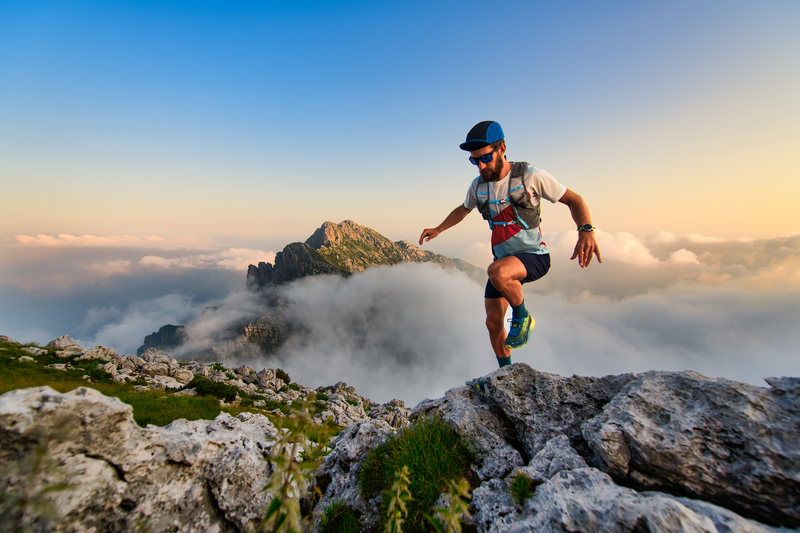
95% of researchers rate our articles as excellent or good
Learn more about the work of our research integrity team to safeguard the quality of each article we publish.
Find out more
REVIEW article
Front. Immunol. , 18 October 2024
Sec. Multiple Sclerosis and Neuroimmunology
Volume 15 - 2024 | https://doi.org/10.3389/fimmu.2024.1459502
This article is part of the Research Topic Use of Big Data and Artificial Intelligence in Multiple Sclerosis View all 9 articles
Artificial intelligence (AI) has meant a turning point in data analysis, allowing predictions of unseen outcomes with precedented levels of accuracy. In multiple sclerosis (MS), a chronic inflammatory-demyelinating condition of the central nervous system with a complex pathogenesis and potentially devastating consequences, AI-based models have shown promising preliminary results, especially when using neuroimaging data as model input or predictor variables. The application of AI-based methodologies to serum/blood and CSF biomarkers has been less explored, according to the literature, despite its great potential. In this review, we aimed to investigate and summarise the recent advances in AI methods applied to body fluid biomarkers in MS, highlighting the key features of the most representative studies, while illustrating their limitations and future directions.
Artificial intelligence (AI) techniques have proved very useful for the diagnosis and prognostication of several conditions around the world (1), including multiple sclerosis (MS) (2). AI methods used in medical research, including MS research, may include machine learning (ML) and deep learning (DL) analyses. Typically, while ML analyses are based on tabulated data as input to the model, DL models use raw data – typically images – as input to the model. Model outputs depend on the type of task that is needed, e.g., a given diagnosis (instead of another one), a certain disability milestone, or the presence of MRI activity in people who are receiving a given drug.
Multiple sclerosis (MS) is a chronic inflammatory-demyelinating condition of the central nervous system (CNS) with heterogeneous genetic and environmental risk factors (3). Disease diagnosis and monitoring strongly rely on routine clinical assessments and the use of conventional brain and spinal cord magnetic resonance imaging (MRI) as a biomarker. A biological marker, or biomarker, is a characteristic that is objectively measured and evaluated as an indicator of normal biological processes, pathogenic processes or pharmacologic responses to a therapeutic intervention (4). Besides MRI, body fluid biomarkers can also provide additional, independent data on MS. AI applications in MS can potentially help us better support the diagnosis, find markers for prognosis, facilitate accurate monitoring, and eventually understand the mechanisms of the disease. Focusing on these main challenges, this review aims to summarise the recent advances in AI applied to blood, serum and CSF biomarkers in MS, highlighting the key features of the most representative studies (Figure 1) (5). This review also aims to illustrate its limitations and future directions.
Figure 1. Main aims of AI-based studies focused on fluid biomarkers. This figure illustrates the main types of input data and the main aims of AI-based studies focused on fluid biomarker data in MS.
We performed a search in PubMed based on the following criteria: (i) search terms: ((multiple sclerosis) or demyelination or (demyelinating disease)) AND ((artificial intelligence) or (deep learning) or (machine learning)) AND (biomarkers OR markers OR (biological markers) OR (fluid biomarkers) OR (body fluid biomarkers)); (ii) language of publication: English; (iv) type of paper: original research. For the purpose of this narrative review, we have focused on three aspects: (i) diagnosis & differential diagnosis; (ii) prediction of clinical outcome; (iii) understanding of pathogenic mechanisms. Thus, after the first literature search, we manually selected the papers if they were included in one of these three categories. Papers not clearly included in any of these categories were not considered in the review. Thus, we did not include papers whose main focus was methodological or animal research, and papers related to fluid biomarkers other than blood, serum and CSF. We also excluded review papers, editorials, and case reports. The PubMed search yielded 206 articles, published between 1996 (and especially between 2009) and 2024, both included (Figure 2). After excluding those not meeting our inclusion criteria, we revised 29 papers for their inclusion in this narrative review (Figure 2). Most of these papers have been published between 2019 and 2024 (Figure 3).
Figure 2. PRISMA chart describing article selection. We have followed a systematic approach for selecting the papers to be considered in our manuscript. After performing a PubMed search with the following terms: (multiple sclerosis or demyelination or demyelinating disease) AND (artificial intelligence or deep learning or machine learning) AND (biomarkers or markers or biological markers or fluid biomarkers or body fluid biomarkers), 206 records were obtained. Of those, only 29 were considered for this review after excluding those not meeting our inclusion criteria.
Figure 3. Distribution of the research papers on AI applied to biomarker data in MS over time. This histogram shows the number of research articles (of those 29 selected) published per year. It is to be noted that most of the papers have been published in the last 4 years.
Once all papers were selected, they were divided into MS diagnosis and differential diagnosis (N=6), prediction of disease evolution (N=14), and understanding mechanisms of damage in MS (N=9). Of note, for some papers we found a degree of overlap and the decision to include them into one or another category depended on the main objectives described by the authors.
The diagnosis of MS relies on integrating clinical, MRI, and laboratory findings and excluding alternative diagnoses, especially in the presence of red flags. Indeed, the diagnosis of MS is not devoid of challenges: other conditions may mimic MS, clinically or radiologically (6). In these circumstances, the use of AI algorithms may be useful (Table 1), especially in body fluid biomarker discovery studies such as those done with “omics” technology.
AI has been implemented to identify genetic susceptibility biomarkers. Pasella et al. (7) used decision trees (DT) to create a predictive tool assessing the likelihood of MS including alleles responsible for human leukocyte antigen (HLA) class I molecules and killer immunoglobulin-like receptor (KIR) genes, responsible for natural killer (NK) lymphocyte receptors. They studied 299 persons with MS (PwMS) and 619 healthy controls (HC). The algorithm accurately identified 80.94% of PwMS and 71.08% HC in the training set and 73.24% and 66.07%, respectively, in the validation set. Guo et al. (8) used Support Vector Machine (SVM) to identify gene expression profiles on the transcriptome of peripheral blood mononuclear cells (PBMC) from 26 PwMS and 18 subjects with other neurological diseases (OND). This approach identified 8 genes differentially expressed between groups with 86% accuracy in the validation study. These genes involved the protein kinase cascade, inactivation of mitogen-activated protein kinases (MAPK), and regulation of signal transduction and apoptosis.
The metabolomes of cells and tissues include lipids, amino acids, sugars and other molecules (9). Andersen et al. (10) used random forests (RF) to identify blood-based metabolite profiles that could discriminate between 12 male PwMS and 13 male controls. The top 6 candidate metabolites informative for MS, defined as having an area under the receiver operating characteristic (ROC) curve (ROC-AUC) >80%, participate in glutathione metabolism, fatty acid metabolism and oxidation, cellular membrane composition, and transient receptor potential channel signalling. Whilst metabolomics focuses on hydrophilic molecules, lipidomics has emerged as an independent “omics” due to its complexity (9). Lötsch et al. (11) used unsupervised ML to compare 43 lipid mediators in serum from 102 PwMS and 301 HC. The analyses showed 98% accuracy to differentiate PwMS from HC. Then, the authors used supervised ML implemented as RF and computed ABC analysis-based feature selection, to create a classifier. This approach identified 8 lipid biomarkers differentially expressed in PwMS with ≥95% accuracy in training and test datasets.
Other studies have focused on CSF biomarkers. Probert et al. (12) used ML to profile metabolites and proteins in CSF samples from 41 PwMS and positive IgG oligoclonal bands (+OCB) and 64 patients with OND and +OCB. Multivariate orthogonal partial least squares discriminant analyses (OPLS-DA) showed that combining connective tissue growth factor/Cysteine-rich protein/Nephroblastoma overexpressed-5 (CCN5), von Willebrand Factor (vWF), glial fibrillary acidic protein (GFAP), and OCB provided the best diagnostic properties to discriminate MS from OND (89% sensitivity, 92% specificity, 91% accuracy). Gaetani et al. (13) used hierarchical clustering to profile 92 immune activation CSF proteins in +OCB relapsing-remitting MS (RRMS) (n=58), -OCB RRMS (n=24), and OND (n=36). Next, they used binomial and multinomial least absolute shrinkage and selection operator (LASSO) regressions to differentiate among these groups. Cluster of differentiation 5 (CD5) (ROC-AUC 0.87) and interleukin 12B (IL-12B) (ROC-AUC 0.81) were the best MS vs OND predictors. The model that best differentiated +OCB RRMS from OND included IL-12B and 4 other proteins (sensitivity 91% and 81%, specificity 94% and 95% in the training and validation sets, respectively). The model that best differentiated -OCB RRMS from OND included CD5, 3 other immune activation proteins as well as NfL, assessed additionally (sensitivity 87% and 56%, specificity 80% and 48% in the training and validation sets, respectively).
One study assessed proteins in both CSF and serum. Martynova et al. (14) used five ML models to study differences in 45 leucocyte-activation regulatory cytokines, measured in serum and CSF of 101 PwMS and in 101 serum and 25 CSF samples from non-MS subjects. Twenty-two cytokines were altered in CSF and 20 in serum, of which 10 were commonly affected. Next, three independent datasets including cytokines affected in CSF and serum, only in CSF, and only in serum were used as input to ML models to predict MS. Diagnostic accuracy was ≥92% when any randomly selected five of any cytokines were used.
The high heterogeneity of MS in terms of disease evolution means that the prognostication in clinical practice is extremely difficult. Although the presence of a high number of inflammatory-demyelinating lesions in the brain (15), and the presence of infratentorial (16), cortical (17), spinal cord (18), lesions at the time of the first attack are well-known predictors of a worse clinical evolution, these associations are only meaningful at a group level. That is, the prediction of the disease at the individual level based on these known predictors is still far from optimal. For that reason, over the years, a number of authors have aimed at predicting MS evolution based on these factors but through the development of AI models, with a much greater potential – at least theoretically – than classical statistical models. In spite of this, though, the ability to currently build (and publish) AI models to predict disease evolution based on MRI and clinical data is still limited. This limited ability becomes evident especially when a model built in a given cohort is applied in a completely unseen, independent, validation cohort, showing a much lower accuracy than expected (much lower than that of the original cohort). This possibly suggests that the variability across people with MS is probably larger than what we thought and that mismatches between accuracies in original (training and testing) cohorts and external validation cohorts may be due to an overfitting of the data by the model in the original cohorts. Additionally, this may also suggest that other aspects apart from MRI and clinical data may be playing a role in the evolution of the disease. Over the last 10 but especially over the last 5 years, some studies using AI models applied to biomarker data to explain concurrent and future disease evolution have started to emerge (Table 2).
Table 2. Summary of selected studies focused on prediction of disease course: relapses and disability accumulation.
Regarding the studies that have focused on the concurrent prediction of clinical outcomes, in 2019, Flauzino et al. (19), published a study where 122 people with MS were tested on several serum biomarkers to predict concurrent disability status. These biomarkers, which were related to the immune-inflammatory response, lipid and protein metabolic pathways, and oxidative stress, were able to predict which patients had an Expanded Disability Status Scale (EDSS) (20) score above or below 3.0 with high accuracy (Area under the ROC curve = 0.842). These results suggest that Immune inflammatory, metabolic and oxidative stress pathways may play a key role in disability accumulation in MS and deserve further research. In another interesting study focused on concurrent prediction, Brummer and colleagues (21) showed how serum neurofilament light (NfL) levels could improve our ability to detect cognitive dysfunction, especially when added to MRI predictors such as grey matter volume. The authors of this study not only built a ML model with high predictive accuracy, but also validated the ML model in an external cohort, supporting the generalisability of the model (21). Finally, we highlight the paper from Jackson and colleagues (22), where ML models based on random forest regression were built to predict a multi-dimensional score of disease severity using genetic variants previously identified as related to MS severity. Interestingly, the results, which could be validated in an external cohort, showed that the 19 most predictive genetic variants were located in 12 genes associated with immune cell regulation, complement activation and functions of neurons (22). This supports the robustness of the results while providing important insights on the mechanisms of progression in MS.
Regarding the studies with a longitudinal design, there is a high variability in terms of the length of the prediction period, ranging from 6 months to 11 years, and in terms of the nature of the predictor data, i.e., the input of the ML model. For instance, there are studies which have used genetic data, focusing on the presence of certain genetic variants or single nucleotide polymorphisms (SNPs) (23, 24). Other studies have focused instead on the presence of certain epigenetic mechanisms, such as DNA methylation (25), and on certain gene expression profiles (26, 27). Also, a few studies have demonstrated the ability of (immune) cellular profiles to predict clinical outcome (23). Finally, there are studies which have based their predictions on the presence of specific serum and CSF proteins and metabolites (28, 29). In relation to the output data, i.e., the outcome of the ML model, most studies focus on disability progression measures (19, 21–23, 25, 28, 30, 31), although some of them have chosen acute activity (generally MRI activity) outcomes (24, 26, 27, 32) and one focused on the development of anti-drug neutralising antibodies (33), known to reduce the effectiveness of the disease-modifying drug (33).
In relation to the studies which have used SNP data to predict future outcome, the article by Andorra et al. (23) is of special interest. In this study, not only SNPs located in Human Leukocyte Antigen (HLA) and non-HLA genes were considered as predictors, but also data on immune cell populations, proteomics, brain MRI, and optic coherence tomography (OCT) data. In this study, whose results were validated in an external cohort, the authors predicted the development of confirmed disability accumulation on different disability outcomes after 2 years of follow-up, with high sensitivity (23).
Among the studies with longest predictive periods, there is the paper by Uphaus et al. (28), which used NfL data to predict 6-year development of relapse-free progression and transition from RRMS to SPMS with high accuracies, especially for the former outcome and especially when combined with age and T2 lesion volume (28). More recently, Everest et al. (31) published a paper where CSF proteomics data was used to predict unfavourable evolutions over an 8-year follow-up period (on average) with very high accuracies. In this paper, which included an external validation analysis, the authors propose several novel candidate CSF protein biomarkers with a promising future in disease prediction modelling (31). Finally, Campagna et al. (25) exploited the DNA methylation profiles of 235 women with MS to predict disease severity over an 11-year period, again with high accuracy. Although this model was not externally validated in an independent cohort, the length of its prediction and the nature of the biomarker used make it especially relevant. Interestingly, those genes with greater levels of methylation seemed to be related to neuronal structure and function (25).
The pathophysiological processes in MS are not completely understood and are believed to be highly heterogeneous across people and disease stages. Fluid biomarker studies using AI to understand pathogenetic mechanisms could contribute to a greater characterisation of MS by expanding the concept of classical phenotypes (Table 3).
PBMCs can bear specific dysregulation in genes at different stages of MS. Acquaviva et al. (34) analysed transcriptomic profiles of PBMCs from individuals with CIS (n=57), RRMS (n=108), SPMS (n=26), PPMS (n=35), OND (n=27), and HC (n=60), divided into training (n=224) and validation (n=89) datasets. They defined classifiers (MS vs non-MS, relapsing vs progressive MS) using nested cross-validation in the training dataset. Then they used ward DT-based algorithms [RF, functional trees (FTs) and adaptive boosting applied to FT (ADAboost-FT) to evaluate their performance in the validation dataset. ADAboost-FT generated the best model to differentiate MS from non-MS (94.3% sensitivity, 87.5% precision). Identified transcripts in MS were related to interferon signalling, chromatin remodelling, and apoptosis. The relapsing vs progressive MS classifier showed 83.3% sensitivity and 93.8% precision. Associated biological themes included cell cycle and T cell activation for both progressive forms; protein ubiquitination, cell migration, and fatty acid metabolism for PPMS; and GTPase activity regulation, locomotor behaviour, and blood coagulation in SPMS.
MicroRNAs (miRNAs) play critical roles in post-transcriptomal gene expression regulation. In MS, miRNAs have been implicated in various aspects of the disease’s pathophysiology (35). Sun et al. (36) proposed a convolutional neural network (CNN)-based model to identify MS-related miRNAs and compared it to other existing methods: DT, SVM, logistic regression, and Gaussian Naïve Bayes. Using the miRNA-MS associations from the Human microRNA Disease Database (HMDD), the CNN model showed the highest ROC-AUC (0.87). Some of the top 10 predicted miRNAs were differentially expressed in RRMS or related to Th17 cell differentiation, whereas another one decreased after initiation of therapy with interferon β.
Lipid metabolism may influence inflammation, neurodegeneration, myelin damage, and repair processes in MS (37). Lötsch et al. (38) used unsupervised ML implemented as emergent self-organising feature maps (ESOM) combined with the U*-matrix visualisation technique to analyse eicosanoids, ceramides, and lysophosphatidic acids in serum of 102 PwMS and 301 HC, to find distance and density-based structures. Clear data structures were observed in eicosanoid and ceramide concentrations. Whereas the classification of MS vs HC yielded a moderate performance with eicosanoids (54% sensitivity, 100% specificity, 77% accuracy) the structures emerging with ceramides resulted in a high performance (89.2% sensitivity, 100% specificity, 94.6% accuracy).
An imbalance of oxidant and antioxidant molecules has been implicated in demyelination and axonal damage in MS. Mezzaroba et al. (39) used supervised ML (neural network analysis [NNA] and SVM with radial basis function [RBF/SVM]) to evaluate discriminatory patterns in plasma of 9 oxidants and antioxidants and zinc serum levels, in 174 PwMS and 182 controls. The combination of low levels of four antioxidants and increased levels of one oxidant yielded the best prediction for MS (sensitivity 98.7%, specificity 91.7%, AUC-ROC 0.990). The SVM analyses obtained 93.51% training and 92.03% validation accuracies (39).
Cytokines play an important role in Th cell differentiation and recruitment of auto-reactive T and B cells in MS. Goyal et al. (40) used four ML models (SVM, DT, RF, and neural networks) to identify serum cytokines predictive of MS. They also assessed the cytokines with age, sex, disease duration, EDSS, and MSSS to classify MS into remitting and non-remitting MS. They used 910 serum samples from PwMS and 199 from HC (total n=1109). Of these, 900 were included in the training set and 209 in the testing set. RF was the model that best predicted MS (sensitivity 75.6%, specificity 85.7%, accuracy 90.91%, ROC-AUC 0.957) and also had the highest accuracy (70%) to differentiate relapsing from non-relapsing MS. In the validation set, the RF model was again the best discriminator (40).
Neurofilament light chain (NfL) is a biomarker of axonal damage in MS (41). Seitz et al. (42) used SVM analysis to test for associations between baseline serum NfL (sNfL) and different retinal thickness measures in 156 early MS patients: 110 with no history of optic neuritis (ON) and 46 with ON. After adjusting for age, sex, disease duration, and EDSS, a significant correlation was found only between high sNfL levels and low outer plexiform layer (OPL) volume in patients with a history of ON. Follow-up OCTs available for 38 subjects with a mean (SD) follow-up of 2.1 (1.4) years showed baseline sNfL correlated with absolute OPL atrophy in ON. sNfL levels predicted OPL volume with 75.9% training and 76.2% testing accuracies. In the longitudinal analysis, sNfL predicted OPL atrophy with 72.5% training and 71.8% testing accuracies.
Other studies have focused on CSF biomarkers. Kosa et al. (43) used RF to search for biomarkers among 1305 proteins in CSF of 227 PwMS to build models predictive of disease severity. To differentiate natural aging and sex effects from MS-related mechanisms they used data from 24 HC. MS severity was assessed using the combinatorial weight-adjusted disability score (CombiWISE)-based MS Disease Severity Score (MS-DSS) measured at baseline and follow-up, and the brain volume deficit (BVD) severity outcome, based on linear regression models of brain parenchymal fraction and age, calculated from MRIs performed within 3 months of CSF collection. Initial analyses demonstrated positive associations of coagulation and complement cascades and negative associations for NOTCH signalling and neuron recognition categories with MS severity. After adjusting for age and sex, the model selected 75 biomarkers explaining 62% of variance for baseline MS-DSS. For follow-up MS-DSS, 34 biomarkers were selected and 35 for BVD explaining 60% of variance. The effect sizes decreased to 17%, 26%, and 22% of variance in the validation cohort (n=98). Using unsupervised cluster analyses, the authors identified seven patient clusters differing in CSF protein concentrations from four protein modules. Of note, one cluster had a predominance of men with progressive MS, a relatively low expression in the CNS repair module and high expression in the myeloid lineage/TNF and complement/coagulation modules. These patients had a higher MS severity.
Cellular characterisation in blood and CSF can help differentiate between CNS disorders and clarify their pathophysiological processes. Gross et al. (44) combined feature selection with dimensionality reduction and unsupervised cluster analyses to investigate parameters altered across autoimmune neuroinflammatory diseases [RRMS n=196, neuromyelitis optica spectrum disorders (NMOSD) n=15, Susac syndrome n=14, autoimmune encephalitis (AE) n=57], other CNS conditions (neurodegenerative n=93, vascular n=97), and non-inflammatory controls (n=74) (total n=546). The validation cohort included 231 additional subjects (neuroinflammatory n=32, neurodegenerative n=156, neurovascular n=8, non-inflammatory controls n=35). Exploratory analyses identified four CSF parameters and one peripheral blood parameter that together discriminated neuroinflammatory diseases from other groups (70% sensitivity, 81% specificity, 76% accuracy, ROC-AUC of 85%). When aiming to differentiate MS from other neuroinflammatory diseases, CSF plasma cells and intrathecal IgG synthesis alone were sufficient to distinguish RRMS from other neuroinflammatory diseases with high accuracy and ROC-AUC (NMOSD: 87.3% and 91.5%; Susac syndrome: 95.3% and 90.7%; AE: 89.4% and 82.7%). Finally, the authors compared cell profiles in RIS, CIS and early RRMS (≤36 months from disease onset) vs late RRMS (>36 months). Alterations in the proportions of CD56dim NK cells and biomarkers of intrathecal inflammation gradually increased during disease evolution. When splitting RRMS based on inflammatory activity, minor effects were shown in most intrathecal parameters, whereas changes in peripheral and intrathecal CD4+CD8+ T cells and intrathecal plasma cells were more pronounced.
AI-based studies using fluid biomarkers in MS offer promising results. However, these studies have limitations which are worth being mentioned. In general, all these studies still have relatively small sample sizes, which, together with the lack of external validation analyses in many of them, limit the generalisability of the results. Also, despite the low number of studies published so far, there is a large methodological variability, which, at times, is not explained in detail, making it very difficult to replicate the analyses done (Tables 1–3). These limitations are common to all AI-based studies that harness biomarker data to improve the diagnosis, predict or understand the disease, thus hampering the application of all these models to clinical practice.
In relation to the specific limitations of those studies focused on diagnosis, the number and types of diseases which have been compared with are limited. Furthermore, many of the tests (biomarkers) used by the authors are not available in routine clinical practice. These aspects reduce the utility of these models in practice, at least in the short term, suggesting the need for more research.
Regarding the studies focused on prediction of disease evolution, apart from the general limitations abovementioned, many of them have cross-sectional designs or, if they have a longitudinal design, there is a relatively short follow-up time in most of the cases. Also, very often, the effect of treatment is not taken into account. Furthermore, most studies were not adjusted for important demographic, clinical and technical aspects, such as race, ethnicity, disease duration, brain volume, and the interval between sampling and relapses or their treatment. Finally, despite the developments in AI-based models in MS which use raw neuroimaging and deep learning techniques to predict clinical outcome, the integration of these into AI-based models which use fluid biomarkers (or the other way around) is still lacking. Little is known about the complementary roles of both types of predictors and the potential synergies between them. However, it is highly likely that only when both are used together in comprehensive models, a real impact on the clinical management of MS can be achieved. Such integration requires, though, intensive methodological research which will hopefully bear fruit in the near future.
Lastly, regarding the limitations of the studies focused on understanding disease mechanisms, many of them are far too focused on certain paths or predictors, therefore not allowing us to explain or understand the whole picture. Also, very importantly, the fact that many of these biomarkers, paths, or predictors, may explain the same variance of a given outcome measure but we are not aware of that – because typically one study tends to focus on a given path – implies that many of the associations found may be reflecting mere epiphenomena rather than causally related events. Whereas this might be less relevant for building predictive models, for those studies which aim at understanding the disease through AI, this may be deleterious.
The application of AI-based methodologies to tackle key challenges in MS is exponentially increasing. However, in this context, the number of studies published in the literature focusing on the use of fluid biomarker data is still small. Most of these publications are focused on serum biomarkers, genetic variants, and gene expression profiles as predictors. Of note, only half of them have included an external validation analysis of the developed AI model, thus hampering a full interpretation of the results and their potential generalisability.
Importantly, after the assessment of the papers published so far, it may be said that the research on AI applied to biomarker data is still quite in its early days and that we are still far from clinical applications. So far, AI methodologies have been very useful for biomarker discovery in MS, but the large heterogeneity of methods and results suggests that we may need many years of research before prototypes can be launched to help healthcare professionals and patients in the clinic.
Along the same lines, even though many studies reported much higher accuracy levels when fluid biomarker, MRI, and clinical data were combined as predictors of diagnosis or disease evolution, large studies combining the most important types of predictor acquired in the clinic are lacking. Only when these take place and are replicated in large independent cohorts will we be able to comprehend their full potential and start considering that a change in patient management thanks to the introduction of those AI-based models is possible. Of note, for these models to be useful in the clinic, they need to use, as input data (predictors), routinely-acquired biomarkers, including laboratory, imaging, and clinical data. On the other hand, it is possible that a branch of AI-based research in MS, i.e., that focused on understanding the pathogenic mechanisms and those processes underlying disability accumulation, continues to exist with the use of less common (non-routinely acquired) biomarkers. This research is also important and will surely bring to light crucial knowledge on the disease, essential for its ultimate eradication. A final conclusion is that all studies carried out so far confirm the leading role of inflammatory pathways in MS.
Future directions include the development of larger studies with validation in independent datasets. Also, future directions should aim at the design of longitudinal studies with longer follow-ups (for those mainly focused on future prediction), hopefully accounting for the complex effects of disease-modifying treatments and other dynamic data, as well as the integration of fluid biomarkers, neuroimaging, optical coherence tomography (OCT) imaging, and clinical predictor data to build robust and powerful models.
Furthermore, forthcoming research endeavours must transition from the current exploratory phase of AI-based methodologies applied to biomarker data in MS to a more translational stage. This shift necessitates thorough evaluation of the clinical utility of the constructed AI models. For that, the future lies in creating guidelines for AI-based analyses to improve the comparability across studies, to shed light on the steps needed to go from discovery to clinical practice implementation, and to evaluate utility of AI-based algorithms in practice. Additionally, we should be able to learn from AI-based investigations on other neurodegenerative diseases (45) to overcome the challenges surrounding these types of studies.
As a final consideration, it is imperative to recognise that addressing ethical and inequality concerns surrounding AI-based analyses is just as crucial as resolving technical challenges. With the exponential growth of AI studies, maintaining research integrity in AI research demands not only initial attention but also ongoing evolution, keeping pace with the rapid advancement of science to meet the needs and expectations of us all.
GA: Conceptualization, Investigation, Methodology, Supervision, Visualization, Writing – original draft, Writing – review & editing. MC: Writing – original draft, Writing – review & editing. CT: Conceptualization, Investigation, Methodology, Supervision, Visualization, Writing – original draft, Writing – review & editing.
The author(s) declare financial support was received for the research, authorship, and/or publication of this article. CT is currently being funded by the Miguel Servet contract, awarded by the Instituto de Salud Carlos III (ISCIII), Spanish Ministry of Science and Innovation (award number: CP23/00117). She has also received research support from the ISCIII through the FORTALECE grant (FORT23/00034).
GA has received compensation for consulting services, speaking honoraria or participation in advisory boards from Merck, Roche, and Horizon Therapeutics; and travel support for scientific meetings from Novartis, Roche, ECTRIMS and EAN. She serves as editor for Europe of the Multiple Sclerosis Journal – Experimental, Translational and Clinical journal; and as a member of the editorial and scientific committee of Acta Neurológica Colombiana. She is a member of the International Women in Multiple Sclerosis iWiMS network executive committee, of the European Biomarkers in Multiple Sclerosis BioMS-eu steering committee, and of the MOGAD Eugene Devic European Network MEDEN steering group.
MC has received compensation for consulting services and speaking honoraria from Bayer Schering Pharma, Merck Serono, Biogen-Idec, Teva Pharmaceuticals, Sanofi-Aventis, Genzyme, and Novartis.
CT is currently being funded by a Miguel Servet contract, awarded by the Instituto de Salud Carlos III ISCIII, Ministerio de Ciencia e Innovación de España CP23/00117. She has also received a 2020 Junior Leader La Caixa Fellowship fellowship code: LCF/BQ/PI20/11760008, awarded by “la Caixa” Foundation ID 100010434, a 2021 Merck’s Award for the Investigation in MS, awarded by Fundación Merck Salud Spain, a 2021 Research Grant PI21/01860 awarded by the ISCIII, Ministerio de Ciencia e Innovación de España, and a FORTALECE research grant FORT23/00034 also by the ISCIII, Ministerio de Ciencia e Innovación de España. In 2015, she received an ECTRIMS Post-doctoral Research Fellowship and has received funding from the UK MS Society. She is a member of the Editorial Board of Neurology Journal and Multiple Sclerosis Journal. She has also received honoraria from Roche, Sanofi, Bristol-Myers Squibb, and Novartis and is a steering committee member of the O’HAND trial and of the Consensus group on Follow-on DMTs.
All claims expressed in this article are solely those of the authors and do not necessarily represent those of their affiliated organizations, or those of the publisher, the editors and the reviewers. Any product that may be evaluated in this article, or claim that may be made by its manufacturer, is not guaranteed or endorsed by the publisher.
1. Schwalbe N, Wahl B. Artificial intelligence and the future of global health. Lancet. (2020) 395:1579–86. doi: 10.1016/S0140-6736(20)30226-9
2. La Rosa F, Wynen M, Al-Louzi O, Beck ES, Huelnhagen T, Maggi P, et al. Cortical lesions, central vein sign, and paramagnetic rim lesions in multiple sclerosis: Emerging machine learning techniques and future avenues. NeuroImage Clin. (2022) 36:103205. doi: 10.1016/j.nicl.2022.103205
3. Reich DS, Lucchinetti CF, Calabresi PA. Multiple sclerosis. N Engl J Med. (2018) 378:169–80. doi: 10.1056/NEJMra1401483
4. Tumani H, Hartung H-P, Hemmer B, Teunissen C, Deisenhammer F, Giovannoni G, et al. Cerebrospinal fluid biomarkers in multiple sclerosis. Neurobiol Dis. (2009) 35:117–27. doi: 10.1016/j.nbd.2009.04.010
5. McCulloch W, Pitts W. A logical calculus of the ideas immanent in nervous activity. Bull Math Biophys. (1943) 5:115–133.
6. Solomon AJ, Arrambide G, Brownlee WJ, Flanagan EP, Amato MP, Amezcua L, et al. Differential diagnosis of suspected multiple sclerosis: an updated consensus approach. Lancet Neurol. (2023) 22:750–68. doi: 10.1016/S1474-4422(23)00148-5
7. Pasella M, Pisano F, Cannas B, Fanni A, Cocco E, Frau J, et al. Decision trees to evaluate the risk of developing multiple sclerosis. Front Neuroinform. (2023) 17:1248632. doi: 10.3389/fninf.2023.1248632
8. Guo P, Zhang Q, Zhu Z, Huang Z, Li K. Mining gene expression data of multiple sclerosis. PloS One. (2014) 9:e100052. doi: 10.1371/journal.pone.0100052
9. Wang R, Li B, Lam SM, Shui G. Integration of lipidomics and metabolomics for in-depth understanding of cellular mechanism and disease progression. J Genet Genomics. (2020) 47:69–83. doi: 10.1016/j.jgg.2019.11.009
10. Andersen SL, Briggs FBS, Winnike JH, Natanzon Y, Maichle S, Knagge KJ, et al. Metabolome-based signature of disease pathology in MS. Mult Scler Relat Disord. (2019) 31:12–21. doi: 10.1016/j.msard.2019.03.006
11. Lötsch J, Schiffmann S, Schmitz K, Brunkhorst R, Lerch F, Ferreiros N, et al. Machine-learning based lipid mediator serum concentration patterns allow identification of multiple sclerosis patients with high accuracy. Sci Rep. (2018) 8:14884. doi: 10.1038/s41598-018-33077-8
12. Probert F, Yeo T, Zhou Y, Sealey M, Arora S, Palace J, et al. Determination of CSF GFAP, CCN5, and vWF levels enhances the diagnostic accuracy of clinically defined MS from non-MS patients with CSF oligoclonal bands. Front Immunol. (2021) 12:811351. doi: 10.3389/fimmu.2021.811351
13. Gaetani L, Bellomo G, Di Sabatino E, Sperandei S, Mancini A, Blennow K, et al. The immune signature of CSF in multiple sclerosis with and without oligoclonal bands: A machine learning approach to proximity extension assay analysis. Int J Mol Sci. (2023) 25(1):139. doi: 10.3390/ijms25010139
14. Martynova E, Goyal M, Johri S, Kumar V, Khaibullin T, Rizvanov AA, et al. Serum and cerebrospinal fluid cytokine biomarkers for diagnosis of multiple sclerosis. Mediators Inflammation. (2020) 2020:2727042. doi: 10.1155/2020/2727042
15. Tintore M, Rovira À, Río J, Otero-Romero S, Arrambide G, Tur C, et al. Defining high, medium and low impact prognostic factors for developing multiple sclerosis. Brain. (2015) 138:1863–74. doi: 10.1093/brain/awv105
16. Chung KK, Altmann D, Barkhof F, Miszkiel KA, Brex PA, O'Riordan J, et al. A 30-Year Clinical and Magnetic Resonance imaging observational study of multiple sclerosis and clinically isolated syndromes. Ann Neurol. (2020) 87(1):63–74. doi: 10.1002/ana.25637
17. Scalfari A, Romualdi C, Nicholas RS, Mattoscio M, Magliozzi R, Morra A, et al. The cortical damage, early relapses, and onset of the progressive phase in multiple sclerosis. Neurology. (2018) 90:e2107–18. doi: 10.1212/WNL.0000000000005685
18. Brownlee WJ, Altmann DR, Prados F, Miszkiel KA, Eshaghi A, Gandini Wheeler-Kingshott CAM, et al. Early imaging predictors of long-term outcomes in relapse-onset multiple sclerosis. Brain. (2019) 142:2276–87. doi: 10.1093/brain/awz156
19. Flauzino T, Simão ANC, de Carvalho Jennings Pereira WL, Alfieri DF, Oliveira SR, Kallaur AP, et al. Disability in multiple sclerosis is associated with age and inflammatory, metabolic and oxidative/nitrosative stress biomarkers: results of multivariate and machine learning procedures. Metab Brain Dis. (2019) 34:1401–13. doi: 10.1007/s11011-019-00456-7
20. Kurtzke JF. Rating neurologic impairment in multiple sclerosis. Neurology. (1983) 33:1444–4. doi: 10.1212/WNL.33.11.1444
21. Brummer T, Muthuraman M, Steffen F, Uphaus T, Minch L, Person M, et al. Improved prediction of early cognitive impairment in multiple sclerosis combining blood and imaging biomarkers. Brain Commun. (2022) 4:fcac153. doi: 10.1093/braincomms/fcac153
22. Jackson KC, Sun K, Barbour C, Hernandez D, Kosa P, Tanigawa M, et al. Genetic model of MS severity predicts future accumulation of disability. Ann Hum Genet. (2020) 84:1–10. doi: 10.1111/ahg.12342
23. Andorra M, Freire A, Zubizarreta I, de Rosbo NK, Bos SD, Rinas M, et al. Predicting disease severity in multiple sclerosis using multimodal data and machine learning. J Neurol. (2024) 271(3):1133–49. doi: 10.1007/s00415-023-12132-z
24. Ferrè L, Clarelli F, Pignolet B, Mascia E, Frasca M, Santoro S, et al. Combining clinical and genetic data to predict response to fingolimod treatment in relapsing remitting Multiple Sclerosis patients: A precision medicine approach. J Pers Med. (2023) 13(1):122. doi: 10.3390/jpm13010122. Epub ahead of print.
25. Campagna MP, Xavier A, Lea RA, Stankovich J, Maltby VE, Butzkueven H, et al. Whole-blood methylation signatures are associated with and accurately classify multiple sclerosis disease severity. Clin Epigenet. (2022) 14:194. doi: 10.1186/s13148-022-01397-2
26. Fagone P, Mazzon E, Mammana S, Di Marco R, Spinasanta F, Basile MS, et al. Identification of CD4+ T cell biomarkers for predicting the response of patients with relapsing−remitting multiple sclerosis to natalizumab treatment. Mol Med Rep. (2019) 20:678–84. doi: 10.3892/mmr.2019.10283
27. Baranzini SE, Madireddy LR, Cromer A, D’Antonio M, Lehr L, Beelke M, et al. Prognostic biomarkers of IFNb therapy in multiple sclerosis patients. Mult Scler. (2015) 21:894–904. doi: 10.1177/1352458514555786
28. Uphaus T, Steffen F, Muthuraman M, Ripfel N, Fleischer V, Groppa S, et al. NfL predicts relapse-free progression in a longitudinal multiple sclerosis cohort study. EBioMedicine. (2021) 72:103590. doi: 10.1016/j.ebiom.2021.103590
29. Herman S, Arvidsson McShane S, Zjukovskaja C, Khoonsari PE, Svenningsson A, Burman J, et al. Disease phenotype prediction in multiple sclerosis. iScience. (2023) 26:106906. doi: 10.1016/j.isci.2023.106906
30. Zhu W, Chen C, Zhang L, Hoyt T, Walker E, Venkatesh S, et al. Association between serum multi-protein biomarker profile and real-world disability in multiple sclerosis. Brain Commun. (2023) 6(1):fcad300. doi: 10.1093/braincomms/fcad300
31. Everest E, Uygunoglu U, Tutuncu M, Bulbul A, Onat UI, Unal M, et al. Prospective outcome analysis of multiple sclerosis cases reveals candidate prognostic cerebrospinal fluid markers. PloS One. (2023) 18:e0287463. doi: 10.1371/journal.pone.0287463
32. Ebrahimkhani S, Beadnall HN, Wang C, Suter CM, Barnett MH, Buckland ME, et al. Serum exosome MicroRNAs predict multiple sclerosis disease activity after fingolimod treatment. Mol Neurobiol. (2020) 57:1245–58. doi: 10.1007/s12035-019-01792-6
33. Waddington KE, Papadaki A, Coelewij L, Adriani M, Nytrova P, Kubala Havrdova E, et al. Using serum metabolomics to predict development of anti-drug antibodies in multiple sclerosis patients treated with IFNβ. Front Immunol. (2020) 11:1527. doi: 10.3389/fimmu.2020.01527
34. Acquaviva M, Menon R, Di Dario M, Dalla Costa G, Romeo M, Sangalli F, et al. Inferring multiple sclerosis stages from the blood transcriptome via machine learning. Cell Rep Med. (2020) 1:100053. doi: 10.1016/j.xcrm.2020.100053
35. Junker A, Hohlfeld R, Meinl E. The emerging role of microRNAs in multiple sclerosis. Nat Rev Neurol. (2011) 7:56–9. doi: 10.1038/nrneurol.2010.179
36. Sun X, Ren X, Zhang J, Nie Y, Hu S, Yang X, et al. Discovering miRNAs associated with Multiple Sclerosis based on network representation learning and deep learning methods. Front Genet. (2022) 13:899340. doi: 10.3389/fgene.2022.899340
37. Lorincz B, Jury EC, Vrablik M, Ramanathan M, Uher T. The role of cholesterol metabolism in multiple sclerosis: From molecular pathophysiology to radiological and clinical disease activity. Autoimmun Rev. (2022) 21:103088. doi: 10.1016/j.autrev.2022.103088
38. Lötsch J, Thrun M, Lerch F, Brunkhorst R, Schiffmann S, Thomas D, et al. Machine-learned data structures of lipid marker serum concentrations in multiple sclerosis patients differ from those in healthy subjects. Int J Mol Sci. (2017) 18(6):1217. doi: 10.3390/ijms18061217
39. Mezzaroba L, Simão ANC, Oliveira SR, Flauzino T, Alfieri DF, de Carvalho Jennings Pereira WL, et al. Antioxidant and anti-inflammatory diagnostic biomarkers in multiple sclerosis: A machine learning study. Mol Neurobiol. (2020) 57:2167–78. doi: 10.1007/s12035-019-01856-7
40. Goyal M, Khanna D, Rana PS, Khaibullin T, Martynova E, Rizvanov AA, et al. Computational intelligence technique for prediction of multiple sclerosis based on serum cytokines. Front Neurol. (2019) 10:781. doi: 10.3389/fneur.2019.00781
41. Khalil M, Teunissen CE, Lehmann S, Otto M, Piehl F, Ziemssen T, et al. Neurofilaments as biomarkers in neurological disorders — towards clinical application. Nat Rev Neurol. (2024) 20(5):269–87. doi: 10.1038/s41582-024-00955-x
42. Seitz CB, Steffen F, Muthuraman M, Uphaus T, Krämer J, Meuth SG, et al. Serum neurofilament levels reflect outer retinal layer changes in multiple sclerosis. Ther Adv Neurol Disord. (2021) 14:17562864211003478. doi: 10.1177/17562864211003478
43. Kosa P, Barbour C, Varosanec M, Wichman A, Sandford M, Greenwood M, et al. Molecular models of multiple sclerosis severity identify heterogeneity of pathogenic mechanisms. Nat Commun. (2022) 13:7670. doi: 10.1038/s41467-022-35357-4
44. Gross CC, Schulte-Mecklenbeck A, Madireddy L, Pawlitzki M, Strippel C, Räuber S, et al. Classification of neurological diseases using multi-dimensional CSF analysis. Brain. (2021) 144:2625–34. doi: 10.1093/brain/awab147
45. Kaur A, Mittal M, Bhatti JS, Thareja S, Singh S. A systematic literature review on the significance of deep learning and machine learning in predicting Alzheimer’s disease. Artif Intell Med. (2024) 154:102928. doi: 10.1016/j.artmed.2024.102928
46. Weideman AM, Barbour C, Tapia-Maltos MA, Tran T, Jackson K, Kosa P, et al. New multiple sclerosis disease severity scale predicts future accumulation of disability. Front Neurol. (2017) 8:598. doi: 10.3389/fneur.2017.00598
Keywords: multiple scleorsis (MS), fluid biomarkers, demyelinating, machine learning and AI, deep learning
Citation: Arrambide G, Comabella M and Tur C (2024) Big data and artificial intelligence applied to blood and CSF fluid biomarkers in multiple sclerosis. Front. Immunol. 15:1459502. doi: 10.3389/fimmu.2024.1459502
Received: 04 July 2024; Accepted: 30 September 2024;
Published: 18 October 2024.
Edited by:
Axel Faes, University of Hasselt, BelgiumReviewed by:
Veronica Popescu, University of Hasselt, BelgiumCopyright © 2024 Arrambide, Comabella and Tur. This is an open-access article distributed under the terms of the Creative Commons Attribution License (CC BY). The use, distribution or reproduction in other forums is permitted, provided the original author(s) and the copyright owner(s) are credited and that the original publication in this journal is cited, in accordance with accepted academic practice. No use, distribution or reproduction is permitted which does not comply with these terms.
*Correspondence: Georgina Arrambide, Z2FycmFtYmlkZUBjZW0tY2F0Lm9yZw==; Carmen Tur, Y3R1ckBjZW0tY2F0Lm9yZw==
Disclaimer: All claims expressed in this article are solely those of the authors and do not necessarily represent those of their affiliated organizations, or those of the publisher, the editors and the reviewers. Any product that may be evaluated in this article or claim that may be made by its manufacturer is not guaranteed or endorsed by the publisher.
Research integrity at Frontiers
Learn more about the work of our research integrity team to safeguard the quality of each article we publish.