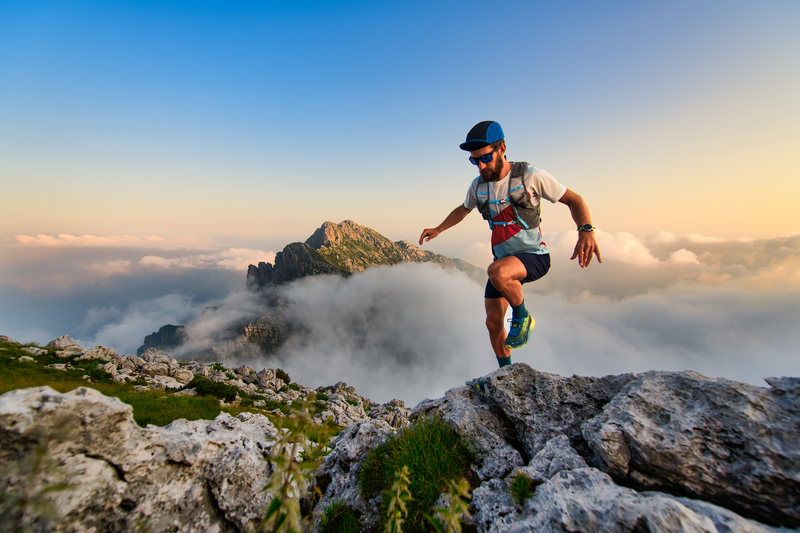
94% of researchers rate our articles as excellent or good
Learn more about the work of our research integrity team to safeguard the quality of each article we publish.
Find out more
ORIGINAL RESEARCH article
Front. Immunol. , 11 December 2024
Sec. Inflammation
Volume 15 - 2024 | https://doi.org/10.3389/fimmu.2024.1486209
Background: Abdominal aortic aneurysm (AAA) is a serious life-threatening vascular disease, and its ferroptosis/cuproptosis markers have not yet been characterized. This study was aiming to identify markers associated with ferroptosis/cuproptosis in AAA by bioinformatics analysis combined with machine learning models and to perform experimental validation.
Methods: This study used three scRNA-seq datasets from different mouse models and a human PBMC bulk RNA-seq dataset. Candidate genes were identified by integrated analysis of scRNA-seq, cell communication analysis, monocle pseudo-time analysis, and hdWGCNA analysis. Four machine learning algorithms, LASSO, REF, RF and SVM, were used to construct a prediction model for the PBMC dataset, the above results were comprehensively analyzed, and the targets were confirmed by RT-qPCR.
Results: scRNA-seq analysis showed Mo/MF as the most sensitive cell type to AAA, and 34 cuproptosis associated ferroptosis genes were obtained. Pseudo-time series analysis, hdWGCNA and machine learning prediction model construction were performed on these genes. Subsequent comparison of the above results showed that only PIM1 appeared in all algorithms. RT-qPCR and western blot results were consistent with sequencing results, showing that PIM1 was significantly upregulated in AAA.
Conclusion: In a conclusion, PIM1 as a novel biomarker associated with cuproptosis/ferroptosis in AAA was highlighted.
Abdominal aortic aneurysm (AAA) is a potentially fatal disease characterized by high morbidity and mortality in the event of aortic rupture. In the past 20 years, there have been major advances in surgical and endovascular repair techniques for AAA. However, drug-based treatments are still scarce, highlighting a real need for a deeper understanding of the molecular mechanisms involved in AAA formation (1). The main pathological features of AAA include capillary smooth muscle degeneration and death, of note, the accumulation of inflammatory cells is also an important feature (2, 3). The inflammatory process plays an integral role in AAA and exerts influence on many determinants of aortic wall remodeling.
Monocytes-macrophages include promonocytes in the bone marrow, monocytes in peripheral blood, and macrophages (MF) in tissues. MF are derived from monocytes in the blood, and monocytes are derived from precursor cells in the bone marrow (2, 4, 5). A deeper understanding of monocytes in the peripheral blood and MF will help improve our understanding of the role of these cells in the immune environment of the disease and make it possible to design new macrophage-targeted therapies (6). However, this part of the research is still not very clear in AAA so far.
Metal ions are important mediators of cellular processes, like regulation of cell death, and their abnormal concentration levels can cause destructive effects in cells. Ferroptosis is an iron-dependent programmed cell death. Excessive accumulation of intracellular iron leads to the accumulation of lipid peroxides, which induce programmed cell death (7, 8). Disorders of iron metabolism are closely related to AAA, leading to extensive research on iron-dependent toxicity (9–11). Like iron ions, copper ions also play a role in cell death. High levels of intracellular copper can cause cytotoxicity, also known as cuproptosis (12, 13). This death mechanism is controlled by copper ions and caused by their direct participation in the tricarboxylic acid cycle pathway, leading to protein aggregation and proteotoxic stress response (13). Less studies have reported the potential role of cuproptosis in the prediction of AAA (14). Moreover, the mechanisms behind cuproptosis-associated AAA and its potential link to ferroptosis remain the subject of ongoing investigation.
Here, in this study, we aimed to identify biomarkers based on cuprotosis-related ferroptosis genes in AAA. Comprehensive bioinformatics analysis and machine learning models found that among cuprotosis-related ferroptosis genes, PIM1 (Pim-1 proto-oncogene, serine/threonine kinase) was significantly upregulated in all in vitro models and patient PBMCs. In addition, our experimental results confirmed that the gene and protein expression levels of PIM1 were also in a significant upregulation trend. Our findings may help improve the personalized treatment and prognosis assessment of AAA.
In this study, we collected mouse single-cell sequencing data from three different AAA models, including CaCl2-induced (GSE164678), elastase-induced (GSE152583), and Ang II-induced (GSE221789) models (15–17). We obtained PBMC bulk RNA transcriptome data from seven patients and seven donors (18). After merging and removing abnormal samples, 13 samples were finally included for analysis. Detailed sample collection information is shown in Supplementary Table S1.
For single-cell datasets, we used the widely validated Seurat’s (R package, V4.4.0) canonical correlation analysis (CCA) integrated dimensionality reduction pipeline (19). First, we filtered the individual data with a filtering threshold of nFeature_RNA > 200, 50000 > nCount_RNA > 1000 and percent.mt < 10. Next, we used the FindIntegrationAnchors function to identify anchors between different datasets and used the IntegrateData function for CCA integration. Subsequently, the top 20 PCs were used for UMAP dimensionality reduction and resolution = 0.3 was used for cluster identification. For cell type identification, we used classical marker genes for different cell types.
For PBMC bulk RNA transcriptome data, we used the principal components analysis (PCA) analysis of DESeq2 (R package, V1.42.1) to filter samples, and we eliminated one AAA sample because the characteristics of this sample were consistent with those of the donor (20).
To identify the cell types most affected by AAA, we performed a cell sensitivity analysis using Augur (R package, V1.0.0). The AUC feature value was calculated using calculate_auc function, with the input being the constructed Seurat object. The codes refer to https://github.com/neurorestore/Augur.
The CellChat package (R package, V1.6.1) was used to construct the intercellular communication network (21). We constructed cellchat objects between the control and AAA samples to better compare and visualize them. The ligand receptor database is provided by the software package.
The schemes for identifying DEGs in single-cell data and bulk RNA-seq data are slightly different. For single-cell data, we used the FindMarkers function of the Seurat software package for identification (19), with a selection threshold of |log2FC| > 0.25 and p < 0.05. For bulk RNA-seq, the DESeq2 software package was used for identification (20), with a selection threshold of |log2FC| > 0.5 and p < 0.05 (22).
In order to explore the functional status of gene sets and select subsequent signal targets as much as possible, we used clusterProfiler (R package, V4.10.1) to perform functional enrichment analysis, including gene ontology (GO), kyoto encyclopedia of genes and genomes (KEGG), gene set enrichment analysis (GSEA) and Reactome Pathway enrichment (23). Terms with p < 0.05 were considered significantly enriched. We used aPEAR (R package, V1.0.0) for some of the visualizations and ggplot2 (R package, V3.5.1) for the rest (24).
To identify the interrelated cuproptosis associated ferroptosis genes in AAA, we obtained ferroptosis genes and cuproptosis from the Ferropoptosis Database (FerrDb, V2, http://www.zhounan.org/ferrdb/current/) (25). Then, we used Hmisc (R package, V5.1-2) to calculate the Pearson correlation between cuproptosis genes and ferroptosis genes. The Pearson correlation coefficient is widely used to measure the degree of correlation between two variables. Its value is between -1 and 1. >0 indicates a positive correlation, and <0 indicates a negative correlation. In this study, we defined |r| > 0.8 as a strong correlation, and we considered |r| > 0.8 and p < 0.05 to be a significant correlation, that is, cuproptosis associated ferroptosis genes.
To characterize the relationships of cuproptosis genes, we performed PPI analysis and visualization using the STRING (V12.0, https://string-db.org/cgi/input.pl?sessionId=0kGn9YYW1ES0&input_page_active_form=multiple_identifiers) database. The input was all cuproptosis genes.
To investigate transcriptional signatures that regulate cell fate transitions, we performed pseudo-time developmental trajectories construction using monocle (R package, V2.24.0) (26). We first constructed the monocle_cds object of monocytes and macrophages, then used the reduceDimension function for trajectory construction and the orderCells function for cell sorting. Notably, the BEAM statistical analysis model was used for cell fate trajectory differential analysis.
WGCNA is widely used to identify the relationship between gene modules and phenotypes (27, 28). In high-dimensional data, such as scRNA-seq, it is difficult to achieve due to the characteristics of the data. The development of hdWGCNA (R package, V0.3.03) software makes it possible to run single-cell datasets (29). Our data object is the single-cell expression matrix of monocytes and macrophages. The relevant analysis code refers to the software tutorial (https://smorabit.github.io/hdWGCNA/).
The screening of clinical biomarkers is the foundation for the early stage of clinical application transformation. In order to identify key biomarkers from massive sequencing data, we used four different machine learning algorithms, including least absolute shrinkage and selection operator (LASSO), recursive feature elimination (RFE), random forest (RF) and support vector machine (SVM). Of note, all machine learning methods were tested on PBMC bulk RNA transcriptome data (18).
The LASSO model was established using the cv.glmnet function of the glmnet (R package, V4.1-8), and the parameters were set as type.measure = ‘mse’, nfolds = 5 and alpha = 1. Then, the minimum lambda value was used as a reference to determine the optimal parameters.
The RFE model was established using the rfe function in caret (R package, V6.0-94), with parameters set as metric = ‘Accuracy’, sizes = 1:(length(candidate.gene)-2).
rfeControl = control, and 10-fold cross validation was used.
The RF model was established using the randomForest function in randomForest (R package, V4.7-1.1), with parameters set as importance=T, proximity=T, ntree= optionTrees.
The SVM model was built using the svmRFE function in e1071 (R package, V1.7-13), with parameters set as k = 5, halve.above = 100.
RT-qPCR was used to measure gene expression levels in PBMC and the steps were as described in our previous publication (30). In a short, gene was amplified and quantitatively analyzed after reverse transcription. The method of 2−ΔΔC was used to obtain the fold change. GAPDH was the housekeeping gene. The gene primers was in the Supplementary Table S2.
Western Blot was used to measure protein expression levels in PBMC and the steps were as described in our previous publication (30). Briefly, cells were lysed with enhanced RIPA lysis buffer and proteins were obtained and then denatured at high temperature. Next, SDS-PAGE electrophoresis and antibody incubation (Abcam, ab300453, the dilution ratio = 1:1000) were performed. Finally, protein chemiluminescence was performed and photos. ImageJ software was selected to calculate protein gray value.
Three independent replicates were performed in all biological experiments and the data were visualized as mean ± SD; Student’s t test was used to identify the differences between AAA and normal using Graphpad Prism 8.0 software. * indicates p < 0.05, compared with the control group.
We collected three different datasets to study the single-cell landscape in AAA. A series of quality control and integration analyses showed that we integrated samples from different groups well to meet the needs of downstream analysis (Supplementary Figure S1A). Next,14,089 cells were divided into 17 cell clusters and annotated into 8 cell types (Supplementary Figure S1B), including 5,746 smooth muscle cells (SMCs, marker genes were Acta2, Tagln and Myh11), 4,609 Fibroblasts (marker genes were Col1a1, Col3a1 and Dcn), 2,394 monocytes and macrophages (Mo/MF, marker genes were Lyz2, Cd68 and C1qb), 491 dendritic cells (DCs, marker genes were Col1a1, Col3a1 and Dcn), 291 Endothelials (marker genes were Pecam1, Vwf and Kdr), 267 T cells (marker genes were Cd3g and Cd28), 153 B cells (marker genes were Cd79a, Cd79b and Ms4a1), and 1,38 Neutrophils (marker genes were S100a8 and S100a9) (Figures 1A, B; Supplementary Figure S1C). We then performed cluster analysis on the expression of top 5 marker genes in different cell types (Figure 1C). UMAP was used to display the dimensionality reduction distribution of different cell types in different samples (Figure 1D). Subsequently, we observed the proportion of different cell types in different samples, and further used bar charts to visualize the cell types in different groups. The results showed that the proportion of SMC in AAA was significantly reduced, while Mo/MF and DCs were significantly increased in AAA (Figures 1E, F). Of note, we used the AUGUR algorithm to calculate cell sensitivity, and the results showed that Mo/MF was the most sensitive to AAA, followed by SMC and DCs (Figure 1G). This result was consistent with the statistical proportion of cell types. The above results showed that the proportion of SMCs decreased in AAA, which may be the result of massive apoptosis of SMCs (31). In addition, Mo/MF and DCs increased significantly, which may be related to their participation in inflammatory response (2). Since Mo/MF are the most sensitive cells, we included them in the subsequent analysis.
Figure 1. The landscape of AAA in a single-cell resolution. (A) UMAP plots show the cell distribution and number of different cell types included in the analysis under the three AAA models. (B) Violin plots show the expression characteristics of marker genes in different cell types (C) Heatmap showing the top 5 genes in different cell types. (D) UMAP plots show the distribution of cell types in different samples. (E) The distribution ratio of different cell types in each sample. (F) The bar graphs show the distribution statistics of different cell types under AAA and Con. Statistics were performed using Student’s T test, ** represents p < 0.01. (G) The circular histogram shows the sensitivity of different cell types to AAA, calculated by Augur. AAA1 means CaCl2-induced model, AAA2 means elastase-induced model and AAA3 means Ang II-induced model.
After integrated analysis of different AAA-induced models, it was found that in AAA, the intensity and frequency of intercellular communication were significantly enhanced, especially between SMC, Mo/MF, Fibroblast and Endothelial (Figures 2A, B). We then compared the differences in different signaling pathways between AAA and normal groups and found that GDF, ANGPT, and CD39 only appeared in AAA, while CD23, CEACAM, etc. only appeared in the normal group (Figure 2C). Since Mo/MF are AAA-sensitive cells and SMC loss is the main pathological feature of AAA, we observed the communication between these two types of cells. The results showed that TNF-α (Tnf-Tnfrsf1a) and TGF-β (Gdf15-Tgfbr2) signals were significantly enhanced in AAA (Figure 2D). There is also strong communication from endothelial cells to Mo/MF. Interestingly, the data showed that upregulation of TNF-α (Tnf-Tnfrsf1a) and TGF-β (Gdf15-Tgfbr2) signals was also observed in Mo/MF-Endoithelial (Supplementary Figure S2A). In addition, we observed the communication between TNF-α and TGF-β signals among different cell types throughout the sample, and the results showed that TNF-α and TGF-β signaling were overall enhanced in AAA (Figures 2E, F).
Figure 2. The cellular communication between SMC and Mo/MF is altered in AAA. (A) The bar graph shows the communication frequency (left) and strength (right) of all cell types in different groups. (B) The network diagram shows the frequency of cell communication for each cell type in different groups. (C) The stacked bar chart shows the differences in each signaling pathway under different groups. (D) The bubble chart shows the differential communication signals between Mo/MF and SMC in different groups. The left side shows the up-regulated signals in AAA, and the right side shows the down-regulated signals. e and f, Network diagram showing the differences in TNF-α (E) and TGF-β (F) signaling in different groups.
In the CaCl2-induced (GSE164678) model, we obtained a total of 1,482 DEGs, of which 944 were downregulated and 538 were upregulated (Figure 3A). GO results showed that these genes may be involved in ‘lymphocyte differentiation’ (Figure 3B). KEGG pathways showed that these genes play a role in regulating ‘apoptosis’, ‘TNF-α signaling pathway’, ‘cell cycle’ and ‘ferroptosis’ (Figure 3C). For elastase-induced (GSE152583) model, there were 4,595 DEGs, and most of them were upregulated, with 4,408 (Figure 3D). The GO annotation of these genes showed that they might be involved in ‘RNA splicing’ and ‘mRNA processing’ (Figure 3E), and the KEGG pathway enrichment showed that the ‘PI3K-AKT signaling pathway’, ‘apoptosis’, ‘cell cycle’, ‘TNF-α signaling pathway’, and ‘ferroptosis’ signaling pathway were significantly enriched (Figure 3F). For Ang II-induced (GSE221789) model, a total of 3209 DEGs were observed (Figure 3G), and their GO results showed that they might be involved in the ‘cytokine-mediated signaling pathway’ (Figure 3H). At the same time, the KEGG functional enrichment entries showed enrichment of ‘apoptosis’, ‘TNF-α signaling pathway’, and ‘ferroptosis’ signaling pathway (Figure 3I). These results suggest that ferroptosis may be involved in regulating the progression of AAA.
Figure 3. The transcriptional dynamics of Mo/MF were altered under different models. (A) The volcano plot shows the DEGs of Mo/MF under CaCl2-induced model, the DEGs screening threshold was |log2FC| > 0.25 and p < 0.05. (B) The GO enrichment analysis results of DEGs, p < 0.05 was considered significantly enriched. (C) The KEGG enrichment analysis results of DEGs, p < 0.05 was considered significantly enriched. D-F, DEGs (D) and related GO (E) and KEGG (F) enrichment analysis results under the elastase-induced model. (G–I), DEGs (G) and related GO (H) and KEGG (I) enrichment analysis results under the Ang II-induced model.
To further investigate the transcriptional signatures of Mo/MF under different induction models to better understand the mechanism of AAA, we first compared the differences in DEGs under different models, and the results showed that there were 617 shared genes (Figure 4A). The GO enrichment of these genes showed that they might be involved in the ‘TNF-α supurfamily cyttokine production’ (Figure 4B). In addition, the functional enrichment results suggested that the ‘TNF-α signaling pathway’ and ‘ferroptosis’ signaling pathway were significantly enriched (Figure 4C). Next, we compared the differences in different KEGG signaling pathways, and the results showed that 67 shared signaling pathways were significantly enriched in different models, such as apoptosis, ferroptosis, etc. (Figure 4D). Interestingly, GSEA showed that the apoptosis signaling pathway was significantly activated in AAA under different models (Figure 4E). Subsequent GO comparative analysis revealed 1,806 common terms (Figure 4F), and GSEA showed that cell death signals and cell killing signals were significantly activated in AAA, while cell population proliferation signals were significantly inhibited (Figure 4G). These results again highlight the role of ferroptosis signaling in AAA.
Figure 4. Comparative analysis of Mo/MF differences under different models. (A) The Venn diagram showing the comparative analysis of differentially expressed genes in Mo/MF under different models. (B, C) The GO (B) and KEGG (C) enrichment results of common DEGs in different models, p < 0.05 was considered significantly enriched. (D) The Venn diagram shows the comparative analysis of KEGG pathways in different models. (E) GSEA results showed the status of the Apoptosis signaling pathway under different models. (F) The Venn diagram shows the comparative analysis of GO terms in different models. (G) GSEA results showed the status of the cell death, cell killing and cell population proliferation signaling pathway under different models. AAA1 means CaCl2-induced model, AAA2 means elastase-induced model and AAA3 means Ang II-induced model.
Since the above results repeatedly suggested the role of ferroptosis in AAA, and taking the crosstalk between cuprotosis and ferroptosis into consideration, we attempted to identify the cuprotosis associated ferroptosis genes. We first explored the PPI network of cuptosis genes themselves. The results showed that PDHA1, DLAT, DLD, PDHB, LIPT1 and SIRT2 had strong interactions (Figure 5A). Reactome pathway enrichment results showed that these cuprotosis genes were involved in ‘protein lipoylation’ and ‘pyruvate metabolism’ (Figure 5B). Next, Pearson correlation analysis was performed to obtain the cuprotosis-ferroptosis gene pairs in AAA, among them, there were 169 negatively correlated (r < -0.8, p< 0.05) and 960 positively correlated (r > 0.8, p< 0.05) gene pairs. Of note, 1129 gene pairs contain 487 cuprotosis-associated ferroptosis genes (Figure 5C; Supplementary Table S3). To narrow down the candidate range, we compared these 487 cuprotosis-associated ferroptosis genes with the Mo/MF shared DEGs in different models and obtained 34 candidate genes for subsequent analysis (Figure 5D).
Figure 5. Identification of cuproptosis-associated ferroptosis genes in AAA. (A) The PPI network shows the interactions among cuprotosis genes. (B) The bar graph shows the Reactome Pathway of cuproptosis genes. (C) The volcano plot shows the correlation between candidate ferroptosis genes and cuproptosis genes. (D) The Venn diagram showing the relationship between candidate ferroptosis genes and DEGs. AAA1 means CaCl2-induced model, AAA2 means elastase-induced model and AAA3 means Ang II-induced model.
To investigate whether cuprotosis-associated ferroptosis genes regulate Mo/MF fate decisions, we performed developmental trajectory construction. The results showed that during Mo/MF development, two fate-determination events occurred and all cells were divided into five states (Figures 6A, B). Next, we observed the distribution of AAA cells in the trajectory and counted the proportion of AAA cells in different states to determine which cell fate determination period affects the functional state of Mo/MF, that is, to explain the driving factors of AAA (Figures 6C, D). The results showed that the proportion of AAA cells was high around the cell fate 2 period, indicating that this stage was driven by AAA. Elucidating the transcriptional dynamics of this fate will help reveal the regulatory mechanism of AAA. Subsequently, we visualized the expression signatures of candidate cuprotosis-associated ferroptosis genes on the developmental trajectory, and the results showed that 33 genes were involved in fate trajectory construction, indicating that ferroptosis is indispensable for AAA progression (Figure 6E). Importantly, we explored the transcriptional signatures that regulate cell fate 2 and revealed four distinct sets of genes involved in driving cell fate 2, including several cuprotosis-associated ferroptosis genes, such as Pim1, Ftl1, and Cd38 (Figure 6F). We investigated the functional characteristics of genes in different datasets and the results showed that the C1 gene set was involved in the ‘IL17 signaling pathway’, ‘ferroptosis’, and ‘apoptosis’. The C2 gene set was also involved in the’ IL17 signaling pathway’ and ‘apoptosis’ (Figure 6G). The above results show the key role of ferroptosis-related genes in the regulation of Mo/MF fate.
Figure 6. Construction of pseudo-development trajectories of Mo/MF cells. (A, B) The pseudo-time trajectories of Mo/MF show different fate trajectories (A) and different cell states (B). (C) Distribution of different groups under pseudo-time trajectory. (D) The stacked bar graphs show the distribution patterns of different groups in different cell states. (E) Expression trends of candidate cuproptosis-associated ferroptosis genes under pseudo-temporal trajectories. (F) The heat map shows the gene expression trends before and after cell fate 2. (G) The KEGG enrichment analysis of gene sets with different patterns in the heatmap.
WGCNA is an analysis algorithm that effectively screens key genes, and we performed hdWGCNA in Mo/MF to identify drivers of AAA. In order to construct a scale-free network, we screened the β value, and the results showed that the most appropriate value was β = 12 (Figure 7A). Then, we divide the genes into modules, and the hierarchical clustering numbers show the modules to which different genes belong (Figure 7B). 11 modules are obtained, and we use different colors to represent the modules (Figure 7C). We further studied the expression characteristics of hub genes within the module and found that these hub genes were cell type specific. In Mo/MF, Cxcl2, Cd83, Pim1 and other hub genes were highly expressed (Figure 7D). Interestingly, we found that different modules have different correlations, such as the yellow module is significantly positively correlated with the pink module, while the red module is negatively correlated with magenta (Figure 7E). This result suggests that different modules drive different biological events, and that there is crosstalk and antagonism between different modules. Moreover, we explored the relationship between different modules and different phenotypes. The results showed that the yellow module was significantly overexpressed in AAA (Figure 7F). We further observed the hub genes within this module and found that Pim1, Cd83 and others were highly connected (Figure 7G). Of note, we constructed a cuprotosis-associated ferroptosis genes regulatory network and discovered five key factors, including Pim1, Jdp2, Zfp36, Cd38 and Parp14 (Figure 7H).
Figure 7. Construction of WGCNA in Mo/MF at the single-cell level. (A) Free-scale network topology analysis for different soft threshold powers. The black circle shows the optimal threshold chosen. (B) Hierarchical clustering numbers show the modules to which genes belong. (C) The genes in each module ranked by kME that iseigengene-based connectivity. (D) The heat map shows the expression characteristics of hub genes in different cell types. (E) Correlation analysis between different modules. (F) The dot plot shows the expression patterns of different modules under different groups. (G) Co-expression network of the top 25 genes in the yellow module. (H) Co-expression network of cuproptosis-associated ferroptosis genes in Mo/MF.
The main purpose of this study was to screen effective biomarkers of cuprotosis-associated ferroptosis genes. Given the difficulty in manipulating tissues, we sought to consider the characteristics of these candidate genes in PBMCs, which are more easily accessible and manipulatable in the clinic. We included PBMC data from 7 donors and 6 AAA patients, and PCA analysis showed that they had obvious transcriptional distinction (Figure 8A). 5,165 DEGs were obtained, and the heat map showed their expression characteristics (Figures 8B, C). Functional enrichment analysis showed that DEGs were involved in a large number of inflammatory signaling pathways, such as ‘TNF-α production’, ‘IL-6 production’. ‘IL-1 production’ (Figure 8D). Furthermore, we analyzed the relationship between DEGs and uprotosis-associated ferroptosis genes, and the results suggested that they had five overlaps, namely Capg, Pgd, Pim1, Pkm and Slc25a37 (Figure 8E).
Figure 8. Analysis of transcriptional differences in PBMCs in AAA. (A) PCA analysis shows the top2 PCs under different groups. (B) The volcano plot shows the distribution of DEGs, and the DEGs screening threshold was |log2FC| > 0.5 and p < 0.05. (C) The heat map showing the expression characteristics of DEGs. (D) KEGG enrichment results of DEGs. (E) The Venn diagram showing the comparative analysis of DEGs and cuproptosis-associated ferroptosis genes. AAA1-6 means different sample.
Machine learning models are a good method for selecting biomarkers. Next, we used four machine models, including LASSO, REF, RF and SVM, to select biomarkers in a PBMC transcriptome data from AAA. We used 34 cuproptosis associated ferroptosis genes as features (Figure 5D), and used AAA and normal as predictive target variables to build the model. According to the LASSO model, four genes were identified as candidate genes, including PIM1, PLIN2, TXNRD1 and NDRG1 (Figures 9A, B). REF obtained five candidate genes, including PIM1, PLIN2, VEGFA, NDRG1 and SLC25A37 (Figure 9C). RF pick out ten candidates, including PIM1, PKM, IRF7, PARP14, PLIN2, CAPG, ENO1, TXN, PER1 and TCF4 (Figure 9D). The accuracy and error rate in the SVM model showed that 33 genes were the most suitable (Figures 9E, F). Significantly, we considered all the data, including three bioinformatics analysis methods and four machine learning algorithms, and finally obtained a candidate gene, namely PIM1 (Figure 9G). As expected, PIM1 was significantly overexpressed in AAA in all sequencing data, which strongly suggested the potential of this gene as a biomarker (Figure 9H). To verify the expression characteristics of PIM1 in patient PBMCs, we used RT-qPCR and protein immunoblotting to verify it at the RNA and protein levels. Excitingly, our experimental results suggest that PIM1 is significantly overexpressed in AAA, both at the RNA and protein levels (Figures 9I, J).
Figure 9. Analysis of transcriptional differences in PBMCs in AAA. (A) and (B) LASSO regression analysis was performed in PBMC data using candidate cuproptosis-associated ferroptosis genes. The minimum value was defined based on 10-fold cross validation, where the best λ yielded 4 cuproptosis-associated ferroptosis genes (A). Coefficient curves were plotted according to (log λ) sequence and lambda value (B). (C) The REF model generated five candidate cuproptosis-associated ferroptosis genes. (D) The top 10 cuproptosis-associated ferroptosis genes generated by RF model evaluation. (E, F) Under the SVM model, the line graph shows the accuracy (E) and error of different genes (F). (G) The Venn diagram shows the comparative analysis of candidate cuproptosis-associated ferroptosis genes obtained by screening with different bioinformatics algorithms and machine models. (H–J) The expression characteristics of PIM1 were examined by sequencing (H), RT-qPCR (I) and western blot (J). AAA1 means CaCl2-induced model, AAA2 means elastase-induced model and AAA3 means Ang II-induced model.
Currently, the only treatment options for AAA are surgery and endovascular repair. Unfortunately, conservative treatment options are lacking (1, 32). Emerging evidence suggests that two newly discovered programmed cell death, cuproptosis and ferroptosis, play crucial roles in the progression of AAA and show promise as effective future treatments for AAA (14, 33–35). However, studies exploring genes associated with cuprotosis and ferroptosis in AAA are lacking. Therefore, it is crucial to focus on potential prognostic studies on the link between cuprotosis and ferroptosis in AAA.
To select appropriate cell types for research, we first analyzed the differences in cell types in different AAA models. Consistent with previous studies, we found that SMCs were significantly reduced in AAA, which can be explained by the pathological characteristics of extensive apoptosis of SMCs in AAA (15–17, 36). The AUGUR algorithm suggests that Mo/MF cells are most affected by AAA, which may be related to the inflammatory response in AAA (2, 5). The functional enrichment results of DEGs in different models suggested that ferroptosis was significantly enriched, and the common DEGs of different models also showed enrichment for ferroptosis, which suggests that ferroptosis plays a role in driving AAA (33). Based on the above reasons, we studied cuprotosis-associated ferroptosis gene features in Mo/MF.
The construction of pseudo-temporal developmental trajectories provides a means to study cell fate determination (26). Our results found that among the genes driving Mo/MF changes in AAA, there are shadows of uprotosis-associated ferroptosis genes. Consistent with previous studies, our results suggest that ferroptosis drives AAA progression and that inflammatory responses occur extensively in Mo/MF at the AAA cell fate stage, which is characterized by the enrichment of the TNF-α signaling pathway. (5, 34, 37). The rise of hdWGCNA has broadened the perspective of single-cell data analysis and provided the possibility for better mining of candidate genes (29). A total of five candidate genes were obtained, including Pim1, Jdp2, Zfp36, Cd38 and Parp14. Currently, studies have reported that the expression level of CD38 is positively correlated with the diameter of the abdominal aorta and that the lack of CD38 in SMCs can inhibit the progression of AAA (38, 39).
Back to our topic, screening for effective AAA biological targets, considering the difficulty in obtaining samples in the clinic, we attempted to study the characteristics of candidate genes in PBMCs to more quickly advance our results to clinical translation.
Monocytes in PBMCs, as the source of MF, can reflect the characteristics of MF in tissues to a certain extent (2, 4, 5). Consistent with previous reports, we found that PBMCs in AAA underwent a substantial inflammatory response (18, 37). Our cross-analysis revealed five candidate genes, including Capg, Pgd, Pim1, Pkm and Slc25a37. Previous studies have reported that different expression forms of PKM affect the progression of AAA (40). These data suggest that the genes we selected have the potential to become biomarkers. Machine learning models have been widely used in predicting biomarkers and building prognostic models (41, 42). Four different machine learning methods, LASSO, RFE, RF and SVM, were selected to predict AAA markers, with candidate genes ranging from 4 to 33. This result shows that different machine learning models have different predictive effects (43).
A single bioinformatics analysis algorithm and machine learning model is not sufficient to screen effective biomarkers. We conducted a comprehensive comparison of all analysis methods, and excitingly, we obtained a potential biomarker, PIM1 (Pim-1 proto-oncogene, serine/threonine kinase), which was obtained in all analyses. PIM1 is a proto-oncogene that encodes a serine/threonine kinase that is primarily involved in cell cycle progression, apoptosis, transcriptional activation, and general signal transduction pathways (44, 45). Due to its critical functions, it has been extensively studied as a therapeutic target in cancer, but there is a lack of reports on its research in AAA. Interestingly, a recent report showed that it could regulate macrophage infiltration and polarization in the tumor microenvironment to enhance anti-PD-1 therapy response (45), which showed its effect on macrophages and also suggested that it might affect macrophages in AAA and thus regulate AAA progression. Indeed, PIM1 has been reported as a marker for pulmonary hypertension (46). These results strongly suggest that PIM1 may serve as an effective biomarker for AAA. To verify it, we characterized the gene and protein expression patterns of AAA in PBMCs of AAA patients. As expected, PIM1 was significantly upregulated in AAA. In a conclusion. PIM1 as a novel biomarker associated with cuproptosis/ferroptosis in AAA was highlighted.
We have to admit that the current study also has some limitations. This study is a hypothesis-driven study based on scRNA-seq or bulk RNA-seq data. Although we have verified the expression pattern of PIM1 in AAA through experimental schemes and confirmed it as an effective biomarker. However, its biological function in AAA has not yet been determined in this study. In future studies, the study of the biological function of PIM1 needs to be strengthened.
In summary, the combined results of our bioinformatics and machine learning models highlighted PIM1 as a valid biomarker for AAA, which was validated by RT-qPCR and western blot.
This study uses public database datasets, including GSE152583 (https://www.ncbi.nlm.nih.gov/geo/query/acc.cgi?acc=GSE152583), GSE164678 (https://www.ncbi.nlm.nih.gov/geo/query/acc.cgi?acc=GSE164678) and GSE221789 (https://www.ncbi.nlm.nih.gov/geo/query/acc.cgi?acc=GSE221789). No new codes were generated in this study, and all codes can be found in the relevant software guidance documents.
The studies involving humans were approved by the Ethics Committee of Shandong Provincial Hospital. The studies were conducted in accordance with the local legislation and institutional requirements. The participants provided their written informed consent to participate in this study.
ZH: Investigation, Methodology, Writing – original draft, Writing – review & editing. XL: Investigation, Writing – review & editing. YH: Investigation, Writing – original draft. TZ: Writing – review & editing. ZZ: Writing – review & editing. JZ: Writing – review & editing. HZ: Project administration, Writing – review & editing.
The author(s) declare financial support was received for the research, authorship, and/or publication of this article. This work was supported by the Shandong postdoctoral innovation project (SDCX-ZG-202303087).
All authors thank the contributors of raw sequencing data, including Huan Yang’s team, Guizhen Zhao’s team, Hong Wu’s team and Daniel P. Zalewski’s team.
Author XL was employed by the company Cisen Pharmaceutical Co., Ltd.
The remaining authors declare that the research was conducted in the absence of any commercial or financial relationships that could be construed as a potential conflict of interest.
All claims expressed in this article are solely those of the authors and do not necessarily represent those of their affiliated organizations, or those of the publisher, the editors and the reviewers. Any product that may be evaluated in this article, or claim that may be made by its manufacturer, is not guaranteed or endorsed by the publisher.
The Supplementary Material for this article can be found online at: https://www.frontiersin.org/articles/10.3389/fimmu.2024.1486209/full#supplementary-material
Supplementary Figure 1 | Supporting for Figure 1. (A, B) UMAP plots show the distribution of samples (A) and seurat_clusters (B) under the three AAA models. (C) UMAP profiles of annotated cell types for each sample.
Supplementary Figure 2 | Supporting for Figure 2. (A) The bubble chart shows the differential communication signals between Mo/MF and endothelial in different groups. The left side shows the up-regulated signals in AAA, and the right side shows the down-regulated signals.
AAA, Abdominal aortic aneurysm; MF, macrophages; SMCs, smooth muscle cells; DCs, dendritic cells; WGCNA, Weighted gene co-expression network analysis; UMAP, Uniform manifold approximate projection; DEGs, differently expressed genes; GO, Gene ontology; KEGG, Kyoto Encyclopedia of Genes and Genomes; PPI, Protein-protein interaction; GSEA, Gene set enrichment analysis; LASSO, Least absolute shrinkage and selection operator; RFE, Recursive feature elimination; RF, Random forest; SVM, Support vector machine.
1. Golledge J. Abdominal aortic aneurysm: update on pathogenesis and medical treatments. Nat Rev Cardiol. (2019) 16:225–42. doi: 10.1038/s41569-018-0114-9
2. Raffort J, Lareyre F, Clément M, Hassen-Khodja R, Chinetti G, Mallat Z. Monocytes and macrophages in abdominal aortic aneurysm. Nat Rev Cardiol. (2017) 14:457–71. doi: 10.1038/nrcardio.2017.52
3. Golledge J, Thanigaimani S, Powell JT, Tsao PS. Pathogenesis and management of abdominal aortic aneurysm. Eur Heart J. (2023) 44:2682–97. doi: 10.1093/eurheartj/ehad386
4. Geissmann F, Manz MG, Jung S, Sieweke MH, Merad M, Ley K. Development of monocytes, macrophages, and dendritic cells. Science. (2010) 327:656–61. doi: 10.1126/science.1178331
5. Potteaux S, Tedgui A. Monocytes, macrophages and other inflammatory mediators of abdominal aortic aneurysm. Curr Pharm design. (2015) 21:4007–15. doi: 10.2174/1381612821666150826093855
6. Ginhoux F, Guilliams M. Tissue-resident macrophage ontogeny and homeostasis. Immunity. (2016) 44:439–49. doi: 10.1016/j.immuni.2016.02.024
7. Li J, Cao F, Yin H-L, Huang Z-J, Lin Z-T, Mao N, et al. Ferroptosis: past, present and future. Cell Death Dis. (2020) 11:88. doi: 10.1038/s41419-020-2298-2
8. Jiang X, Stockwell BR, Conrad M. Ferroptosis: mechanisms, biology and role in disease. Nat Rev Mol Cell Biol. (2021) 22:266–82. doi: 10.1038/s41580-020-00324-8
9. Filiberto AC, Ladd Z, Leroy V, Su G, Elder CT, Pruitt EY, et al. Resolution of inflammation via RvD1/FPR2 signaling mitigates Nox2 activation and ferroptosis of macrophages in experimental abdominal aortic aneurysms. FASEB journal: Off Publ Fed Am Societies Exp Biol. (2022) 36:e22579. doi: 10.1096/fj.202201114R
10. Wang K, Song Y, Li H, Song J, Wang S. Identification of differentially expressed ferroptosis-related genes in abdominal aortic aneurysm: bioinformatics analysis. Front Cardiovasc Med. (2022) 9:991613. doi: 10.3389/fcvm.2022.991613
11. Chen L, Liu Y, Wang Z, Zhang L, Xu Y, Li Y, et al. Mesenchymal stem cell-derived extracellular vesicles protect against abdominal aortic aneurysm formation by inhibiting NET-induced ferroptosis. Exp Mol Med. (2023) 55:939–51. doi: 10.1038/s12276-023-00986-2
12. Tang D, Chen X, Kroemer G. Cuproptosis: a copper-triggered modality of mitochondrial cell death. Cell Res. (2022) 32:417–8. doi: 10.1038/s41422-022-00653-7
13. Tsvetkov P, Coy S, Petrova B, Dreishpoon M, Verma A, Abdusamad M, et al. Copper induces cell death by targeting lipoylated TCA cycle proteins. Science. (2022) 375:1254–61. doi: 10.1126/science.abf0529
14. Hu J, Xue S, Xu Z, Wu Z, Xu X, Wang X, et al. Identification of core cuprotosis-correlated biomarkers in abdominal aortic aneurysm immune microenvironment based on bioinformatics. Front Immunol. (2023) 14:1138126. doi: 10.3389/fimmu.2023.1138126
15. Yang H, Zhou T, Stranz A, Deroo E, Liu B. Single-cell RNA sequencing reveals heterogeneity of vascular cells in early stage murine abdominal aortic aneurysm—brief report. Arteriosclerosis thrombosis Vasc Biol. (2021) 41:1158–66. doi: 10.1161/ATVBAHA.120.315607
16. Zhao G, Lu H, Chang Z, Zhao Y, Zhu T, Chang L, et al. Single-cell RNA sequencing reveals the cellular heterogeneity of aneurysmal infrarenal abdominal aorta. Cardiovasc Res. (2021) 117:1402–16. doi: 10.1093/cvr/cvaa214
17. Wu H, Xie C, Wang R, Cheng J, Xu Q, Zhao H. Comparative analysis of thoracic and abdominal aortic aneurysms across the segment and species at the single-cell level. Front Pharmacol. (2023) 13:1095757. doi: 10.3389/fphar.2022.1095757
18. Zalewski DP, Ruszel KP, Stępniewski A, Gałkowski D, Bogucki J, Kołodziej P, et al. Identification of transcriptomic differences between lower extremities arterial disease, abdominal aortic aneurysm and chronic venous disease in peripheral blood mononuclear cells specimens. Int J Mol Sci. (2021) 22:3200. doi: 10.3390/ijms22063200
19. Hao Y, Hao S, Andersen-Nissen E, Mauck WM, Zheng S, Butler A, et al. Integrated analysis of multimodal single-cell data. Cell. (2021) 184:3573–3587. e3529. doi: 10.1016/j.cell.2021.04.048
20. Love M, Huber W, Anders S. Moderated estimation of fold change and dispersion for RNA-seq data with DESeq2. Genome Biol. (2014) 15, 550. doi: 10.1186/s13059-014-0550-8
21. Jin S, Guerrero-Juarez CF, Zhang L, Chang I, Ramos R, Kuan C-H, et al. Inference and analysis of cell-cell communication using CellChat. Nat Commun. (2021) 12:1088. doi: 10.1038/s41467-021-21246-9
22. Yin H, Chu A, Liu S, Yuan Y, Gong Y. Identification of DEGs and transcription factors involved in H. pylori-associated inflammation and their relevance with gastric cancer. PeerJ. (2020) 8:e9223. doi: 10.7717/peerj.9223
23. Wu T, Hu E, Xu S, Chen M, Guo P, Dai Z, et al. clusterProfiler 4.0: A universal enrichment tool for interpreting omics data. Innovation. (2021) 2(3):100141. doi: 10.1016/j.xinn.2021.100141
24. Kerseviciute I, Gordevicius J. aPEAR: an R package for autonomous visualization of pathway enrichment networks. Bioinformatics. (2023) 39:btad672. doi: 10.1093/bioinformatics/btad672
25. Zhou N, Yuan X, Du Q, Zhang Z, Shi X, Bao J, et al. FerrDb V2: update of the manually curated database of ferroptosis regulators and ferroptosis-disease associations. Nucleic Acids Res. (2023) 51:D571–82. doi: 10.1093/nar/gkac935
26. Cao J, Spielmann M, Qiu X, Huang X, Ibrahim DM, Hill AJ, et al. The single-cell transcriptional landscape of mammalian organogenesis. Nature. (2019) 566:496–502. doi: 10.1038/s41586-019-0969-x
27. Zhang F-L, Zhang S-E, Sun Y-J, Wang J-J, Shen W. Comparative transcriptomics uncover the uniqueness of oocyte development in the donkey. Front Genet. (2022) 13:839207. doi: 10.3389/fgene.2022.839207
28. Zhang F-L, Li W-D, Zhang G, Zhang M, Liu Z-J, Zhu K-X, et al. Identification of unique transcriptomic signatures through integrated multispecies comparative analysis and WGCNA in bovine oocyte development. BMC Genomics. (2023) 24:265. doi: 10.1186/s12864-023-09362-w
29. Morabito S, Reese F, Rahimzadeh N, Miyoshi E, Swarup V. hdWGCNA identifies co-expression networks in high-dimensional transcriptomics data. Cell Rep Methods. (2023) 3(6), 100498. doi: 10.1016/j.crmeth.2023.100498
30. Han Z-L, Wang H-Q, Zhang T-S, He Y-X, Zhou H. Up-regulation of exosomal miR-106a may play a significant role in abdominal aortic aneurysm by inducing vascular smooth muscle cell apoptosis and targeting TIMP-2, an inhibitor of metallopeptidases that suppresses extracellular matrix degradation. Eur Rev Med Pharmacol Sci. (2020) 24(15), p8087. doi: 10.26355/eurrev_202008_22493
31. Qian G, Adeyanju O, Olajuyin A, Guo X. Abdominal aortic aneurysm formation with a focus on vascular smooth muscle cells. Life. (2022) 12:191. doi: 10.3390/life12020191
32. Buck DB, Van Herwaarden JA, Schermerhorn ML, Moll FL. Endovascular treatment of abdominal aortic aneurysms. Nat Rev Cardiol. (2014) 11:112–23. doi: 10.1038/nrcardio.2013.196
33. Zhang F, Li K, Zhang W, et al. Ganglioside GM3 protects against abdominal circulation. Circulation. (2024) 149(11):843–859. doi: 10.1161/CIRCULATIONAHA.123.066110
34. He X, Xiong Y, Liu Y, Li Y, Zhou H, Wu K. Ferrostatin-1 inhibits ferroptosis of vascular smooth muscle cells and alleviates abdominal aortic aneurysm formation through activating the SLC7A11/GPX4 axis. FASEB J. (2024) 38:e23401. doi: 10.1096/fj.202300198RRR
35. Yang Z, Feng R, Zhao H. Cuproptosis and Cu: a new paradigm in cellular death and their role in non-cancerous diseases. Apoptosis. (2024) 29, 1330–60. doi: 10.1007/s10495-024-01993-y
36. Thompson RW, Liao S, Curci JA. Vascular smooth muscle cell apoptosis in abdominal aortic aneurysms. Coronary artery Dis. (1997) 8:623–32. doi: 10.1097/00019501-199710000-00005
37. Batra R, Suh MK, Carson JS, Dale MA, Meisinger TM, Fitzgerald M, et al. IL-1β (interleukin-1β) and TNF-α (tumor necrosis factor-α) impact abdominal aortic aneurysm formation by differential effects on macrophage polarization. Arteriosclerosis Thrombosis Vasc Biol. (2018) 38:457–63. doi: 10.1161/ATVBAHA.117.310333
38. Sánchez-Infantes D, Nus M, Navas-Madroñal M, Fité J, Pérez B, Barros-Membrilla AJ, et al. Oxidative stress and inflammatory markers in abdominal aortic aneurysm. Antioxidants. (2021) 10:602. doi: 10.3390/antiox10040602
39. Yu Z-P, Wang Y-K, Wang X-Y, Gong L-N, Tan H-L, Jiang M-X, et al. Smooth-muscle-cell-specific deletion of CD38 protects mice from angII-induced abdominal aortic aneurysm through inhibiting vascular remodeling. Int J Mol Sci. (2024) 25:4356. doi: 10.3390/ijms25084356
40. Zhang Y, Li L, Ma L. Integrative analysis of transcriptome-wide association study and mRNA expression profile identified candidate genes and pathways associated with aortic aneurysm and dissection. Gene. (2022) 808:145993. doi: 10.1016/j.gene.2021.145993
41. Hirata K, Nakaura T, Nakagawa M, Kidoh M, Oda S, Utsunomiya D, et al. Machine learning to predict the rapid growth of small abdominal aortic aneurysm. J Comput Assisted Tomography. (2020) 44:37–42. doi: 10.1097/RCT.0000000000000958
42. Zhu J, Zhang J, Lou Y, Zheng Y, Zheng X, Cen W, et al. Developing a machine learning-based prognosis and immunotherapeutic response signature in colorectal cancer: insights from ferroptosis, fatty acid dynamics, and the tumor microenvironment. Front Immunol. (2024) 15:1416443. doi: 10.3389/fimmu.2024.1416443
43. Xiong T, Lv X-S, Wu G-J, Guo Y-X, Liu C, Hou F-X, et al. Single-cell sequencing analysis and multiple machine learning methods identified G0S2 and HPSE as novel biomarkers for abdominal aortic aneurysm. Front In Immunol. (2022) 13:907309. doi: 10.3389/fimmu.2022.907309
44. Merkel AL, Meggers E, Ocker M. PIM1 kinase as a target for cancer therapy. Expert Opin investigational Drugs. (2012) 21:425–36. doi: 10.1517/13543784.2012.668527
45. Chen X, Zhou J, Wang Y, Wang X, Chen K, Chen Q, et al. PIM1/NF-κB/CCL2 blockade enhances anti-PD-1 therapy response by modulating macrophage infiltration and polarization in tumor microenvironment of NSCLC. Oncogene. (2024) 43, 2517–30. doi: 10.1038/s41388-024-03100-6
Keywords: PIM1, ferroptosis, cuproptosis, abdominal aortic aneurysm, WGCNA
Citation: Han Z, Lu X, He Y, Zhang T, Zhou Z, Zhang J and Zhou H (2024) Integration of bulk/scRNA-seq and multiple machine learning algorithms identifies PIM1 as a biomarker associated with cuproptosis and ferroptosis in abdominal aortic aneurysm. Front. Immunol. 15:1486209. doi: 10.3389/fimmu.2024.1486209
Received: 25 August 2024; Accepted: 18 November 2024;
Published: 11 December 2024.
Edited by:
Yu’e Liu, Boston Children’s Hospital and Harvard Medical School, United StatesReviewed by:
Jin-Ming Zhang, University of Texas Health Science Center at Houston, United StatesCopyright © 2024 Han, Lu, He, Zhang, Zhou, Zhang and Zhou. This is an open-access article distributed under the terms of the Creative Commons Attribution License (CC BY). The use, distribution or reproduction in other forums is permitted, provided the original author(s) and the copyright owner(s) are credited and that the original publication in this journal is cited, in accordance with accepted academic practice. No use, distribution or reproduction is permitted which does not comply with these terms.
*Correspondence: Hua Zhou, emhvdWh1YTMyNjkyOUAxNjMuY29t
Disclaimer: All claims expressed in this article are solely those of the authors and do not necessarily represent those of their affiliated organizations, or those of the publisher, the editors and the reviewers. Any product that may be evaluated in this article or claim that may be made by its manufacturer is not guaranteed or endorsed by the publisher.
Research integrity at Frontiers
Learn more about the work of our research integrity team to safeguard the quality of each article we publish.