- 1Healthcare Research Institute Hospital 12 de Octubre (imas12), Hospital Universitario 12 de Octubre, Madrid, Spain
- 2Immunology Department, Hospital Universitario 12 de Octubre, Madrid, Spain
- 3Internal Medicine Department, Hospital Universitario 12 de Octubre, Madrid, Spain
- 4Faculty of Medicine, Universidad Complutense, Madrid, Spain
- 5Red de Infecciones en Inmunodeprimidos no VIH e infecciones relacionadas con la asistencia sanitaria (CIBERINFEC), Instituto de Salud Carlos III, Madrid, Spain
- 6Cell Biology Department, Faculty of Medicine, Universidad Complutense, Madrid, Spain
- 7Microbiology Department, Hospital Universitario 12 de Octubre, Madrid, Spain
- 8Biochemistry Department, Hospital Universitario 12 de Octubre, Madrid, Spain
- 9Red de Centros de Investigación Biomédica en Epidemiología y Salud Pública (CIBERESP), Instituto de Salud Carlos III, Madrid, Spain
Introduction: Influenza virus infection can cause a range of clinical symptoms, including respiratory failure (RF) and even death. The mechanisms responsible for the most severe forms of the disease are not yet well understood. The objective is to assess the initial immune response upon admission and its potential impact on infection progression.
Methods: We conducted a prospective observational study of patients with influenza virus infection who required admission to a tertiary hospital in the 2017/18 and 2018/19 flu seasons. Immune markers, surrogate markers of neutrophil activation, and blood levels of DNase I and Apolipoprotein-H (ApoH) were determined in the first serum sample available during hospital care. Patients were followed until hospital discharge or death. Initially, 792 patients were included. From this group, 107 patients with poor evolution were selected, and a random control group was matched by day of admission.
Results: Patients with poor outcomes had significantly reduced ApoH levels, a soluble protein that regulate both complement and coagulation pathways. In multivariate analysis, low plasma levels of ApoH (OR:5.43; 2.21-13.4), high levels of C- reactive protein (OR:2.73: 1.28-5.4), hyperferritinemia (OR:2.83; 1.28-5.4) and smoking (OR:3.41; 1.04-11.16), were significantly associated with a worse prognosis. RF was independently associated with low levels of ApoH (OR: 5.12; 2.02-1.94), while high levels of IL15 behaved as a protective factor (OR:0.30; 0.12-0.71).
Discussion: Therefore, in hospitalized influenza patients, a dysregulated early immune response is associated with a worse outcome. Adequate plasma levels of ApoH are protective against severe influenza and RF and High levels of IL15 protect against RF.
1 Introduction
Influenza is a contagious disease due to influenza viruses characterized by involvement of the respiratory tract that appears in seasonal epidemics and annually affect between 8 and 10% of the population (1, 2). Clinical manifestations present a wide spectrum of clinical profiles, from a self-limited mild infection to complications such as severe pneumonia, respiratory failure (RF), multiple organ failure or even death, reported in 20% of serious infections (3, 4). The mechanisms and factors associated with poor outcomes are not yet well understood.
Variables associated with complications have been identified that could serve as possible prognostic factors, including age, delay in the administration of antiviral treatment, pneumonia, thrombocytopenia, lymphopenia and PaO2/FiO2<200 mmHg (5–8).
As has already been reported in other infections, the evolution of the pathology based on the immune response established around the infection by influenza virus could be a determining factor in the evolution of patients (9–11). The secretion of cytokines by respiratory epithelial cells during infection is a determining factor in the disease's prognosis. An imbalance of cytokines can lead to ineffective and exaggerated adaptive immune responses (12–14), which could result in multi-organ failure and lung tissue damage (15).
Neutrophil extracellular traps (NETs) are a form of immediate response against any type of infection that consists of the release of DNA and molecules from the neutrophil cytoplasm, mainly antimicrobial peptides and proteases, which are organized in a network where pathogens are trapped and destroyed (16). Although NETs can prevent the spread of the infectious disease, their release and degradation by DNases after infection must be tightly regulated to effectively control the infection but avoiding excessive inflammatory reactions (17, 18). High levels of NETs causes tissue damage due to the activity of enzymes associated with NETs, such as mieloperoxidase (MPO) and elastases and correlates with poor prognosis of severe Influenza A infection (19). Dysregulation of the NETs formation process has been associated with the initiation and progression of autoimmune and autoinflammatory diseases (20). Various soluble proteins of the innate immune system such as C-reactive protein (CRP), ferritin, DNase-I and apolipoprotein H (ApoH) could play an important role, both systemically and locally, in the early stages of infection, to contain it, until a specific response is developed (21–23). During this early immune response, there is an increase in hepatic synthesis and in the plasmatic concentration of a group of proteins, which are involved in the inflammatory process as immune regulators (24, 25).
ApoH, also known as Beta 2 Glycoprotein 1 (β2GPI), is a soluble protein that regulates both complement and coagulation (26–28). ApoH also plays an important role as an opsonin: it can bind to microvesicles containing phosphatidylserine, such as microorganisms, viruses, vesicles or apoptotic bodies with proinflammatory activity (29). ApoH mediates virus and debris clearance (30) and is especially known to be the main antigenic target of antiphospholipid antibodies, present in patients with antiphospholipid syndrome (28).
The activity of the molecules involved in early immune responses must be strictly regulated to guarantee infection control and avoid triggering excessive inflammatory reactions that can lead to multiple organ failure or favour the appearance of numerous pathologies such as systemic lupus erythematosus (SLE) (17, 31, 32). In the present work we study the presence of the main plasma markers related to the innate immune response in the first moments of influenza infection and their relationship with patient's evolution.
2 Materials and methods
2.1 Study design
A prospective observational study enrolled adults (>18 years) with confirmed influenza at Hospital 12 de Octubre (Madrid, Spain) during 2017/18 and 2018/19 seasons (December to March in both periods). For inclusion, it was mandatory that a serum sample drawn within 24 hours of admission was available. Patients were followed up until hospital discharge or death. Demographic and clinical data were collected from electronic records. Collection of demographic and clinical laboratory data was obtained from the electronic medical record. Laboratory parameters included the absolute number of leukocytes, lymphocytes, platelets, C-reactive protein (CRP) and ferritin. Admission occurred with a median of 2 days after symptom manifestation.
2.2 Patients
From a total of 792 patients that were recruited, 107 had a poor evolution (see definition below) and the rest had an uneventful evolution until discharge.
Due to the impossibility of evaluating the early immune response in all patients, a two-cohort study was carried out. In this way, it was possible to study all the patients with poor evolution and compare them with a similar number of patients with good evolution.
In the first cohort, all patients with severe complications were incorporated. The second cohort was formed by a control group built with 107 patients with good evolution. For each patient with severe flu, a control was randomly selected from among the mild patients with sample availability who were admitted on the same day as the severe patient. In 8 of the 107 patients with poor evolution the serum sample was insufficient to be able to carry out an adequate immunological study, so they were discarded although their corresponding control remained in the study. Finally, 206 patients with influenza were part of the study, 99 with poor evolution and 107 with good evolution. See arrangement and algorithm in Figure 1.
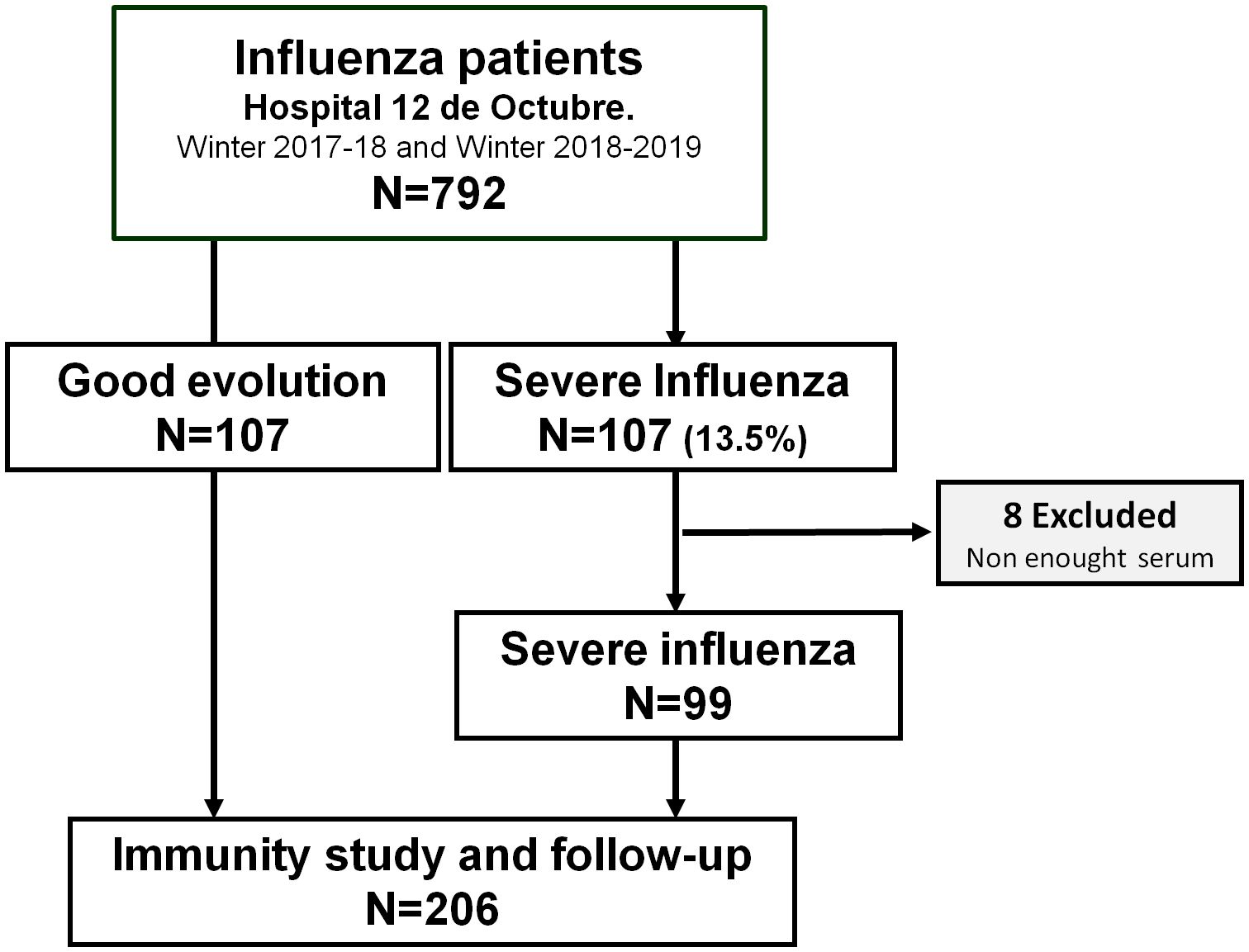
Figure 1 Algorithm of the distribution of patients. From the total group of 792 patients, 107 presented poor evolution. For each patient with severe influenza, a control was randomly selected from among the mild patients group.
In addition, a reference group made up of 41 anonymous blood donors was created to compare the profile of cytokines and other factors of innate immunity between healthy people and patients. This control group included healthy people who went to our hospital to donate blood. All of them tested negative for influenza virus and signed a questionnaire declaring that they did not suffer from any disease.
2.3 Study definitions
Severe Influenza (bad evolution) included RF, Intensive Care Unit (ICU) admission, or death during hospitalization. Good evolution was defined as the absence of any of these complications.
Respiratory failure (RF) was defined as PaO2/FiO2 ratio <200 mmHg or the need for mechanical ventilation, including those patients who had a clinical indication for ventilatory support but for any reason were finally not ventilated. If PaO2 was unavailable, estimated PaO2/FiO2 ratio was calculated using SpO2/FiO2 ratio (33).
The cut-off points used for the haematological abnormalities were as follows:
Leukocytosis (leukocytes >11,000/µL), leukopenia (leukocytes <4,000/µL), lymphopenia (lymphocytes <1,000/µL), severe lymphopenia (<500/µL), and thrombocytopenia (platelet <140,000/µL).
Obesity was defined as a Body Mass Index (BMI) >30.
2.4 Samples
Serum samples were collected and processed during the first 24 hours after admission to the emergency department. Admission occurred with a median of 2 days after the manifestation of clinical symptoms, without significant differences between patients with good or poor evolution (p=0.803).
2.5 Immune parameters
Cytokine determination assays Serum cytokine profile, including IFNα, IFNγ, IL-2, IL-6, IL-10, and IL-15, was determined by the Human Cytokine Magnetic Beads Panel Kit (EMD Millipore Corporation, St. Charles, MO, 63304, USA) using a LABScan ™ 100 Luminex. The results were analyzed with the Luminex xPONENT42 v3.1 software. In addition, serum IL-8 was assessed by Flex ELISA: Human IL-8 CXCL8 (Mabtech AB, Nacka Strand, Sweden). The experimental procedure was carried out following the manufacturer's recommendations and automated in a Triturus® Analyzer (Diagnostics Grifols SA, Barcelona, Spain).
Consideration of elevated or decreased immune-markers levels (cytokines, DNase, ApoH and MPO) for dichotomization and use as potential biomarkers was performed using the mean ± 2 standard deviation (STD) of each marker in a group of 41 healthy blood donors as the cutoff. The cutoff points for cytokines are described in Supplementary Table S1).
ApoH determination assay Serum β2GPI levels were quantified using ELISA Pro Human ApoH (Mabtech AB, following the manufacturer's instructions. The ELISA procedures were carried out in a Triturus® Analyzer (Diagnostics Grifols SA). Low levels of β2GPI were considered to be values ≤86 mg/l, corresponding to the mean levels previously described in healthy people (178 ± 46 mg/l) minus twice the STD (34).
2.6 DNase activity assay
Serum DNase activity was measured with a functional technique that assesses DNA hydrolysis in a semisolid medium. The plates were prepared with PBS Buffer (with Ca and Mg, pH 7.4) to which 10 mg/mL of agarose, 0.13 mg/mL of DNA and 0.2 mg/mL Syber Green (Invitrogen, Carlsbad, California, USA) were added. Small cylindrical holes (2 mm diameter) were created in which 10uL of the serum sample were placed and incubated for 16h at 37°C. The halos resulting from DNA hydrolysis were evaluated in a Syngene™ LED Blue Light transilluminator (Syngene, Bangalore, India). DNase levels were calculated by relating them to the activity of pure DNase (Manufacturer Invitrogen, Carlsbad, California, USA) diluted to known concentrations. Serum DNase activity levels ≤ 12 ng/ml were considered low.
2.7 MPO determination assay
Human myeloperoxidase (MPO) is the main biocidal protein in NETs (35). For this reason, we determined levels of MPO in serum as an indirect measurement of NETosis activity using Human MPO Quantikine ELISA kit (R&D Systems, Minneapolis, MN, USA). The procedure was performed following the manufacturer's instructions. Briefly, samples were diluted 50-fold in assay diluent and incubated for 2 hours at room temperature. Subsequently, the samples were then washed, the conjugate was added to the plate and incubated for 2 hours. After new washes, substrate solution was added and incubated for 30 minutes. Samples were read at 450 nm. Elevated levels of MPO in the blood were considered to be values > 1783 ng/mL (mean value + 2 STD observed in a sample of 41 blood donors).
2.8 Microbiological methods
For influenza molecular diagnosis, the Allplex™ respiratory Panel 1 (Seegene, Seoul, South Korea) was utilized. This panel enables the simultaneous detection of Flu A, Flu B, RSV A, RSV B and Flu A subtypes H1, H1pdm09 and H3. Nucleic acid extraction (200 μl assay volume) was carried out using the MicrolabStarlet IVD with the STARMag 96 x 4 Universal Cartridge Kit (Seegene, Seoul, South Korea). The rRT-PCR, was performed on the CFX96™ system (Bio-Rad Laboratories, Hercules, CA, USA). Analysis of the results was done using Seegene viewer software.
2.9 Ethics statement
This study was carried out in accordance with the principles of the Declaration of Helsinki and was approved by the Clinical Research Ethics Committee of Hospital Universitario 12 de Octubre (reference numbers 20/117, 18/182 and 18/009). The patients signed informed consent.
2.10 Statistical analysis
The results of the scaled variables were expressed as median with interquartile range (IQR) in brackets. For comparisons between two groups, the Wilcoxon-Mann-Whitney test was used. To compare the variability in the continuous variables between cohorts, the Hodges-Lehmann median difference and the relative difference of the Hodges Lehman medians (RMD) were used. The RMD was calculated by dividing the Hodges Lehman median difference by the highest value of the cohort medians. This index varies between 0 and 1, with the values closest to zero indicating very small (irrelevant) differences. When more than two groups were compared, the Kruskal–Wallis test was used.
The results of the qualitative variables were expressed in absolute frequency and percentage. The association between variables was determined using the Chi-square test or Fisher's exact test, when appropriate. The relative size of an effect is expressed as an odds ratio (OR). Multivariate analyzes were performed using a logistic regression model. Data were analyzed with MedCalc for Windows version 20.1 (MedCalc Software, Ostend, Belgium), Microsoft Excel (Microsoft Corp, Redmond, WA, USA), and GraphPad Prism version 9 (GraphPad Software,Boston, MA, USA). Probabilities less than 0.05 were considered significant.
3 Results
3.1 Characteristics of the patients and controls
Analysing the whole group of influenza patients, the median age was 75.5 (IQR: 62-85) years with a balanced distribution by gender (women 54.4%). The most prevalent comorbidities were ex-smoking habit (25.2%), Diabetes Mellitus (23.8%) and obesity (22.3%) (Supplementary Table S2). In 94% of cases, the infection was caused by influenza A, while influenza B accounted for the remaining 6%. Among cases of influenza A, the most common subtype was H3N2 (79%), with the remaining 21% caused by subtype H1N1pmd09.
Comparing all influenza patients with healthy population (Supplementary Table S3), influenza patients presented a lower concentration of alpha and gamma interferons (IFN α: 1.3 vs 7.0 pg/mL p<0.001; IFNγ: 0.5 vs 4.8 pg/mL, p=0.002) and a higher concentration of IL-10 (10.8 vs 0.9 pg/mL, p<0.001) and IL-15 (2.3 vs 0.2 pg/mL, p<0.001). MPO levels were higher in patients with influenza: 1154 (486-2379) vs 396 (266-708) ng/ml, p<0.001.
Influenza patients presented a decreased DNase activity compared to healthy controls: 8 (4-8) vs 108 (77-138) ng/mL, p<0.001 and a slightly lower ApoH concentration although the differences were not significant: 177 (103-281) vs 209 (168-256) ug/mL, p=0.079 (Supplementary Table S3).
3.2 Patients with influenza according to the severity of the disease
The comparison of the demographic data of patients with severe influenza with those who had a favourable evolution showed that patients with severe influenza were younger: 71 (57-83) vs 80 (68-86) years old (p=0.004) having also a higher smoking prevalence (16.2% vs 4.7%. p=0.007) and a lower obesity proportion, (15.2% vs 28.9%. p=0.017). Additionally, severe patients showed a higher number of leukocytes per µL: 9500 (6900-13100) vs 7700 (5825-10900; p=0.012), CRP: 13 (5.3-26.25) vs 7 (3-13) µg/mL (p<0.001), ferritin: 452 (186-959) vs 235 (149-428), ng/mL (p<0.001), MPO: 1571 (673-2884) vs. 1038 (473-1733) ng/ml (p=0.017) and IL-8: 22 (3.5-61.5) vs 3.5 (3.5-24.4), pg/mL, (p= 0.001) (Table 1).
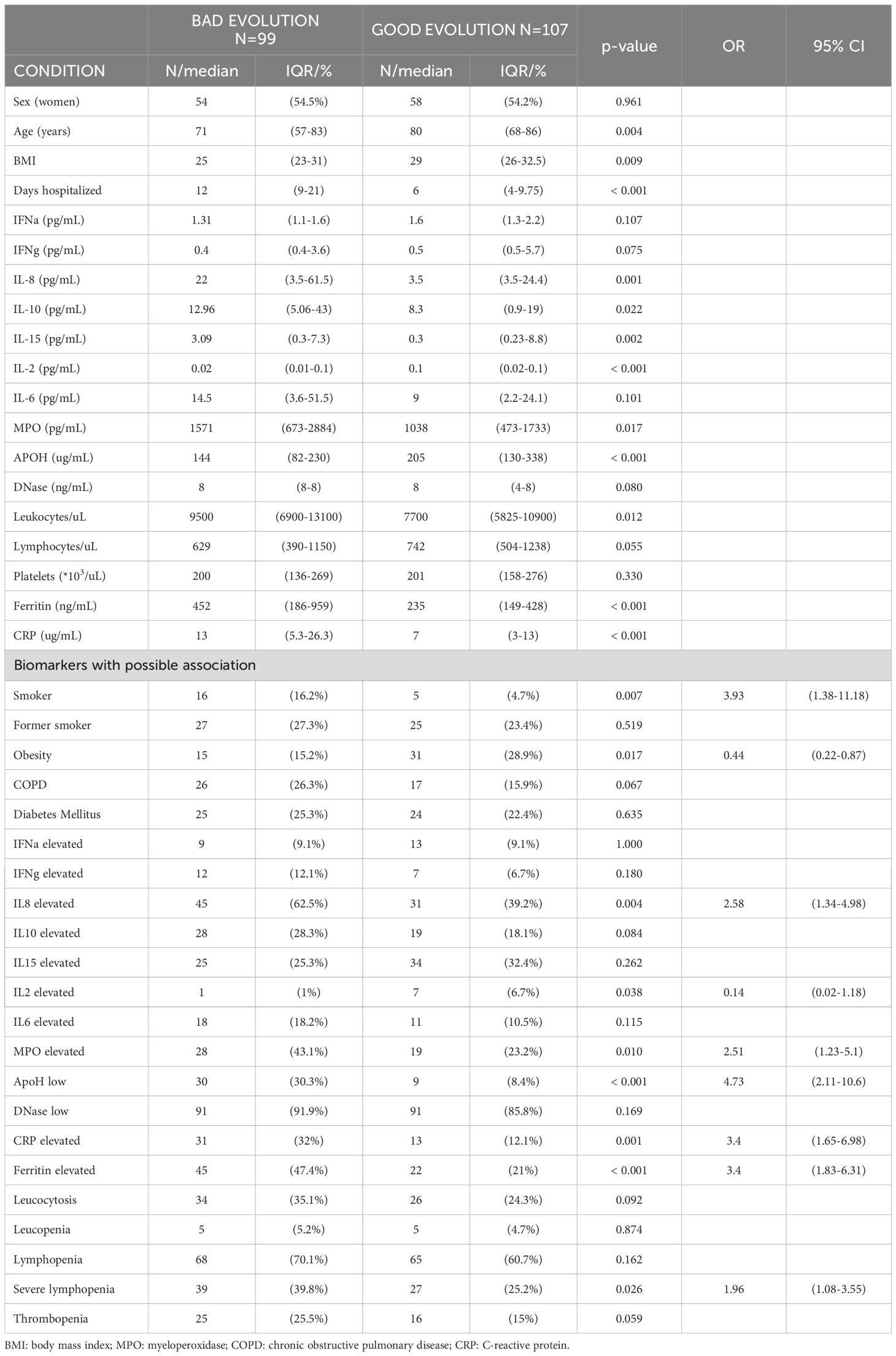
Table 1 Comparison of the input parameters of patients with influenza who had a poor outcome versus those who had a course without serious complications.
Comparing the cytokine profile between patients with severe form and those with good evolution, it was detected that those with severe form presented an altered immune serum-proteins profile (Table 1).
Severe patients showed a marked reduction of ApoH levels compared to those with good evolution: 144 (82-230) vs 205 (130-338) µg/mL (p<0.01) and compared with healthy controls: 209 (168-256) µg/mL (p=0.001) (Figure 2). The proportion of patients with low ApoH levels was higher in patients with severe form than in those with good evolution (30.3% vs 8.4%, p <0.001, Table 1). No significant differences were observed in the levels of DNase activity and in the proportion of patients with low DNase between patients with severe or mild forms of the disease.
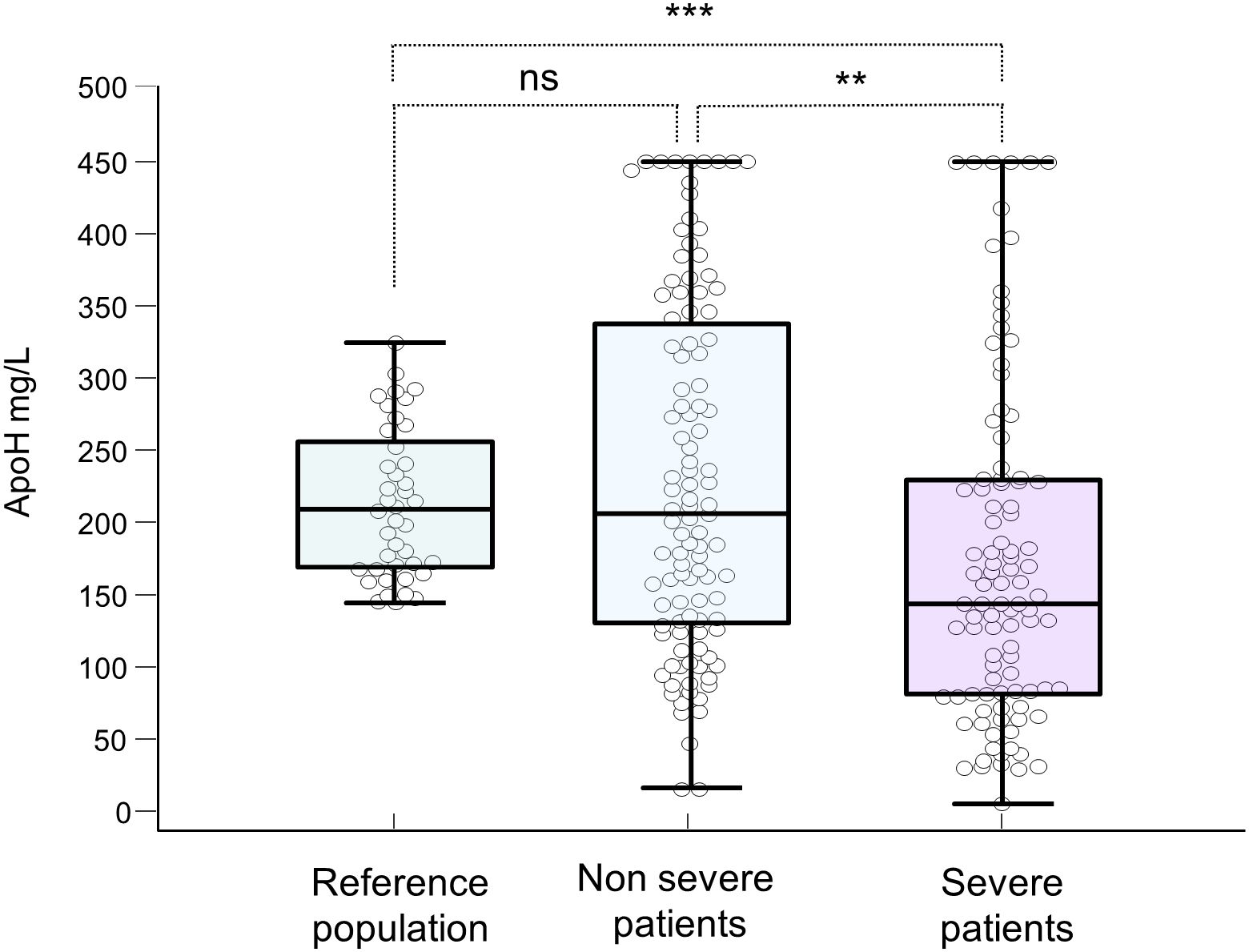
Figure 2 Blood levels of Apolipoprotein H in the blood (mg/L) of healthy people, mild and severe patients. **: p-value ≤ 0.01; ***:p-value ≤ 0.001; ns: not significant. p-value > 0.05.
A first multivariate analysis was performed with those variables associated with poor outcome that had shown a p value < 0.05 in the previous univariate analysis (Table 2A), in which, elevated levels of IL8, MPO and ferritin did not behave as independent variables. Since these three variables are associated with the formation of NETs and increase together in its formation (would be interdependent), it was performed a new multivariate analysis in which only one of the variables associated with NETs was assessed: ferritin levels (see discussion below). In this second multivariate analysis, smoking habit (OR 3.41), low levels of ApoH (OR 5.43) and high levels of Ferritin (OR 2.63) and CRP (OR 2.73) behaved as independent risk factors associated with poor outcome (Table 2B).
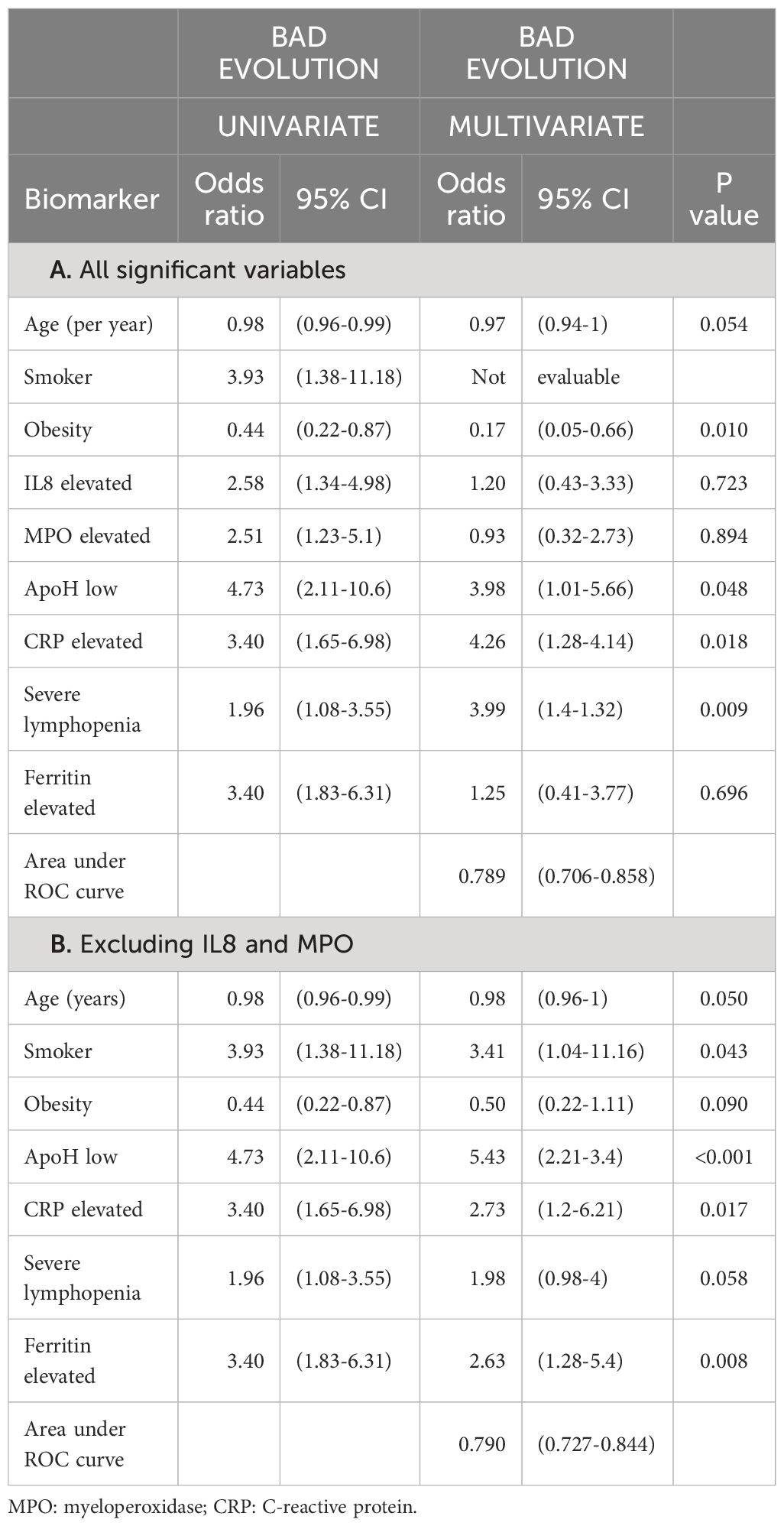
Table 2 Multivariate analysis of the markers that were associated with poor flu outcomes detected in a first univariate analysis of influenza patients.
Besides, although not significant in the multivariate study, there was a higher incidence of the H1N1pmd09 subtype in patients with a worse prognosis (27% vs 13%, p<0.001) (Supplementary Table S4).
3.3 Patients with respiratory failure
Sixty-one patients suffered RF. Patients with RF were younger than the rest: 70 (57-80) vs 79 (66-86) years, (p<0.001) and had higher rates of smoking (23% vs 4.8%, p<0.001) and COPD (32.8% vs 15.9%, p=0.006). Also had a higher prevalence of high levels of CRP, IL8, IL10 and MPO than in those with normal ventilatory function (OR 2.42, 2.69, 2.36 and 2.33 respectively). Patients with RF presented higher proportion of patients with low ApoH levels (OR: 4.25, p <0.001) (Table 3).
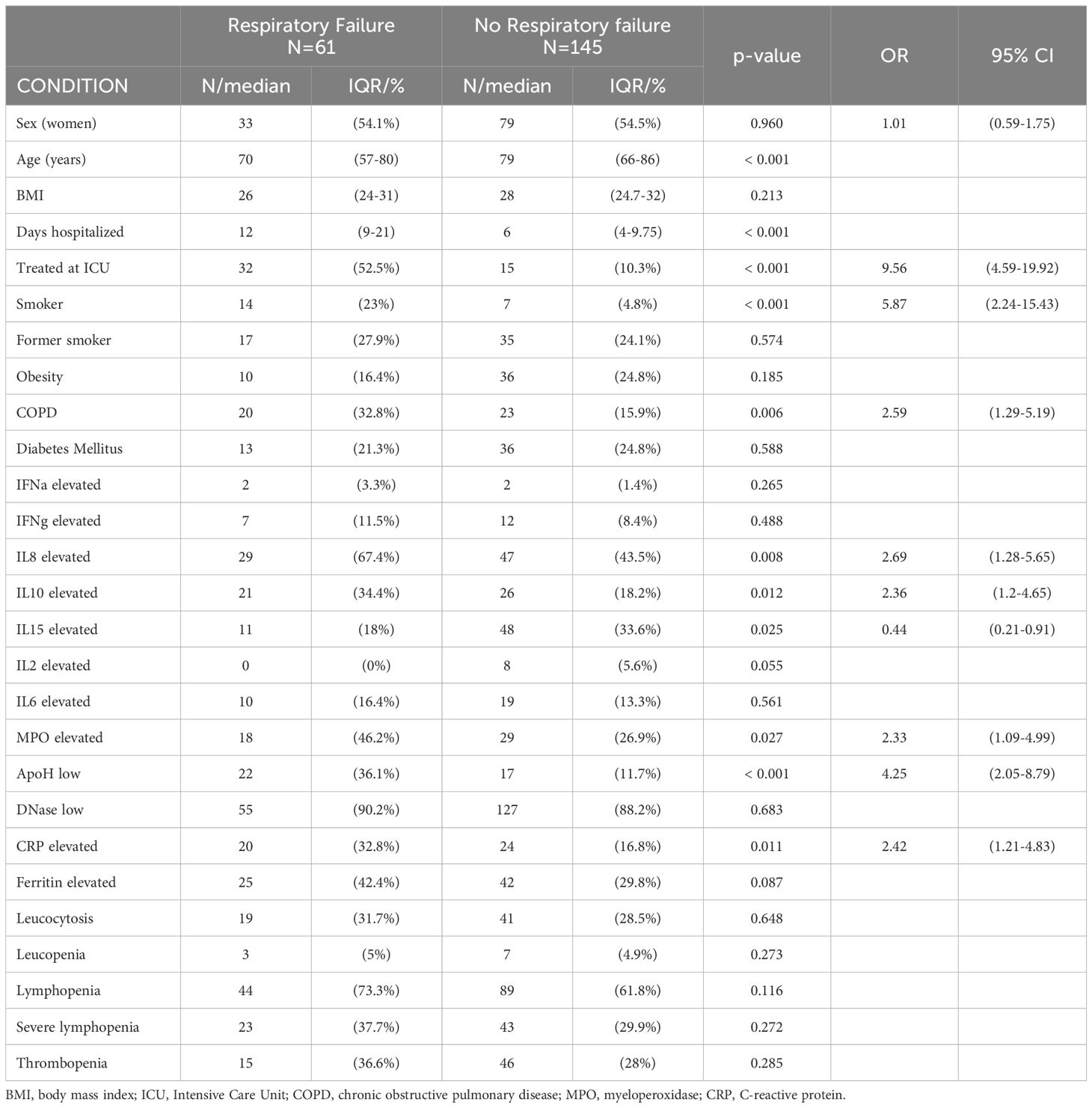
Table 3 Characteristics of influenza patients with respiratory failure versus those who did not have respiratory complications.
A first multivariate analysis was performed including the variables that in a previous univariate analysis were significant. Four variables were identified as independent, all with statistical significance: smoking, COPD and low ApoH, behaved as independent risk factors and high levels of IL15 behaved as a protective factor (Supplementary Table S5). As eight variables were analysed over 61 events, with a ratio of only 7.6 events per variable (in the literature 10 are recommended) (36), a second analysis was carried out, restricting to six variables. The variables smoking, low levels of ApoH, high levels of CRP and high levels of IL10 behaved as independent and significant risk factors (OR: 5.25, 5.12, 2.97 and 3.12, p<0.01) while high levels of IL15 behaved as an independent protective factor (OR: 0.30, p<0.01) (area under the ROC curve 0.788, 95%CI: 0.725-0.842) (Table 4).
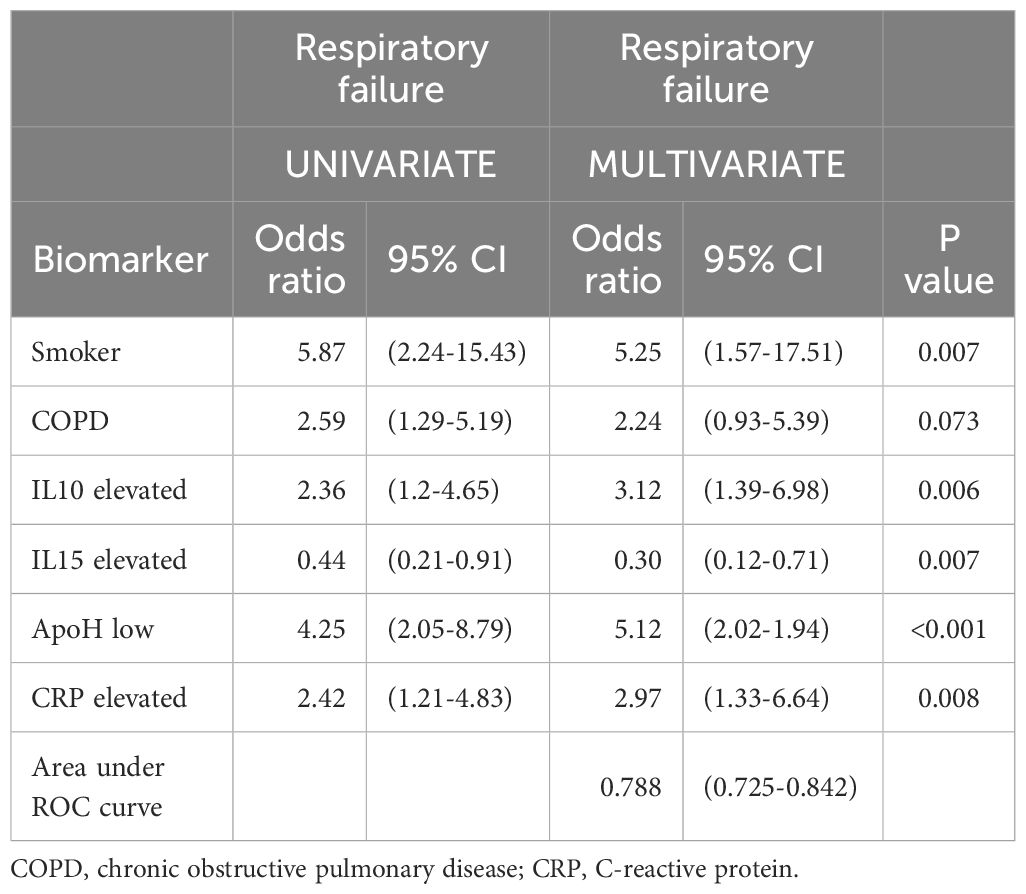
Table 4 Multivariate analysis of the markers associated with respiratory failure in the 61 influenza patients who had this evolution.
4 Discussion
Our work would suggest that the initial state of innate immunity during influenza infection may have a significant impact on clinical progression. It is noteworthy that in our study, patients with severe forms were younger and less obese than those who suffered a less severe disease. These results contrast with those who reported that age could be related to disease severity (37, 38). This discrepancy may arise from potential biases in the hospital admission of older or fatter people, who are admitted even if they suffer from very mild forms because they have a greater risk of suffering complications, in order to be able to act quickly if problems arise. These results could suggest that elderly, who are supposed to present more complications, are well monitored and controlled seasonally. In this study, the decision on the need for hospital admission was made by the Emergency Department physicians before the patient was assessed and included by the research team.
CRP stands out as independent risk factor for disease severity aligning with previous findings in both influenza and SARS-CoV-2 (34, 39, 40). In the same way high levels of ferritin have been identified as an independent risk factor for disease severity, as previously reported. The identification of high ferritin levels as an independent risk factor for severe influenza is consistent with previous studies (41). Ferritin is a key regulator in iron homeostasis and it is considered an acute inflammatory reactant. Nowadays it is suggested that ferritin is an important modulator of the innate and adaptive immune system (42–44).
Severe patients presented significantly higher levels of IL-8 as previously reported (15). The measurement of IL-8 during the acute phase proves crucial, as it correlates strongly with clinical complications. IL-8 is a potent chemoattractant and activator for myeloid leukocytes and neutrophils which acts through CXCR1, CXCR2 and NF-κB (15, 45). IL-8 is implicated in neutrophils' recruitment to infectious or tumorigenic areas, promoting either protective or harmful immune responses (45). During respiratory viral infections, epithelial respiratory cells secrete a vast number of cytokines including IL-8 (46).
One of the main causes of NETosis is the potent activation of neutrophils via IL-8. Inside their granules exists a weaponry of antimicrobial molecules. Bacterial, viral or fungal infection are able to activate neutrophils, favouring the secretion of NETs (16). Although NETs are beneficial for the clearance of infections, they have been related to pathological conditions like pulmonary diseases, immunothrombosis or autoimmunity (47).
Recently it has been described that ferritin induces the formation of NETs in a way mediated by Macrophage Scavenger Receptor 1 (Msr1). Ferritin acts as a MSr1 ligand and trigger the NET formation pathway (48). This mechanism explains why in our study the biomarkers associated with NET activation (MPO or IL8) do not behave as independent variables when the hyperferritinemia variable is also present in the analysis. For this reason, in the definitive multivariate analysis, NET markers were excluded and represented with ferritin levels.
One of the major important regulators of NETosis is the DNase I activity, which elicits the crumbling of the traps to recover homeostasis (49). In influenza patients its activity is deficient suggesting that it is a defense mechanism to increase the persistence of NETs and, therefore, their microbicidal activity.
High IL-8 levels and low DNase I activity would facilitate a substantial influx of neutrophils to the lungs, forming long-stable NETs that contribute to infection clearance but also collateral damage to host cells due to the production of toxic molecules for own cells like reactive oxygen species (15). This provokes the destruction of alveolar epithelium and contributes to the development of cytokine storm. In that tendency, the administration of recombinant DNase or biological treatments against the IL-8 axis have reported important benefits in the prognosis of acute respiratory distress syndrome and cystic fibrosis (50, 51).
The analysis of blood levels of ApoH in influenza patients showed that low levels were associated with severe forms, indicating that this molecule behaves as a protective factor for the development of severe forms of the disease, especially those related to RF and hospitalization to ICU. Furthermore, ApoH levels in the entire cohort of influenza patients were significantly lower than those observed in healthy controls. These results are in line with previous publications from our group, where a significant reduction in ApoH levels was observed in COVID-19 and also demonstrated the role of this protein as a protective factor for RF (34). The association of low ApoH levels with severity in infectious disease has been previously described in patients with COVID-19 (34) and sepsis (52). In the same way the presence of ApoH has been identified as a protective factor for organ dysfunction and mortality in sepsis (53). The causes of the reduction of ApoH levels in infections are not well established yet. Among the known facts it stands out that ApoH expression is downregulated both in patients with severe influenza and COVID-19 (54).
ApoH is a key factor in the clearance of apoptotic/necrotic cells avoiding the inherent proinflammatory activity (55). Thus, the consumption of ApoH in the elimination of cellular debris would be added to the effect of the Virus-induced ApoH deregulation causing a situation of partial ApoH deficiency, especially at the local level, which could be related to poor control of proinflammatory cytokines.
The enhancement of the debris removal process has been proposed as a possible therapeutic intervention in inflammatory diseases (56). ApoH replacement therapy in patients with severe influenza and very low levels of the protein may be a suitable alternative. There is currently no possibility of administering recombinant ApoH although fresh plasma (not convalescent) can be used as an indirect way to replenish ApoH with good results in COVID-19 (57, 58). Low levels of ApoH behaved as the independent variable with the greatest statistical strength associated with RF. High levels of IL15 behaved as an independent protective variable. This finding is in contrast to the observation in COVID-19, where elevated levels of IL15 are associated with poor outcome (10), suggesting that the deregulation of the immune response in COVID 19 is different to influenza. Functionally, IL-15 is similar to IL-2 favouring the activation and proliferation of cell-mediated immunity. It may also play a role in the innate immune response to influenza infection (59). If its expression is excessive or chronically deregulated contribute to tissue damage and can be involved in the pathophysiology of the disease (60).
This work presents several limitations. The number of patients recruited is not large enough to confirm in a multivariate analysis subtype-related prognosis differences. Elderly people were considered at risk from a clinical point of view to consider admission to the ICU, although they did not have objective risk criteria. This bias was partially neutralized thanks to the multivariate analysis that allowed the role of each variable to be assessed independently of the age of the individual. The method used for DNase I activity detection exhibits low sensitivity, potentially explaining the lack of differences between severe and non-severe patients. The mRNA expression of ApoH should be assessed in parallel with the quantification of circulating molecule to figure out the reason of the deficiency.
Data availability statement
The raw data supporting the conclusions of this article will be made available by the authors, without undue reservation.
Ethics statement
The studies involving humans were approved by Clinical Research Ethics Committee of Hospital Universitario 12 de Octubre. The studies were conducted in accordance with the local legislation and institutional requirements. The participants provided their written informed consent to participate in this study.
Author contributions
SG: Formal Analysis, Methodology, Supervision, Validation, Writing – original draft, Writing – review & editing. AL: Conceptualization, Data curation, Formal Analysis, Supervision, Writing – original draft, Writing – review & editing. FG-E: Methodology, Writing – review & editing. RD-S: Data curation, Writing – review & editing. IL: Methodology, Writing – review & editing. AM: Methodology, Writing – review & editing. BD-B: Methodology, Writing – review & editing. LN: Methodology, Writing – review & editing. OC-M: Methodology, Writing – review & editing. DP: Methodology, Writing – review & editing. MR-R: Data curation, Writing – review & editing. BA: Data curation, Writing – review & editing. EA: Data curation, Writing – review & editing. DF: Methodology, Writing – review & editing. EP-A: Methodology, Writing – review & editing. CC: Data curation, Writing – review & editing. CL: Data curation, Writing – review & editing. AS: Conceptualization, Formal Analysis, Funding acquisition, Investigation, Resources, Supervision, Validation, Writing – original draft, Writing – review & editing. MS: Formal Analysis, Writing – review & editing.
Funding
The author(s) declare financial support was received for the research, authorship, and/or publication of this article. This study was supported by grants from Fundación Mutua Madrileña (Award number(s): P177122021); Instituto de Salud Carlos III (Award number(s): 17/01129 and 20/01361). This study was supported by grants from Fundación Mutua Madrileña (P177122021) and from the Spanish Ministry of Economy and Competitiveness, Instituto de Salud Carlos III (Fondo de Investigaciones Sanitarias [FIS] 17/01129 and [FIS] 20/01361).
Conflict of interest
The authors declare that the research was conducted in the absence of any commercial or financial relationships that could be construed as a potential conflict of interest.
Publisher’s note
All claims expressed in this article are solely those of the authors and do not necessarily represent those of their affiliated organizations, or those of the publisher, the editors and the reviewers. Any product that may be evaluated in this article, or claim that may be made by its manufacturer, is not guaranteed or endorsed by the publisher.
Supplementary material
The Supplementary Material for this article can be found online at: https://www.frontiersin.org/articles/10.3389/fimmu.2024.1443096/full#supplementary-material
References
1. Dawood FS, Iuliano AD, Reed C, Meltzer MI, Shay DK, Cheng PY, et al. Estimated global mortality associated with the first 12 months of 2009 pandemic influenza a H1n1 virus circulation: A modelling study. Lancet Infect Dis. (2012) 12:687–95. doi: 10.1016/S1473-3099(12)70121-4
2. Tokars JI, Olsen SJ, Reed C. Seasonal incidence of symptomatic influenza in the United States. Clin Infect Dis. (2018) 66:1511–8. doi: 10.1093/cid/cix1060
3. Shah NS, Greenberg JA, McNulty MC, Gregg KS, Riddell J, Mangino JE, et al. Severe influenza in 33 us hospitals, 2013-2014: Complications and risk factors for death in 507 patients. Infect Control Hosp Epidemiol. (2015) 36:1251–60. doi: 10.1017/ice.2015.170
4. Ortiz JR, Neuzil KM, Shay DK, Rue TC, Neradilek MB, Zhou H, et al. The burden of influenza-associated critical illness hospitalizations. Crit Care Med. (2014) 42:2325–32. doi: 10.1097/CCM.0000000000000545
5. Chien YS, Su CP, Tsai HT, Huang AS, Lien CE, Hung MN, et al. Predictors and outcomes of respiratory failure among hospitalized pneumonia patients with 2009 H1n1 influenza in Taiwan. J Infect. (2010) 60:168–74. doi: 10.1016/j.jinf.2009.12.012
6. Loubet P, Samih-Lenzi N, Galtier F, Vanhems P, Loulergue P, Duval X, et al. Factors associated with poor outcomes among adults hospitalized for influenza in France: A three-year prospective multicenter study. J Clin Virol. (2016) 79:68–73. doi: 10.1016/j.jcv.2016.04.005
7. Ribeiro AF, Pellini AC, Kitagawa BY, Marques D, Madalosso G, de Cassia Nogueira Figueira G, et al. Risk factors for death from influenza a(H1n1)Pdm09, state of Sao Paulo, Brazil, 2009. PLoS One. (2015) 10:e0118772. doi: 10.1371/journal.pone.0118772
8. Lalueza A, Folgueira D, Diaz-Pedroche C, Hernandez-Jimenez P, Ayuso B, Castillo C, et al. Severe lymphopenia in hospitalized patients with influenza virus infection as a marker of a poor outcome. Infect Dis (Lond). (2019) 51:543–6. doi: 10.1080/23744235.2019.1598572
9. Gil-Etayo FJ, Garcinuno S, Utrero-Rico A, Cabrera-Marante O, Arroyo-Sanchez D, Mancebo E, et al. An early th1 response is a key factor for a favorable covid-19 evolution. Biomedicines. (2022) 10(2):296. doi: 10.3390/biomedicines10020296
10. Gil-Etayo FJ, Suarez-Fernandez P, Cabrera-Marante O, Arroyo D, Garcinuno S, Naranjo L, et al. T-helper cell subset response is a determining factor in Covid-19 progression. Front Cell Infect Microbiol. (2021) 11:624483. doi: 10.3389/fcimb.2021.624483
11. Garcinuno S, Gil-Etayo FJ, Mancebo E, Lopez-Nevado M, Lalueza A, Diaz-Simon R, et al. Effective natural killer cell degranulation is an essential key in Covid-19 evolution. Int J Mol Sci. (2022) 23(12):6577. doi: 10.3390/ijms23126577
12. Bermejo-Martin JF, Ortiz de Lejarazu R, Pumarola T, Rello J, Almansa R, Ramirez P, et al. Th1 and th17 hypercytokinemia as early host response signature in severe pandemic influenza. Crit Care. (2009) 13:R201. doi: 10.1186/cc8208
13. Hagau N, Slavcovici A, Gonganau DN, Oltean S, Dirzu DS, Brezoszki ES, et al. Clinical aspects and cytokine response in severe H1n1 influenza a virus infection. Crit Care. (2010) 14:R203. doi: 10.1186/cc9324
14. Turner RB, Weingand KW, Yeh CH, Leedy DW. Association between interleukin-8 concentration in nasal secretions and severity of symptoms of experimental rhinovirus colds. Clin Infect Dis. (1998) 26:840–6. doi: 10.1086/513922
15. Ramos I, Fernandez-Sesma A. Modulating the innate immune response to influenza a virus: Potential therapeutic use of anti-inflammatory drugs. Front Immunol. (2015) 6:361. doi: 10.3389/fimmu.2015.00361
16. Vorobjeva NV, Chernyak BV. Netosis: Molecular mechanisms, role in physiology and pathology. Biochem (Mosc). (2020) 85:1178–90. doi: 10.1134/S0006297920100065
17. Angeletti A, Volpi S, Bruschi M, Lugani F, Vaglio A, Prunotto M, et al. Neutrophil extracellular traps-dnase balance and autoimmunity. Cells. (2021) 10(10):2667. doi: 10.3390/cells10102667
18. Demkow U. Molecular mechanisms of neutrophil extracellular trap (Nets) degradation. Int J Mol Sci. (2023) 24(5):4896. doi: 10.3390/ijms24054896
19. Zhu L, Liu L, Zhang Y, Pu L, Liu J, Li X, et al. High level of neutrophil extracellular traps correlates with poor prognosis of severe influenza a infection. J Infect Dis. (2018) 217:428–37. doi: 10.1093/infdis/jix475
20. Ravindran M, Khan MA, Palaniyar N. Neutrophil extracellular trap formation: Physiology, pathology, and pharmacology. Biomolecules. (2019) 9(8):365. doi: 10.3390/biom9080365
21. Johnson EE, Wessling-Resnick M. Iron metabolism and the innate immune response to infection. Microbes Infect. (2012) 14:207–16. doi: 10.1016/j.micinf.2011.10.001
22. Ganz T. Iron in innate immunity: Starve the invaders. Curr Opin Immunol. (2009) 21:63–7. doi: 10.1016/j.coi.2009.01.011
23. Nakayama T, Sonoda S, Urano T, Yamada T, Okada M. Monitoring both serum amyloid protein a and C-reactive protein as inflammatory markers in infectious diseases. Clin Chem. (1993) 39:293–7. doi: 10.1093/clinchem/39.2.293
24. Mehdi H, Kaplan MJ, Anlar FY, Yang X, Bayer R, Sutherland K, et al. Hepatitis B virus surface antigen binds to apolipoprotein H. J Virol. (1994) 68:2415–24. doi: 10.1128/jvi.68.4.2415-2424.1994
25. Ehlting C, Wolf SD, Bode JG. Acute-phase protein synthesis: A key feature of innate immune functions of the liver. Biol Chem. (2021) 402:1129–45. doi: 10.1515/hsz-2021-0209
26. Watanabe-Kusunoki K, Nakazawa D, Ishizu A, Atsumi T. Thrombomodulin as a physiological modulator of intravascular injury. Front Immunol. (2020) 11:575890. doi: 10.3389/fimmu.2020.575890
27. Fay WP. Linking inflammation and thrombosis: role of C-reactive protein. World J Cardiol. (2010) 2:365–9. doi: 10.4330/wjc.v2.i11.365
28. McDonnell T, Wincup C, Buchholz I, Pericleous C, Giles I, Ripoll V, et al. The role of beta-2-glycoprotein I in health and disease associating structure with function: More than just aps. Blood Rev. (2020) 39:100610. doi: 10.1016/j.blre.2019.100610
29. Clarke MC, Figg NL, Bennett MR. Vascular smooth muscle cell apoptosis induces interleukin-1-directed inflammation: effects of hyperlipidemia-mediated inhibition of phagocytosis. Circ Res. (2010) 106:363–72. doi: 10.1161/CIRCRESAHA.109.208389
30. Arreola-Diaz R, Majluf-Cruz A, Sanchez-Torres LE, Hernandez-Juarez J. The pathophysiology of the antiphospholipid syndrome: A perspective from the blood coagulation system. Clin Appl Thromb Hemost. (2022) 28:10760296221088576. doi: 10.1177/10760296221088576
31. Noris M, Remuzzi G. Overview of complement activation and regulation. Semin Nephrol. (2013) 33:479–92. doi: 10.1016/j.semnephrol.2013.08.001
32. Perez L. Acute phase protein response to viral infection and vaccination. Arch Biochem Biophys. (2019) 671:196–202. doi: 10.1016/j.abb.2019.07.013
33. Rice TW, Wheeler AP, Bernard GR, Hayden DL, Schoenfeld DA, Ware LB. Comparison of the spo2/fio2 ratio and the pao2/fio2 ratio in patients with acute lung injury or ards. Chest. (2007) 132:410–7. doi: 10.1378/chest.07-0617
34. Serrano M, Espinosa G, Lalueza A, Bravo-Gallego LY, Diaz-Simon R, Garcinuno S, et al. Beta-2-glycoprotein-I deficiency could precipitate an antiphospholipid syndrome-like prothrombotic situation in patients with coronavirus disease 2019. ACR Open Rheumatol. (2021) 3:267–76. doi: 10.1002/acr2.11245
35. Garley M, Omeljaniuk WJ, Motkowski R, Ratajczak-Wrona W, Jablonska E, Filipkowski D, et al. Immunoaging - the effect of age on serum levels of net biomarkers in men: A pilot study. Int J Occup Med Environ Health. (2023) 36:333–48. doi: 10.13075/ijomeh.1896.02125
36. Vittinghoff E, McCulloch CE. Relaxing the rule of ten events per variable in logistic and cox regression. Am J Epidemiol. (2007) 165:710–8. doi: 10.1093/aje/kwk052
37. Paget J, Staadegaard L, Wang X, Li Y, van Pomeren T, van Summeren J, et al. Global and national influenza-associated hospitalisation rates: Estimates for 40 countries and administrative regions. J Glob Health. (2023) 13:4003. doi: 10.7189/jogh.13.04003
38. Kalil AC, Thomas PG. Influenza virus-related critical illness: Pathophysiology and Epidemiology. Crit Care. (2019) 23:258. doi: 10.1186/s13054-019-2539-x
39. Laguna-Goya R, Utrero-Rico A, Talayero P, Lasa-Lazaro M, Ramirez-Fernandez A, Naranjo L, et al. Il-6-based mortality risk model for hospitalized patients with Covid-19. J Allergy Clin Immunol. (2020) 146:799–807 e9. doi: 10.1016/j.jaci.2020.07.009
40. Serino M, Melo N, Caldas JP, Ferreira A, Garcia D, Lourenco P. Predictors of severity and in-hospital mortality in patients with influenza. Monaldi Arch Chest Dis. (2021) 92(1). doi: 10.4081/monaldi.2021.1876
41. Lalueza A, Ayuso B, Arrieta E, Trujillo H, Folgueira D, Cueto C, et al. Elevation of serum ferritin levels for predicting a poor outcome in hospitalized patients with influenza infection. Clin Microbiol Infect. (2020) 26:1557 e9– e15. doi: 10.1016/j.cmi.2020.02.018
42. Kernan KF, Carcillo JA. Hyperferritinemia and inflammation. Int Immunol. (2017) 29:401–9. doi: 10.1093/intimm/dxx031
43. Rosario C, Zandman-Goddard G, Meyron-Holtz EG, D'Cruz DP, Shoenfeld Y. The hyperferritinemic syndrome: Macrophage activation syndrome, still's disease, septic shock and catastrophic antiphospholipid syndrome. BMC Med. (2013) 11:185. doi: 10.1186/1741-7015-11-185
44. Ruscitti P, Di Cola I, Di Muzio C, Italiano N, Ursini F, Giacomelli R, et al. Expanding the spectrum of the hyperferritinemic syndrome, from pathogenic mechanisms to clinical observations, and therapeutic implications. Autoimmun Rev. (2022) 21:103114. doi: 10.1016/j.autrev.2022.103114
45. Teijeira A, Garasa S, Ochoa MC, Villalba M, Olivera I, Cirella A, et al. Il8, neutrophils, and nets in a collusion against cancer immunity and immunotherapy. Clin Cancer Res. (2021) 27:2383–93. doi: 10.1158/1078-0432.CCR-20-1319
46. Skoner DP, Gentile DA, Patel A, Doyle WJ. Evidence for cytokine mediation of disease expression in adults experimentally infected with influenza a virus. J Infect Dis. (1999) 180:10–4. doi: 10.1086/314823
47. Njeim R, Azar WS, Fares AH, Azar ST, Kfoury Kassouf H, Eid AA. Netosis contributes to the pathogenesis of diabetes and its complications. J Mol Endocrinol. (2020) 65:R65–76. doi: 10.1530/JME-20-0128
48. Jia J, Wang M, Meng J, Ma Y, Wang Y, Miao N, et al. Ferritin triggers neutrophil extracellular trap-mediated cytokine storm through msr1 contributing to adult-onset still's disease pathogenesis. Nat Commun. (2022) 13:6804. doi: 10.1038/s41467-022-34560-7
49. Katkar GD, Sundaram MS, NaveenKumar SK, Swethakumar B, Sharma RD, Paul M, et al. Netosis and lack of dnase activity are key factors in echis carinatus venom-induced tissue destruction. Nat Commun. (2016) 7:11361. doi: 10.1038/ncomms11361
50. Teijeira A, Garasa S, Ochoa MDC, Cirella A, Olivera I, Glez-Vaz J, et al. Differential interleukin-8 thresholds for chemotaxis and netosis in human neutrophils. Eur J Immunol. (2021) 51:2274–80. doi: 10.1002/eji.202049029
51. Uddin M, Watz H, Malmgren A, Pedersen F. Netopathic inflammation in chronic obstructive pulmonary disease and severe asthma. Front Immunol. (2019) 10:47. doi: 10.3389/fimmu.2019.00047
52. Schrijver IT, Kemperman H, Roest M, Kesecioglu J, de Lange DW. Beta-2-glycoprotein I as a biomarker for sepsis in critically ill patients in the intensive care unit: A prospective cohort study. Crit Care. (2020) 24:341. doi: 10.1186/s13054-020-03066-3
53. Ruiz-Sanmartin A, Ribas V, Sunol D, Chiscano-Camon L, Palmada C, Bajana I, et al. Characterization of a proteomic profile associated with organ dysfunction and mortality of sepsis and septic shock. PLoS One. (2022) 17:e0278708. doi: 10.1371/journal.pone.0278708
54. Ackermann M, Verleden SE, Kuehnel M, Haverich A, Welte T, Laenger F, et al. Pulmonary vascular endothelialitis, thrombosis, and angiogenesis in Covid-19. N Engl J Med. (2020) 383:120–8. doi: 10.1056/NEJMoa2015432
55. Barth ND, Marwick JA, Vendrell M, Rossi AG, Dransfield I. The "Phagocytic synapse" and clearance of apoptotic cells. Front Immunol. (2017) 8:1708. doi: 10.3389/fimmu.2017.01708
56. Poon IK, Lucas CD, Rossi AG, Ravichandran KS. Apoptotic cell clearance: Basic biology and therapeutic potential. Nat Rev Immunol. (2014) 14:166–80. doi: 10.1038/nri3607
57. Khamis F, Al-Zakwani I, Al Hashmi S, Al Dowaiki S, Al Bahrani M, Pandak N, et al. Therapeutic plasma exchange in adults with severe Covid-19 infection. Int J Infect Dis. (2020) 99:214–8. doi: 10.1016/j.ijid.2020.06.064
58. Zhang L, Zhai H, Ma S, Chen J, Gao Y. Efficacy of therapeutic plasma exchange in severe Covid-19 patients. Br J Haematol. (2020) 190:e181–e3. doi: 10.1111/bjh.16890
59. Verbist KC, Rose DL, Cole CJ, Field MB, Klonowski KD. Il-15 participates in the respiratory innate immune response to influenza virus infection. PLoS One. (2012) 7:e37539. doi: 10.1371/journal.pone.0037539
Keywords: influenza, flu, apolipoprotein H, ApoH, β2GPI, IL15, IL8, early immune response
Citation: Garcinuño S, Lalueza A, Gil-Etayo FJ, Díaz-Simón R, Lizasoain I, Moraga A, Diaz-Benito B, Naranjo L, Cabrera-Marante O, Pleguezuelo DE, Ruiz-Ruigomez M, Ayuso B, Arrieta E, Folgueira D, Paz-Artal E, Cueto C, Lumbreras C, Serrano A and Serrano M (2024) Immune dysregulation is an important factor in the underlying complications in Influenza infection. ApoH, IL-8 and IL-15 as markers of prognosis. Front. Immunol. 15:1443096. doi: 10.3389/fimmu.2024.1443096
Received: 03 June 2024; Accepted: 15 July 2024;
Published: 26 July 2024.
Edited by:
Heribert Stoiber, Innsbruck Medical University, AustriaReviewed by:
Theocharis Konstantinidis, Democritus University of Thrace, GreeceChristopher Jondle, Western Michigan University, United States
Copyright © 2024 Garcinuño, Lalueza, Gil-Etayo, Díaz-Simón, Lizasoain, Moraga, Diaz-Benito, Naranjo, Cabrera-Marante, Pleguezuelo, Ruiz-Ruigomez, Ayuso, Arrieta, Folgueira, Paz-Artal, Cueto, Lumbreras, Serrano and Serrano. This is an open-access article distributed under the terms of the Creative Commons Attribution License (CC BY). The use, distribution or reproduction in other forums is permitted, provided the original author(s) and the copyright owner(s) are credited and that the original publication in this journal is cited, in accordance with accepted academic practice. No use, distribution or reproduction is permitted which does not comply with these terms.
*Correspondence: Antonio Serrano, autoimmunity.imas12@h12o.es
†These authors have contributed equally to this work and share first authorship