- Third Institute of Oceanography, Ministry of Natural Resources, Xiamen, China
The Antarctic krill (Euphausia superba) fishery is the largest fishery in the Southern Ocean, and it has been operating for over 45 years. In the past decade, the spatial distribution of the krill fishery has focused on the Bransfield Strait off the Antarctic Peninsula (subarea 48.1). However, the high fishing effort and climate change have placed great pressure on krill resources in this region, and conservation concerns have been raised. Because aging krill is difficult and uncertain, we developed an integrated size-structured model to estimate the fishing mortality, recruitment and spawning biomass of krill. The results indicated that in 1992–2011, the average spawning biomass of krill ranged from 1.14 × 106 to 1.45 × 106 tons, the estimated biomass of the maximum sustainable yield (BMSY) ranged from 3.96 × 105 to 4.90 × 105 tons and the estimated average recruitment ranged from 2.3 × 1012 to 5.03 × 1012 individuals in the research area. We explored the effect of different data weighting schemes for the length data on the assessment output. However, our estimates also have uncertainties. In addition, an attempt was made to analyze the correlation between model-estimated recruitment and mature biomass with climate change. Future stock assessments of krill resources should be comprehensive and multimethod, and the management of krill resources should be based on science that considers the demand of fishing communities and ecosystem protection.
Introduction
The American naturalist James Dwight Dana gave an initial description of the Antarctic krill (Euphausia superba) in 1850. Antarctic krill are marine crustaceans with a circumpolar distribution (Hofmann and Hüsrevoğlu, 2003; Siegel, 2005; Atkinson et al., 2009); they often congregate in different forms (path, shoal, swarm, and school) on the edges of continental shelves, near sea ice and around islands and exhibit diurnal vertical movement behavior (Hardy, 1936). Generally, krill are considered to live for 5–7 years, the spawning population is 2–3 years old, and the adult body length is 40–65 mm (Ikeda, 1985). However, due to the biological characteristics of molting and shortening body length when krill face adverse environments, determining their longevity is an unsolved and difficult scientific problem. Antarctic krill is one of the largest single-species resources in the world, with a very large biomass of 300–500 million tons (Nicol and Foster, 2003; Kawaguchi and Nicol, 2007; Nicol et al., 2012). Antarctic krill play a very important role in the Southern Ocean ecosystem, mainly because it is the main prey of many marine predators (penguins, seals, whales and fish), and it is also an important herbivore of autotrophic and heterotrophic plankton undertaking high and low trophic levels in the food chain (Howard, 1989; Voronina, 1998). The overall change in Antarctic krill resources will directly affect the resource status of other marine organisms in the Southern Ocean and play a key role in biogeochemical cycles such as carbon exports and the iron cycle. In addition, the Antarctic krill fishery is one of the key fisheries in the Southern Ocean, has high economic value and is of concern to many countries.
Antarctic krill has been fished commercially in the Southern Ocean since the 1970s, and the Antarctic krill fishery has consistently been the largest fishery, by tonnage, in the region (Budzinksi et al., 1985; Nicol and Foster, 2003). In 1990s, due to the problems of changing krill fishing technologies and the development of the main fishing countries, the catch of krill gradually decreased. With the development of krill fisheries in new countries, the catch of krill has increased in recent years.
As 70% of the Antarctic krill resources are concentrated in the southwest Atlantic region of the Southern Ocean, the northwest Antarctic Peninsula is an important fishing ground and an important active area for krill fishing. In recent decades, the krill fishery has experienced a series of spatiotemporal changes. In the 1990s and early 21st century, the krill fishery near Elephant Island and the South Shetland Islands began to expand to the south of the center of the Bransfield Strait, and a fishing hot spot was established (Kawaguchi et al., 2009). During 2009–2014, 65–90% of the seasonal fishing came from this fishing hot spot. Other studies show that the catches in subarea 48.1 are now more concentrated in space and time than ever before (Trathan et al., 2018; Kawaguchi and Nicol, 2020; Meyer et al., 2020). The demand for krill will likely increase, driven by at least two industries (marine products and nutraceutical products).
The southwest Atlantic is not only the area where the Antarctic krill fishery is concentrated but also the key ecological area of the krill population, especially in the northern Antarctic Peninsula and Bransfield Strait (subarea 48.1 is included in this area), which is the main spawning area, breeding area and wintering area of krill (Spiridonov, 1995; Siegel et al., 2004, 2013; Piñones and Fedorov, 2016; Perry et al., 2019). Therefore, based on the information from the fishery and ecology, it can be seen that the Bransfield Strait region in the northern part of the Antarctic Peninsula is an important area (it can be considered a “key area”) where Antarctic krill fishery resources overlap with important processes in its life cycle.
At present, the key area described above is one of the sea areas significantly affected by global climate change. Due to the rapid change in global climate and the reduction in sea ice coverage, the fishing time for Antarctic krill in the key area has been prolonged, and the fishing season has changed. Previous research also points out that the average body length of Antarctic krill in the southwest Atlantic Ocean has increased, which indicates that the recruitment of Antarctic krill in the key area tends to decrease (Loeb et al., 1997, 2009; Atkinson et al., 2004, 2019; Trivelpiece et al., 2011; Flores et al., 2012; Saba et al., 2014; Loeb and Santora, 2015; Cox et al., 2019; Hill et al., 2019). With the increase in fishing spatial aggregation and the gradual change in fishing time distribution, as well as the importance of Antarctic krill resources in the Southern Ocean ecosystem, it is very important to manage and protect Antarctic krill resources in the key area.
For the current fishery, the Commission for the Conservation of Antarctic Marine Living Resources (CCAMLR) adopts a fishing quota to manage Antarctic krill resources, and the total catch of Antarctic krill is limited to 620,000 tons per year, and this preventive limit is based on a simulation method using the general yield model (Karlsen et al., 2006). However, with the emergence of new technologies, krill fishing in new member countries and environmental changes, the management system of the Antarctic krill fishery will face new challenges.
The long-term aim of CCAMLR has been the development of a feedback management procedure for the krill fishery that includes adaptive management in which management measures are regularly adjusted based on ecosystem monitoring indices. Implementing this sort of management requires developing an integrated stock assessment (Nicol and de La Mare, 1993; Fabra and Gascon, 2008). At present, for Antarctic krill resources, the main stock assessment method is acoustic. However, the disadvantage of the acoustic assessment method is that there is uncertainty in the parameter setting in the process of analyzing acoustic data, and the acquisition of acoustic data also costs considerable labor and financial resources. The acoustic assessment method can assess only the overall level of resources, and the assessment result cannot consider the life stage of the krill population. Stock assessment methodologies are rapidly developing, but some of these methods are difficult to implement for krill given their biological characteristics. Krill, like many invertebrates, are difficult to age, so age-structured assessments can be difficult to fit to available data sources. One of CCAMLR’s key aims is the development of integrated assessment methodologies that incorporate many different data sources in the assessment (e.g., acoustic surveys and trawl surveys). Kinzey et al. (2015) developed an integrated, age-structured model that incorporated acoustic survey data to estimate the stock status of krill, and key parameters were analyzed. This model from Kinzey et al. (2015) provided a good foundation for the stock assessment of Antarctic krill based on the integrated method. An integrated assessment combines all available data into a single modeling framework, thereby providing more comprehensive estimates of model parameters as well as biological reference points (Maunder and Punt, 2013; Punt et al., 2013). The integrated assessment method can not only effectively use data but also confirm the development direction of krill stock assessment in the future.
Therefore, this study establishes an integrated, size-structured model based on krill fishery and scientific survey data to evaluate the Antarctic krill resource status in the key area and explore the body length composition, recruitment and mature biomass (spawning biomass). A preliminary analysis of the relationship between estimated recruitment, mature biomass and climate variability in the Southern Ocean is also presented. Our goal is to demonstrate how systematically collected data from different sources could be used in an integrated framework and to build an integrated model suitable for krill resources. It is also developed to balance the use of different data sources by means of data weighting, so that the integrated model can have a better prospect of convergence in the future. This research can further develop krill stock assessments to provide advice for the management of this fishery.
Materials and Methods
Research Area
The study area includes the western side of the South Shetland Islands, the Bransfield Strait, and the water between Elephant Island and the Joinville Islands at the northern tip of the Antarctic Peninsula (Figure 1).
From an ecological point of view, the study area includes the main spawning area, breeding area and wintering area of Antarctic krill in the southwest Atlantic Ocean; from the perspective of the krill fishery, the study area contains an important fishing ground for Antarctic krill, subarea 48.1. Especially in recent years, the catch of Antarctic krill in subarea 48.1 has been more concentrated in time and space than ever before.
Data Collection
Survey and Fishery Data
The independent survey data (including survey abundance and length composition data) used in this assessment were collected by the United States Antarctic Living Marine Resources (AMLR) Program of the National Marine Fisheries Service, Southwest Fisheries Science Center (AMLR1). The surveys occurred in the austral summer from 1992 to 2011, the sample data were collected by zooplankton net.
The fishery-dependent data (including length composition data and catch biomass) come from CCAMLR’s public data.2 Catch biomass data are available from 1992 to 2011, but length composition data are available for only 2001–2002 and 2004–2011. Data details is in Table 1.
Climate Index
It has been noted in previous studies that SAM and ENSO affect sea ice and duration around the Antarctic Peninsula and may have an impact on the Antarctic krill recruitment (Loeb et al., 2009; Loeb and Santora, 2015). Therefore, two climate indices, SAM and ENSO, were chosen for this study. SAM describes the north-south motion of the westerly winds around Antarctica, and ESNO deals with the temperature variations in the eastern and central Pacific Ocean that affect the wind and storm patterns around Antarctica.
Two measures of ENSO were used in this research, the Nino 3.4 Index, a measure of sea-surface temperature anomalies in the equatorial Pacific, and the Southern Oscillation Index (SOI), a measure of large-scale air pressure fluctuations that coincide with EI NINO and La Nina events. We obtained SAM data from the British Antarctic Survey’s Ice and Climate Division,3 and obtained the El Niño 3.4 sea-surface temperature and SOI from the NOAA Climate Prediction Center.4
Antarctic Krill Distribution and History
The distribution of krill is circumpolar, and South Georgia, Bird Island, South Orkney Island, Elephant Island, the Bransfield Strait and the Ross Sea have higher biomass than other areas of Antarctica (Hofmann and Hüsrevoğlu, 2003; Siegel, 2005; Atkinson et al., 2009). Krill often congregate in different forms (path, shoal, swarm, and school) on the edge of the continental shelf, near sea ice and around islands and exhibit diurnal vertical movement behavior (Watkins et al., 2000). Krill have obvious vertical movement behavior in the day and night, inhabiting deep water at depths of 200–500 m in the daytime and rising to the surface or upper 0–200 m at night. In the development stage, krill are concentrated in deep water, and they rise to the surface with development. Generally, krill are considered to live for 5–7 years, and the spawning population is 2–3 years old (Ikeda, 1985). At present, researchers usually consider Antarctic krill under 1 year old as a recruitment of Antarctic krill, and krill with body lengths over 35 mm are considered adults, which grow rapidly in summer (Reiss, 2016).
Integrated Size-Structured Stock Assessment Model
Assessment methods should be selected to produce the types of outputs needed for management purposes and to utilize the available data to the maximum extent possible.
For Antarctic krill, aging is difficult. However, size-structured methods are now preferred because they can make full use of size-composition data, are able to integrate multiple sources of data, and produce the types of outputs that are needed for management purposes. An advantage of size-based models over age-based models is that all processes can be size-based, and these processes can modify the (unmodeled) size-at-age distribution (Punt et al., 2013).
We developed an integrated size-structured assessment method, similar to Walters and Post (1993). Our assessment modeled a single population, did not discriminate between sexes, and operated at a yearly time step. Krill were tracked by size and classified into 5 mm length bins. The model included four key parts (Eq. 1):
where Ni is a column vector of length H (number of size classes) containing the numbers-at-length at the start of the time step I; Xi is the size transition matrix for time-step I; Hi is the survival at size during time step I; and Ri+1 is a column vector of the recruitment to each size-class during time step i. The initial numbers of krill in the length bins (N1) are estimated as a parameter vector. In total, 61 parameters were estimated in this assessment. The assessment model was coded in AD Model Builder (Fournier et al., 2012) and estimates quantities that are important for fishery management, such as mature biomass, recruitment, fishing mortality, and selectivity. Maximum likelihood methods were used to estimate all parameters.
The above size-structured, integrated model, which tracked Antarctic krill by body length in surveys and fisheries, catch in fisheries, abundance in surveys, and maturity state, was coded in AD Model Builder (Fournier et al., 2012) to estimate the trends of recruitment, spawning biomass, fishing mortality and selectivity from 1992 to 2011. The model equations are provided in the Appendix. The estimated and fixed parameters setting are provided in Tables A1, A2, respectively.
Exploring the Drivers of Recruitment Dynamics
In this study, we use the Beverton-Holt (Beverton and Holt, 1957) model (Eq. 2) and Ricker spawner-recruit model (Ricker, 1954) (Eq. 3) to fit the estimates of krill spawning biomass and recruitment and compare them to the null model with the small sample size corrected Akaike information criterion (AICc) (Eq. 4) (Akaike, 1974; Burnham and Anderson, 2002).
where Rt is the estimated recruitment at time t; Pt is the estimated spawning biomass at time t; α and β are estimated parameters; k is the number of parameters estimated in the model; and n is the number of data points.
Quantifying Uncertainty and Data Weighting
Likelihood profiles were calculated for key parameters (e.g., natural mortality and mean recruitment). Calculation of appropriate sample sizes was difficult because no data describing how the samples were collected were available (Pennington et al., 2002).
Many length frequency sample data were taken from surveys (sample size was more than 30,000) and fisheries (sample size was more than 5,000), length data range from 11 to 61 mm. There were no available data on how the sample data were collected at the time of assessment, so estimating the effective sample size was not possible. In this study, the length frequency data were down weighted from the sample size to 20 (initial smoothness weighting was 20). The coefficients of variation (CVs) from catches are not known but should be relatively small and were set to 0.01. The weights of the multiplier for the effective sample size for size data are 0.25, 0.5, and 1.
Impacts of Climate Change on Estimated Resources
To further investigate the impact of climate change on Antarctic krill resources and to pave the way for how environmental factors can be better incorporated into assessment models in the future, this study used correlation analysis to examine the relationship between relevant climate factors and the model-assessed Antarctic krill recruitment and mature biomass in time series.
Sequential t-test analyses of regime shifts (STARS) are used to examine changes in climate indices and assessed resources over time series. In order to eliminate the influence of the autocorrelation of the leap nodes in the time series, the original time series were preprocessed using the “pre-whitening” method of the STARS method (Rodionov, 2006). The results of the STARS analysis are affected by the cut-off length and the Huber weighting coefficient. The cut-off length used in this study was 3, and the Huber weighting coefficient was 1, with a significance level of 0.1, based on the time range selection and trial settings analyzed. STARS was programmed using the Visual Basic for Application (VBA) function in EXCEL.5
Results
Model Fits
Model estimation ends when a convergent Hessian matrix is obtained, and all data sources are fit by the maximum likelihood estimates from the assessment method. The trajectory of predicted survey numbers was relatively consistent among the three weighting scenarios (Figure 2). In general, the years in which large survey abundances were observed were poorly fit, mainly in 2002–2003, 2008, and 2010 year. Fits to catch biomass were relatively good under all scenarios (Figure 3). Overall, the survey length composition data were better fit than the catch length composition data (compare Figure 4A to 4B). Some years with notably poor fits in the catch composition data were 2005, 2007, 2008, and 2011 (Figure 4B). In 2005 and 2011, the predicted catch length composition displayed a peak in large size classes that was not observed. In 2007 and 2008, the predicted catch length composition displayed a peak in small size classes that was not observed in the data. Differences in fits among weighting scenarios were minor for both survey and catch length composition data.
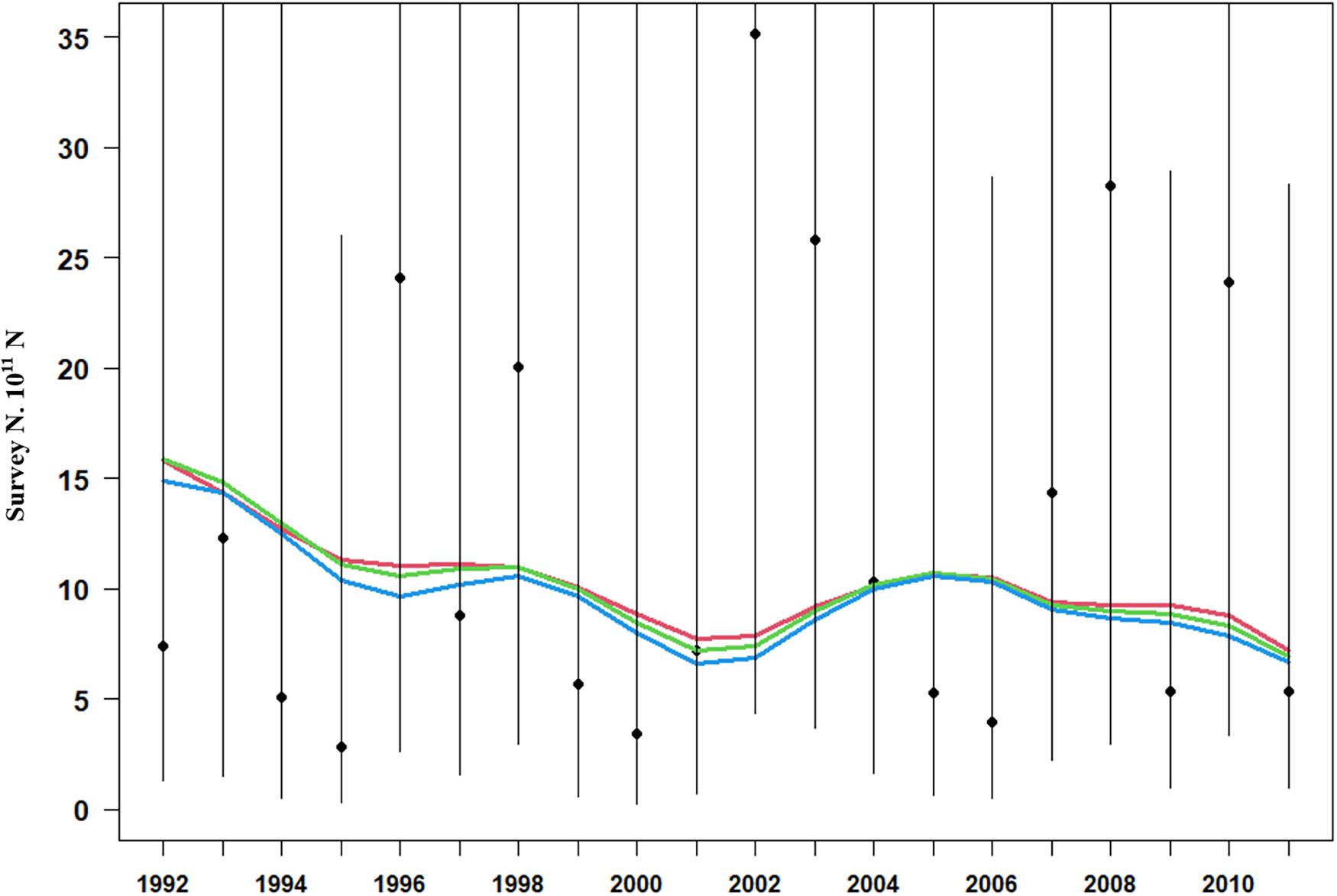
Figure 2. Model fits (three solid line, red line present weights in 0.25, green line present weight in 0.5, blue line present weight in 1) to observed survey number (black dots) with 95% confidence intervals (black lines).
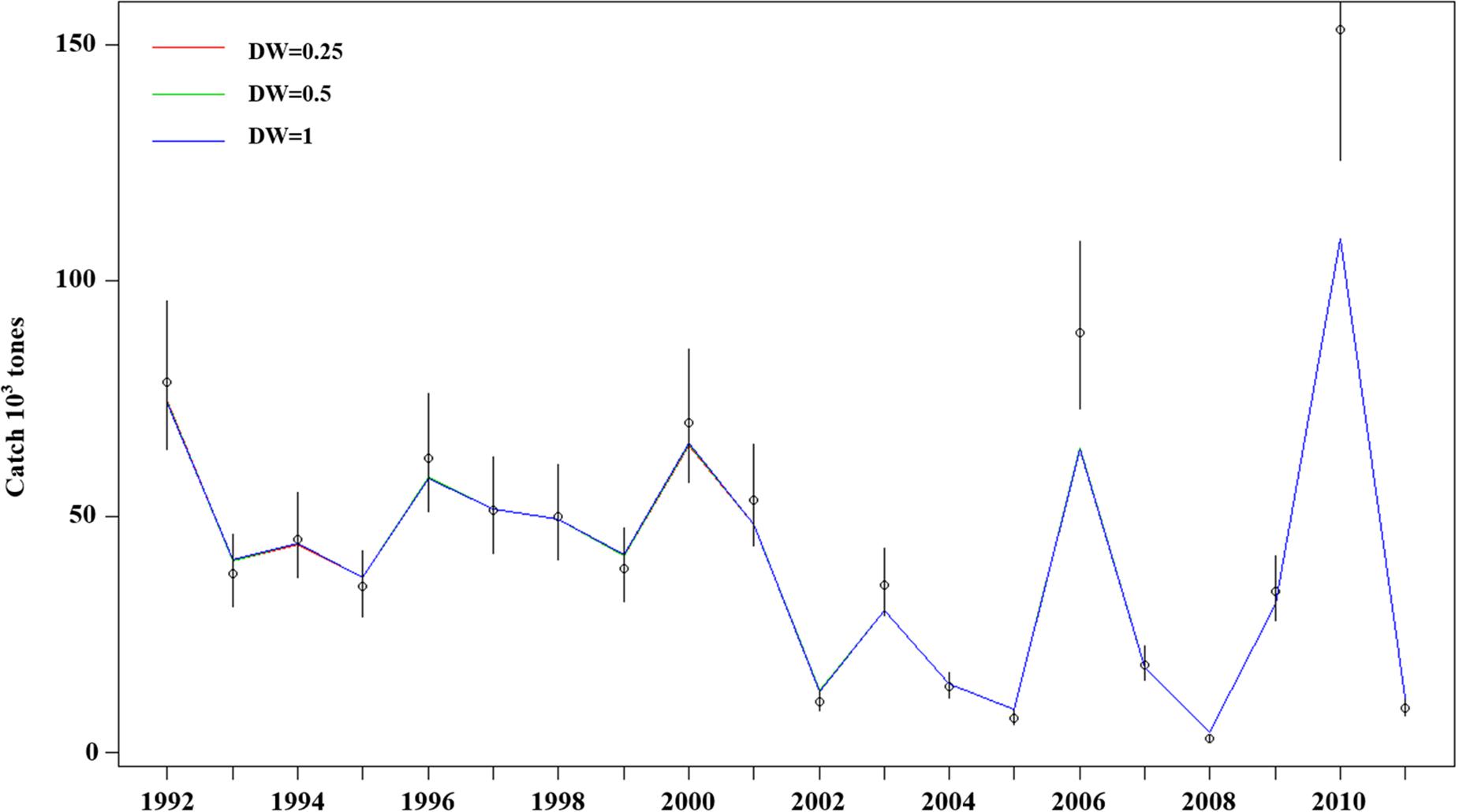
Figure 3. Model fits (three solid line, red line present weights in 0.25, green line present weight in 0.5, blue line present weight in 1) to observed catch biomass (black dots) with the 95% confidence intervals (black line). The assumed CVs for the catch data are very small, so it is difficult to see the error bars and the fits to the data are quite close.
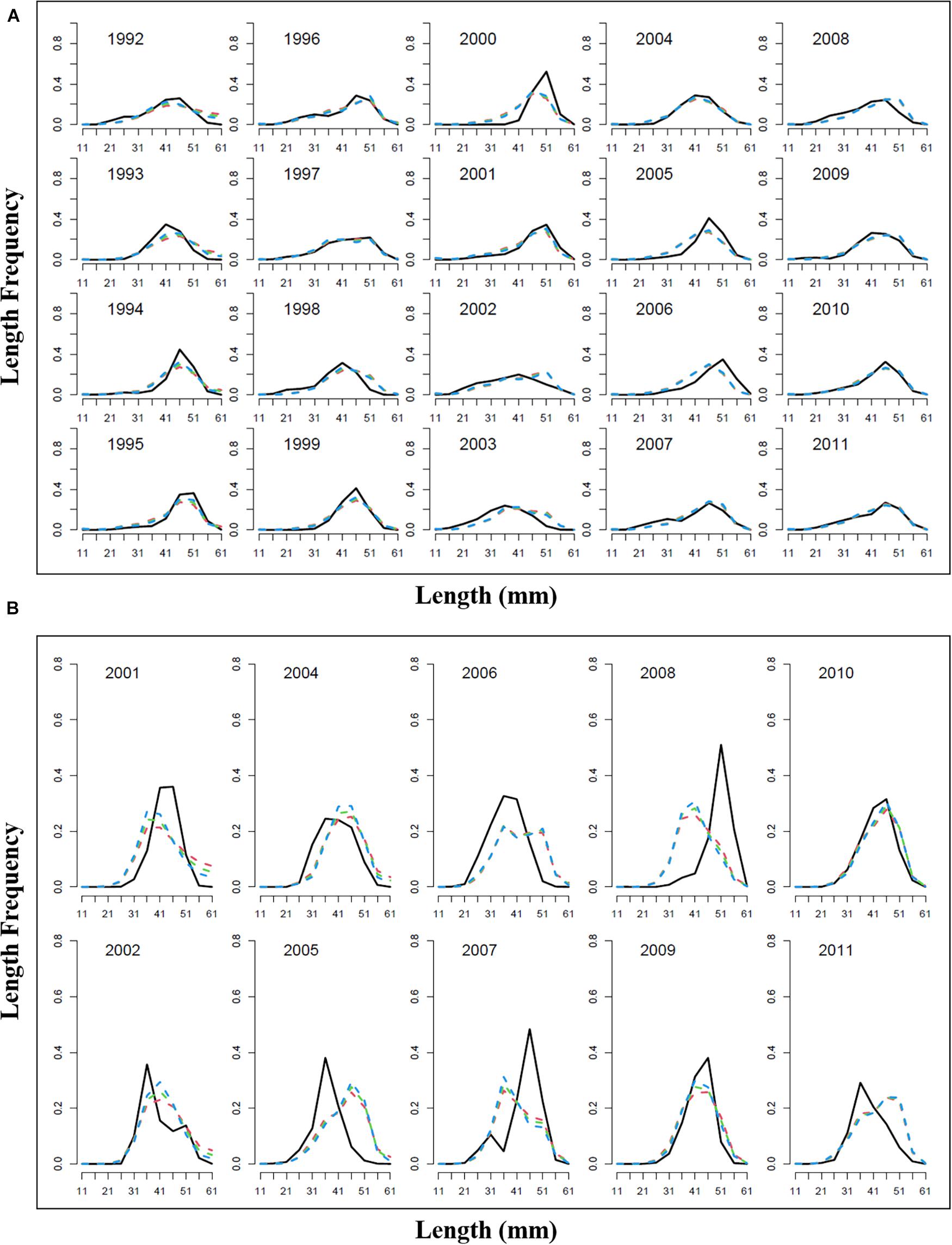
Figure 4. Observed length frequency length (black solid line) and predicted length frequency (dotted red line present weights in 0.25, dotted green line present weight in 0.5, dotted blue line present weight in 1); (A) survey length frequency; (B) catch length frequency.
Assessment
Recruitment, fishing mortality, natural mortality, mature biomass, biomass of the maximum sustainable yield (BMSY) and selectivity of survey and fishing were estimated by the integrated model under three kinds of data weightings. Under the three weighting scenarios, the results show that estimates of recruitment have same trend of change, and the highest and lowest values were located in 1994 and 1997 year, respectively (Figure 5), the assessment results for each year are shown in Table 2; estimates of fishing mortality range from 0.0036 to 0.2380, with an average fishing mortality of 0.05 year–1, and the values are very close under the three weighting scenarios (Figure 6); estimated mature biomass also have same trend of change, and the highest and lowest values were located in 1998 and 2001 year, respectively (Figure 7), the assessment details for each year are shown in Table 3. The estimated results of natural mortality, biomass of the maximum sustainable yield (BMSY) and selectivity of survey and fishing are detailed in Table 4. The change of the survey and fishing selectivity estimated by the model are shown in Figure 8. In addition, the estimation result of the increment per year at length and size transition matrix by the model are shown in Figure 9.
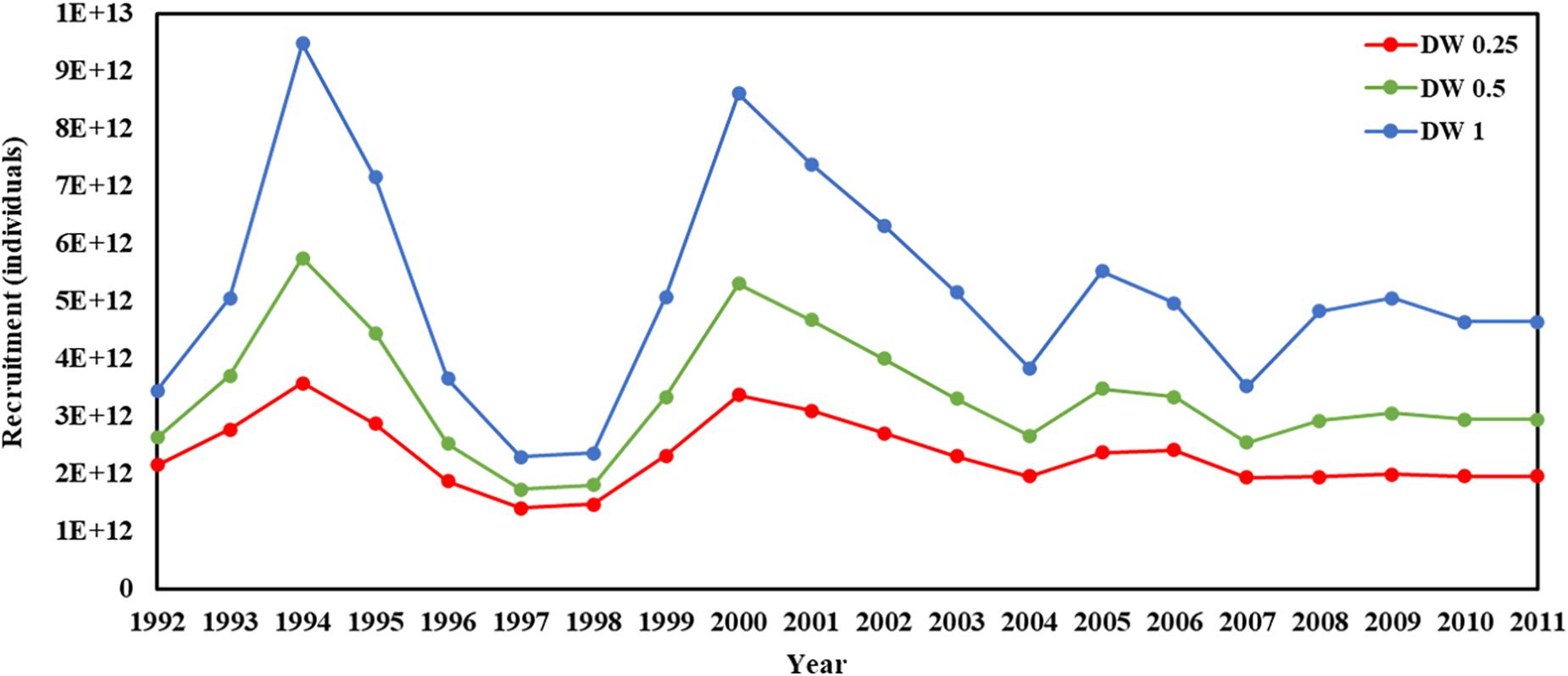
Figure 5. Recruitment in 1992–2011 years (red line present weights in 0.25, green line present weight in 0.5, blue line present weight in 1).
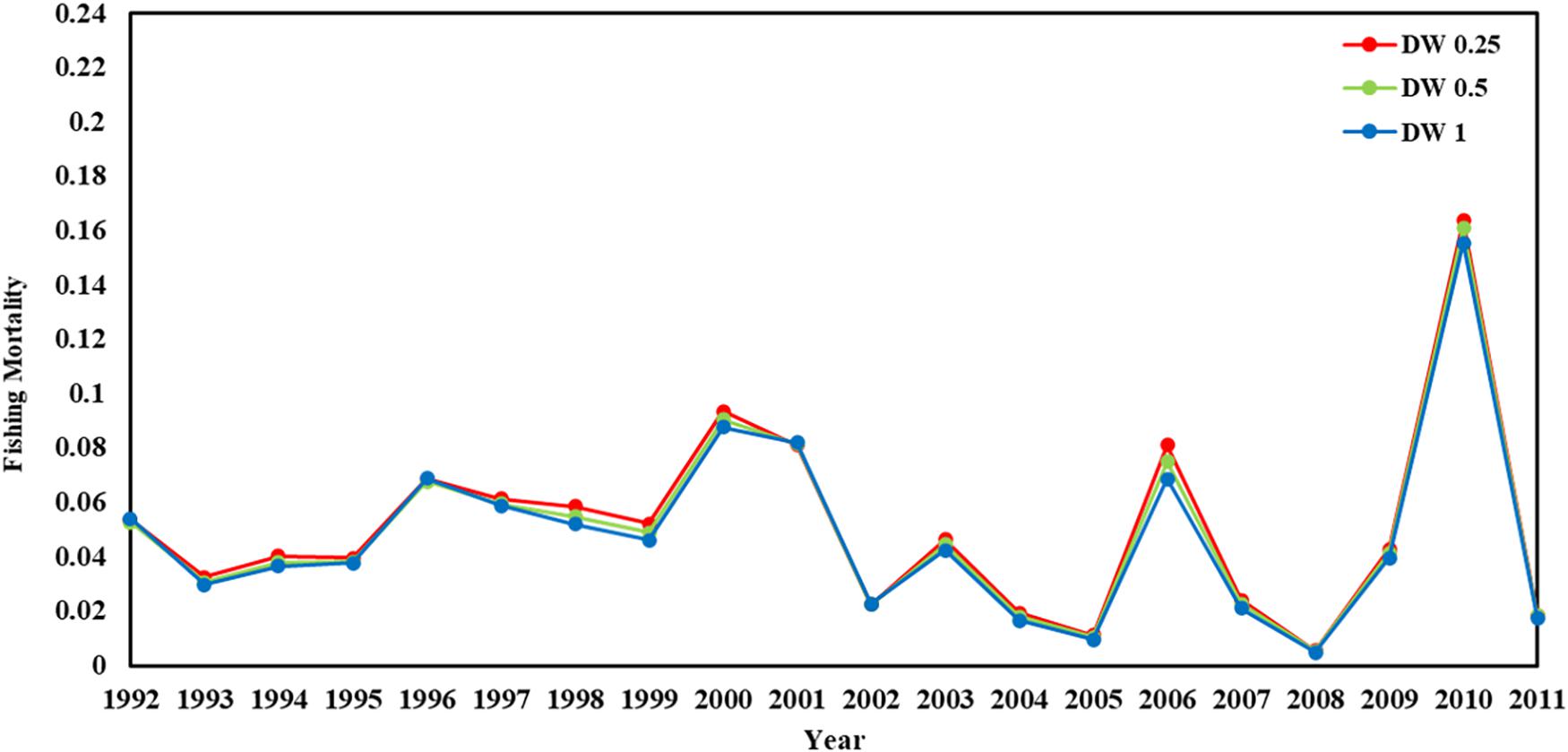
Figure 6. Fishing mortality in 1992–2011 years (red line present weights in 0.25, green line present weight in 0.5, blue line present weight in 1).
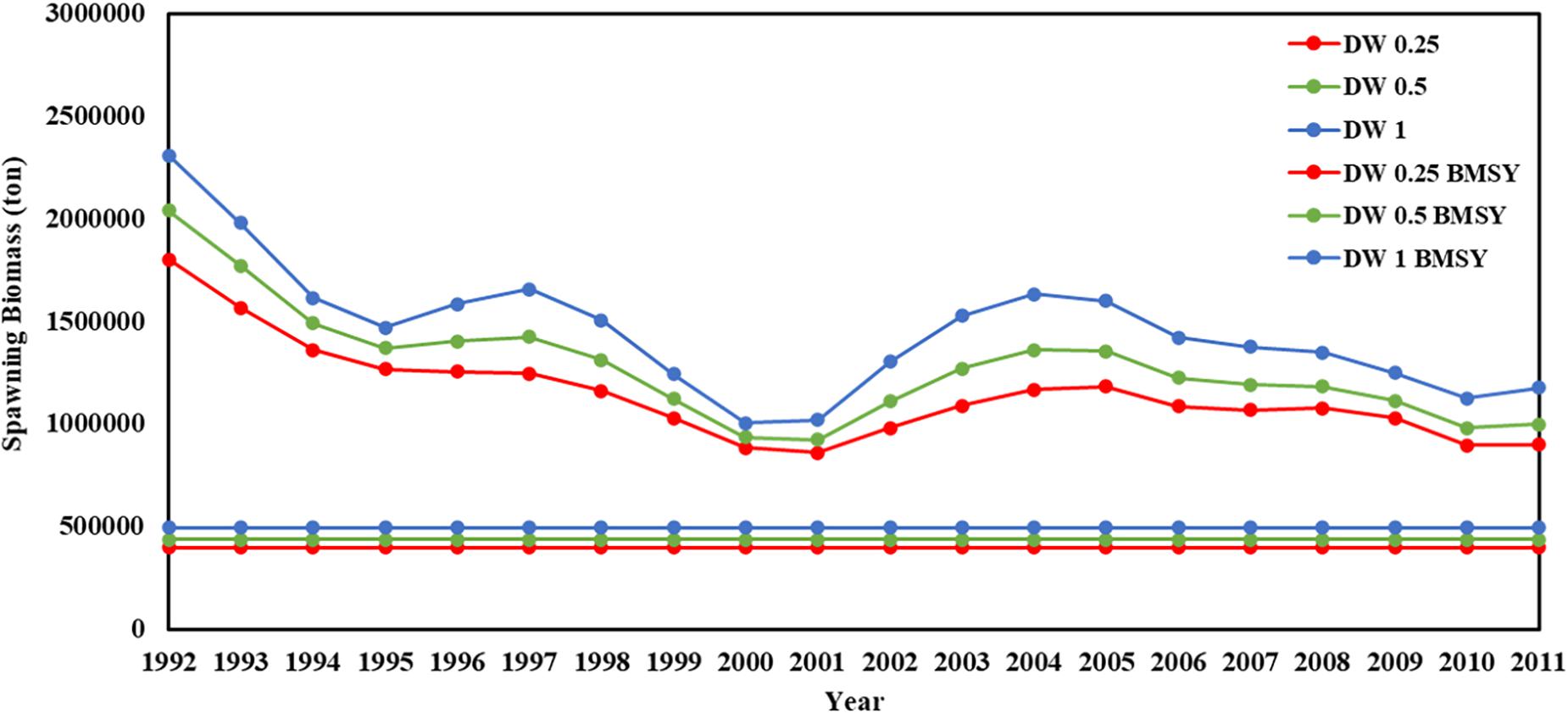
Figure 7. Spawning biomass and BMSY in 1992–2011 years (red line present weights in 0.25, green line present weight in 0.5, blue line present weight in 1).
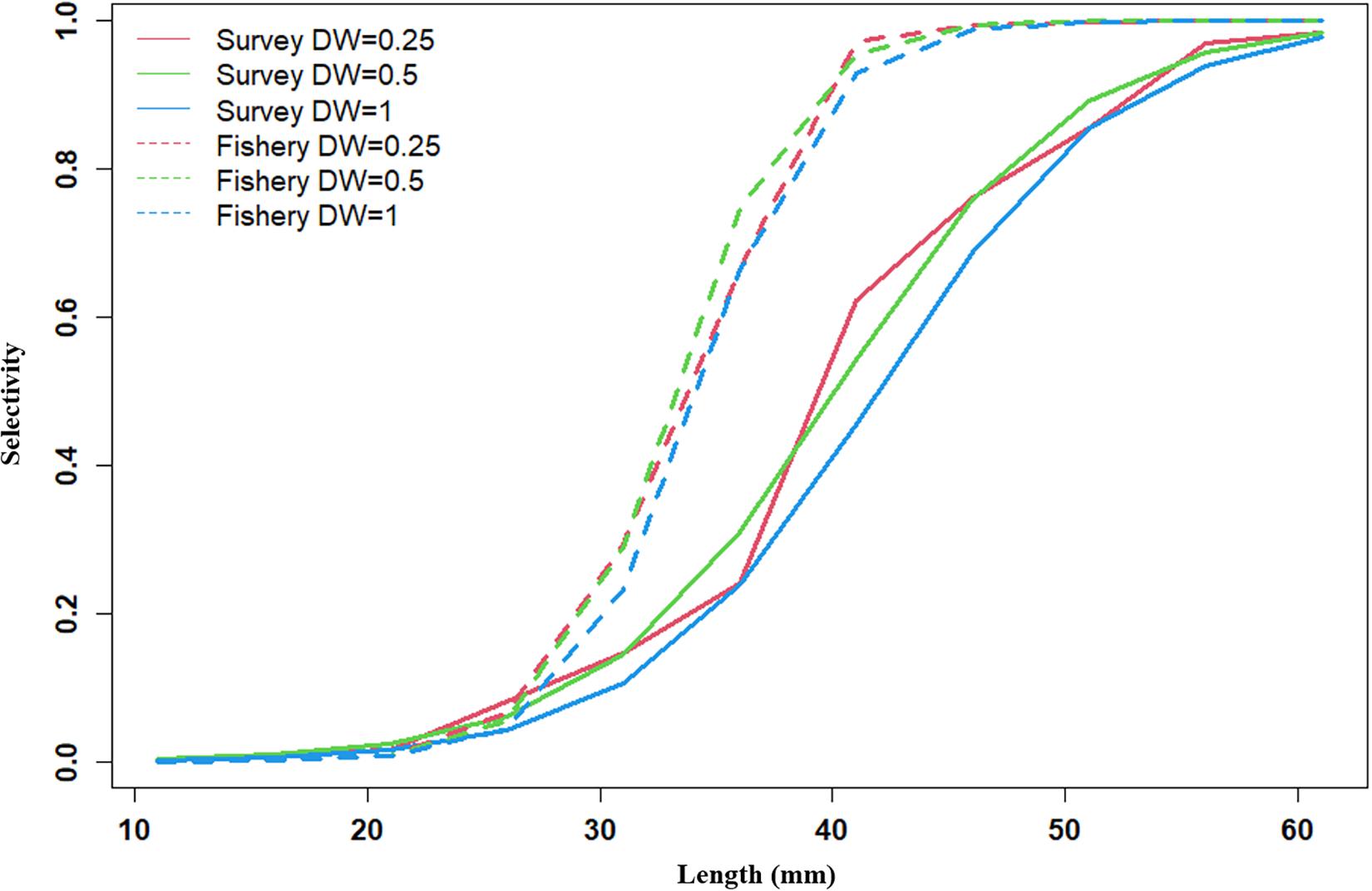
Figure 8. Survey selectivity and fishing selectivity in 1992–2011 years (red line present weights in 0.25, green line present weight in 0.5, blue line present weight in 1).
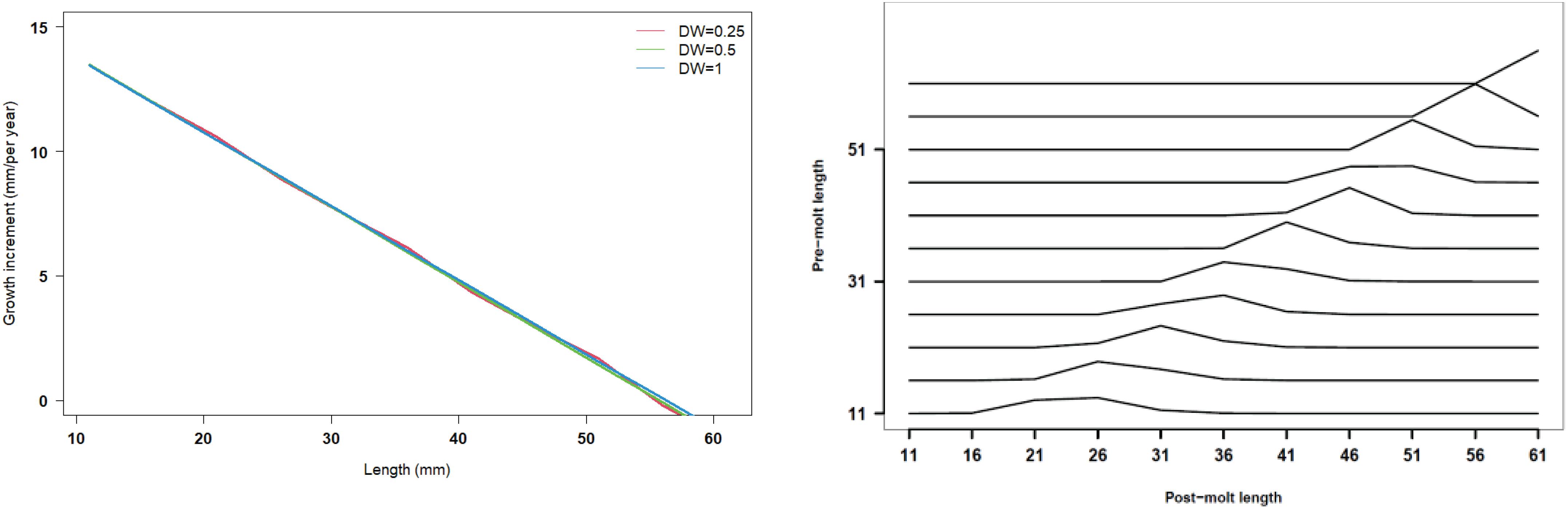
Figure 9. Length increment per year at length and size transition matrix under three kinds of data weightings (red line present weights in 0.25, green line present weight in 0.5, blue line present weight in 1).
The Relationship Between Spawning Biomass and Recruitment
We used the Beverton-Holt and Ricker models to fit the relationship between spawning biomass and estimated recruitment, and the results indicated that there was no significant correlation between spawning biomass and estimated recruitment (Figure 10).
Relationship of Estimated Recruitment, Spawning Biomass and Climate Change
The distance level, cumulative sum, and interannual variation of estimated Antarctic krill recruitment and spawning biomass are shown in Figures 11A,B. Based on the results of the STARS analysis, the jumping change in estimated recruitment occurred in 1995/96 and 1999/2000 year, and the jumping change in estimated mature biomass occurred in 1993/94, 1998/99, 2003/04, and 2009/10 year. The distance, cumulative sum, and interannual variation of SAM, SOI, and SST Nino 3.4 are shown in Figures 11C–E. The interannual leapfrog in SAM occurred in 1997/98 year, the interannual leapfrog in SOI occurred in 1998/99 year, and the interannual leapfrog in SST occurred in 1998/99 year.
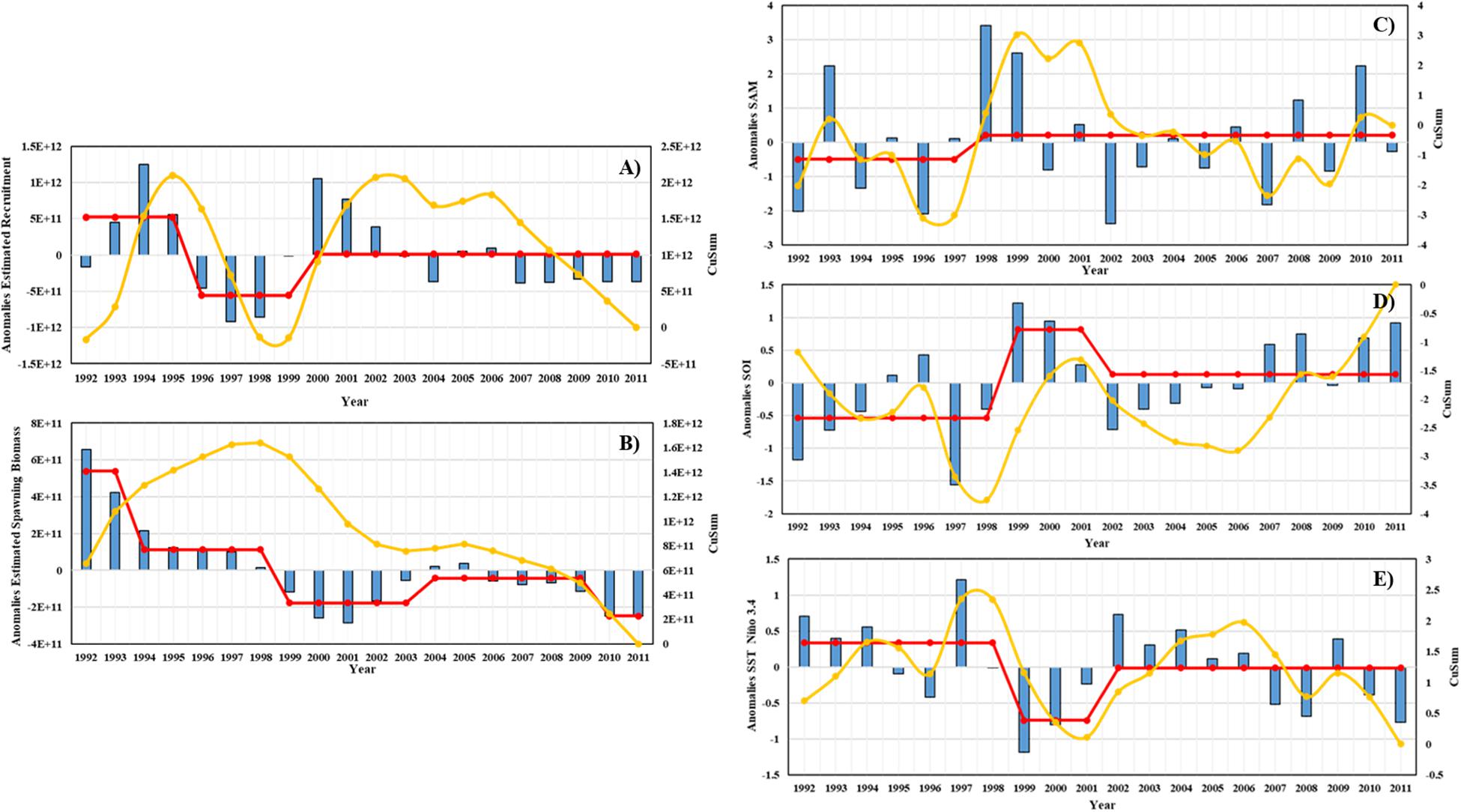
Figure 11. Interannual variability of estimated Antarctic krill recruitment, spawning biomass and three climate indices. (A) Estimated recruitment, (B) Estimated spawning biomass, (C) SAM, (D) SOI, (E) SST in Nino 3.4. The histogram is the distance level, the yellow line is the cumulative, the red line is the steady-state transition obtained from the STARS analysis.
In general, there is a certain lag in the effect of climate change on marine organisms. Therefore, comparing the estimated Antarctic krill recruitment, mature biomass and the leap in the same time series with the three climate factors, we can find that the climate changes have influence on the estimated Antarctic krill recruitment and mature biomass to some extent, but the pattern of the influence is not very obvious.
Discussion
Diversity of Antarctic Krill Stock Assessment
Stock Assessment
In this study, we used a new method to assess krill resources in subarea 48.1 near the Antarctic Peninsula and obtained important information about the recruitment, spawning biomass, fishing mortality and natural mortality of krill. The recruitment, spawning biomass and fishing mortality of krill were estimated to have greatly changed during 1992–2011. Under the three kinds of data weightings, there were some differences in the estimated recruitment of krill, but the trends were consistent The average levels of the estimated recruitment were 2.33 × 1012, 3.36 × 1012, and 5.16 × 1012, the highest estimated values were 3.58 × 1012, 5.76 × 1012, and 9.49 × 1012 in 1994, and the lowest estimated values were 1.47 × 1012, 1.81 × 1012, and 2.36 × 1012 in 1998. In addition, the estimated recruitment of krill presents a cyclical variation, with an average period of 3–4 years, this variation in the cycle of change is related to the physical environment of the polar ecosystem (Kinzey et al., 2019), but also to the behavior of its predators (Fraser et al., 1992; Fraser and Trivelpiece, 1995; Reid et al., 1999, 2002).
Kinzey et al. (2015) estimated the recruitment of krill based on an integrated age structured model using the same survey data and acoustic survey data in the same area. The results showed that the recruitment of krill ranged from 3.85 × 106 to 0.58 × 108, which was lower than the estimated recruitment of krill in our assessment model. For krill resources, recruitment estimation is difficult, and it is normal that the estimated results of different assessment models are different. In the observation time range of this study and other related studies, there was a phenomenon in which the recruitment of krill was very low in 1 year and very high in the following year (Siegel, 1988). For krill resources, this rapid change might not be completely attributed to the biological properties of the krill population (mainly including growth and reproduction) and the level of spawning biomass; instead, it could be influenced more by environmental changes. The greatest challenge to further understand the population dynamics of krill recruitment variability is to separate the effects of oceanography and the environment forcing on krill abundance from factors affecting the population dynamics of the species that has a circumpolar distribution, and yet shows little evidence of genetic stock structure (Bortolotto et al., 2011). In addition, from the perspective of the ecological status of Antarctic krill in the Southern Ocean, in the future, an in-depth study of the changes in trophic levels (food web positions) of Antarctic krill in the ecosystem over a certain time scale, using the law of conservation of energy as a guideline, could provide information for exploring the mechanisms of changes in its recruitment and its changes in time and space.
Compared with the estimated recruitment of krill, the estimated mature biomass had relatively small differences under the three kinds of data weightings, and the trends over time were consistent. The estimated average mature biomass was 1.15 × 106, 1.28 × 106, and 1.46 × 106 tons, the highest value appeared in 1992, and the lowest value appeared in 2001. Compared with the assessment results from Kinzey et al. (2015), the differences in the estimated mature biomass were not very significant. However, similar to the estimated recruitment of krill, the estimated mature biomass varied greatly within the observation time frame of this study. Comparing the estimated mature biomass with the corresponding catch, there is no obvious relationship between them. Judging from the characteristics of krill resources, the variability of krill resources is mainly due to changes in krill distribution rather than changes in abundance and biomass. Changes in the structure of the krill population have also be observed in related studies. Therefore, the density dependence of krill resources and the transport mechanism of krill populations in the Southern Ocean are issues that need to been understood in depth. The research results of these issues can further provide help for stock assessments of krill.
Different from the estimated recruitment and the mature biomass, the estimated fishing mortality showed almost no difference under the three data weightings, and the estimated average fishing mortality was 0.05. In general, the level of catch corresponds to the level of fishing mortality. However, when comparing the fishing mortality estimated by the model with the corresponding yield of krill, there was no obvious correlation between the level of krill catch and the level of fishing mortality within the observation range. In years with high krill catch, the corresponding fishing mortality was not very high. The cause of this phenomenon is mainly due to the predation of krill by marine mammals such as whales and seals, which is much higher than the fishing mortality of krill. Therefore, the different kinds of data weightings that were used for the model estimation had little effect on the estimated fishing mortality. In addition, in recent years, there has been less discussion about the risks that fisheries bring to krill resources (Meyer et al., 2020; Watters et al., 2020). This lack of attention also reflects the view that the current fishing level cannot have an appreciable impact on krill resources, and the catch was only a small part of the total biomass of krill.
The natural mortality and 50 and 95% fishery and survey selectivity have certain differences under the three data weightings. Natural mortality is a relatively important parameter; it is estimated by this study to be between 0.47 and 0.60 under the three weightings. The natural mortality range estimated by this study is smaller than the range of natural mortality estimated according to the catch curve method (Siegel, 1986, 1989), Pauly empirical formula method (Basson, 1994), von Bertalanffy (VB) equation method (Basson and Beddington, 1989; Basson, 1994), and B-H method (Siegel, 1987; Knox, 2006), and it is close to the natural mortality estimated by the length cumulative frequency method (Siegel, 1986, 1991) and the predator curve method (Siegel, 1992). Natural mortality is very difficult to accurately estimate for a mobile resource such as marine living resources, and natural mortality is not a constant value; it will change with the different life history stages of the organism and the environment in which it is located (Virtue et al., 1996). Different estimation methods and different parameter settings during estimation will affect the estimation results.
Climate Change Implication for Krill Dynamics
In the analysis of the leapfrog of Antarctic krill recruitment, mature biomass and three climate indices, it can be seen that the leapfrog of Antarctic krill recruitment and mature biomass are correlated with the leapfrog of three climate indices, which is consistent with the results of other studies, that is, climate change affects the recruitment and abundance of Antarctic krill to some extent. The correlation results demonstrate the accuracy of the model results and further confirm the impact of climate change on Antarctic krill, which paves the way for the future inclusion of climate factors as parameters in the integrated size-structured model.
Relationship Between Recruitment and Mature Biomass
Within the observed population size range, there was no obvious relationship between recruitment and spawning biomass estimated by the model. This phenomenon is very common in the case of using commercial fishing data for stock assessment (Punt et al., 2013). This is mainly due to the lack of information on recruitment of krill. In addition, compared with other resources, krill are small in size and live in polar regions that are not conducive to long-term surveys. Whether through scientific surveys or commercial fishing, it is difficult to obtain group information on krill recruitment. From the perspective of population biology, the lack of an obvious relationship between the estimated recruitment and spawning biomass of krill is mainly due to two reasons. First, it is generally believed that populations or resource groups with relatively small changes in their numbers will have a close relationship between their recruitment and spawning biomass. However, for populations or resource groups with drastic changes in their numbers, the relationship between their recruitment and spawning biomass does not seem to be clearly linked, and to be more precise, the relationship between the recruitment and spawning biomass is less direct, and the strong influence brought by some factors obscures the relationship between the recruitment and spawning biomass. The results of this study show that within the observed range, the estimated recruitment and spawning biomass of krill vary greatly, and the correlation is not obvious. Second, previous studies have pointed out that the strength of the relationship between the recruitment of bait species in one year and the spawning biomass in subsequent years is uncertain. The level of recruitment can be controlled by factors such as the environment, ecosystems or some external driving factors, and these factors have a great influence on bait species. Krill resources are bait resources and are preyed by whales, penguins, seals and seabirds in the Southern Ocean. In addition to the above two main factors, the status of krill resource density may also affect the estimation of the spawning-recruitment relationship, but because there are too many factors involved, there are few studies that can clearly prove this point of view.
From the perspective of model estimation, the Ricker and B-H models that are currently commonly used and used in this article are completely based on the dependence of population resource density. In many cases, they may not be very suitable. A more complete reproduction model should consider not only the recruitment and spawning biomass but also the role of environmental factors in the process from recruitment to spawning biomass. For some species, the impact of fishing activities must also be considered. For Antarctic krill, a bait resource with large changes in resources and strong environmental impacts, these two models cannot effectively reflect the relationship between recruitment and spawning biomass, which is relatively normal. In future research, improving the quality of collected data and selecting important influencing factors to bring into the estimation model are two very important tasks.
Application to Fishery Management
When evaluating any kind of fishery resource, the evaluation method is selected to make the results of the evaluation meet the reference data required for production management as much as possible and to maximize the use of existing data (Punt et al., 2013). For krill resources, due to the special geographic location where they live, it is very difficult to obtain scientific survey data for long-term and continuous scientific investigations. However, as an important fishery resource in the Southern Ocean, krill has more catch data. The integrated size structure model used in this article makes full use of the scientific survey data and fishery data of krill and balances the difference in model estimation caused by the effective sample size of body length data by means of data weighting. The model does not require the assumption of deterministic growth or the assumption that the population is stable, and it can express the uncertainty related to the model output in a variety of ways (Punt et al., 2013). However, our research may not be feasible or necessary for future fishery management. Even so, at present, the estimated result indeed lays the foundation for effective fishery management in the key area of 48.1 and provides a background for the biology of krill in the 48.1 area. We developed a new type of krill assessment method on the basis of previous studies, and the uncertainty of the estimation is also reflected in the posterior distribution. Based on the estimated results obtained in this article, the mature biomass, sustainable fishing mature biomass, and fishery selectivity assessed by the model can provide scientific references for future krill management in subarea 48.1. Comparing the global catch data of krill published by CCAMLR with the mature biomass of krill estimated by the model, the global catch is approximately 10–13% of the estimated mature biomass, and the estimated BMSY is approximately three times higher than the fishing limit (1.5 × 105 tons) set by CCAMLR for subarea 48.1 (Nicol et al., 2012). Combined with the estimated fishing mortality, it can be considered that the current krill resources in subarea 48.1 are not overfished, and this view is also consistent with the current belief of most scholars; that is, the output of krill accounts for only a small part of its resources. From a macro perspective, this result is indeed the case, but the recruitment and mature biomass estimated in this study show slight downward trends. In addition, studies have shown that the recruitment of krill near the Antarctic Peninsula is decreasing, the average body length of the krill that are caught is larger, and climate change (mainly reflected by the reduction in sea ice coverage and time) is becoming increasingly unfavorable for the formation of krill recruitment (Loeb et al., 1997; Siegel et al., 1997; Quetin et al., 2007; Wiedenmann et al., 2008, 2009). The current fishing situation will have a poorly quantified and poorly controlled impact on local krill resources. Therefore, in the future management of Antarctic krill resources in the 48.1 zone, fishing control in smaller units may be a better choice (Constable and Nicol, 2002; Miller, 2002).
Our research has developed an assessment model for Antarctic krill, and it has certain advantages. However, the estimated value of the resource state is generally sensitive to the data input and the structure of the evaluation model. From the perspective of data sources, the main problems in data collection in this study include three points: (1) the collected length data have systematic differences, and the information about the effective sample data is not clear, for example, the survey sample data volume in 2001 was 167, while the survey sample data volume in 2010 was only 59, and the contribution of these two parts of data to the model estimation will be different; (2) the fishery report is not complete, which is mainly reflected in the unclear information about some covariates of catch data; (3) some parameters used in the evaluation are poorly selected, for example, the body length-weight relationship of krill is calculated based on an empirical formula, and no site-specific research has been conducted. From the perspective of model design, the time interval set by the model, bin size of length data, etc., will affect the output of the model. In this study, the model we built did not consider the spatial dimension, so the estimated fishing mortality and reference point have bias. The results of this study can lay a foundation for the development of future assessment methods to manage this resource more scientifically, but there are also uncertainties. In future krill resource assessments, it is very important to collect data through scientific and rigorous experimental design.
Leaving aside Antarctic krill resources, other marine crustacean resources such as crab, shrimp, and abalone also form rich and valuable fisheries, and these resources also hamper the assessment work because they are different from fish (Punt et al., 2013). When surveying these resources, in addition to their abundance data, the most available data is their size data, and we have developed an integrated size structure model in which all processes are based on size data, and multiple data sources can be used in combination to maximize the use of data. Although all processes of the model can be based on size data, they are not limited to it, and the model can still be applied flexibly through transformation for biological resources with known length-age relationships. Therefore, the development of this model not only complements the methodologies for the assessment of Antarctic krill resources, but also provides a reference for the assessment of similar resource groups.
Data Availability Statement
The original contributions presented in the study are included in the article/Supplementary Material, further inquiries can be directed to the corresponding authors.
Author Contributions
RW done the modeling, data analyses, and wrote the first draft. PS helped modeling, mapping, and revised the manuscript. PS, YL, and LL revised the manuscript. All authors participated in the discussion to finalize the manuscript.
Funding
This study was supported by the response and feedback of the Southern Ocean to climate change (RFSOCC2020-2025).
Conflict of Interest
The authors declare that the research was conducted in the absence of any commercial or financial relationships that could be construed as a potential conflict of interest.
Publisher’s Note
All claims expressed in this article are solely those of the authors and do not necessarily represent those of their affiliated organizations, or those of the publisher, the editors and the reviewers. Any product that may be evaluated in this article, or claim that may be made by its manufacturer, is not guaranteed or endorsed by the publisher.
Acknowledgments
We are very grateful to Cody S. Szuwalski for helping us modify the assessment model, and his comments greatly improved this manuscript. We also acknowledge the Doug Kinzey for sharing the related data required to conduct this study.
Supplementary Material
The Supplementary Material for this article can be found online at: https://www.frontiersin.org/articles/10.3389/fmars.2021.710544/full#supplementary-material
Footnotes
- ^ https://swfsc.noaa.gov/AERD-Data/
- ^ https://www.ccamlr.org/en/data/ccamlr-data
- ^ http://www.nerc-bas.ac.uk/icd/gjma/sam.html
- ^ https://www.cpc.ncep.noaa.gov/data/indices/
- ^ http://www.Beringclimate.noaa.gov/regimes/index.html
References
Akaike, H. (1974). A new look at the statistical model identification. IEEE Trans. Automat. Control 19, 716–723. doi: 10.1007/978-1-4612-1694-0_16
Atkinson, A., Hill, S. L., Pakhomov, E. A., Siegel, V., Reiss, C. S., Loeb, V. J., et al. (2019). Krill (Euphausia superba) distribution contracts southward during rapid regional warming. Nat. Climate Change 9, 142–147. doi: 10.1038/s41558-018-0370-z
Atkinson, A., Siegel, V., Pakhomov, E. A., Jessopp, M. J., and Loeb, V. (2009). A re-appraisal of the total biomass and annual production of Antarctic krill. Deep Sea Res. I Oceanogr. Res. Pap. 56, 727–740. doi: 10.1016/j.dsr.2008.12.007
Atkinson, A., Siegel, V., Pakhomov, E., and Rothery, P. (2004). Long-term decline in krill stock and increase in salps within the Southern Ocean. Nature 432, 100–103. doi: 10.1038/nature02996
Basson, M. (1994). Towards a Distribution of M/K for Krill (Euphausia superba) Required for the Stochastic Krill Yield Model. CCAMLR WG-Krill-94/11. Hobart, TAS.
Basson, M., and Beddington, J. R. (1989). “Preliminary analysis of growth and mortality rates of Antarctic krill (Euphausia superba) from length frequency samples,” in Proceedings of the British Antarctic Survey Antarctic Special Topic Scheme Symposium 9-10 November 1988. University Research in Antarctica, ed. R. B. Heywood (Cambridge: British Antarctic Survey), 51–55. Available online at: http://nora.nerc.ac.uk/id/eprint/520965/
Beverton, R., and Holt, S. J. (1957). On the dynamics of exploited fish populations. fishery investigations, ministry of agriculture, fishery and food: great britain. Copeia 46:533. doi: 10.2307/2333556
Bortolotto, E., Bucklin, A., Mezzavilla, M., Zane, L., and Patarnello, T. (2011). Gone with the currents: lack of genetic differentiation at the circum-continental scale in the antarctic krill Euphausia superba. BMC Genet. 12:32. doi: 10.1186/1471-2156-12-32
Budzinksi, E., Bykowski, P., and Dutkiewicz, D. (1985). Possibilities of Processing and Marketing of Products Made from Antarctic krill. FAO Fisheries Technical Paper.No. 268.
Burnham, K. P., and Anderson, D. R. (2002). A Practical Information-Theoretic Approach. Model Selection and Multimodel Inference, 2nd Edn. New York, NY: Springer. doi: 10.2307/2669574
Clark, W. G. (1991). Groundfish exploitation rates based on life history parameters. Can. J. Fish. Aquat. Sci. 48, 734–750. doi: 10.1139/f91-088
Constable, A. J., and Nicol, S. (2002). Defining smaller-scale management units to further develop the ecosystem approach in managing large-scale pelagic krill fisheries in Antarctica. CCAMLR Sci. 9, 117–131.
Cox, M. J., Candy, S., De la Mare, W. K., Nicol, S., Kawaguchi, S., and Gales, N. (2019). Clarifying trends in the density of Antarctic krill Euphausia superba Dana, 1850 in the South Atlantic. A response to Hill et al. J. Crustac. Biol. 39, 323–327. doi: 10.1093/jcbiol/ruz010
Fabra, A., and Gascon, V. (2008). The convention on the conservation of Antarctic marine living resources (CCAMLR) and the ecosystem approach. Int. J. Mari. Coast. Law 23, 567–598. doi: 10.1163/092735208X331854
Flores, H., Atkinson, A., Kawaguchi, S., Krafft, B. A., Milinevsky, G., Nicol, S., et al. (2012). Impact of climate change on Antarctic krill. Mari. Ecol. Prog. Ser. 458, 1–19. doi: 10.3354/meps09831
Fournier, D. A., Skaug, H. J., Ancheta, J., Ianelli, J., Magnusson, A., Maunder, M. N., et al. (2012). AD model builder: using automatic differentiation for statistical inference of highly parameterized complex nonlinear models. Optimization Methods Softw. 27, 233–249. doi: 10.1080/10556788.2011.597854
Fraser, W. R., and Trivelpiece, W. Z. (1995). Relationships between variability in sea-ice coverage, krill recruitment, and the foraging ecology of Adelie penguins. Antarctic J. U.S. 30, 271–272.
Fraser, W. R., Trivelpiece, W. Z., Ainley, D. G., and Trivelpiece, S. G. (1992). Increases in Antarctic penguin populations: reduced competition with whales or a loss of sea ice due to global warming? Polar Biol. 11, 525–531. doi: 10.1007/BF00237945
Hardy, A. C. (1936). Observation on the uneven distribution of oceanic plankton. Discovery Rep. 11, 511–538.
Hewitt, R. P., Watkins, J., Naganobu, M., Sushin, V., Brierley, A. S., Demer, D., et al. (2004). Biomass of Antarctic krill in the Scotia Sea in January/February 2000 and its use in revising an estimate of precautionary yield. Deep Sea Res. II Top. Stud. Oceanogr. 51, 1215–1236. doi: 10.1016/j.dsr2.2004.06.011
Hill, S. L., Atkinson, A., Pakhomov, E. A., and Siegel, V. (2019). Evidence for a decline in the population density of Antarctic krill Euphausia superba still stands. a comment on Cox. J. Crust. Biol. 39, 316–322. doi: 10.1093/jcbiol/ruz004
Hofmann, E. E., and Hüsrevoğlu, Y. S. (2003). A circumpolar modeling study of habitat control of Antarctic krill (Euphausia superba) reproductive success. Deep Sea Res. Part II: Top. Stud. Oceanogr. 50, 3121–3142. doi: 10.1016/j.dsr2.2003.07.012
Howard, M. (1989). The convention on the conservation of Antarctic marine living resources: a five-year review. Int. Comp. Law Q. 38, 104–150. doi: 10.1093/iclqaj/38.1.104
Ikeda, T. (1985). Life history of Antarctic krill Euphausia superba: a new look from an experimental approach. Bull. Mari. Sci. 37, 599–608.
Ikeda, T., and Dixon, P. (1982). Body shrinkage as a possible over-wintering mechanism of the Antarctic krill, Euphausia superba Dana. J. Exp. Mar. Biol. Ecol. 62, 143–151. doi: 10.1016/0022-0981(82)90088-0
Karlsen, Ø, Suontama, J., and Olsen, R. E. (2006). Effect of Antarctic krillmeal on quality of farmed Atlantic cod (Gadus morhua L.). Aquac. Res. 37, 1676–1684. doi: 10.1111/j.1365-2109.2006.01615.x
Kawaguchi, S., and Nicol, S. (2007). Learning about Antarctic krill from the fishery. Antarctic Sci. 19, 219–230. doi: 10.1017/S0954102007000296
Kawaguchi, S., and Nicol, S. (2020). Krill fishery. Fish. Aquac 9:137. doi: 10.1093/oso/9780190865627.003.0006
Kawaguchi, S., Nicol, S., and Press, A. J. (2009). Direct effects of climate change on the Antarctic krill fishery. Fish. Manage. Ecol. 16, 424–427. doi: 10.1111/j.1365-2400.2009.00686.x
Kinzey, D., Watters, G. M., and Reiss, C. S. (2015). Selectivity and two biomass measures in an age-based assessment of Antarctic krill (Euphausia superba). Fish. Res. 168, 72–84. doi: 10.1016/j.fishres.2015.03.023
Kinzey, D., Watters, G. M., and Reiss, C. S. (2019). Estimating recruitment variability and productivity in Antarctic krill. Fish. Res. 217, 98–107. doi: 10.1016/j.fishres.2018.09.027
Loeb, V., Siegel, V., Holm-Hansen, O., Hewitt, R., Fraser, W., Trivelpiece, W., et al. (1997). Effects of sea-ice extent and krill or salp dominance on the Antarctic food web. Nature 387, 897–900. doi: 10.1038/43174
Loeb, V. J., Hofmann, E. E., Klinck, J. M., Holm-Hansen, O., and White, W. B. (2009). ENSO and variability of the Antarctic peninsula pelagic marine ecosystem. Antarctic Sci. 21:636. doi: 10.1017/S0954102008001636
Loeb, V. J., and Santora, J. A. (2015). Climate variability and spatiotemporal dynamics of five Southern Ocean krill species. Prog. Oceanogr. 134, 93–122. doi: 10.1016/j.pocean.2015.01.002
Maunder, M. N., and Punt, A. E. (2013). A review of integrated analysis in fisheries stock assessment. Fish. Res. 142, 61–74. doi: 10.1016/j.fishres.2012.07.025
Meyer, B., Atkinson, A., Bernard, K. S., Brierley, A. S., Driscoll, R., Hill, S. L., et al. (2020). Successful ecosystem-based management of Antarctic krill should address uncertainties in krill recruitment, behaviour and ecological adaptation. Commun. Earth Environ. 1, 1–12. doi: 10.1038/s43247-020-00026-1
Miller, D. G. M. (2002). Antarctic krill and ecosystem management–from Seattle to Siena. CCAMLR Sci. 9, 175–212.
Nicol, S., and de La Mare, W. (1993). Ecosystem management and the Antarctic krill. Am. Sci. 81, 36–47.
Nicol, S., and Foster, J. (2003). Recent trends in the fishery for Antarctic krill. Aquatic Living Res. 16, 42–45. doi: 10.1016/S0990-7440(03)00004-4
Nicol, S., Foster, J., and Kawaguchi, S. (2012). The fishery for Antarctic krill–recent developments. Fish Fish. 13, 30–40. doi: 10.1111/j.1467-2979.2011.00406.x
Pennington, M., Burmeister, L. M., and Hjellvik, V. (2002). Assessing the precision of frequency distributions estimated from trawl-survey samples. Fish. Bull. 100, 74–80.
Perry, F. A., Atkinson, A., Sailley, S. F., Tarling, G. A., Hill, S. L., Lucas, C. H., et al. (2019). Habitat partitioning in Antarctic krill: spawning hotspots and nursery areas. PLoS One 14:e0219325. doi: 10.1371/journal.pone.0219325
Piñones, A., and Fedorov, A. V. (2016). Projected changes of Antarctic krill habitat by the end of the 21st century. Geophys. Res. Lett. 43, 8580–8589. doi: 10.1002/2016GL069656
Punt, A. E., Huang, T., and Maunder, M. N. (2013). Review of integrated size-structured models for stock assessment of hard-to-age crustacean and mollusc species. ICES J. Mari. Sci. 70, 16–33. doi: 10.1093/icesjms/fss185
Quetin, L. B., Ross, R. M., Fritsen, C. H., and Vernet, M. (2007). Ecological responses of Antarctic krill to environmental variability: can we predict the future? Antarctic Sci. 19:253. doi: 10.1017/S0954102007000363
Reid, K., Watkins, J. L., Croxall, J. P., and Murphy, E. J. (1999). Krill population dynamics at South Georgia 1991–1997, based on data from predators and nets. Mari. Ecol. Prog. Ser. 177, 103–114. doi: 10.3354/meps177103
Reid, K. R., Murphy, E. J., Loeb, V., and Hewitt, R. P. (2002). Krill population dynamics in the scotia sea: variability in growth and mortality within a single population. J. Mari. Syst. 36, 1–10. doi: 10.1016/S0924-7963(02)00131-8
Reiss, C. S. (2016). “Age, growth, mortality, and recruitment of Antarctic krill, Euphausia superba,” in Biology and Ecology of Antarctic Krill, ed. V. Siegel (Springer International Publishing), 101–144. doi: 10.1007/978-3-319-29279-3_3
Ricker, W. E. (1954). Stock and recruitment. J. Fish. Board Canada 11, 559–623. doi: 10.1139/f54-039
Rodionov, S. N. (2006). Use of prewhitening in climate regime shift detection. Geophys. Res. Lett. 33:904. doi: 10.1029/2006GL025904
Saba, G. K., Fraser, W. R., Saba, V. S., Iannuzzi, R. A., Coleman, K. E., Doney, S. C., et al. (2014). Winter and spring controls on the summer food web of the coastal west Antarctic Peninsula. Nat. Commun. 5:4318. doi: 10.1038/ncomms5318
Siegel, V. (1986). Investigations on the biology of Antarctic krill, Euphausia superba, in the bransfield strait and adjacent areas. Mitt Inst. Seefisch Hamburg 38, 1–24.
Siegel, V. (1987). Age and growth of Antarctic Euphausiacea (Crustacea) under natural conditions. Mari. Biol. 96, 483–495. doi: 10.1007/BF00397966
Siegel, V. (1988). “A concept of seasonal variation of krill (Euphausia superba) distribution and abundance west of the Antarctic Peninsula,” in Antarctic Ocean and Resources Variability, ed. D. Sahrhage (Berlin: Springer), 219–230. doi: 10.1007/978-3-642-73724-4_19
Siegel, V. (1989). Winter and spring distribution and status of the krill stock in Antarctic peninsula waters. Archiv fü Fischereiwissenschaft 39(Suppl. 1), 45–72.
Siegel, V. (1991). Estimation of krill (Euphausia superba) mortality and production rate in the Antarctic Peninsula region. Selected Sci. Papers 1991, 145–155.
Siegel, V. (1992). Assessment of the krill (Euphausia superba) spawning stock off the Antarctic Peninsula. Arc. Fischereiwiss 41, 101–130.
Siegel, V. (2005). Distribution and population dynamics of Euphausia superba: summary of recent findings. Polar Biol. 29, 1–22. doi: 10.1007/s00300-005-0058-5
Siegel, V., De la Mare, W. K., and Loeb, V. (1997). Long-term monitoring of krill recruitment and abundance indices in the elephant Island area (Antarctic Peninsula). CCAMLR Sci. 4, 19–35. doi: 10.2307/1422825
Siegel, V., Kawaguchi, S., Ward, P., Litvinov, F., Sushin, V., Loeb, V., et al. (2004). Krill demography and large-scale distribution in the southwest Atlantic during January/February 2000. Deep Sea Res. Part II: Top. Stud. Oceanogr. 51, 1253–1273. doi: 10.1016/j.dsr2.2004.06.013
Siegel, V., Reiss, C. S., Dietrich, K. S., Haraldsson, M., and Rohardt, G. (2013). Distribution and abundance of Antarctic krill (Euphausia superba) along the Antarctic Peninsula. Deep Sea Res. Part I: Oceanogr. Res. Papers 77, 63–74. doi: 10.1016/j.dsr.2013.02.005
Spiridonov, V. A. (1995). Spatial and temporal variability in reproductive timing of antarctic krill (Euphausia superba dana). Polar Biol. 15, 161–174. doi: 10.1007/BF00239056
Tarling, G. A., Shreeve, R. S., Hirst, A. G., Atkinson, A., Pond, D. W., Murphy, E. J., et al. (2006). Natural growth rates in Antarctic krill (Euphausia superba): I. improving methodology and predicting intermolt period. Limnol. Oceanogr. 51, 959–972. doi: 10.2307/3841103
Trathan, P. N., Warwick-Evans, V., Hinke, J. T., Young, E. F., Murphy, E. J., Carneiro, A. P. B., et al. (2018). Managing fishery development in sensitive ecosystems: identifying penguin habitat use to direct management in Antarctica. Ecosphere 9:2392. doi: 10.1002/ecs2.2392
Trivelpiece, W. Z., Hinke, J. T., Miller, A. K., Reiss, C. S., Trivelpiece, S. G., and Watters, G. M. (2011). Variability in krill biomass links harvesting and climate warming to penguin population changes in Antarctica. Proc. Natl. Acad. Sci. U.S.A. 108, 7625–7628. doi: 10.1073/pnas.1016560108
Virtue, P., Nichols, P. D., Nicol, S., and Hosie, G. (1996). Reproductive trade-off in male Antarctic krill, Euphausia superba. Mari. Biol. 126, 521–527. doi: 10.1007/BF00354634
Voronina, N. M. (1998). Comparative abundance and distribution of major filter-feeders in the Antarctic pelagic zone. J. Mari. Syst. 17, 375–390. doi: 10.1016/S0924-7963(98)00050-5
Walters, C. J., and Post, J. R. (1993). Density-dependent growth and competitive asymmetries in size-structured fish populations: a theoretical model and recommendations for field experiments. Trans. Am. Fish. Soc. 122, 34–45. doi: 10.1577/1548-865919931222.3.CO;2
Watkins, J., Macaulay, M., and Everson, I. (2000). Sampling Krill. In Krill Biology, Ecology and Fisheries. London: Blackwell Science, 8–39. doi: 10.1002/9780470999493.ch2
Watters, G. M., Hinke, J. T., and Reiss, C. S. (2020). Long-term observations from Antarctica demonstrate that mismatched scales of fisheries management and predator-prey interaction lead to erroneous conclusions about precaution. Sci. Rep. 10, 1–9. doi: 10.1038/s41598-020-59223-9
Wiedenmann, J., Cresswell, K., and Mangel, M. (2008). Temperature-dependent growth of Antarctic krill: predictions for a changing climate from a cohort model. Mari. Ecol. Prog. Ser. 358, 191–202. doi: 10.3354/meps07350
Keywords: Antarctic krill, integrated size-structured model, stock assessment, Antarctic Peninsula, data weighting, climate change
Citation: Wang R, Song P, Li Y and Lin L (2021) An Integrated, Size-Structured Stock Assessment of Antarctic Krill, Euphausia superba. Front. Mar. Sci. 8:710544. doi: 10.3389/fmars.2021.710544
Received: 16 May 2021; Accepted: 11 October 2021;
Published: 09 November 2021.
Edited by:
Simone Libralato, Istituto Nazionale di Oceanografia e di Geofisica Sperimentale, ItalyReviewed by:
Cameron Hodgdon, University of Maine, United StatesMatthew Baker, North Pacific Research Board, United States
Copyright © 2021 Wang, Song, Li and Lin. This is an open-access article distributed under the terms of the Creative Commons Attribution License (CC BY). The use, distribution or reproduction in other forums is permitted, provided the original author(s) and the copyright owner(s) are credited and that the original publication in this journal is cited, in accordance with accepted academic practice. No use, distribution or reproduction is permitted which does not comply with these terms.
*Correspondence: Yuan Li, bGl5dWFuQHRpby5vcmcuY24=; Longshan Lin, bGlubHNoQHRpby5vcmcuY24=