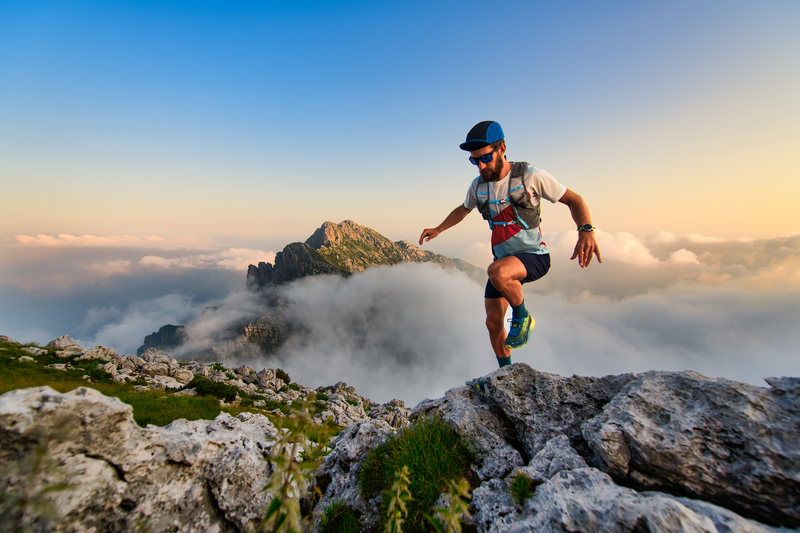
94% of researchers rate our articles as excellent or good
Learn more about the work of our research integrity team to safeguard the quality of each article we publish.
Find out more
ORIGINAL RESEARCH article
Front. Immunol. , 09 October 2024
Sec. Cancer Immunity and Immunotherapy
Volume 15 - 2024 | https://doi.org/10.3389/fimmu.2024.1444091
This article is part of the Research Topic Clinical prediction models in cancer through bioinformatics View all 4 articles
Background: The prognostic value and immune significance of T-cell proliferation regulators (TCRs) in hepatocellular carcinoma (HCC) have not been previously reported. This study aimed to develop a new prognostic model based on TCRs in patients with HCC.
Method: This study used The Cancer Genome Atlas-Liver Hepatocellular Carcinoma (TCGA-LIHC) and International Cancer Genome Consortium-Liver Cancer-Riken, Japan (ICGC-LIRI-JP) datasets along with TCRs. Differentially expressed TCRs (DE-TCRs) were identified by intersecting TCRs and differentially expressed genes between HCC and non-cancerous samples. Prognostic genes were determined using Cox regression analysis and were used to construct a risk model for HCC. Kaplan-Meier survival analysis was performed to assess the difference in survival between high-risk and low-risk groups. Receiver operating characteristic curve was used to assess the validity of risk model, as well as for testing in the ICGC-LIRI-JP dataset. Additionally, independent prognostic factors were identified using multivariate Cox regression analysis and proportional hazards assumption, and they were used to construct a nomogram model. TCGA-LIHC dataset was subjected to tumor microenvironment analysis, drug sensitivity analysis, gene set variation analysis, and immune correlation analysis. The prognostic genes were analyzed using consensus clustering analysis, mutation analysis, copy number variation analysis, gene set enrichment analysis, and molecular prediction analysis.
Results: Among the 18 DE-TCRs, six genes (DCLRE1B, RAN, HOMER1, ADA, CDK1, and IL1RN) could predict the prognosis of HCC. A risk model that can accurately predict HCC prognosis was established based on these genes. An efficient nomogram model was also developed using clinical traits and risk scores. Immune-related analyses revealed that 39 immune checkpoints exhibited differential expression between the high-risk and low-risk groups. The rate of immunotherapy response was low in patients belonging to the high-risk group. Patients with HCC were further divided into cluster 1 and cluster 2 based on prognostic genes. Mutation analysis revealed that HOMER1 and CDK1 harbored missense mutations. DCLRE1B exhibited an increased copy number, whereas RAN exhibited a decreased copy number. The prognostic genes were significantly enriched in tryptophan metabolism pathways.
Conclusions: This bioinformatics analysis identified six TCR genes associated with HCC prognosis that can serve as diagnostic markers and therapeutic targets for HCC.
Hepatocellular carcinoma (HCC), a major health issue, is the sixth most common malignancy and the third most common cause of cancer-related mortality (1). The incidence and mortality rates of HCC are high in Asian and African populations, which account for approximately 75% of new HCC cases worldwide. The annual incidence of new HCC cases is approximately 780,000, with approximately 600,000 deaths worldwide. China accounts for 55% of the global HCC incidence (2). The high HCC incidence in China is closely linked to various factors, such as viral hepatitis, chronic alcohol abuse, obesity, and diabetes (3). As the symptoms are not prominent in the early stages, 70%–80% of patients are diagnosed with advanced-stage HCC (4). Currently, the therapeutic modalities for HCC include liver transplantation, surgical resection, radiofrequency ablation, transarterial chemoembolization (TACE), and targeted drug therapy. The 5-year overall survival (OS) rate of patients with early-stage HCC after radical surgery can be as high as 80%. However, the survival rate of patients with advanced-stage HCC is less than 20% (5–9). Recently, immunotherapy, especially T-cell-related therapies involving immune checkpoint inhibitors (ICIs), has revolutionized the treatment landscape for advanced HCC. However, the overall objective response rate of patients undergoing ICI therapy is low (10, 11), which can be because the role of the immune microenvironment in HCC pathogenesis has not been elucidated.
T cells, including cytotoxic T cells, T helper cells, and T regulatory (Treg) cells, play a crucial role in the tumor microenvironment (12). Expression of inhibitory molecules, such as programmed death ligand 1 (PD-L1), promotes the malignant proliferation of HCC cells. The binding of PD-L1 to its receptor (PD-1) suppresses T-cell activation and proliferation, impedes the ability of immune cells to attack tumors, and promotes malignant growth (13). ICIs, which are a type of immunotherapeutic agents, target the PD-1/PD-L1 pathway in the immune microenvironment and can potentiate anti-tumor effects by boosting the immune response of T cells (14). Systemic therapies, including ICIs, have significantly improved the OS rate and quality of life of patients with HCC. However, the tumor microenvironment and the immune escape mechanism confer HCC with primary or adaptive resistance to these systemic therapies (14, 15). Only 10%–35% of patients experience sustained clinical benefits from these therapies (16). Currently, the 5-year survival rate of patients with advanced and intermediate HCC is less than 20% (11). Therefore, targeting the immune microenvironment of HCC, especially the activation and inhibition of T cells in this microenvironment, can significantly impact HCC therapy response and improve the prognosis of patients.
T cell proliferation regulators (TCRs), which are a diverse group of molecules, including proteins, enzymes, receptors, and signaling molecules, are involved in T-cell development, differentiation, proliferation, and function (17). In the tumor immune environment, the Expression and function of TCRs may be dysregulated, which can impair the ability of T cells to recognize and clear tumor cells. For example, CDK1 can influence tumor immunity by regulating the migration of immune cells into the bladder cancer microenvironment. CDK1 is also associated with tumor mutational burden (TMB) and microsatellite instability (18). CXCL12 is highly enriched in fibroblasts and can promote the proliferation of T cells in bladder cancer microenvironment. CXCL12 specifically interacts with CXCR4 expressed on the surface of T cells and macrophages (19). Cell cycle suppressor proteins, such as p27 and p21, play a major role in T-cell proliferation (20). The Expression of immune checkpoint molecules (such as PD-1 and CTLA-4) also affects the function of TCRs, which subsequently influence the anti-tumor activity of T cells (21). Therefore, targeting or modulating specific TCRs can potentially enhance the anti-tumor activity of T cells or improve the efficacy of immunotherapy. For example, inhibiting negative regulators in the tumor microenvironment or enhancing the Expression of positive regulators improves the cytotoxic effects of T cells against tumors. Additionally, TCRs serve as a marker for tumors to predict prognosis and guide treatment. In clear cell renal cell carcinoma (ccRCC), T-cell proliferation-related regulatory genes (TPRGs) are used to predict prognosis, identify tumor types (such as hot and cold tumors), and guide treatment (22). Previously, we analyzed the expression characteristics of 35 TPRGs and their somatic mutations in TPRG-associated subtypes, OS, biological pathways, and immunity in lung adenocarcinoma (LUAD) and developed a TPRG-associated risk model based on six genes. This model could accurately predict TPRG prognosis and immunotherapy response (23). However, the specific mechanism of TCRs in the tumor microenvironment and their roles in the immunotherapy response and prognosis of HCC have not been elucidated.
This study examined the role of TCRs in HCC using The Cancer Genome Atlas-Liver Hepatocellular Carcinoma (TCGA-LIHC) and International Cancer Genome Consortium-Liver Cancer-Riken, Japan (ICGC-LIRI-JP) datasets. The intersection of TCRs and differentially expressed genes (DEGs) between HCC and non-cancerous samples provided differentially expressed TCRs (DE-TCRs). Prognostic genes were identified using Cox regression analysis to establish an HCC risk model. The roles of these prognostic genes in HCC and their impact on prognosis were analyzed. Additionally, the mutational spectrum, immune cell infiltration, immunotherapy efficacy, and chemotherapy efficacy were examined. This study aimed to elucidate the effects of TCRs on the progression and prognosis of HCC and offer novel insights into HCC treatment strategies.
The RNA-sequencing data (HTSeq-Counts and HTSeq-FPKM) and clinical characteristics of the TCGA-LIHC cohort were extracted from the UCSC Xena database (http://xena.ucsc.edu/). TCGA-LIHC dataset, comprising the data of 50 non-cancerous and 365 HCC samples, served as the training set. The RNA-sequencing data and clinical information of the ICGC-LIRI-JP cohort were obtained from the ICGC database. The ICGC-LIRI-JP dataset, comprising the survival and gene expression data of 232 patients with HCC, served as the testing set. This study retrieved 35 TCRs from previous studies (19, 24). The immunophenoscore (IPS) and tumor immune dysfunction and exclusion (TIDE) scores of patients with HCC were obtained from The Cancer Imaging Archive and TIDE databases, respectively.
DEGs between non-cancerous and HCC samples in TCGA-LIHC datasets were identified using the R package DEseq2 (p < 0.05 and |log2 fold-change (FC)| > 0.5) (25). These DEGs were then overlapped with TCRs using the R package ggvenn (26) to obtain differentially expressed TCRs (DE-TCRs). The DE-TCRs were subjected to Gene Ontology (GO) and Kyoto Encyclopedia of Genes and Genomes (KEGG) using the clusterProfiler package with a significance threshold set at p < 0.05 (27).
A risk model for HCC was developed using TCGA-LIHC and ICGC-LIRI-JP datasets. TCGA-LIHC dataset was subjected to univariate Cox regression analysis to identify DE-TCRs using the coxph function in the R package “survival” (27). Prognosis-related genes were screened based on the following conditions: hazard ratio (HR) ≠ 1, p < 0.05. Subsequently, the least absolute shrinkage and selection operator (LASSO) algorithm was employed to identify prognostic genes (family = “cox,” nfold = 10) (28). A risk model was established using TCGA-LIHC dataset. The risk score was calculated as follows: . The HCC samples were divided into high-risk and low-risk groups based on the median risk score, and Kaplan-Meier (K-M) survival analysis was performed to assess the difference in survival between the two groups. Additionally, to further verify the validity of the risk model, we plotted receiver operating characteristic (ROC) curve and calculated the area under the curve (AUC) of the model to assess its accuracy. Meanwhile, validation was performed with the ICGC-LIRI-JP dataset. Finally, the significance of differential prognostic gene expression levels between HCC and non-cancerous samples in the TCGA-LIHC dataset was analyzed using the Wilcoxon test.
Univariate and multivariate Cox regression analyses and proportional hazards assumption were used to identify independent prognostic factors. The clinicopathological factors (N-stage, risk score, gender, T-stage, race, age, M-stage, and grade) of the HCC cohort (TCGA-LIHC) were systematically analyzed (29). A nomogram model was constructed using these independent prognostic factors with the R package rms (30). ROC, calibration, and decision curve analysis (DCA) curves were generated to further verify the validity of the model.
To investigate the biological pathways of prognostic genes, the KEGG background gene set (c2.cp.kegg.v2023.1.Hs.symbols.gmt), which was extracted from the Molecular Signature Database (MSigDB), was subjected to gene set variation analysis (GSVA) (p < 0.05) (27). The background gene set h.all.v2023.1.Hs.symbols.gmt was downloaded from the MSigDB to examine differential regulatory pathways between the high-risk and low-risk groups in TCGA-LIHC cohort (27). All genes in the risk groups were subjected to GSVA using the R package GSVA (31) and differential analysis using the R package limma (32).
To evaluate the association between the risk score and immune cell infiltration in patients with HCC, six types of immune cells were analyzed using the Tumor Immune Estimation Resource algorithm in the R package IOBR (33). To further assess differences in immune status among patients with HCC, immune-related pathways, and immune cell infiltration were analyzed using the single-sample gene set enrichment analysis (ssGSEA) algorithm within the R package ‘GSVA’ (significance threshold: p < 0.05) (31). The correlations between the risk score and immune cells, as well as between prognostic genes and differential immune checkpoints, were determined using Spearman’s rank correlation analysis.
Patient response to immunotherapy was assessed using the IPS and TIDE scores. Immunotherapy responses in the high-risk and low-risk groups in the TCGA-LIHC dataset were evaluated based on outcomes predicted by the TIDE algorithm. Additionally, the half-maximal inhibitory concentration (IC50) values of 138 drugs against HCC were evaluated using the R package pRRophetic (34) to determine the sensitivity of patients with HCC to these drugs.
To identify molecular subtypes based on prognostic genes in HCC samples from TCGA-LIHC dataset, consensus clustering was performed using the R package ‘ConsensusClusterPlus’ (35). The K-M curve was plotted using the R package survminer (36) to evaluate the differential OS between patients with different subtypes (p < 0.05). Additionally, differential immune cell infiltration statuses between these subtypes were analyzed (p < 0.05).
The data on the single nucleotide polymorphism mutation site of patients with HCC were extracted from the TCGA database. The R package maftools was used to analyze the mutation data of high-risk, low-risk, and prognostic genes (37). To elucidate the relationship between TMB and prognostic genes, the TMB of patients with HCC was calculated using the R package survminer (36). Based on the median risk score and the median TMB, patients with HCC in TCGA-LIHC dataset were grouped as follows to examine survival differences: H_TMB-H_risk, H_TMB-L_risk, L_TMB-H_risk, and L_TMB-L_risk. Additionally, somatic gene copy number data of TCGA-LIHC dataset were downloaded from the UCSC database (https://genome.ucsc.edu/). The R package OmicCircos (27) was used to analyze the chromosomal location of CNV in prognostic genes.
miRNAs targeting prognostic genes were predicted using the miRDB database (https://mirdb.org/). Subsequently, microRNAs (miRNAs) targeting long non-coding RNAs (lncRNAs) were predicted using the miRNet database (https://www.mirnet.ca/) to establish the mRNA-miRNA-lncRNA regulatory network. Transcription factors (TFs) regulating the prognostic genes were predicted using the Transcriptional Regulatory Relationships Unraveled by Sentence-based Text mining database. The mRNA-miRNA-lncRNA and miRNA/TF-gene regulatory networks were visualized using Cytoscape software.
Five pairs of non-cancerous and HCC samples were collected from the General Hospital of Ningxia Medical University. Informed consent was obtained from all participants. This study was approved by the Ethics Committee of the General Hospital of Ningxia Medical University (approval number: KYLL-2021-229).
Total RNA was extracted using the TRIzol reagent (Invitrogen, USA), following the manufacturer’s instructions. The isolated RNA was reverse-transcribed into complementary DNA (cDNA) using the SureScript First-strand cDNA Synthesis kit (Servicebio, China). The qRT-PCR analysis was performed with CFX Connect Thermal Cycler (Bio-Rad, USA). The relative expression levels of mRNAs were calculated using the 2−ΔΔCt method. The sequences of all primers are shown in Supplementary Table 1.
Bioinformatics analyses were performed using R language (v. 4.2.2). The relationship between two variables was assessed using Spearman correlation analysis. Cox regression analysis was performed to determine the factors influencing the survival status. LASSO regression analysis was performed to reduce model complexity and avoid overfitting. K-M curves were plotted to determine the differential survival between the high-risk and low-risk groups. The significant differences between the survival curves were determined using the Log-rank test. ROC curves were plotted to assess the validity of the risk model. Data from different groups were compared using the Wilcoxon test (P < 0.05).
In total, 8,525 DEGs were identified between HCC and non-cancerous samples (5,853 upregulated genes and 2,672 downregulated genes) (Figures 1A, B). The DEGs were intersected with TCRs to obtain 18 DE-TCRs (Figure 1C). DE-TCRs were enriched in various GO terms, including telomeric region, chromosome, positive regulation of protein localization to the nucleus, and amyloid-beta binding (Figure 1D). Additionally, DE-TCRs were enriched in several KEGG pathways, such as amphetamine addiction, alcoholism, and cytokine-cytokine receptor interaction (Figure 1E).
Figure 1. Identification and functional enrichment analysis of differentially expressed T-cell proliferation regulators (DE-TCRs). (A) The heatmap of differentially expressed genes. In the middle annotation bar, non-cancerous and HCC samples are indicated in blue and red colors, respectively. The intensity of the color in the heatmap signifies gene expression density per sample, with darker colors indicating higher density. The y-axis of the lower heatmap represents genes (red and blue colors indicate upregulation and downregulation, respectively). (B) Volcano plot of differentially expressed genes. The x-axis shows the log2 fold-change (FC) values, while the y-axis shows the −log10 (adjusted p-values). Each point represents a gene. The horizontal reference line represents −log10 (0.05) = 1.3, while the vertical reference line represents log2 (FC) = ± 0.5. Divided by the reference line, upper right genes are upregulated genes (red), and upper left genes are downregulated genes (blue). Gray represents genes with non-significant differences in expression levels. The top 10 upregulated genes and the top 10 downregulated genes with the largest log2 (FC) values are marked. (C) Venn diagram for DE-TCR identification. Pink and blue represent genes unique to the differentially expressed gene set and the TCR set, respectively. (D) Gene Ontology (GO) enrichment (Top 5). Each column represents a GO term. The color of the column represents different GO categories. The length represents the number of genes enriched in the term. (E) Kyoto Encyclopedia of Genes and Genomes (KEGG) enrichment. The color transition from red (high) to blue (low) indicates log2 (FC) values of the genes. Each pathway is represented by a distinct color.
Univariate Cox regression analysis of 18 DE-TCRs revealed the following 10 prognosis-related genes (HR ≠ 1 and p < 0.05): DCLRE1B, RAN, HOMER1, ADA, CDK1, IL1RN, CLIC1, BATF, LIG3, and CYP27A1 (Figure 2A). LASSO Cox regression analysis identified the following six prognostic genes: DCLRE1B, RAN, HOMER1, ADA, CDK1, and IL1RN (Figure 2B). GSEA revealed that the prognostic genes were significantly enriched in pathways related to primary bile acid biosynthesis (Figure 2C). All prognostic genes, except IL1RN, were upregulated in HCC (Figure 2D).
Figure 2. Prognostic gene screening and analysis. (A) Forest plot of Cox univariate analysis results. The leftmost side lists the prognostic genes. The three columns of numbers on the right represent the hazard ratio (HR) values corresponding to the gene, the 95% confidence interval of the HR value, and the p-value. (B) Least absolute shrinkage and selection operator (LASSO) analysis. The left figure demonstrates cross-validation with the middle line marking the minimum error. The optimal log (lambda) value is determined. Key genes and their coefficients are then identified in the right figure based on the lambda value. (C) Gene set enrichment analysis (GSEA) (Top 5) of characteristic genes. The figure has three sections. The top section displays the enrichment score calculated for each gene, linked into a line graph. The middle section visualizes the rank of each gene within the set. The bottom section depicts the overall rank distribution of all genes. (D) Analysis of the Expression of characteristic genes in hepatocellular carcinoma (HCC) versus control samples.
Figure 3A shows the distribution of HCC samples in the two risk groups in TCGA-LIHC cohort. DCLRE1B, RAN, HOMER1, ADA, and CDK1 were upregulated, whereas IL1RN was downregulated in the high-risk group (Figure 3B). K-M survival curve analysis indicated that patients in the high-risk group exhibited decreased survival probabilities (Figure 3C). Time-dependent ROC validation confirmed the efficacy of the model (area under the curve (AUC) values for predicting 1-year, 2-year, and 3-year survival were 0.75, 0.68, and 0.66, respectively) (Figure 3D). The findings of the ICGC-LIRI-JP dataset analysis were consistent with those of TCGA-LIHC dataset analysis (Figures 3E–G). The AUC values for predicting 1-year, 2-year, and 3-year survival in the ICGC-LIRI-JP dataset were 0.71, 0.77, and 0.80, respectively (Figure 3H).
Figure 3. Predictive performance of the risk model. (A, E) Risk curve and survival status distribution of hepatocellular carcinoma (HCC) samples in the training and validation sets. The x-axis represents the risk score, increasing from left to right. In the upper part, red and blue points indicate high-risk and low-risk patients, respectively. In the lower part, red and blue dots denote deceased and surviving patients, respectively. (B, F) Characteristic gene expression analysis in the training and validation sets. The y-axis lists six characteristic genes. Red and blue colors indicate upregulation and downregulation, respectively. High-risk and low-risk groups are shown in red and blue colors, respectively. (C, G) Kaplan-Meier survival curves of patients in the training and validation sets. Red and blue represent high-risk and low-risk groups, respectively. (D, H) Receiver operating characteristic (ROC) curve of the training and validation sets.
Cox regression analysis identified the risk score and T-stage were independent prognostic factors (Figure 4A). A nomogram model was constructed based on these independent prognostic factors (Figure 4B). The calibration curves for 1-year, 2-year, and 3-year survival revealed that the predicted outcomes were consistent with the actual outcomes, indicating that the model can accurately predict HCC prognosis (Figure 4C). The AUC values of the nomogram for predicting 1-year, 2-year, and 3-year survival were more than 0.6. Thus, the predictive accuracy of the nomogram was higher than that of the risk score or T-stage alone (Figure 4D). In the high-risk group, 14 pathways were activated, including those related to immunization, such as HALLMARK_NOTCH_SIGNALING and HALLMARK_WNT_BETA_CATENIN_SIGNALING. In contrast, 21 pathways were inhibited in the high-risk group, including HALLMARK_COAGULATION and HALLMARK_ XENOBIOTIC_METABOLISM (Figure 4E).
Figure 4. Application of the nomogram model for clinical hepatocellular carcinoma (HCC) cases. (A) Results of univariate and multivariate Cox regression analysis: The leftmost column shows the risk score and clinical characteristics, while the two right columns show the corresponding p-values and hazard ratio (HR) values. (B) Nomogram. (C) Nomogram calibration curves for predicting 1-year, 2-year, and 3-year survival rates. The x-axis shows the predicted event rate, while the y-axis shows the observed event rate (both ranging from 0 to 1). (D) The receiver operating characteristic (ROC) curves for predicting the 1-year, 2-year, and 3-year survival rates. (E) The upper panel shows the pathways activated and inhibited in the high-risk and low-risk groups (Top 5). The horizontal axis represents the samples (pink and blue colors indicate the high-risk and low-risk groups, respectively). The vertical axis represents the pathway (red and blue colors indicate the enriched and suppressed pathways, respectively). The lower panel shows the gene set variant analysis (GSVA) results.
The development of tumors is closely linked to the immune microenvironment. Immune cell infiltration was positively correlated with the risk score in patients with HCC. The infiltration levels of six immune cell types were upregulated in the high-risk group (Figure 5A). Meanwhile, the infiltration levels of 11 immune cell types, including eosinophil, varied between the high-risk and low-risk groups. The infiltration levels of most of these 11 immune cell types were upregulated in the high-risk group (Figure 5B). Additionally, the levels of five immune-related pathways, including the type II IFN response, varied between the high-risk and low-risk groups (Figure 5C).
Figure 5. Correlation between risk scores and the tumor microenvironment of hepatocellular carcinoma (HCC). (A) The Tumor Immune Estimation Resource (TIMER) algorithm assesses the relationship between the risk score and immune cell infiltration. Yellow and blue represent the high-risk and low-risk groups, respectively. Each image is divided into two parts: correlation analysis (left) and differential analysis (right). (B) Analysis of differential immune cell infiltration levels using the single-sample gene set enrichment analysis (ssGSEA) algorithm. (C) Analysis of differential immune-related pathways using the ssGSEA algorithm. * p< 0.05, ** p< 0.01, *** p< 0.001, **** p< 0.0001.
Immune checkpoints play a crucial role in mitigating autoimmune effects. In TCGA-LIHC cohort, 39 immune checkpoints were differentially expressed between the high-risk and low-risk groups (Figure 6A). The expression levels of most prognostic genes were positively correlated with those of 39 immune checkpoints. ADA exhibited the strongest positive correlation with TNFRSF18 (cor = 0.58), whereas IL1RN exhibited the strongest negative correlation with NRP1 (cor = −0.23) (Figure 6B). The levels of CTLA4-negative response and PD-1-negative response (ips_ctla4_neg_pd1_neg) and CTLA4-positive and PD-1-negative response (ips_ctla4_pos_pd1_neg) were downregulated in the high-risk group (Figure 6C). Furthermore, the high-risk group exhibited increased exclusion scores and decreased dysfunction scores, indicating that immune escape in this group was predominantly due to immune exclusion, which contributes to decreased responses to immunotherapy (Figures 6D, E).
Figure 6. Prognostic genes can affect the immunotherapy efficacy in patients with hepatocellular carcinoma (HCC). (A) Expression of immune checkpoints in the high-risk and low-risk groups. (B) Correlation between characteristic genes and differential immune checkpoints (Dot size represents significance; red and blue colors indicate positive and negative correlations, respectively; darker shades indicate stronger correlations). (C) Immunephenoscore (IPS) of the high-risk and low-risk groups (red and green colors indicate high-risk and low-risk, respectively). Each image features a density plot at the top and a scatter plot with a box plot at the bottom. (D) Tumor immune dysfunction and exclusion (TIDE) scores of the high-risk and low-risk groups (yellow and blue colors indicate the high-risk and low-risk groups, respectively). (E) Immune response ratio of patients in the high-risk and low-risk groups. * p< 0.05, ** p< 0.01, *** p< 0.001, **** p< 0.0001.
HCC samples were categorized into the following two subtypes via consensus clustering: cluster 1, comprising 156 samples; and cluster 2, comprising 209 samples. The t-distributed stochastic neighbor embedding (tSNE) dimensionality reduction analysis distinguished the two subtypes, revealing variations in prognostic gene expression (Figure 7A). The K-M curve analysis revealed that the OS significantly varied between cluster 1 and cluster 2 with cluster 1 exhibiting poor survival (Figures 7B, C). Additionally, the infiltration levels of 11 immune cell types, including eosinophils, varied between cluster 1 and cluster 2 (Figure 7D).
Figure 7. Differential characteristics of different subtypes of hepatocellular carcinoma (HCC). (A, B) Consistent clustering of characteristic genes in patients with HCC. (C) Kaplan-Meier (K-M) curves of HCC subtypes. The x-axis represents overall survival time in days, while the y-axis shows survival probability. Different colors represent different HCC subtypes. (D) Differential immune cell infiltration levels in HCC subtypes. * p< 0.05, ** p< 0.01, **** p< 0.0001.
Chemotherapy is a common treatment for malignant tumors. Analysis of 138 anti-cancer drugs revealed that the IC50 values of 108 drugs varied between the high-risk and low-risk groups. Figure 8A shows the 10 drugs (including A.443654, BI.2536, and BIRB.0796) with the most marked differences in IC50 values (Figure 8A). The frequency of mutations, predominantly missense mutations, in genes, such as TP53, TTN, and CTNNB1, varied between the high-risk and low-risk groups (Figure 8B). Additionally, the two prognostic genes CDK1 and HOMER1 also exhibited mutations. The frequency of transition (Ti) mutations was higher than that of transversion (Tv) and missense mutations (Figure 8C). CNV analysis revealed that the most frequent copy number increase was observed in DCLRE1B, whereas the most frequent copy number decrease was observed in RAN (Figure 8D).
Figure 8. Differential drug sensitivity and mutation profiles between the high-risk and low-risk groups. (A) The half-maximal inhibitory concentration (IC50) values of the top 10 drugs in patients belonging to the high-risk and low-risk groups (Top 10). (B) Mutation profiles of the high-risk and low-risk groups (the top and bottom sections represent the low-risk and high-risk groups, respectively). (C) Analysis of characteristic gene mutations and Kaplan-Meier (K-M) curves based on tumor mutational burden (TMB) and risk score. (D) Copy number variation of characteristic genes (red and blue represent amplification and deletion, respectively). **** p< 0.0001.
The lncRNA-miRNA-mRNA regulatory network comprised 350 nodes and 838 edges. The nodes included five prognostic genes (RAN, HOMER1, IL1RN, DCLRE1B, and CDK1), 21 miRNAs (such as hsa-miR-181a-5p and hsa-miR-122-5p), and 324 lncRNAs. This network featured competing endogenous RNA (ceRNA) interactions, such as DCLRE1B-hsa-miR-101-3p-XIST and IL1RN-hsa-miR-122-5p-ASB16-AS1 interactions (Supplementary Figure 1A). The miRNA/TF-prognostic gene regulatory network graph comprised 224 nodes and 227 edges. The nodes included three prognostic genes (ADA, CDK1, and IL1RN), 15 TFs (such as SP1 and RB1), and 206 miRNAs. Examples of the miRNA/TF-gene interactions were ADA-SP1 and CDK1-hsa-miR-374c-5p interactions (Supplementary Figure 1B).
qRT-PCR analysis indicated that the mRNA expression levels of HOMER1, ADA, and CDK1 in HCC samples were significantly higher than those in non-cancerous samples. However, the mRNA expression levels of IL1RN and DCLRE1B were not significantly different between HCC and non-cancerous samples (Figure 9). The expression patterns of HOMER1, ADA, and CDK1 determined using qRT-PCR analysis were consistent with those determined using bioinformatic analysis.
Figure 9. Validation of prognostic genes. The mRNA expression levels of five prognostic genes in cancer and para-cancerous tissues. * p< 0.05.
HCC is the predominant form of primary liver cancer, accounting for more than 90% of primary liver cancer cases. Globally, HCC is the sixth most common cancer and the third leading cause of cancer-related deaths (38). The poor prognosis of HCC is because patients with early-stage HCC do not exhibit prominent symptoms. Thus, HCC is mostly diagnosed at an advanced stage, which is associated with resistance to conventional chemotherapy and radiotherapy. The survival rates of patients with HCC are low due to the development of treatment resistance and disease recurrence (39). The emergence of cancer immunotherapy, which stimulates the immune system, especially T cells, to target and kill tumor cells, has revolutionized HCC treatment, offering new hope to patients with HCC (40, 41). The proliferation of T cells, which aggregate and cluster in the tumor microenvironment, serves as a marker of tumor reactivity and is positively correlated with the outcomes of ICI therapy (42–44). However, T-cell proliferation is not an infallible measure of tumor reactivity and outcomes (45–50). Recently, Legut et al., who were the first to identify TPRGs (24), identified several TPRGs that enhance T-cell function. The understanding of the role of TCRs in HCC immunotherapy response can aid in guiding the selection of immunotherapy regimens for patients with HCC.
This study systematically investigated the Expression of TCRs and their relationship with OS in HCC. In total, 8,525 DEGs were identified between HCC and non-cancerous samples (5,853 upregulated genes and 2,672 downregulated genes) in TCGA-LIHC dataset. Based on the filtering criteria for TCRs, 18 DE-TCRs were selected. Next, a novel prognostic model comprising six prognostic genes (DCLRE1B, RAN, HOMER1, ADA, CDK1, and IL1RN) was established using LASSO regression analysis.
DCLRE1B, a 5’-3’ exonuclease belonging to the metallo-β-lactamase superfamily, is one of the evolutionarily conserved genes and is involved in DNA interstrand cross-linking (ICL) repair (51). DNA ICL induces tumor cell death (52). Lee et al. reported that the Oldenlandia diffusa extract promotes cell death in cisplatin-resistant ovarian cancer cells by regulating DCLRE1B. Additionally, the upregulated Expression of DCLRE1B in the liver, kidney, and pancreatic cancers is associated with poor prognosis (53). Li et al. reported that DCLRE1B expression was significantly correlated with immune cells in various cancers. The authors reported that DCLRE1B expression was associated with immune checkpoint gene expression and immune therapy sensitivity (54). In vitro experiments demonstrated that DCLRE1B facilitates the proliferation and migration of pancreatic cancer cells. The upregulated Expression of DCLRE1B in human cancers promotes cancer initiation and progression by modulating processes, such as immune cell infiltration (54). Recent studies have confirmed the role of DCLRE1B in immune regulation and tumor immunotherapy response (53). In this study, DCLRE1B was upregulated in HCC and regulated HCC occurrence and progression by modulating T cells, which was consistent with the findings of recent studies.
HOMER1, a member of the Homer family of dendritic proteins, is a scaffold protein that regulates glutamatergic synapses and spine morphogenesis (55). In this study, HOMER1 was upregulated in HCC and was negatively correlated with OS. This finding was consistent with that of Yang et al. (23), who demonstrated that HOMER1 is a T-cell proliferation-related regulatory gene in LUAD. Experimental validation in LUAD cells revealed that HOMER1 can inhibit tumor cell proliferation, migration, and invasion (23).
RAN, a small GTP-binding protein of the RAS superfamily, is crucial for protein transport through the nuclear pore complex (56). Elsalahaty et al. revealed that the RAN*rs14035 variant may be an independent risk factor for HCC and that RAN is involved in miRNA synthesis and promoting the development of various cancers, including HCC (57). In this study, RAN was upregulated in HCC and regulated tumor development and progression by influencing T lymphocyte responses in the tumor microenvironment. CDK1, a serine/threonine protein kinase, plays a crucial role in the G1/S and G2/M phase transitions of the cell cycle (58, 59). The upregulated Expression of CDK1 in HCC is associated with poor prognosis. The downregulation or the inhibition of CDK1 overexpression can improve survival outcomes (60–62). Chen et al. reported that IRF-1 can regulate the transcription of CDK1, playing a crucial role in both pathological and physiological phenomena, such as viral infection, carcinogenesis, pro-inflammatory damage, and immune system development (63). In this study, CDK1 was upregulated in HCC, serving as a risk gene. CDK1 was upregulated in patients with high-risk scores and was significantly associated with poor prognosis.
ADA, which is critical for purine nucleoside and DNA metabolism, plays key roles in the immune and vascular systems (64). The activity of ADA is reported to be upregulated in patients with cancer. The upregulated ADA activity is associated with the staging of gastric, bladder, breast, colorectal, and renal cancers (65–73). In liver cancer, elevated ADA is correlated with serum atezolizumab concentrations and impaired functions of CD8-positive T cells, including suppressed secretion of interferon-γ and tumor necrosis factor-α. This suggests that high ADA levels may decrease atezolizumab exposure, potentially reducing its anti-cancer efficacy (74). Immune cell death and DNA damage response play significant roles in cancer progression and prognosis (75). Zhang et al. identified ADA as a prognostic gene associated with immune cell death and DNA damage response and that ADA is upregulated in HCC and contributes to the anti-tumor immune response in liver cancer (76). IL1RN, which serves as a natural interleukin-1 receptor antagonist (77), is negatively correlated with the proliferation of bladder cancer cells (78). Zhang et al. demonstrated that the upregulation of IL1RN-201/203 and anakinra treatment in KRAS-mutant intrahepatic cholangiocarcinoma mice significantly enhanced the anti-tumor immune response by altering neutrophil recruitment and phenotype. The upregulation of IL1RN-201/203 levels was associated with a favorable response to anti-PD-1 immunotherapy (79). Additionally, one study examining genes related to metabolic risk factors in non-alcoholic fatty liver disease and HCC reported that IL1RN is a protective prognostic gene for HCC. However, the biological function of IL1RN in HCC has not been elucidated (80, 81). In this study, IL1RN was downregulated in HCC, indicating its role as a protective gene. The Expression of IL1RN was downregulated in high-risk patients. These results are consistent with those of previous studies.
These findings indicated that TCRs play crucial roles in various processes, including T-cell proliferation and tumor immunotherapy response, highlighting the validity of the prognosis model developed in this study. To further validate the bioinformatics analysis results, the mRNA levels of five genes were examined in HCC tissues and adjacent tissues. The expression trends of HOMER1, ADA, and CDK1 were consistent between qRT-PCR and bioinformatics analyses. However, the IL1RN and DCLRE1B mRNA levels were not significantly different between HCC and non-cancerous samples, which can be attributed to sample heterogeneity. Thus, the experimental results were consistent with the bioinformatics analysis results, indicating the reliability of the model.
GSVA revealed that the DNA replication and repair pathways were significantly enriched in high-risk patients, suggesting increased genomic alterations. Genomic mutation analysis revealed an increased frequency of mutations, including TP53, TTN, and CTNNB1 mutations, in high-risk cases, indicating increased genomic instability. Somatic mutations in TP53, which are the most common alterations in human cancers, are associated with poor prognosis (82, 83). The increased frequency of these mutations in the high-risk group suggests decreased survival duration. Previous studies have suggested that TP53 mutations affect the progression and prognosis of HCC and are associated with the immune microenvironment of HCC (84, 85). Therefore, CTNNB1 mutations can serve as biomarkers for evaluating the effectiveness of immunotherapy in HCC (86–88). Huo et al. demonstrated that CTNNB1 mutations are associated with poor prognosis and decreased disease-free survival (89). Consistently, this study demonstrated that the rate of CTNNB1 mutations was upregulated in the high-risk group and that the increased rate of CTNNB1 mutations was associated with decreased OS. Additionally, one study reported that TP53 and TTN exhibited the highest mutation rates and that these mutations served as cancer-driving factors in hepatitis B virus-related HCC (90). These findings suggest that patients in the high-risk group are sensitive to DNA-damaging agent-based therapies due to enhanced genomic instability.
The tumor microenvironment exerts regulatory effects on tumor phenotypes. Immune cell infiltration, which is crucial for the immune evasion of tumor cells and the induction of inflammation, is a key feature of the tumor environment (91). This study examined the differential immune cell infiltration levels between the high-risk and low-risk groups. The risk scores were positively correlated with the presence of B cells, CD4+ T cells, CD8+ T cells, macrophages, neutrophils, and dendritic cells. Previous studies have demonstrated that CD4+ and CD8+ T cells suppress HCC development through the induction of anti-tumor immune responses (92). The proportion and quantity of CD4+ T cells are reported to be significantly upregulated in the peritumoral area of HCC tissues, promoting HCC progression (93). The presence of CD8+ T cells is associated with prolonged OS (94). Recent studies have reported a close correlation between intra-tumoral dendritic cell infiltration and poor prognosis in patients with HCC (95), which is consistent with the findings of this study. The infiltration of neutrophils in HCC is linked to adverse clinical outcomes (96). Neutrophils, which are involved in the activation, regulation, and effector functions of immune cells (97), accelerate HCC progression by secreting various cytokines (100). Increased macrophage infiltration is associated with poor prognosis in HCC (98). Macrophage infiltration in the tumor microenvironment promotes tumor growth, angiogenesis, invasion, and metastasis (99). Targeting macrophages is a promising adjuvant immunotherapy approach for patients with HCC (100, 101). The enrichment of immune-related pathways varied between the high-risk and low-risk groups. The TIDE score in the low-risk group was lower than that in the high-risk group. This suggests that patients in the low-risk group can potentially benefit from immunotherapy. In high-risk patients with HCC, high levels of immune cell infiltration may not indicate that the immune system can effectively control or clear tumor cells. Immune cell functions are suppressed in the immunosuppressive microenvironment or the cancer cells evade immune system recognition and attack through multiple mechanisms, impairing the anti-tumor functions of immune cells. Previous studies have demonstrated that myeloid-derived suppressor cells exert immunosuppressive effects in HCC by expanding immune checkpoint signaling and suppressing the cytotoxic activity of natural killer cells. Additionally, cancer cells evade T-cell recognition due to the lack of a transporter protein or β2 macroglobulin associated with major histocompatibility complex class 1 antigen presentation (102, 103). Thus, this study contributed to the elucidation of unique mechanisms of the HCC immune microenvironment, which may aid in developing novel therapeutic strategies, including immunotherapeutic strategies, for HCC (104). The results of this study are consistent with those of previous studies on immune infiltration in HCC. This study offers valuable direction and guidance for understanding the mechanisms of immune cells in HCC. However, further studies are needed to elucidate specific mechanisms.
Increasing the sensitivity of HCC to various drugs can benefit patients, although further studies are needed to elucidate the specific underlying mechanisms (105). Analysis of drug sensitivity and risk scores revealed that the IC50 values of 5-fluorouracil, sorafenib, and VX-11e were low in the high-risk group, indicating their enhanced efficacy in this group. Conversely, the IC50 values of gefitinib, ceritinib, and sunitinib were high in the low-risk group, suggesting their decreased efficacy in this group (106). Thus, the prognostic model established in this study may facilitate the development of improved treatment strategies for HCC.
Six prognostic genes were used to construct a model to quantitatively assess the prognosis of patients with HCC. Patients with high-risk scores exhibited significantly decreased OS. The AUC values for predicting 1-year, 2-year, and 3-year OS were 0.75, 0.68, and 0.66, respectively. Univariate and multivariate analyses revealed that the risk scores and T-stage were independent prognostic factors. This indicated the relevance of the prognostic model for patients with late-stage HCC. Gene testing data and clinical characteristics should be integrated in the future for a comprehensive assessment. In this study, a nomogram combining risk scores and clinical factors was developed to predict the 1-year, 2-year, and 3-year OS of patients with HCC. The AUC values for predicting 1-year, 2-year, and 3-year were more than 0.7. Calibration curves revealed that the predicted survival rates concurred with the actual survival rates. The ROC curves demonstrated excellent predictive performance of the nomogram. Thus, the model developed in this study can quantitatively predict the prognosis of patients with HCC and enable the customization of treatment plans. This scoring system will aid healthcare professionals in predicting survival and selecting optimal treatment options.
This study has several limitations. The sample size was small in this study. Additionally, the data were obtained from public datasets. Furthermore, the accuracy and stability of the prognostic model were not validated through clinical studies. Finally, only the transcript levels were evaluated in this study, which may not reflect protein levels. Future studies must focus on overcoming these limitations.
In conclusion, this study established a novel prognostic model based on TCRs to predict the clinical outcomes of patients with HCC. Six TCR genes were correlated with HCC prognosis. The findings of this study may enable the development of novel diagnostic and therapeutic strategies for HCC.
The raw data supporting the conclusions of this article will be made available by the authors, without undue reservation.
LH: Data curation, Formal analysis, Methodology, Resources, Writing – original draft. XB: Data curation, Formal analysis, Methodology, Resources, Software, Writing – original draft. XL: Data curation, Formal analysis, Methodology, Resources, Software, Writing – review & editing. SL: Data curation, Formal analysis, Methodology, Resources, Software, Writing – review & editing. ZM: Formal analysis, Methodology, Resources, Software, Visualization, Writing – review & editing. LM: Formal analysis, Methodology, Resources, Software, Visualization, Writing – review & editing. XD: Conceptualization, Funding acquisition, Investigation, Project administration, Supervision, Validation, Writing – review & editing.
The author(s) declare financial support was received for the research, authorship, and/or publication of this article. This study was supported by financially supported by Ningxia Natural Science Foundation (Grant No.: 2023AAC03584), Ningxia Natural Science Foundation Key Project (Grant No.: 2022AAC02065) and Ningxia Key Research and Development Program (Grant No.: 2022BEG02039).
We acknowledge Dr. Xuming Mao from the University of Pennsylvania for valuable suggestions and revision of the manuscript. The language used in this study has been professionally edited by ExEditing.com.
ZM is employed by Weiluo Microbial Pathogens Monitoring Technology Co., Ltd. of Beijing.
The remaining authors declare that the research was conducted in the absence of any commercial or financial relationships that could be construed as a potential conflict of interest.
All claims expressed in this article are solely those of the authors and do not necessarily represent those of their affiliated organizations, or those of the publisher, the editors and the reviewers. Any product that may be evaluated in this article, or claim that may be made by its manufacturer, is not guaranteed or endorsed by the publisher.
The Supplementary Material for this article can be found online at: https://www.frontiersin.org/articles/10.3389/fimmu.2024.1444091/full#supplementary-material
Supplementary Figure 1 | Construction of competing endogenous RNA (ceRNA) and microRNA (miRNA)/transcription factor (TF)-gene networks. (A) ceRNA network of characteristic genes (red squares, blue diamonds, and yellow circles indicate characteristic genes, miRNAs, and long non-coding RNAs (lncRNAs) (degree ≥ 8), respectively). (B) miRNA/TF-gene network (red squares, purple diamonds, and yellow circles indicate characteristic genes, miRNAs (degree > 1), and TFs, respectively).
1. Oura K, Morishita A, Hamaya S, Fujita K, Masaki T. The roles of epigenetic regulation and the tumor microenvironment in the mechanism of resistance to systemic therapy in hepatocellular carcinoma. Int J Mol Sci. (2023) 24(3):2805. doi: 10.3390/ijms24032805
2. Konyn P, Ahmed A, Kim D. Current epidemiology in hepatocellular carcinoma. Expert Rev Gastroenterol Hepatol. (2021) 15:1295–307. doi: 10.1080/17474124.2021.1991792
3. Zhang CH, Cheng Y, Zhang S, Fan J, Gao Q. Changing epidemiology of hepatocellular carcinoma in Asia. Liver International: Off J Int Assoc Study Liver. (2022) 42:2029–41. doi: 10.1111/liv.15251
4. Singal AG, Llovet JM, Yarchoan M, Mehta N, Heimbach JK, Dawson LA, et al. AASLD Practice Guidance on prevention, diagnosis, and treatment of hepatocellular carcinoma. Hepatol (Baltimore Md). (2023) 78:1922–65. doi: 10.1097/HEP.0000000000000466
5. Li J, Zhu Y. Recent advances in liver cancer stem cells: non-coding RNAs, oncogenes and oncoproteins. Front Cell Dev Biol. (2020) 8:548335. doi: 10.3389/fcell.2020.548335
6. Feng D, Wang M, Hu J, Li S, Zhao S, Li H, et al. Prognostic value of the albumin-bilirubin grade in patients with hepatocellular carcinoma and other liver diseases. Ann Trans Med. (2020) 8:553. doi: 10.21037/atm.2020.02.116
7. Thompson JA, Schneider BJ, Brahmer J, Achufusi A, Armand P, Berkenstock MK, et al. Management of immunotherapy-related toxicities, version 1.2022, NCCN clinical practice guidelines in oncology. J Natl Compr Cancer Network: JNCCN. (2022) 20:387–405. doi: 10.6004/jnccn.2022.0020
8. Clinical Practice Guidelines EASL. Management of hepatocellular carcinoma. J Hepatol. (2018) 69:182–236. doi: 10.1016/j.jhep.2018.03.019
9. Liu Z, Liu X, Liang J, Liu Y, Hou X, Zhang M, et al. Immunotherapy for hepatocellular carcinoma: current status and future prospects. Front Immunol. (2021) 12:765101. doi: 10.3389/fimmu.2021.765101
10. Zhu AX, Finn RS, Edeline J, Cattan S, Ogasawara S, Palmer D, et al. Pembrolizumab in patients with advanced hepatocellular carcinoma previously treated with sorafenib (KEYNOTE-224): a non-randomised, open-label phase 2 trial. Lancet Oncol. (2018) 19:940–52. doi: 10.1016/S1470-2045(18)30351-6
11. Cheng AL, Hsu C, Chan SL, Choo SP, Kudo M. Challenges of combination therapy with immune checkpoint inhibitors for hepatocellular carcinoma. J Hepatol. (2020) 72:307–19. doi: 10.1016/j.jhep.2019.09.025
12. Ostroumov D, Fekete-Drimusz N, Saborowski M, Kühnel F, Woller N. CD4 and CD8 T lymphocyte interplay in controlling tumor growth. Cell Mol Life sciences: CMLS. (2018) 75:689–713. doi: 10.1007/s00018-017-2686-7
13. Zhang Y, Zhang Z. The history and advances in cancer immunotherapy: understanding the characteristics of tumor-infiltrating immune cells and their therapeutic implications. Cell Mol Immunol. (2020) 17:807–21. doi: 10.1038/s41423-020-0488-6
14. Schoenfeld AJ, Hellmann MD. Acquired resistance to immune checkpoint inhibitors. Cancer Cell. (2020) 37:443–55. doi: 10.1016/j.ccell.2020.03.017
15. Bagchi S, Yuan R, Engleman EG. Immune checkpoint inhibitors for the treatment of cancer: clinical impact and mechanisms of response and resistance. Annu Rev Pathol. (2021) 16:223–49. doi: 10.1146/annurev-pathol-042020-042741
16. Whiteside SK, Grant FM, Alvisi G, Clarke J, Tang L, Imianowski CJ, et al. Acquisition of suppressive function by conventional T cells limits antitumor immunity upon T(reg) depletion. Sci Immunol. (2023) 8:eabo5558. doi: 10.1126/sciimmunol.abo5558
17. Hwang JR, Byeon Y, Kim D, Park SG. Recent insights of T cell receptor-mediated signaling pathways for T cell activation and development. Exp Mol Med. (2020) 52:750–61. doi: 10.1038/s12276-020-0435-8
18. Yang Y, Liu Q, Guo X, Yuan Q, Nian S, Kang P, et al. Systematic pan-cancer analysis identifies CDK1 as an immunological and prognostic biomarker. J Oncol. (2022) 2022:8115474. doi: 10.1155/2022/8115474
19. Hou J, Wen X, Lu Z, Wu G, Yang G, Tang C, et al. A novel T-cell proliferation-associated regulator signature pre-operatively predicted the prognostic of bladder cancer. Front Immunol. (2022) 13:970949. doi: 10.3389/fimmu.2022.970949
20. Patsoukis N, Sari D, Boussiotis VA. PD-1 inhibits T cell proliferation by upregulating p27 and p15 and suppressing Cdc25A. Cell Cycle (Georgetown Tex). (2012) 11:4305–9. doi: 10.4161/cc.22135
21. Llovet JM, Castet F, Heikenwalder M, Maini MK, Mazzaferro V, Pinato DJ, et al. Immunotherapies for hepatocellular carcinoma. Nat Rev Clin Oncol. (2022) 19:151–72. doi: 10.1038/s41571-021-00573-2
22. Krishna C, DiNatale RG, Kuo F, Srivastava RM, Vuong L, Chowell D, et al. Single-cell sequencing links multiregional immune landscapes and tissue-resident T cells in ccRCC to tumor topology and therapy efficacy. Cancer Cell. (2021) 39:662–77.e6. doi: 10.1016/j.ccell.2021.03.007
23. Yang Q, Zhu W, Gong H. Subtype classification based on t cell proliferation-related regulator genes and risk model for predicting outcomes of lung adenocarcinoma. Front Immunol. (2023) 14:1148483. doi: 10.3389/fimmu.2023.1148483
24. Legut M, Gajic Z, Guarino M, Daniloski Z, Rahman JA, Xue X, et al. A genome-scale screen for synthetic drivers of T cell proliferation. Nature. (2022) 603:728–35. doi: 10.1038/s41586-022-04494-7
25. Love MI, Huber W, Anders S. Moderated estimation of fold change and dispersion for RNA-seq data with DESeq2. Genome Biol. (2014) 15:550. doi: 10.1186/s13059-014-0550-8
26. Mao W, Ding J, Li Y, Huang R, Wang B. Inhibition of cell survival and invasion by Tanshinone IIA via FTH1: A key therapeutic target and biomarker in head and neck squamous cell carcinoma. Exp Ther Med. (2022) 24:521. doi: 10.3892/etm.2022.11449
27. Wu T, Hu E, Xu S, Chen M, Guo P, Dai Z, et al. clusterProfiler 4.0: A universal enrichment tool for interpreting omics data. Innovation (Cambridge (Mass)). (2021) 2:100141. doi: 10.1016/j.xinn.2021.100141
28. Friedman J, Hastie T, Tibshirani R. Regularization paths for generalized linear models via coordinate descent. J Stat Software. (2010) 33:1–22. doi: 10.18637/jss.v033.i01
29. Butcher SC, Essayagh B, Steyerberg EW, Benfari G, Antoine C, Grigioni F, et al. Factors influencing post-surgical survival in degenerative mitral regurgitation. Eur Heart J. (2023) 44:871–81. doi: 10.1093/eurheartj/ehad004
30. Liu TT, Li R, Huo C, Li JP, Yao J, Ji XL, et al. Identification of CDK2-related immune forecast model and ceRNA in lung adenocarcinoma, a pan-cancer analysis. Front Cell Dev Biol. (2021) 9:682002. doi: 10.3389/fcell.2021.682002
31. Sun Y, Zhang C, Fang Q, Zhang W, Liu W. Abnormal signal pathways and tumor heterogeneity in osteosarcoma. J Trans Med. (2023) 21:99. doi: 10.1186/s12967-023-03961-7
32. Ritchie ME, Phipson B, Wu D, Hu Y, Law CW, Shi W, et al. limma powers differential expression analyses for RNA-sequencing and microarray studies. Nucleic Acids Res. (2015) 43:e47. doi: 10.1093/nar/gkv007
33. Yu S, Chen M, Xu L, Mao E, Sun S. A senescence-based prognostic gene signature for colorectal cancer and identification of the role of SPP1-positive macrophages in tumor senescence. Front Immunol. (2023) 14:1175490. doi: 10.3389/fimmu.2023.1175490
34. Geeleher P, Cox N, Huang RS. pRRophetic: an R package for prediction of clinical chemotherapeutic response from tumor gene expression levels. PLoS One. (2014) 9:e107468. doi: 10.1371/journal.pone.0107468
35. Wilkerson MD, Hayes DN. ConsensusClusterPlus: a class discovery tool with confidence assessments and item tracking. Bioinf (Oxford England). (2010) 26:1572–3. doi: 10.1093/bioinformatics/btq170
36. Shi Y, Wang Y, Dong H, Niu K, Zhang W, Feng K, et al. Crosstalk of ferroptosis regulators and tumor immunity in pancreatic adenocarcinoma: novel perspective to mRNA vaccines and personalized immunotherapy. Apoptosis. (2023) 28:1423–35. doi: 10.1007/s10495-023-01868-8
37. Mayakonda A, Lin DC, Assenov Y, Plass C, Koeffler HP. Maftools: efficient and comprehensive analysis of somatic variants in cancer. Genome Res. (2018) 28:1747–56. doi: 10.1101/gr.239244.118
38. Chakraborty E, Sarkar D. Emerging therapies for hepatocellular carcinoma (HCC). Cancers. (2022) 14(11):2798. doi: 10.3390/cancers14112798
39. Luo XY, Wu KM, He XX. Advances in drug development for hepatocellular carcinoma: clinical trials and potential therapeutic targets. J Exp Clin Cancer research: CR. (2021) 40:172. doi: 10.1186/s13046-021-01968-w
40. Sangro B, Sarobe P, Hervás-Stubbs S, Melero I. Advances in immunotherapy for hepatocellular carcinoma. Nat Rev Gastroenterol Hepatol. (2021) 18:525–43. doi: 10.1038/s41575-021-00438-0
41. Celsa C, Giuffrida P, Stornello C, Grova M, Spatola F, Rizzo GEM, et al. Systemic therapies for hepatocellular carcinoma: the present and the future. Recenti Progressi Medicina. (2021) 112:110–6. doi: 10.1701/3559.35371
42. Tumeh PC, Harview CL, Yearley JH, Shintaku IP, Taylor EJ, Robert L, et al. PD-1 blockade induces responses by inhibiting adaptive immune resistance. Nature. (2014) 515:568–71. doi: 10.1038/nature13954
43. Scheper W, Kelderman S, Fanchi LF, Linnemann C, Bendle G, de Rooij MAJ, et al. Low and variable tumor reactivity of the intratumoral TCR repertoire in human cancers. Nat Med. (2019) 25:89–94. doi: 10.1038/s41591-018-0266-5
44. Pasetto A, Gros A, Robbins PF, Deniger DC, Prickett TD, Matus-Nicodemos R, et al. Tumor- and neoantigen-reactive T-cell receptors can be identified based on their frequency in fresh tumor. Cancer Immunol Res. (2016) 4:734–43. doi: 10.1158/2326-6066.CIR-16-0001
45. Li H, van der Leun AM, Yofe I, Lubling Y, Gelbard-Solodkin D, van Akkooi ACJ, et al. Dysfunctional CD8 T cells form a proliferative, dynamically regulated compartment within human melanoma. Cell. (2019) 176:775–89.e18. doi: 10.1016/j.cell.2018.11.043
46. Guo X, Zhang Y, Zheng L, Zheng C, Song J, Zhang Q, et al. Global characterization of T cells in non-small-cell lung cancer by single-cell sequencing. Nat Med. (2018) 24:978–85. doi: 10.1038/s41591-018-0045-3
47. Yost KE, Satpathy AT, Wells DK, Qi Y, Wang C, Kageyama R, et al. Clonal replacement of tumor-specific T cells following PD-1 blockade. Nat Med. (2019) 25:1251–9. doi: 10.1038/s41591-019-0522-3
48. Zhang L, Yu X, Zheng L, Zhang Y, Li Y, Fang Q, et al. Lineage tracking reveals dynamic relationships of T cells in colorectal cancer. Nature. (2018) 564:268–72. doi: 10.1038/s41586-018-0694-x
49. Savas P, Virassamy B, Ye C, Salim A, Mintoff CP, Caramia F, et al. Single-cell profiling of breast cancer T cells reveals a tissue-resident memory subset associated with improved prognosis. Nat Med. (2018) 24:986–93. doi: 10.1038/s41591-018-0078-7
50. van der Leun AM, Thommen DS, Schumacher TN. CD8(+) T cell states in human cancer: insights from single-cell analysis. Nat Rev Cancer. (2020) 20:218–32. doi: 10.1038/s41568-019-0235-4
51. Diene SM, Pinault L, Keshri V, Armstrong N, Khelaifia S, Chabrière E, et al. Human metallo-β-lactamase enzymes degrade penicillin. Sci Rep. (2019) 9:12173. doi: 10.1038/s41598-019-48723-y
52. Demuth I, Digweed M, Concannon P. Human SNM1B is required for normal cellular response to both DNA interstrand crosslink-inducing agents and ionizing radiation. Oncogene. (2004) 23:8611–8. doi: 10.1038/sj.onc.1207895
53. Lee YK, Lim J, Yoon SY, Joo JC, Park SJ, Park YJ. Promotion of cell death in cisplatin-resistant ovarian cancer cells through KDM1B-DCLRE1B modulation. Int J Mol Sci. (2019) 20(53):2443. doi: 10.3390/ijms20102443
54. Li L, Wang F, Deng Z, Zhang G, Zhu L, Zhao Z, et al. DCLRE1B promotes tumor progression and predicts immunotherapy response through METTL3-mediated m6A modification in pancreatic cancer. BMC Cancer. (2023) 23:1073. doi: 10.1186/s12885-023-11524-8
55. Yoon S, Piguel NH, Khalatyan N, Dionisio LE, Savas JN, Penzes P. Homer1 promotes dendritic spine growth through ankyrin-G and its loss reshapes the synaptic proteome. Mol Psychiatry. (2021) 26:1775–89. doi: 10.1038/s41380-020-00991-1
56. Krol J, Loedige I, Filipowicz W. The widespread regulation of microRNA biogenesis, function and decay. Nat Rev Genet. (2010) 11:597–610. doi: 10.1038/nrg2843
57. Elsalahaty MI, Salama AF, Diab T, Ghazy M, Toraih E, Elshazli RM. Unleash multifunctional role of miRNA biogenesis gene variants (XPO5*rs34324334 and RAN*rs14035) with susceptibility to hepatocellular carcinoma. J Pers Med. (2023) 13(6):959. doi: 10.3390/jpm13060959
58. Tandon R, Cunningham LL, White DK, Herford AS, Cicciu M. Overexpression of cyclin A in oral dysplasia: An international comparison and literature review. Indian J Cancer. (2014) 51:502–5. doi: 10.4103/0019-509X.175324
59. Ohashi R, Gao C, Miyazaki M, Hamazaki K, Tsuji T, Inoue Y, et al. Enhanced expression of cyclin E and cyclin A in human hepatocellular carcinomas. Anticancer Res. (2001) 21:657–62. doi: 10.1071/ZO08011
60. Wu CX, Wang XQ, Chok SH, Man K, Tsang SHY, Chan ACY, et al. Blocking CDK1/PDK1/β-Catenin signaling by CDK1 inhibitor RO3306 increased the efficacy of sorafenib treatment by targeting cancer stem cells in a preclinical model of hepatocellular carcinoma. Theranostics. (2018) 8:3737–50. doi: 10.7150/thno.25487
61. Zhou J, Han S, Qian W, Gu Y, Li X, Yang K. Metformin induces miR-378 to downregulate the CDK1, leading to suppression of cell proliferation in hepatocellular carcinoma. OncoTargets Ther. (2018) 11:4451–9. doi: 10.2147/OTT.S167614
62. Liu HM, Tan HY, Lin Y, Xu BN, Zhao WH, Xie YA. MicroRNA-1271-5p inhibits cell proliferation and enhances radiosensitivity by targeting CDK1 in hepatocellular carcinoma. J Biochem. (2020) 167:513–24. doi: 10.1093/jb/mvz114
63. Chen S, Shen B, Wu Y, Shen L, Qi H, Cao F, et al. Identification of prognosis-related cyclin-dependent kinases and potential response drugs in hepatocellular carcinoma. J Cancer Res Ther. (2023) 19:108–16. doi: 10.4103/jcrt.jcrt_1703_22
64. Zhu Z, Zhou J, Xiong X, Liu D, Zheng Y, Ding X, et al. Prognostic value of serum liver enzymes in oral and oropharynx squamous cell carcinomas. J Oral Pathol medicine: Off Publ Int Assoc Oral Pathologists Am Acad Oral Pathol. (2019) 48:36–42. doi: 10.1111/jop.2019.48.issue-1
65. Vannoni D, Bernini A, Carlucci F, Civitelli S, Di Pietro MC, Leoncini R, et al. Enzyme activities controlling adenosine levels in normal and neoplastic tissues. Med Oncol (Northwood London England). (2004) 21:187–95. doi: 10.1385/MO:21:2:187
66. Durak I, Cetin R, Canbolat O, Cetin D, Yurtarslani Z, Unal A. Adenosine deaminase, 5’-nucleotidase, guanase and cytidine deaminase activities in gastric tissues from patients with gastric cancer. Cancer Lett. (1994) 84:199–202. doi: 10.1016/0304-3835(94)90376-X
67. Durak I, Ormeci N, Akyol O, Canbolat O, Kavutçu M, Bülbül M. Adenosine deaminase, 5’-nucleotidase, xanthine oxidase, superoxide dismutase, and catalase activities in gastric juices from patients with gastric cancer, ulcer, and atrophic gastritis. Digestive Dis Sci. (1994) 39:721–8. doi: 10.1007/BF02087413
68. Pirinççi N, Geçit I, Güneş M, Yüksel MB, Kaba M, Tanık S, et al. Serum adenosine deaminase, catalase and carbonic anhydrase activities in patients with bladder cancer. Clinics (Sao Paulo Brazil). (2012) 67:1443–6. doi: 10.6061/clinics/2012(12)15
69. Durak I, Perk H, Kavutçu M, Canbolat O, Akyol O, Bedük Y. Adenosine deaminase, 5’nucleotidase, xanthine oxidase, superoxide dismutase, and catalase activities in cancerous and noncancerous human bladder tissues. Free Radic Biol Med. (1994) 16:825–31. doi: 10.1016/0891-5849(94)90199-6
70. FAİSAL A, Taha M. Serum adenosine deaminase activity in Iraqi patients with breast cancer on tamoxifen therapy. Gaziantep Tip Dergisi. (2012) 18:139-142. doi: 10.5455/GMJ-30-2012-96
71. Aghaei M, Karami-Tehrani F, Salami S, Atri M. Diagnostic value of adenosine deaminase activity in benign and Malignant breast tumors. Arch Med Res. (2010) 41:14–8. doi: 10.1016/j.arcmed.2009.10.012
72. Pirinççi N, Kaya TY, Kaba M, Ozan T, Geçit İ, Özveren H, et al. Serum adenosine deaminase, catalase, and carbonic anhydrase activities in patients with renal cell carcinoma. Redox report: Commun Free Radical Res. (2017) 22:252–6. doi: 10.1080/13510002.2016.1207364
73. Kutryb-Zajac B, Harasim G, Jedrzejewska A, Krol O, Braczko A, Jablonska P, et al. Macrophage-derived adenosine deaminase 2 correlates with M2 macrophage phenotype in triple negative breast cancer. Int J Mol Sci. (2021) 22(7):3764. doi: 10.3390/ijms22073764
74. Kim C, Yang H, Kim I, Kang B, Kim H, Kim H, et al. Association of high levels of antidrug antibodies against atezolizumab with clinical outcomes and T-cell responses in patients with hepatocellular carcinoma. JAMA Oncol. (2022) 8:1825–9. doi: 10.1001/jamaoncol.2022.4733
75. Krysko DV, Garg AD, Kaczmarek A, Krysko O, Agostinis P, Vandenabeele P. Immunogenic cell death and DAMPs in cancer therapy. Nat Rev Cancer. (2012) 12:860–75. doi: 10.1038/nrc3380
76. Zhang X, Wen J, Zhang G, Fan W, Tan J, Liu H, et al. Identification and validation of novel immunogenic cell death- and DNA damage response-related molecular patterns correlated with immune status and prognosis in hepatocellular carcinoma. Trans Oncol. (2023) 27:101600. doi: 10.1016/j.tranon.2022.101600
77. Pan JH, Zhou H, Cooper L, Huang JL, Zhu SB, Zhao XX, et al. LAYN is a prognostic biomarker and correlated with immune infiltrates in gastric and colon cancers. Front Immunol. (2019) 10:6. doi: 10.3389/fimmu.2019.00006
78. Schneider L, Liu J, Zhang C, Azoitei A, Meessen S, Zheng X, et al. The role of interleukin-1-receptor-antagonist in bladder cancer cell migration and invasion. Int J Mol Sci. (2021) 22(11):5875. doi: 10.3390/ijms22115875
79. Zhang M, Huang Y, Pan J, Sang C, Lin Y, Dong L, et al. An inflammatory checkpoint generated by IL1RN splicing offers therapeutic opportunity for KRAS-mutant intrahepatic cholangiocarcinoma. Cancer Discov. (2023) 13:2248–69. doi: 10.1158/2159-8290.CD-23-0282
80. Salnikova O, Breuhahn K, Hartmann N, Schmidt J, Ryschich E. Endothelial plasticity governs the site-specific leukocyte recruitment in hepatocellular cancer. Int J Cancer. (2013) 133:2372–82. doi: 10.1002/ijc.v133.10
81. Shen B, Zhang G, Liu Y, Wang J, Jiang J. Identification and analysis of immune-related gene signature in hepatocellular carcinoma. Genes. (2022) 13(10):1834. doi: 10.3390/genes13101834
82. Wang L, Yan K, Zhou J, Zhang N, Wang L. Relationship of liver cancer with LRP1B or TP53 mutation and tumor mutation burden and survival. J Clin Oncol. (2019) 37:1573. doi: 10.1200/JCO.2019.37.15_suppl.1573
83. Wang W, Lu Z, Wang M, Liu Z, Wu B, Yang C, et al. The cuproptosis-related signature associated with the tumor environment and prognosis of patients with glioma. Front Immunol. (2022) 13:998236. doi: 10.3389/fimmu.2022.998236
84. Woo HG, Wang XW, Budhu A, Kim YH, Kwon SM, Tang ZY, et al. Association of TP53 mutations with stem cell-like gene expression and survival of patients with hepatocellular carcinoma. Gastroenterology. (2011) 140:1063–70. doi: 10.1053/j.gastro.2010.11.034
85. Zhao Y, Zhu C, Chang Q, Yang J, Liu Y, Peng P, et al. TP53 rs28934571 polymorphism increases the prognostic risk in hepatocellular carcinoma. Biomarkers Med. (2021) 15:615–22. doi: 10.2217/bmm-2020-0418
86. Xiao X, Mo H, Tu K. CTNNB1 mutation suppresses infiltration of immune cells in hepatocellular carcinoma through miRNA-mediated regulation of chemokine expression. Int Immunopharmacol. (2020) 89:107043. doi: 10.1016/j.intimp.2020.107043
87. Chen L, Zhou Q, Liu J, Zhang W. CTNNB1 alternation is a potential biomarker for immunotherapy prognosis in patients with hepatocellular carcinoma. Front Immunol. (2021) 12:759565. doi: 10.3389/fimmu.2021.759565
88. Deldar Abad Paskeh M, Mirzaei S, Ashrafizadeh M, Zarrabi A, Sethi G. Wnt/β-catenin signaling as a driver of hepatocellular carcinoma progression: an emphasis on molecular pathways. J Hepatocellular Carcinoma. (2021) 8:1415–44. doi: 10.2147/JHC.S336858
89. Huo J, Wu L, Zang Y. Development and validation of a CTNNB1-associated metabolic prognostic model for hepatocellular carcinoma. J Cell Mol Med. (2021) 25:1151–65. doi: 10.1111/jcmm.16181
90. Kong F, Kong D, Yang X, Yuan D, Zhang N, Hua X, et al. Integrative analysis of highly mutated genes in hepatitis B virus-related hepatic carcinoma. Cancer Med. (2020) 9:2462–79. doi: 10.1002/cam4.2903
91. Tower H, Ruppert M, Britt K. The immune microenvironment of breast cancer progression. Cancers. (2019) 11(9):1375. doi: 10.3390/cancers11091375
92. Mossanen JC, Tacke F. Role of lymphocytes in liver cancer. Oncoimmunology. (2013) 2:e26468. doi: 10.4161/onci.26468
93. Yang XH, Yamagiwa S, Ichida T, Matsuda Y, Sugahara S, Watanabe H, et al. Increase of CD4+ CD25+ regulatory T-cells in the liver of patients with hepatocellular carcinoma. J Hepatol. (2006) 45:254–62. doi: 10.1016/j.jhep.2006.01.036
94. Itoh S, Yoshizumi T, Yugawa K, Imai D, Yoshiya S, Takeishi K, et al. Impact of immune response on outcomes in hepatocellular carcinoma: association with vascular formation. Hepatol (Baltimore Md). (2020) 72:1987–99. doi: 10.1002/hep.31206
95. Zhou ZJ, Xin HY, Li J, Hu ZQ, Luo CB, Zhou SL. Intratumoral plasmacytoid dendritic cells as a poor prognostic factor for hepatocellular carcinoma following curative resection. Cancer Immunol Immunother. (2019) 68:1223–33. doi: 10.1007/s00262-019-02355-3
96. Kuang DM, Zhao Q, Wu Y, Peng C, Wang J, Xu Z, et al. Peritumoral neutrophils link inflammatory response to disease progression by fostering angiogenesis in hepatocellular carcinoma. J Hepatol. (2011) 54:948–55. doi: 10.1016/j.jhep.2010.08.041
97. Mantovani A, Cassatella MA, Costantini C, Jaillon S. Neutrophils in the activation and regulation of innate and adaptive immunity. Nat Rev Immunol. (2011) 11:519–31. doi: 10.1038/nri3024
98. Tian Z, Hou X, Liu W, Han Z, Wei L. Macrophages and hepatocellular carcinoma. Cell Biosci. (2019) 9:79. doi: 10.1186/s13578-019-0342-7
99. Wu SD, Ma YS, Fang Y, Liu LL, Fu D, Shen XZ. Role of the microenvironment in hepatocellular carcinoma development and progression. Cancer Treat Rev. (2012) 38:218–25. doi: 10.1016/j.ctrv.2011.06.010
100. Mantovani A, Marchesi F, Malesci A, Laghi L, Allavena P. Tumour-associated macrophages as treatment targets in oncology. Nat Rev Clin Oncol. (2017) 14:399–416. doi: 10.1038/nrclinonc.2016.217
101. Degroote H, Van Dierendonck A, Geerts A, Van Vlierberghe H, Devisscher L. Preclinical and clinical therapeutic strategies affecting tumor-associated macrophages in hepatocellular carcinoma. J Immunol Res. (2018) 2018:7819520. doi: 10.1155/2018/7819520
102. Oura K, Morishita A, Tani J, Masaki T. Tumor immune microenvironment and immunosuppressive therapy in hepatocellular carcinoma: A review. Int J Mol Sci. (2021) 22(11):5801. doi: 10.3390/ijms22115801
103. Li X, Yan X, Wang Y, Kaur B, Han H, Yu J. The Notch signaling pathway: a potential target for cancer immunotherapy. J Hematol Oncol. (2023) 16:45. doi: 10.1186/s13045-023-01439-z
104. Ruf B, Heinrich B, Greten TF. Immunobiology and immunotherapy of HCC: spotlight on innate and innate-like immune cells. Cell Mol Immunol. (2021) 18:112–27. doi: 10.1038/s41423-020-00572-w
105. Zhang X, Wu L, Xu Y, Yu H, Chen Y, Zhao H, et al. Microbiota-derived SSL6 enhances the sensitivity of hepatocellular carcinoma to sorafenib by down-regulating glycolysis. Cancer Lett. (2020) 481:32–44. doi: 10.1016/j.canlet.2020.03.027
Keywords: T-cell proliferation regulators, hepatocellular carcinoma, bioinformatic, GEO, prognostic model
Citation: Hai L, Bai X-Y, Luo X, Liu S-W, Ma Z-M, Ma L-N and Ding X-C (2024) Prognostic modeling of hepatocellular carcinoma based on T-cell proliferation regulators: a bioinformatics approach. Front. Immunol. 15:1444091. doi: 10.3389/fimmu.2024.1444091
Received: 05 June 2024; Accepted: 23 September 2024;
Published: 09 October 2024.
Edited by:
Wenlin Yang, University of Florida, United StatesCopyright © 2024 Hai, Bai, Luo, Liu, Ma, Ma and Ding. This is an open-access article distributed under the terms of the Creative Commons Attribution License (CC BY). The use, distribution or reproduction in other forums is permitted, provided the original author(s) and the copyright owner(s) are credited and that the original publication in this journal is cited, in accordance with accepted academic practice. No use, distribution or reproduction is permitted which does not comply with these terms.
*Correspondence: Xiang-Chun Ding, MTM2MTk1MTE3NjhAMTYzLmNvbQ==; Li-Na Ma, MTM2MTk1MTE3NThAMTYzLmNvbQ==
†These authors have contributed equally to this work
Disclaimer: All claims expressed in this article are solely those of the authors and do not necessarily represent those of their affiliated organizations, or those of the publisher, the editors and the reviewers. Any product that may be evaluated in this article or claim that may be made by its manufacturer is not guaranteed or endorsed by the publisher.
Research integrity at Frontiers
Learn more about the work of our research integrity team to safeguard the quality of each article we publish.