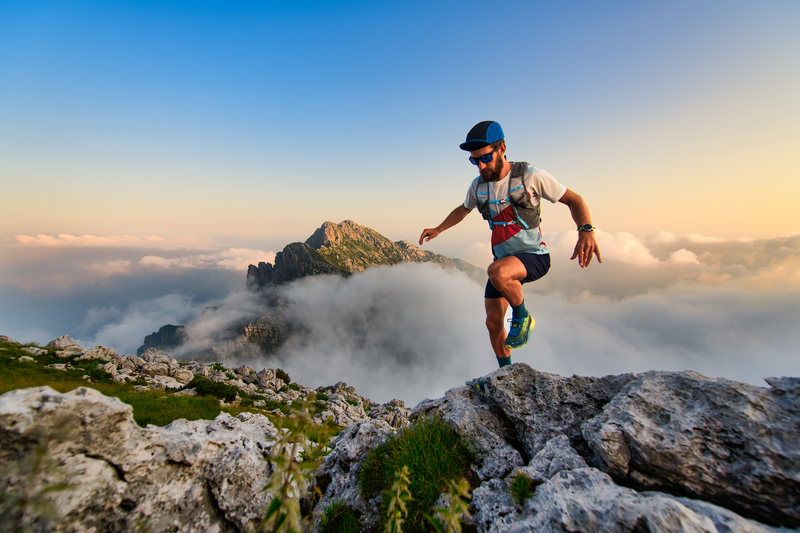
94% of researchers rate our articles as excellent or good
Learn more about the work of our research integrity team to safeguard the quality of each article we publish.
Find out more
ORIGINAL RESEARCH article
Front. Mar. Sci. , 22 June 2021
Sec. Marine Ecosystem Ecology
Volume 8 - 2021 | https://doi.org/10.3389/fmars.2021.669372
This article is part of the Research Topic Advances in 3D Habitat Mapping of Marine Ecosystem Ecology and Conservation View all 15 articles
Recent advances in deep-sea exploration with underwater vehicles have led to the discovery of vertical environments inhabited by a diverse sessile fauna. However, despite their ecological importance, vertical habitats remain poorly characterized by conventional downward-looking survey techniques. Here we present a high-resolution 3-dimensional habitat map of a vertical cliff hosting a suspension-feeding community at the flank of an underwater glacial trough in the Greenland waters of the Labrador Sea. Using a forward-looking set-up on a Remotely Operated Vehicle (ROV), a high-resolution multibeam echosounder was used to map out the topography of the deep-sea terrain, including, for the first time, the backscatter intensity. Navigational accuracy was improved through a combination of the USBL and the DVL navigation of the ROV. Multi-scale terrain descriptors were derived and assigned to the 3D point cloud of the terrain. Following an unsupervised habitat mapping approach, the application of a K-means clustering revealed four potential habitat types, driven by geomorphology, backscatter and fine-scale features. Using groundtruthing seabed images, the ecological significance of the four habitat clusters was assessed in order to evaluate the benefit of unsupervised habitat mapping for further fine-scale ecological studies of vertical environments. This study demonstrates the importance of a priori knowledge of the terrain around habitats that are rarely explored for ecological investigations. It also emphasizes the importance of remote characterization of habitat distribution for assessing the representativeness of benthic faunal studies often constrained by time-limited sampling activities. This case study further identifies current limitations (e.g., navigation accuracy, irregular terrain acquisition difficulties) that can potentially limit the use of deep-sea terrain models for fine-scale investigations.
Deep-water vertical and overhanging cliffs are important marine habitats that often host diverse and abundant communities (Huvenne et al., 2011; Johnson et al., 2013; Morris et al., 2013; Robert et al., 2020), including ecosystem engineers such as cold-water corals and deep-sea sponges (Ramirez-Llodra et al., 2010). Vertical cliffs are often associated with broadscale geomorphic features, such as continental margin troughs (Edinger et al., 2011), canyons (Freiwald et al., 2009; Huvenne et al., 2011, 2012; Gori et al., 2013; Brooke and Ross, 2014; Brooke et al., 2017; Robert et al., 2017; Pearman et al., 2020) and fjords (Haedrich and Gagnon, 1991; Gasbarro et al., 2018). Ecological studies usually assume that habitats providing a variety of environmental niches also promote enhanced biodiversity (MacArthur and Wilson, 1967; Kohn and Leviten, 1976). Vertical habitats are known to be hotspots of biodiversity (Robert et al., 2017, 2020) because of a number of interacting processes such as the complex small-scale topography defined by the geomorphology (Althaus et al., 2012; Carter et al., 2018), the depth (Stewart et al., 1985), hydrodynamics (Frederiksen et al., 1992; Mortensen et al., 2001; Kiriakoulakis et al., 2007; Lim et al., 2020; Pearman et al., 2020) and the substrate type (e.g., hard substrate for cold-water coral settlement; Gass and Roberts, 2006; Buhl-Mortensen et al., 2017; Davies et al., 2017).
One of the biggest anthropogenic threats to deep-sea benthic communities is the destructive action of bottom trawling. Deep-sea trawling activities severely damage the biogenic structure formed by epibenthic species such as sponges and cold-water corals (Hall-Spencer et al., 2002; Wheeler et al., 2005; Malecha and Heifetz, 2017). However, these environments are often characterized by increased concentrations of commercial fishes resulting in exploration and active management of Northwest Atlantic areas known to host structure-forming fauna, classified as “Vulnerable Marine Ecosystems” (VMEs, as defined by the United Nations General Assembly (UNGA) resolution 61/105 of 2006) (Costello et al., 2005; Ross and Quattrini, 2007). Crucially, deep-sea trawling activities do not have access to vertical habitats and it has been suggested that deep-water vertical habitats may provide refugia for species under trawling pressure elsewhere (e.g., Huvenne et al., 2011). Therefore, mapping the habitats and identifying the factors driving species distribution on vertical structures are of high importance for defining conservation plans in complex deep-sea environments.
Habitat mapping ‘enables to represent or predict biological patterns’ (Brown et al., 2011) as habitats reflect particular physico-chemical conditions that are delineated in space and that influence species distribution (Lamarche et al., 2016). Since the geomorphology and the substrate of the seabed are important proxies for explaining benthic species distribution, the recent advances in acoustic mapping (using multibeam echosounders [MBES] or sidescan sonars) make it a cost-effective remote sensing tool (LaFrance et al., 2014; Lamarche et al., 2016) widely used to map and characterize seafloor habitats (e.g., Greene et al., 1999; Kostylev et al., 2001; Brown and Blondel, 2009; Verfaillie et al., 2009; Brown et al., 2011; Hill et al., 2014; Ismail et al., 2015; Hogg et al., 2016; Vassallo et al., 2018; Zelada Leon et al., 2020). Typically, acoustic surveys are combined with groundtruthing validation of the benthic habitats (Micallef et al., 2012; LaFrance et al., 2014). However, acoustic surveys remain a technical challenge in deep vertical environments.
In many seabed studies, shipboard MBES is used to produce a broad-scale bathymetric map of the seabed that can serve for habitat mapping (Brown and Blondel, 2009; Costello et al., 2010; Harris and Whiteway, 2011). However, the poor resolution of deep-water bathymetry data (∼25–100 m pixel size) overlooks smaller-scale complexity (∼0.1–5 m) of the seabed. In addition to being overlooked by vessel acoustic surveys, vertical marine habitats are historically undersampled and rarely visited (e.g., Haedrich and Gagnon, 1991). Recent advances in remotely operated technology and underwater vehicles have now increased our capability for the exploration of deep-sea vertical environments (Wynn et al., 2014; Huvenne et al., 2018). In particular, Remotely Operated Vehicles (ROVs) offer the potential to map vertical habitats with a fine-scale resolution (Huvenne et al., 2012, 2018; Robert et al., 2017). The selection of the appropriate configuration of the mapping equipment is crucial, as a downward-facing orientation can limit the acquisition of fine-scale features of steep environments in digital terrain models (Huvenne et al., 2016). Outcrops and overhanging features obstruct downward acoustic measurements in complex vertical habitats (Robert et al., 2017) meaning forward-facing data acquisition is optimal for the terrain reconstruction of vertical features (Yoerger et al., 1997).
At centimetric scales, photogrammetry methods integrate biological information with the fine-scale heterogeneity and the complexity of the benthic habitat (Gerdes et al., 2019; Price et al., 2019; Girard et al., 2020; Lim et al., 2020). Acoustic data acquisition can achieve 3D reconstructions of the terrain from a decimeter to a meter resolution over larger areas for the same amount of time than photogrammetry methods (Robert et al., 2017; Huvenne et al., 2018). Recent studies using forward-looking MBES mounted on ROVs have retrieved digital models of near-vertical walls of >60,000 m2. They have been used to assess the geomorphology of the vertical walls to investigate landslide processes (Huvenne et al., 2016) and in relation to the small-scale distribution of biological communities for ecological studies (Huvenne et al., 2011; Robert et al., 2017).
Substrate properties (e.g., grain size and stability) are important features affecting cold-water coral and sponge community composition and density (Wilborn et al., 2018; De Clippele et al., 2019). MBES offers the opportunity to quantify the backscatter echo intensity as a proxy for substrate roughness, composition and texture. The backscatter corresponds to the overall “inner and micro-scale” material properties of the seabed (Jackson and Briggs, 1992; Brown and Blondel, 2009; Micallef et al., 2012). So far, this aspect of acoustic vertical mapping has not yet been investigated, nor has it been used for the study of substrate characteristics and their distribution at vertical geomorphological features.
This study uses acoustic data collected with a MBES front-mounted onto a ROV at a deep-sea wall located offshore Western Greenland with the aim to (i) improve the workflow to obtain well-navigated vertical bathymetry and retrieve substrate information, (ii) map out the habitat diversity by applying an unsupervised habitat mapping method based on abiotic terrain variables and (iii) test if the unsupervised abiotic classes contain different benthic communities characterized with ROV photography.
On July 20th 2017, the ROV Isis was deployed from the RRS Discovery during the DY081 expedition (Dive 333), and explored the terrain of an underwater wall off the Greenland west coast (63°51.9′N, 53°16.9′W) in the Labrador Sea (Figure 1A; Hendry, 2017; Hendry et al., 2019). The vertical feature represented a portion of a north-facing cliff which marked the transition between a 900 m-deep glacial trough and the more elevated seabed of the Greenland continental shelf (Figure 1B). This site was then selected to investigate habitat characteristics following an unsupervised habitat mapping approach using small-scale descriptors derived from a near-vertical terrain mapped in high resolution during the Isis Dive D334 of July 21st 2017.
Figure 1. (A) Location map of the study site on the W Greenland continental margin. The red boxes indicate the position of the underwater wall (63°51.9′N, 53°16.9′W) in the Labrador Sea, where the dives D333 and D334 of the ROV Isis took place. The bathymetry displayed was acquired on board the RRS Discovery (DY081; Hendry, 2017; Hoy et al., 2018). The background bathymetry (contour lines) was retrieved from ETOPO (NOAA). The wall marks the boundary between a cross-shelf glacial trough and the Greenland continental shelf. (B) 3D repositioning of the deep-sea wall according to the shipboard bathymetry (DY081; Hendry, 2017; Hoy et al., 2018). Panel (B) was computed on ArcScene (v. 10.8.1). (C) The underwater wall with segments representing the ROV tracks carried out at depths of 740, 765, and 790 m with a multibeam echosounder (MBES) front-mounted on the underwater vehicle Isis. The ROV was piloted 25 m off the vertical terrain with headings of: 137° (green lines) and 214° (red lines).
A portion of the underwater wall was mapped using a Reson7125 multibeam echosounder (MBES; 400 kHz, max. 140° swath angle, 512 beams) front-mounted onto the ROV Isis piloted from the RRS Discovery (Dive D334). Over 2h40, the ROV performed 7 survey lines at a constant distance (25 m) parallel to the wall. Horizontal survey lines were achieved at three depths, 25 m apart (Figure 1C). They were undertaken with two different headings (i.e., 137 and 214°) to ensure better coverage of the different parts of the wall, as the terrain displayed different orientations (Figure 1C). In total, seven survey lines were carried out by keeping Isis’ attitude as constant as possible. The MBES system was operated through the Seabat7K software, while the data were recorded with PDS2000, v.3.9. Supplementary Figure 1 details the workflow followed to create the vertical terrain point cloud.
The depth of Isis was recorded by a Parascientific Digiquartz pressure sensor. The position of the ROV was recorded at a frequency of < 1 Hz with an Ultra Short Baseline acoustic positioning system (Sonardyne USBL) and a Doppler Velocity Log (DVL). The USBL has a positioning error of 1% of the vehicle depth. At great depth, this can result in noisy ROV positioning. The DVL is an inertial system that uses dead-reckoning to calculate the ROV position. Over time, this may result in a gradual drift of the ROV navigation. The DVL positions are therefore characterized by high precision but lower accuracy than the USBL (e.g., Supplementary Figure 2). Reconstructing the terrain model therefore required merging of the USBL and DVL navigation to best reconstruct the fine-scale topography (Kwasnitschka et al., 2013; Huvenne et al., 2018). Corrected navigation was acquired by adding the coordinates recorded by the DVL to the average offset between the DVL and the USBL recordings, calculated using a 180-s interval rolling average (e.g., Supplementary Figure 2).
Acoustic data files were converted from the .pds format to .s7k files using PDS2000 (version 3.7), and then transferred into the CARAIBES software (Ifremer) for computing of the terrain point cloud of the wall. As established by Huvenne et al. (2016) and Robert et al. (2017), smoothed navigation coordinates were transformed to a metric coordinate reference system (UTM Mercator) for rotation in R (version 3.2.3; R Core Team, 2013) in order to simulate a conventional downward-looking configuration for processing the acoustic data, as to date no acoustic processing software offers the option of processing forward-looking MBES data. Furthermore, attitude data were transformed to comply with the new downward-looking configuration of the navigation (see in Huvenne et al., 2016).
The datasets of the survey lines recorded with similar heading were merged after aberrant soundings were manually removed in CARAIBES. This resulted in two 0.3 m-resolution point clouds, one for each part of the wall, which were exported as point clouds in .txt and back-rotated to their initial reference system in R (Robert et al., 2017). The software CloudCompare (v.2.11; 2019) was used to spatially combine the point clouds collected with different ROV headings. Small lateral adjustments (<10 m) had to be made as slight offsets of latitude and longitude arose between both point clouds, possibly as a result of the smoothing operations of the navigation.
Backscatter intensity was corrected in the Seabat7k software for spherical spreading and absorption losses based on the water temperature and salinity at depth. The acoustic signal amplitudes recorded in the .s7k files did not represent the actual reflectivity in dB, but nominal values (i.e., no unit). The backscatter extraction with the function Epremo of CARAIBES simply relays that information while the function Ereamo performs the projection in the 3D space. No correction accounting for the true incidence angle on the seafloor was applied. A mosaic was created using the smoothed and rotated navigation coordinates, and further exported in a point cloud with a resolution of 0.3 m. The backscatter was also back-rotated in R, and merged with the bathymetry point cloud by averaging the four nearest backscatter values based on the X,Y,Z coordinates of the bathymetric points.
For further information on the bathymetry and backscatter extraction workflow in CARAIBES, Supplementary Figure 1 details the complete processing workflow.
Topographic descriptors were computed using a kernel radius centered on each point of the point cloud using different kernel radii to account for multi-scale variability of the terrain (Ismail et al., 2015). Topographic descriptors were calculated using Kernel radii of 0.9, 3, and 9 m, representing approximately an exponential series starting from the 0.3 m initial resolution of the point cloud. The maximum kernel size was constrained by the average extent of our study area and represented 1/15th of the height of the vertical wall (e.g., Robert et al., 2017). Topographic descriptors were chosen to reflect the bathymetry (depth), the steepness (slope), the variability (roughness and Terrain Ruggedness Index, TRI), orientation (northness and eastness), curvature (Gaussian and mean curvatures) and relative topographic position (Bathymetric Position Index, BPI) of the terrain in addition to the backscatter values which were used as a proxy for substrate physical characteristics (Wilson et al., 2007; Brown et al., 2011). Normal vectors were computed with a quadric function to derive multi-scale topographic variables (Table 1) and were transformed to “dip/dip direction” for computing the slope and the aspect from which the roughness, the mean and the Gaussian curvatures were derived in CloudCompare following Robert et al. (2017). Terrain Ruggedness Index (TRI), Orientation and Topographic Position Index (TPI) were computed in R [R Core Team, 2013; code provided from Robert et al. (2017)]. Abiotic descriptors were calculated for each point of the point cloud. This produced the input dataset for the subsequent clustering: a matrix where each point (i.e., rows) were assigned a specific depth, longitude, latitude, backscatter intensity and its terrain derivatives values (i.e., columns).
Unsupervised habitat mapping was achieved following a procedure established by Verfaillie et al. (2009) and modified by Ismail et al. (2015) and Hogg et al. (2016). The distribution of each variable was centered on a zero mean and scaled to a unit variance to give each input variable the same weight in a Principal Component Analysis (PCA). PCA is useful to reduce the number of variables into a new set of linearly independent variables called Principal Components (PCs). PCs consist of a linear combination of the initial variables hence discarding collinearity of the variables. Only PCs with eigenvalues > 1 were retained for the clustering analysis following the Kaiser-Guttman criterion (Legendre and Legendre, 1998). Varimax rotation was performed on the retained PCs resulting in Rotated Components (RCs) which were used as input data for the clustering analysis (package ‘psych’; Revelle and Revelle, 2015). Orthogonal rotation improves the PCs’ independence by maximizing the variance shared among items related to one factor therefore enabling easier interpretation of the factor loading pattern.
In unsupervised classification, a critical step is to define the optimal number of clusters to consider in the analysis. Typically, indices based on the proportion of variance explained by a given number of clusters are used in order to find a tradeoff between the model output complexity (i.e., number of clusters) and the clusters inertia (i.e., variability of observations in relation to the cluster center indicated by the within-cluster sum of squares; WSS). The Elbow and Caliński-Harabasz (C-H; Caliński and Harabasz, 1974) criteria were calculated over a range from 2 to 15 clusters in order to determine the optimal number of clusters (Milligan and Cooper, 1985; Milligan, 1996). The Elbow criterion aims to identify the optimal number (K) of clusters based on a decrease, or a local maximum in the gradient, of the total WSS when increasing the number of clusters (Legendre and Legendre, 1998). The C-H criterion seeks to find a local maximum in the ratio of the between-cluster sum of squares and the WSS as the number of clusters is increased (package vegan; Oksanen et al., 2013).
The RCs were used as input variables for an unsupervised clustering. The K-means clustering method (Lance and Williams, 1967; MacQueen, 1967) has been extensively used for classifying features of the seabed (e.g., Legendre et al., 2002; Verfaillie et al., 2009). The K-means algorithm first randomly positions K cluster centers (Hartigan, 1975; Hartigan and Wong, 1979; Milligan and Cooper, 1987). Subsequently, (i) every data point is assigned temporarily to the closest center in the Euclidean space defined by the RCs, and (ii) each cluster center is then repositioned to the average coordinates of the temporary cluster. Both operations (i) and (ii) are repeated iteratively until the positions of the cluster centers converge below a chosen threshold.
As the cluster centers converge to fixed coordinates, the distance between each individual sample and each cluster centroid is calculated as a measure of the similarity of the sample to each cluster (Bezdek, 1974). The membership of each point to its cluster can be expressed as a distance ratio (Burrough et al., 1997; Lucieer and Lucieer, 2009) by the following expression adapted by Ismail et al. (2015).
where μik is the membership value of the i-th data point to cluster k, which results in , dik is the distance between the i-th point and the cluster center k in the Euclidean space built by the RCs, n is the number of clusters defined in section 2.4.
An evaluation of the certainty of assigning the point i to the cluster k and not to another is performed using the confusion index (CI; Burrough et al., 1997). The CI is expressed as the ratio between the data point memberships with the second-closest cluster and the cluster to which it was allocated by the K-means clustering.
Where μ(max–1)_i is the membership value of the point i with the second-closest cluster center in the Euclidean space of the RCs, while μ(max)_i is the membership value of that same point with the closest cluster center (i.e., to which it was assigned by the K-means clustering algorithm). The CI holds the property to tend to 0 when the membership value for the cluster to which it was allocated is high whereas it tends to 1 when the distance-based allocation of one point to the cluster was not well justified compared to the distance with the second-closest cluster.
The abiotic clusters represent an unsupervised summary of a combination of environmental factors. Unsupervised clusters therefore describe the multidimensional environmental space that the fauna experiences and that may potentially contribute to driving community differences. Starting from this hypothesis, we tested for significant differences between a-priori unsupervised abiotic clusters in terms of community composition metrics derived from photograph annotations. In other words, the null hypothesis posits that there was no difference of assemblage composition among unsupervised clusters.
The biological data were extracted from seabed images collected during DY081, Dive D333 (Hendry, 2017; Culwick et al., 2020). In total, 159 images were extracted across a depth gradient (715 to 807 m) explored during two vertical transects that crossed the flank of the cross-shelf glacial trough (Figure 2; Broad, 2020; see methodology within). The position of the images corresponded to the area mapped using the forward-facing MBES during Dive D334. Images of the wall were collected every 30 s using the ROV Isis at an approximate horizontal distance from the wall of 2.5 m. The ROV is equipped with a forward-facing camera “Scorpio” (Insite Pacific Inc.) which is offset from the ROV frame at a down-facing angle of 22.5° and carries parallel lasers spaced 0.1 m apart. Due to the near-vertical orientation of the substrate, estimations of seabed area were calculated as if the images were obtained from a down-facing lens across a flat substrate. Annotation of marine megafauna and estimation of the seabed area within each image were carried out using the online BIIGLE 2.0 platform (Langenkämper et al., 2017). A morphospecies approach was used to characterize the diversity of the epibenthic megafauna, as standardized taxonomic classification of species from deep-sea imagery is not always accurate (Howell et al., 2019).
Figure 2. Front view of the high-resolution (0.3 m) terrain point cloud of the underwater wall marking the boundary between a trough and the West Greenland continental shelf (63°51.9′N, 53°16.9′W). The depth ranges from –685 to –820 m and is displayed as a color gradient. The deep-sea cliff terrain was mapped using a forward-looking MBES mounted onto the ROV Isis (Dive D334) and is displayed as a point cloud using the software CloudCompare. Pink dots locate the position of the ROV when taking seabed groundtruthing pictures (Dive D333) used to assess for assemblage differences among abiotic clusters.
The area of the point cloud that corresponded with the position of each image was spatially identified by projecting a 2.25 m2 square in the 3D space, originating from centralized coordinates recorded by the ROV USBL. This area was consistent with the average area captured by seabed images (2.33 m2). The ROV heading, combined with the sum of the pitch and the inclination of the Scorpio camera, were used to orientate the projection of the 2-dimensional footprint of the initial image squares onto the point cloud, delimiting the estimated field of view recorded by the camera. The terrain points within each field of view did not always display a homogenous affiliation to a particular K-means cluster, therefore we applied a majority filter to create a single assignment of each photograph to a majority cluster.
In many cases, individual images of the seabed are not representative of localized species composition, as they sample too small an area (Benoist et al., 2019). Therefore we found it necessary to compile composite replicate samples to accurately account for faunal patchiness over scales larger than captured in single images (Benoist et al., 2019). Individual images were assigned to a majority cluster established by the method described in section “Generation of Point Cloud Majority Clusters.” Following Broad (2020), images were then pooled at random within their respective majority cluster and aggregated into composite samples representing a seabed area of 20 m2 (±SD, 1.30 m2). The aim was to pool the fauna according to similar conditions they experience within the multivariable environmental space (i.e., environmental proximity) rather than spatial proximity (Benoist et al., 2019; Broad, 2020). Morphospecies abundances were summed in each composite sample. To test for differences in the biological assemblages characterizing each majority cluster, morphospecies abundance data within composite samples were Hellinger transformed and investigated with nonmetric multidimensional scaling plots (nMDS) using a Bray-Curtis dissimilarity matrix calculated in R with the vegan package (Oksanen et al., 2013). An Analysis of Similarities (ANOSIM) and Similarity Percentages (SIMPER) were calculated in PRIMER Version 7 to identify significant differences between majority clusters and the morphospecies responsible for pairwise dissimilarity (Anderson et al., 2008).
The high-resolution point cloud (0.3 m average resolution, 292,577 points; Figure 2) characterized the morphology of the wall. The average topography of the point cloud displayed a slope of 60° (±SD, 18°) oriented north, extending from a depth of 820 to 685 m. The area mapped was ca. 276 m wide with a planar area of 35,880 m2. Groundtruthing pictures are positioned in the terrain point cloud in Supplementary Figure 3 to visualize coinciding fine-scale features and different terrain habitat types.
The deepest part of the wall exhibited a smoother slope of ∼50° at 780 to 818 m depth (Figure 3A), stretching over the whole width of the wall (>200 m). This illustrated a homogeneous horizontal geomorphic transition within the wall (Figure 2). Above that smooth depth band, the underwater cliff displayed areas with steeper slopes reaching on average 60°, but with local gradients up to 90°.
Figure 3. Spatial distribution of terrain derivatives at the finest scale of calculation: (A) Slope (0.9 m), (B) Terrain Ruggedness Index (0.9 m), (C) Bathymetric Position Index (0.9 m), (D) Roughness (3 m), (E) Gaussian curvature (3 m), (F) Mean curvature (3 m), (G) Eastness (3 m), (H) Northness (3 m).
The upper cliff was characterized by a more heterogeneous relief with near-vertical areas (Figure 3A) and zones with slopes < 45°, resulting in higher values of the TRI and roughness variables in areas of a few square meters (Figures 3B,D). The BPI was rather homogeneous throughout the wall, but it underlined elongated near-horizontal features corresponding to transitions between areas with different slopes presented above (Figure 3C). The Gaussian curvature, although it displayed a few localized high values, was generally low (Figure 3E), in contrast to the mean curvature (Figure 3F) which did not exhibit such a homogeneous pattern.
The underwater cliff was not characterized by a homogeneous orientation (Figures 3G,H). Areas with a distinct orientation demonstrated the presence of elongated and protruding features visible (25 m width) in the cliff (Figure 2). Lower backscatter values on the upper sections of the point cloud suggested sediment accumulation (Figure 4). In fact, lower backscatter intensities are typically associated with finer-grained and well-sorted substrata, while higher backscatter intensities are correlated with coarse or hard substrata. These low-backscatter areas also extended on the sides of the protruding features, appearing like incisions in the backscatter map (Figure 4). They may be interpreted as local sediment buildups originating from sediment flow processes.
Figure 4. Spatial distribution of the backscatter intensity retrieved from the MBES. The gray scale refers to untransformed, nominal values retrieved from CARAIBES (Ifremer) with darker gray depicting lower backscatter intensity. Low backscatter intensity indicates a substrate characterized by a combination of smooth and/or soft properties of the “inner” material.
The point cloud displayed fine-scale vertical stripes or ‘ribbing,’ perpendicular to the ROV survey tracks. Such across-track artifacts can arise when mapping the terrain, particularly when using high-frequency acoustic sonar and high ping rates, and can be caused by several types of dynamic errors related to the time series recordings of the attitude sensors and the sonar’s relative angle (Hugues Clarke, 2003). These regular artificial stripes can also arise from noisy USBL recordings in the case of underwater vehicles (e.g., Robert et al., 2017) and can be removed through post-processing using cosine filters. However, meter-scale 3-dimensional structures were observed in images of bedrock veneer indicating an unsupervised filtering could clean out real terrain features that may be important for ecological studies. Hence this was not applied here.
Other artifacts arose when calculating terrain derivatives such as the slope (Figure 3A) and the mean curvature (Figure 3F) in the form of sections of the cliff displaying highly heterogeneous values. We inferred two different causes for these issues. Firstly, the merging of the two point clouds was not perfect as small-scale offsets (∼m) in the reconstructed point clouds remained. This resulted in higher spatial variability of the terrain derivatives along the section where the two point clouds overlapped. Secondly, many sections exhibiting high local variability in the terrain descriptor values coincided with areas with high eastness (Figure 3G) and low northness (Figure 3H) but also with low point density (Supplementary Figure 4). Therefore, we hypothesize these artifacts to be related to the orientation of the cliff: as the ROV kept a constant heading during survey lines, sections of the cliff that were not locally facing the sonar swath were more overlooked as fewer beams were scanning these areas. This explains a locally poorer resolution of the point cloud, which makes it more sensitive to variability in the data when calculating the terrain descriptors with a given Kernel radius size. Similarly, low backscatter intensity (Figure 4) also locally corresponded with low-density areas, and may be caused by the local orientation of the cliff away from the sonar (Supplementary Figure 4).
The Principal Component analysis (PCA) was performed on 10 terrain variables calculated at different scales (Table 1). Five RCs with eigenvalues > 1 were retained and explained 58% of the total variance. Factor loads are displayed in the component’s matrix (Table 2) and allow to investigate the correlation between the terrain variables and the RCs retained. Factor loads (Table 2) rarely exceeded a value of 0.5 which indicates a poor one-to-one relationship (Hogg et al., 2016). Terrain variables computed at different scales displayed similar factor loads. Overall, except for the northness and the backscatter, all variables displayed an exclusive relationship with the RCs. The slope and the TRI accounted for the highest factor loads of RC1 (Table 2); backscatter for RC2; eastness for RC3; TPI for RC4; backscatter and roughness for RC5. The mean and the Gaussian curvatures did not exhibit high loads in the five RCs retained by the K-means algorithm.
Table 2. Component matrix showing correlation between the Varimax rotated principal components (RC) and the terrain input variables computed at different scales.
A K-means clustering was performed on 292,557 data points with the five RCs. The Elbow criterion exhibited a decrease in the gradient of the WSS at 4 clusters (Supplementary Figure 5). The C-H criterion confirmed this observation with a maximum at 4 and 6 clusters (Supplementary Figure 5). We favored the least-complex clustering result with a low number of groups (i.e., four clusters).
The four clusters provided by the unsupervised method of data partitioning were mapped in the 3D space as each point of the point cloud was assigned to one of the four clusters (Figure 5). Broadly speaking, clusters T1, T2 and T3 were related to different depth bands, also characterized by differences in slope and backscatter. Cluster T4 exhibited a more discontinuous spatial distribution (Figure 5), suggesting it was related to variability in terrain characteristics at the scale of the point cloud resolution (i.e., 0.3 m).
Figure 5. Spatial distribution of the four abiotic clusters computed with a K-means clustering based on depth, backscatter intensity and terrain derivatives. Colors refer to points assigned to one of the habitat clusters (T1–T4).
The terrain characteristics of each cluster can also be described using violin plots in order to link differences in the data distribution and range of each input variable to each cluster (Figure 6). All data in this section are also presented with their mean ± standard deviation in the table in Supplementary Table 1. As noted above, depth appeared to be an important variable in constraining the clustering of the point cloud of the underwater cliff. T1 was characterized by the shallowest portion of the vertical wall (−733 ± 19 m) whereas T3 was positioned in the deepest areas (−781 ± 26 m; Figure 6) and T2 was located at intermediate depths (−758 ± 22 m). T4 did not occur in a preferential depth range (−751 ± 27 m; Figures 5, 6). Low backscatter values of T1 (36.1 ± 7.2, nominal units) possibly described a different substrate in comparison to T2, T3 and T4 (>50 ± 6, nominal units; Figure 6). T3 contained terrain data with smoother slopes (44.7 ± 12.6°) and lower TRI (0.29 ± 0.06 m), while T2 reached the highest values (70.9 ± 12.5° and 0.37 ± 0.04 m; Figure 6). The slopes of T1 and T4 were much more variable but still higher in average than those of T3. T4 showed higher and more variable values of roughness (59.83 ± 36.31 10–2 m) compared to T1 which exhibited the second-highest roughness (28.69 ± 27.79 10–2 m; Figure 6). The BPI did not show any clear distinction between clusters notably due to its high variability within clusters. Similarly, the curvatures displayed a relatively similar distribution (Figure 6) although the distinction between T1–2–3 and T4 was more pronounced in the case of the Gaussian curvature, with the latter being less positively skewed in the case of T4 (Figure 6). Eastness and northness were distributed in an opposite way overall, while no distinct patterns could be observed between clusters (Figure 6).
Figure 6. Violin boxplots showing the distribution of terrain variables for each cluster. In the box of the violin boxplot, the middle line is the median, the lower and the upper box boundaries are the first and third quartiles. No statistical outliers are presented. These abiotic variables were used as input variables for the PCA and subsequent K-means clustering that computed the clusters T1–T4. Cluster colors are synchronized with those displayed in Figure 5. Terrain variables presented are A. Depth (0.3 m) [m], B. Backscatter (0.3 m) [nominal values], C. Slope (0.9 m) [°], D. Terrain Ruggedness Index (TRI, 0.3 m) [m], E. Roughness (3 m) [m], F. Bathymetric Position Index (BPI, 0.9 m) [m], G. Mean curvature (3 m) [m–1), H. Gaussian curvature (3 m) [m–1], I. Eastness (3 m) [–], J. Northness (3 m) [–]. Scales of computation are presented in parentheses and units of the abiotic variable is specified in square brackets as well as in the subtitle of the figure panels.
Confusion between clusters can be monitored using the distribution of the CI values (Table 3). On average, no clear distinction characterized the CI distribution of the different clusters although T4 reached the highest mean CI and T2 held the lowest mean CI followed by T3 (Table 3).
Confidence in the clustering outcome can also be assessed considering the spatial distribution of the CI values (Figure 7). The lower part of the cliff displayed very low CI demonstrating a clear distinction between T2 and T3 in this area (Figure 7). This deeper depth band also exhibited some small-scale variation of CI (i.e., abrupt increase) coinciding with spatial transitions between T2 and T3 (Figures 5, 7). These features correspond to local changes of the topography suggested by the slope spatial distribution (Figure 3A) and other bathymetry derivatives (TRI, BPI, curvatures; Figure 3). The upper part of the cliff displayed higher CI values overall (Figure 7) coinciding with a mixed spatial arrangement of the clusters in small-scale patches. This supports the interpretation of a more heterogeneous habitat in this area.
Figure 7. Spatial distribution of CI (Confusion Index) values calculated for the four abiotic clusters. The color gradient refers to uncertainty of clustering based on the membership of a point to the cluster it was assigned by the K-means algorithm. Value of 1/0 (yellow/blue) refers to high/low uncertainty.
The wall supported a diverse community of generalist boreal benthic fauna. Occurring in high abundance were encrusting demosponge morphotypes, crinoids, ophiuroids and soft coral species in the family of Nephtheidae. A number of specialist ecosystem engineers (e.g., the scleractinian cold-water coral Desmophyllum pertusum) were observed in isolated patches on rocky outcrops but remained rare in comparison to the generalist community (Table 4). Supplementary Figure 3 illustrates different species and associated habitats found on the wall.
Table 4. Results of ANOSIM and SIMPER analysis on a Bray-Curtis dissimilarity matrix of transformed morphospecies abundance data.
Characterization of epibenthic megafauna observed in the majority clusters showed a general partitioning of the community mapped in the point cloud (Figure 8). However, the low ANOSIM global R statistic did not indicate a strong aggregation of the assemblages within the clusters (ANOSIM Global R: 0.54, p = 0.001; Table 4). The ANOSIM global significance could be attributed to the cluster T4 which was characterized by the exclusive presence of D. pertusum and a reduced abundance of Drifa glomerata. Pairwise analyses also indicated T1 and T2 and T1 and T3 communities were similar in composition (p ≥ 0.05, Table 4). However, despite the larger distribution of data points within T1 (Figure 8), only T2 and T3 were significantly different (p < 0.01). The increased abundance of Acesta sp. clams (T2, Table 4) and of the carnivorous sponge Asbestopluma pennatula (T3, Table 4) appeared to be contributing the most toward the dissimilarity. Echinoderm morphospecies that contributed to pairwise dissimilarities (Table 4) were likely driven by high abundance values promoting their contribution toward dissimilarity.
Figure 8. Non-metric MDS ordination of Hellinger transformed Bray-Curtis dissimilarity of assemblage compositions across the wall. Factors T1–T4 refer to the K-means majority clusters. Cluster colors are synchronized with those displayed in Figure 5.
This study successfully mapped and characterized the fine-scale topography of a vertical wall located in deep Greenland waters (760 m). Additionally, our study also extracted the backscatter information from the MBES data and used it together with terrain variables calculated in the 3D space, to create the first habitat map of a deep-sea vertical wall. The subsequent comparison with the faunal communities identified in groundtruthing images indicated that the initial unsupervised classification resulted in habitat categories holding ecological relevance.
Global bathymetry maps are the essential input information for various disciplines such as hazard studies, ocean circulation models, seafloor engineering and marine conservation (Wölfl et al., 2019). However, by 2015 less than 18% of the seabed had been mapped with a resolution of 1 km prompting a global endeavor to acquire, standardize and share bathymetric maps (e.g., Seabed2030; Mayer et al., 2018). Still, most of those mapping initiatives are carried out at fairly coarse resolutions (∼100–500 m pixel size or more). This means that the true heterogeneity of the seabed usually remains underestimated (Costello et al., 2010). At finer scales, terrain characterization at a meter-scale resolution is of importance for ecological investigations. For example, the presence of features increasing seabed roughness can have an influence on ecological modeling output (Robert et al., 2017).
While shipboard bathymetry of the Greenland margin provided a 25 m-pixel map of the area (Figure 1; Hendry, 2017; Hoy et al., 2018), the bathymetric and backscatter map supplied by the ROV reached ∼100 times that resolution. A total of 2h40 were needed to map 35,880 m2 of vertical surface with a resolution of 0.3 m. This is in the same order of magnitude as other studies that mapped deep-sea vertical cliffs with a high resolution (<1 m) using forward-looking acoustic sonar for ecological studies (e.g. 9,000 to 15,000 m2 per hour in Robert et al., 2017). Fine-scale geomorphological descriptions have applications for studying geohazard events (e.g., Sichi et al., 2005; Huvenne et al., 2016; Carter et al., 2018). This study identified different geomorphic facies over a range of spatial scales (e.g., 2 horizontal bands >200 m wide, with distinct steepness, 25 m protruding features, meter-scale heterogeneity revealed by unsupervised cluster T4) that provide new insights in the geomorphology of the flank of a deep-sea glacial trough, and that could not be mapped from the shipboard MBES data. These geomorphic features result from differential erosion processes affecting on the long term the geomorphology of the bedrock exposed. The terrain will in turn affect the benthic community composition by shaping local dynamics of the sediment and of the currents and by influencing the stability of the terrain (e.g., friability; Edinger et al., 2011; Robert et al., 2017, 2020). Finally, backscatter data acquired together with multibeam bathymetry at a resolution under a meter have potential in ecological modeling studies focusing on fine-scale influences of terrain heterogeneity usually captured with imagery (e.g., Wilborn et al., 2018; Corbera et al., 2019; De Clippele et al., 2019) or in spatial modeling of dynamic sedimentary processes (e.g., Huvenne et al., 2007; Lastras et al., 2011). While understanding of these processes requires combination with larger-scale investigations, this case study demonstrates current abilities for more extensive mapping combined with a decimeter-scale resolution, even if survey time at the study site currently remains a limiting factor in such deep-sea investigation.
Establishing a robust link between backscatter echo intensity and seabed properties usually requires groundtruthing information to establish what property actually drives the relative spatial differences in backscatter response (e.g., by using images, Micallef et al., 2012; Lucieer et al., 2013; using sediment cores, Lo Iacono et al., 2008; De Falco et al., 2010; and geomorphological maps, Lucieer and Lamarche, 2011) since the backscatter response results from a combination of factors that remain difficult to disentangle without groundtruthing validation (i.e., seabed roughness and substrate properties such as grain size and porosity; Jackson and Briggs, 1992). Interpretation of spatial differences in the backscatter at deep underwater cliffs can therefore be challenging since they can result from confounding variables poorly described in these environments. Images of the seabed did show slight differences in seabed type that could affect the acoustic backscatter (e.g., presence of pebbles, thin layers of sediment) while the effect of dense biogenic structures such as D. pertusum framework can have an influence on < 1 m resolution backscatter (e.g., Masson et al., 2003, see Supplementary Figure 3 for localization of the mentioned features). However, calibrating quantitatively the link with the backscatter would have required sampling or a specific groundtruthing investigation as images only picture the superficial layer of the substrate, while backscatter intensity is affected by the substratum characteristics down to a certain depth (the so-called ‘volume effect,’ depending on acoustic frequency; Lurton and Lamarche, 2015). Nowadays, with improvement of sonar technology, there is a greater interest to integrate backscatter as a substrate surrogate in investigations aiming to characterize the seabed, as shown by recent effort for relating quantitatively the backscatter with the seabed properties (Brown and Blondel, 2009; Lucieer and Lamarche, 2011). Backscatter post-processing usually requires geometric and radiometric corrections to remove artifacts produced by the operating device settings (Lamarche et al., 2016; Lurton et al., 2018). While these steps were not tackled in the framework of forward-looking acoustic acquisition at vertical terrains, this work remains a first attempt to aim for backscatter extraction. This study opens space for further development to characterize the backscatter response of vertical features using acquisition and processing tools specifically tailored to vertical mapping configurations (see recommendations in Lurton and Lamarche, 2015; Lamarche and Lurton, 2018).
Other limitations have been identified. In the same way that downward-looking sonar overlooks fine-scale details of steeply sloping terrains, vertical cliffs exhibiting different orientations cannot be evenly mapped using a single ROV heading, as was illustrated by the merging of two sections of the cliff here in this study. To overcome this issue, separate point clouds can be acquired from survey lines with different heading orientations. The data for each survey section are processed separately and back-rotated, after which they can be merged in the overall point cloud. Occasionally small offsets build up between the separate sections. If the point clouds overlap in relatively homogeneous areas displaying only smaller-scale features, these offsets can trigger local inaccuracies in terrain descriptors. This can have an influence on the unsupervised habitat mapping, especially with clustering algorithms relying on variance partitioning methods.
The reason for these small point cloud offsets remains uncertain, but we suggest it may arise from artifacts or inaccuracies occurring in the navigation and attitude recordings. Although pre-processing of underwater vehicle navigation (Rigby et al., 2006; Batista et al., 2012; Kwasnitschka et al., 2013) and attitude (Hugues Clarke, 2003) allows to optimize the quality of fine-scale terrain reconstruction, the latter is intrinsically dependent on the data quality primarily acquired by motion sensors (i.e., spatial accuracy, lower recording time step than acoustic soundings and temporal synchronization). Accuracy and precision of underwater vehicle positioning in the deep sea remains nevertheless a major technological challenge that may particularly affect high-resolution terrain reconstruction efforts. Noisy USBL navigation data of underwater vehicles can create abrupt artifacts in the MBES bathymetry that may lead to inaccurate fine-scale terrain models. In this study, we merged the overall accuracy of the USBL navigation with the precision of the DVL records, to achieve the optimal navigation dataset to avoid terrain artifacts in a vertical reconstruction. To our understanding, this was a necessary step in the workflow of acoustic data processing since the decisions to remove soundings at the manual cleaning stage remain difficult to make, particularly in complex terrain. Meter-scale features such as outcrops could easily be erased from the point cloud which could lead to a reduction of the terrain complexity. Similarly, navigational uncertainty can remain between separate ROV dives (e.g., between the MBES and photography dives), and can cause difficulties in linking groundtruthing data to acoustic datasets.
In this study, we applied an unsupervised clustering method, as proposed by Verfaillie et al. (2009), to partition the terrain descriptors in a reasonable number of categories displaying distinct characteristics based on the computation of RCs. The cluster analysis delineated four abiotic groups or so-called ‘potential habitats’ characterized by depth, backscatter, slope and roughness. These groups revealed (with high clustering confidence) contiguous zones of the cliff geomorphology even if no information on the spatial autocorrelation of the variables was provided to the cluster algorithm. The habitats included the ‘talus’ located at the bottom of the cliff, the steepest section of the cliff, the upper, more sedimented parts, and a few large protruding geomorphological features. Mapping such geomorphological features is of importance when characterizing the vertical habitat, particularly of sessile species, as they directly influence other aspects of the abiotic environment (e.g., sedimentation, slope, hydrodynamics). They may also reflect some of the geological processes (e.g., erosion) that shaped the vertical cliff as a result of its geological composition (Edinger et al., 2011).
Some aspects of the K-means partitioning method may constrain the interpretation of the habitat mapping outcome. Firstly, the K-means clustering method is based on spherical partitioning resulting in the computation of clusters of similar size in the multidimensional environmental space. This may not always be useful if delineating terrain groups with different sizes is required (Hogg et al., 2016). Density-based clustering (e.g., DBSCAN; Ester et al., 1996) is an alternative approach as it is capable of identifying patterns with arbitrary sizes in datasets even containing noise and outliers (Khan et al., 2014). Secondly, some clusters did locally exhibit patchy and discontinuous distributions also linked with lower confidence levels nested in the upper part of the underwater cliff. The clustering algorithm works on examining the best data partitioning according to the input variables’ variance but not on their spatial coherence and continuity. Spatial coherence and continuity can be met with a clustering method that accounts for spatial proximity between data points (e.g., ST-DBSCAN; Birant and Kut, 2007). Recurrent terrain patterns could also be investigated using approaches based on signal decomposition (e.g., Empirical Orthogonal Function; Preisendorfer and Mobley, 1988).
As a last step in this study, we tested the ecological relevance of the unsupervised habitat categories summarizing a multivariable environment (i.e., depth, terrain and substrate) with the biological information provided by groundtruthing imagery. Abiotic clusters are regularly used as a proxy to reflect the habitat of certain species or groups of species (Brown et al., 2011), but this assumption requires validation in poorly understood ecosystems such as the deep Greenland waters studied here. Although some terrain descriptors may be greatly affected by biogenic structures (e.g., cold-water coral reefs will affect backscatter at < 1 m resolution, Masson et al., 2003; terrain heterogeneity at 0.1 m resolution, Huvenne et al., 2011), such biogenic structures themselves create habitat for other species, and hence can be considered part of the initial habitat characterization, particularly if it has to be based on remote sensing data, with little or no groundtruthing data available for quantitative validation.
Several clusters did show differences in assemblage composition (Figure 8), although sometimes only explained by few species. Being a rare species, D. pertusum drove most of the significant differences related to the high-roughness habitat class T4. D. pertusum live framework was observed at rocky outcrops which is comparable with previous observations made at steep terrains (Huvenne et al., 2011; Pearman et al., 2020). Patches of Acesta sp. grew attached to the hard substrate of vertical features similar to sightings reported in Northeast Atlantic canyons (Johnson et al., 2013; Robert et al., 2017; Pearman et al., 2020). However, community composition differences were not strong, nor was any particular species predominantly contributing to assemblage dissimilarity. For example, on the one hand, D. glomerata showed higher abundances in the high-roughness cluster T4, while on the other hand it still co-occurred with A. pennatula, which was more sighted on smoother terrain with low backscatter (T1). Linked together, these results suggest assemblage differences explained by a few rarer species occurring at particular terrain features, whereas the small extent of the study area may have contributed to assemblage similarity as the sessile communities tended to overlap in space. A more extensive characterization of the wall communities is required to confirm these patterns whereas species distribution models may be useful to investigate specific spatial distributions independently from the rest of the community.
Linking abiotic habitats with assemblage composition using unsupervised habitat mapping may not always result in strong delineation of communities, but is one of the only ways to carry out a first-level interpretation of a seabed area if biological data are sparse or non-existent (Hogg et al., 2018). At least 3 additional factors possibly played a role in the outcome of this community analysis. (1) The sampling, which took place before the habitat mapping work was completed, was not designed to collect images according to the spatial arrangement of the abiotic clusters. Vertical ROV tracks aimed to investigate communities across depths, but this can mislead the validity of comparing biological communities across other abiotic terrain categories because of a difference in sampling strategy between both. The terrain investigation resulted in abiotic clusters which did not fully distribute according to depth. Other terrain characteristics, not distributed along depth, also influenced the unsupervised clustering patterns and did influence the presence of a few particular species (e.g., slope, roughness, backscatter intensity). (2) Terrain descriptors that did not strongly explain clustering results may be more important in driving assemblage composition, for example by influencing current exposure through orientation or by positioning the species at keystone structures (e.g., overhangs revealed by BPI (Robert et al., 2017, 2020). Other environmental factors which were not measured may have been at play but remained excluded from the K-means clustering (e.g., hydrodynamics, physical and chemical properties of the water column). Furthermore, at such a fine scale, biological factors act on assemblage structure, such as biotic interactions or the presence of structuring species (Buhl-Mortensen et al., 2010). In ecology, spatial autocorrelation in species distribution is a natural reality triggered not only by the presence of the correct ecological niche, but also by migration of mobile species and dispersion of sessile organisms (Legendre and Legendre, 1998). Therefore, observations may not always reflect the ecological niche a species can occupy. (3) Habitat clusters are discrete categories that may not reflect transitional patterns between communities; especially abiotic clusters were locally patchy and discontinuous. Considering only the majority cluster located within an image area may overlook fine-scale habitat heterogeneity. Local diversity of habitats may influence the presence of a more diverse panel of species than if the seabed habitat were more homogeneous. So-called ‘fuzzy classification’ approaches that reflect point membership in the point cloud to several clusters may allow a more realistic mapping of transitional habitats or ‘ecotones’ (Lucieer and Lamarche, 2011).
This study presents an application of high-resolution habitat mapping of vertical cliffs in the deep sea. Pre-existing information about deep-water vertical walls in most cases is sparse or non-existent because of their inaccessibility and the difficulties in mapping such terrains with conventional methods. In such cases, an initial unsupervised habitat mapping approach is appropriate to obtain a first-level interpretation of the habitat structure (Hogg et al., 2018). The spatial distribution of clusters will then be useful to objectively and rapidly inform the user regarding the heterogeneity/similarity of the habitat. This initial information can help the definition of a robust sampling design that optimizes habitat representativeness of the area of interest (LaFrance et al., 2014), which is especially useful during exploration activities or in poorly characterized environments such as in deep waters, where sampling time is limited and costly. Defined on that objective information, groundtruth sampling will help to build a refined habitat map by validating the level of (dis)similarity and ecological relevance between habitats delineated by the first seafloor classification.
This study demonstrated our ability to capture fine-scale seabed characteristics of vertical habitats in the deep sea using forward-looking acoustic survey methods (bathymetry and backscatter) on underwater platforms. Unsupervised habitat mapping based on K-means clustering was applied to delineate similarities across the vertical seabed and to summarize the multidimensionality of the benthic substrate variables. The latter revealed terrain differences linked with geomorphological features >200 and >25 m in size, and meter-scale heterogeneity (e.g., roughness). Groundtruthing photographs partitioned among the abiotic clusters indicated dissimilarities of benthic community composition. However, these differences remained attenuated therefore calling for a more representative sampling and characterization of the faunal assemblages based on a better sampling scheme. Simultaneously, this study stresses the need for an investigation into alternative clustering approaches that may describe the environmental conditions in a more adequate way. Furthermore, the extraction of the backscatter intensity at vertical underwater terrain remaining at its infancy, it demonstrates the need for further development to ensure accurate acquisition of this proxy of the substrate properties.
Nevertheless, this investigation demonstrates the need and possibilities of this method for multidisciplinary investigations of vertical features at fine scales in geology, ecology and habitat prediction, especially when adding the backscatter information. Meter-scale unsupervised terrain mapping remains a cost-effective and objective tool to inform relevant and representative field sampling strategies in remote environments where no a priori knowledge is available, such as at deep underwater cliffs. However, acquisition of robust groundtruthing data remains necessary to fully characterize the faunal communities, especially in the undersampled deep-sea benthic habitat of Greenland waters. In practice, uncertainties in ROV positioning and attitude recording are still some of the major challenges when working in this type of environment and with high-resolution terrain characterization. While we proposed post-processing methods that help to limit error propagation, positional errors can still affect the habitat mapping outcomes and possibly constrain the spatial accuracy when linking abiotic and biotic datasets. Further investigations and development in vehicle navigation are needed to improve high-resolution habitat mapping in complex deep-sea environments.
The original contributions presented in the study are included in the article/Supplementary Material, further inquiries can be directed to the corresponding author/s. Original data (terrain and biotic variables) are provided https://doi.org/10.1594/PANGAEA.931687.
VH conceived the study. VH and KH carried out the field work. LV performed abiotic data analyses and drafted the manuscript. EB conducted biotic data analyses. All authors contributed to editing the final manuscript.
This work was initially part of a thesis supported by the International Master of Science in Marine Biological Resources (IMBRSea; LV). IMBRSea is a Joint Master’s Degree under Erasmus Mundus coordinated by Ghent University (FPA 574482-EPP-1-2016-1-BE-EPPKA1-JMD-MOB). Funding for DY081 was from the European Research Council (ERC Starting Grant 678371 ICY-LAB). VH was supported by the NERC National Capability Program CLASS (Grant No. NE/R015953/1). EU H2020 project iAtlantic (Grant No. 818123) also supported VH and LV. Paper publication costs were supported by NERC National Capability funding.
The authors declare that the research was conducted in the absence of any commercial or financial relationships that could be construed as a potential conflict of interest.
We acknowledge the Captain and the crew of the RRS Discovery as well as the ROV Isis technical team of the National Oceanography Centre (NOC). We gratefully thank Tabitha Pearman, Tim Le Bas, Catherine Wardell, Guillem Corbera, Michael Faggetter, David Price, and Pål Buhl-Mortensen for their help and advice and Erik Simon-Lledó for his assistance on the BIIGLE platform. We would like to thank Katleen Robert who provided R scripts for preprocessing and Evan Edinger for reviewing the initial version of our work. We appreciated the constructive feedback of two reviewers who improved the earlier versions of the manuscript.
The Supplementary Material for this article can be found online at: https://www.frontiersin.org/articles/10.3389/fmars.2021.669372/full#supplementary-material
Althaus, F., Williams, A., Kloser, R. J., Seiler, J., and Bax, N. J. (2012). “Evaluating geomorphic features as surrogates for benthic biodiversity on Australia’s western continental margin,” in Seafloor Geomorphology as Benthic Habitat, eds P. T. Harris and E. K. Baker (Amsterdam: Elsevier), 665–679. doi: 10.1016/B978-0-12-385140-6.00048-7
Anderson, M. J., Gorley, R. N., and Clarke, K. R. (2008). PERMANOVA+ for PRIMER: Guide to Software and Statistical Methods. Plymouth: PRIMER-E.
Batista, P., Silvestre, C., and Oliveira, P. (2012). “GES integrated LBL/USBL navigation system for underwater vehicles,” in Proceedings of the 2012 IEEE 51st IEEE Conference on Decision and Control (CDC), (Maui, HI: IEEE), 6609–6614. doi: 10.1109/CDC.2012.6426614
Benoist, N. M. A., Morris, K. J., Bett, B. J., Durden, J. M., Huvenne, V. A. I., Le Bas, T. P., et al. (2019). Monitoring mosaic biotopes in a marine conservation zone by autonomous underwater vehicle. Conserv. Biol. 33, 1174–1186. doi: 10.1111/cobi.13312
Bezdek, J. C. (1974). Numerical taxonomy with fuzzy sets. J. Math. Biol. 1, 57–71. doi: 10.1007/BF02339490
Birant, D., and Kut, A. (2007). ST-DBSCAN: an algorithm for clustering spatial-temporal data. Data Knowl. Eng. 60, 208–221. doi: 10.1016/j.datak.2006.01.013
Broad, E. (2020). The Community Structure of Epibenthic Megafauna on the Continental Slope in Southwest Greenland, Master’s Thesis. Southampton: The University of Southampton, 63.
Brooke, S., and Ross, S. W. (2014). First observations of the cold-water coral Lophelia pertusa in mid-Atlantic canyons of the USA. Deep. Res. Part II 104, 245–251. doi: 10.1016/j.dsr2.2013.06.011
Brooke, S. D., Watts, M. W., Heil, A. D., Rhode, M., Mienis, F., Duineveld, G. C. A., et al. (2017). Distributions and habitat associations of deep-water corals in Norfolk and Baltimore Canyons, Mid-Atlantic Bight, USA. Deep Res. Part II 137, 131–147. doi: 10.1016/j.dsr2.2016.05.008
Brown, C. J., and Blondel, P. (2009). Developments in the application of multibeam sonar backscatter for seafloor habitat mapping. Appl. Acoust. 70, 1242–1247. doi: 10.1016/j.apacoust.2008.08.004
Brown, C. J., Smith, S. J., Lawton, P., and Anderson, J. T. (2011). Benthic habitat mapping: a review of progress towards improved understanding of the spatial ecology of the seafloor using acoustic techniques. Estuar. Coast. Shelf Sci. 92, 502–520. doi: 10.1016/j.ecss.2011.02.007
Buhl-Mortensen, L., Vanreusel, A., Gooday, A. J., Levin, L. A., Priede, I. G., Buhl-Mortensen, P., et al. (2010). Biological structures as a source of habitat heterogeneity and biodiversity on the deep ocean margins. Mar. Ecol. 31, 21–50. doi: 10.1111/j.1439-0485.2010.00359.x
Buhl-Mortensen, P., Buhl-Mortensen, L., and Purser, A. (2017). “Trophoic ecology and habitat provision in cold-water coral ecosystems,” in Marine Animal Forests, eds S. Rossi, L. Bramanti, A. Gori, and C. Orejas (Cham: Springer), 919–944. doi: 10.1007/978-3-319-17001-5
Burrough, P. A., Van Gaans, P. F. M., and Hootsmans, R. (1997). Continuous classification in soil survey: Spatial correlation, confusion and boundaries. Geoderma 77, 115–135. doi: 10.1016/S0016-7061(97)00018-9
Caliński, T., and Harabasz, J. (1974). A dendrite method for cluster analysis. Commun. Stat. 3, 1–27.
Carter, G. D. O., Huvenne, V. A. I., Gales, J. A., Lo, C., Marsh, L., Ougier-simonin, A., et al. (2018). Ongoing evolution of submarine canyon rockwalls; examples from the Whittard Canyon, Celtic Margin (NE Atlantic). Prog. Oceanogr. 169, 79–88. doi: 10.1016/j.pocean.2018.02.001
Corbera, G., Lo, C., Gràcia, E., Grinyó, J., Pierdomenico, M., Huvenne, V. A. I., et al. (2019). Ecological characterisation of a Mediterranean cold-water coral reef: Cabliers Coral Mound Province (Alboran Sea, western Mediterranean). Prog. Oceanogr. 175, 245–262. doi: 10.1016/j.pocean.2019.04.010
Costello, M. J., Cheung, A., and De Hauwere, N. (2010). Surface area and the seabed area, volume, depth, slope, and topographic variation for the world’s seas, oceans, and countries. Environ. Sci. Technol. 44, 8821–8828. doi: 10.1021/es1012752
Costello, M. J., Mccrea, M., Freiwald, A., Lundälv, T., Jonsson, L., Bett, B. J., et al. (2005). “Role of cold-water Lophelia pertusa coral reefs as fish habitat in the NE Atlantic,” in Cold-water Corals and Ecosystems, eds A. Freiwald and J. M. Roberts (Berlin: Springer-Verlag), 771–805.
Culwick, T., Phillips, J., Goodwin, C., Rayfield, E. J., and Hendry, K. R. (2020). Sponge density and distribution constrained by fluid forcing in the deep sea. Front. Mar. Sci. 7:395. doi: 10.3389/fmars.2020.00395
Davies, J. S., Guillaumont, B., Tempera, F., Vertino, A., Beuck, L., Ólafsdóttir, S. H., et al. (2017). A new classification scheme of European cold-water coral habitats: implications for ecosystem-based management of the deep sea. Deep Res. Part II Top. Stud. Oceanogr. 145, 102–109. doi: 10.1016/j.dsr2.2017.04.014
De Clippele, L. H., Huvenne, V. A. I., Molodtsova, T. N., and Roberts, J. M. (2019). The diversity and ecological role of non-scleractinian corals (Antipatharia and Alcyonacea) on scleractinian cold-water coral mounds. Front. Mar. Sci. 6:184. doi: 10.3389/fmars.2019.00184
De Falco, G., Tonielli, R., Di Martino, G., Innangi, S., Simeone, S., and Michael Parnum, I. (2010). Relationships between multibeam backscatter, sediment grain size and Posidonia oceanica seagrass distribution. Cont. Shelf Res. 30, 1941–1950. doi: 10.1016/j.csr.2010.09.006
Edinger, E. N., Sherwood, O. A., Piper, D. J. W., Wareham, V. E., Baker, K. D., Gilkinson, K. D., et al. (2011). Geological features supporting deep-sea coral habitat in Atlantic Canada. Cont. Shelf Res. 31, S69–S84. doi: 10.1016/j.csr.2010.07.004
Ester, M., Kriegel, H. P., Sander, J., and Xu, X. (1996). “A density-based algorithm for discovering clusters in large spatial databases with noise,” in KDD’96: Proceedings of the Second International Conference on Knowledge Discovery and Data Mining, (Miinche: AAAI), 226–231. doi: 10.1016/B978-044452701-1.00067-3
Frederiksen, R., Jensen, A., and Westerber, H. (1992). The distribution of the scleractinian coral Lophelia pertusa around the Faroe Islands and the relation to internal tidal mixing. Sarsia 77, 157–171.
Freiwald, A., Beuck, L., Rüggeberg, A., Taviani, M., and Hebbeln, D. (2009). The white coral community in the central mediterranean sea revealed by ROV surveys. Oceanography 22, 58–74. doi: 10.5670/oceanog.2009.06
Gasbarro, R., Wan, D., and Tunnicliffe, V. (2018). Composition and functional diversity of macrofaunal assemblages on vertical walls of a deep northeast Pacific fjord. Mar. Ecol. Prog. Ser. 597, 47–64.
Gass, S. E., and Roberts, J. M. (2006). The occurrence of the cold-water coral Lophelia pertusa (Scleractinia) on oil and gas platforms in the North Sea: colony growth, recruitment and environmental controls on distribution. Mar. Pollut. Bull. 52, 549–559. doi: 10.1016/j.marpolbul.2005.10.002
Gerdes, K., Arbizu, P. M., Schwarz-Schampera, U., Schwentner, M., and Kihara, T. C. (2019). Detailed mapping of hydrothermal vent fauna: a 3d reconstruction approach based on video imagery. Front. Mar. Sci. 6:96. doi: 10.3389/fmars.2019.00096
Girard, F., Sarrazin, J., Arnaubec, A., Cannat, M., Sarradin, P.-M., Wheeler, B., et al. (2020). Currents and topography drive assemblage distribution on an active hydrothermal edifice. Prog. Oceanogr. 187:102397. doi: 10.1016/j.pocean.2020.102397
Gori, A., Orejas, C., Madurell, T., Bramanti, L., Martins, M., Quintanilla, E., et al. (2013). Bathymetrical distribution and size structure of cold-water coral populations in the Cap de Creus and Lacaze-Duthiers canyons (northwestern Mediterranean). Biogeosciences 10, 2049–2060. doi: 10.5194/bg-10-2049-2013
Greene, H. G., Yoklavich, M. M., Starr, R. M., O’Connell, V. M., Wakefield, W. W., Sullivan, D. E., et al. (1999). A classification scheme for deep for seafloor habitats. Oceanol. Acta 22, 663–678.
Haedrich, R. L., and Gagnon, J. M. (1991). Rock wall fauna in a deep Newfoundland fiord. Cont. Shelf Res. 11, 1199–1207.
Hall-Spencer, J., Allain, V., Fossa, J. H., and Copernic, P. N. (2002). Trawling damage to Northeast Atlantic ancient coral reefs. Proc. R. Soc. Lond. Ser. B Biol. Sci. 269, 507–511. doi: 10.1098/rspb.2001.1910
Harris, P. T., and Whiteway, T. (2011). Global distribution of large submarine canyons: geomorphic differences between active and passive continental margins. Mar. Geol. 285, 69–86. doi: 10.1016/j.margeo.2011.05.008
Hartigan, J. A., and Wong, M. A. (1979). A K-means clustering algorithm: algorithm AS 136. Appl. Stat. 28, 126–130.
Hendry, K. R., Huvenne, V. A. I., Robinson, L. F., Annett, A., Badger, M., Jacobel, A. W., et al. (2019). The biogeochemical impact of glacial meltwater from Southwest Greenland. Prog. Oceanogr. 176:102126. doi: 10.1016/j.pocean.2019.102126
Hill, N. A., Lucieer, V., Barrett, N. S., Anderson, T. J., and Williams, S. B. (2014). Estuarine, coastal and shelf science filling the gaps: predicting the distribution of temperate reef biota using high resolution biological and acoustic data. Estuar. Coast. Shelf Sci. 147, 137–147. doi: 10.1016/j.ecss.2014.05.019
Hogg, O. T., Huvenne, V. A. I., Griffiths, H. J., Dorschel, B., and Linse, K. (2016). Landscape mapping at sub-Antarctic South Georgia provides a protocol for underpinning large-scale marine protected areas. Sci. Rep. 6:33163. doi: 10.1038/srep33163
Hogg, O. T., Huvenne, V. A. I., Griffiths, H. J., and Linse, K. (2018). On the ecological relevance of landscape mapping and its application in the spatial planning of very large marine protected areas. Sci. Total Environ. 626, 384–398. doi: 10.1016/j.scitotenv.2018.01.009
Howell, K. L., Davies, J. S., Louise Allcock, A., Braga-Henriques, A., Buhl-Mortensen, P., Carreiro-Silva, M., et al. (2019). A framework for the development of a global standardised marine taxon reference image database (SMarTaR-ID) to support image-based analyses. bioRxiv [Preprint] doi: 10.1101/670786
Hoy, S. K., Hendry, K. R., and Huvenne, V. A. I. (2018). North Atlantic EM-122 multibeam swath bathymetry collected during RRS Discovery cruise DY081DY081 (links to raw files and gridded data). Bremen: PANGAEA, doi: 10.1594/PANGAEA.892825
Hugues Clarke, J. E. (2003). Dynamic motion residuals in swath sonar data: ironing out the creases. Int. Hydrogr. Rev. 4, 6–23.
Huvenne, V. A. I., Georgiopoulou, A., Chaumillon, L., Lo Iacono, C., and Wynn, R. B. (2016). “Novel method to map the morphology of submarine landslide headwall scarps using remotely operated vehicles,” in Advances in Natural and Technological Hazards Research, eds G. Lamarche, J. Mountjoy, S. Bull, T. Hubble, S. Krastel, E. Lane, et al. (Cham: Springer US), 135–144. doi: 10.1007/978-3-319-20979-1_13
Huvenne, V. A. I., Huenerbach, V., Blondel, P., and Le Bas, T. P. (2007). “Detailed mapping of shallow-water environments using image texture analysis on sidescan sonar and multibeam backscatter imagery,” in Proceedings of the International Conference “Underwater Acoustic Measurements: Technologies & Results, eds J. S. Papadakis and L. Bjorno (Heraklion: Foundation for Research & Technology), 879–886.
Huvenne, V. A. I., Pattenden, A. D. C., Masson, D. G., and Tyler, P. A. (2012). “Habitat heterogeneity in the Nazaré deep-sea canyon offshore Portugal,” in Seafloor Geomorphology as Benthic Habitat, eds P. T. Harris and E. K. Baker (Amsterdam: Elsevier), 691–701. doi: 10.1016/B978-0-12-385140-6.00050-5
Huvenne, V. A. I., Robert, K., Marsh, L., Lo Iacono, C., Le Bas, T., and Wynn, R. B. (2018). “ROVs and AUVs,” in Springer Geology, eds A. Micallef, S. Krastel, and A. Savini (New York, NY: Springer US), 93–108. doi: 10.1007/978-3-319-57852-1_7
Huvenne, V. A. I., Tyler, P. A., Masson, D. G., Fisher, E. H., Hauton, C., Hühnerbach, V., et al. (2011). A picture on the wall: Innovative mapping reveals cold-water coral refuge in submarine canyon. PLoS One 6:e28755. doi: 10.1371/journal.pone.0028755
Ismail, K., Huvenne, V. A. I., and Masson, D. G. (2015). Objective automated classification technique for marine landscape mapping in submarine canyons. Mar. Geol. 362, 17–32. doi: 10.1016/j.margeo.2015.01.006
Jackson, D. R., and Briggs, K. B. (1992). High-frequency bottom backscattering: roughness versus sediment volume scattering. J. Acoust. Soc. Am. 92, 962–977. doi: 10.1121/1.403966
Johnson, M. P., White, M., Wilson, A., Würzberg, L., Schwabe, E., Folch, H., et al. (2013). A vertical wall dominated by Acesta excavata and Neopycnodonte zibrowii, part of an undersampled group of deep-sea habitats. PLoS One 8:e79917. doi: 10.1371/journal.pone.0079917
Khan, K., Rehman, S. U., Aziz, K., Fong, S., and Sarasvady, S. (2014). “DBSCAN: Past, Present and Future. in The fifth international conference on the applications of digital information and web technologies,” in Proceedings of the The Fifth International Conference on the Applications of Digital Information and Web Technologies (ICADIWT 2014), (Bangalore: IEEE), 232–238.
Kiriakoulakis, K., Freiwald, A., Fisher, E., and Wolff, G. A. (2007). Organic matter quality and supply to deep-water coral / mound systems of the NW European Continental Margin. Int. J. Earth Sci. 96, 159–170. doi: 10.1007/s00531-006-0078-6
Kohn, A. J., and Leviten, P. J. (1976). Effect of habitat complexity on population density and species richness in tropical intertidal predatory gastropod assemblages. Oecologia 25, 199–210.
Kostylev, V. E., Todd, B. J., Fader, G. B. J., Courtney, R. C., Cameron, G. D. M., and Pickrill, R. A. (2001). Benthic habitat mapping on the Scotian Shelf based on multibeam bathymetry, surficial geology and sea floor photographs. Mar. Ecol. Prog. Ser. 219, 121–137. doi: 10.3354/meps219121
Kwasnitschka, T., Hansteen, T. H., Devey, C. W., and Kutterolf, S. (2013). Doing fieldwork on the seafloor: photogrammetric techniques to yield 3D visual models from ROV video. Comput. Geosci. 52, 218–226. doi: 10.1016/j.cageo.2012.10.008
LaFrance, M., King, J. W., Oakley, B. A., and Pratt, S. (2014). A comparison of top-down and bottom-up approaches to benthic habitat mapping to inform offshore wind energy development. Cont. Shelf Res. 83, 24–44. doi: 10.1016/j.csr.2014.04.007
Lamarche, G., and Lurton, X. (2018). Recommendations for improved and coherent acquisition and processing of backscatter data from seafloor-mapping sonars. Mar. Geophys. Res. 39, 5–22. doi: 10.1007/s11001-017-9315-6
Lamarche, G., Orpin, A. R., Mitchell, J. S., and Pallentin, A. (2016). “Benthic habitat mapping,” in Biological Sampling in the Deep Sea, eds M. R. Clark, M. Consalvey, and A. A. Rowden (Hoboken, NJ: John Wiley & Sons), 80–102.
Lance, G. N., and Williams, W. T. (1967). A general theory of classificatory sorting strategies: 1. Hierarchical systems. Comput. J. 9, 373–380. doi: 10.1093/comjnl/9.4.373
Langenkämper, D., Zurowietz, M., Schoening, T., and Nattkemper, T. W. (2017). BIIGLE 2 . 0 – browsing and annotating large marine image collections. Front. Mar. Sci. 4:83. doi: 10.3389/fmars.2017.00083
Lastras, G., Canals, M., Amblas, D., Lavoie, C., Church, I., De Mol, B., et al. (2011). Understanding sediment dynamics of two large submarine valleys from seafloor data: Blanes and La Fonera canyons, northwestern Mediterranean Sea. Mar. Geol. 280, 20–39. doi: 10.1016/j.margeo.2010.11.005
Legendre, P., Ellingsen, K. E., Bjørnbom, E., and Casgrain, P. (2002). Acoustic seabed classification: improved statistical method. Can. J. Fish. Aquat. Sci. 59, 1085–1089. doi: 10.1139/f02-096
Lim, A., Wheeler, A. J., Price, D. M., O’Reilly, L., Harris, K., and Conti, L. (2020). Influence of benthic currents on cold-water coral habitats: a combined benthic monitoring and 3D photogrammetric investigation. Sci. Rep. 10:19433. doi: 10.1038/s41598-020-76446-y
Lo Iacono, C., Gràcia, E., Diez, S., Bozzano, G., Moreno, X., Dañobeitia, J., et al. (2008). Seafloor characterization and backscatter variability of the Almería Margin (Alboran Sea, SW Mediterranean) based on high-resolution acoustic data. Mar. Geol. 250, 1–18. doi: 10.1016/j.margeo.2007.11.004
Lucieer, V., Hill, N. A., Barrett, N. S., and Nichol, S. (2013). Do marine substrates “look” and “sound” the same? Supervised classification of multibeam acoustic data using autonomous underwater vehicle images. Estuar. Coast. Shelf Sci. 117, 94–106. doi: 10.1016/j.ecss.2012.11.001
Lucieer, V., and Lamarche, G. (2011). Unsupervised fuzzy classification and object-based image analysis of multibeam data to map deep water substrates, Cook Strait, New Zealand. Cont. Shelf Res. 31, 1236–1247. doi: 10.1016/j.csr.2011.04.016
Lucieer, V., and Lucieer, A. (2009). Fuzzy clustering for seafloor classification. Mar. Geol. 264, 230–241. doi: 10.1016/j.margeo.2009.06.006
Lurton, X., Eleftherakis, D., and Augustin, J. M. (2018). Analysis of seafloor backscatter strength dependence on the survey azimuth using multibeam echosounder data. Mar. Geophys. Res. 39, 183–203. doi: 10.1007/s11001-017-9318-3
Lurton, X., and Lamarche, G. (2015). Backscatter Measurements by Seafloor – Mapping Sonars. Guidelines and Recommendations. Eastsound, WA: GEOHAB.
MacArthur, R. H., and Wilson, E. O. (1967). The Theory of Island Biogeography. Princeton, NJ: Princeton University Press, 1.
MacQueen, J. (1967). “Some methods for classification and analysis of multivariate observations,” in Proceedings of the Fifth Berkeley Symposium on Mathematical Statistics and Probability, Vol. 1, eds L. M. Le Cam and J. Neyman (Berkeley, CA: University of California Press), 281–297.
Malecha, P., and Heifetz, J. (2017). Long-term effects of bottom trawling on large sponges in the Gulf of Alaska. Cont. Shelf Res. 150, 18–26. doi: 10.1016/j.csr.2017.09.003
Masson, D. G., Bett, B. J., Billett, D. S. M., Jacobs, C. L., Wheeler, A. J., and Wynn, R. B. (2003). The origin of deep-water, coral-topped mounds in the northern Rockall Trough, Northeast Atlantic. Mar. Geol. 194, 159–180. doi: 10.1016/S0025-3227(02)00704-1
Mayer, L., Jakobsson, M., Allen, G., Dorschel, B., Falconer, R., Ferrini, V., et al. (2018). The Nippon foundation-GEBCO seabed 2030 project: the quest to see the world’s oceans completely mapped by 2030. Geosci. 8, 1–18. doi: 10.3390/geosciences8020063
Micallef, A., Le Bas, T. P., Huvenne, V. A. I., Blondel, P., Hühnerbach, V., and Deidun, A. (2012). A multi-method approach for benthic habitat mapping of shallow coastal areas with high-resolution multibeam data. Cont. Shelf Res. 39, 14–26. doi: 10.1016/j.csr.2012.03.008
Milligan, G. W. (1996). “Clustering validation: results and implications for applied analyses,” in Clustering and Classification, eds P. Arabie, L. J. Hubert, and G De Soete (Singapore: World Scientific Publishing), 341–375.
Milligan, G. W., and Cooper, M. C. (1985). An examination of procedures for determining the number of clusters in a data set. Psychometrika 50, 159–179. doi: 10.1007/BF02294245
Milligan, G. W., and Cooper, M. C. (1987). Methodology review: clustering methods. Appl. Psychol. Meas. 11, 329–354. doi: 10.1177/014662168701100401
Morris, K. J., Tyler, P. A., Masson, D. G., Huvenne, V. A. I., and Rogers, A. D. (2013). Distribution of cold-water corals in the Whittard Canyon, NE Atlantic Ocean. Deep Res. Part II 92, 136–144. doi: 10.1016/j.dsr2.2013.03.036
Mortensen, P. B., Hovland, M. T., Fossa, J. H., and Furevik, D. M. (2001). Distribution, abundance and size of Lophelia pertusa coral reefs in mid-Norway in relation to seabed characteristics. J. Mar. Biol. Assoc. 81, 581–597.
Oksanen, J., Blanchet, F. G., Friendly, M., Kindt, R., Legendre, P., McGlinn, D., et al. (2013). Package “vegan”. Community Ecology Package, Version 2.
Pearman, T. R. R., Robert, K., Callaway, A., Hall, R., Lo Iacono, C., and Huvenne, V. A. I. (2020). Improving the predictive capability of benthic species distribution models by incorporating oceanographic data – Towards holistic ecological modelling of a submarine canyon. Prog. Oceanogr. 184:102338. doi: 10.1016/j.pocean.2020.102338
Preisendorfer, R. W., and Mobley, C. D. (1988). Principal Component Analysis in Meteorology and Oceanography. New York, NY: ScienceOpen.
Price, D. M., Robert, K., Callaway, A., Lo lacono, C., Hall, R. A., and Huvenne, V. A. I (2019). Using 3D photogrammetry from ROV video to quantify cold-water coral reef structural complexity and investigate its influence on biodiversity and community assemblage. Coral Reefs 38, 1007–1021. doi: 10.1007/s00338-019-01827-3
R Core Team (2013). R: A Language and Environment for Statistical Computing. Vienna: R Foundation for Statistical Computing, 201.
Ramirez-Llodra, E., Brandt, A., Danovaro, R., De Mol, B., Escobar, E., German, C. R., et al. (2010). Deep, diverse and definitely different: Unique attributes of the world’s largest ecosystem. Biogeosciences 7, 2851–2899. doi: 10.5194/bg-7-2851-2010
Rigby, P., Pizarro, O., and Williams, S. B. (2006). “Towards geo-referenced AUV navigation through fusion of USBL and DVL measurements,” in Proceedings of the Oceans 2006, (Boston, MA: IEEE), 1–6. doi: 10.1109/OCEANS.2006.306898
Robert, K., Huvenne, V. A. I., Georgiopoulou, A., Jones, D. O. B., Marsh, L., Carter, D. O. G., et al. (2017). New approaches to high-resolution mapping of marine vertical structures. Sci. Rep. 7:9005. doi: 10.1038/s41598-017-09382-z
Robert, K., Jones, D. O. B., Georgiopoulou, A., and Huvenne, V. A. I. (2020). Cold-water coral assemblages on vertical walls from the Northeast Atlantic. Divers. Distrib. 26, 284–298. doi: 10.1111/ddi.13011
Ross, S. W., and Quattrini, A. M. (2007). The fish fauna associated with deep coral banks off the southeastern United States. Deep. Res. Part I 54, 975–1007. doi: 10.1016/j.dsr.2007.03.010
Sichi, O. G., Blondel, P. H., and Gràcia, E. (2005). “Acoustic textures and seafloor characterisation of submarine landslides – an example from the Sw Iberian Margin,” in Boundary Influences In High Frequency, Shallow Water Acoustics, eds N. G. Pace and P. Blondel (Bath: University of Bath), 271–278.
Stewart, P. L., Pocklington, P., and Cunjak, R. A. (1985). Distribution, abundance and diversity of Benthic Macroinvertebrates on the Canadian continental shelf and slope of Southern Davis Strait and Ungava Bay. Arctic 38, 281–291.
Vassallo, P., Bianchi, C. N., Paoli, C., Holon, F., Navone, A., Bavestrello, G., et al. (2018). A predictive approach to benthic marine habitat mapping: efficacy and management implications. Mar. Pollut. Bull. 131, 218–232. doi: 10.1016/j.marpolbul.2018.04.016
Verfaillie, E., Degraer, S., Schelfaut, K., Willems, W., and Van Lancker, V. (2009). A protocol for classifying ecologically relevant marine zones, a statistical approach. Estuar. Coast. Shelf Sci. 83, 175–185. doi: 10.1016/j.ecss.2009.03.003
Wheeler, A. J., Bett, B. J., Billett, D. S. M., Masson, D. G., and Mayor, D. J. (2005). “The impact of demersal trawling on northeast Atlantic deepwater coral habitats: the case of the Darwin mounds, United Kingdom,” in Proceedings of the American Fisheries Society Symposium, (Bethesda, MD: American Fisheries Society), 807–818.
Wilborn, R., Rooper, C. N., Goddard, P., Li, L., Williams, K., and Towler, R. (2018). The potential effects of substrate type, currents, depth and fishing pressure on distribution, abundance, diversity, and height of cold-water corals and sponges in temperate, marine waters. Hydrobiologia 811, 251–268. doi: 10.1007/s10750-017-3492-9
Wilson, M. F. J., O’Connell, B., Brown, C., Guinan, J. C., and Grehan, A. J. (2007). Multiscale terrain analysis of multibeam bathymetry data for habitat mapping on the continental slope. Mar. Geod. 30, 3–35. doi: 10.1080/01490410701295962
Wölfl, A. C., Snaith, H., Amirebrahimi, S., Devey, C. W., Dorschel, B., Ferrini, V., et al. (2019). Seafloor mapping – the challenge of a truly global ocean bathymetry. Front. Mar. Sci. 6:283. doi: 10.3389/fmars.2019.00283
Wynn, R. B., Huvenne, V. A. I., Le Bas, T. P., Murton, B. J., Connelly, D. P., Bett, B. J., et al. (2014). Autonomous Underwater Vehicles (AUVs): Their past, present and future contributions to the advancement of marine geoscience. Mar. Geol. 352, 451–468. doi: 10.1016/j.margeo.2014.03.012
Yoerger, D. R., Kelley, D. S., and Delaney, J. R. (1997). Fine-scale three-dimensional mapping of a deep-sea hydrothermal vent site using the Jason ROV system. Int. J. Rob. Res. 19, 1000–1014.
Keywords: marine habitat mapping, deep-water vertical cliff, ROV, multibeam echosounder, terrain point cloud, Greenland glacial trough, suspension-feeding community, underwater exploration
Citation: Van Audenhaege L, Broad E, Hendry KR and Huvenne VAI (2021) High-Resolution Vertical Habitat Mapping of a Deep-Sea Cliff Offshore Greenland. Front. Mar. Sci. 8:669372. doi: 10.3389/fmars.2021.669372
Received: 18 February 2021; Accepted: 04 May 2021;
Published: 22 June 2021.
Edited by:
Andrew J. Davies, University of Rhode Island, United StatesReviewed by:
Benjamin Misiuk, Memorial University of Newfoundland, CanadaCopyright © 2021 Van Audenhaege, Broad, Hendry and Huvenne. This is an open-access article distributed under the terms of the Creative Commons Attribution License (CC BY). The use, distribution or reproduction in other forums is permitted, provided the original author(s) and the copyright owner(s) are credited and that the original publication in this journal is cited, in accordance with accepted academic practice. No use, distribution or reproduction is permitted which does not comply with these terms.
*Correspondence: Loïc Van Audenhaege, bG9pYy52YW5hdWRlbmhhZWdlQGdtYWlsLmNvbQ==
Disclaimer: All claims expressed in this article are solely those of the authors and do not necessarily represent those of their affiliated organizations, or those of the publisher, the editors and the reviewers. Any product that may be evaluated in this article or claim that may be made by its manufacturer is not guaranteed or endorsed by the publisher.
Research integrity at Frontiers
Learn more about the work of our research integrity team to safeguard the quality of each article we publish.