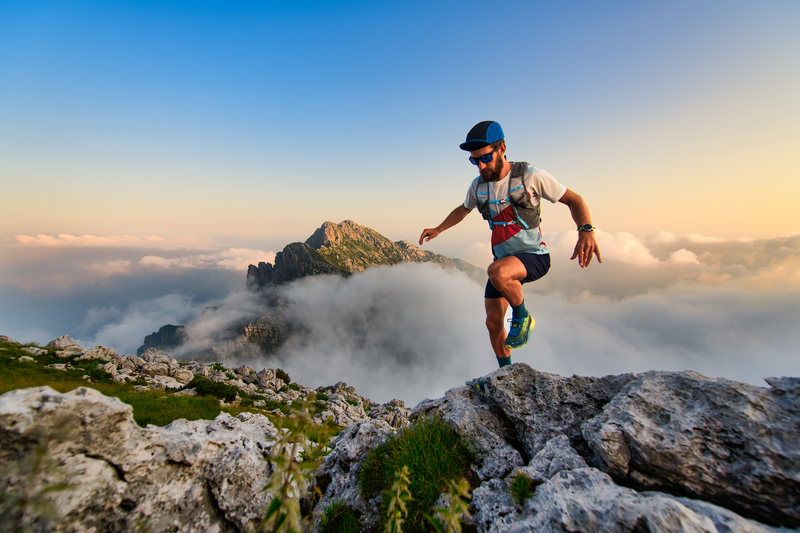
94% of researchers rate our articles as excellent or good
Learn more about the work of our research integrity team to safeguard the quality of each article we publish.
Find out more
ORIGINAL RESEARCH article
Front. Immunol. , 07 February 2025
Sec. Autoimmune and Autoinflammatory Disorders: Autoinflammatory Disorders
Volume 16 - 2025 | https://doi.org/10.3389/fimmu.2025.1458341
This article is part of the Research Topic New Targets and Strategies for the Prevention and Treatment of Organ Fibrosis, Volume III View all 10 articles
Background: Idiopathic pulmonary fibrosis (IPF) is a degenerative respiratory condition characterized by significant mortality rates and a scarcity of available treatment alternatives. Cuproptosis, a novel form of copper-induced cell death, has garnered attention for its potential implications. The study aimed to explore the diagnostic value of cuproptosis-related hub genes in patients with IPF. Additionally, multiple bioinformatics analyses were employed to identify immune-related biomarkers associated with the diagnosis of IPF, offering valuable insights for future treatment strategies.
Methods: Four microarray datasets were selected from the Gene Expression Omnibus (GEO) collection for screening. Differentially expressed genes (DEGs) associated with IPF were analyzed. Additionally, weighted gene coexpression network analysis (WGCNA) was employed to identify the DEGs most associated with IPF. Ultimately, we analyzed five cuproptosis-related hub genes and assessed their diagnostic value for IPF in both the training and validation sets. Additionally, four immune-related hub genes were screened using a protein–protein interaction (PPI) network and evaluated through the receiver operating characteristic (ROC) curve. Lastly, single-cell RNA-seq was employed to further investigate differential gene distribution.
Results: We identified a total of 92 DEGs. Bioinformatics analysis highlighted five cuproptosis-related genes as candidate biomarkers, including three upregulated genes (CFH, STEAP1, and HDC) and two downregulated genes (NUDT16 and FMO5). The diagnostic accuracy of these five genes in the cohort was confirmed to be reliable. Additionally, we identified four immune-related hub genes that demonstrated strong diagnostic performance for IPF, with CXCL12 showing an AUROC of 0.90. We also examined the relationship between these four genes and immune cells. CXCL12 was significantly negatively associated with neutrophils, while CXCR2 was associated exclusively with neutrophils, consistent with our single-cell sequencing results. CTSG showed a primarily positive association with follicular helper T, and SPP1 was most strongly associated with macrophages. Finally, our single-cell sequencing data revealed that in patients with IPF, CXCL12 was highly expressed in the endothelial cell subset (ECs), while SPP1 exhibited high expression in multiple cellular populations. The expression of the CTSG showed statistically significant differences in monocyte macrophages.
Conclusion: The research methodically depicted the intricate interplay among five cuproptosis-related genes, four immune-related hub genes, and IPF, offering new ideas for diagnosing and treating patients with IPF.
Idiopathic pulmonary fibrosis (IPF) represents a long-term, advancing pulmonary condition with scarce therapeutic choices, high mortality, and poor prognosis, with a median survival of only 2.5–3.5 years from the time of diagnosis (1–3). Epidemiologic surveys show that the global incidence of IPF ranges from 0.09 to 1.30 per 10,000 people, with an increasing trend over the years (4). At present, the pathophysiological mechanism of IPF has not been fully elucidated. It has been suggested that the occurrence of IPF may be closely related to persistent or repetitive injury to alveolar epithelial cells. Dysregulated epithelial cells interact with mesenchymal cells, immune cells, and endothelial cells through various signaling mechanisms, which contribute to tissue scarring, modification of the alveolar structure, and irreversible loss of lung function (5). Immune cells play a pivotal role in the onset and progression of fibrogenesis by promoting or exacerbating tissue structural remodeling (6, 7). Continued damage to alveolar epithelial cells leads to an increase in neutrophils and monocytes, triggering inflammatory responses (8). Similarly, macrophages are crucial in the initiation and progression of IPF (9). In addition, the wound healing process involves an inflammatory response that recruits fibroblasts, activates myofibroblasts, and deposits extracellular matrix in the form of collagen and other proteins (10). Although pirfenidone and nintedanib are recommended in the guidelines, their efficacy is limited. Therefore, further exploration of novel therapeutic strategies for IPF is particularly important (11).
Copper is one of the essential trace elements in the human body and plays a key role in many biological processes (12). It is involved in multiple physiological processes, including the regulation of energy metabolism, mitochondrial respiration, and antioxidant activity (13–15). Copper ion levels maintain a dynamic equilibrium, and a loss of this balance can lead to oxidative stress and abnormal cellular autophagy (16, 17), causing a variety of copper or copper ion-related diseases. Cuproptosis is a recently discovered, unique form of cell death. Upon disruption of the mitochondrial respiratory chain, the lipoylated components of the tricarboxylic acid cycle are directly bound by copper ions, forming aggregates that ultimately lead to cell death (13). Furthermore, to date, no studies have demonstrated a relationship between cuproptosis and fibrosis. Previous studies have shown that the signaling pathway mediated by the fibrotic cytokine transforming growth factor β1 (TGF-β1) plays a significant role in lung fibrosis. TGF-β1 reduces Nicotinamide adenine dinucleotide (NADH) and NADH/NAD levels, possibly due to alterations in the tricarboxylic acid cycle, which results in decreased ATP levels and impaired oxidative phosphorylation in lung fibroblasts (18). Therefore, we hypothesized that cuproptosis may play a role in the development of IPF. Genes associated with cuproptosis could serve as new targets for IPF therapy, although their precise mechanisms remain to be elucidated. In this study, we conducted bioinformatics analyses to identify novel biomarkers of IPF that may be associated with cuproptosis and immunity.
The study flow diagram is shown in Figure 1. Five datasets were obtained from the Gene Expression Omnibus database (https://www.ncbi.nlm.nih.gov/geo/). Specifically, GSE24206, GSE35145, GSE53845, and GSE68239 were utilized as the training sets, while GSE70866 served as the validation set. Initially, the four training sets were combined using the R package inSilicoMerging (19). Subsequently, the method proposed by Johnson et al. (20) was applied to eliminate batch effects, resulting in a unified Gene Expression Omnibus (GEO) dataset consisting of 99 samples from 71 IPF patients and 28 normal controls. This unified dataset was then utilized to identify differentially expressed genes (DEGs). In this study, differential analysis was performed using the R package limma (version 3.40.6) to identify genes that exhibit differential expression between various control groups. Genes meeting the criteria of an adjusted p-value < 0.05 and |log2 fold change (FC)| > 1.5 were classified as DEGs. The volcano plot and heatmap displayed the expression data of 92 DEGs. Additionally, the density plot and Uniform Manifold Approximation and Projection (UMAP) plot results indicated the successful elimination of batch effects. The microarray preprocessing outcomes were visualized through a boxplot. Gene ontology (GO) enrichment analysis and Kyoto Encyclopedia of Genes and Genomes (KEGG) pathway analysis were conducted using the org.Hs.eg.db (version 3.1.0) and “clusterProfiler” (version 3.14.3) package in R to explore the biological roles of DEGs. The GO annotation and KEGG pathway analysis were performed with a significance threshold of p < 0.05.
The development of IPF is closely linked to the immune system, and an immune response plays a crucial role at every stage of fibrosis (21). The CIBERSORT tool was used to explore the differences in the proportions of 22 immunocyte types between the IPF group and the controls (22). Using a known reference set, this approach generates a set of gene expression profiles for 22 immune cell subtypes.
Using gene expression patterns, we first calculated Media Absolute Deviation (MAD) for each gene, removed the top 50% of genes with the smallest MAD values, and excluded outlier genes and samples using the weighted gene coexpression network analysis (WGCNA) GoodSamplesGenes approach in the R package. We then constructed a scale-free coexpression network using WGCNA, setting the soft-threshold power to 4. To further analyze the module, we calculated the dissimilarity of module eigengenes, selected a cut line for the module dendrogram, and merged certain modules. In addition, we merged modules with distances less than 0.25, resulting in 11 coexpression modules. The gray module was identified as a collection of genes that could not be assigned to a singular module. Among these, the yellow module was found to be the most relevant for subsequent research projects.
A total of 2,181 cuproptosis-related genes (CRGs) were identified in GeneCard (https://www.genecards.org), and genes with correlation coefficients above the median were selected, resulting in 1,090 genes. Additionally, following the guidelines of Tsvetkov et al. (13), we identified another 2,978 genes associated with cuproptosis. After combining the two gene sets to remove duplicates, a total of 3,538 CRGs were selected for subsequent analysis in this article.
A compilation of immune-related (IRGs) was obtained from the Immunology Database and Analysis Portal (ImmPort, https://www.immport.org/shared/genelists). A total of 24 differently expressed immune-related genes (DEIRGs) were identified by intersecting the previously obtained DEGs with IRGs.
Protein–protein interaction (PPI) network was constructed using the STRING database (http://string-db.org) to identify key genes. Cytoscape software (version 3.9.1) was utilized to visualize the PPI networks, and the Cytoscape plugin CytoNCA was employed to identify genes associated with hub genes.
Each sample was fixed in a 4% neutral formaldehyde solution and embedded in paraffin wax. The tissue was sectioned into 4 µM slices, which were then dewaxed and rehydrated. Initially, the slices were placed in a preheated repair solution at 65°C, heated to 90°C for a 30-min incubation, and subsequently cooled to 70°C. Following this, the slices were washed with PBS and incubated with hydrogen peroxide for 10 min. The primary antibodies used for the analysis included STEAP1 (bs-1901R, Bioss, Beijing, China), CFH (bs-9525R, Bioss, Beijing, China), HDC (bs-1054R, Bioss, Beijing, China), FMO5 (bs13187R, Bioss, Beijing, China), CXCL12 (bs-4938R, Bioss, Beijing, China), CXCR2 (abs-133162, Absin, Shanghai, China), and SPP1 (AF0227, Affinity, Jiangsu, China). The primary antibody reactions were carried out for 30 min, followed by a 20-min reaction with the secondary antibody (PV-6000, OriGene, Wuxi, China). The sections were counterstained with hematoxylin, dehydrated, cleared, and sealed according to conventional protocols. Images were then captured under a light microscope, and cells stained brown were considered positive.
This study included eight human lung specimens, comprising three from healthy controls and five from patients with IPF. The healthy control specimens were derived from residual biopsy specimens deemed unsuitable for lung transplantation, while the IPF samples were obtained during lung transplantation procedures. The inclusion criteria for IPF patients were based on the diagnostic standards set by the American Thoracic Society/European Respiratory Society. All participants provided written informed consent after receiving a comprehensive explanation of the study.
Pulmonary nonparenchymal cells were isolated from fresh lung specimens. Lung samples were sliced into 1–2 mm sheets and incubated in 2 ml of GEXSCOPE® Tissue Dissociation Solution at 37°C for 15 min to maintain warmth. The resulting single-cell mixture was dispensed onto an array and incubated for an additional 15 min. After digestion, filter the sample through a 40 µm sterile mesh filter, and centrifuge the filtrate at 1000 rpm for 5 minutes. Discard the supernatant and resuspend the cells with 1 ml of PBS (HyClone). Add 2 mL of GEXSCOP® Erythrocyte Lysis Buffer (Singleron, Nanjing, China) and allow to stand for 10 minutes at 25°C. Centrifuge at 500 g for 5 minutes, and resuspend the cells using 1 ml of PBS. Then stain with Trypan Blue (Sigma) and count the viable cells and total cells under a microscope.
Single-cell suspensions preserved in PBS at a concentration of 1 × 105 cells/ml were prepared and loaded onto a microfluidic chip. scRNA-seq libraries were constructed using the GEXSCOPE® Single-Cell RNA Library Kit (Singleron Biotechnologies, Nanjing, China) in accordance with the Singleron GEXSCOP® Operating Instructions. The libraries were then diluted to 4 nM and sequenced on the Illumina Novaseq6000 sequencing platform using 150 bp paired-end sequencing mode.
Raw sequencing data obtained from the sequencing run were processed using Singleron’s internal analysis pipeline to generate a gene expression matrix. Briefly, reads1 without poly-T sequences were filtered out, and valid cell barcodes and UMIs were extracted. Reads2 were filtered to remove adapters and poly-A tails (using fastp V1). The data were then aligned and quantified against the reference genome from the Ensembl database using STAR (v2.5.3a) and featureCounts (v1.6.2). The pipeline group reads, UMIs, and genes sharing the same cell barcode and calculates the number of UMIs for each gene in every cell for subsequent analysis. The Seurat package (version 3.0.1) was used for cell type identification and clustering analysis of the RNA sequencing data. The expression matrix was imported into R using the read.table function, and cell clustering analysis was performed using the FindClusters function (with a resolution parameter set to 0.6). DEGs between different samples or continuous clusters were identified using the findMarkers function.
The study was reviewed and approved by the Ethics Committee of The Second Affiliated Hospital of Hainan Medical University.
SPSS 22.0 was used to process and statistically analyze the data. p-values of less than 0.05 were taken as the criterion for statistical significance. Comparisons of continuous variables were verified using the Student’s t-test Kruskal–Wallis H test. The Chi-square test or Fisher’s exact test was used for categorical variables. Associations between pivotal genes and immune cells were analyzed using Spearman’s rank sum test or Pearson’s correlation coefficient. Receiver operating characteristic (ROC) analysis was performed using the R package pROC (version 1.17.0.1) to determine the diagnostic accuracy of the hub genes. The results were expressed as the area under the ROC curve (AUROC) with 95% CI. AUROC ≥ 0.9 indicates exceptional discrimination, 0.8 ≤ AUROC < 0.9 indicates excellent discrimination, 0.7 ≤ AUROC < 0.8 indicates acceptable discrimination, 0.5 ≤ AUROC < 0.7 indicates limited diagnostic effectiveness, and ROC = 0.5 indicates no discrimination). Spearman’s rank test or Pearson’s correlation coefficient was used to analyze the associations between hub genes and immune cells.
After filtering the GEO microarray datasets, we retained four datasets: GSE24206, GSE35145, GSE53845, and GSE68239 (Figure 2A). These were merged to create an internal dataset. The box plots illustrate the distribution of differences and confirm the standardization of the internal datasets (Figure 2B). The results from the primary density studies indicate a high level of consistency in the internal dataset after excluding batch effects (Figures 2C, D). Furthermore, the UMAP plot demonstrates a convergence of data distribution across datasets following the elimination of batch effects (Figures 2E, F).
Figure 2. DEG dataset collection and pre-processing. (A) Detailed information about the collected datasets. (B) Boxplot of normalized microarray data. (C, D) Density plots showing differences in sample distribution before and after debatching. (E, F) UMAP visualizations illustrating how samples clustered before and after the removal of the batch effect.
DEGs were analyzed in RNA samples from IPF and normal controls using the “limma” software package, identifying a total of 92 DEGs, with 41 downregulated and 51 upregulated genes. A volcanic graph was generated using a 1.5-fold change criterion (Figure 3A). Figure 3B illustrates the heatmap for DEGs. To investigate the potential functions of these genes, GO and KEGG enrichment pathway analyses were conducted using the R clusterProfiler package. The KEGG analysis revealed that the DEGs were enriched in the following pathways: cytokine–cytokine receptor interaction, hematopoietic cell lineage, and mineral absorption (Figure 3C). The GO-BP analysis (Figure 3D) showed significant enrichment in the immune system process, immune response, metal ion homeostasis, and cation homeostasis. In the GO-CC analysis (Figure 3E), we observed enrichment in the extracellular matrix, cell surface, and extracellular space. Furthermore, the GO-MF enrichment analysis (Figure 3F) revealed fibronectin binding, Wnt-protein binding, interleukin-1 receptor activity, and transforming growth factor beta-activated receptor activity. These findings suggest that the DEGs play a crucial role in IPF and warrant further investigation.
Figure 3. Functional and pathway enrichment analysis of DEGs. (A) Volcano plot of DEGs: blue nodes represent downregulation in IPF, red nodes represent upregulation, and gray nodes represent no significant difference from controls. (B) Heatmap of 92 IPF-related DEGs. (C) Gene ontology (GO) molecular function pathway. (D) GO biological processes pathway. (E) GO cellular component pathway. (F) GO molecular function pathway.
Since immune cells play a key role in the development and initiation of fibrosis, we analyzed immune cell infiltration. Layered histograms display the abundance distribution of 22 types of immune cells across each sample (Figure 4A). Different immune cell types are represented by distinct colors, with the height of each color indicating the proportion of cells. Figure 4B highlights a significant difference in the expression levels of 10 different immune cell marker types. Six immune cell markers (memory B cells, follicular helper T cells, activated NK cells, M1 macrophages, resting dendritic cells, and resting mast cells) were significantly upregulated in the IPF group. Concurrently, the expression levels of plasma cells, dormant NK cells, M1 macrophages, and neutrophils were reduced compared to the control group.
Figure 4. (A) Stacked histogram comparing immune cell percentages between IPF and control samples. (B) Boxplot showing the abundance of 22 immune cell types.
To study the key genes in depth, we selected a soft threshold of 4 (Figure 5A), developed a gene coexpression network utilizing WGCNA technology, and identified the modules most closely related to IPF (Figure 5B). In total, 11 gene modules were obtained, with the yellow module showing a significant correlation with IPF (correlation coefficient = 0.63, p < 0.001) (Figure 5C). The yellow module, identified as the key module, contains 291 genes. Figure 5D displays the scatter plot of module eigengenes in the yellow module.
Figure 5. (A) The power index of 4 was chosen as the appropriate soft threshold, achieving a scale-free coexpression network. (B) The branches of the dendrogram correspond to 11 gene modules. (C) The correlation coefficients and corresponding p-values between each module and IPF. (D) Scatter plot of module eigengenes in the yellow module.
We identified 2,978 CRGs from a previous study (13). A total of 2,181 CRGs were extracted from GeneCards, and 1,090 genes were obtained after taking the median number. After merging the two genes and removing duplicates, we ultimately ended up with 3,538 CRGs.
Five cuproptosis-related hub genes (NUDT16, FMO5, CFH, HDC, and STEAP1) were identified by crossing the candidate genes obtained from the DEGs, CRGs, and WGCNA models (Figure 6A). Figure 6B displays the comprehensive expression patterns of the hub genes when comparing IPF samples with normal samples. CFH, HDC, and STEAP1 exhibited prominent expression in IPF, while the expression of NUDT16 and FMO5 was reduced in the IPF group (all p < 0.001). The ROC curve showed that the AUROC for NUDT16 was 0.92 (95% CI = 0.86–0.98), with sensitivity and specificity of 0.79 and 0.92 (Figures 6C, H). For FMO5, the AUROC was 0.90 (95% CI = 0.81–0.98), and the sensitivity and specificity were 0.86 and 0.86 (Figures 6D, H). The AUROC for CFH in the diagnosis of IPF was 0.87 (95% CI = 0.80–0.95), with sensitivity and specificity of 0.96 and 0.65 (Figures 6E, H). The AUROC of HDC was 0.86 (95% CI = 0.77–0.94), with sensitivity and specificity of 0.86 and 0.75 (Figures 6F, H). The sensitivity, specificity, and AUROC of STEAP1 were 0.86, 0.68, and 0.78 (95% CI = 0.70–0.88), respectively (Figures 6G, H).
Figure 6. (A) Venn diagram showing the intersection of diagnostic markers obtained from the three algorithms. Performance of the five hub genes in diagnosing IPF in the validation set. (B) Expression differences of the five hub genes in IPF and control groups. (C–G) ROC curves of the five hub genes in IPF and control groups. (H) Diagnostic value of the five hub genes for differentiating between IPF and control groups. (I) Correlation between the five hub genes. PPV, positive predictive value; NPV, negative predictive value; AUROC, area under the receiver operating characteristic curve. ****p < 0.0001.
We also performed a correlation analysis of the five hub genes (Figure 6I). The results showed that NUDT16 was only positively correlated with FMO5 (r = 0.70, p < 0.001), significantly negatively correlated with HDC (r = 0.65, p < 0.001), and moderately negatively correlated with CFH (r = 0.56, p < 0.001) and STEAP1 (r = 0.45, p < 0.001). FMO5 was negatively correlated with CFH (r = 0.60, p < 0.001), HDC (r = 0.62, p < 0.001), and STEAP1 (r = 0.66, p < 0.001). HDC was moderately positively associated with STEAP1 (r = 0.43, p < 0.001). In summary, the results suggest that most of the five genes are highly correlated.
The diagnostic efficacy of the five genes in the validation set (GSE70866) was also excellent (Figure 7). The validation group consisted of alveolar lavage fluid specimens, with 196 specimens in total, including 176 from patients with IPF. Figure 7A shows that CFH, HDC, and STEAP1 (all p < 0.001) were significantly overexpressed in IPF, with AUROC of 0.73 (95% CI = 0.62–0.85), 0.77 (95% CI = 0.66–0.88), and 0.75 (95% CI = 0.68–0.83), respectively (Figures 7D, F). However, the expressions of NUDT16 (p = 0.05) and FMO5 (p < 0.001) were significantly lower in the IPF group compared to controls. The AUROC for these genes were 0.63 (95% CI = 0.55–0.71) and 0.79 (95% CI = 0.70–0.87) (Figures 7B, C), which is consistent with the expression we obtained earlier.
Figure 7. Performance of the five hub genes in diagnosing IPF in the validation set. (A) Expression differences of the five hub genes between IPF and control groups. (B–F) ROC curves of the five hub genes in IPF and control groups. (G) Diagnostic value of the four hub genes for differentiating between IPF and control groups. PPV, positive predictive value; NPV, negative predictive value; AUROC, area under the receiver operating characteristic curve.
Not coincidentally, immunohistochemistry of IPF and normal tissues also demonstrated similar results. The immunohistochemistry (IHC) analysis revealed positive expression of CFH, STEAP1, and HDC in the IPF group (all p < 0.01), while FMO5 was overexpressed in the control group (p < 0.05) (Figures 8A, B). Unfortunately, we did not detect NUDT16 expression.
Figure 8. (A) Representative images of immunohistochemical staining of CFH, STEAP1, HDC, and FMO5 in lung tissues. (B) The areas of CFH, STEAP1, HDC, and FMO5. *p < 0.05; **p < 0.001.
Firstly, we extracted 1,793 immune genes associated with IPF from the “Immport Shared Data”. As shown in Figure 9A, 24 DEIRGs were screened after intersecting the 92 DEGs with the 1,793 IRGs. In the KEGG analysis (Figure 9B), the most enriched pathways were cytokine–cytokine receptor interaction, neuroactive and ligand–receptor interaction, and viral protein interaction with cytokine–cytokine receptor, among others.
Figure 9. (A) Venn diagram showing the intersection of diagnostic markers obtained from DEGs and IRGs. (B) Top 10 KEGG pathways. (C) Top 10 gene ontology (GO) biological process pathways. (D) Top 10 GO cellular component pathways. (E) Top 10 GO molecular function pathways.
In GO-BP analysis (Figure 9C), the major pathways were regulation of response to stimulus, defense response, and immune response. The results of enrichment analysis in GO-CC (Figure 9D) revealed enrichment in the extracellular region, an integral component of the plasma membrane, and others. GO-MF analysis (Figure 9E) indicated that the major pathways included signaling receptor activity and cytokine binding, among others.
We used the STRING online website for PPI network analysis. In total, a PPI network containing 15 nodes and 23 edges was obtained. Two of these genes lack association with other molecules and fail to form a molecular network. Altogether, a PPI network comprising 15 nodes and 23 edges was acquired (Figure 10A). The network was configured using the standard threshold (interaction score > 0.4). The Cytoscpe plugin CytoNCA was used to identify hub genes. Hub genes (CXCL12, CXCR2, CTSG, SPP1) were selected based on their top four scores (Figure 10B). Figure 10C shows the value of the four hub genes in the diagnosis of IPF in the training set. CXCL12, CTSG, and SPP1 were significantly upregulated in the IPF group, while CXCR2 was highly expressed in the normal group. Moreover, the AUROC values were 0.92 (95% CI = 0.86–0.97) (Figures 10D, H), 0.74 (95% CI = 0.64–0.85) (Figures 10E, H), 0.79 (95% CI = 0.70–0.88) (Figures 10F, H), and 0.71 (95% CI = 0.59–0.82) (Figures 10G, H). CXCL12 emerged as the gene most strongly associated with IPF.
Figure 10. (A) PPI network showing the interactions of 15 immune-related genes. (B) Cytoscape analysis using the CytoNCA plugin identifying hub genes and their expression differences. (C) Expression differences of the four hub genes between IPF and control groups. (D–G) ROC curves of the four hub genes in IPF and control groups. (H) Diagnostic value of the four hub genes for differentiating between IPF and control groups. PPV, positive predictive value; NPV, negative predictive value; AUROC, area under the receiver operating characteristic curve.
The correlation between four hub genes and immune cells is shown in Figure 11A. CXCL12 was significantly correlated with five cell types, including a significant negative correlation with neutrophils (r = − 0.64, p < 0.001) and a significant positive correlation with resting mast (r = 0.47, p < 0.001). The only notable association observed between CXCR2 and neutrophils was (r = 0.66, p < 0.001). There was an inverse relationship between CTSG and three types of immune cells, most notably with neutrophils (r = − 0.39, p < 0.001), and it was only positively correlated with follicular helper T (r = 0.33, p < 0.001). SPP1 was significantly positively correlated with three immune cells and negatively correlated with two immune cells. The strongest correlation was with Macrophages_M0 (r = 0.58, p < 0.001). Meanwhile, we found a mild correlation between these four genes (Figure 11B).
Figure 11. (A) Matrix correlation between immune-related hub genes and immune cell abundance. (B) Correlation analysis between the four hub genes. ***p < 0.001; ****p < 0.0001.
In the validation set (GSE70866), the expression levels of genes CXCL12, CTSG, and SPP1 were consistent with those in the experimental set (Figure 12A); however, we regretfully were unable to detect the expression of CXCR2. The diagnostic efficacy of the three genes also yielded excellent results. The AUROC of CXCL12 was 0.66 (95% CI = 0.56–0.76), with sensitivity and specificity of 0.45 and 0.95, respectively (Figures 12B, E). For CTSG, the AUROC was 0.65 (95% CI = 0.52–0.79), and the sensitivity and specificity were 0.96 and 0.35 (Figures 12C, E). The AUROC of SPP1 in the diagnosis of IPF was 0.96 (95% CI = 0.39–0.99), and the sensitivity and specificity were 0.94 and 0.90, respectively (Figures 12D, E). We employed immunohistochemical methods to examine the expression profiles of four genes in both IPF and normal tissues. The results revealed that CXCL12, CTSG, and SPP1 were significantly upregulated in IPF tissues, whereas CXCR2 showed higher expression in normal tissues, which is consistent with our analytical findings (Figures 12F, G).
Figure 12. (A) Expression differences of the five hub genes between IPF and control groups. (B–E) ROC curves of the four hub genes in IPF and control groups. (F) Representative images of immunohistochemical of CXCL12, CTSG, CXCR2, and SPP1 in lung tissues. (G) The areas of the four hub genes. PPV, positive predictive value; NPV, negative predictive value; AUROC, area under the receiver operating characteristic curve. *p < 0.05; **p < 0.01; ***p < 0.001.
In this study, a total of eight lung tissue specimens were collected, comprising five cases diagnosed with IPF who underwent lung transplantation and three cases that underwent pulmonary nodule surgery but were ultimately determined to be benign. After sequencing the lung tissue cell suspensions using the 10× Genomics platform, a total of 90,722 single-cell high-quality data points were obtained. Following quality control and filtering, 75,613 cells were utilized for subsequent analysis (Table 1). After accounting for batch effects across samples, 26 distinct cellular populations were identified (Figure 13A). Based on previous research on canonical cell markers, these 26 cell clusters were categorized into 11 distinct cell types (Figure 13B). Including epithelial cells, endothelial cell subsets (ECs), fibroblasts, mural cells, prolific cells, B cells, plasma cells, T and NK cells, neutrophils, mast cells, and mononuclear phagocytes (MPs). Figures 13C–F depict the expression of immune-related hub genes across various specimens, with cell populations represented through a violin plot.
Figure 13. (A) UMAP plot of all cells from 26 cell clusters. (B) UMAP plot displaying the cell types in the two groups. (C) Gene expression of CXCL12 in IPF and normal groups. (D) Gene expression of CXCR2 in IPF and normal groups. (E) Gene expression of CTSG in IPF and normal groups. (F) Gene expression of SPP1 in IPF and normal groups.
IPF is a chronic, progressive, and devastating disease, primarily characterized by fibrosis, structural deformation, honeycomb lungs, fibrosis of the lamellar lung parenchyma, and the generation of fibroblastic foci, leading to poor prognosis and shorter survival (23). The incidence of IPF has risen in the past few years, with over 5,000 new confirmed cases annually in the UK (24). Aging is a major risk factor for IPF, with incidence rates doubling every decade after the age of 50 (4). Currently, there are no clinically effective treatments to stop or reverse pulmonary fibrosis (25). Most patients experience a gradual decline in lung function, which ultimately leads to severe respiratory failure and can be fatal (1). Due to the variability and uncertainty of IPF in the clinical process, early assessment and intervention of disease progression are particularly important.
Almost all cell types require copper for a multitude of physiological processes, and maintaining the equilibrium of copper in cells is critical for cellular physiology and endurance (26). Abnormalities in copper metabolism, as a novel mode of cell death, are closely linked to the emergence of numerous human illnesses (27–29). In addition, research has indicated that certain fibrotic conditions may be influenced by the levels of copper ions. One reason is that elevated copper ions in cells activate lysine oxidase, enhancing the cross-linking of collagen and elastin (30, 31). Therefore, this study links cuproptosis to the pathogenesis of IPF, with bioinformatics analysis identifying potential crucial genes and investigating prospective treatment targets.
In this study, we investigated the gene expression levels in the normal group and IPF patients using the GEO database, identifying a total of 92 DEGs. GO and KEGG enrichment analyses revealed enrichment in immune system processes, the extracellular matrix, fibronectin binding, transforming growth factor beta-activated receptor activity, and the cytokine–cytokine receptor interaction pathway. In addition, there was a notable difference in the percentage of immune cells between the IPF-affected group and the normal group. Our study showed that six immune cell markers—memory B cells, follicular helper T cells, activated NK cells, M1 macrophages, resting dendritic cells, and resting mast cells—were significantly highly expressed in the IPF group. Consistent with previous research, the expression of memory B cells, follicular T cells, and mast cells was in agreement with the study (32). However, the role of M1 macrophages in the pathogenesis of IPF remains controversial. Our results support the involvement of M1 macrophages in fibrosis. The mechanisms of action of activated NK cells and dormant DC cells in IPF were not identified and warrant further exploration.
Using bioinformatics analysis, we identified five cuproptosis-related hub genes that showed significant differences between the IPF and healthy groups, demonstrating high diagnostic efficacy. These results were validated in the Alveolar Irrigation Validation (GSE70866), further supporting the reliability of our findings.
CFH primarily inhibits the complement substitution pathways by accelerating the attenuation of the complement alternative pathway, specifically the C3-converting enzyme C3bBb, and impairing the production of novel C3b (33). Furthermore, C3b interacts with other proteins to promote the restoration of normal immune system functioning (34). Previous studies have shown that immune disorders drive the pathophysiology of IPF (35). In our study, CFH was highly expressed in the tissues of IPF patients, suggesting it may act as a potential disruptor. These findings lead us to hypothesize that CFH could play a role in IPF as an immune system suppressor. Previous studies have shown that STEAP1 expression is upregulated in lung adenocarcinoma cells, where it regulates cellular epithelial–mesenchymal transition (EMT) through the (JAK2/STAT3) signaling pathway (36). In addition, studies have shown that STEAP1 is closely linked to abnormal copper metabolism (37). However, it is still unclear whether abnormal copper metabolism in epithelial cells plays a role in mediating EMT. Similarly, in lung adenocarcinoma research, factors derived from HDC + pmn-mdscs may influence EMT cell behavior through paracrine models. Blocking these factors inhibits metastasis in lung adenocarcinoma (38). The study suggests that HDC knockout improves the progression of liver fibrosis (39). However, no related literature currently exists regarding IPF, which may offer new directions for future research. NUDT16 is a (deoxygenated) creatine diphosphatase, primarily responsible for protecting cells from the harmful effects of inosine triphosphate (ITP) in the nucleus. The ITP receptor facilitates the transformation of lung fibroblasts into myofibroblasts, potentially contributing to lung fibrosis (40). Our results suggest that NUDT16 is poorly expressed in IPF patients, indicating that NUDT16 may act as a protective factor in the mechanisms of IPF. FMO5, a member of the FMO protein family, is involved in the upregulation of the NRF2-mediated oxidative stress response (41). NRF2 has been shown to attenuate renal fibrosis through the PI3K/AKT signaling pathway (42). Additionally, NRF2 activation has been demonstrated to protect against various lung diseases, including IPF (43). This validates our findings. Furthermore, we analyzed the correlation among these five genes and identified significant synergistic or antagonistic interactions between them.
All stages of IPF involve both innate and adaptive immune responses (21). IRGs are essential for immune cells to respond to immune stimulation and infiltration (44). However, the regulatory mechanism of IPF through IRG expression remains unclear. From the IMMport database, we identified 1,793 IRGs and intersected them with previously identified DEGs, resulting in 24 DEIRGs. KEGG analysis revealed that these DEIRGs are primarily associated with the cytokine–cytokine receptor interaction pathway. GO enrichment analysis indicated the following: defense response, immune system process, and regulation of immune system process (biological processes); extracellular (cellular composition); and enrichment of cytokine binding (molecular function). These findings align with our initial hypothesis. Subsequently, four hub genes (CXCL12, CXCR2, CTSG, and SPP1) were identified in this study using PPI network analysis. Among these, CXCL12, CTSG, and SPP1 were highly expressed in IPF, while CXCR2 was expressed at lower levels, consistent with immunohistochemistry results. Similarly, the AUROC analysis showed a moderate diagnostic capability for these genes.
CXCL12 has garnered widespread attention as the sole ligand of CXCR4, a major chemokine receptor on fibroblasts (45). Research indicates that the CXCL12-CXCR4 axis plays a role in multiple pathological processes of fibrosis, including inflammation, immune responses, epithelial–mesenchymal transitions, and the formation of new blood vessels (46). Our bioinformatics analysis found that CXCL12 expression was upregulated in IPF patients, a finding that was validated by previous studies (47). In our single-cell sequencing, we found that CXCL12 is predominantly enriched in ECs, providing new evidence for exploring IPF. CTSG is a protein-coding gene, and single-cell sequencing showed statistically significant differences in CTSG expression, primarily in monocyte macrophages, which regulate damage and repair in various fibrosis models. A recent study showed that macrophages from both mice and humans promote fibrosis by overexpressing repair mechanisms in alveolar damage (48). The study (49) suggests that CTSG is upregulated in the peripheral blood of IPF patients, consistent with our findings. Previous research (50) reports that macrophages orchestrate fibroblast activation via Spp1, Fn1, and Sema3 crosstalk. SPP1 has been found to play an important role in fibrosis in multiple organs, including the heart, lungs, and skin (51, 52). Our single-cell sequencing revealed significant differences in SPP1 expression in multiple cell types, including ECS, EPI, and NK, with the largest differences observed in EC subsets. Therefore, we hypothesize that SPP1 may regulate fibrosis not only through macrophages but also through ECs, providing a new direction for subsequent analysis. CXCR2 is a receptor for interleukin 8 (IL-8), which mediates neutrophil migration to inflammatory sites (53). Our bioinformatics analysis results suggest that CXCR2 is highly expressed in normal tissues, and single-cell sequencing results further confirm that this high expression is predominantly concentrated in neutrophils. In contrast to some of the current findings (54), we suggest that the high expression of CXCR2 in normal tissues stimulates the recruitment of more neutrophils, rapidly suppresses inflammation, prevents excessive repair of postinflammatory tissue, and thus reduces fibrosis. However, this is merely a hypothesis, and further research is needed to confirm it.
Additionally, we examined the relationship between four specific genes and immune cells. CXCL12 was significantly negatively associated with neutrophils, while CTSG and SPP1 were significantly positively correlated with resting mast cells and macrophages, respectively. CXCR2 was only significantly positively associated with neutrophils, which is consistent with our single-cell sequencing results. A moderate correlation was observed between the four genes. These findings suggest an important association between IPF and IRGs and may provide novel perspectives and directions for future research.
This study has some limitations. First, databases of genes associated with CRGs and IRGs are limited, and more data need to be mined. Second, the single-cell sequencing results are based on single-center clinical specimens with a limited sample size, and additional cases may be needed for validation due to patient heterogeneity.
This study confirms that the development of IPF may be associated with cuproptosis and identifies five key related genes (CFH, STEAP1, HDC, NUDT16, and FMO5). Additionally, we found a strong relationship between IPF and immune cells, leading to the identification of four important genes (FMO5, CFH, HDC, and STEAP1). Single-cell sequencing results further elucidated their expression in relevant cell clusters. Therefore, these selected genes may serve as potential biomarkers and therapeutic targets for future IPF research.
The data supporting the findings of this study are deposited in GEO (http://www.ncbi.nlm.nih.gov/geo), GeneCard (https://www.genecards.org), ImmPort (https://www.immport.org/shared/genelists) database.
The studies involving humans were approved by Ethics Committee of the Second Affiliated Hospital of Hainan Medical University. The studies were conducted in accordance with the local legislation and institutional requirements. The participants provided their written informed consent to participate in this study. Written informed consent was obtained from the individual(s) for the publication of any potentially identifiable images or data included in this article.
JinL: Writing – review & editing, Resources. JW: Writing – review & editing, Funding acquisition, Resources. CJ: Conceptualization, Methodology, Software, Writing – review & editing. JiaL: Writing – original draft. QL: Investigation, Writing – original draft. LZ: Data curation, Writing – original draft. SZ: Supervision, Writing – original draft. QF: Formal analysis, Writing – original draft. YL: Formal Analysis, Writing – original draft. YZ: Validation, Writing – original draft. QN: Validation, Writing – original draft.
The author(s) declare financial support was received for the research, authorship, and/or publication of this article. We thank the Open Foundation of the NHC Key Laboratory of Tropical Disease Control, Hainan Medical University (2020-PT310-009); the National Natural Science Foundation of China (No. 81760005); the Natural Science Foundation of Hainan Province (No. 822QN471 and No. 821QN408); and the project supported by the Hainan Province Clinical Medical Center.
The authors declare that the research was conducted in the absence of any commercial or financial relationships that could be construed as a potential conflict of interest.
All claims expressed in this article are solely those of the authors and do not necessarily represent those of their affiliated organizations, or those of the publisher, the editors and the reviewers. Any product that may be evaluated in this article, or claim that may be made by its manufacturer, is not guaranteed or endorsed by the publisher.
The Supplementary Material for this article can be found online at: https://www.frontiersin.org/articles/10.3389/fimmu.2025.1458341/full#supplementary-material
1. Ley B, Collard HR, King TE Jr. Clinical course and prediction of survival in idiopathic pulmonary fibrosis. Am J Respir Crit Care Med. (2011) 183:431–40. doi: 10.1164/rccm.201006-0894CI
2. Strongman H, Kausar I, Maher TM. Incidence, prevalence, and survival of patients with idiopathic pulmonary fibrosis in the UK. Adv Ther. (2018) 35:724–36. doi: 10.1007/s12325-018-0693-1
3. Raghu G, Collard HR, Egan JJ. An official ATS/ERS/JRS/ALAT statement: idiopathic pulmonary fibrosis: evidence-based guidelines for diagnosis and management. Am J Respir Crit Care Med. (2011) 183:788–824. doi: 10.1164/rccm.2009-040GL
4. Maher TM, Bendstrup E, Dron L. Global incidence and prevalence of idiopathic pulmonary fibrosis. Respir Res. (2021) 22:197. doi: 10.1186/s12931-021-01791-z
5. Mei Q, Liu Z, Zuo H, Yang Z, Qu J. Idiopathic pulmonary fibrosis: an update on pathogenesis. Front Pharmacol. (2021) 12:797292. doi: 10.3389/fphar.2021.797292
6. Pakshir P, Hinz B. The big five in fibrosis: Macrophages, myofibroblasts, matrix, mechanics, and miscommunication. Matrix Biol. (2018) 68-69:81–93. doi: 10.1016/j.matbio.2018.01.019
7. Wynn TA, Vannella KM. Macrophages in tissue repair, regeneration, and fibrosis. Immunity. (2016) 44:450–62. doi: 10.1016/j.immuni.2016.02.015
8. Richeldi L, Collard HR, Du Bois RM, Jenkins G, Kolb M, Maher TM, et al. Mapping the future for pulmonary fibrosis: report from the 17th International Colloquium on Lung and Airway Fibrosis. Eur Respir J. (2013) 42:230–8. doi: 10.1183/09031936.00038613
9. Cai Y, Sugimoto C, Arainga M, Alvarez X, Didier ES, Kuroda MJ. In vivo characterization of alveolar and interstitial lung macrophages in rhesus macaques: implications for understanding lung disease in humans. J Immunol. (2014) 192:2821–9. doi: 10.4049/jimmunol.1302269
10. Moss BJ, Ryter SW, Rosas IO. Pathogenic mechanisms underlying idiopathic pulmonary fibrosis. Annu Rev Pathol. (2022) 17:515–46. doi: 10.1146/annurev-pathol-042320-030240
11. Spagnolo P, Tzouvelekis A, Bonella F. The management of patients with idiopathic pulmonary fibrosis. Front Med (Lausanne). (2018) 5:148. doi: 10.3389/fmed.2018.00148
12. Rehman M, Liu L, Wang Q. Copper environmental toxicology, recent advances, and future outlook: a review. Environ Sci pollut Res Int. (2019) 26:18003–16. doi: 10.1007/s11356-019-05073-6
13. Tsvetkov P, Coy S, Petrova B. Copper induces cell death by targeting lipoylated TCA cycle proteins. Cell Death. (2022) 375(6586):1254–61. doi: 10.1126/science.abf0529
14. Kardos J, HÉJA L, Simon Á. Copper signalling: causes and consequences. Cell Communication Signaling. (2018) 16:71. doi: 10.1186/s12964-018-0277-3
15. Ozcelik. D, Ozaras. R, Gurel. Z. Copper-mediated oxidative stress in rat liver. Biol Trace Elem Res. (2003) 96:209–15. doi: 10.1385/BTER:96:1-3:209
16. Guo H, Wang Y, Cui H. Copper induces spleen damage through modulation of oxidative stress, apoptosis, DNA damage, and inflammation. Biol Trace Elem Res. (2022) 200:669–77. doi: 10.1007/s12011-021-02672-8
17. Jian Z, Guo H, Liu H. Oxidative stress, apoptosis and inflammatory responses involved in copper-induced pulmonary toxicity in mice. Res Paper. (2020) 12:16867–86. doi: 10.18632/aging.103585
18. Lee HY, Nam S, Kim MJ. Butyrate prevents TGF-beta1-induced alveolar myofibroblast differentiation and modulates energy metabolism. Metabolites. (2021) 11. doi: 10.3390/metabo11050258
19. Taminau J, Meganck S, Lazar C, Steenhoff D, Coletta A, Molter C, et al. Unlocking the potential of publicly available microarray data using inSilicoDb and inSilicoMerging R/Bioconductor packages. BMC Bioinf. (2012) 13. doi: 10.1186/1471-2105-13-335
20. Johnson WE, Li C, Rabinovic A. Adjusting batch effects in microarray expression data using empirical Bayes methods. Biostatistics. (2007) 8:118–27. doi: 10.1093/biostatistics/kxj037
21. Heukels P, Moor CC, Von Der Thusen JH. Inflammation and immunity in IPF pathogenesis and treatment. Respir Med. (2019) 147:79–91. doi: 10.1016/j.rmed.2018.12.015
22. Newman AM, Liu CL, Green MREA. Robust enumeration of cell subsets from tissue expression profiles. Nat Methods. (2015) 12:453–7. doi: 10.1038/nmeth.3337
23. Richeldi L, Collard HR, Jones MG. Idiopathic pulmonary fibrosis. Lancet. (2017) 389:1941–52. doi: 10.1016/S0140-6736(17)30866-8
24. Navaratnam V, Fleming KM, West J, Smith CJ, Jenkins RG, Fogarty A, et al. The rising incidence of idiopathic pulmonary fibrosis in the U.K. Thorax. (2011) 66:462–7. doi: 10.1136/thx.2010.148031
25. Hutchinson J, Fogarty A, Hubbard R, Mckeever T. Global incidence and mortality of idiopathic pulmonary fibrosis: a systematic review. Eur Respir J. (2015) 46:795–806. doi: 10.1183/09031936.00185114
26. Chen L, Min J, Wang F. Copper homeostasis and cuproptosis in health and disease. Signal Transduct Target Ther. (2022) 7:378. doi: 10.1038/s41392-022-01229-y
27. Yuan Y, Fu M, Li N, Ye M. Identification of immune infiltration and cuproptosis-related subgroups in Crohn's disease. Front Immunol. (2022) 13:1074271. doi: 10.3389/fimmu.2022.1074271
28. Zhang G, Sun J, Zhang X. A novel Cuproptosis-related LncRNA signature to predict prognosis in hepatocellular carcinoma. Sci Rep. (2022) 12:11325. doi: 10.1038/s41598-022-15251-1
29. Bian Z, Fan R, Xie L. A novel cuproptosis-related prognostic gene signature and validation of differential expression in clear cell renal cell carcinoma. Genes (Basel). (2022) 13. doi: 10.3390/genes13050851
30. Janssen R, De Brouwer B, Von Der Thusen JH, Wouters EFM. Copper as the most likely pathogenic divergence factor between lung fibrosis and emphysema. Med Hypotheses. (2018) 120:49–54. doi: 10.1016/j.mehy.2018.08.003
31. Niu YY, Zhang YY, Zhu Z, Zhang XQ, Liu X, Zhu SY, et al. Elevated intracellular copper contributes a unique role to kidney fibrosis by lysyl oxidase mediated matrix crosslinking. Cell Death Dis. (2020) 11:211. doi: 10.1038/s41419-020-2404-5
32. Li X, Huang Y, Ye N, He J. Analysis of immune-related genes in idiopathic pulmonary fibrosis based on bioinformatics and experimental verification. Ann Palliat Med. (2021) 10:11598–614. doi: 10.21037/apm-21-2676
33. Wu J, Wu YQ, Ricklin D, Janssen BJ, Lambris JD, Gros P. Structure of complement fragment C3b-factor H and implications for host protection by complement regulators. Nat Immunol. (2009) 10:728–33. doi: 10.1038/ni.1755
34. Danobeitia JS, Ziemelis M, Ma X, Zitur LJ, Zens T, Chlebeck PJ, et al. Complement inhibition attenuates acute kidney injury after ischemia-reperfusion and limits progression to renal fibrosis in mice. PLoS One. (2017) 12:e0183701. doi: 10.1371/journal.pone.0183701
35. Shenderov K, Collins SL, Powell JD, Horton MR. Immune dysregulation as a driver of idiopathic pulmonary fibrosis. J Clin Invest. (2021) 131. doi: 10.1172/JCI143226
36. Huo SF, Shang WL, Yu M, Ren XP, Wen HX, Chai CY, et al. STEAP1 facilitates metastasis and epithelial-mesenchymal transition of lung adenocarcinoma via the JAK2/STAT3 signaling pathway. Biosci Rep. (2020) 40. doi: 10.1042/BSR20193169
37. Knutson MD. Steap proteins: implications for iron and copper Metabolism. Nutr Rev. (2007) 65:335–40. doi: 10.1301/nr.2007.jul.335–340
38. Fu X-G, Deng J, Xu W-J. Histidine decarboxylase-expressing PMN-MDSC-derived TGF-β1 promotes the epithelial-mesenchymal transition of metastatic lung adenocarcinoma. Int J Clin Exp Pathol. (2020) 13:1361–74.
39. Kennedy L, Meadows V, Demieville J, Hargrove L, Virani S, Glaser S, et al. Biliary damage and liver fibrosis are ameliorated in a novel mouse model lacking l-histidine decarboxylase/histamine signaling. Lab Invest. (2020) 100:837–48. doi: 10.1038/s41374-020-0405-8
40. Iyama T, Abolhassani N, Tsuchimoto D, Nonaka M, Nakabeppu Y. NUDT16 is a (deoxy)inosine diphosphatase, and its deficiency induces accumulation of single-strand breaks in nuclear DNA and growth arrest. Nucleic Acids Res. (2010) 38:4834–43. doi: 10.1093/nar/gkq249
41. Phillips IR, Veeravalli S, Khadayate S, Shephard EA. Metabolomic and transcriptomic analyses of Fmo5-/- mice reveal roles for flavin-containing monooxygenase 5 (FMO5) in NRF2-mediated oxidative stress response, unfolded protein response, lipid homeostasis, and carbohydrate and one-carbon metabolism. PLoS One. (2023) 18:e0286692. doi: 10.1371/journal.pone.0286692
42. Wang J, Zhu H, Huang L, Zhu X, Sha J, Li G, et al. Nrf2 signaling attenuates epithelial-to-mesenchymal transition and renal interstitial fibrosis via PI3K/Akt signaling pathways. Exp Mol Pathol. (2019) 111:104296. doi: 10.1016/j.yexmp.2019.104296
43. Liu Q, Gao Y, Ci X. Role of nrf2 and its activators in respiratory diseases. Oxid Med Cell Longev. (2019) 2019:7090534. doi: 10.1155/2019/7090534
44. Sun M, Zhang T, Wang Y, Huang W, Xia L. A novel signature constructed by immune-related lncRNA predicts the immune landscape of colorectal cancer. Front Genet. (2021) 12:695130. doi: 10.3389/fgene.2021.695130
45. Suzuki Y, Rahman M, Mitsuya H. Diverse transcriptional response of CD4+ T cells to stromal cell-derived factor SDF-1: cell survival promotion and priming effects of SDF-1 on CD4+ T cells. J Immunol. (2001) 167:3064–73. doi: 10.4049/jimmunol.167.6.3064
46. Wu X, Qian L, Zhao H, Lei W, Liu Y, Xu X, et al. CXCL12/CXCR4: An amazing challenge and opportunity in the fight against fibrosis. Ageing Res Rev. (2023) 83:101809. doi: 10.1016/j.arr.2022.101809
47. Jaffar J, Griffiths K, Oveissi S, Duan M, Foley M, Glaspole I, et al. CXCR4(+) cells are increased in lung tissue of patients with idiopathic pulmonary fibrosis. Respir Res. (2020) 21:221. doi: 10.1186/s12931-020-01467-0
48. Ishikawa G, Liu A, Herzog EL. Evolving perspectives on innate immune mechanisms of IPF. Front Mol Biosci. (2021) 8:676569. doi: 10.3389/fmolb.2021.676569
49. Yang IV, Luna LG, Cotter J, Talbert J, Leach SM, Kidd R, et al. The peripheral blood transcriptome identifies the presence and extent of disease in idiopathic pulmonary fibrosis. PLoS One. (2012) 7:e37708. doi: 10.1371/journal.pone.0037708
50. Hoeft K, Schaefer GJL, Kim H, Schumacher D, Bleckwehl T, Long Q, et al. Platelet-instructed SPP1(+) macrophages drive myofibroblast activation in fibrosis in a CXCL4-dependent manner. Cell Rep. (2023) 42:112131. doi: 10.1016/j.celrep.2023.112131
51. Cheng Q, Chen M, Wang H, Chen X, Wu H, Du Y, et al. MicroRNA-27a-3p inhibits lung and skin fibrosis of systemic sclerosis by negatively regulating SPP1. Genomics. (2022) 114:110391. doi: 10.1016/j.ygeno.2022.110391
52. Lin R, Wu S, Zhu D, Qin M, Liu X. Osteopontin induces atrial fibrosis by activating Akt/GSK-3beta/beta-catenin pathway and suppressing autophagy. Life Sci. (2020) 245:117328. doi: 10.1016/j.lfs.2020.117328
53. Korbecki J, Kupnicka P, Chlubek M, Goracy J, Gutowska I, Baranowska-Bosiacka I. CXCR2 receptor: regulation of expression, signal transduction, and involvement in cancer. Int J Mol Sci. (2022) 23. doi: 10.3390/ijms23042168
Keywords: idiopathic pulmonary fibrosis disease, cuproptosis -related genes, immune-related genes, immune infiltration, single-cell RNA-seq
Citation: Jin C, Li J, Li Q, Zhang L, Zheng S, Feng Q, Li Y, Zheng Y, Nie Q, Liang J and Wang J (2025) Contribution of cuproptosis and immune-related genes to idiopathic pulmonary fibrosis disease. Front. Immunol. 16:1458341. doi: 10.3389/fimmu.2025.1458341
Received: 02 July 2024; Accepted: 14 January 2025;
Published: 07 February 2025.
Edited by:
Zhihong Chen, Fudan University, ChinaCopyright © 2025 Jin, Li, Li, Zhang, Zheng, Feng, Li, Zheng, Nie, Liang and Wang. This is an open-access article distributed under the terms of the Creative Commons Attribution License (CC BY). The use, distribution or reproduction in other forums is permitted, provided the original author(s) and the copyright owner(s) are credited and that the original publication in this journal is cited, in accordance with accepted academic practice. No use, distribution or reproduction is permitted which does not comply with these terms.
*Correspondence: Jin Liang, bGlhbmdqaW4wMTAyQDE2My5jb20=; Jing Wang, dGxmd2pAMTYzLmNvbQ==
†These authors have contributed equally to this work
Disclaimer: All claims expressed in this article are solely those of the authors and do not necessarily represent those of their affiliated organizations, or those of the publisher, the editors and the reviewers. Any product that may be evaluated in this article or claim that may be made by its manufacturer is not guaranteed or endorsed by the publisher.
Research integrity at Frontiers
Learn more about the work of our research integrity team to safeguard the quality of each article we publish.