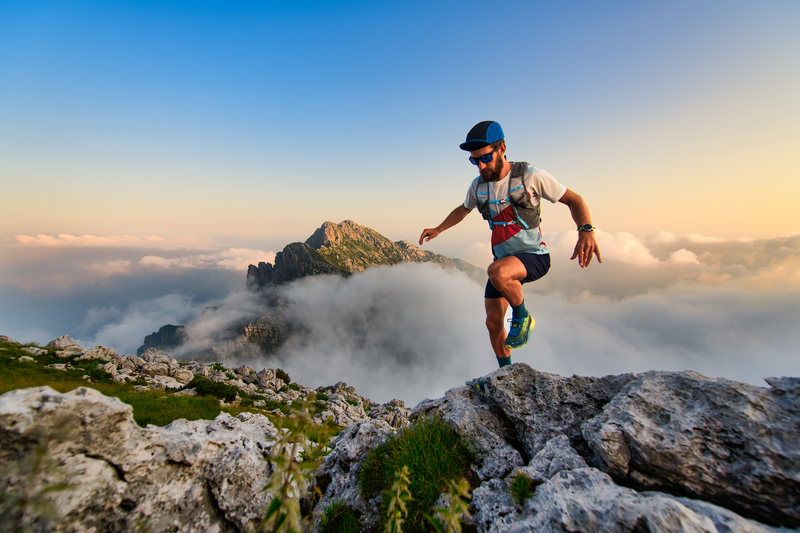
95% of researchers rate our articles as excellent or good
Learn more about the work of our research integrity team to safeguard the quality of each article we publish.
Find out more
ORIGINAL RESEARCH article
Front. Mar. Sci. , 12 January 2022
Sec. Coastal Ocean Processes
Volume 8 - 2021 | https://doi.org/10.3389/fmars.2021.792294
This article is part of the Research Topic Coastal Wetlands Dynamics View all 15 articles
Understanding the microbial community assembly is an essential topic in microbial ecology. Coastal wetlands are an important blue carbon sink, where microbes play a key role in biogeochemical cycling of nutrients and energy transformation. However, the drivers controlling the distribution patterns and assembly of bacterial and archaeal communities in coastal wetland are unclear. Here we examined the diversity, co-occurrence network, assembly processes and environmental drivers of bacterial and archaeal communities from inshore to offshore sediments by the sequencing of 16S rRNA gene amplicons. The value of α- and β-diversity of bacterial and archaeal communities generally did not change significantly (P > 0.05) between offshore sites, but changed significantly (P < 0.05) among inshore sites. Sediment pH and salinity showed significant effects on the diversity and keystone taxa of bacterial and archaeal communities. The bacterial and archaeal co-occurrence networks were inextricably linked with pH and salinity to formed the large network nodes, suggesting that they were the key factors to drive the prokaryotic community. We also identified that heterogeneous and homogeneous selection drove the bacterial and archaeal community assembly, while the two selections became weaker from offshore sites to inshore sites, suggesting that deterministic processes were more important in offshore sites. Overall, these results suggested that the environmental filtering of pH and salinity jointly governed the assembly of prokaryotic community in offshore sediments. This study advances our understanding of microbial community assembly in coastal wetland ecosystems.
Microbes are an essential component of ecosystems and drive a variety of elements cycles and substances transformation in natural ecosystems, including carbon mineralization, nitrogen sequestration and phosphorus release (Leff et al., 2015; Fierer, 2017; Wei et al., 2020; Yu et al., 2020, 2021a). Coastal ecosystems store large amounts of blue carbon due to their high primary productivity rates and slow decomposition rates (Donato et al., 2011; McLeod et al., 2011; Alongi, 2014). Meanwhile, coastal ecosystems exhibit special environmental conditions such as high salinity, aerobic-anaerobic exchange, and rich organic matter, supporting that various unique microbes for biogeochemical cycling of carbon, nitrogen and sulfur (Lin et al., 2019; Zhang C. J. et al., 2019, 2020; Behera et al., 2020; Liu X. et al., 2020; Li et al., 2021). Previous studies indicated that the diversity, assembly and functional traits of microbial communities varied across coastal ecosystems (Lauber et al., 2009; Rousk et al., 2010; Rath and Rousk, 2015; Ma et al., 2017; Zhong et al., 2017; Yu et al., 2020). However, much less is known about the drivers of microbial community assembly in coastal ecosystems.
Understanding the assembly processes of microbial communities remain a central issue in community ecology (Nemergut et al., 2013; Zhou and Ning, 2017). Two types of processes (i.e., deterministic and stochastic) generally impact the diversity, assembly and co-occurrence patterns of species in microbial communities (Zhou et al., 2014; Dini-Andreote et al., 2015). Traditional niche theory assumes that deterministic processes, including environmental filtering (e.g., pH, nutrients, moisture, temperature) and biotic factors (e.g., species traits, interspecies interactions), govern the microbial community assembly (Chesson, 2000; Fargione et al., 2003). Conversely, neutral theory hypothesizes that all species are ecologically equivalent, and stochastic processes, including dispersal and ecological drift, control the microbial community assembly (Chave, 2004; Rosindell et al., 2011). Currently, it is accepted that both deterministic and stochastic processes govern the microbial community assembly, and their relative importance to microbial communities depend on the located environmental conditions (Yan et al., 2017; Mo et al., 2018; Jiao et al., 2020; Li et al., 2020; Ning et al., 2020). For example, determinism played a stronger influence than stochasticity on the bacterial community assembly in a lake wetland (Logares et al., 2018), while stochastic processes were more important in the archaeal community assembly of mangrove ecosystems (Liu J. et al., 2020). However, the relative importance of deterministic and stochastic processes was difficult to quantitatively evaluate in different ecosystems (Chase, 2010; Zhou et al., 2014; Jiao et al., 2020). One limitation is that environmental conditions often change systematically with space-time such that these variables are difficult to assess (Tripathi et al., 2018).
Environmental factors have significant effects on driving microbial community assembly (Rath and Rousk, 2015; Jiao and Lu, 2020a; Yu et al., 2021b). Previous studies indicated that pH could influence the diversity and structure of microbial communities at local (Tripathi et al., 2014; Yu et al., 2020; Zhong et al., 2020), regional (Griffiths et al., 2011; Tripathi et al., 2012; Jiao et al., 2019) and global scales (Fierer and Jackson, 2006). Recent studies found that pH regulated the balance between stochastic and deterministic processes of microbial community assembly in freshwater lakes (Ren et al., 2015) and successional soils (Tripathi et al., 2018). Also, salinity exerts substantial influence on microbial communities in different ecosystems (Rath and Rousk, 2015; Rath et al., 2019). For instance, salinity was the strongest factor for bacterial community dissimilarity and components in desert ecosystems (Campbell and Kirchman, 2013; Zhang K. et al., 2019). High salinity was often associated with the shift toward an archaeal dominated community (Ventosa et al., 2014; Vavourakis et al., 2016; Zhong et al., 2016). However, how these environmental factors mediate the microbial community assembly remains unclear.
Microbial interactions (e.g., competition, mutualism, predation and mutualism) can help us reveal microbial responses to environmental variations (Deng et al., 2012; Weiss et al., 2016; Ma et al., 2020). Network analysis is a useful method to decipher possible microbial interactions and identify keystone taxa (Wang B. et al., 2020), which provide comprehensive insights into co-occurrence patterns of microbial communities and assembly mechanisms (Berry and Widder, 2014; Banerjee et al., 2018; Mamet et al., 2019). Recent studies indicated that the changes in keystone taxa and interactions were closely associated with the shift of microbial community diversity, structure and its ecological functions (Herren and McMahon, 2018; Ma et al., 2020). The competitive interactions among keystone taxa could increase the diversity of bacterial and fungal communities to decreased loss of soil organic carbon (Chen et al., 2019). Thus, a comprehensive understanding of the effects of key environment factors on co-occurrence networks of microbial communities could help to reveal microbial assembly mechanisms.
Since coastal wetlands are influenced by tides periodically, the dispersal effects of seawater may become weaker from inshore sites to offshore sites. Therefore, in this study, we aimed to understand the microbial community assembly and their driving factors in coastal ecosystems. We tested the distribution patterns (e.g., the diversity, composition, co-occurrence patterns and keystone taxa) and assembly processes of bacterial and archaeal communities in coastal sediments with different concentrations of salt and pH values. Our results showed that environmental filtering of pH and salinity jointly drove the prokaryotic community assembly, which largely expanded our understanding of the role of environmental drivers on the assembly of prokaryotic communities in coastal ecosystems.
All samples were collected from the Qi’ao Island (113°36′∼113°39′E, 22°23′∼22°27′N) located on the western side of the mouth of the Pearl River Estuary in Guangdong province, Southern China. The annual average temperature and precipitation are 22.4°C and 1,964 mm, respectively. The area of this island is 24 km2 and the sediment is mainly composed of clayey silt (Wu et al., 2017). We collected five field samples from inshore sites (randomly named CM, SAP, and KO sites) to offshore sites (randomly named MF, SA sites) in July 2018 (Supplementary Figure S1). In each sites, we established six about 2 m × 2 m mudflats and a sediment core was sampled in each plot using a custom-made sampler (8 cm in diameter, 10 cm in depth). In total, 30 samples (5 sites × 6 replicates) were collected, then all samples were sealed in sterile bags and transported to the laboratory in ice boxes within 12 h. Each sample was divided into two sub-samples: one was kept at 4°C for sediment properties analysis, and the other was stored at –80°C for DNA extraction within 24 h.
The sediment properties were measured as previously described (Yu et al., 2020). Briefly, the temperature in situ was determined by a temperature meter (PHBJ-260, INESA instrument, China). The fresh sediment (2.0 g) was centrifuged at 3500 rpm for 10 min. The supernatant was collected to measure the contents of ammonium nitrogen (NH4+-N), nitrate nitrogen (NO3–-N), and nitrite nitrogen (NO2–-N) by a continuous flow analyzer (SKALAR San++, Skalar Co., Netherlands) (Hong et al., 2019). The water content was measured after drying 5.0 g fresh sediments at 105°C to a constant weight. The sediments before measuring pH, salinity, total carbon (TC) and total nitrogen (TN) were dried at 65°C to a constant weight. TC and TN were detected with 40 mg dry sediment using an elemental analyzer (Vario TOC, Elemental, Germany). The pH was determined by a pH meter (SevenCompact210, Mettler-Toledo, United States) with water and sediment ratio of 2.5:1. Salinity was measured by a salinity meter (EUTECH SALT6+, Thermo Scientific, United States) with water and sediment ratio of 5:1.
Microbial community DNA was extracted from the sediment using a modified sodium dodecyl sulfate extraction method (Zhou et al., 1996) combined with a Power Soil DNA kit (Mo Bio Laboratories, Carlsbad, CA, United States) following the manufacturer’s instructions. Briefly, sediment samples had been fully ground with liquid nitrogen before DNA extraction. Then, DNA was extracted from 0.5 g sediment by using the same method described above. The quality of DNA was determined by a NanoDrop spectrophotometer (NanoDrop One, Thermo Fisher Scientific, United States) with ratios of 260/280 and 260/230, and then diluted into 2 ng μL–1 for subsequent PCR amplification.
The primers 338F (5′-ACTCCTACGGGAGGCAGCA-3′) and 806R (5′-GGACTACHVGGGTWTCTAAT-3′) were used for amplifying of V3-V4 hypervariable region of bacterial 16S rRNA gene with an expected fragment size of 468 bp (Wang et al., 2019), and the primers Arch349F (5′-GYGCASCAGKCGMGAAW-3′) and Arch806R (5′-GGACTACVSGGGTATCTAAT-3′) were used to amplify V3-V4 hypervariable region of archaeal 16S rRNA gene with a correct size of 458 bp (Frank et al., 2013). Amplicon sequencing was performed with an Illumina HiSeq platform 2500 (Illumina, Inc., CA, United States) (2 × 250 paired ends) in Biomarker Technologies Corporation, Beijing, China. Raw 16S rRNA gene sequences data were deposited in the NCBI Sequence Read Archives1 with accession numbers PRJNA666027 and PRJNA666234, respectively.
The trimming process of rRNA gene amplicon sequences were conducted by a publicly available pipeline2 (Yu et al., 2021b). In brief, each sample was assigned based on unique barcode and the primers were removed using Cutadapt (Martin, 2011). Low-quality sequences (length < 350 bp, with the ambiguous base “N,” and average base quality score < 30) and poorly overlapped were filtered by Trimmomatic (Bolger et al., 2014). Potential chimeric reads were identified and removed from the dataset using UCHIME in reference database mode (Edgar, 2013). The high-quality sequences were clustered into operational taxonomic units (OTUs) at a 97% identity threshold by UPARSE (Edgar, 2013) and singleton OTUs were not involved in subsequent analysis. Resampling was performed for bacterial sequences (53,166) and archaeal sequences (76,548) to make sure that the sequencing depth is same for all the samples (Supplementary Table S1). Taxonomy was identified by the Ribosomal Database Project classifier at a confidence level of 80% (Wang et al., 2007; Zhong et al., 2017).
To understand possible interactions among different taxa of microbial community and identify potential keystone taxa, the networks of bacterial and archaeal communities were constructed by the Molecular Ecological Network Analysis (MENA) pipeline3 (Deng et al., 2012). In each network, the size of each node is proportional to the number of connections (i.e., degree). Nodes represented individual microbial taxa and edges indicate the pairwise correlations between nodes. The connectivity of each node was estimated by its within-module connectivity (Zi) and among-module connectivity (Pi). The nodes with either a high value of Zi or Pi were identified as potential keystone taxa, including module hubs (Zi ≥ 2.5, Pi < 0.62), connectors (Zi < 2.5, Pi ≥ 0.62), and network hubs (Zi ≥ 2.5, Pi ≥ 0.62) (Guimera and Amaral, 2005). The final visualization of networks was generated by Gephi software (Bastian et al., 2009).
Structural equation modeling (SEM) is theory-oriented and capable of evaluating network of causal hypotheses via testing simultaneous influences rather than individual (bivariate) causes (Grace et al., 2012; Eisenhauer et al., 2015). A large amount of studies confirm that it is a powerful tool to reveal the synergistic relationships between multiple environmental factors and the diversity or keystone taxa of microbial communities (Chen et al., 2019; Mamet et al., 2019; Li et al., 2020). To untangle the relationship between the two most important sediment parameters (pH and salinity), keystone taxa and microbial diversity, we conducted SEM analysis in AMOS 22.0 (AMOS IBM, United States), and the fitness was evaluated by a non-significant chi-square test (χ2, P > 0.05), the goodness-of-fit index (GFI) and the root mean square error of approximation (RMSEA) (Byrne, 2016).
To quantitatively evaluate the contribution of major ecological processes to bacterial and archaeal community assembly, we used a null-model-based framework (Stegen et al., 2013; Dini-Andreote et al., 2015). The dynamics of phylogenetic and taxonomic diversity were measured with the null model-based beta nearest taxon indices (βNTI) and Bray–Curtis-based Raup–Crick (RCBray) metrics, respectively (Stegen et al., 2013). The βNTI > 2 was interpreted as heterogeneous selection, and βNTI < –2 was denoted as homogeneous selection. When the | βNTI| < 2 and RCBray < –0.95, the community assembly is assembled primarily by the homogenizing dispersal. Whereas | βNTI| < 2 and RCBray > 0.95, the community is mainly driven by the dispersal limitation. When the | βNTI| < 2 and | RCBray| < 0.95, that means no dominant assembly process (Stegen et al., 2013). All above ecological processes analyses were performed in a publicly available pipeline (see footnote 2).
Before statistical analysis, normal distribution was examined and non-normal data were transformed to normal ones using Blom’s formula under equivalent command Rank Cases (Li et al., 2016). The α-diversity was evaluated by Chao1 and Shannon indices, and the β-diversity was estimated by Bray–Curtis distances. The community dissimilarity was visualized using principal co-ordinates analysis (PCoA) based on the Bray–Curtis distances, and the statistical significance was tested by permutational multivariate analysis (PERMANOVA) and analysis of similarities (ANOSIM) using adonis and anosim functions in the R package vegan (Yu et al., 2021a). The relationships between sediment parameters and microbial communities were analyzed by Mantel tests and redundancy analysis (RDA). Variation partition analysis (VPA) was used to quantify the relative contributions of the physicochemical factors to bacterial and archaeal communities with the vegan package (Yu et al., 2021b). Differences among sites were evaluated by one-way analysis of variance (ANOVA) in SPSS 18.0 software.
To determined variations of the environmental factors, the sediment physicochemical parameters (pH, salinity, temperature and moisture content) and nutrient contents (TC, TN, NH4+-N, NO2–-N, and NO3–-N) from offshore sites (MF, SA) and offshore sites (CM, SAP, and KO) were analyzed (Supplementary Figure S1). The results showed that pH was significantly (P < 0.05) higher in MF sites than in CM and KO sites, while the salinity was significantly (P < 0.05) higher in MF, SA sites than in CM and SAP sites (Table 1), indicating a significant increased sediment pH and salinity in offshore sites. For nutrient contents, the concentrations of TC, TN, and NH4+-N were significantly (P < 0.05) higher in CM, SAP, and KO sites than in MF and SA sites, indicating a high nutrient level in inshore sites.
We obtained a total of 2,828,732 and 3,827,293 high-quality bacterial and archaeal sequences, respectively (Supplementary Table S1). The Chao1 value and Shannon indices of both bacterial and archaeal communities were highest in KO sites, followed by MF, SA, CM sites, and lowest in SAP sites, while there were no significant (P < 0.05) differences between MF, SA, and CM sites (Figures 1A–D). The β-diversity (Bray–Curtis dissimilarity) within bacterial communities was significantly (P < 0.05) lower in MF sites than other sites (Figure 1E), and the β-diversity of archaeal communities was significantly (P < 0.05) lower in MF, SA, and CM sites than in SAP and KO sites (Figure 1F). The results indicated that α- and β-diversity of prokaryotic communities generally did not change significantly (P > 0.05) between offshore sites, but changed significantly (P < 0.05) among inshore sites.
Figure 1. The α-diversity (Chao1 and Shannon indices) of bacterial (A,C) and archaeal (B,D) communities in the coastal wetland. The β-diversity within one site was based on the Bray–Curtis distance of bacterial (E) and archaeal (F) communities. Different lowercase letters indicate a significant difference at the level of P < 0.05 (n = 6).
Principal coordinates analysis (PCoA) showed that bacterial and archaeal communities were well separated across five sites (Figures 2A,B). Two non-parametric tests (Adonis and Anosim) showed consistently significant (P < 0.001) variations in bacterial and archaeal communities among five sites, indicating that sediment factors shifted the structure of prokaryotic communities. The bacterial communities were dominated by phyla Proteobacteria (36.4%), Chloroflexi (21.1%) and Acidobacteria (7.8%) (Supplementary Figure S2A). The majority of archaea belonged to phyla Crenarchaeota (61.3%), Euryarchaeota (32.4%), and Parvarchaeota (6.0%) (Supplementary Figure S2B). The dominant bacterial genera included Nitrospira (1.3%), Rhodoplanes (0.8%), and Desulfococcus (0.6%) (Supplementary Figure S3A). The relative abundance of Nitrospira, Rhodoplanes, and Desulfococcus was significantly (P < 0.05) higher in MF, SAP, and KO sites, respectively, than other sites (Figure 2C). For archaeal communities, the majority of classes belonged to Bathyarchaeota (34.3%), Methanomicrobia (27.9%), Thaumarchaeota (25.7%), Parvarchaea (5.8%), and Thermoplasmata (3.4%) (Supplementary Figure S3B). Specifically, the order of Methanosarcina in SA and CM sites was significantly (P < 0.05) higher than that in MF, SAP, and KO sites (Supplementary Table S2). The top 11 genera belonged to methanogens, including Methanosaeta (19.3%), Candidatus Methanoregula (2.7%), and Methanolinea (2.7%) (Supplementary Figure S3B). The relative abundance of Methanosaeta and Candidatus Methanoregula was higher in SA and CM sites than other three sites (Figure 2D), indicating that methanogens dominated in SA and CM sites.
Figure 2. Successional dynamics of prokaryotic communities in the coastal wetlands. Principal coordinates analysis (PCoA) of bacterial (A) and archaeal (B) communities. The relative abundance of top four bacterial (C) and archaeal (D) genera; values in brackets are percentages of explained variations for PCoA1 and PCoA2 of bacterial and archaeal communities. Different lowercase letters indicate a significant difference at the level of P < 0.05 (n = 6).
To identify potential interactions and keystone taxa of microbial communities in coastal wetland sediments, we constructed co-occurrence networks of bacterial and archaeal communities, respectively (Supplementary Figure S4 and Supplementary Table S3). The proportions of positive correlations between nodes in both bacterial and archaeal networks were 64.7 and 99.3%, respectively (Figures 3A,C), indicating that cooperation was fundamental in bacterial and archaeal communities. The microbial community co-occurrence networks showed strong positive and negative links with salinity and pH, respectively (Figures 3B,D). For bacteria, we detected 16 keystone OTUs and the most dominant taxa (relative abundance > 0.1%) were derived from Gammaproteobacteria (OTU5 and OTU124) and Betaproteobacteria (OTU12 and OTU82) (Supplementary Figure S4A). The relative abundances of the four OTUs were significantly (P < 0.05) higher in MF and SA sites than in CM, SAP and KO sites (Supplementary Figure S4C). For archaea, we identified four keystone OTUs, including two module hubs belonging to class Bathyarchaeota (OTU7884) and Heimdallarchaeota (OTU28), and two connectors derived from Bathyarchaeota (OTU37 and OTU100) (Supplementary Figure S4B). The relative abundances of these four OTUs were significantly (P < 0.05) higher in MF and SA sites than in CM and SAP sites (Supplementary Figure S4D). Especially, the top four keystone OTUs of bacteria were significantly (P < 0.05) positive correlation with pH and salinity, and the top four keystone OTUs of archaea were significantly (P < 0.05) positive correlation with salinity (Supplementary Figure S5). Collectively, the bacterial and archaeal keystone taxa were mainly distributed in offshore sites and had close links with pH and salinity.
Figure 3. Co-occurrence networks patterns of bacterial (A) and archaeal (C) communities and their relationships with the environmental parameters (B,D), respectively in the coastal wetland. A connection indicates a strong (Spearman’s |r| > 0.8) and significant (P < 0.01) correlation. Each node represents an OTU. The green line represent a positive correlation, while red line represent a negative correlation. A summary of node-edge statistics was also given, and numbers represent the nodes or edges.
To reveal the relationship between sediment factors and the microbial community structure, their correlations were analyzed by Mantel tests (Supplementary Table S4) and Pairwise Spearman’s correlation (Supplementary Figure S6). The factors with significant (P < 0.05) influence were further assessed by RDA. The results showed that pH, TC and NH4+ were the major factors to explain bacterial community variations (Figure 4A), while pH were strongly correlated with archaeal communities (Figure 4B). In addition, the salinity showed more stronger effects on the prokaryotic community in offshore sites than that in inshore sites (Figures 4A,B). VPA further indicated that sediment pH and salinity clearly contributed to a large proportion of variation to bacterial (12.3%, 40.6%) and archaeal (22.7%, 33.8%) community, respectively (Figures 4C,D). Also, linear regression analysis showed that the α-diversity of bacterial and archaeal communities was significantly (P < 0.05) positively associated with salinity (Supplementary Figures S7A,B). The β-diversity showed a significantly (P < 0.05) positive correlation with pH and salinity (Supplementary Figure S7C). In addition, pH and salinity showed strong effects on the relative aboundance of bacterial and archaeal taxa. For example, pH was significantly (P < 0.05) associated with the top four bacterial phyla (Supplementary Figure S8A), the top three archaeal classes (Supplementary Figure S8B), and the top four archaeal genera (Supplementary Figure S9B). Collectively, these results indicated that sediment pH and salinity could influence the diversity, composition and structure of bacterial and archaeal communities.
Figure 4. Drivers of the prokaryotic communities in coastal wetlands. Redundancy analysis of environmental variables for bacterial (A) and archaeal (B) communities. Variation partition analysis (VPA) showed the effects of environmental factors on the bacterial (C) and archaeal (D) community structure. N value is less than 0.1%. TC, total carbon; MC, moisture content; NH4+-N, ammonium nitrogen; NO3–-N, nitrate nitrogen. Other sediment parameters including TC, MC, NH4+-N, NO3–-N, NO2–-N, and temperature.
To better understand the effects of pH and salinity on the diversity and keystone taxa of microbial communities, we further constructed SEM. For bacterial communities, the result showed that sediment pH and salinity had significant (P < 0.01) positive effects on bacterial keystone taxa (Figure 5A). Also, salinity had significant positive effects on the α- and β-diversity of bacterial community (standardized effects = 0.47, P < 0.01; 0.38, P < 0.05). However, sediment pH could affect bacterial keystone taxa to indirectly inhibit the β-diversity of bacterial community. For archaeal communities, sediment salinity showed significantly (P < 0.01) positive effects on the α-diversity and keystone taxa (Figure 5B). Moreover, salinity had indirect effects on the α-diversity of archaeal community through influencing their keystone taxa (P < 0.001). Sediment pH had significant negative effects on the α-diversity of archaeal communities (standardized effects = –0.25, P < 0.05). Overall, these results indicated that sediment pH and salinity could affect the diversity and keystone taxa of bacterial and archaeal communities.
Figure 5. Structural equation modeling (SEM) analysis showing the direct and indirect effects of pH and salinity on the diversity and keystone taxa of bacterial (A) and archaeal (B) communities. Values along the arrows are standardized regression coefficients. R2 represents the proportion of variance explained for the dependent variable. Green and red arrow indicate a significant positive and negative path coefficient, respectively. The thickness represents the strength of path coefficients. The β-diversity is represented by the average Bray–Curtis dissimilarity among all samples. The keystone OTUs and α-diversity are represented by the first principal component (PCA1) of keystone OTU relative abundance and α-diversity (Chao1 and Shannon indices), respectively. ***P < 0.001; **P < 0.01; *P < 0.05.
The abundance-weighted beta nearest taxon index (βNTI) showed that selection was one of essential processes in bacterial and archaeal communities assembly (Figure 6A). For bacterial community, the modified Raup–Crick (RCbray) played a principal role in inshore and offshore sites (Figure 6B). However, heterogeneous selection (21.6%) and homogeneous selection (6.0%) were higher in offshore sites than that in inshore sites, indicating that deterministic processes were more important in offshore sites. The relative contribution of stochastic process to the archaeal community was the highest in all sites (93.4%) (Figure 6C). The proportion of homogeneous selection to the archaeal community was the highest in inshore (95.6%) and offshore sites (81.9%), while dispersal limitation and homogenizing dispersal almost could be ignored. Therefore, stochastic process was major driving factor in the assembly of prokaryotic communities in offshore sites.
Figure 6. The quantified major ecological processes governing the prokaryotic communities in the coastal wetland. Patterns of βNTI from inshore sites to offshore sites (A). The relative contribution percentage of each process to bacterial (B) and archaeal (C) community assembly was governed primarily by various deterministic (heterogeneous and homogeneous selection) and stochastic processes.
In this study, we found that sediment pH and salinity could affect the diversity, structure, co-occurrence network and keystone taxa of bacterial and archaeal communities, suggesting that pH and salinity were key factors driving the prokaryotic community assembly. Moreover, the diversity pattern of bacterial and archaeal communities shifted from offshore sites to inshore sites largely due to the influence of selection processes. The heterogeneous and homogeneous selection became weak from offshore sites to inshore sites. These results demonstrated that the distribution pattern and assembly of prokaryotic communities were affected by the environmental filtering of pH and salinity in the coastal wetland ecosystem.
The deterministic processes govern microbial communities assembly through altering their diversity, composition, structure and keystone taxa in ecosystems (Roguet et al., 2015; Burns et al., 2016; Aguilar and Sommaruga, 2020). In this study, the α- and β-diversity of prokaryotic communities were lower in offshore sites than that in inshore sites. A major reason may be that the selection processes were stronger in offshore sites. Moreover, as coastal sediments are influenced by tides periodically, seawater flow brings dispersal (Cermeno and Falkowski, 2009; Langenheder and Lindstrom, 2019), and the inundated time is longer in offshore sites than inshore sites. Thus, it could make a stronger environmental filtering effect on the local microbial community structure (Mouquet and Loreau, 2003; Leibold et al., 2004). Moreover, low sediment nutrients may limit community variation or turnover in offshore sites, resulting in low diversity and low number of keystone taxa of prokaryotic communities (Stegen et al., 2013, 2015; Zhou and Ning, 2017). For offshore sites, sediments in water-unsaturated conditions inhibited dispersal apacity and represented a higher dispersal limitation for bacterial communities (Jiao et al., 2019). Therefore, a stronger selection processes was observed in offshore sites than inshore sites, which could explain a high keystone taxa and diversity of prokaryotic communities in inshore sites.
Sediment pH play a key role in driving the prokaryotic community assembly (Zhang K. et al., 2019; Liu X. et al., 2020). Although pH could alter the diversity and composition of prokaryotic communities (Yu et al., 2021b), it does not directly affect the prokaryotic community and mainly through affecting the structure of microbial cell membrane and availability of soil nutrients (Gabler et al., 2017). A slightly acidic or neutral microenvironment is conducive to the decomposition of plant litter, which could affect nutrient contents in coastal wetland sediments (Wang Y. et al., 2020). Previous studies indicated that pH was negatively associated with microbial α-diversity (Jiao and Lu, 2020b; Zhong et al., 2020). In this study, a neutral pH (from 6 to 7.5) could lead to a high α-diversity of bacterial and archaeal communities. The intracellular pH level of most microorganisms is closed to a neutral value (Ren et al., 2015; Tripathi et al., 2018). Thus, acidic pH may impose a strong stress on cell membrane, resulting in rapid loss of the highly sensitivity species (Gabler et al., 2017). Moreover, high pH values can reduce the microbial β-diversity through influencing their keystone OTUs. For example, the top four bacterial keystone OTUs were affiliated with Gammaproteobacteria and Betaproteobacteria, which showed an optimal pH of 6.0 (Lauber et al., 2009). The pH value of offshore sites was higher than 6.0, which may be benefit for the diversification of bacterial keystones taxa, consequently resulting in lower bacterial β-diversity (Herren and McMahon, 2018; Ma et al., 2020). Besides, sediment availability of nutrients such as organic carbon can regulate the composition, structure and diversity of microbial communities (Hu et al., 2014; Yang et al., 2016). Previous studies reported that coastal wetlands (e.g., mangroves and salt marshes) have high carbon sequestration rates, and usually maintain soil organic carbon stocks, which plays an important role in shaping microbial community structures (Sun et al., 2019; Xia et al., 2021). Neutral sediment pH showed a positive relationship with the organic carbon content, which contributes to utilization of the organic carbon by microorganisms (Sun et al., 2019). Although the content of sediment organic carbon was not detected in this study, the organic carbon should be an important factor influencing the prokaryotic community assembly.
We also found that sediment salinity was an important factor in driving the prokaryotic community assembly in the coastal wetland. It is generally expected that high salinity may present a high osmotic stress (Oren, 2011; Rath and Rousk, 2015), which may be beneficial for marine microbes and unfavorable to terrestrial microorganisms. For example, a previous study found that salinity was negatively associated with microbial α-diversity and regulated the microbial community assembly in desert ecosystem (Zhang K. et al., 2019). In this study, the salinity of sediment (about 2‰) showed a significant positive correlation with the α-diversity of bacterial and archaeal communities. This is reasonable because coastal sediment microorganisms generally adapt to the saline environments, thus promoting the microbial α-diversity and activity (Morrissey et al., 2014). Low salinity sediments may provide appropriate mineral elements for microbial growth and promote their biodiversity (Jia et al., 2020). Furthermore, the increased relative abundances of keystone OTUs could contribute to the α-diversity of bacterial and archaeal communities. The microbial keystones can adapt to osmotic stress in marine sediment, resulting in a high α-diversity in coastal sediments. For example, three of four archaeal keystone OTUs were from Bathyarchaeota (Meng et al., 2014), which was mainly distributed in marine environments and involved in protein and cellulose degradation (Lloyd et al., 2013; Lazar et al., 2016; Baker et al., 2020), carbon dioxide fixation (McLeod et al., 2011) and methanogenesis (Evans et al., 2015; McKay et al., 2017). Therefore, sediment salinity may drive the prokaryotic community assembly via affecting the microbial diversity in coastal wetland.
Increasing intensity of human activities might dramatically affect the structure and function of microbial communities (Zhang et al., 2016; Huang et al., 2020). External agricultural inputs such as animal feces, and mineral fertilizers might subsequently be transferred to rivers, coastal wetland and sediments (McIsaac and Hu, 2004; Zhang et al., 2016). Thus, microbial communities in coastal wetland sediments would shift their structure and ecological function under the effects of long-term human activity (Huang et al., 2020). In this study, the abundance of Methanosaeta and Candidatus Methanoregula decreased from offshore sites to inshore sites due to that methanogens may be affected by local agricultural inputs (Long et al., 2021). Although we did not assess the microbial response to human activities, the variation of prokaryotic diversity and the number of keystone taxa in inshore sites indicated prokaryotic communities might be more vulnerable to anthropogenic activities. Collectively, the changes in the traits of prokaryotic communities in coastal wetlands should be due to the comprehensive effect of environmental factors and human activities.
In summary, this study illustrated that sediments pH and salinity can drive the distribution patterns and assembly processes of prokaryotic communities in the coastal wetland ecosystem. These results indicated that sediment pH and salinity were key environmental factors to jointly control the assembly processes of bacterial and archaeal communities via influencing their diversity, composition, co-occurrence networks patterns and keystone taxa. We also found that the assembly of bacterial and archaeal community was mainly driven by homogeneous and heterogeneous selection, while the deterministic processes became weaker from offshore sites to inshore sites due to the decrease of pH and salinity. This study improves our understanding of the prokaryotic community assembly in coastal wetland sediments.
The datasets presented in this study can be found in online repositories. The names of the repository/repositories and accession number(s) can be found below: NCBI (accession: PRJNA666027 and PRJNA666234).
HY: conceptualization, investigation, data curation, and writing – review and editing. QZ: conceptualization, investigation, data curation, and writing – original draft. YP: conceptualization, methodology, and writing – review and editing. XZ and FX: methodology, data curation, and writing – review and editing. BW and XY: methodology and writing – review and editing. ZL: investigation and writing – review and editing. LS: writing – review and editing. CW and QY: conceptualization and writing – review and editing. ZH: conceptualization, supervision, funding acquisition, and writing – review and editing. All authors: contributed to the article and approved the submitted version.
This study was supported by the National Natural Science Foundation of China (32001178, 91951207, 31770539, 31870469, 32000070, and 52070196), the Innovation Group Project of Southern Marine Science and Engineering Guangdong Laboratory (Zhuhai) (311021006), the Southern Marine Science and Engineering Guangdong Laboratory (Zhuhai) (SML2020SP0004), the China Postdoctoral Science Foundation (2018M643296), and the project from Sun Yat-sen University (38000-42150006).
The authors declare that the research was conducted in the absence of any commercial or financial relationships that could be construed as a potential conflict of interest.
All claims expressed in this article are solely those of the authors and do not necessarily represent those of their affiliated organizations, or those of the publisher, the editors and the reviewers. Any product that may be evaluated in this article, or claim that may be made by its manufacturer, is not guaranteed or endorsed by the publisher.
The Supplementary Material for this article can be found online at: https://www.frontiersin.org/articles/10.3389/fmars.2021.792294/full#supplementary-material
Aguilar, P., and Sommaruga, R. (2020). The balance between deterministic and stochastic processes in structuring lake bacterioplankton community over time. Mol. Ecol. 29, 3117–3130. doi: 10.1111/mec.15538
Alongi, D. M. (2014). Carbon cycling and storage in mangrove forests. Ann. Rev. Mar. Sci. 6, 195–219. doi: 10.1146/annurev-marine-010213-135020
Baker, B. J., De Anda, V., Seitz, K. W., Dombrowski, N., Santoro, A. E., and Lloyd, K. G. (2020). Diversity, ecology and evolution of Archaea. Nat. Microbiol. 5, 887–900. doi: 10.1038/s41564-020-0715-z
Banerjee, S., Schlaeppi, K., and van der Heijden, M. G. A. (2018). Keystone taxa as drivers of microbiome structure and functioning. Nat. Rev. Microbiol. 16, 567–576. doi: 10.1038/s41579-018-0024-1
Bastian, M., Heymann, S., and Jacomy, M. (2009). “Gephi: an open source software for exploring and manipulating networks,” in Proceedings of the 3rd International AAAI Conference on Weblogs and Social Media, Menlo Park, CA.
Behera, P., Mohapatra, M., Kim, J. Y., and Rastogi, G. (2020). Benthic archaeal community structure and carbon metabolic profiling of heterotrophic microbial communities in brackish sediments. Sci. Total Environ. 706:135709. doi: 10.1016/j.scitotenv.2019.135709
Berry, D., and Widder, S. (2014). Deciphering microbial interactions and detecting keystone species with co-occurrence networks. Front. Microbiol. 5:219. doi: 10.3389/fmicb.2014.00219
Bolger, A., Lohse, M., and Usadel, B. (2014). Trimmomatic: a flexible trimmer for Illumina sequence data. Bioinformatics 30, 2114–2120. doi: 10.1093/bioinformatics/btu170
Burns, A. R., Stephens, W. Z., Stagaman, K., Wong, S., Rawls, J. F., Guillemin, K., et al. (2016). Contribution of neutral processes to the assembly of gut microbial communities in the zebrafish over host development. ISME J. 10, 655–664. doi: 10.1038/ismej.2015.142
Byrne, B. M. (2016). Structural Equation Modeling With Amos: Basic Concepts, Applications, and Programming, 3rd Edn. Milton Park: Taylor & Francis.
Campbell, B. J., and Kirchman, D. L. (2013). Bacterial diversity, community structure and potential growth rates along an estuarine salinity gradient. ISME J. 7, 210–220. doi: 10.1038/ismej.2012.93
Cermeno, P., and Falkowski, P. G. (2009). Controls on diatom biogeography in the ocean. Science 325, 1539–1541. doi: 10.1126/science.1174159
Chase, J. M. (2010). Stochastic community assembly causes higher biodiversity in more productive environments. Science 328, 1388–1391. doi: 10.1126/science.1187820
Chave, J. (2004). Neutral theory and community ecology. Ecol. Lett. 7, 241–253. doi: 10.1111/j.1461-0248.2003.00566.x
Chen, L. J., Jiang, Y. J., Liang, C., Luo, Y., Xu, Q. S., Han, C., et al. (2019). Competitive interaction with keystone taxa induced negative priming under biochar amendments. Microbiome 7, 1–18. doi: 10.1186/s40168-019-0693-7
Chesson, P. (2000). Mechanisms of maintenance of species diversity. Annu. Rev. Ecol. Evol. 31, 343–366. doi: 10.1146/annurev.ecolsys.31.1.343
Deng, Y., Jiang, Y. H., Yang, Y., He, Z., Luo, F., and Zhou, J. Z. (2012). Molecular ecological network analyses. BMC Bioinformatics 13:113. doi: 10.1186/1471-2105-13-113
Dini-Andreote, F., Stegen, J. C., van Elsas, J. D., and Salles, J. F. (2015). Disentangling mechanisms that mediate the balance between stochastic and deterministic processes in microbial succession. Proc. Natl. Acad. Sci. U.S.A. 112, E1326–E1332. doi: 10.1073/pnas.1414261112
Donato, D. C., Kauffman, J. B., Murdiyarso, D., Kurnianto, S., Stidham, M., and Kanninen, M. (2011). Mangroves among the most carbon-rich forests in the tropics. Nat. Geosci. 4, 293–297. doi: 10.1038/NGEO1123
Edgar, R. C. (2013). UPARSE: highly accurate OTU sequences from microbial amplicon reads. Nat. Methods 10, 996–998. doi: 10.1038/NMETH.2604
Eisenhauer, N., Bowker, M. A., Grace, J. B., and Powell, J. R. (2015). From patterns to causal understanding: structural equation modeling (SEM) in soil ecology. Pedobiologia 58, 65–72. doi: 10.1016/j.pedobi.2015.03.002
Evans, P. N., Parks, D. H., Chadwick, G. L., Robbins, S. J., Orphan, V. J., Golding, S. D., et al. (2015). Methane metabolism in the archaeal phylum Bathyarchaeota revealed by genome-centric metagenomics. Science 350, 434–438. doi: 10.1126/science.aac7745
Fargione, J., Brown, C. S., and Tilman, D. (2003). Community assembly and invasion: an experimental test of neutral versus niche processes. Proc. Natl. Acad. Sci. U.S.A. 100, 8916–8920. doi: 10.1073/pnas.1033107100
Fierer, N. (2017). Embracing the unknown: disentangling the complexities of the soil microbiome. Nat. Rev. Microbiol. 15, 579–590. doi: 10.1038/nrmicro.2017.87
Fierer, N., and Jackson, R. B. (2006). The diversity and biogeography of soil bacterial communities. Proc. Natl. Acad. Sci. U.S.A. 103, 626–631. doi: 10.1073/pnas.0507535103
Frank, K. L., Rogers, D. R., Olins, H. C., Vidoudez, C., and Girguis, P. R. (2013). Characterizing the distribution and rates of microbial sulfate reduction at middle valley hydrothermal vents. ISME J. 7, 1391–1401. doi: 10.1038/ismej.2013.17
Gabler, C. A., Osland, M. J., Grace, J. B., Stagg, C. L., Day, R. H., Hartley, S. B., et al. (2017). Macroclimatic change expected to transform coastal wetland ecosystems this century. Nat. Clim. Change 7, 142–147. doi: 10.1038/NCLIMATE3203
Grace, J. B., Schoolmaster, D. R., Guntenspergen, G. R., Little, A. M., Mitchell, B. R., Miller, K. M., et al. (2012). Guidelines for a graph-theoretic implementation of structural equation modeling. Ecosphere 3, 1–44. doi: 10.1890/ES12-00048.1
Griffiths, R. I., Thomson, B. C., James, P., Bell, T., Bailey, M., and Whiteley, A. S. (2011). The bacterial biogeography of British soils. Environ. Microbiol. 13, 1642–1654. doi: 10.1111/j.1462-2920.2011.02480.x
Guimera, R., and Amaral, L. A. N. (2005). Functional cartography of complex metabolic networks. Nature 433, 895–900. doi: 10.1038/nature03288
Herren, C. M., and McMahon, K. D. (2018). Keystone taxa predict compositional change in microbial communities. Environ. Microbiol. 20, 2207–2217. doi: 10.1111/1462-2920.14257
Hong, Y., Wu, J., Guan, F., Guan, F. J., Yue, W. Z., and Long, A. M. (2019). Nitrogen removal in the sediments of the Pearl River estuary, China: evidence from the distribution and forms of nitrogen in the sediment cores. Mar. Pollut. Bull. 138, 115–124. doi: 10.1016/j.marpolbul.2018.11.040
Hu, Y., Wang, L., Tang, Y., Li, Y. L., Chen, J. H., Xi, X. F., et al. (2014). Variability in soil microbial community and activity between coastal and riparian wetlands in the Yangtze River estuary–potential impacts on carbon sequestration. Soil Biol. Biochem. 70, 221–228. doi: 10.1016/j.soilbio.2013.12.025
Huang, L., Bai, J., Wen, X., Zhang, G. L., Zhang, C. D., Cui, B. S., et al. (2020). Microbial resistance and resilience in response to environmental changes under the higher intensity of human activities than global average level. Glob. Change Biol. 26, 2377–2389. doi: 10.1111/gcb.14995
Jia, Y., Niu, C. T., Lu, Z. M., Zhang, X. J., Chai, L. Y., Shi, J. S., et al. (2020). A bottom-up approach to develop simplified microbial community model with desired functions: application for efficient fermentation of broad bean paste with low salinity. Appl. Environ. Microb. 86:e00306-20. doi: 10.1128/AEM.00306-20
Jiao, S., and Lu, Y. (2020a). Abundant fungi adapt to broader environmental gradients than rare fungi in agricultural fields. Glob. Chang. Biol. 26, 4506–4520. doi: 10.1111/gcb.15130
Jiao, S., and Lu, Y. (2020b). Soil pH and temperature regulate assembly processes of abundant and rare bacterial communities in agricultural ecosystems. Environ. Microbiol. 22, 1052–1065. doi: 10.1111/1462-2920.14815
Jiao, S., Xu, Y., Zhang, J., and Lu, Y. (2019). Environmental filtering drives distinct continental atlases of soil archaea between dryland and wetland agricultural ecosystems. Microbiome 7, 1–13. doi: 10.1186/s40168-019-0630-9
Jiao, S., Yang, Y., Xu, Y., Zhang, J., and Lu, Y. (2020). Balance between community assembly processes mediates species coexistence in agricultural soil microbiomes across eastern China. ISME J. 14, 202–216. doi: 10.1038/s41396-019-0522-9
Langenheder, S., and Lindstrom, E. S. (2019). Factors influencing aquatic and terrestrial bacterial community assembly. Environ. Microbiol. Rep. 11, 306–315. doi: 10.1111/1758-2229.12731
Lauber, C. L., Hamady, M., Knight, R., and Fierer, N. (2009). Pyrosequencing-based assessment of soil pH as a predictor of soil bacterial community structure at the continental scale. Appl. Environ. Microbiol. 75, 5111–5120. doi: 10.1128/AEM.00335-09
Lazar, C. S., Baker, B. J., Seitz, K., Hyde, A. S., Dick, G. J., Hinrichs, K. U., et al. (2016). Genomic evidence for distinct carbon substrate preferences and ecological niches of Bathyarchaeota in estuarine sediments. Environ. Microbiol. 18, 1200–1211. doi: 10.1111/1462-2920.13142
Leff, J. W., Jones, S. E., Prober, S. M., Barberan, A., Borer, E. T., Firn, J. L., et al. (2015). consistent responses of soil microbial communities to elevated nutrient inputs in grasslands across the globe. Proc. Natl. Acad. Sci. U.S.A. 112, 10967–10972. doi: 10.1073/pnas.1508382112
Leibold, M. A., Holyoak, M., Mouquet, N., Amarasekare, P., Chase, J. M., Hoopes, M. F., et al. (2004). The community concept: a framework for multi-scale community ecology. Ecol. Lett. 7, 601–613. doi: 10.1111/j.1461-0248.2004.00608.x
Li, M., Zhang, J., Yang, X., Zhou, Y., Zhang, L., and Yang, Y. (2021). Responses of ammonia-oxidizing microorganisms to biochar and compost amendments of heavy metals-polluted soil. J. Environ. Sci. 102, 263–272. doi: 10.1016/j.jes.2020.09.029
Li, S. P., Wang, P., Chen, Y., Wilson, M. C., Yang, X., Ma, C., et al. (2020). Island biogeography of soil bacteria and fungi: similar patterns, but different mechanisms. ISME J. 14, 1886–1896. doi: 10.1038/s41396-020-0657-8
Li, W., Guan, W., Chen, H., Liao, B., Hu, J., Peng, C., et al. (2016). Archaeal communities in the sediments of different mangrove stands at Dongzhaigang, China. J. Soil Sediment 16, 1995–2004. doi: 10.1007/s11368-016-1427-0
Lin, X., Hetharua, B., Lin, L., Xu, H., Zheng, T., He, Z., et al. (2019). Mangrove sediment microbiome: adaptive microbial assemblages and their routed biogeochemical processes in Yunxiao mangrove national nature reserve, China. Microb. Ecol. 78, 57–69. doi: 10.1007/s00248-018-1261-6
Liu, J., Zhu, S., Liu, X., Yao, P., Ge, T., and Zhang, X. H. (2020). Spatiotemporal dynamics of the archaeal community in coastal sediments: assembly process and co-occurrence relationship. ISME J. 14, 1463–1478. doi: 10.1038/s41396-020-0621-7
Liu, X., Yang, C., Yu, X., Yu, H., Zhuang, W., Gu, H., et al. (2020). Revealing structure and assembly for rhizophyte-endophyte diazotrophic community in mangrove ecosystem after introduced Sonneratia apetala and Laguncularia racemosa. Sci. Total Environ. 721:137807. doi: 10.1016/j.scitotenv.2020.137807
Lloyd, K. G., Schreiber, L., Petersen, D. G., Kjeldsen, K. U., Lever, M. A., Steen, A. D., et al. (2013). Predominant archaea in marine sediments degrade detrital proteins. Nature 496, 215–218. doi: 10.1038/nature12033
Logares, R., Tesson, S. V. M., Canback, B., Pontarp, M., Hedlund, K., and Rengefors, K. (2018). Contrasting prevalence of selection and drift in the community structuring of bacteria and microbial eukaryotes. Environ. Microbiol. 20, 2231–2240. doi: 10.1111/1462-2920.14265
Long, Y., Jiang, J., Hu, X., Hu, J., Ren, C. J., and Zhou, S. Q. (2021). The response of microbial community structure and sediment properties to anthropogenic activities in Caohai wetland sediments. Ecotoxicol. Environ. Safe. 211:111936. doi: 10.1016/j.ecoenv.2021.111936
Ma, B., Dai, Z., Wang, H., Dsouza, M., Liu, X., He, Y., et al. (2017). Distinct biogeographic patterns for archaea, bacteria, and fungi along the vegetation gradient at the continental scale in eastern China. mSystems 2:e00174-16. doi: 10.1128/mSystems.00174-16
Ma, B., Wang, Y., Ye, S., Liu, S., Stirling, E., Gilbert, J. A., et al. (2020). Earth microbial co-occurrence network reveals interconnection pattern across microbiomes. Microbiome 8, 1–12. doi: 10.1186/s40168-020-00857-2
Mamet, S. D., Redlick, E., Brabant, M., Lamb, E. G., Helgason, B. L., Stanley, K., et al. (2019). Structural equation modeling of a winnowed soil microbiome identifies how invasive plants re-structure microbial networks. ISME J. 13, 1988–1996. doi: 10.1038/s41396-019-0407-y
Martin, M. J. (2011). Cutadapt removes adapter sequences from high-throughput sequencing reads. EMBnet. J. 17, 10–12. doi: 10.14806/ej.17.1.200
McIsaac, G. F., and Hu, X. (2004). Net N input and riverine N export from Illinois agricultural watersheds with and without extensive tile drainage. Biogeochemistry 70, 253–273.
McKay, L. J., Hatzenpichler, R., Inskeep, W. P., and Fields, M. W. (2017). Occurrence and expression of novel methyl-coenzyme M reductase gene (mcrA) variants in hot spring sediments. Sci. Rep. 7:7252. doi: 10.1038/s41598-017-07354-x
McLeod, E., Chmura, G. L., Bouillon, S., Salm, R., Björk, M., Duarte, C. M., et al. (2011). A blueprint for blue carbon: toward an improved understanding of the role of vegetated coastal habitats in sequestering CO2. Front. Ecol. Environ. 9:552–560. doi: 10.1890/110004
Meng, J., Xu, J., Qin, D., He, Y., Xiao, X., and Wang, F. (2014). Genetic and functional properties of uncultivated MCG archaea assessed by metagenome and gene expression analyses. ISME J. 8, 650–659. doi: 10.1038/ismej.2013.174
Mo, Y., Zhang, W., Yang, J., Lin, Y., Yu, Z., and Lin, S. (2018). Biogeographic patterns of abundant and rare bacterioplankton in three subtropical bays resulting from selective and neutral processes. ISME J. 12, 2198–2210. doi: 10.1038/s41396-018-0153-6
Morrissey, E. M., Gillespie, J. L., Morina, J. C., and Franklin, R. B. (2014). Salinity affects microbial activity and soil organic matter content in tidal wetlands. Glob. Change Biol. 20, 1351–1362. doi: 10.1111/gcb.12431
Mouquet, N., and Loreau, M. (2003). Community patterns in source-sink metacommunities. Am. Nat. 162, 544–557. doi: 10.1086/378857
Nemergut, D. R., Schmidt, S. K., Fukami, T., O’Neill, S. P., Bilinski, T. M., Stanish, L., et al. (2013). Patterns and processes of microbial community assembly. Microbiol. Mol. Biol. Rev. 77, 342–356. doi: 10.1128/MMBR.00051-12
Ning, D., Yuan, M., Wu, L., Zhang, Y., Guo, X., Zhou, X., et al. (2020). A quantitative framework reveals ecological drivers of grassland microbial community assembly in response to warming. Nat. Commun. 11:4717. doi: 10.1038/s41467-020-18560-z
Oren, A. (2011). Thermodynamic limits to microbial life at high salt concentrations. Environ. Microbiol. 13, 1908–1923. doi: 10.1111/j.1462-2920.2010.02365.x
Rath, K. M., Fierer, N., Murphy, D. V., and Rousk, J. (2019). Linking bacterial community composition to soil salinity along environmental gradients. ISME J. 13, 836–846. doi: 10.1038/s41396-018-0313-8
Rath, K. M., and Rousk, J. (2015). Salt effects on the soil microbial decomposer community and their role in organic carbon cycling: a review. Soil Biol. Biochem. 81, 108–123. doi: 10.1016/j.soilbio.2014.11.001
Ren, L., Jeppesen, E., He, D., Wang, J., Liboriussen, L., Xing, P., et al. (2015). pH influences the importance of niche-related and neutral processes in lacustrine bacterioplankton assembly. Appl. Environ. Microbiol. 81, 3104–3114. doi: 10.1128/AEM.04042-14
Roguet, A., Laigle, G. S., Therial, C., Bressy, A., Soulignac, F., Catherine, A., et al. (2015). Neutral community model explains the bacterial community assembly in freshwater lakes. FEMS Microbiol. Ecol. 91:fiv125. doi: 10.1093/femsec/fiv125
Rosindell, J., Hubbell, S. P., and Etienne, R. S. (2011). The unified neutral theory of biodiversity and biogeography at age ten. Trends Ecol. Evol. 26, 340–348. doi: 10.1016/j.tree.2011.03.024
Rousk, J., Baath, E., Brookes, P. C., Lauber, C. L., Lozupone, C., Caporaso, J. G., et al. (2010). Soil bacterial and fungal communities across a pH gradient in an arable soil. ISME J. 4, 1340–1351. doi: 10.1038/ismej.2010.58
Stegen, J. C., Lin, X., Fredrickson, J. K., Chen, X., Kennedy, D. W., Murray, C. J., et al. (2013). Quantifying community assembly processes and identifying features that impose them. ISME J. 7, 2069–2079. doi: 10.1038/ismej.2013.93
Stegen, J. C., Lin, X., Fredrickson, J. K., and Konopka, A. E. (2015). Estimating and mapping ecological processes influencing microbial community assembly. Front. Microbiol. 6:370. doi: 10.3389/fmicb.2015.00370
Sun, H., Jiang, J., Cui, L., Feng, W. T., Wang, Y. G., and Zhang, J. C. (2019). Soil organic carbon stabilization mechanisms in a subtropical mangrove and salt marsh ecosystems. Sci. Total. Environ. 673, 502–510. doi: 10.1016/j.scitotenv.2019.04.122
Tripathi, B. M., Kim, M., Singh, D., Lee-Cruz, L., Lai-Hoe, A., Ainuddin, A. N., et al. (2012). Tropical soil bacterial communities in Malaysia: pH dominates in the equatorial tropics too. Microb. Ecol. 64, 474–484. doi: 10.1007/s00248-012-0028-8
Tripathi, B. M., Lee-Cruz, L., Kim, M., Singh, D., Go, R., Shukor, N. A. A., et al. (2014). Spatial scaling effects on soil bacterial communities in malaysian tropical forests. Microb. Ecol. 68, 247–258. doi: 10.1007/s00248-014-0404-7
Tripathi, B. M., Stegen, J. C., Kim, M., Dong, K., Adams, J. M., and Lee, Y. K. (2018). Soil pH mediates the balance between stochastic and deterministic assembly of bacteria. ISME J. 12, 1072–1083. doi: 10.1038/s41396-018-0082-4
Vavourakis, C. D., Ghai, R., Rodriguez-Valera, F., Sorokin, D. Y., Tringe, S. G., and Hugenholtz, P. (2016). Metagenomic insights into the uncultured diversity and physiology of microbes in four hypersaline soda lake brines. Front. Microbiol. 7:211. doi: 10.3389/fmicb.2016.00211
Ventosa, A., Beatriz Fernandez, A., Jose Leon, M., Sanchez-Porro, C., and Rodriguez-Valera, F. (2014). The Santa Pola saltern as a model for studying the microbiota of hypersaline environments. Extremophiles 18, 811–824. doi: 10.1007/s00792-014-0681-6
Wang, B., Zheng, X., Zhang, H., Xiao, F., He, Z., and Yan, Q. (2020). Keystone taxa of water microbiome respond to environmental quality and predict water contamination. Environ. Res. 187:109666. doi: 10.1016/j.envres.2020.109666
Wang, J., Cai, C., Li, Y., Hua, M., Wang, J., Yang, H., et al. (2019). Denitrifying anaerobic methane oxidation: a previously overlooked methane sink in intertidal zone. Environ. Sci. Technol. 53, 203–212. doi: 10.1021/acs.est.8b05742
Wang, Q., Garrity, G. M., Tiedje, J. M., and Cole, J. R. (2007). Naive bayesian classifier for rapid assignment of rRNA sequences into the new bacterial taxonomy. Appl. Environ. Microbiol. 73, 5261–5267. doi: 10.1128/AEM.00062-07
Wang, Y., Wang, K., Huang, L., Dong, P., Wang, S., Chen, H., et al. (2020). Fine-scale succession patterns and assembly mechanisms of bacterial community of Litopenaeus vannamei larvae across the developmental cycle. Microbiome 8, 1–16. doi: 10.1186/s40168-020-00879-w
Wei, G., Li, M., Shi, W., Tian, R., Chang, C., Wang, Z., et al. (2020). Similar drivers but different effects lead to distinct ecological patterns of soil bacterial and archaeal communities. Soil Biol. Biochem. 144:107759. doi: 10.1016/j.soilbio.2020.107759
Weiss, S., Van Treuren, W., Lozupone, C., Faust, K., Friedman, J., Deng, Y., et al. (2016). Correlation detection strategies in microbial data sets vary widely in sensitivity and precision. ISME J. 10, 1669–1681. doi: 10.1038/ismej.2015.235
Wu, Z., Ren, D., Zhou, H., Gao, H., and Li, J. (2017). Sulfate reduction and formation of iron sulfide minerals in nearshore sediments from Qi’ao Island, Pearl River Estuary, Southern China. Quat. Int. 452, 137–147. doi: 10.1016/j.quaint.2016.06.003
Xia, S., Wang, W., Song, Z., Kuzyakov, Y., Guo, L. D., Zwieten, L. V., et al. (2021). Spartina alterniflora invasion controls organic carbon stocks in coastal marsh and mangrove soils across tropics and subtropics. Global Change Biol. 27, 1627–1644. doi: 10.1111/gcb.15516
Yan, Q., Stegen, J. C., Yu, Y., Deng, Y., Li, X., Wu, S., et al. (2017). Nearly a decade-long repeatable seasonal diversity patterns of bacterioplankton communities in the eutrophic Lake Donghu (Wuhan, China). Mol. Ecol. 26, 3839–3850. doi: 10.1111/mec.14151
Yang, W., Yan, Y., Jiang, F., Leng, X., Cheng, X. L., and An, S. Q. (2016). Response of the soil microbial community composition and biomass to a short-term Spartina alterniflora invasion in a coastal wetland of eastern China. Plant Soil 408, 443–456. doi: 10.1007/s11104-016-2941-y
Yu, H., Liu, X. Y., Yang, C., Peng, Y. S., Yu, X. L., Gu, H., et al. (2021a). Co-symbiosis of arbuscular mycorrhizal fungi (AMF) and diazotrophs promote biological nitrogen fixation in mangrove ecosystems. Soil Biol. Biochem. 161:108382. doi: 10.1016/j.soilbio.2021.108382
Yu, H., Zheng, X. F., Weng, W. L., Yan, X. Z., Chen, P. B., Liu, X. Y., et al. (2021b). Synergistic effects of antimony and arsenic contaminations on bacterial, archaeal and fungal communities in the rhizosphere of Miscanthus sinensis: insights for nitrification and carbon mineralization. J. Hazard Mater. 411:125094. doi: 10.1016/j.jhazmat.2021.125094
Yu, X., Yang, X., Wu, Y., Peng, Y., Yang, T., Xiao, F., et al. (2020). Sonneratia apetala introduction alters methane cycling microbial communities and increases methane emissions in mangrove ecosystems. Soil Biol. Biochem. 144:107775. doi: 10.1016/j.soilbio.2020.107775
Zhang, C. J., Pan, J., Duan, C. H., Wang, Y. M., Liu, Y., Sun, J., et al. (2019). Prokaryotic diversity in mangrove sediments across southeastern China fundamentally differs from that in other biomes. mSystems 4:e00442-19. doi: 10.1128/mSystems.00442-19
Zhang, C. J., Pan, J., Liu, Y., Duan, C. H., and Li, M. (2020). Genomic and transcriptomic insights into methanogenesis potential of novel methanogens from mangrove sediments. Microbiome 8:94. doi: 10.1186/s40168-020-00876-z
Zhang, K., Shi, Y., Cui, X., Yue, P., Li, K., Liu, X., et al. (2019). Salinity is a key determinant for soil microbial communities in a desert ecosystem. mSystems 4:e00225-18. doi: 10.1128/mSystems.00225-18
Zhang, X., Gu, Q., Long, X. E., Zhao, L. L., Liu, D. X., Ye, D. H., et al. (2016). Anthropogenic activities drive the microbial community and its function in urban river sediment. J. Soil. Sediments 16, 716–725. doi: 10.1007/s11368-015-1246-8
Zhong, Q., Chen, H., Liu, L., He, Y., Zhu, D., Jiang, L., et al. (2017). Water table drawdown shapes the depth-dependent variations in prokaryotic diversity and structure in Zoige peatlands. FEMS Microbiol. Ecol. 93:fix049. doi: 10.1093/femsec/fix049
Zhong, Q., Xue, D., Chen, H., Liu, L., He, Y., Zhu, D., et al. (2020). Structure and distribution of nitrite-dependent anaerobic methane oxidation bacteria vary with water tables in Zoige peatlands. FEMS Microbiol. Ecol. 96:fiaa039. doi: 10.1093/femsec/fiaa039
Zhong, Z., Liu, Y., Miao, L., Wang, F., Chu, L., Wang, J., et al. (2016). Prokaryotic community structure driven by salinity and ionic concentrations in plateau lakes of the Tibetan Plateau. Appl. Environ. Microbiol. 82, 1846–1858. doi: 10.1128/AEM.03332-15
Zhou, J., Bruns, M. A., and Tiedje, J. M. (1996). DNA recovery from soils of diverse composition. Appl. Environ. Microbiol. 62, 316–322. doi: 10.1128/AEM.62.2.316-322.1996
Zhou, J., Deng, Y., Zhang, P., Xue, K., Liang, Y., Van Nostrand, J. D., et al. (2014). Stochasticity, succession, and environmental perturbations in a fluidic ecosystem. Proc. Natl. Acad. Sci. U.S.A. 111, E836–E845. doi: 10.1073/pnas.1324044111
Keywords: microbial community assembly, deterministic processes, environmental filtering, prokaryotic communities, coastal wetland
Citation: Yu H, Zhong Q, Peng Y, Zheng X, Xiao F, Wu B, Yu X, Luo Z, Shu L, Wang C, Yan Q and He Z (2022) Environmental Filtering by pH and Salinity Jointly Drives Prokaryotic Community Assembly in Coastal Wetland Sediments. Front. Mar. Sci. 8:792294. doi: 10.3389/fmars.2021.792294
Received: 10 October 2021; Accepted: 07 December 2021;
Published: 12 January 2022.
Edited by:
Nicoletta Leonardi, University of Liverpool, United KingdomReviewed by:
Wenjin Hao, Nantong University, ChinaCopyright © 2022 Yu, Zhong, Peng, Zheng, Xiao, Wu, Yu, Luo, Shu, Wang, Yan and He. This is an open-access article distributed under the terms of the Creative Commons Attribution License (CC BY). The use, distribution or reproduction in other forums is permitted, provided the original author(s) and the copyright owner(s) are credited and that the original publication in this journal is cited, in accordance with accepted academic practice. No use, distribution or reproduction is permitted which does not comply with these terms.
*Correspondence: Qingyun Yan, eWFucXk2QG1haWwuc3lzdS5lZHUuY24=; Zhili He, aGV6aGlsaUBtYWlsLnN5c3UuZWR1LmNu
†These authors share first authorship
Disclaimer: All claims expressed in this article are solely those of the authors and do not necessarily represent those of their affiliated organizations, or those of the publisher, the editors and the reviewers. Any product that may be evaluated in this article or claim that may be made by its manufacturer is not guaranteed or endorsed by the publisher.
Research integrity at Frontiers
Learn more about the work of our research integrity team to safeguard the quality of each article we publish.