- 1Marine Biotechnology Group, National Institute of Ocean Technology, Ministry of Earth Sciences, Government of India, Chennai, India
- 2Biological Oceanography Division, Council of Scientific and Industrial Research - National Institute of Oceanography (CSIR-NIO), Dona Paula, Goa, India
Deep sea bacterial communities demonstrate remarkable adaptability to high-pressure environments coupled with low temperatures which has sparked curiosity about their diversity and exceptional metabolic pathways. Additionally, bacteria in the deep sea exert a substantial influence over various biogeochemical processes. To date, we have relatively very little information about the deep-sea bacterial communities and, they remain largely unexplored. We investigated the variability in the physicochemical conditions, heavy metals and their influence on deep-sea bacterial community structure across three different depths in the Bay of Bengal. The structural and metabolic diversity of deep-sea sediment microbial communities were examined through culture-based sequencing of 16S rRNA genes, ecto-enzymatic studies, and community-level physiological profiling. Bacillota was the most dominant phylum representing 61% of the cultured bacterial isolates, while the remaining belonged to Actinomycetota and Pseudomonodata. Five potential novel species belonging to the genera Fictibacillus, Lysinibacillus, Salinicola, Robertmurraya and Blastococcus were identified. The extracellular enzymatic activity was positive for >50% of the bacterial isolates, wherein the genera Bacillus and Micromonospora exhibited versatile profiles. High metabolic diversity was recorded through the carbon substrate utilization profiles indicating that microbial communities are active participants in biogeochemical cycles in the deep sea. The most prominently utilized carbon substrates were α-cyclodextrin, glucose-1-phosphate, D-xylose, glycogen, and 2-hydroxy benzoic acid which serve as organic substrates for microbial metabolism, facilitating the decomposition of organic matter and, recycling carbon in deep-sea ecosystems. Multivariate statistical analyses confirmed that the environmental variables had a profound influence on the bacterial community. The findings shed light on spatial variability in the bacterial community structure, enzyme activity and metabolic profiles, and enhance our understanding of Bay of Bengal deep-sea sedimentary microbial ecology.
Introduction
Earth is often referred to as the “blue planet”, owing to the fact that ~71% of the planet is covered by the oceans. It is estimated that ~95% of the total ocean volume comprises of the deep sea (Nemergut et al., 2011) lying below 200m depth (Rogers, 2015) with predominantly high hydrostatic pressures; low temperatures (2-4°C) and lack of sunlight (Nogi, 2017). The deep sea plays a notable role in carbon sequestration helping to mitigate the impact of anthropogenic carbon emissions on the climate (Thurber et al., 2014). The microbial oxidation of methane in the deep sea prevents the release of potent greenhouse gases in the atmosphere (Jiang et al., 2022). Additionally, the role of the deep sea in nutrient regeneration and driving global biogeochemical cycles is essential for the sustenance of primary and secondary production in the oceans (Danovaro, 2018). Furthermore, the deep sea is also a reservoir of huge fish stocks, mineral resources, oil and gas, offering enormous potential for bioprospecting (Kato et al., 2011; Thurber et al., 2014; Sweetman et al., 2017).
The prevalence of extremities in the deep-sea environment supports unique habitats and biota (Sogin et al., 2006; Ramirez-Llodra et al., 2010). The deep-sea microorganisms, in particular, exhibit remarkable metabolic adaptations that enable them to thrive in extreme and often harsh environments (Padmanaban et al., 2017; Garel et al., 2021). Due to the absence of sunlight in the deep ocean, these microbes rely on chemosynthesis rather than photosynthesis for energy production. They harness chemical compounds such as hydrogen sulfide, methane, and dissolved iron as energy sources, deriving metabolic energy from the oxidation of these compounds (McCollom, 2000; Jørgensen and Boetius, 2007). These microorganisms are essentially heterotrophs that play a pivotal role in carbon and nutrient cycling by breaking down organic matter (OM), recycling essential elements, and contributing to the overall resilience and stability of the deep-sea ecosystem (Falkowski et al., 2008; Zehr and Kudela, 2011; Acinas et al., 2021).
The bacterial diversity of the deep sea has gained global attention in recent years encompassing the world oceans. Studies have shown that the deep sea sediments harbour a rich diversity of bacteria including, Pseudomonodata, Planctomycetota, Bacteroidota, Acidobacteriota, Actinomycetota, Chloroflexota, Nitrospirota, Gemmatimonadota and Bacillota (Sogin et al., 2006; Jørgensen and Boetius, 2007; Lauro and Bartlett, 2008; Zinger et al., 2011; Corinaldesi, 2015; Walsh et al., 2016; Gawas et al., 2019). However, a significant proportion of microbes in the deep-sea sediments remain taxonomically unidentified, implying that a sizable fraction of the global microbial diversity is undiscovered (Scheckenbach et al., 2010; Zinger et al., 2011; Danovaro et al., 2016). Moreover, the paucity of cultured representatives from the deep-sea sediments poses a significant challenge in studying biodiversity and hinders our ability to accurately assess their role in ecological processes (Dong et al., 2019).
The Bay of Bengal (BOB) forming the northeastern part of the Indian Ocean is the largest triangular basin in the world, that is approximately 2090 kilometres long, 1600 kilometres wide and has an average depth of approximately 2600 metres (Jasna et al., 2020). Multiple riverine influxes (1.6 × 1012 m3 yr–1; UNESCO, 1979) from the Indian subcontinent and Himalayan range, such as the Ganges, Brahmaputra, Irrawaddy, and Salween on the north and Mahanadi, Godavari, Krishna, and Kaveri on the west, deposit sediments in BOB, supporting a complex marine microbiome (Varkey et al., 1996; Padmanaban et al., 2017; Angelova et al., 2019). Studies in the BOB focussing on benthic bacterial diversity remain underrepresented and have gained impetus only in recent years (Verma et al., 2017; Rajpathak et al., 2018; Angelova et al., 2019; Padmanaban et al., 2019; Lincy and Manohar, 2020; Parvathi et al., 2020; Gu et al., 2022; Guo et al., 2022; Marimuthu et al., 2022). Despite the critical importance of deep-sea microbenthos, our knowledge is limited and lags significantly behind that of coastal ecosystems due to several factors, including the logistical challenges of accessing deep-sea environments. Additionally, the extreme conditions of the deep sea pose unique challenges for studying these organisms. Therefore, efforts to bridge this knowledge gap and understand the biodiversity and ecological processes of deep-sea ecosystems are crucial for a comprehensive understanding of microbial interactions in the deep sea.
The present study focuses on a comparative analysis of the prevailing environmental conditions and resultant bacterial diversity across three different depths of the BOB. Community-level physiological profiling based on sole carbon substrates was carried out to investigate the metabolic capacities of the deep-sea microbial communities. Additionally, distinct preferences of the bacterial communities for different culture media and ecto-enzyme activity were studied. Understanding these preferences can provide valuable information for studying the microbial ecology and biogeochemical processes in the BOB. The results of this study can have implications for various fields, including biotechnology and environmental conservation, as deep-sea bacteria hold enormous untapped potential for novel enzymes and bioremediation strategies.
Methods
Sample collection and analysis of sediment characteristics
Sediment samples were collected on-board the ocean research vessel Sagar Nidhi (Cruise No. SN142), from three different locations in the northern Bay of Bengal (BOB) during June 2019 (Figure 1). The sampling was carried out using the gravity corer from the depths of 2725m, 2191m, and 955m for the stations BOB16N, BOB17N, and BOB20N, respectively. Physical properties were measured using Ocean Seven 320Plus CTD equipped with Idronaut sensors. The detection limits for temperature, salinity, pH, and pressure were 0.001°C, 0.001 PSU (calculated), 0.001, and 0.05 dbar, respectively.
To estimate the concentration of heavy metals, sediment samples were initially dried, ground using an agate pestle and mortar, and subsequently sieved through a 2 mm sieve following standard procedures (USEPA, 2001). Analytical-grade chemicals were utilized, and Milli-Q water (Millipore, USA) was used to make the solutions. The Teflon and polypropylene containers were soaked in 5% HNO3 for 24 hours, followed by rinsing with Milli-Q water and drying. In a microwave digestion system (Anton Paar), one gram of dried sediment was digested at 140°C with 5 ml HNO3 (Suprapur, Merck) as per APHA, 2005. The digested, clear solution was transferred to centrifuge tubes, and 10 ml of Milli-Q water (Millipore, resistivity: 18.2 MΩ/cm) was added to make up the volume (Cortada and Collins, 2013). The concentrations of metals were determined using Inductively Coupled Plasma Mass Spectrometry (ICP-MS) (Agilent-7500). Replicate analytical blanks were analyzed alongside certified reference materials to check for potential contamination, by following protocol adopted by Jha et al. (2019). The total organic carbon (TOC) in sediment was determined using the modified Walkley-Black wet oxidation method (Gaudette et al., 1974), and OM was calculated by multiplying the TOC by a factor of 1.724 (Trask, 1939). The sediment texture was analysed by wet sieve and pipette method to classify it into sand, silt, and clay (Buchanan, 1984).
Isolation of bacteria
Sterile 15ml polypropylene conical tubes were used to collect three replicate sediment sub-samples of 5cm layer from each core. For the isolation of heterotrophic bacteria, on-board processing of samples was done by addition of 2g of sediment to 18ml sterile seawater. The slurry was vigorously vortexed three times for 1 minute each with an intermittent gap of 30 seconds and kept at a shaker (200rpm) for 20 minutes. The particles were allowed to settle for 5 minutes and diluted at four gradients from 100 to 10-4. 100μL of each dilution was spread plated in triplicate on different media (HiMedia, India), ZoBell marine agar 2216 (ZMA), starch casein agar (SCA), ISP7, and Streptomyces agar (SMA), and incubated at 20°C in the dark for 3-14 days. Considering low-nutrient deep-sea conditions, growth medium were used at 1/5 of their original strength in sterile seawater. Morphologically unique bacterial colonies were counted and individually chosen from each culture plate through visual examination using a stereomicroscope (Nikon SMZ 800).
Axenic strains were achieved through three quadrant streaking cycles on specific media. Pure isolates were preserved in 30% (v/v) glycerol with 50% (v/v) culture media broth at -80°C and maintained on media slants at 4°C for further analysis. Strains were grouped based on the colony characteristics like morphology, pigment production, and Gram-staining, and a representative from each phenotype was subjected to molecular identification and extracellular enzyme screening.
DNA extraction and 16S rDNA amplification
The phenol-chloroform-isoamyl alcohol (25:24:1) extraction method with proteinase K treatment was used to extract the genomic DNA from the axenic cultures (Sambrook and Russell, 2001). The bacterial cells were initially lysed by bead-beating with ceramic beads to break open and release the DNA physically. To eliminate any remaining salt or impurities, the extracted DNA pellet was washed twice with 70% ethanol, air-dried, and dissolved in TE buffer. The primers, 27F (5′-AGRGTTTGATCMTGGCTCAG-3′) and 1525R (5′-AAGGAGGTGWTCCARCC-3′) or 1492R (5′-TACGGYTACCTTGTTACGACTT-3′) were used for 16S rDNA amplification of bacteria. The 50µl PCR reaction mixture contains 1.2µl (~ 60 ng) of template DNA, 0.5pmol/µl of each primer, 10% BSA of concentration 10mg/ml, and Emerald AMP GT PCR Master Mix (Takara, Japan) at 1X final concentration. Reactions without templates were used as negative control. The PCR conditions were as follows: a 5-minute initial denaturation at 95°C, 35 cycles of 1 minute at 94°C, 1 minute at 55°C, and 1.2 minutes at 72°C, and a 10-minute final extension at 72°C. After ethidium bromide staining, the reaction products were observed on an agarose gel (1%) under UV illumination and purified using the QIAquick gel extraction kit (Qiagen) according to the manufacturer’s instructions.
Sequencing and taxonomic assignment
The purified PCR products were sequenced after setting sequencing reactions with BigDye Terminator Kit v. 3.1 (Applied Biosystems). The initial PCR primers along with additional internal primers 704F (5′-GTAGCGGTGAAATGCGTAGA-3′) and 907R (5′-CCGTCAATTCCTTTGAGTTT-3′) were used for setting up the sequencing reactions individually and after purification the run was carried out in Applied Biosystems 3730 Genetic Analyzer. ChromasPro version 1.5 software (www.technelysium.com.au/ChromasPro) was used to manually assemble and edit the partial 16S rDNA sequences of each strain to produce near full-length sequences. The taxonomic assessment of sequences was carried out using the BLASTN (Altschul et al., 1997) program against the EzBioCloud database containing type strains with validly published prokaryotic names (http://www.ezbiocloud.net; Yoon et al., 2017). The top three sequences with the highest similarity were downloaded for each strain and the multi-sequence alignment of nucleotide sequences was carried out using ClustalW in MEGA11 alignment explorer (Kumar et al., 2018). The Neighbor-Joining method in MEGA11 was used to infer the evolutionary history, the evolutionary distances were computed using the Maximum Composite Likelihood method and are in the units of the number of base substitutions per site. The final dataset contained 1621 positions and all ambiguous positions were removed for each sequence pair. The robustness of the topology of phylogenetic trees was evaluated by using bootstrap analysis with 1000 resamplings.
Ectoenzymatic hydrolytic activity
Screening for the production of extracellular hydrolytic enzymes was carried out by spotting bacterial isolates onto respective agar medium. A solid medium containing marine agar 2216 supplemented with 2% (w/v) of sterile skim milk, extra pure gelatin, and soluble starch, respectively, was used to assess the generation of extracellular hydrolases like caseinase, gelatinase, and amylase, respectively (Bjerga et al., 2014). The hydrolytic zone formations were monitored on the plates for up to two weeks while they were incubated at 20°C. The plates were flooded with acidic mercuric chloride (Balan et al., 2012) and Lugol’s iodine solution to determine gelatinase and amylase activity respectively.
Metabolic diversity of microbial communities
The community-level physiological profiling (CLPP) of the deep-sea sediment samples in triplicates was performed using BIOLOG® EcoPlates™ to assess the ability of heterotrophic microbial communities to utilize different carbon substrates. The Ecoplates contain 31 single carbon sources and tetrazolium dye. The utilization of any carbon source by the microbial community results in the respiration-dependent reduction of the dye and purple colour formation that can be quantified and monitored over time (Garland, 1997). For CLPP, 2 g of sediment was mixed with 18 ml sterile 50% artificial seawater and kept on the shaker for 30 min. After settling for 5 minutes,150 μL of suspension was inoculated in each well, and the plates were incubated at 20°C for 144 h. Every 24 h, optical density (OD) at 590 nm was measured using a microplate reader to monitor the carbon source utilization by microbial communities. Each Ecoplate contains the control well in triplicate, which is devoid of any carbon sources. The average well-color development (AWCD) was measured to represent the metabolic proficiency of microbes. The AWCD was calculated as:
where Ci is the absorbance value of each well at 590 nm, R is the absorbance value of the blank control well, and n is representative of the number of wells. When plotted over time, the AWCD of EcoPlate represented the activity or overall carbon substrate utilization potential of heterotrophic microbial communities (Garland and Mills, 1991).
Data analysis
To assess the structural properties of the bacterial community, the most broadly utilized univariate diversity indices such as number of taxa (S), species richness (Margalef’s d), Shannon-Wiener diversity index (H′, loge), Simpson’s index (1- Lambda′), and Pielou’s evenness (J′) were estimated using PRIMER v 6.1 (Clarke and Gorley, 2006). A two-way ANOVA was conducted to investigate the differences in microbial diversity indices between different stations and the culture media used. In case of significant interaction between the stations and media used, an analysis of simple main effects for media was performed with statistical significance receiving a Bonferroni adjustment for multiple pairwise comparisons between media at each station. Prior to conducting the two-way ANOVA, all the assumptions were checked for the data. The data had no outliers, normality was assessed using Shapiro-Wilk’s test, and homogeneity of variances was assessed using Levene’s test. The microbial community’s metabolic diversity (Shannon index H′) and substrate richness (number of carbon substrates utilized, R) were calculated using BIOLOG® with a threshold AWCD value of ≥0.35 (OD 590nm). To identify carbon sources which were driving the variability in the metabolic patterns of bacterial communities, Principal Component Analysis (PCA) was carried out on standardized OD 590nm values for each substrate using the PASW Statistics 18 software. A correlation matrix based on the Kaiser-Meyer-Olkin measure of sampling adequacy and Bartlett’s test of Sphericity was used for factor analysis. The microbial abundance data was transformed to log(x+1) values to smooth the extremes in data and allow less represented taxa to exert same influence while simultaneously reducing the potentially overwhelming impact of the most exorbitant taxa. The Bray-Curtis resemblance matrix was created using the transformed data, and non-metric multi-dimensional scaling (nMDS) was used to observe the bacterial taxa assemblage pattern (Clarke and Warwick, 1994). Whereas, the relationship between taxa and the environmental parameters within the sites was assessed by a direct gradient analysis of the multivariate linear regression ordination technique (canonical correspondence analysis, CCA).
Data availability
The 16S rDNA sequence data generated for all the deep-sea bacterial strains obtained in the study was submitted in the NCBI GenBank database (http://www.ncbi.nlm.nih.gov/GenBank/index.html) under the accession numbers OR623173 – OR623227 and are included in the Supplementary Table 1.
Results and discussion
Environmental factors and heavy metal distribution in deep-sea
It is well-established that microbes inhabiting deep-sea sediments represent a significant proportion of the biosphere (Danovaro et al., 2016). Their abundance and diversity are largely dependent on environmental conditions such as OM content and sediment texture (Parkes et al., 2014). Table 1A, summarizes the spatial variation of environmental characteristics of deep-sea in the three study locations. The water temperature showed an inverse relationship with the depth, the lowest recorded temperature (1.90 °C) was at the depth of 2725m in BOB16N, and <1 °C rise in temperature (2.41 °C) was observed at a depth of 2191m in BOB17N. The maximum temperature (7.18 °C) was recorded in BOB20N at a depth of 955m. In the case of salinity (34.77 to 34.99 PSU) and pH (7.95 to 8.13), minimum variations were noted across the three locations. OM varied between 0.68% to 2.45% with the highest concentration in BOB20N and the least in BOB17N. The study observed lower concentrations of OM at increasing depths in the deep-sea or in the middle region of Bengal Fan in comparison to the upper region. The Northeastern Indian Ocean is intensely influenced by one of the largest fluvial systems on Earth comprising of the Ganga-Brahmaputra-Meghna rivers (Contreras-Rosales et al., 2016). Transporting substantial sediment and nutrient loads, these rivers significantly elevate the OM concentration, particularly noticeable in the study location BOB20N, as compared to BOB16N and BOB17N (Figure 1). The sediment texture analyses revealed that BOB16N was mostly clayey, BOB17N possessed a mixture of silt and clay and lastly, BOB20N was silty. The obtained results revealed a clear demarcation in sediment texture between the three different depths among the study locations in the BOB. Recent studies have suggested that sediment provenance in the BOB is mostly composed of terrestrial detrital matter with minor contributions of marine autogenous OM, and wind dust (Weber et al., 2003; Tripathy et al., 2014). The variation in sediment textures may be attributed to a combination of factors such as sediment sources, transportation processes and depositional environments which change as we go deeper into the seafloor (Ye et al., 2022). Closer to the coast, sediments tend to be coarser and more variable due to proximity to terrestrial sources, wave action, and river input resulting in a good proportion of sand, gravel and mud (Gunaratna et al., 2019). In contrast, deep-sea sediments are typically composed of fine-grained particles such as silt and clay which accumulate slowly over time and are influenced by factors such as ocean currents, nutrient availability, and the presence of OM (Liu et al., 2023).

Table 1 (A) Geographical details and environmental characteristics (mean ± SD) of the Bay of Bengal deep-sea samples; (B) Trace metals concentration (ppm) in the deep-sea sediment samples in the present study, average crustal abundances, and other deep sea locations; (C) Diversity and richness estimate indices for the samples and growth media.
The concentration of metals in the sea is regulated by natural processes such as biological uptake, particulate matter scavenging, advection of water masses and Aeolian transport of terrestrial materials (Jones and Murray, 1984; Wangersky, 1986). While some heavy metals, including Al, Fe, and Mn, have a lithogenic origin, human activity is largely responsible for mobilising heavy metals like Pb, Cd, Zn, Cr, Cu, and Ni. (Serrano et al., 2011). In addition to this, factors like ocean circulation patterns, temperature and water chemistry can affect the transport, dispersion and precipitation of heavy metals (Tuohy et al., 2015). Majority of the heavy metal concentrations in all three locations retained a trend in the following order: Fe>Al>Mn>Zn>Ni (Table 1B). Among all the heavy metals determined, significantly high concentrations were observed for Fe (avg. 25584.43 ppm) and Al (avg. 14920.37 ppm). The OM and sediment grain size in the coastal environment are important controllers of metal bioavailability in the coastal environment (Jha et al., 2021). As the proportion of mud (silt and clay) increased at greater depths i.e., BOB16N and BOB17N a corresponding elevation in the concentrations of Fe, Co, Pb, and Ni was observed indicating that these metals may be adsorbed by fine sediment particles and such pattern has also been documented in coastal regions where muddy sediment due to the smaller size, represents a higher adsorption capacity for metals (Pandey et al., 2021).
Table 1B provides a comparison of the average range of trace metal concentrations found in the sediments of the present study with those found in other deep-sea sediments. The cumulative data shows that the concentrations of Fe and Al were the highest in comparison to the other metals indicating that they are abundant in marine sediments. Additionally, the abundance of Fe and Al in the study locations can be explained by their status as the third and fourth most abundant elements on Earth, primarily originating from lithogenic sources (Serrano et al., 2011). This suggests that the high concentrations of Fe and Al in deep-sea ecosystems are likely derived from natural geological processes rather than human activities. Inconsistencies in the concentration of Mn within the current study locations as well as in the reported studies could be attributed to variability in the reductive dissolution of Mn in the sediment (Pakhomova et al., 2007). The sampling sites are also under the influence of riverine discharge in the Bengal Fan region, which is the largest submarine fan on Earth. Additionally, the geological and tectonic forces can lead to variations in the underlying rock composition and mineral deposits, affecting the availability of heavy metals (Cheng et al., 2022). Conversely, the concentrations of Pb, Cd, Zn, Cr, Cu, and Ni remained well within the average crustal abundance concentration. Overall, the concentrations of heavy metals exhibit significant spatial variation in different deep-sea regions as a result of complex interactions between natural geological processes, local environmental conditions and the unique characteristics of each marine region (Budianto and Lestari, 2018; Sattarova and Aksentov, 2021; Marimuthu et al., 2022).
Deep-sea bacterial community identification and taxonomy
The deep-sea sediments host the largest fraction of microbenthos on Earth which play a vital role in ocean productivity (Corinaldesi, 2015; Danovaro et al., 2016). The average number of heterotrophic bacteria (colony-forming units, CFUs) obtained in the deep-sea sediment samples was highest at station BOB20N (1.67×105 CFU/g), followed by BOB16N (6.06×104 CFU/g) and BOB17N (5.65×104 CFU/g). A total of 76 morphologically distinct bacterial strains were isolated from the BOB16N, BOB17N, and BOB20N sediment samples on different growth media, and based on standard colony morphological characteristics these strains were classified into 55 groups cumulatively for all three stations. The growth medium Streptomyces agar and Zobell Marine agar showed major population of heterotrophic bacteria, 1.49×105 and 1.21×105 CFU/g, respectively in comparison to other media such as ISP7 (6.99×104 CFU/g), and Starch casein agar (5.75×104 CFU/g). The 16S rRNA gene sequence has overwhelmingly emerged as the most frequently employed genetic marker for bacterial phylogenetics and taxonomy, serving as a standard housekeeping gene. By utilizing a variety of sequencing primers, we achieved successful sequencing of more than 1350 base pairs of the 16S rRNA gene for all the bacterial groups obtained in this study (Supplementary Table 1). The strains were identified by comparing the 16S rRNA gene sequence to nucleotide sequences within the NCBI database (http://www.ncbi.nlm.nih.gov/) using the Basic Local Alignment Search Tool (BLAST) and EzBioCloud database containing type strains sequences. We obtained a total of 55 bacterial strains which belonged to 39 species and 24 genera. The majority of the bacterial isolates clustered with the phyla Bacillota (61.54%), while the remaining belonged to Actinomycetota (20.51%), and Pseudomonodata (17.95%). Padmanaban et al. (2019) also reported that the majority of isolated microorganisms from deep-sea sediments in the Bay of Bengal and the Andaman Sea belonged to Bacillota. It was found to be the second dominating phylum in deep-sea sediments from the Bay of Bengal and volcanic Barren Island in the Andaman Sea, according to a culture independent study by Verma et al. (2017). Among the 14 families obtained, Bacillaceae, had the highest representation with 21 different species (53.84%) (Figure 2). Bacillus was the dominant genus discovered within this phylum and formed four distinct clades in the phylogenetic tree (Figure 2), its members are known for their remarkable metabolic versatility and capacity to flourish under extreme conditions. While the wide distribution of the genus Bacillus is known, this study reaffirmed its presence in deep-sea sediments.
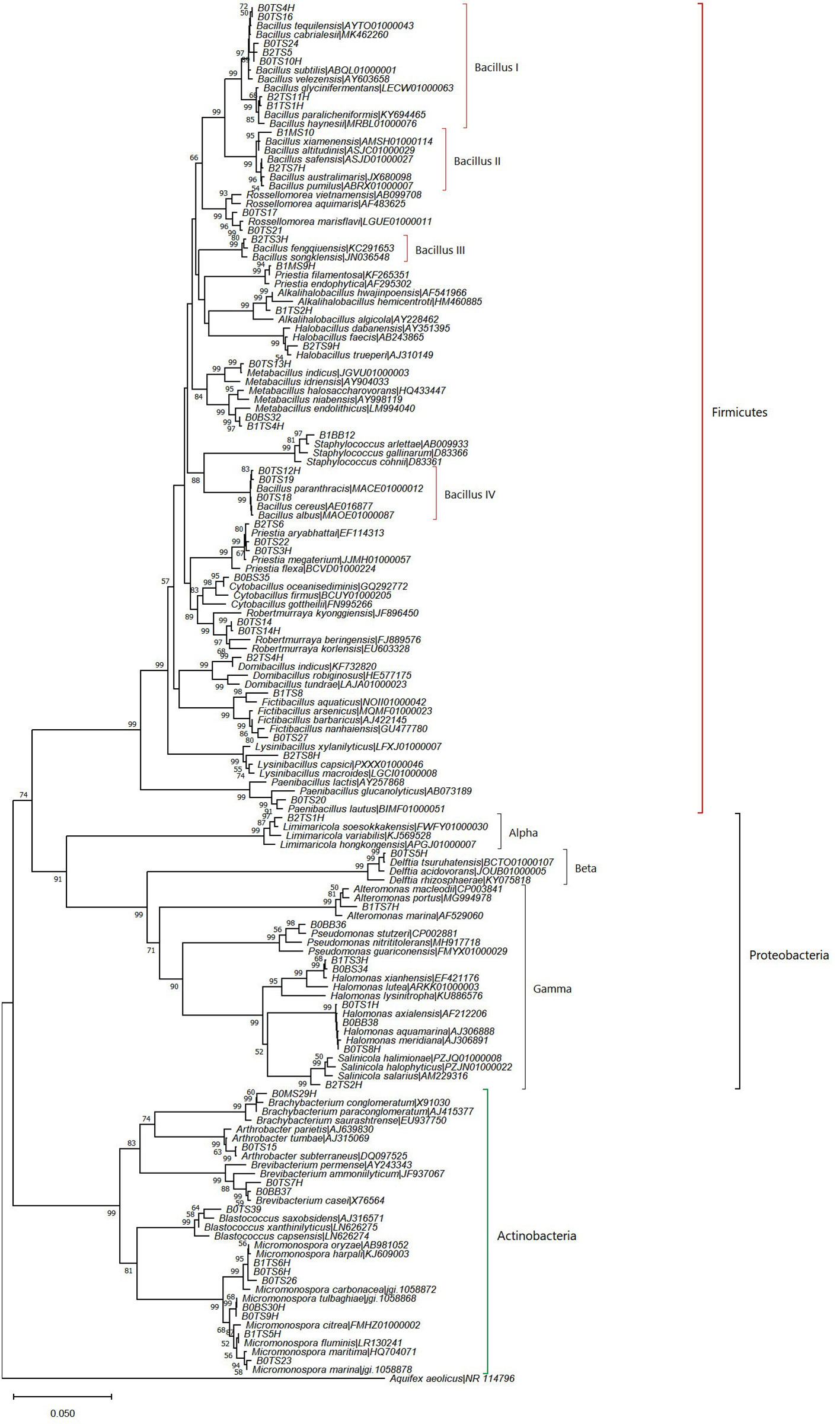
Figure 2 Neighbor-joining phylogenetic tree based on nearly complete and aligned 16S rDNA sequences of isolated bacteria and their nearest type strains. Bootstrap values (≥50%) of 1000 resamplings are shown at branch points. Aquifex aeolicus (NR 114796) was used as an outgroup.
The dominance of Actinomycetota in deep-sea sediment has been reported from the Southwest Indian Ridge, CIOB (Chen et al., 2016) , BOB (Verma et al., 2017) and the Southern Okinawa trough, China Sea (Dang et al., 2009). Several investigations from other marine environments, like the CIOB (Gawas et al., 2019), the Atlantic Ocean (da Silva et al., 2013), and the Mariana Trench (Ma et al., 2022), also report the isolation of Actinomycetota from deep-sea sediment. Moreover, microbial diversity studies done through culture-independent metagenomes from the sediments of the Arabian Sea (Nair et al., 2017; Vipindas et al., 2020) and the Pacific Ocean (Hongxiang et al., 2008) revealed the presence of Actinomycetota. In the present study, the dominant genus found within the Actinomycetota phylum is Micromonospora. Species of this genus are reported from deep-sea sediment in the Mediterranean Sea, Mariana Trench in the Pacific Ocean, and other marine regions. Its members have well-developed pathways for the biosynthesis of secondary metabolites for non-ribosomal peptides and polyketides, (Gärtner et al., 2016). In a previous study on the cultured biodiversity of deep-sea sediment samples from the Atlantic Ocean (da Silva et al., 2013), and the Mariana Trench (Ma et al., 2022), the prevalence of Pseudomonodata were found as major groups. Moreover, culture-independent approaches revealed the presence of bacterial communities belonging to Pseudomonodata in sediments from the central Mediterranean Sea (Mahmoudi et al., 2020), Arabian Sea (Nair et al., 2017; Vipindas et al., 2020), Pacific Ocean (Hongxiang et al., 2008), and China sea (Chen et al., 2023). In this study, within Pseudomonodata, the class Gammaproteobacteria showed the highest representation (71.42%). The genus Halomonas of this class was most frequently isolated from our study locations which can be attributed to the vast range of salinity, temperature, and pressure that Halomonas can thrive in as a result of its physiological and metabolic adaptability (da Silva et al., 2013).
Regarding the specific study locations, we observed variations in the number of identified bacterial species. The highest number of bacterial species, 24 in total, were found at BOB20N. This count decreased significantly to 13 species at BOB17N and further reduced to the lowest count of 11 species at BOB16N. A similar trend was observed in the distribution of bacterial genera. In BOB20N, the bacterial species were diverse and represented 16 different genera. In contrast, at BOB17N, the number of genera reduced to 10, and at BOB16N, it decreased further to only 8 genera (Supplementary Table 1). This pattern indicates a decreasing trend in both species and genera diversity as we move from BOB20N to BOB17N and then to BOB16N. Furthermore, the bacterial species from BOB20N could be further categorized into 3 phyla, 4 classes, 9 orders, and 10 families. BOB17N bacterial species belonged to 3 phyla, 4 classes, 5 orders, and 6 families. Lastly, BOB16N bacterial species belonged to 3 phyla, 4 classes, 4 orders, and 5 families. Out of 24 bacterial species observed in BOB20N, 16 which were exclusively present are, Arthrobacter subterraneus, Bacillus cabrielesii, B. cereus, B. tequilensis, Blastococcus saxobsidens, Brachybacterium paraconglomeratum, Brevibacterium casei, Cytobacillus oceanisediminis, Fictibacillus barbaricus, Halomonas meridiania, Metabacillus indicus, Micromonospora marina, M. tulbaghiae, Paenibacillus lautus, Robertmurraya beringensis and Rossellomorea marisflavi. The bacterial species unique to BOB17N were Alkalihalobacillus hwajinpoensis, Alteromonas macleodii, Fictibacillus aquaticus, Micromonospora fluminis, Priestia filamentosa and Staphylococcus arlettae. Moreover, the bacterial species encountered only in BOB16N were Bacillus fengqiuensis, B. safensis, Domibacillus indicus, Halobacillus trueperi, Limimaricola soesokkakensis, Lysinibacillus capsica and Salinicola salarius.
The role of the 16S rRNA gene sequence similarity threshold has been pivotal in the classification of prokaryotes, particularly in recognizing new species. Initially, the suggested threshold value was 97%, as proposed by Stackebrandt and Goebel. (1994). However, this value has faced challenges from various research groups. Kim et al. (2014), proposed a revised threshold of 98.65% based on an extensive analysis of over a million comparisons between genome sequences and complete 16S rRNA gene sequences. In light of this criterion, our study has yielded noteworthy results. Specifically, we identified five potential novel isolates with 16S rRNA gene sequence similarity values below the established threshold. Strain B1TS8, with a 98% bootstrap-supported cluster in the phylogenetic tree, was found as a potential novel species closely related to Fictibacillus aquaticus, sharing 98.4% similarity (Figure 2). Strain B2TS8H formed a divergent node in the genus Lysinibacillus clade and shared 98.2% similarity with L. capsici. Strain B2TS2H, showed 98.3‐98.4% similarity of 16S rDNA with multiple species of genus Salinicola and formed a tight cluster with S. halophyticus, S. halimionae and S. salarius. Strains of S. salarius are reported as piezophilic bacterium that requires pressures of 102 MPa to grow and bacteria belonging to this genus have been reported earlier from the deep-sea sediments. Strains B0TS14 and B0TS14H showed 98.7 and 98.6% similarity with Robertmurraya beringensis and formed a distinct group in the genus Robertmurraya clade. Strain B0TS39, showed 98.6% similarity of 16S rDNA with Blastococcus saxobsidens. It formed a moderate bootstrap-supported (64%) cluster in a phylogenetic tree reflecting similarity with multiple species. Members of this genus have been isolated from sandstone monuments, sea, soil, plant, and snow samples.
Bacterial diversity and abundance in deep-sea
The diversity indices offer a comprehensive view of the distribution of the bacterial population in the sediment samples of the BOB (Table 1C). The diversity indices indicate that BOB20N had the highest values for number of species, S; species richness, d; Shannon diversity, H′; and Simpson’ estimate, 1- Lambda’ except for evenness, J’. However, it was noted that J’ had the highest value in BOB16N. The obtained values suggest that BOB20N harbours a rich and diverse bacterial community, with a high probability of encountering distinct genotypes within the population. The distribution of bacterial species further indicates a balanced ecological niche, potentially contributing to the overall stability and resilience of the ecosystem in BOB20N. Considering all the samples together, the results suggest that ZMA was the most favourable culture medium for obtaining different bacteria species, as it showed the highest values for S, d, J’, H’, and 1-Lambda’. On the other hand, SMA was found to be the most suitable medium for promoting bacterial growth, as it recorded the peak density among all the media used. Regarding the optimal growth medium for specific study locations, it was observed that ZMA displayed the highest values for S, d, J’, H’ and 1- Lambda’. Conversely, in the case of BOB20N, SMA medium exhibited the highest population density among all the tested growth media (Supplementary Figure 1). In BOB17N, the SMA medium exhibited highest values for density, S, d, H’ and 1-Lambda’, however, the highest value for J’ was observed with the ISP7 medium. Conversely, in BOB16N, the ZMA medium displayed the highest values for density, S, d, H’ and 1-Lambda’ and maximum in ISP7 and SMA for J’.
It is noteworthy that the SCA medium recorded the lowest values across majority of the diversity indices, indicating that it was the least favourable medium for bacterial diversity and abundance in the BOB deep-sea sediment samples examined in this study. These findings indicate that among the culture media tested, ZMA exhibited the most favourable conditions for bacterial growth, as it yielded the highest counts of species, evenness, richness, and genotypic diversity. Meanwhile, SMA demonstrated the highest abundance. The combination of ZMA’s high species diversity and SMA’s peak abundance suggests that different media can provide complementary insights into the microbial community composition and dynamics in BOB20N.
The two-way ANOVA confirmed significant spatial variation between the study locations and the media for the diversity indices (p<0.05) (Supplementary Table 2). The simple main effect of “media” on the station’s diversity indices was statistically significant for each station (p< 0.0001), except for J’ in BOB20N and 1-Lambda’ in BOB17N. Moreover, there is a statistically significant difference in density at BOB16N bacteria cultured in ISP7, ZMA, SMA or SCA, F(3, 24) = 4.09, p = 0.018. The same holds true for BOB17N, F(3, 24) = 39.1, p< 0.0001 and BOB20N, F(3, 24) = 74.4, p< 0.0001. The pairwise comparisons for species richness, evenness, Shannon index, Simpson index and density showed a combination of significant as well as insignificant values for every media in the different sampling stations (Supplementary Figure 1). The obtained results of two-way ANOVA and pairwise comparisons confirmed that the choice of media significantly affects the diversity indices of bacteria at each station. These findings suggest that the bacterial communities in the BOB sediments have distinct preferences for different media, which may be indicative of specific ecological niches or resource availability.
Ecto-enzymatic activities of deep-sea sedimentary bacteria and nutrient cycling
The microbial communities of deep-sea sediment meet their substrate needs by breaking down organic components deposited at the bottom of the sea (Mahmoudi et al., 2020). The primary step in the breakdown of OM is the production of extracellular enzymes that make high molecular weight compounds easily accessible for bacterial consumption (Polymenakou et al., 2008). The polymer mineralization and regeneration of deep-sea nutrients are indicated by the production of these enzymes from marine bacteria (Raghukumar et al., 2001). Thus, the production of extracellular hydrolytic enzymes by deep-sea sedimentary bacteria plays a significant role in the biogeochemical processes through decomposition of OM (Dang et al., 2009). In our study, we investigated the enzymatic activities of 55 bacterial strains. Among these strains, 28 exhibited positive activity for caseinase, indicating their capacity to hydrolyze protein polymer. Additionally, 31 strains displayed positive gelatinase activity, suggesting their ability to break down gelatin, a protein derived from collagen (Supplementary Table 1). These findings have important implications for deep-sea environments, as they highlight the potential role of these bacteria in protein degradation, leading to an increase in free amino acid availability, as previously discussed in the works of Dang et al. (2009) and Saunders et al. (2022).
In the case of amylolytic activity, 28 bacterial isolates were positive indicating their role in carbohydrate polymer degradation (Supplementary Table 1). Complex polysaccharide degradation is particularly pertinent to the study of microbial metabolism since they are the major elements of phytoplankton, marine particles, and sediments (Raghukumar et al., 2001; Arnosti et al., 2005). Notably, sedimentary bacteria of genus Bacillus and Micromonospora exhibited all three enzyme activities, indicating their versatility in enzymatic degradation and crucial roles in nutrient cycling in the deep-sea environment. Limimaricola, Brevibacterium, Robertmurraya, Blastococcus, and some cultures of Metabacillus were negative for all enzyme activities (Supplementary Table 1).
Assemblage pattern of bacterial community and relationship with environmental factors
The 2D nMDS (stress 0.01) ordination plot showed the assemblage pattern of bacteria from three deep-sea stations cultured using different growth media (Figure 3). Specifically, for BOB20N, it was observed that the bacterial species grown in SMA, ZMA and ISP7 formed a cluster in the plot, indicating their similarity in terms of community composition. However, SCA appeared as a separate point on the plot, suggesting that it had a distinct bacterial community composition compared to the other three media. Similarly, for BOB17N, SMA, ZMA and SCA were clustered closely together, whereas, ISP7 appeared as a separate point in the plot. Lastly, in the case of BOB16N, ZMA, SMA and SCA were grouped and ISP7 was positioned far away in the plot indicating that the latter had a distinct bacterial community composition compared to the other media and study locations.
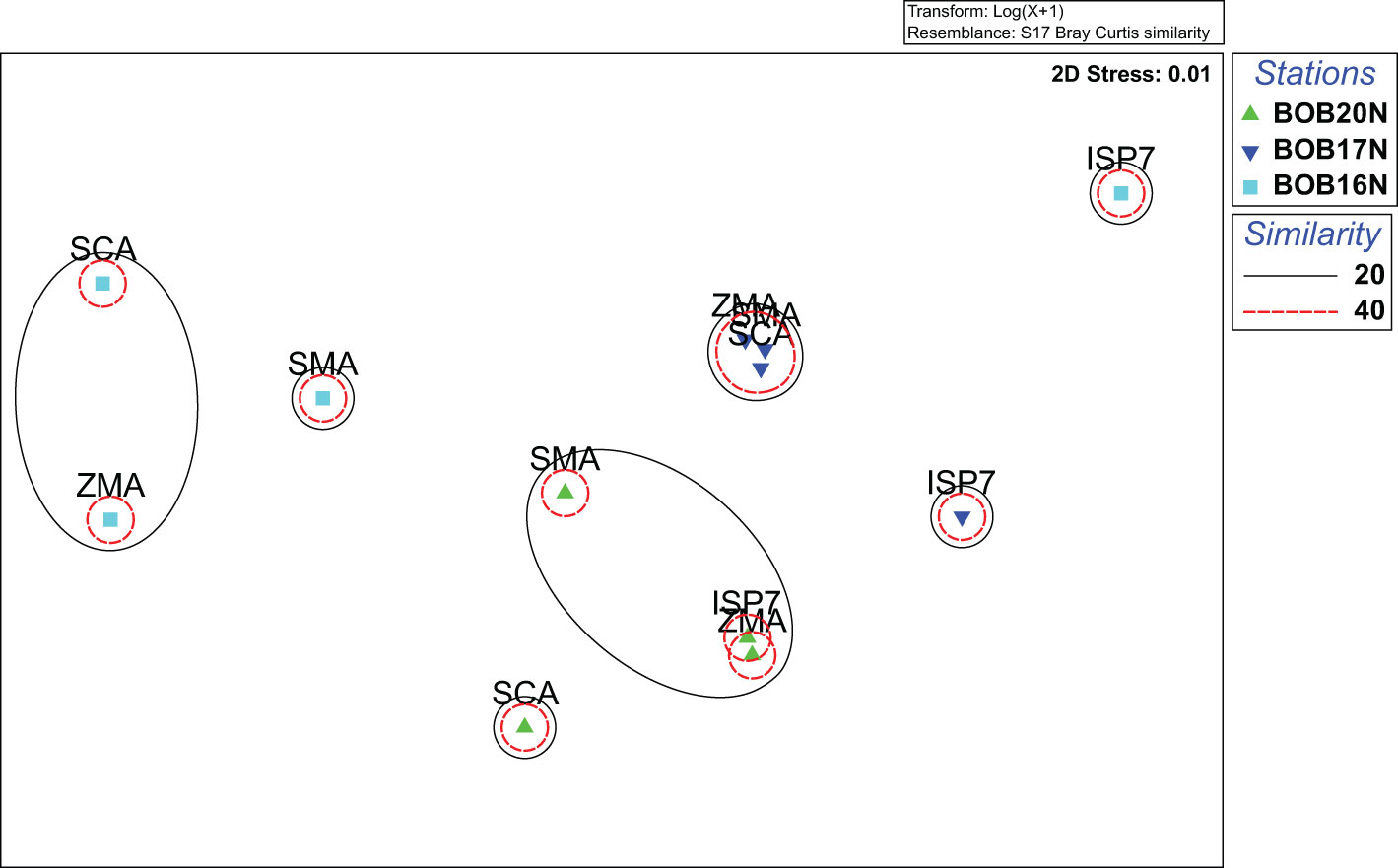
Figure 3 nMDS plot highlighting the distinct clustering patterns of bacterial strains isolated on different growth media from deep sea samples.
The nMDS analysis visually highlighted the distinct clustering patterns among bacterial species cultured on different growth media. These distinct clustering patterns indicate that the composition and abundance of bacterial species can vary significantly depending on the growth media used. Based on the obtained results, ZMA, SMA and SCA promote more similar communities, while ISP7 support the growth of distinct bacterial communities.
The CCA ordination plotted for bacterial families against the environmental variables and heavy metals revealed the influence of variables on bacterial families. The CCA plot suggests that both the environmental variables and heavy metals have a parallel influence on the spatial variability of the bacterial community in the study locations. (Figure 4). A clear demarcation was observed on axis-2 between the bacterial families related to BOB20N and much deeper stations BOB16N & BOB17N. Bacterial families related to BOB20N i.e., Halomonadaceae, Paenibacillaceae, Dermabacteraceae, Geodermatophilaceae, Pseudomonadaceae and Bacillaceae were primarily influenced by the sandy texture of the sediment, OM, temperature, and salinity. In addition, Zn and Cu were two metals that were also important in structuring the bacterial community in the sediment samples of BOB20N. Sediment with distinct textures exhibit differences in their surface area available for bacterial attachment and growth including its ability to retain oxygen, leading to changes in the composition of the bacterial community (Wang et al., 2013; Zheng et al., 2014). Comamonadaceae, Micrococcaceae and Micromonosporaceae were positively correlated to silt, Cr and Al. Rhodobacteraceae, Brevibacteraceae and Planococcaceae were dominant in BOB16N and related to depth, clay, Co, Fe, Ni and Pb whereas in BOB17N, Alteromonadaceae and Staphylococcaceae were dominant and showed correlation mainly with silty sediment, Cu and Mn on the first axis on CCA plot. The higher diversity in BOB20N may be attributed to the availability of high OM content that supports the growth of bacteria. The prevalence of both positive and negative correlations between the bacterial community, physical and chemical parameters indicate a complex and dynamic relationship between microbial communities and the ambient environment. Positive correlations suggest the existence of bacterial species that thrive in metal-rich conditions, possibly due to their specialized metal resistance or metabolism (Yin et al., 2015). These bacteria might play essential roles in metal cycling, accumulation, or transformation within the ecosystem. Conversely, negative correlations could signify the sensitivity or inhibition of certain bacterial taxa in the presence of high metal concentrations, potentially reflecting their vulnerability to metal toxicity.
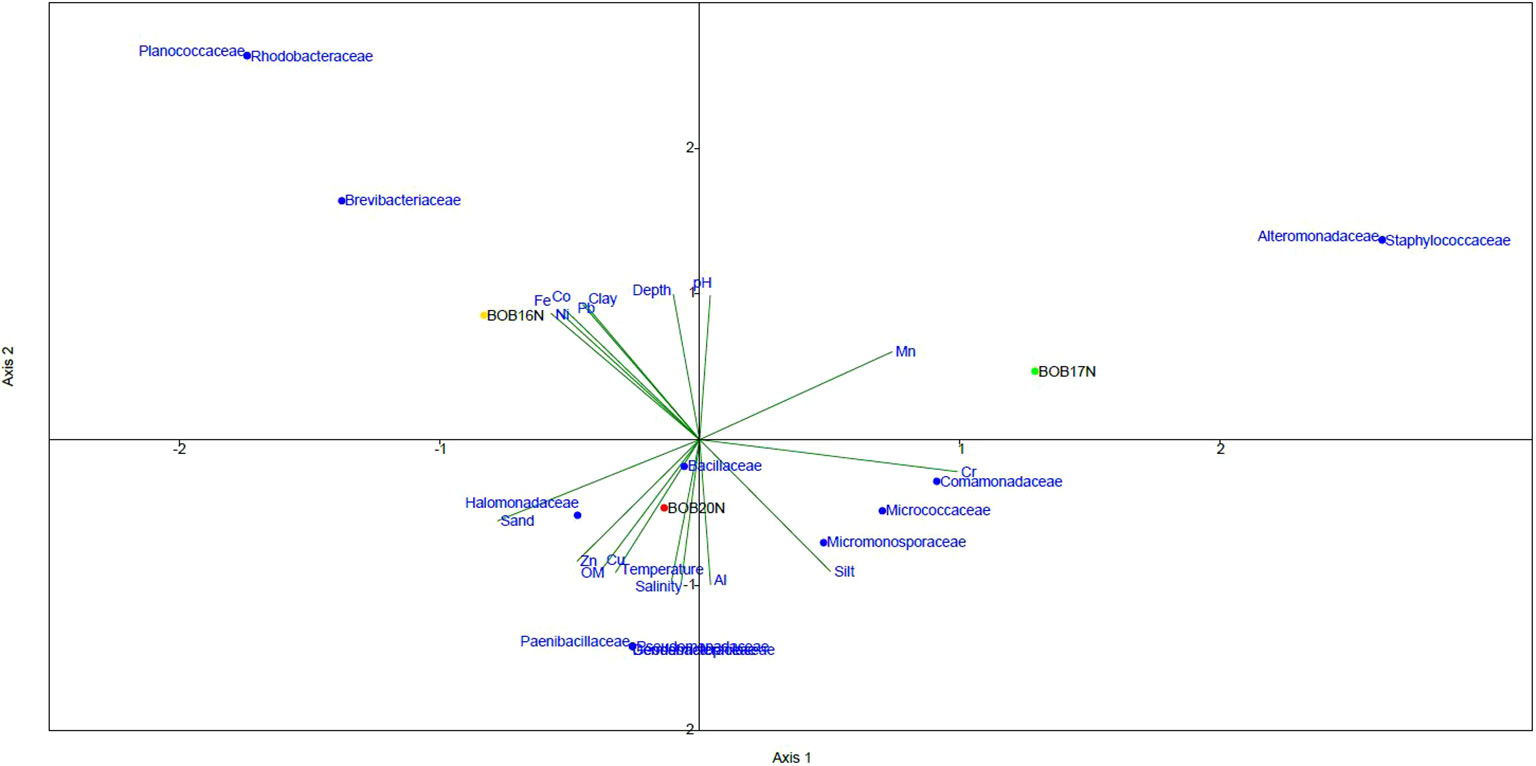
Figure 4 The CCA relationship between bacterial families, deep-sea sediment characteristics and environmental variables.
Carbon metabolic characteristics of bacterial communities
Biolog plates have served as valuable tools for evaluating the functional diversity of microorganisms in various environments ever since their initial proposal by Garland and Mills (1991). Biolog Ecoplates, which are particularly well-suited for ecological investigations, were subsequently introduced by Insam (1997). These Ecoplates are designed with a selection of carbon sources that are highly pertinent to environmental contexts. The fundamental principle behind Biolog plates is to undertake miniaturized enrichment experiments wherein bacterial growth on a single carbon source is concurrently assessed across 31 different carbon sources, and the analysis is carried out in triplicate. While it’s important to acknowledge that the methodology of Biolog Ecoplates does have its limitations, which have been thoughtfully examined in the work of Preston-Mafham et al. (2002). It is worth noting that these Ecoplates have been effectively employed for assessing the utilization of carbon sources in various environmental settings, such as freshwater (Christian and Lind, 2006; Comte and Del Giorgio, 2009), brackish water (Behera et al., 2018) and marine environments (Sinha et al., 2019; Wang et al., 2022).
The EcoPlateTM analysis of carbon metabolism in the deep-sea communities of the BOB unveiled significant metabolic diversity. Over a 6-day incubation period, the bacterial community demonstrated the utilization of 28 out of the 31 different carbon sources tested in at least one of the sediment samples. Notably, 10 carbon substrates (β-methyl-D-glucoside, α-D-lactose, α-cyclodextrin, N-acetyl-D-glucosamine, tween 80, glucose-1-phosphate, D-xylose, pyruvic acid methyl ester, glycogen, and 2-hydroxy benzoic acid) were consistently utilized across all sediment samples, with an average well color development (AWCD) of ≥ 0.2 OD at 590nm for each substrate.
The increase in net AWCD (Absorbance at 590nm) over time provides insight into the metabolic activity of heterotrophic bacterial communities in different samples. Within 24 hours, BOB20N’s bacterial community achieved an AWCD of 0.35 OD, indicating a high rate of carbon substrate utilization. In contrast, BOB17N reached the 0.35 OD threshold after 72 hours, and the bacterial communities in the BOB16N sample couldn’t reach this threshold even after 6 days of incubation (Figure 5A). This suggests that the deeper samples in BOB16N exhibited lower and more selective utilization of substrates. The functional diversity as reflected by mean H′ was 2.9 (range 2.7–3.1) indicating a high metabolic diversity in the heterotrophic bacterial communities. R ranged from 20 to 28 (average 24) for all samples, which indicates that the deep-sea sediment communities utilized a substantial number of carbon substrates. The richness of substrate utilization was 1.4 and 1.17-fold higher in BOB20N as compared to BOB16N and BOB17N samples, respectively (Figure 5B).
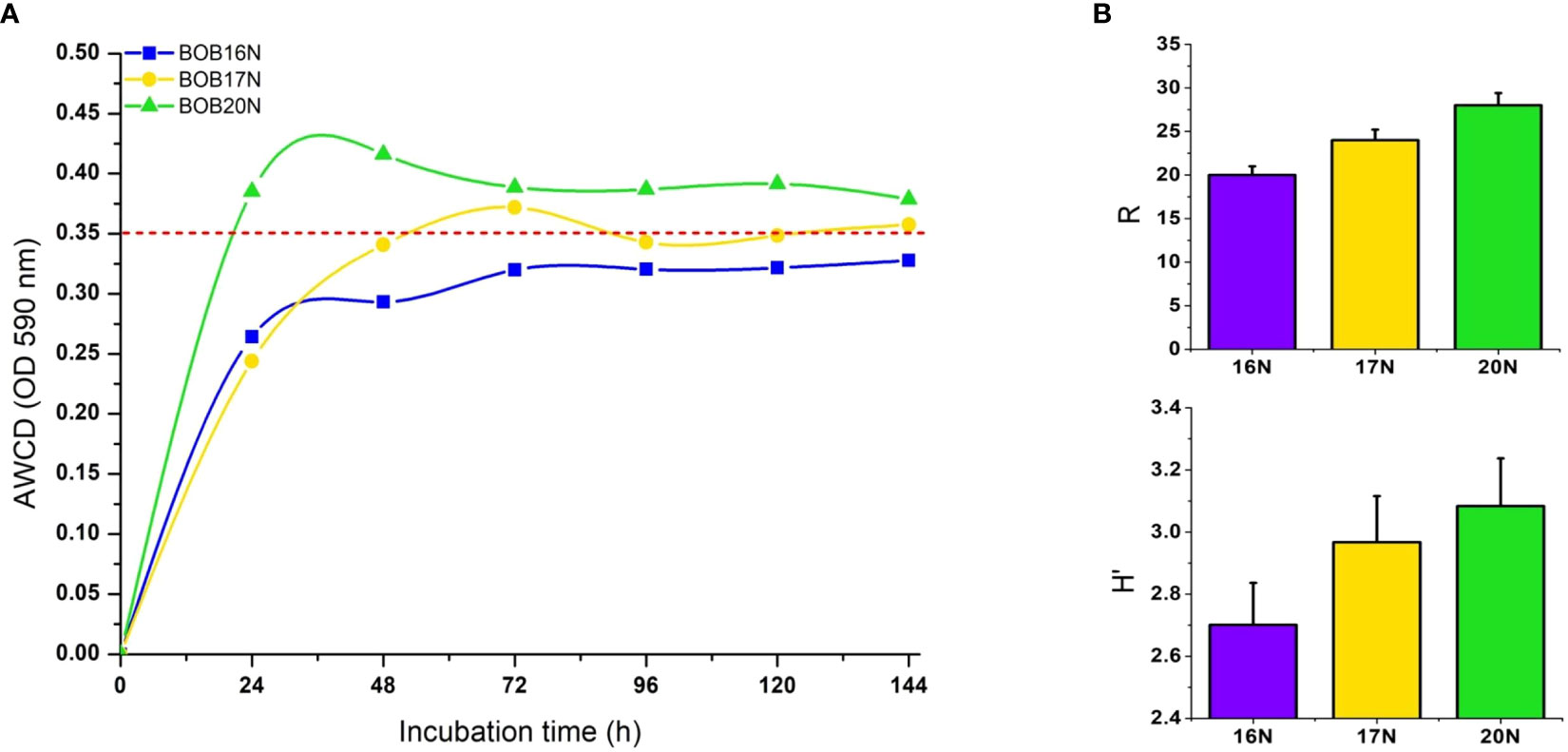
Figure 5 (A) AWCD by heterotrophic deep-sea bacterial communities in Biolog EcoPlates, red dashed line indicates the threshold value (OD590nm ≥ 0.35). (B) Richness (R), and Shannon diversity index (H′) of deep-sea sediment bacterial communities carbon substrates utilization. Errors bars indicate the standard error.
The higher carbon substrate utilization rate along with higher metabolic diversity and carbon substrate richness in the Northern Bay of Bengal sediment sample indicated these bacterial communities were more active and better adapted to quickly metabolize diverse carbon substrates than the BOB16N and BOB17N sediments. The higher rate of carbon substrate utilization in BOB20N sediment could also be attributed to higher bacterial abundances than the BOB16N and BOB17N sediments.
According to their biochemical properties and molecular composition, the 31 carbon sources in the EcoPlate were divided into six categories, including amines (AM), amino acids (AA), carbohydrates (CH), carboxylic acids (CA), phenolic compounds (PC), and polymers (PO). The average substrate utilization of CH, and CA groups were closely related in all the deep-sea sediment samples studied, while substrates of PO group showed fairly increasing trend of utilization from BOB16N< BOB17N< BOB20N. The mean utilization of carbon substrates groups, amine (AM), amino acid (AA) and phenolic compounds (PC) was higher (1.3 to 1.4-fold) in the BOB20N sediment compared to the deeper depth southern samples, indicating that shallow heterotrophic microbial communities have a preference for these specific carbon substrate groups (Figure 6A). When we analyzed the average colour development of all the sediment samples collectively, we found that the wells containing the substrates, α-cyclodextrin (polymer), glucose-1-phosphate (carbohydrate), D-xylose (carbohydrate), glycogen (polymer), D-galacturonic acid (carboxylic acid), and 2-hydroxy benzoic acid (phenols) had the highest AWCD (> 0.3 OD) at 590nm among the 31 carbon sources tested which presents preferential utilisation of these carbon substrates by the deep-sea bacterial communities.
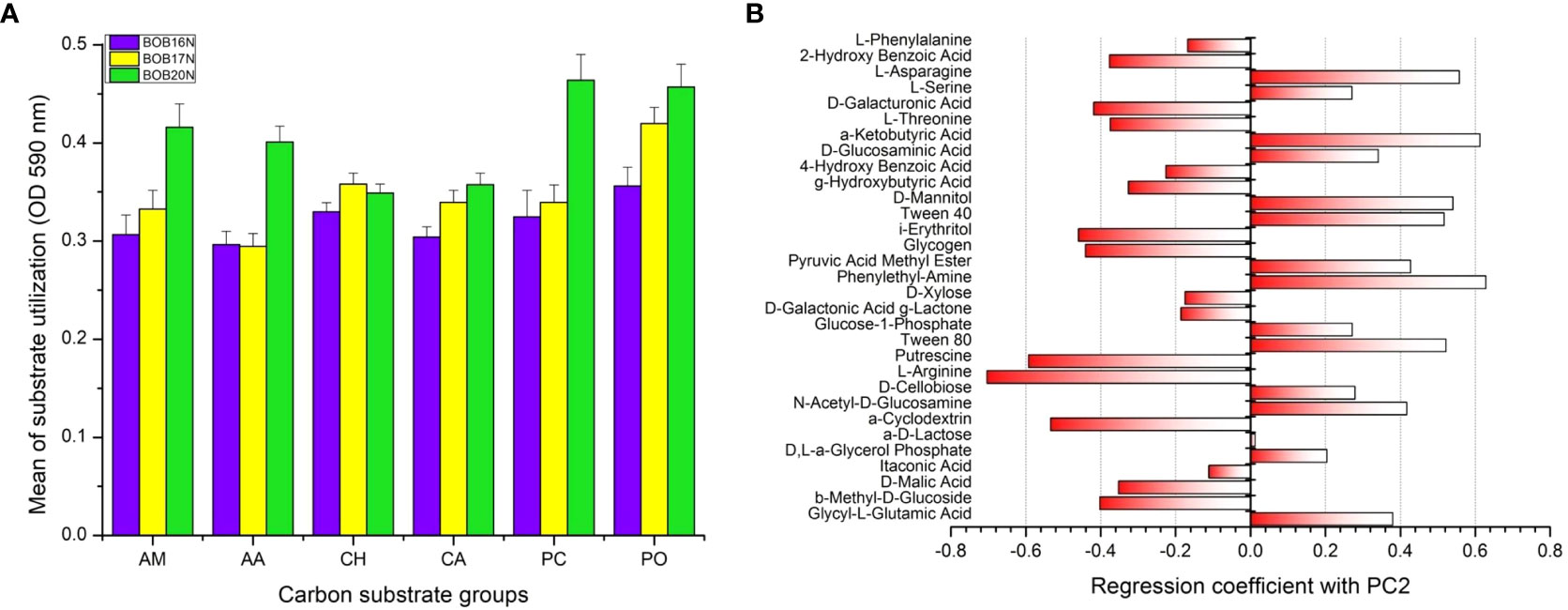
Figure 6 (A) Carbon substrate utilization of different substrate groups by the deep-sea bacterial communities. Errors bars indicate the standard error. AM, amines; AA, amino acids; CH, carbohydrates; CA, carboxylic acids; PC, phenolic compounds, PO, polymers. (B) PCA loading plot showing positive and negative correlation of carbon substrates with PC2.
PCA was applied to investigate relationships among different deep-sea sediment samples based on their carbon substrate utilization profiles. PCA revealed that the two principal components (PC1 and PC2) together accounted for 58.02% of the variation in the carbon metabolic profiles of the deep-sea bacterial communities. To determine the most significant carbon sources that were driving the differences in metabolic profiles, loading analysis was carried out by analyzing the correlations between original variables (31 carbon sources) to the PC axes values (Garland and Mills, 1991) (Figure 6B, Supplementary Table 3). The results of the correlation matrix showed that the KMO measure of sampling adequacy was 0.72 and Bartlett’s test of sphericity was 2317.06 (P< 0.0001), presenting an adequate sample size for the factor analysis. The carbon sources which were significantly correlated with PC2 are shown in the PCA Figure 6B. Carbon sources namely, Tween 80, Phenylethyl-amine, Tween 40, D-mannitol, α-ketobutyric acid, and L-asparagine showed significant positive correlation. Whereas, α-cyclodextrin, L-arginine and Putrescine were negatively correlated with PC2 axis suggesting that separation of samples in PCA plot can be attributed to the differences in the utilization of these important carbon sources by the deep-sea bacterial communities.
In summary, our study revealed notable differences in microbial metabolism both within individual samples and among different samples, underscoring the existence of microbial metabolic diversity. Specifically, across all deep-sea sediment samples, the prominent carbon substrates that exhibited consistent utilization included α-cyclodextrin, glucose-1-phosphate, D-xylose, glycogen, and 2-hydroxy benzoic acid. In this study, we found that the deep-sea bacterial community utilized 28 carbon substrates, displaying significant functional diversity that is essential to effectively decompose the heterogeneous organic carbon pool that accumulates in benthic sediments. Since, the heterotrophic bacteria breakdown complex OM; they can regulate the availability of carbon in benthic sediments (Säwström et al., 2016). Consequently, the higher OM content observed in BOB20N sediments, in comparison to BOB16N and BOB17N, can be linked to the elevated metabolic rates and greater diversity of heterotrophic bacterial communities.
Conclusion
The knowledge of the deep-sea microbiota holds the key to understanding the intricate complexities and ecological interactions as they can have significant impacts on ecosystem functioning and resilience. However, the limited literature on the deep-sea microbial biota emphasizes the expansive need for exploration of their unknown diversity, their ecological roles and untapped potential. The present study revealed a strong correlation between the physicochemical parameters and the bacterial community in the deep sea indicating that the ambient environment exerts a significant influence over the benthic bacterial community structure and composition. The bacterial groups encountered in this study were Bacilli, Actinomycetia, Alphaproteobacteria, Betaproteobacteria and Gammaproteobacteria. Distinct spatial variations observed in the bacterial diversity and retrievable heterotrophic counts may be indicative of environmental gradients, where bacterial composition shifts in response to varying metal levels, and these changes might be influenced by ecological factors, including, OM, sediment texture or competing microbial species. The carbon substrates primarily utilized across all the deep-sea sediment samples were α-cyclodextrin (polymer), glucose-1-phosphate (carbohydrate), D-xylose (carbohydrate), glycogen (polymer), and 2-hydroxy benzoic acid (phenols) presenting widespread presence of related pathways. The utilisation of >90% of the carbon substrates tested by the deep-sea microbenthos in at least one of the sediment samples indicates highly efficient metabolic activity which is essential to maintain the overall functioning and productivity of the ecosystem. Besides, the production of extracellular enzymes is also indicative of the role of deep-sea bacteria in the breakdown of complex OM and nutrient regeneration that is crucial for metabolic activity. The findings of this study provide insights into the adaptations and interactions of bacteria in extreme conditions, contributing to our understanding of complex web of life in deep-sea ecosystems.
Data availability statement
The datasets presented in this study can be found in online repositories. The names of the repository/repositories and accession number(s) can be found in the article/Supplementary Material.
Author contributions
PV: Conceptualization, Formal analysis, Investigation, Methodology, Writing – original draft, Writing – review & editing. VP: Formal analysis, Investigation, Software, Writing – review & editing. SS: Formal analysis, Investigation, Writing – original draft, Writing – review & editing. AA: Investigation, Methodology, Writing – original draft. GD: Project administration, Resources, Writing – review & editing.
Funding
The author(s) declare financial support was received for the research, authorship, and/or publication of this article. The authors thank the Ministry of Earth Sciences, Govt. of India, Deep Ocean Mission (DOM)- Technological innovations for exploration and conservation of deep-sea biodiversity program for the funding support.
Acknowledgments
The authors are grateful to the Ministry of Earth Sciences, Govt. of India, for providing support to carry out the present study. We are thankful to the Director, NIOT, Chennai, for his constant encouragement, support, and guidance. We are thankful to the former group head, Dr. R. Kirubagaran, for his constant support and guidance. The NIOT Vessel Management Cell and crew members of the ORV Sagar Nidhi (SN142) are acknowledged for their support. We thank the scientific and supporting staff of NIOT, Chennai, India, for their support in the field and in the laboratory during this study.
Conflict of interest
The authors declare that the research was conducted in the absence of any commercial or financial relationships that could be construed as a potential conflict of interest.
Publisher’s note
All claims expressed in this article are solely those of the authors and do not necessarily represent those of their affiliated organizations, or those of the publisher, the editors and the reviewers. Any product that may be evaluated in this article, or claim that may be made by its manufacturer, is not guaranteed or endorsed by the publisher.
Supplementary material
The Supplementary Material for this article can be found online at: https://www.frontiersin.org/articles/10.3389/fmars.2023.1308953/full#supplementary-material
References
Acinas S. G., Sánchez P., Salazar G., Cornejo-Castillo F. M., Sebastián M., Logares R., et al. (2021). Deep ocean metagenomes provide insight into the metabolic architecture of bathypelagic microbial communities. Commun. Biol. 4 (1), 604. doi: 10.1038/s42003-021-02112-2
Altschul S. F., Madden T. L., Schäffer A. A., Zhang J., Zhang Z., Miller W., et al. (1997). Gapped BLAST and PSI-BLAST: a new generation of protein database search programs. Nucleic Acids Res. 25 (17), 3389–3402. doi: 10.1093/nar/25.17.3389
Angelova A. G., Ellis G. A., Wijesekera H. W., Vora G. J. (2019). Microbial composition and variability of natural marine planktonic and biofouling communities from the Bay of Bengal. Front. Microbiol. 10, 2738. doi: 10.3389/fmicb.2019.02738
APHA (2005). Standard methods of water and wastewater. 21st Edn (Washington, DC.: American Public Health Association), 2–61, ISBN: ISBN: 0875530478.
Arnosti C., Durkin S., Jeffrey W. H. (2005). Patterns of extracellular enzyme activities among pelagic marine microbial communities: implications for cycling of dissolved organic carbon. Aquat. Microb. Ecol. 38, 135–145. doi: 10.3354/ame038135
Balan S. S., Nethaji R., Sankar S., Jayalakshmi S. (2012). Production of gelatinase enzyme from Bacillus spp isolated from the sediment sample of Porto Novo Coastal sites. Asian Pacific J. Trop. Biomedicine 2 (3), S1811–S1816. doi: 10.1016/S2221-1691(12)60500-0
Behera P., Mohapatra M., Adhya T. K., Suar M., Pattnaik A. K., Rastogi G. (2018). Structural and metabolic diversity of rhizosphere microbial communities of Phragmites karka in a tropical coastal lagoon. Appl. Soil Ecol. 125, 202–212. doi: 10.1016/j.apsoil.2017.12.023
Bjerga G. E. K., Hjerde E., De Santi C., Williamson A. K., Smalås A. O., Willassen N. P., et al. (2014). High-quality draft genome sequence of Streptomyces sp. strain AW19M42 isolated sea squirt Northern Norway. Standards Genomic Sci. 9 (3), 676–686. doi: 10.4056/sigs.5038901
Buchanan J. B. (1984). “Sediment analysis,” in Methods for the study of marine benthos (UK: Wiley Blackwell), 41–65.
Budianto F., Lestari (2018). Trace metal in sediment from a deep-sea floor of Makassar Strait. IOP Conf. Series: Earth Environ. Sci. 118, 012057. doi: 10.1088/1755-1315/118/1/012057
Chen X., Cai R., Zhuo X., Chen Q., He C., Sun J., et al. (2023). Niche differentiation of microbial community shapes vertical distribution of recalcitrant dissolved organic matter in deep-sea sediments. Environ. Int. 178, 108080. doi: 10.1016/j.envint.2023.108080
Chen P., Zhang L., Guo X., Dai X., Liu L., Xi L., et al. (2016). Diversity, biogeography, and biodegradation potential of actinobacteria in the deep-sea sediments along the southwest Indian ridge. Front. Microbiol. 7, 1340. doi: 10.3389/fmicb.2016.01340
Cheng N., Shi M., Hou Q., Wang J., Pan J. (2022). The impact of tectonic stress chemistry on mineralization processes: A review. Solid Earth Sci. 7 (2), 151–166. doi: 10.1016/j.sesci.2021.11.002
Christian B. W., Lind O. T. (2006). Key issues concerning Biolog use for aerobic and anaerobic freshwater bacterial community-level physiological profiling. Int. Rev. hydrobiology 91 (3), 257–268. doi: 10.1002/iroh.200510838
Clarke K. R., Warwick R. M. (1994). Similarity-based testing for community pattern: the two-way layout with no replication. Mar. Biol. 118, 167–176. doi: 10.1007/BF00699231
Comte J., Del Giorgio P. A. (2009). Links between resources, C metabolism and the major components of bacterioplankton community structure across a range of freshwater ecosystems. Environ. Microbiol. 11 (7), 1704–1716. doi: 10.1111/j.1462-2920.2009.01897.x
Contreras-Rosales L. A., Schefuß E., Meyer V., Palamenghi L., Lückge A., Jennerjahn T. C. (2016). Origin and fate of sedimentary organic matter in the northern Bay of Bengal during the last 18 ka. Global Planetary Change 146, 53–66. doi: 10.1016/j.gloplacha.2016.09.008
Corinaldesi C. (2015). New perspectives in benthic deep-sea microbial ecology. Front. Mar. Sci. 2, 17. doi: 10.3389/fmars.2015.00017
Cortada U., Collins M. (2013). The nature and contamination of sediments in the Plentzia estuary (Biscay province, Spain). Geogaceta 54, 147–150.
Dang H., Zhu H., Wang J., Li T. (2009). Extracellular hydrolytic enzyme screening of culturable heterotrophic bacteria from deep-sea sediments of the Southern Okinawa Trough. World J. Microbiol. Biotechnol. 25, 71–79. doi: 10.1007/s11274-008-9865-5
Danovaro R. (2018). Climate change impacts on the biota and on vulnerable habitats of the deep Mediterranean Sea. Rendiconti Lincei. Sci. Fisiche e Naturali 29 (3), 525–541. doi: 10.1007/s12210-018-0725-4
Danovaro R., Molari M., Corinaldesi C., Dell’Anno A. (2016). Macroecological drivers of archaea and bacteria in benthic deep-sea ecosystems. Sci. Adv. 2 (4), e1500961. doi: 10.1126/sciadv.1500961
da Silva M. A. C., Cavalett A., Spinner A., Rosa D. C., Jasper R. B., Quecine M. C., et al. (2013). Phylogenetic identification of marine bacteria isolated from deep-sea sediments of the eastern South Atlantic Ocean. SpringerPlus 2 (1), 1–10. doi: 10.1186/2193-1801-2-127
Dong X., Greening C., Rattray J. E., Chakraborty A., ChuvoChina M., Mayumi D., et al. (2019). Metabolic potential of uncultured bacteria and archaea associated with petroleum seepage in deep-sea sediments. Nat. Commun. 10 (1), 1816. doi: 10.1038/s41467-019-09747-0
Falkowski P. G., Fenchel T., Delong E. F. (2008). The microbial engines that drive Earth's biogeochemical cycles. science 320 (5879), 1034–1039. doi: 10.1126/science.1153213
Garel M., Panagiotopoulos C., Boutrif M., Repeta D., Sempere R., Santinelli C., et al. (2021). Contrasting degradation rates of natural dissolved organic carbon by deep-sea prokaryotes under stratified water masses and deep-water convection conditions in the NW Mediterranean Sea. Mar. Chem. 231, 103932. doi: 10.1016/j.marchem.2021.103932
Garland J. L. (1997). Analysis and interpretation of community-level physiological profiles in microbial ecology. FEMS Microbiol. Ecol. 24, 289–300. doi: 10.1111/j.1574-6941.1997.tb00446.x
Garland J. L., Mills A. L. (1991). Classification and characterization of heterotrophic microbial communities on the basis of patterns of community-level sole-carbon-source utilization. Appl. Environ. Microbiol. 57, 2351–2359. doi: 10.1128/aem.57.8.2351-2359.1991
Gärtner A., Wiese J., Imhoff J. F. (2016). Diversity of Micromonospora strains from the deep Mediterranean Sea and their potential to produce bioactive compounds[J]. AIMS Microbiol. 2 (2), 205–221. doi: 10.3934/microbiol.2016.2.205
Gaudette H. E., Flight W. R., Toner L., Folger D. W. (1974). An inexpensive titration method for the determination of organic carbon in recent sediments. J. Sedimentary Res. 44 (1), 249–253. doi: 10.1306/74D729D7-2B21-11D7-8648000102C1865D
Gawas V. S., Shivaramu M. S., Damare S. R., Pujitha D., Meena R. M., Shenoy B. D. (2019). Diversity and extracellular enzyme activities of heterotrophic bacteria from sediments of the Central Indian Ocean Basin. Sci. Rep. 9 (1), 9403.
Gu B., Liu J., Cheung S., Ho N. H. E., Tan Y., Xia X. (2022). Insights into prokaryotic community and its potential functions in nitrogen metabolism in the Bay of Bengal, a pronounced Oxygen Minimum Zone. Microbiol. Spectr. 10 (3), e00892–e00821. doi: 10.1128/spectrum.00892-21
Gunaratna T., Suzuki T., Yanagishima S. (2019). Cross-shore grain size and sorting patterns for the bed profile variation at a dissipative beach: Hasaki Coast, Japan. Mar. Geology 407, 111–120. doi: 10.1016/j.margeo.2018.10.008
Guo R., Ma X., Zhang J., Liu C., Thu C. A., Win T. N., et al. (2022). Microbial community structures and important taxa across oxygen gradients in the Andaman Sea and eastern Bay of Bengal epipelagic waters. Front. Microbiol. 13, 1041521. doi: 10.3389/fmicb.2022.1041521
Hongxiang X., Min W., Xiaogu W., Junyi Y., Chunsheng W. (2008). Bacterial diversity in deep-sea sediment from northeastern Pacific Ocean. Acta Ecologica Sin. 28 (2), 479–485. doi: 10.1016/S1872-2032(08)60026-8
Insam H. (1997). Microbial communities: functional versus structural approaches. Eds. Insam H., Rangger A. (Germany: Springer Science and Business Media), 259–260.
Jasna V., Nathan V. K., Parvathi A. (2020). Comparison of bacterial community structure in coastal and offshore waters of the Bay of Bengal, India. Regional Stud. Mar. Sci. 39, 101414. doi: 10.1016/j.rsma.2020.101414
Jha D. K., Dharani G., Verma P., Ratnam K., Kumar R. S., Rajaguru S. (2021). Evaluation of factors influencing the trace metals in Puducherry and Diu coasts of India through multivariate techniques. Mar. pollut. Bull. 167, 112342. doi: 10.1016/j.marpolbul.2021.112342
Jha D. K., Ratnam K., Rajaguru S., Dharani G., Devi M. P., Kirubagaran R. (2019). Evaluation of trace metals in seawater, sediments, and bivalves of Nellore, southeast coast of India, by using multivariate and ecological tool. Mar. pollut. Bull. 146, 1–10. doi: 10.1016/j.marpolbul.2019.05.044
Jiang Q., Jing H., Liu H., Du M. (2022). Biogeographic distributions of microbial communities associated with anaerobic methane oxidation in the surface sediments of deep-sea cold seeps in the South China Sea. Front. Microbiol. 13, 1060206. doi: 10.3389/fmicb.2022.1060206
Jones C. J., Murray J. W. (1984). Nickel, cadmium, and copper in the northeast Pacific off the coast of Washington 1, 2. Limnology Oceanography 29 (4), 711–720. doi: 10.4319/lo.1984.29.4.0711
Jørgensen B. B., Boetius A. (2007). Feast and famine—microbial life in the deep-sea bed. Nat. Rev. Microbiol. 5 (10), 770–781. doi: 10.1038/nrmicro1745
Kato Y., Fujinaga K., Nakamura K., Takaya Y., Kitamura K., Ohta J., et al. (2011). Deep-sea mud in the Pacific Ocean as a potential resource for rare-earth elements. Nat. Geosci. 4 (8), 535–539. doi: 10.1038/ngeo1185
Kim M., Oh H. S., Park S. C., Chun J. (2014). Towards a taxonomic coherence between average nucleotide identity and 16S rRNA gene sequence similarity for species demarcation of prokaryotes. Int. J. Systematic Evolutionary Microbiol. 64 (Pt_2), 346–351. doi: 10.1099/ijs.0.059774-0
Kumar S., Stecher G., Li M., Knyaz C., Tamura K. (2018). MEGA X: molecular evolutionary genetics analysis across computing platforms. Mol. Biol. Evol. 35 (6), 1547. doi: 10.1093/molbev/msy096
Lauro F. M., Bartlett D. H. (2008). Prokaryotic lifestyles in deep sea habitats. Extremophiles 12, 15–25. doi: 10.1007/s00792-006-0059-5
Lincy J., Manohar C. S. (2020). A comparison of bacterial communities from OMZ sediments in the Arabian Sea and the Bay of Bengal reveals major differences in nitrogen turnover and carbon recycling potential. Mar. Biol. Res. 16 (8-9), 656–673. doi: 10.1080/17451000.2020.1840593
Liu S., Ye W., Zhang H., Cao P., Li J., Sun X., et al. (2023). Sediment provenances shift driven by sea level and Indian monsoon in the southern Bay of Bengal since the last glacial maximum. Front. Mar. Sci. 10, 1106663. doi: 10.3389/fmars.2023.1106663
Ma Y., Ding W., Wang Y., Chen P., Zhou H., Li X., et al. (2022). Environmental factors shape the culturable bacterial diversity in deep-sea water and surface sediments from the Mariana Trench. bioRxiv. doi: 10.1101/2022.01.13.476188
Mahmoudi N., Hagen S. M., Hazen T. C., Steen A. D. (2020). Patterns in extracellular enzyme activity and microbial diversity in deep-sea Mediterranean sediments. Deep Sea Res. Part I: Oceanographic Res. Papers 158, 103231.
Marimuthu J., Rangamaran V. R., Subramanian S. H. S., Balachandran K. R. S., Kulasekaran N. T., Vasudevan D., et al. (2022). Deep-sea sediment metagenome from Bay of Bengal reveals distinct microbial diversity and functional significance. Genomics 114 (6), 110524. doi: 10.1016/j.ygeno.2022.110524
McCollom T. M. (2000). Geochemical constraints on primary productivity in submarine hydrothermal vent plumes. Deep Sea Res. Part I: Oceanographic Res. Papers 47 (1), 85–101. doi: 10.1016/S0967-0637(99)00048-5
Nair H. P., Puthusseri R. M., Vincent H., Bhat S. G. (2017). 16S rDNA-based bacterial diversity analysis of Arabian Sea sediments: A metagenomic approach. Ecol. Genet. Genomics 3, 47–51. doi: 10.1016/j.egg.2017.09.001
Nemergut D. R., Costello E. K., Hamady M., Lozupone C., Jiang L., Schmidt S. K., et al. (2011). Global patterns in the biogeography of bacterial taxa. Environ. Microbiol. 13 (1), 135–144. doi: 10.1111/j.1462-2920.2010.02315.x
Nogi Y. (2017). “Microbial life in the deep sea: Psychropiezophiles,” in Psychrophiles: from biodiversity to biotechnology (Cham: Springer), 133–152.
Padmanaban V. P., Verma P., Gopal D., Sekar A. K., Ramalingam K. (2019). Phylogenetic identification and metabolic potential of bacteria isolated from deep sea sediments of Bay of Bengal and Andaman Sea. Indian J. Exp. Biol. 57, 561–572.
Padmanaban V. P., Verma P., Venkatabaskaran S., Keppayan T., Gopal D., Sekar A. K., et al. (2017). Antimicrobial potential and taxonomic investigation of piezotolerant Streptomyces sp. NIOT-Ch-40 isolated from deep-sea sediment. World J. Microbiol. Biotechnol. 33, 1–11. doi: 10.1007/s11274-016-2193-2
Pakhomova S. V., Hall P. O., Kononets M. Y., Rozanov A. G., Tengberg A., Vershinin A. V. (2007). Fluxes of iron and manganese across the sediment–water interface under various redox conditions. Mar. Chem. 107 (3), 319–331. doi: 10.1016/j.marpolbul.2021.112031
Pandey V., Venkatnarayanan S., Kumar P. S., Ratnam K., Jha D. K., Rajaguru S., et al. (2021). Assessment of ecological health of Swarnamukhi river estuary, southeast coast of India, through AMBI indices and multivariate tools. Mar. Pollut. Bull. 164, 112031. doi: 10.1016/j.marpolbul.2021.112031
Parkes R. J., Cragg B., Roussel E., Webster G., Weightman A., Sass H. (2014). A review of prokaryotic populations and processes in sub-seafloor sediments, including biosphere: geosphere interactions. Mar. Geol. 352, 409–425. doi: 10.1016/j.marpolbul.2021.112031
Parvathi A., Jasna V., Aswathy V. K., Aparna S., Nathan V. K., Jyothibabu R. (2020). Dominance of Wolbachia sp. in the deep-sea sediment bacterial metataxonomic sequencing analysis in the Bay of Bengal, Indian Ocean. Genomics 112 (1), 1030–1041. doi: 10.1016/j.ygeno.2019.06.019
Polymenakou P. N., Lampadariou N., Tselepides A. (2008). Exo-enzymatic activities and organic matter properties in deep-sea canyon and slope systems off the southern Cretan margin. Deep Sea Res. Part I: Oceanographic Res. Papers 55 (10), 1318–1329. doi: 10.1016/j.dsr.2008.05.010
Preston-Mafham J., Boddy L., Randerson P. F. (2002). Analysis of microbial community functional diversity using sole-carbon-source utilisation profiles–a critique. FEMS Microbiol. Ecol. 42 (1), 1–14. doi: 10.1111/j.1574-6941.2002.tb00990.x
Raghukumar C., Sheelu G., Loka Bharathi P. A., Nair S., Mohandass C. (2001). Microbial biomass and organic nutrients in the deep-sea sediments of the Central Indian Ocean Basin. Mar. Georesources Geotechnol. 19 (1), 1–16.
Rajpathak S. N., Banerjee R., Mishra P. G., Khedkar A. M., Patil Y. M., Joshi S. R., et al. (2018). An exploration of microbial and associated functional diversity in the OMZ and non-OMZ areas in the Bay of Bengal. J. Biosci. 43, 635–648. doi: 10.1007/s12038-018-9781-2
Ramirez-Llodra E., Brandt A., Danovaro R., De Mol B., Escobar E., German C. R., et al. (2010). Deep, diverse and definitely different: unique attributes of the world's largest ecosystem. Biogeosciences 7 (9), 2851–2899. doi: 10.5194/bg-7-2851-2010
Rogers A. D. (2015). Environmental change in the deep ocean. Annu. Rev. Environ. Resour. 40, 1–38. doi: 10.1146/annurev-environ-102014-021415
Sambrook J., Russell D. W. (2001). Molecular cloning-sambrook & Russel-vol. 1, 2, 3 (Long Island, NY, USA: Cold Springs Harbor Lab Press).
Sattarova V. V., Aksentov K. I. (2021). Trace metals in deep-sea sediments collected from Kuril Basin (Sea of Okhotsk) and Kuril-Kamchatka Trench area. Mar. pollut. Bull. 164, 112055. doi: 10.1016/j.marpolbul.2021.112055
Saunders J. K., McIlvin M. R., Dupont C. L., Kaul D., Moran D. M., Horner T., et al. (2022). Microbial functional diversity across biogeochemical provinces in the central Pacific Ocean. Proc. Natl. Acad. Sci. 119 (37), e2200014119. doi: 10.1073/pnas.2200014119
Säwström C., Serrano O., Rozaimi M., Lavery P. S. (2016). Utilization of carbon substrates by heterotrophic bacteria through vertical sediment profiles in coastal and estuarine seagrass meadows. Environ. Microbiol. Rep. 8 (5), 582–589. doi: 10.1111/1758-2229.12406
Scheckenbach F., Hausmann K., Wylezich C., Weitere M., Arndt H. (2010). Large-scale patterns in biodiversity of microbial eukaryotes from the abyssal sea floor. Proc. Natl. Acad. Sci. 107 (1), 115–120. doi: 10.1073/pnas.0908816106
Serrano O., Mateo M. A., Dueñas-Bohórquez A., Renom P., López-Sáez J. A., Cortizas A. M. (2011). The Posidonia oceanica marine sedimentary record: A Holocene archive of heavy metal pollution. Sci. Total Environ. 409 (22), 4831–4840. doi: 10.1016/j.scitotenv.2011.08.001
Sinha R. K., Krishnan K. P., Thomas F. A., Binish M. B., Mohan M., Kurian P. J. (2019). Polyphasic approach revealed complex bacterial community structure and function in deep sea sediment of ultra-slow spreading Southwest Indian Ridge. Ecol. Indic. 96, 40–51. doi: 10.1016/j.ecolind.2018.08.063
Sogin M. L., Morrison H. G., Huber J. A., Welch D. M., Huse S. M., Neal P. R., et al. (2006). Microbial diversity in the deep sea and the underexplored “rare biosphere”. Proc. Natl. Acad. Sci. 103 (32), 12115–12120. doi: 10.1073/pnas.0605127103
Stackebrandt E., Goebel B. M. (1994). Taxonomic note: a place for DNA-DNA reassociation and 16S rRNA sequence analysis in the present species definition in bacteriology. . Int. J. Syst. Bacteriol 44, 846–849. doi: 10.1099/00207713-44-4-846
Sweetman A. K., Thurber A. R., Smith C. R., Levin L. A., Mora C., Wei C.-L., et al. (2017). Major impacts of climate change on deep-sea benthic ecosystems. Elem Sci. Anth 5, 4. doi: 10.1525/elementa.203
Taylor S. R. (1964). Abundance of chemical elements in the continental crust: a new table. Geochimica cosmochimica Acta 28 (8), 1273–1285. doi: 10.1016/0016-7037(64)90129-2
Thurber A. R., Sweetman A. K., Narayanaswamy B. E., Jones D. O., Ingels J., Hansman R. L. (2014). Ecosystem function and services provided by the deep sea. Biogeosciences 11 (14), 3941–3963. doi: 10.5194/bg-11-3941-2014
Trask P. D. (1939). Organic content of recent marine sediments: part 6. Special features of sediments. Amer. Assoc. Petrol. Geologists, Tulsa. 428–453
Tripathy G. R., Singh S. K., Ramaswamy V. (2014). Major and trace element geochemistry of Bay of Bengal sediments: Implications to provenances and their controlling factors. Palaeogeography Palaeoclimatology Palaeoecol. 397, 20–30. doi: 10.1016/j.palaeo.2013.04.012
Tuohy A., Bertler N., Neff P., Edwards R., Emanuelsson D., Beers T., et al. (2015). Transport and deposition of heavy metals in the Ross Sea Region, Antarctica. J. Geophysical Research: Atmospheres 120 (20), 10–996. doi: 10.1002/2015JD023293
UNESCO (1979). Discharge of selected rivers of the world: A contribution to the international hydrological decade (Paris: UNESCO), 104.
USEPA (2001). Supplemental guidance for developing soil screening levels for superfund sites (Office of Soild Waste and Emergency Response). OSWER USEPA, 9355.4-24.
Varkey M. J., Murty V. S. N., Suryanarayana A. (1996). Physical oceanography of the bay of Bengal and Andaman sea. Oceanogr. Mar. Biol. Annu. Rev. 34, 1–70.
Verma P., Raghavan R. V., Jeon C. O., Lee H. J., Priya P. V., Dharani G., et al. (2017). Complex bacterial communities in the deep-sea sediments of the Bay of Bengal and volcanic Barren Island in the Andaman Sea. Mar. Genomics 31, 33–41. doi: 10.1016/j.margen.2016.08.003
Vipindas P. V., Mujeeb R. K. M., Jabir T., Thasneem T. R., Hatha A. M. (2020). Diversity of sediment bacterial communities in the South Eastern Arabian Sea. Regional Stud. Mar. Sci. 35, 101153. doi: 10.1016/j.rsma.2020.101153
Walsh E. A., Kirkpatrick J. B., Rutherford S. D., Smith D. C., Sogin M., D'Hondt S. (2016). Bacterial diversity and community composition from seasurface to subseafloor. ISME J. 10 (4), 979–989. doi: 10.1038/ismej.2015.175
Wang L., Liu L., Zheng B., Zhu Y., Wang X. (2013). Analysis of the bacterial community in the two typical intertidal sediments of Bohai Bay, China by pyrosequencing. Mar. Pollut. Bull. 72 (1), 181–187. doi: 10.1016/j.marpolbul.2013.04.005
Wang F., Zhang Y., Jing H., Liu H. (2022). Spatial variation and metabolic diversity of microbial communities in the surface sediments of the Mariana Trench. Front. Microbiol. 13, 1051999. doi: 10.3389/fmicb.2022.1051999
Wangersky P. J. (1986). Biological control of trace metal residence time and speciation: a review and synthesis. Mar. Chem. 18 (2-4), 269–297. doi: 10.1016/0304-4203(86)90013-7
Weber M. E., Wiedicke-Hom M., Kudrass H. R., Erlenkeuser H. (2003). Bengal Fan sediment transport activity and response to climate forcing inferred from sediment physical properties. Sedimentary Geology 155 (3-4), 361–381. doi: 10.1016/S0037-0738(02)00187-2
Ye W., Liu S., Fan D., Zhang H., Cao P., Pan H. J., et al. (2022). Evolution of sediment provenances and transport processes in the central Bay of Bengal since the Last Glacial Maximum. Quaternary Int. 629, 27–35. doi: 10.1016/j.quaint.2020.12.007
Yin H., Niu J., Ren Y., Cong J., Zhang X., Fan F., et al. (2015). An integrated insight into the response of sedimentary microbial communities to heavy metal contamination. Sci. Rep. 5 (1), 14266. doi: 10.1038/srep14266
Yoon S. H., Ha S. M., Kwon S., Lim J., Kim Y., Seo H., et al. (2017). Introducing EzBioCloud: a taxonomically united database of 16S rRNA gene sequences and whole-genome assemblies. Int. J. Syst. Evol. Microbiol. 67 (5), 1613–1617. doi: 10.1099/ijsem.0.001755
Zehr J. P., Kudela R. M. (2011). Nitrogen cycle of the open ocean: from genes to ecosystems. Annu. Rev. Mar. Sci. 3, 197–225. doi: 10.1146/annurev-marine-120709-142819
Zheng B., Wang L., Liu L. (2014). Bacterial community structure and its regulating factors in the intertidal sediment along the Liaodong Bay of Bohai Sea, China. Microbiological Res. 169 (7-8), 585–592. doi: 10.1016/j.micres.2013.09.019
Keywords: deep-sea sediment, microbial community, benthos, spatial variation, heavy metals, ecto-enzyme activity, metabolic profile
Citation: Verma P, Pandey V, Seleyi SC, Alagarsamy A and Dharani G (2024) Exploring the hidden treasures: Deep-sea bacterial community structure in the Bay of Bengal and their metabolic profile. Front. Mar. Sci. 10:1308953. doi: 10.3389/fmars.2023.1308953
Received: 07 October 2023; Accepted: 29 November 2023;
Published: 08 January 2024.
Edited by:
Ganesh Thiruchitrambalam, Port Blair Campus, IndiaReviewed by:
Gopalakrishnan Thilagam, Pachaiyappa's College for Men, IndiaSatheeswaran Thangaraj, China University of Geosciences Wuhan, China
Nithyanandam Marimuthu, Zoological Survey of India, India
Copyright © 2024 Verma, Pandey, Seleyi, Alagarsamy and Dharani. This is an open-access article distributed under the terms of the Creative Commons Attribution License (CC BY). The use, distribution or reproduction in other forums is permitted, provided the original author(s) and the copyright owner(s) are credited and that the original publication in this journal is cited, in accordance with accepted academic practice. No use, distribution or reproduction is permitted which does not comply with these terms.
*Correspondence: Pankaj Verma, vermabios@gmail.com; Gopal Dharani, dhara@niot.res.in