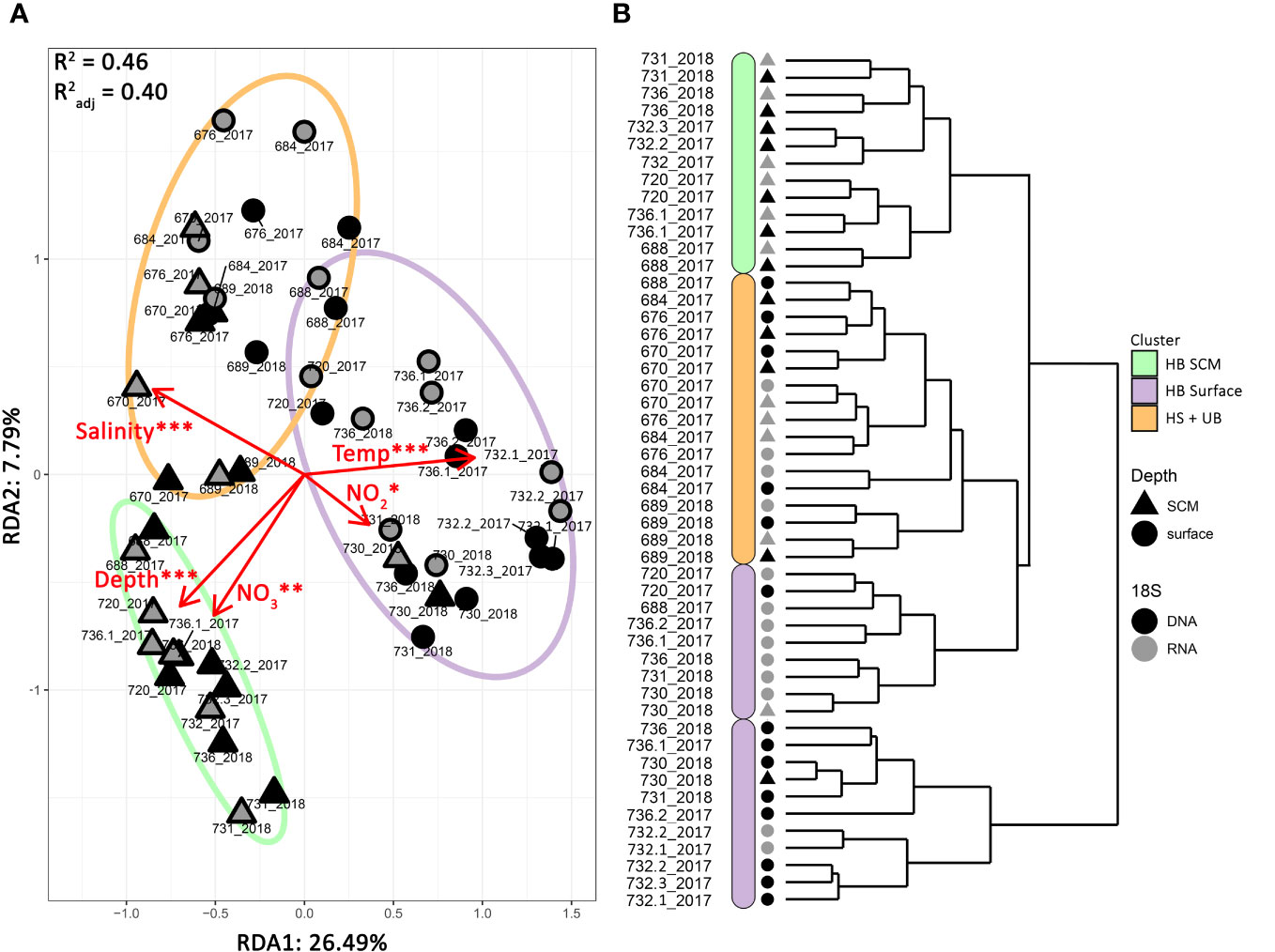
Figure 2 Summer protist community structure. (A) Ordination plot of distance-based redundancy analysis (db-RDA) of microbial eukaryote communities using a Bray-Curtis distance. Symbol shapes indicate the depth layer, with nucleotide source in grey or black. Statistically significant environmental variables are shown (ANOVA test). P-value: *** = 0; ** < 0.001, * <0.01. (B) Hierarchical clustering analysis. The dendrograms were constructed with Bray–Curtis distance using the “ward.D2” method. Environmental clusters are designated by colored bars.
A distance-based redundancy analysis (db-RDA) was used to seek the environmental parameters associated with the spatial repartition of the communities. Together, temperature, salinity, depth, nitrite, and nitrate concentrations explained 40% of the total variance (R2 adj = 0.40; Figure 2A). Phosphate was also significant but was excluded from the final analysis due to its high positive correlation with nitrate (Pearson’s correlation = 0.75; P-value = 0.03) and because nitrate is expected to be the main limiting nutrient for phytoplankton growth in Hudson Bay (Tremblay et al., 2019). The surface waters in the HB clearly separated from HS+UB along the first canonical axis explaining 26.49% of the total variance. Higher temperatures and nitrite concentrations were associated with the surface waters of HB, and saltier conditions were associated with the surface and SCM samples in the HS+UB. Temperature explained most of the variance in our dataset (F = 19.65; P-value < 0.001; Table S1). The second axis, which explained 7.79% of the total variance indicated that communities at the SCM in HB were associated with greater depth and the higher nitrate concentrations that occur below the halocline.
Dinoflagellates, diatoms, and to a lesser extent ciliates accounted for the greatest number of sequences in rDNA and rRNA datasets (Figure 3). The dinoflagellates and diatoms contributed about equal proportions in the rDNA data set of the SCM in HB, with relatively lower representation of dinoflagellates and more diatoms in the rRNA data. The HS+UB communities had a similar pattern with fewer dinoflagellates and more diatoms in the rRNA compared to the rDNA. Dinoflagellate read relative abundance was greatest in the HB surface especially in the rDNA dataset with relatively fewer diatoms in both the rDNA and rRNA compared to HS+UB.
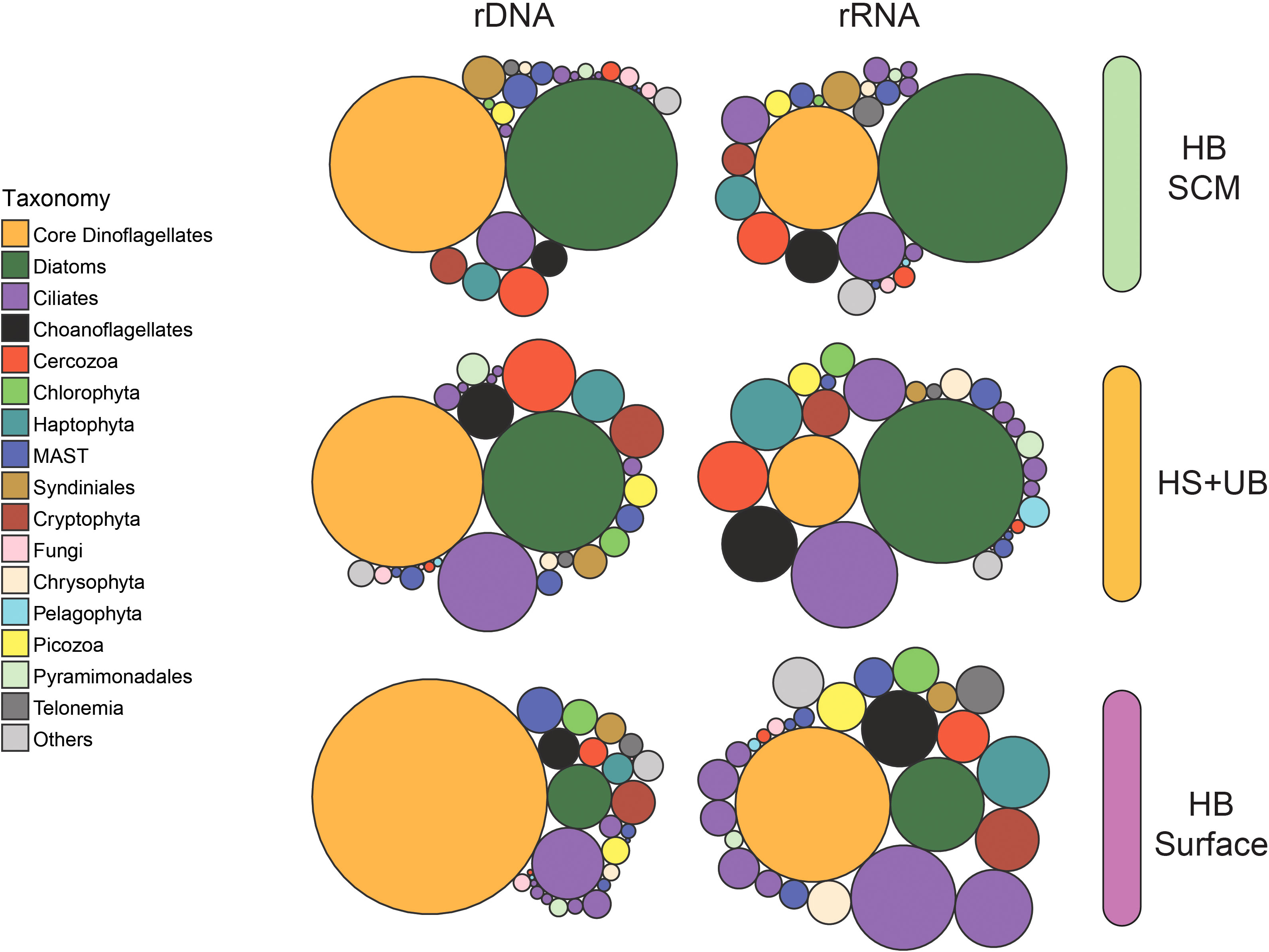
Figure 3 Relative abundance of major microbial eukaryote groups in the rDNA compared to rRNA. The size of the bubble is proportional to the relative abundance of the PR2 group at the Class level taxonomy. Colored bars on the right indicate clusters defined according to the hierarchical clustering analysis from Figure 2.
To identify the principal protist groups underlying separation of the communities in the db-RDA (Figure 2A), we used Pearson’s correlation between environmental parameters and relative abundance of 252 protist groups that were placed at the same classification level in the PR2 database (Guillou et al., 2013). Only correlations with P-values less than 0.001 were considered as significant. Among the 252 groups tested, 39 showed at least one significant correlation with an environmental parameter in both rDNA and rRNA datasets (Figure S1). Ciliate reads classified in the Laboea, Rimostrombidium group A, Strombidiida group F, as well as the dinoflagellates Heterocapsa and Gymnodinium were highly correlated with decreasing salinity in both rDNA and rRNA datasets. Increasing temperature was also correlated with increased representation of small photosynthetic taxa such as Bathycoccus and Micromonas in rDNA and the dinoflagellates Margalefidinium in both rDNA and rRNA. Lower temperatures were associated with an increase in diatom relative abundance from the genus Thalassiosira. Linear regression models tended to confirm these patterns and showed opposite trends for the diatom Thalassiosira, compared to the dinoflagellates and ciliates (Figure S2). Reflecting this, warmer temperatures and lower salinity were associated with significantly higher proportions of Gymnodiniales, Heterocapsa and Strombidiida F reads. Again, this contrasted with the diatom reads that increased with salinity and decreased with warmer temperatures. The macronutrients, phosphate, nitrate and silicate, were correlated with several of the groups (Figure S1). Especially, higher nitrate concentrations were correlated with higher proportions of the diatom Pseudo-nitzschia in the rRNA dataset.
At a finer taxonomic level, distribution of the top 50 species level taxa also showed the biogeographic patterns in the surface layer (Figures 4A, B). Together, these top 50 taxa represented between 82% and 95% of the total community in surface samples from the rDNA dataset. Most of the diatom species, especially Porosira glacialis, Pseudo-nitzschia, Navicula sp., Thalassiosira antarctica, Thalassiosira nordenskioeldii, Chaetoceros neogracilis and an unidentified ASV of Mediophyceae were more abundant in HS+UB, with high proportions at st689 in HS and at st676 and st670 in Ungava Bay. Among the diatoms, particularly for sequences classified as Pseudo-nitzschia spp., Thalassiosira spp. and Chaetoceros spp., reads at the SCM in HB were proportionately higher compared to the surface for both rDNA and rRNA (Figure S3). Prymnesiophyceae from the environmental clade F, and Phaeocystis pouchetii showed a significant relative increase in HS+UB compared to HB (Figure 4A). Most of the dinoflagellate ASVs except for taxa from the family Heterocapsaceae, were more abundant in HB. Gymnodiniales and Peridiniales were overrepresented in the rDNA compared to rRNA, (Figure S3). Ciliates tended to have a pattern of increasing relative abundance in HS+UB compared to HB, particularly for ASVs in the Strombidiidae family.
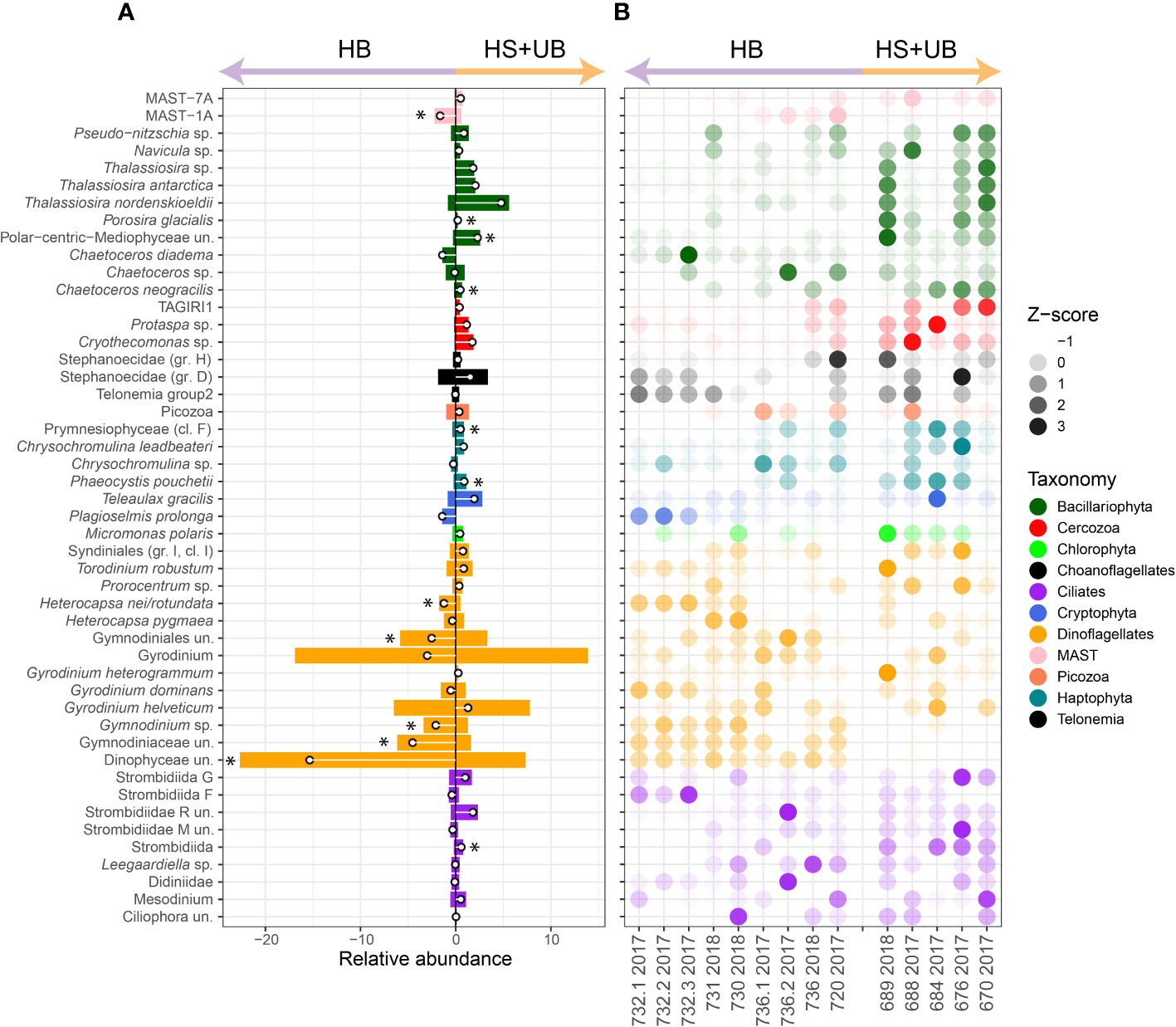
Figure 4 Relative abundance of the 50 most abundant surface protist ASVs grouped at equivalent species level in the rDNA. (A) The mean relative abundance in HB and HS+UB. Asterisk indicate significant differences in relative abundance between HB and HS+UB (Student’s P-value < 0.05). (B) Bubble plot of z-score standardized distribution of taxa along the sampling transect (Figure 1). un., unclassified.
HAB species
Our results based on metabarcoding showed no evidence of any HAB event at the time of sampling, but our targeted search indicated a widespread occurrence of reads from species reported as HABs. Pseudo-nitzschia spp. were present at all sampled stations, but especially in the rRNA dataset at the SCM in the HB samples where they may have been particularly active (Figure S3B). Despite their low relative abundance, six putative harmful dinoflagellate species were detected. Among these 6 taxa, Margalefidinium fulvescens, Protoceratium reticulatum and Phalacroma rotundatum had unique best blast hits > 99% of identity and annotation at the species level, while Tripos, Dinophysis and Alexandrium were not resolved to species based on best blast hits to multiple species (see Figure 5; Table S2). Specifically, the distribution of Alexandrium sp. was restricted to HB with a peak at st732 in 2017 where it contributed to more than 4% of the total rRNA reads (Figure 5). The relatively high number of sequences of Alexandrium sp. at st732 coincided with the highest water temperature (9.49°C) recorded during our sampling of the area (Table 1; Figure 1B).
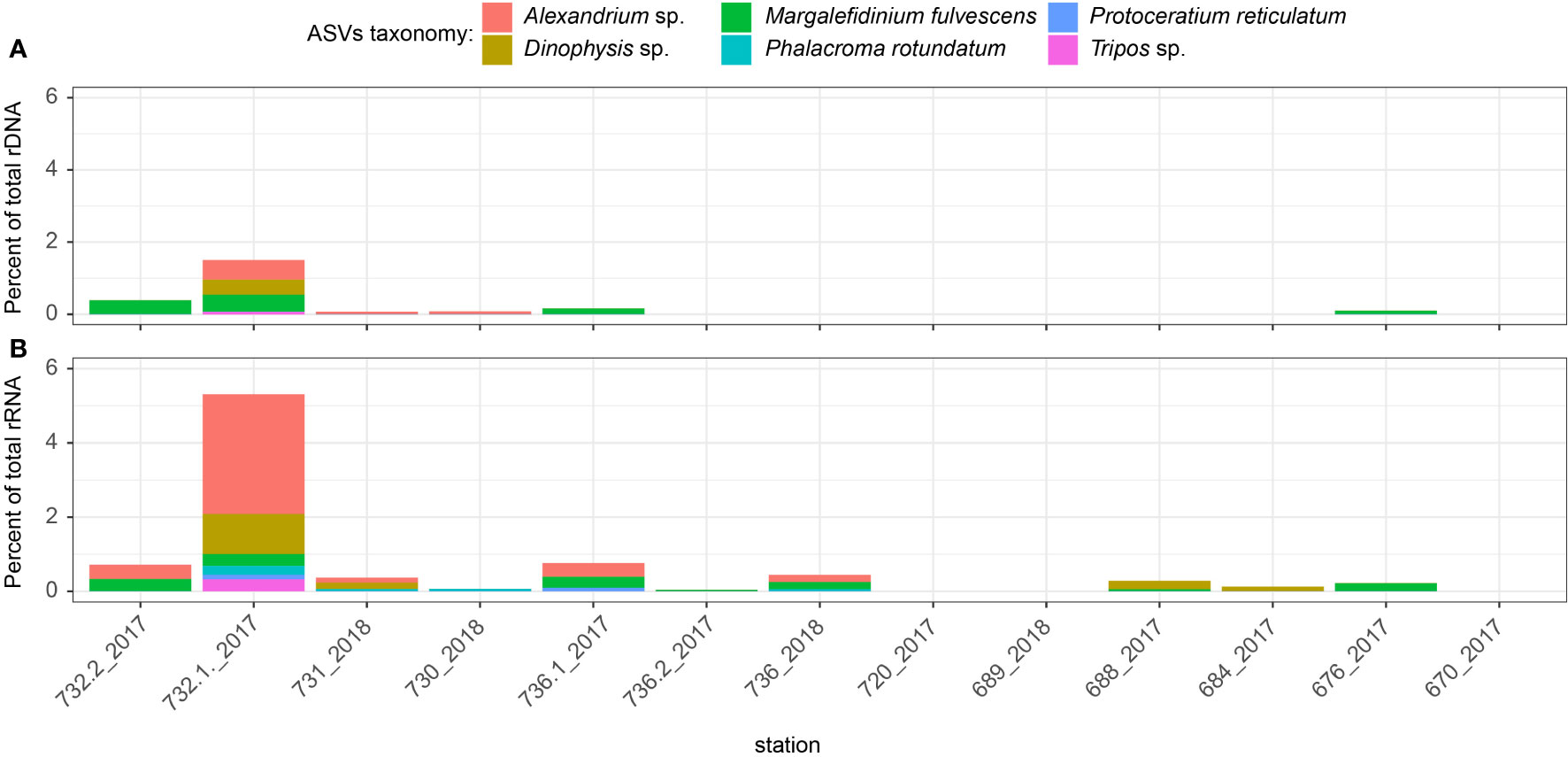
Figure 5 Proportion (as %) of potential harmful dinoflagellates detected at surface in rDNA (A) and rRNA dataset (B).
Discussion
Marine microbial eukaryotes respond rapidly to changing environmental conditions and can have a direct influence on the energy and carbon transfer to higher trophic levels (Worden et al., 2015). Here we showed that the structure of protist assemblages recovered from 18S rDNA and rRNA distinguished the surface and SCM as two distinct communities in eastern HB, with a single more uniform HS+UB community. The differences in species composition between the eastern HB compared to northern and western stations surrounding the HS+UB were consistent with hydrological conditions in the two marine regions (Figures 1, 2).
For the stations that were sampled during both years, rRNA and rDNA showed little or no differences in the microbial community structure, suggesting our observations were characteristic of summer conditions in this part of the HB complex. Surface temperatures at st688 were the highest in 2017 in HS+UB, which may have influenced the clustering of the rRNA sample in the HB group. Overall, the strength of the stratification was a key driver of microbial eukaryotic community structure and distribution, especially in HB (Table 1). The communities in eastern HB were vertically separated in keeping with greater stratification associated with a fresher warmer summer surface mixed layer above a well-established SCM (Figures 1, 2). The development of an SCM dominated by diatoms in summer is a characteristic feature of the HB (Roff and Legendre, 1986; Ferland et al., 2011; Matthes et al., 2021). The SCM was particularly well-defined in 2017 and was mainly associated with Thalassiosira spp. and Chaetoceros spp., two genera that also occur at the SCM after sea ice breakup in central HB (Jacquemot et al., 2022).
The low salinity and warm temperature in the surface waters of southeastern HB compared to HS+UB suggested a strong influence of river runoff in the region at the time of sampling, especially in 2018 (Figure 1; Table 1). During our sampling in 2017 and 2018, most of the nutrients at the surface were depleted, with low inorganic nutrient conditions in the surface mixed layer (Figure 1), consistent with the post spring bloom timing of our sample collection. The conditions were favorable for dinoflagellates which accounted for a high proportion of reads at the surface, particularly in the rDNA dataset where they exceeded 70% of the total reads at st732, st731, st730 and st736 (Figures 3; S3A). The presence of multiple gene copies of the 18S rRNA gene in dinoflagellates might result in an overestimation of their relative abundance in metabarcoding studies (Zhu et al., 2005; Medinger et al., 2010). However, variations in their relative abundance across different regions and depths can offer valuable insights into the preferential ecological niche of dinoflagellates. Compared to HS+UB, the dominance of reads associated with specific ASVs of dinoflagellates in HB suggest that some genera or species were particularly successful despite the low nutrient waters, but consistent with being primarily heterotrophic. For example, the marine stramenopiles from the MAST-1A clade, the Peridiniales Heterocapsa rotundata and the Gymnodiniales Gyrodinium sp. and Gymnodinium sp. had high relative representation in the surface waters of HB compared to the HS+UB (Figure 4). Moreover, both rDNA and rRNA were higher for Heterocapsa and Gymnodinium in the HB than in the HS+UB, suggesting a high potential for growth and acclimatation of these two genera in the warmer waters of the HB surface (Figure S3A). Arctic Heterocapsa spp. have chloroplasts (Rintala et al., 2010) but the genus is considered mixotrophic (Millette et al., 2017) and able to photosynthesize and graze on bacteria. While some species of Gymnodiniales are mixotrophic (Li et al., 2000), Gyrodinium spp. are mostly heterotrophic dinoflagellates that graze on small phytoplankton and bacteria (Hoppenrath, 2017). Both genera are nearly always reported in Arctic waters in summer (Lovejoy et al., 2006; Kubiszyn and Wiktor, 2016; Onda et al., 2017). The significant correlation of Gymnodiniales, Gymnodinium and Heterocapsa relative abundance with increasing temperature and decreasing salinity suggest that the warmer and fresher waters in the surface mixed layer were favorable to the growth of these dinoflagellates (Figures S1, S2). This is in keeping with reports of salinity and temperature having the greatest influence on the growth rate and biomass accumulation of diverse dinoflagellate species (Kim et al., 2004; Dhib et al., 2013). In a recent study, Amiraux et al. (2022) showed that the higher concentrations of dinoflagellates in eastern HB resulted in lower but more diverse content of bioactive molecules being transferred to higher trophic levels. Because the open water season is becoming longer and freshwater inputs are expected to increase in HB, our results suggest heterotrophic groups such as MAST-1A and heterotrophic/mixotrophic dinoflagellates from the Order Peridiniales and Gymnodinales may become more prevalent in summer in coastal HB, with potential influence on the local marine food web.
Diatoms in Hudson Strait and Ungava Bay
Samples from the surface and SCM clustered together in HS+UB, suggesting that the communities were driven by similar environmental parameters in the two layers (Figure 2). Unlike HB, the chl a fluorescence profiles in the HS+UB showed that phytoplankton were abundant and likely growing in the upper surface waters. The dominance of photosynthetic species is in keeping with a less stratified water column, as nutrients would be entrained into the photic zone (Figure 1; Table 1). Larger diatom species such as Thalasssiosira spp., Porosira glacialis, and the smaller Chaetoceros neogracilis seemed to be favored, but small photosynthetic flagellates including P. pouchettii and M. polaris were also relatively abundant (Figures 4; S3). Together, these results indicate that environmental conditions at the time of sampling were favorable for the growth of a mixed phytoplankton assemblage in HS+UB. The occurrence of diatoms in the moderately nitrate-depleted surface waters may have been unexpected since nitrate limits the biological production in HB (Kuzyk et al., 2010; Tremblay et al., 2019). However, the weak stratification in the HS+UB suggest that phytoplankton persistence over summer could be supported by regular supply of nitrate from the deeper waters. These pulses of nutrients are favored by hydrodynamic processes driven by wind and tidal mixing in southern HS (Harvey et al., 1997; Straneo and Saucier, 2008; Ferland et al., 2011). The dominant taxa seen in the metabarcodes was consistent with the notion of ongoing nutrient pulses as Chaetoceros and Thalassiosira can quickly become dominant and persist in polar waters when nutrients become available (Lovejoy et al., 2002).
Stratification provides suitable habitat for HAB species
Identifying phytoplankton community composition is of concern for inhabitants of Nunavik as local communities consume marine resources, whose quality could be affected by the taxonomic composition of primary producers (Rapinski et al., 2018; Amiraux et al., 2022). Conditions in temperate coastal regions that promote different inshore communities during summer are reasonably well studied and factors leading to different phytoplankton communities include temperature, stratification regimes, intertidal mixing and the extent of allochthonous inputs, which bring in nutrients but can also change light conditions associated with particles and colored dissolved organic material from the land (Peperzak, 2003; Wells et al., 2015; Joli et al., 2018; Boivin-Rioux et al., 2022). High nutrients and stratification due to warmer surface water temperatures can trigger community transition towards dominance by harmful algal bloom forming species (Anderson et al., 2021). The marine Arctic has traditionally been considered unfavorable for toxin-producing marine harmful algal blooms (HABs), but numerous potentially harmful algae species have been reported to occur in Canadian Arctic waters, including Hudson Bay (Poulin et al., 2011; Pućko et al., 2019; McKenzie et al., 2020; Dhifallah et al., 2021). Toxins produced by many of these algae accumulate in filter-feeding marine bivalves and can be transferred up the food chain with severe health consequences for marine wildlife such as sea birds and marine mammals, in addition to humans (Beltrán et al., 1997; Scholin et al., 2000; Hendrix et al., 2021). Of greatest concern in northern waters are domoic acid and saxitoxin produced respectively by species of Pseudo-nitzschia and Alexandrium that are causative agents of human illness, amnesic and paralytic shellfish poisoning, respectively (Rue and Bruland, 2001). Because HABs are common in temperate and tropical regions, warming Arctic Ocean conditions raise growing concern for the potential expansion of HAB into these regions (Anderson et al., 2021; Hendrix et al., 2021). Moreover, the expected increase of shipping activity associated with the longer open water season, make the coastal HB system particularly vulnerable to the importation of toxic algae from ship ballast and other accidental introductions (Hochheim and Barber, 2014; Andrews et al., 2018).
Our results showed that, although we did not detect any HAB events, Hudson Bay in summer could be a suitable habitat for several toxin producers. Pseudo-nitzschia spp. were present at all sampled stations, especially at the SCM (Figure S3B). Not all species of Pseudo-nitzschia are toxic but toxigenic representatives have been recorded from northern temperate waters, with some events leading to the closure of harvesting sites in Eastern Canada (Bates, 1998; Trainer et al., 2012). In the Arctic, the presence of toxic Pseudo-nitzschia species has been reported from Arctic regions of the United States (Alaska), Greenland, Canadian and Russian coastal waters (Hansen et al., 2011; Poulin et al., 2011; Harðardóttir et al., 2015; Bruhn et al., 2021). Pseudo-nitzschia blooms can be triggered by upwelling events or nutrient inputs from increasing river discharge (reviewed in Trainer et al., 2012).
Five of the six potential harmful dinoflagellates detected in this study; Protoceratium reticulatum, Phalacroma rotundatum, Tripos sp., Alexandrium sp. and Dinophysis sp., have been previously reported in HB (Pućko et al., 2019; Dhifallah et al., 2021). Margalefidinium fulvescens has been documented in the Beaufort and Chukchi Seas (Galaska et al., 2023), but to our knowledge, this is the first record of this species in HB. Previous occurrences of Alexandrium sp. were reported throughout the Canadian Arctic and in HB, particularly in Churchill Harbor and in ballast tanks at Deception Bay Harbor near HS in Nunavik (Pućko et al., 2019; McKenzie et al., 2020; Dhifallah et al., 2021). Because of their capacity to form cysts, Alexandrium spp. are highly adaptable to new regions and can lay dormant in sediments until environmental conditions are suitable for germination and growth (Jensen and Moestrup, 1997; Anderson et al., 2018). Interestingly, the increased number of sequences of Dinophysis sp. and Alexandrium sp. at st732 coincided with the highest water temperature (9.49°C) recorded in our sampling campaign (Table 1; Figure 1). Temperature also showed significant positive correlation with relative abundance of Dinophysis, Alexandrium and Margalefidinium genera (Figure S1). Although mechanisms triggering dinoflagellate blooms are not well understood, several studies have brought out the importance of nutrient ratios and short-term warming events (Granéli et al., 2011; Laabir et al., 2011; Du et al., 2016; Clark et al., 2019). Compared to rDNA, rRNA reads associated to Alexandrium sp. were detected at a greatest number of stations in HB and their higher relative abundance of rRNA at station 732 suggest that this species has the potential to rapidly synthesize proteins and could respond to favorable conditions.
In the Arctic, the warming ocean has been associated with the expansion of the spatial and temporal windows for harmful algal growth. As continuing sea ice loss and longer open water can result in warmer surface waters, the presence of these harmful algae raises valid concerns about future exposure risks of people living near the coast, as these indigenous communities rely on marine resources for basic food security. Monitoring of HAB species during warmer periods in coastal HB should become a priority to ensure food safety. The utilization of metabarcoding targeting the V4 region of the 18S rRNA enables the detection of various harmful taxa. However, as highlighted by Dzhembekova et al. (2017), the V4 region has limited taxonomic resolution at the species level for some genera, as evidenced by multiple species appearing as best hits for Dinophysis, Alexandrium and Tripos genera (Table S2). As sequencing technology becomes more efficient and affordable, the implementation of long-read sequencing using multiple assays could be a practical solution for rapidly identifying and distinguishing harmful algae species (Hatfield et al., 2020), and would limit the need for trained taxonomists.
Conclusion
This study provides the first extensive characterization of microbial eukaryotes in coastal Eastern Hudson Bay, Hudson Strait and the western side of Ungava Bay using a metabarcoding approach. We found that the stability of the water column drove the composition of protist assemblages at the surface with a dinoflagellate-dominated community in the stratified Hudson Bay and a community enriched in diatoms in the more well mixed waters of Hudson Strait. These results support the view that increasing stratification, under the influence of climate warming would favor a more heterotrophic/mixotrophic food web in coastal Hudson Bay. We showed that metabarcoding on the 18S rRNA gene is a suitable tool to detect the occurrence of several potential harmful microalgae. This study provides a time sensitive reference to track the potential introduction of new non-indigenous microbial species into the Hudson Bay System. Future efforts should move toward making DNA metabarcoding in near real time a standard practice to track harmful species in coastal Arctic regions in summer.
Data availability statement
The datasets presented in this study can be found in online repositories. The names of the repository/repositories and accession number(s) can be found below: https://www.ncbi.nlm.nih.gov/, PRJNA627250.
Author contributions
LJ: Conceptualization, Data curation, Formal analysis, Investigation, Methodology, Software, Visualization, Writing – original draft, Writing – review & editing. J-ET: Conceptualization, Funding acquisition, Project administration, Resources, Supervision, Validation, Writing – review & editing, Data curation. CM: Data curation, Formal analysis, Methodology, Validation, Writing – review & editing. CL: Conceptualization, Funding acquisition, Investigation, Methodology, Project administration, Resources, Supervision, Validation, Visualization, Writing – original draft, Writing – review & editing.
Funding
The author(s) declare financial support was received for the research, authorship, and/or publication of this article. This project was conducted as part of the BaySys project funded by Natural Science and Engineering Council of Canada (NSERC) and Manitoba Hydro, with additional support from the project NTRAIN within ArcticNet, a Network of Centers of Excellence of Canada. CL received additional funds from NSERC Discovery and Northern Supplement grants. The authors acknowledge support from the Canada First Research Excellence Fund to Sentinelle Nord for funding CL as part of theme 3 on marine microbiomes and Fonds de Recherche du Québec Nature et Technologies (FRQNT) to Québec Océan. Conductivity, temperature, and depth data were made available through the Amundsen Science program, supported by the Canada Foundation for Innovation and the NSERC. The analyses were conducted using Compute Canada facilities.
Acknowledgments
The authors thank the Canadian Coast Guard crew of the of the CCGS Amundsen for their dedicated and professional logistic support, P. Guillot for processing CTD data and G. Deslongchamps and J. Gagnon for collecting and processing nutrient data. We thank the personnel at the Plateforme d’Analyses Genomiques (IBIS, Université Laval) for aid and advice. Compute Canada provided infrastructure, support, and advice for data analysis. We also acknowledge Marianne Potvin for technical advice and laboratory work.
Conflict of interest
The authors declare that the research was conducted in the absence of any commercial or financial relationships that could be construed as a potential conflict of interest.
Publisher’s note
All claims expressed in this article are solely those of the authors and do not necessarily represent those of their affiliated organizations, or those of the publisher, the editors and the reviewers. Any product that may be evaluated in this article, or claim that may be made by its manufacturer, is not guaranteed or endorsed by the publisher.
Supplementary material
The Supplementary Material for this article can be found online at: https://www.frontiersin.org/articles/10.3389/fmars.2024.1321604/full#supplementary-material
References
Amiraux R., Lavaud J., Cameron-Bergeron K., Matthes L. C., Peeken I., Mundy C. J., et al. (2022). Content in fatty acids and carotenoids in phytoplankton blooms during the seasonal sea ice retreat in Hudson Bay complex, Canada. Elem. Sci. Anthr. 10, 106. doi: 10.1525/elementa.2021.00106
Anderson D. M., Fachon E., Pickart R. S., Lin P., Fischer A. D., Richlen M. L., et al. (2021). Evidence for massive and recurrent toxic blooms of Alexandrium catenella in the Alaskan Arctic. Proc. Natl. Acad. Sci. U. S. A. 118, e210738711. doi: 10.1073/pnas.2107387118
Anderson D. M., Richlen M. L., Lefebvre K. A. (2018). “Harmful algal blooms in the Arctic,” in NOAA Arctic Report Card (Silver Spring: NOAA), 81–87. Available at: https://arctic.noaa.gov/report-card/report-card-2018/harmful-algal-blooms-in-the-arctic/.
Andrews S. (2010). FastQC a quality control tool for high throughput sequence data. Available at: https://www.bioinformatics.babraham.ac.uk/projects/fastqc/.
Andrews J., Babb D., Barber D. G. (2018). Climate change and sea ice: Shipping in Hudson Bay, Hudson Strait, and Foxe Basin, (1980–2016). Elem. Sci. Anthr. 6, 19. doi: 10.1525/elementa.281
Arrigo K. R., Robinson D., Worthen D., Dunbar R., DiTullio G. R., VanWoert M., et al. (1999). Phytoplankton community structure and the drawdown of nutrients and CO2 in the Southern Ocean. Science 283, 365–367. doi: 10.1126/science.283.5400.365
Bates S. S. (1998). Ecophysiology and metabolism of ASP toxin production. Phycol. Ecol. Harmful Algal Blooms 41, 405–426.
Behrenfeld M. J., O’Malley R. T., Siegel D. A., McClain C. R., Sarmiento J. L., Feldman G. C., et al. (2006). Climate-driven trends in contemporary ocean productivity. Nature 444, 752–755. doi: 10.1038/nature05317
Beltrán A. S., Palafox-Uribe M., Grajales-Montiel J., Cruz-Villacorta A., Ochoa J. L. (1997). Sea bird mortality at Cabo San Lucas, Mexico: Evidence that toxic diatom blooms are spreading. Toxicon 35, 447–453. doi: 10.1016/S0041-0101(96)00140-7
Blazewicz S. J., Barnard R. L., Daly R. A., Firestone M. K. (2013). Evaluating rRNA as an indicator of microbial activity in environmental communities: Limitations and uses. ISME J. 7, 2061–2068. doi: 10.1038/ismej.2013.102
Boivin-Rioux A., Starr M., Chassé J., Scarratt M., Perrie W., Long Z., et al. (2022). Harmful algae and climate change on the Canadian East Coast: Exploring occurrence predictions of Dinophysis acuminata, D. norvegica, and Pseudo-nitzschia seriata. Harmful Algae 112, 102183. doi: 10.1016/j.hal.2022.102183
Breitburg D., Levin L. A., Oschlies A., Grégoire M., Chavez F. P., Conley D. J., et al. (2018). Declining oxygen in the global ocean and coastal waters. Science 359, eaam7240. doi: 10.1126/science.aam7240
Bruhn C. S., Wohlrab S., Krock B., Lundholm N., John U. (2021). Seasonal plankton succession is in accordance with phycotoxin occurrence in Disko Bay, West Greenland. Harmful Algae 103, 101978. doi: 10.1016/j.hal.2021.101978
Callahan B. J., McMurdie P. J., Rosen M. J., Han A. W., Johnson A. J. A., Holmes S. P. (2016). DADA2: High-resolution sample inference from Illumina amplicon data. Nat. Methods 13, 581–583. doi: 10.1038/nmeth.3869
Caporaso J. G., Kuczynski J., Stombaugh J., Bittinger K., Bushman F. D., Costello E. K., et al. (2010). QIIME allows analysis of high- throughput community sequencing data. Nat. Publ. Group 7, 335–336. doi: 10.1038/nmeth0510-335
Carmack E. C. (2007). The alpha/beta ocean distinction: A perspective on freshwater fluxes, convection, nutrients and productivity in high-latitude seas. Deep-Sea Res. Part II Top. Stud. Oceanogr 54, 2578–2598. doi: 10.1016/j.dsr2.2007.08.018
Clark S., Hubbard K. A., Anderson D. M., McGillicuddy D. J., Ralston D. K., Townsend D. W. (2019). Pseudo-nitzschia bloom dynamics in the Gulf of Maine: 2012–2016. Harmful Algae 88, 101656. doi: 10.1016/j.hal.2019.101656
Comeau A. M., Li W. K., Tremblay J.É., Carmack E. C., Lovejoy C. (2011). Arctic Ocean microbial community structure before and after the 2007 record sea ice minimum. PloS One 6, e27492. doi: 10.1371/journal.pone.0027492
Déry S. J., Wood E. F. (2005). Decreasing river discharge in northern Canada. Geophys. Res. Lett. 32, 1–4. doi: 10.1029/2005GL022845
Dhib A., Frossard V., Turki S., Aleya L. (2013). Dynamics of harmful dinoflagellates driven by temperature and salinity in a northeastern Mediterranean lagoon. Environ. Monit. Assess. 185, 3369–3382. doi: 10.1007/s10661-012-2797-4
Dhifallah F., Rochon A., Simard N., McKindsey C. W., Gosselin M., Howland K. L. (2021). Dinoflagellate communities in high-risk Canadian Arctic ports. Estuar. Coast. Shelf Sci. 266, 107731. doi: 10.1016/j.ecss.2021.107731
Drinkwater K. F., Jones E. P. (1987). Density stratification, nutrient and chlorophyll distributions in the Hudson Strait region during summer and their relation to tidal mixing. Cont. Shelf Res. 7, 599–607. doi: 10.1016/0278-4343(87)90025-2
Du X., Peterson W., Fisher J., Hunter M., Peterson J. (2016). Initiation and development of a toxic and persistent Pseudo-nitzschia bloom off the Oregon coast in spring/summer 2015. PloS One 11, e0163977. doi: 10.1371/journal.pone.0163977
Dzhembekova N., Urusizaki S., Moncheva S., Ivanova P., Nagai S. (2017). Applicability of massively parallel sequencing on monitoring harmful algae at Varna Bay in the Black Sea. Harmful Algae 68, 40–51. doi: 10.1016/j.hal.2017.07.004
Ferland J., Gosselin M., Starr M. (2011). Environmental control of summer primary production in the Hudson Bay system: The role of stratification. J. Mar. Syst. 88, 385–400. doi: 10.1016/j.jmarsys.2011.03.015
Galaska M. P., Brown S. D., McAllister S. M. (2023). Monitoring biodiversity impacts of a changing arctic through environmental DNA. Oceanography 13, 109–113. doi: 10.5670/oceanog.2023.221
Granéli E., Vidyarathna N. K., Funari E., Cumaranatunga P. R. T., Scenati R. (2011). Can increases in temperature stimulate blooms of the toxic benthic dinoflagellate Ostreopsis ovata? Harmful Algae 10, 165–172. doi: 10.1016/j.hal.2010.09.002
Granskog M. A., Kuzyk Z. Z. A., Azetsu-Scott K., Macdonald R. W. (2011). Distributions of runoff, sea-ice melt and brine using δ18O and salinity data - a new view on freshwater cycling in Hudson Bay. J. Mar. Syst. 88, 362–374. doi: 10.1016/j.jmarsys.2011.03.011
Granskog M. A., Macdonald R. W., Kuzyk Z. Z. A., Senneville S., Mundy C.-J., Barber D. G., et al. (2009). Coastal conduit in southwestern Hudson Bay (Canada) in summer: Rapid transit of freshwater and significant loss of colored dissolved organic matter. J. Geophys. Res. 114, 1–15. doi: 10.1029/2009jc005270
Grasshoff K., Kremling K., Ehrhardt M. (1999). “Determination of nutrients,” in Methods of Seawater Analysis: Third, Completely Revised and Extended Edition (Hoboken: Wiley-VCH), 159–228. doi: 10.1002/9783527613984.ch10
Guillou L., Bachar D., Audic S., Bass D., Berney C., Bittner L., et al. (2013). The Protist Ribosomal Reference database (PR2): A catalog of unicellular eukaryote Small Sub-Unit rRNA sequences with curated taxonomy. Nucleic Acids Res. 41, 597–604. doi: 10.1093/nar/gks1160
Hansen L. R., Soylu S.Í, Kotaki Y., Moestrup Ø., Lundholm N. (2011). Toxin production and temperature-induced morphological variation of the diatom Pseudo-nitzschia seriata from the Arctic. Harmful Algae 10, 689–696. doi: 10.1016/j.hal.2011.05.004
Harðardóttir S., Pančić M., Tammilehto A., Krock B., Møller E. F., Nielsen T. G., et al. (2015). Dangerous relations in the arctic marine food web: Interactions between toxin producing Pseudo-nitzschia diatoms and Calanus copepodites. Mar. Drugs 13, 3809–3835. doi: 10.3390/md13063809
Harvey M., Therriault J. C., Simard N. (1997). Late-summer distribution of phytoplankton in relation to water mass characteristics in Hudson Bay and Hudson Strait (Canada). Can. J. Fish. Aquat. Sci. 54, 1937–1952. doi: 10.1139/cjfas-54-8-1937
Hatfield R. G., Batista F. M., Bean T. P., Fonseca V. G., Santos A., Turner A. D., et al. (2020). The application of nanopore sequencing technology to the study of dinoflagellates: A proof of concept study for rapid sequence-based discrimination of potentially harmful algae. Front. Microbiol. 11. doi: 10.3389/fmicb.2020.00844
Hendrix A. M., Lefebvre K. A., Quakenbush L., Bryan A., Stimmelmayr R., Sheffield G., et al. (2021). Ice seals as sentinels for algal toxin presence in the Pacific Arctic and subarctic marine ecosystems. Mar. Mammal Sci. 37, 1–17. doi: 10.1111/mms.12822
Hochheim K. P., Barber D. G. (2014). An update on the ice climatology of the Hudson Bay System. Arct. Antarct. Alp. Res. 46, 66–83. doi: 10.1657/1938-4246-46.1.66
Hoppenrath M. (2017). Dinoflagellate taxonomy — a review and proposal of a revised classification. Mar. Biodivers. 47, 381–403. doi: 10.1007/s12526-016-0471-8
Jacquemot L., Vigneron A., Tremblay J.-É., Lovejoy C. (2022). Contrasting sea ice conditions shape microbial food webs in Hudson Bay (Canadian Arctic). ISME Commun. 2, 1–10. doi: 10.1038/s43705-022-00192-7
Jensen M.Ø., Moestrup Ø. (1997). Autecology of the toxic dinoflagellate Alexandrium ostenfeldii: Life history and growth at different temperatures and salinities. Eur. J. Phycol. 32, 9–18. doi: 10.1080/09541449710001719325
Joli N., Gosselin M., Ardyna M., Babin M., Onda D. F., Tremblay J.É., et al. (2018). Need for focus on microbial species following ice melt and changing freshwater regimes in a Janus Arctic Gateway. Sci. Rep. 8, 1–11. doi: 10.1038/s41598-018-27705-6
Kassambara A. (2019). ggcorrplot: Visualization of a Correlation Matrix using 'ggplot2'. CRAN, R package version 0.1.4. 1–5. Available at: https://CRAN.R-project.org/package=ggcorrplot.
Kim D. I., Matsuyama Y., Nagasoe S., Yamaguchi M., Yoon Y. H. O., Oshima Y., et al. (2004). Effects of temperature, salinity and irradiance on the growth of the harmful red tide dinoflagellate Cochlodinium polykrikoides Margalef (Dinophyceae). J. Plankton Res. 26, 61–66. doi: 10.1093/plankt/fbh001
Kubiszyn A. M., Wiktor J. M. (2016). The Gymnodinium and Gyrodinium (Dinoflagellata: Gymnodiniaceae) of the West Spitsbergen waters, (1999–2010): biodiversity and morphological description of unidentified species. Polar Biol. 39, 1739–1747. doi: 10.1007/s00300-015-1764-2
Kuzyk Z. Z. A., Macdonald R. W., Tremblay J.É., Stern G. A. (2010). Elemental and stable isotopic constraints on river influence and patterns of nitrogen cycling and biological productivity in Hudson Bay. Cont. Shelf Res. 30, 163–176. doi: 10.1016/j.csr.2009.10.014
Laabir M., Jauzein C., Genovesi B., Masseret E., Grzebyk D., Cecchi P., et al. (2011). Influence of temperature, salinity and irradiance on the growth and cell yield of the harmful red tide dinoflagellate Alexandrium catenella colonizing Mediterranean waters. J. Plankton Res. 33, 1550–1563. doi: 10.1093/plankt/fbr050
Li G., Cheng L., Zhu J., Trenberth K. E., Mann M. E., Abraham J. P. (2020). Increasing ocean stratification over the past half-century. Nat. Clim. Change 10, 1116–1123. doi: 10.1038/s41558-020-00918-2
Li A., Stoecker D. K., Coats D. W. (2000). Mixotrophy in Gyrodinium galatheanum (Dinophyceae): grazing responses to light intensity and inorganic nutrients. J. Phycol. 36, 33–45. doi: 10.1046/j.1529-8817.2000.98076.x
Lovejoy C., Legendre L., Price N. M. (2002). Prolonged diatom blooms and microbial food web dynamics: experimental results from an Arctic polynya. Aquat. Microb. Ecol. 29, 267–278. doi: 10.3354/ame029267
Lovejoy C., Massana R., Pedrós-Alió C. (2006). Diversity and distribution of marine microbial eukaryotes in the Arctic Ocean and adjacent seas. Appl. Environ. Microbiol. 72, 3085–3095. doi: 10.1128/AEM.72.5.3085-3095.2006
Macdonald R. W., Kuzyk Z. Z. A. (2011). The Hudson Bay system: A northern inland sea in transition. J. Mar. Syst. 88, 337–340. doi: 10.1016/j.jmarsys.2011.06.003
Matear R. J., Hirst A. C. (2003). Long-term changes in dissolved oxygen concentrations in the ocean caused by protracted global warming. Glob. Biogeochem. Cycles 17, 1125. doi: 10.1029/2002gb001997
Matthes L. C., Ehn J. K., Dalman L. A., Babb D. G., Peeken I., Harasyn M., et al. (2021). Environmental drivers of spring primary production in Hudson Bay. Elem. Sci. Anthr. 9, 160. doi: 10.1525/elementa.2020.00160
McKenzie C. H., Bates S. S., Martin J. L., Haigh N., Howland K. L., Lewis N. I., et al. (2020). Three decades of Canadian marine harmful algal events: Phytoplankton and phycotoxins of concern to human and ecosystem health. Harmful Algae 102, 101852. doi: 10.1016/j.hal.2020.101852
McMurdie P. J., Holmes S. (2013). Phyloseq: an R package for reproducible interactive analysis and graphics of microbiome census data. PloS One 84, e61217. doi: 10.1371/journal.pone.0061217
Medinger R., Nolte V., Pandey R. V., Jost S., Ottenwälder B., Schlötterer C., et al. (2010). Diversity in a hidden world: potential and limitation of next‐generation sequencing for surveys of molecular diversity of eukaryotic microorganisms. Mol. Ecol. 19, 32–40. doi: 10.1111/j.1365-294X.2009.04478.x
Millette N. C., Pierson J. J., Aceves A., Stoecker D. K. (2017). Mixotrophy in Heterocapsa rotundata: A mechanism for dominating the winter phytoplankton. Limnol. Oceanogr. 62, 836–845. doi: 10.1002/lno.10470
Morency C., Jacquemot L., Potvin M., Lovejoy C. (2022). A microbial perspective on the local influence of Arctic rivers and estuaries on Hudson Bay (Canada). Elem. Sci. Anthr. 10, 9. doi: 10.1525/elementa.2021.00009
Onda D. F. L., Medrinal E., Comeau A. M., Thaler M., Babin M., Lovejoy C. (2017). Seasonal and interannual changes in ciliate and dinoflagellate species assemblages in the Arctic Ocean (Amundsen Gulf, Beaufort Sea, Canada). Front. Mar. Sci. 4. doi: 10.3389/fmars.2017.00016
Peperzak L. (2003). Climate change and harmful algal blooms in the North Sea. Acta Oecologica 24, S139–S144. doi: 10.1016/S1146-609X(03)00009-2
Poulin M., Daugbjerg N., Gradinger R. (2011). The pan-Arctic biodiversity of marine pelagic and sea-ice unicellular eukaryotes: a first-attempt assessment. Mar. Biodivers. 41, 13–28. doi: 10.1007/s12526-010-0058-8
Pućko M., Dionne K., Michel C. (2019). Occurrence of toxin-producing marine algae in the Canadian Arctic and adjacent waters (Winnipeg: Fisheries and Oceans Canada), 27p. Available at: https://publications.gc.ca/site/eng/9.874970/publication.html.
Rapinski M., Cuerrier A., Harris C., Lemire M. (2018). Inuit Perception of Marine Organisms: From folk classification to food harvest. J. Ethnobiol. 38, 333–355. doi: 10.2993/0278-0771-38.3.333
Rintala J.-M., Hällfors H., Hällfors S., Hällfors G., Majaneva M., Blomster J. (2010). Heterocapsa arctica Subsp. frigida Subsp. Nov. (peridiniales, Dinophyceae)—Description of a new dinoflagellate and its occurrence in the Baltic Sea. J. Phycol. 46, 751–762. doi: 10.1111/j.1529-8817.2010.00868.x
Roff J. C., Legendre L. (1986). Physico-chemical and biological oceanography of Hudson Bay. Elsevier Oceanogr. Ser. 44, 265–292. doi: 10.1016/S0422-9894(08)70907-3
Rue E., Bruland K. (2001). Domoic acid binds iron and copper: A possible role for the toxin produced by the marine diatom Pseudo-nitzschia. Mar. Chem. 76, 127–134. doi: 10.1016/S0304-4203(01)00053-6
Schlitzer R. (2002). Interactive analysis and visualization of geoscience data with Ocean Data View. Comput. Geosciences 28, 1211–1218. doi: 10.1016/S0098-3004(02)00040-7
Scholin C. A., Gulland F., Doucette G. J., Benson S., Busman M., Chavez F. P., et al. (2000). Mortality of sea lions along the central California coast linked to a toxic diatom bloom. Nature 403, 80–84. doi: 10.1038/47481
Stadnyk T. A., MacDonald M. K., Tefs A., Déry S. J., Koenig K., Gustafsson D., et al. (2020). Hydrological modeling of freshwater discharge into Hudson Bay using HYPE. Elem. Sci. Anthr. 8, 43. doi: 10.1525/elementa.439
Straneo F., Saucier F. (2008). The outflow from Hudson Strait and its contribution to the Labrador Current. Deep-Sea Res. Part Oceanogr. Res. Pap. 55, 926–946. doi: 10.1016/j.dsr.2008.03.012
Trainer V. L., Bates S. S., Lundholm N., Thessen A. E., Cochlan W. P., Adams N. G., et al. (2012). Pseudo-nitzschia physiological ecology, phylogeny, toxicity, monitoring and impacts on ecosystem health. Harmful Algae 14, 271–300. doi: 10.1016/j.hal.2011.10.025
Tremblay J.É., Gagnon J. (2009). “The effects of irradiance and nutrient supply on the productivity of Arctic waters: A perspective on climate change,” in Influence of climate change on the changing arctic and sub-arctic conditions. Ed. Nihoul J. (The Netherlands: Springer), 73–93.
Tremblay J.É., Lee J.-H., Gosselin M., Belanger S. (2019). “Nutrient dynamic and marine biological productivity in the greater Hudson Bay marine region,” in An integrated regional impact study (IRIS) ArcticNet. University of Manitoba and ArcticNet (University of Manitoba, Winnipeg), 225–244.
Wells M. L., Trainer V. L., Smayda T. J., Karlson B. S. O., Trick C. G., Kudela R. M., et al. (2015). Harmful algal blooms and climate change: Learning from the past and present to forecast the future. Harmful Algae 49, 68–93. doi: 10.1016/j.hal.2015.07.009
Worden A. Z., Follows M. J., Giovannoni S. J., Wilken S., Zimmerman A. E., Keeling P. J. (2015). Rethinking the marine carbon cycle: factoring in the multifarious lifestyles of microbes. Science 347, 1257594. doi: 10.1126/science.1257594
Keywords: stratification, Hudson bay complex, coastal protists, diatoms, dinoflagellates, harmful algae
Citation: Jacquemot L, Tremblay J-É, Morency C and Lovejoy C (2024) Stratification and summer protist communities in the Arctic influenced coastal systems of Nunavik (Québec, Canada). Front. Mar. Sci. 11:1321604. doi: 10.3389/fmars.2024.1321604
Received: 14 October 2023; Accepted: 10 January 2024;
Published: 29 January 2024.
Edited by:
Zhang-Xian Xie, Xiamen University, ChinaReviewed by:
Yunyun Zhuang, Ocean University of China, ChinaJean-François Mangot, Spanish National Research Council (CSIC), Spain
Copyright © 2024 Jacquemot, Tremblay, Morency and Lovejoy. This is an open-access article distributed under the terms of the Creative Commons Attribution License (CC BY). The use, distribution or reproduction in other forums is permitted, provided the original author(s) and the copyright owner(s) are credited and that the original publication in this journal is cited, in accordance with accepted academic practice. No use, distribution or reproduction is permitted which does not comply with these terms.
*Correspondence: Loïc Jacquemot, bG9pYy5qYWNxdWVtb3QuMUB1bGF2YWwuY2E=