- 1Department of Laboratory Medicine, The First Hospital of Jilin University, Changchun, China
- 2Cancer Center, The First Hospital of Jilin University, Changchun, China
- 3Prenatal Diagnosis Center, Reproductive Medicine Center, The First Hospital of Jilin University, Changchun, China
Background: The majority of patients with lung cancer exhibit drug resistance after anti-PD-1 immunotherapy, leading to shortened patient survival time. Previous studies have suggested an association between epigenetic abnormalities such as methylation and clinical response to anti-PD-1 immunotherapy, while the role of SUMOylation in resistance to anti-PD-1 antibody immunotherapy is still unclear.
Methods: Here, the mRNA expression of 15 SUMO machinery components in PBMC from lung cancer patients receiving anti-PD-1 immunotherapy were analyzed using real-time PCR. Base on the percentage change in mRNA levels, the relationship between the expression of SUMO machinery components and outcomes of anti-PD-1 immunotherapy, and the influencing factors of SUMOylation were evaluated. PBMC was treated with different concentrations of 2-D08 (a specific inhibitor of SUMOylation) in vitro, and analyzed the activation and the death rates of lymphocyte subsets by flow cytometry analysis.
Results: A predictive method, base on the gene expression of three SUMO machinery components (SUMO1, SUMO3 and UBE2I), were developed to distinguish non-responders to PD-1 inhibitors. Furthermore, the number of lymphocytes in peripheral blood significantly reduced in the dysregulated SUMOylation groups (the percentage change >100 or -50 ~ -100 groups). In vitro studies confirmed that lightly low SUMOylation level improved the activation status of T and NK lymphocytes, but extremely low SUMOylation level lead to the increased death rates of lymphocytes.
Conclusion: Our findings implied that dysregulated gene expression of SUMO machinery components could induce the resistance of anti-PD-1 immunotherapy in lung cancer by upregulating the death of peripheral blood lymphocytes. These data might provide effective circulating biomarkers for predicting the efficacy of anti-PD-1 immunotherapy, and uncovered a novel regulatory mechanism of resistance to anti-PD-1 immunotherapy.
Introduction
Lung cancer is one of the malignant diseases with high incidence and mortality (1, 2). Since most patients with advanced lung cancer lose the opportunity for surgical resection, they often require anti-tumor immunotherapy, such as anti-programmed cell death-1 (PD-1) immunotherapy (3). However, the majority of patients remained unresponsive or worsening of disease after PD-1 blockade, which is known as the resistance to anti-PD-1 immunotherapy (4). Research indicates that resistance is a common cause of shortened survival and increased mortality in patients with anti-PD-1 immunotherapy (5). Therefore, it is important to comprehend mechanisms of the resistance to anti-PD-1 immunotherapy and identify patients who may potentially benefit from therapeutic schedule.
Numerous studies on anti-PD-1 immunotherapy have shown that epigenetic aberrations may lead to the resistance to PD-1 inhibition, thereby leading to poor prognosis (6, 7). Small ubiquitin-like modification (SUMOylation) is an epigenetic modification gaining most of the research interest recently (8). During SUMOylation, small ubiquitin-like modifiers (SUMO) are covalently attached to target proteins via an enzymatic cascade that requires the sequential action of SUMO activating enzyme, SUMO conjugating enzyme and SUMO ligases (9, 10). Similar to other epigenetic modifications, the covalent conjugation of SUMO to protein substrates is a reversible modification and cleaved by SUMO specific proteases (SENPs), i.e., deSUMOylation (11). Cumulative studies have indicated that SUMOylation regulates a number of biological processes, including carcinogenesis, cell cycle progression, apoptosis and immune responses (12). To date, five categories of SUMO machinery components had been identified, including SUMO isoforms (SUMO1~4), SUMO activating enzyme (SAE1 and UBA2), SUMO conjugating enzyme (Ubc9, encoded by UBE2I), SUMO ligases (PIAS1, etc) and SUMO specific proteases (SENP1, 2, 3, 5, 6, 7) (9, 10). Owing to the critical roles of SUMO machinery components in maintaining the steady-state level between SUMOylated and deSUMOylated in substrate proteins, it is conceivable that altered expression of these components can lead to various diseases, particularly cancer (13). For example, in glioblastoma (GBM), SUMO machinery components are upregulated, such as SUMO activating enzyme (SAE1), SUMO conjugating enzyme (Ubc9) and SUMO specific protease (SENP1), promoting tumor progression (14). Similarly, upregulated SUMO conjugating enzyme (Ubc9) promoted transcription factor Slug SUMOylation play a crucial role in hypoxia-induced lung cancer progression (15). Recently, epigenetic drugs have been shown to reduce the resistance of certain cancer patients to PD-1 inhibitors (16). For example, inhibitors of histone deacetylase or inhibitors of DNA Methyltransferase effectively overcome the resistance to anti-PD-1 immunotherapy in breast cancer and melanoma (17, 18). However, the role of SUMOylation in the resistance to anti-PD-1 immunotherapy is still unclear.
In anti-PD-1 immunotherapy, treatment with the PD-1 blockade interrupted the inhibitory effect mediated by PD-1/PD-L1 axis and restored activity of lymphocytes, which are the main effector cells with an anti-tumor function (19). Recent studies have found that, during anti-PD-1 immunotherapy, only a small fraction of tumor-infiltrating lymphocytes can specifically recognize and attack cancer cells to achieve therapeutic effects (20, 21). When they are exhausted, the continuous recruitment of a large number of peripheral blood lymphocytes into tumors for supplementing tumor infiltrating lymphocytes may be important for subsequent anti-PD-1 treatment responsiveness (22, 23). At present, the number of peripheral blood lymphocytes has been adopted as a clinical prediction tool for the therapeutic efficacy of anti-PD-1 immunotherapy (24, 25). Therefore, analyzing the factors that cause changes in peripheral blood lymphocyte count during anti-PD-1 immunotherapy may help to understand the mechanism of the resistance to anti-PD-1 immunotherapy and improve treatment efficacy.
In this study, we focused attention on the mRNA expression of 15 SUMO machinery components, including four SUMO genes (SUMO1~4), two SUMO activating enzyme genes (SAE1 and UBA2), a SUMO conjugating enzyme gene (UBE2I), two SUMO ligase genes (PIAS1 and PIAS2) and six SUMO specific protease genes (SENP 1,2,3,5,6,7), in peripheral blood mononuclear cells (PBMC). By analyzing these genes of 105 patients with lung cancer during anti-PD-1 immunotherapy, we developed a predictive method, based on the SUMO1, SUMO3 and UBE2I, to distinguish non-responders with a progressive disease. Further analysis showed that dysregulated gene expression of SUMO machinery components was associated with decreased peripheral lymphocyte counts during the resistance process of anti-PD-1 immunotherapy in lung cancer. The in vitro experiments validated that dysregulated SUMOylation were one reason for inducing increased lymphocyte death. Together, these results indicated that dysregulated gene expression of SUMO machinery components may be a potential underlying cause of developing resistance to anti-PD-1 immunotherapy, and effective circulating biomarkers for predicting the efficacy of this treatment.
Materials and methods
Study design
Patients were enrolled from conventional treatments at the First Hospital of Jilin University, Changchun, China, from January 2023 to December 2023. For inclusion criteria: patients were diagnosed with late-stage lung cancer (stage III/IV, including local, regional and distant recurrence). The treatment regimens were determined by clinicians based on the patient’s condition. All enrolled patients received PD-1 mAb intravenously once every 3 weeks until disease progression or unacceptable toxicity. Based on Response Evaluation Criteria in Solid Tumors (RECIST) V.1.1 criteria, these patients were divided into a responder (R) group and a non-responder (NR) group. Responders were defined as a patient who achieved complete response (CR), partial response (PR) or stable disease (SD) after anti-PD-1 immunotherapy for more than 24 weeks. Non-responders were defined as a patient who had progressive disease (PD) after anti-PD-1 immunotherapy for less than 24 weeks. Patients with other malignancies or comorbidities (e.g., heart failure, severe diabetes mellitus) were excluded. The study was approved by the Human Ethics Committee of the First Hospital of Jilin University (23K155-001). All participants had signed informed consent forms before collecting samples.
Real-time PCR
PBMC were isolated from lung cancer patients who received anti-PD-1 immunotherapy for 9 weeks by using Ficoll-Hypaque (Sigma Aldrich, USA) density gradient centrifugation. The 9th week is in line with the recommended time node for the first response assessment of tumor according to the guidelines and consensus (26–28). Total RNA was extracted from PBMC using MolPure Cell RNA Kit (YEASEN Biotech Co., Ltd, China) following the manufacturer’s instructions. cDNA synthesis was carried out by using Hifair III 1st Strand cDNA Synthesis SuperMix for qPCR (YEASEN Biotech Co., Ltd, China). The mRNA expression of 15 SUMO machinery components were analyzed by real-time PCR using the SYBR Green PCR Master Mix (YEASEN Biotech Co., Ltd, China). Considering the comparability of data, we combined the mRNA expression levels of PBMC from 10 untreated patients with lung cancer as the baseline value and defined it as 1. The relative mRNA expression levels of 15 SUMO machinery components in PBMC were calculated using the 2–ΔΔCT method. GAPDH served as an internal control. The sequences of real-time PCR primers were shown as Supplementary Table 1.
Prediction model
We calculated the percentage changes in relative mRNA level of 15 SUMO machinery components in PBMC from 105 lung cancer patients as the following formula: [(express value-baseline value)/baseline value]×100%. The utilization of the percentage change formula for data normalization had been implemented in multiple studies searching for biomarkers of cancer immunotherapy efficacy (29–31). We developed a risk score base on different percentage changes in SUMO machinery components, which could be used to identify the high risk patients for developing NR. The risk score of patient was defined as 2, if the percentage change in mRNA level of SUMO machinery components in PBMC was greater than 100 times or less than -50~-100 times. The risk score of patient was defined as 1, if the percentage change in mRNA level of SUMO machinery components in PBMC was less than 100 times and greater than -50. Receiver operating characteristic (ROC) curves were used to assess diagnostic accuracy of changes in SUMO machinery components in predicting the probability of NR.
PBMC under treatment with the SUMOylation inhibitor
To investigate the effect of SUMOylation inhibition on lymphocytes in peripheral blood, we chose 2-D08 (YEASEN Biotech Co., Ltd, China) to treat PBMC. 2-D08 is a specific inhibitor of SUMOylation that blocks the transfer of SUMO protein from the SUMO-Conjugating Enzyme (Ubc9) thioester conjugate to substrates. In the in vitro culture system, PBMCs isolated from 27 lung cancer patients treated with PD-1 antibody were cultured in RPMI1640 medium containing 10% fetal bovine serum (FBS; Thermo fisher scientific, USA). Along with the concentration of 2-D08 gradually increased, we observed the dose effect of SUMOylation inhibition on lymphocytes. After 24 hours of adding 2-D08, PBMCs were harvested and analyzed by flow cytometry.
Flow cytometry analysis
Samples were tested by 10-colour/three laser flow cytometer (FACSCantoTM, BD Bioscience, USA) and analyzed by BD FACSDiva™ software (BD Bioscience, USA). The following fluorescently-conjugated antibodies were used for cell phenotypic analysis: CD45- PerCP (BD Bioscience, USA; Cat#652803), CD3-FITC (BD Bioscience, USA; Cat#349201), CD4-APC-Cy7 (BD Bioscience, USA; Cat#557871), CD8-PE-Cy7 (BD Bioscience, USA; Cat#557746), CD19-APC (BD Bioscience, USA; Cat#652804), CD56- BV510 (BD Bioscience, USA; Cat#563041), CD69-Alexa Fluor 700 (BD Bioscience, USA; Cat#560739). The death of lymphocytes was measured by staining with Annexin V-PE antibody (BD Bioscience, USA; Cat#559763). All antibodies for flow cytometry in this study were purchased from BD Biosciences. The gating strategy for cell subsets were shown in corresponding figures.
Statistical analysis
Differences among normally distributed variables were analyzed using a Student’s t-test. For variables that were not normally distributed, a Mann-Whitney U test or Kruskal-Wallis ANOVA test was used. Statistical significance was shown as: * P< 0.05, ** P< 0.01. *** P< 0.001. Statistical analyses were performed using Prism 10.0 (GraphPad Prism, RRID: SCR_002798) and SPSS 27.0 statistical software package (SPSS, RRID: SCR_002865).
Results
The relationship between dysregulated gene expression of SUMO machinery components in PBMC and the prevalence rates of NR
To search for potential regulatory points that may influence clinical response to anti-PD-1 immunotherapy, we explored the relative mRNA expression of 15 SUMO machinery components in PBMC from 105 lung cancer patients (53 responders and 52 non-responders) for 9 weeks after anti-PD-1 immunotherapy (Figures 1A–C; Supplementary Figures 1, 2). We found that the average expression levels of each gene in PBMC was not significantly different between R group and NR group. However, compared with the R group, the dots in the scatter plot of gene expression in the NR group appeared to be more scattered. Subsequently, based on analysis of variance, the discretization of relative mRNA expression of 15 SUMO machinery components between the R and NR groups was investigated. As expected, except for SUMO4, SAE1, PIAS1 and SENP5, the discretization of the other 11 genes in the NR group was significantly higher than that in the R group (all p values < 0.05).
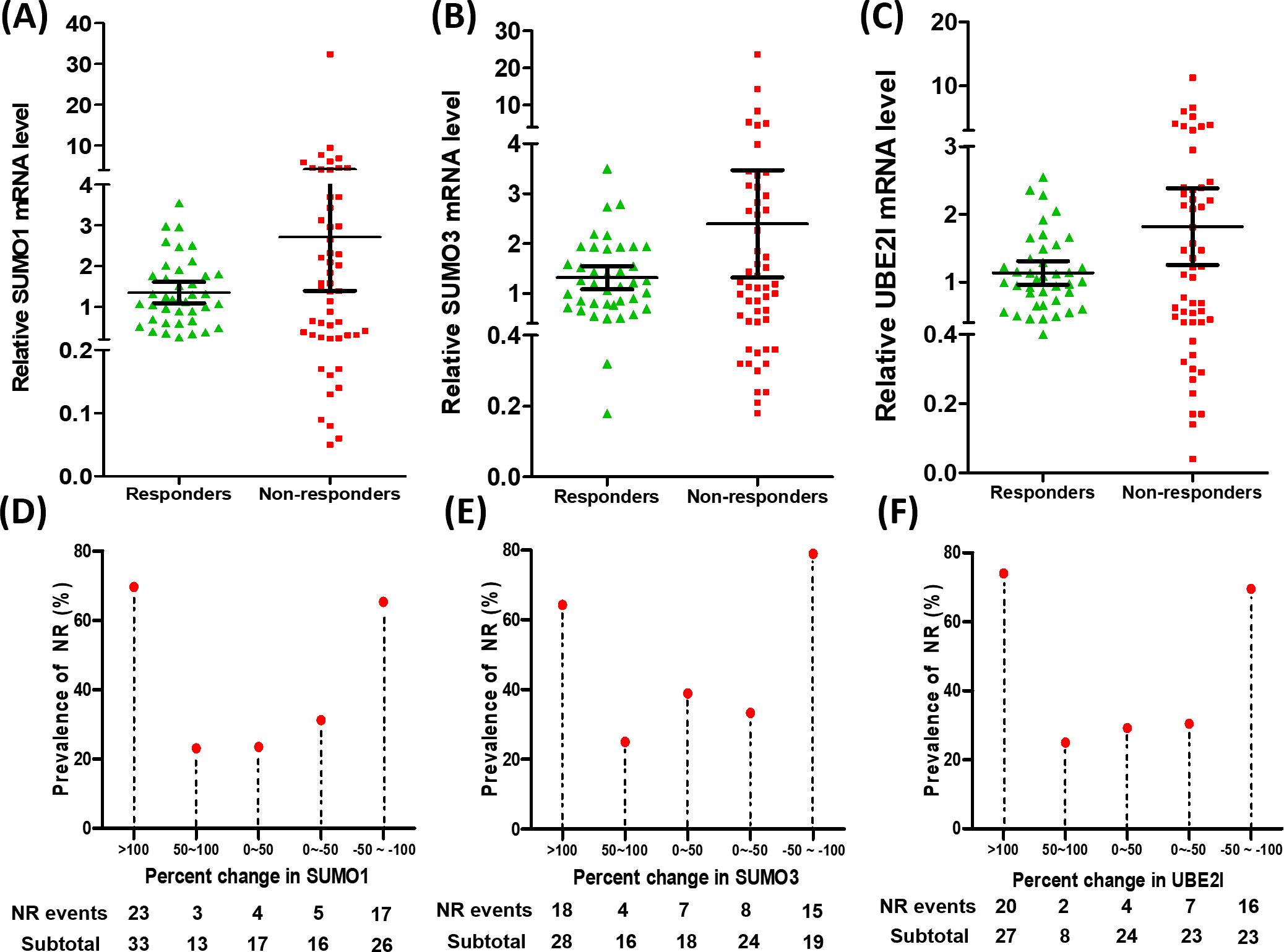
Figure 1. Dysregulated gene expression of SUMO machinery components in PBMC was associated with the prevalence rates of NR. (A–C) The relative mRNA expression of SUMO1 (A), SUMO3 (B) and UBE2I (C) in PBMC from responders (n=53) and non-responders (n=52). Data were expressed as mean ± 95%CI. (D–F) The percentage change in the relative mRNA expression of three SUMO machinery components (SUMO1, SUMO3 and UBE2I) was associated with the prevalence rates of NR.
In order to accurately evaluate the relationship between the mRNA expression of SUMO machinery components and outcomes of anti-PD-1 immunotherapy, we used the formula [(express value-baseline value)/baseline value] ×100% to calculate the relative percentage change in SUMO machinery component mRNA levels after implementation of anti PD-1 immunotherapy. The baseline value is the average mRNA expression level of corresponding SUMO machinery component in PBMCs of 10 untreated lung cancer patients. By calculating, we obtained the percentage change in relative mRNA levels and used it as an indicator of the severity of SUMOylation dysfunction (32–34). Based on the distribution of percentage change data, we roughly divided it into five groups, which are >100, 50~100, 0~50, 0~-50, -50~-100. Then the prevalence rates of NR based on specific percentage changes in relative mRNA levels were analyzed. The results showed that the prevalence rates of NR had significant differences among diverse percentage change groups of SUMO1 (Figure 1D, p=0.001), SUMO3 (Figure 1E, p=0.003) and UBE2I (Figure 1F, p<0.001). Notably, the prevalence rates of NR significantly increased in the highest percentage change group (>100) and the lowest percentage change group (-50~-100), compared with other three groups. On the contrary, the percentage change of other 12 genes had no significant correlation with the prevalence rates of NR (Supplementary Figures 1, 2). These results indicate that the dysregulation of SUMO1, SUMO3, and UBE2I expression in PBMC was positively correlated with the occurrence of NR and played an important role in the resistance process of anti PD-1 immunotherapy.
The predictive value of the mRNA levels of SUMO1, SUMO3 and UBE2I in PBMC for clinical response to anti-PD-1 immunotherapy
To evaluate the predictive ability of SUMO1, SUMO3 and UBE2I for clinical response to anti-PD-1 therapy, we developed a risk score based on different percentage changes in mRNA level of three genes. The patients were grouped into the high-risk group and scored as 2, if the percentage change in mRNA level of three genes in PBMC was greater than 100 times or less than -50~-100 times. The patient was grouped into the low-risk group and scored as 1, if the percentage change in mRNA level of three genes in PBMC was less than 100 times and greater than -50. ROC curves was used to assess diagnostic accuracy of the percentage change in three genes to predict the probability of NR (Figure 2). SUMO1 predicted the probability of NR with a sensitivity of 75.0% and specificity of 67.9% (cut-off value: 1.5; area under the curve [AUC]: 0.715; 95% confidence interval [CI]: 0.614 to 0.815; P <0.001). The AUC for SUMO3 to predict NR was 0.704 (cut-off value: 1.5; 95% CI: 0.603 to 0.805; P < 0.001), and the sensitivity and specificity were 63.5% and 77.4%, respectively. ROC analysis revealed that UBE2I (cut-off value: 1.5; AUC: 0.733; 95% CI 0.635-0.831; P < 0.001; sensitivity: 69.2%; specificity: 77.4%) had best predictive power for the probability of NR among three genes. Moreover, the analysis of combined three genes (cut-off value, AUC, sensitivity and specificity of 5.5, 0.791, 57.7% and 96.2%, respectively) yielded a higher diagnostic accuracy than that of single gene in NR diagnosis. These results suggested that the percentage change in mRNA level of 3 SUMO machinery components (SUMO1, SUMO3 and UBE2I), either alone or in combination, could to some extent predict clinical response to anti-PD-1 immunotherapy.
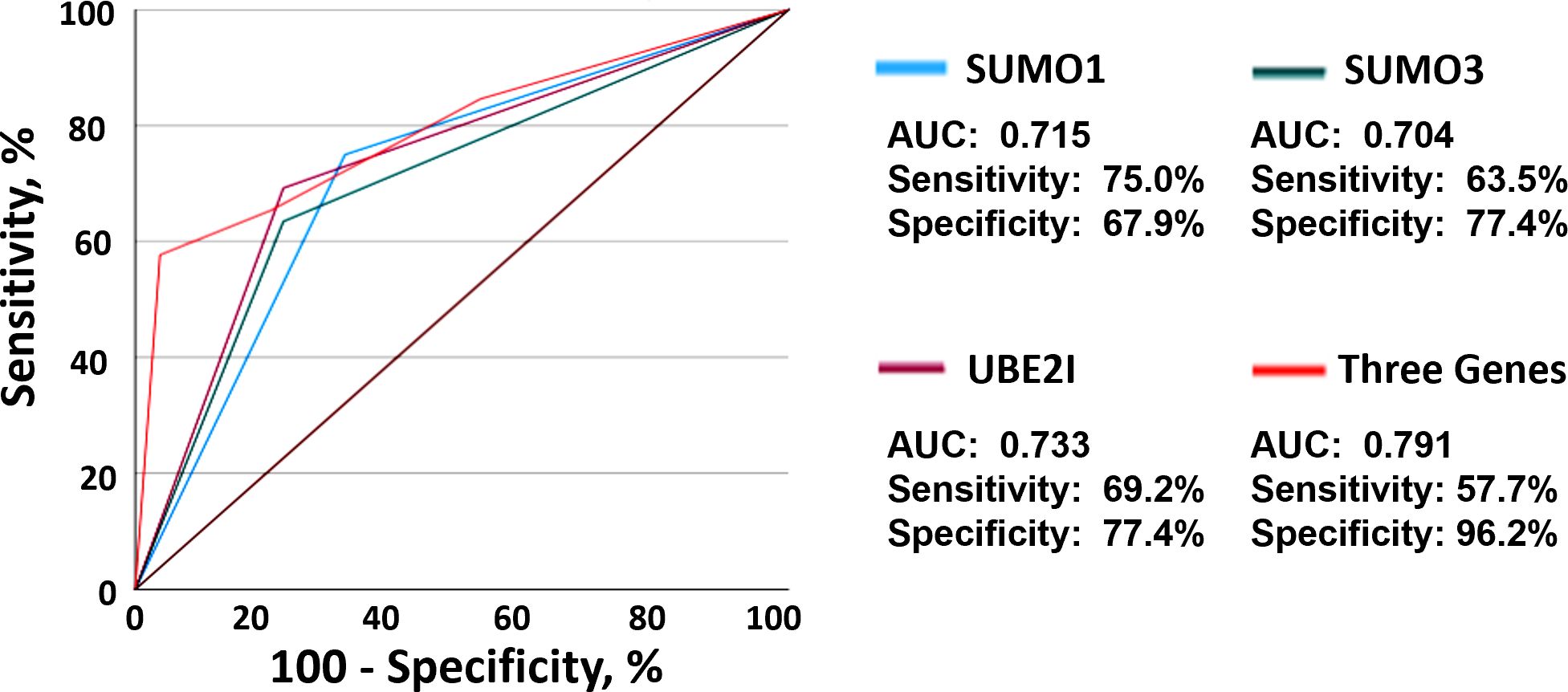
Figure 2. The mRNA levels of three SUMO machinery components predicted clinical response to anti-PD-1 immunotherapy. ROC analysis based on SUMO1 (blue), SUMO3 (green), UBE2I (purple) and combined three genes (red) for the diagnosis of non-responders was shown.
The influencing factors of dysregulated SUMO machinery components in peripheral blood of lung cancer patients
Due to the best predictive ability of UBE2I for NR among SUMO machinery components, we attempted to identify the influencing factors of SUMOylation based on the percentage change in mRNA level of UBE2I. The enrolled 105 patients were divided into five groups for evaluation of sociodemographic, clinical, and peripheral leukocyte characteristics. As shown in Table 1, the results showed that the percentage change in mRNA level of UBE2I was not associated with age, sex, histology, disease stage and treatment. By analyzing peripheral leukocyte characteristics, we found that the number of leukocytes (white blood cells, WBC) (Figure 3A), neutrophils (NEU) (Figure 3B) and monocytes (MON) (Figure 3C) had no significant differences among five groups. However, as shown in Figure 3D, the number of lymphocytes (LYM) were significantly reduced in the highest percentage change group (>100) and the lowest percentage change group (-50~-100). Subsequently, we analyzed the data of lymphocyte subsets from 90 patients who underwent peripheral lymphocyte subset analysis. The results showed that there was no significant differences in the proportion of various lymphocyte subsets among five groups (Figures 3E–H). Furthermore, based on the percentage change in mRNA level of SUMO1 (Supplementary Figure 3) and SUMO3 (Supplementary Figure 4), the characteristics of peripheral leukocytes were similar with UBE2I. Our data indicated that SUMOylation related non-response to anti-PD-1 immunotherapy might be associated with decreased peripheral lymphocyte count.
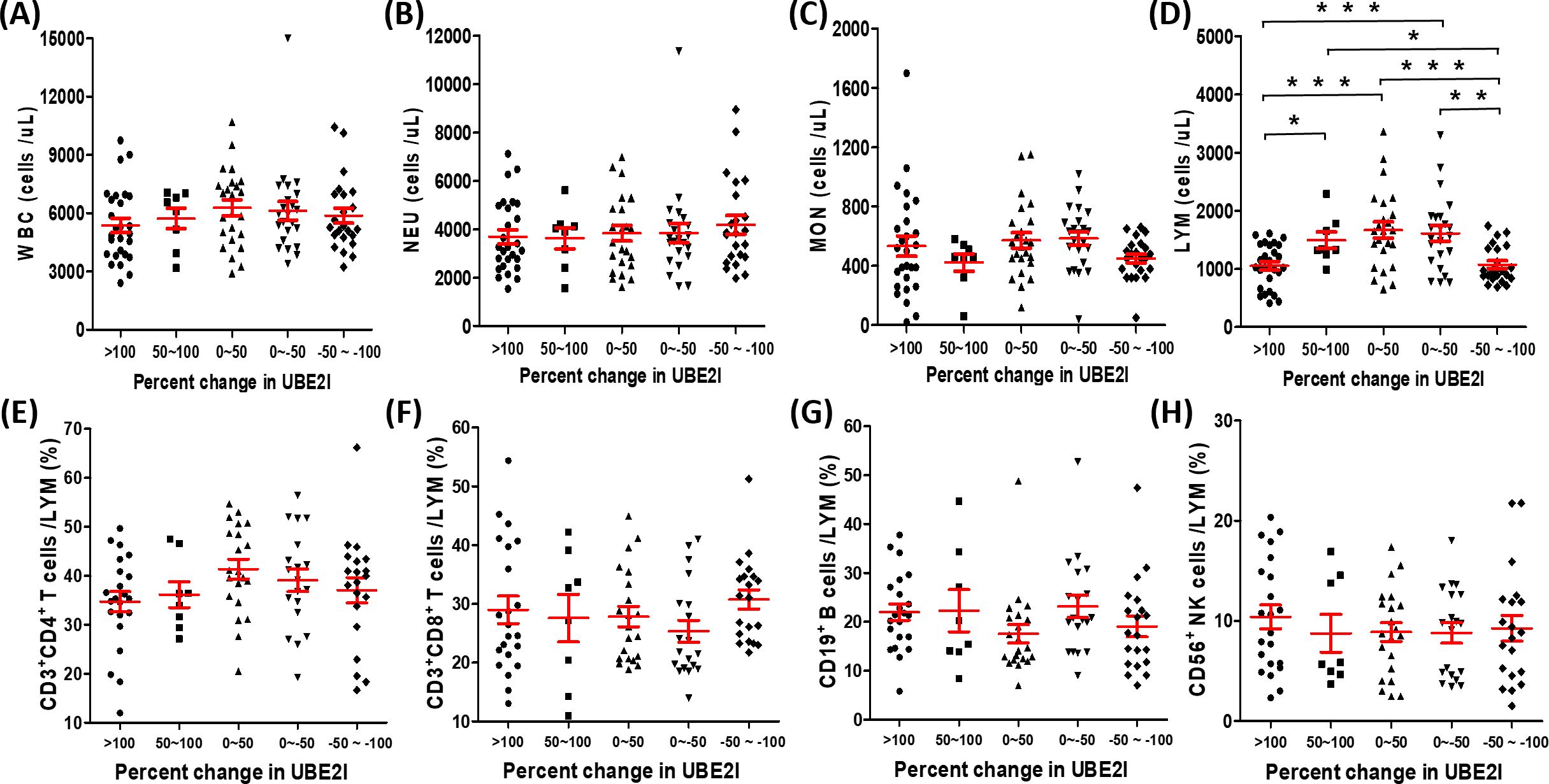
Figure 3. Association between the percentage change in UBE2I mRNA level and different white blood cell populations in peripheral blood of lung cancer patients. (A–D) The absolute counts of white blood cell (WBC), neutrophils (NEU), monocytes (MON) and lymphocytes (LYM) were compared among different groups base on the percentage change of UBE2I. (E–H) The percentages of lymphocyte subsets were compared among different groups base on the percentage change of UBE2I. Student’s paired t-test, * P < 0.05, ** P < 0.01, *** P < 0.001.
The regulatory effect of SUMOylation inhibition on lymphocyte activity and death
To investigate the effect of SUMOylation on lymphocytes in peripheral blood, we treated PBMC of 27 lung cancer patients receiving PD-1 treatment with different concentrations of 2-D08 (a specific inhibitor of SUMOylation) in vitro, and analyzed the activation status (CD69+) and the death rates (Annexin V+) of different lymphocyte subsets by flow cytometry analysis. The gating strategy was presented in Figure 4A. We found that the percentages of CD45+CD3+CD4+CD69+T cells (Figure 4B), CD45+CD3+CD8+CD69+T cells (Figure 4C) and CD45+CD3-CD56+CD69+NK cells (Figure 4D) were significantly up-regulated in a 2-D08 dose-dependent manner, but the gradually increasing concentrations of 2-D08 had no effect on the percentages of CD45+CD3-CD19+CD69+B cells (Figure 4E). Similarly, 2-D08 increased the percentage of CD45+CD3+CD4+Annexin V+T cells (Figure 4B), CD45+CD3+CD8+Annexin V+T cells (Figure 4C), and CD45+CD3-CD19+Annexin V+B cells (Figure 4E) in a dose-dependent manner at concentrations greater than 10μM. However, it was worth noting that 2-D08 reduced the percentage of these cells at 5μM concentrations (Figures 4B, C, E). Besides, CD45+CD3-CD56+NK cells also displayed an increased percentage of Annexin V+ cells at higher concentrations of 2-D08 (≥20μM) (Figure 4D). These results suggested that although inhibition of SUMOylation levels could improve the activation status of T and NK lymphocytes, extreme decline in SUMOylation levels would lead to the increased death rates of lymphocytes.
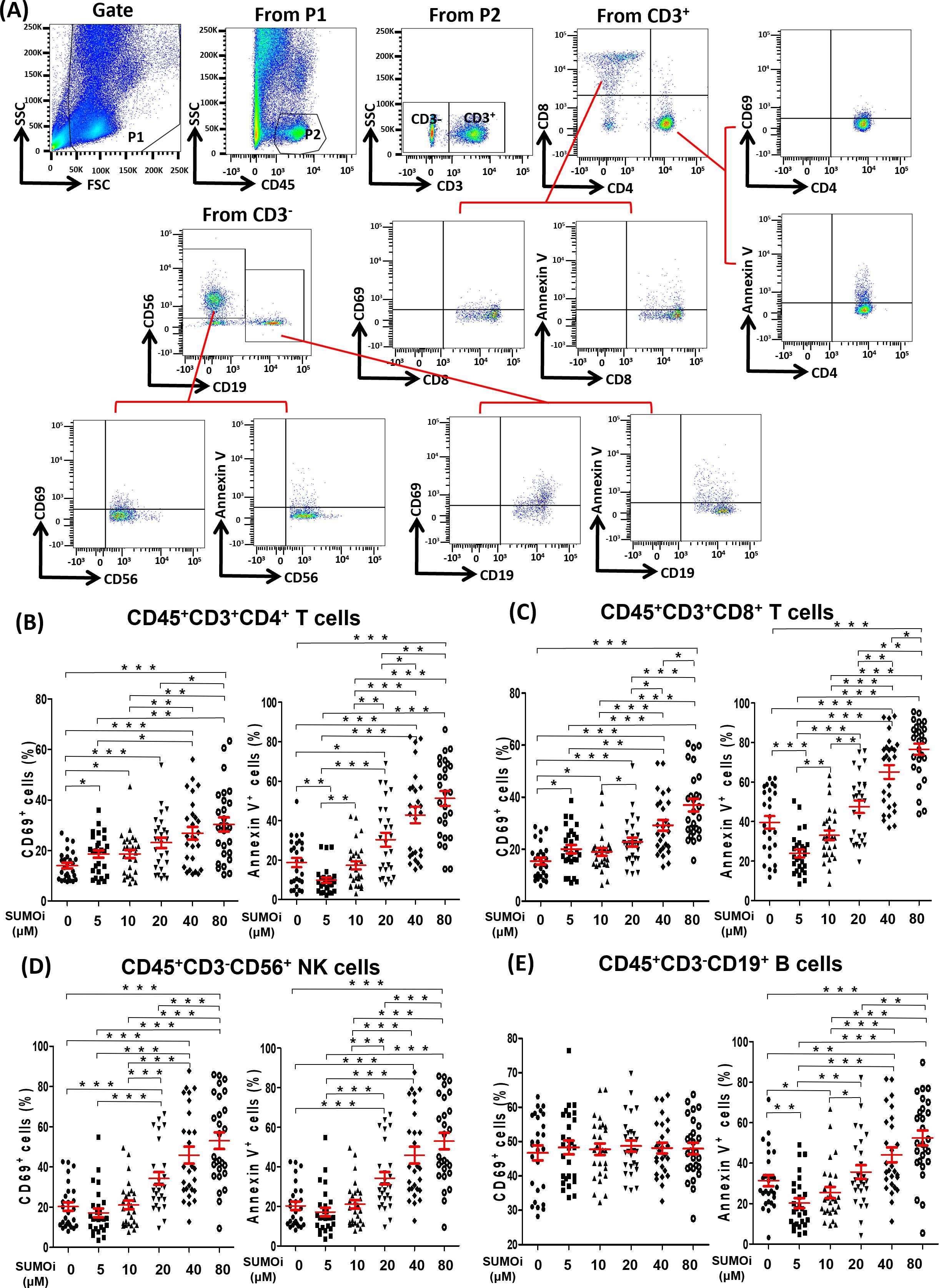
Figure 4. The relationship between the SUMOylation inhibition and the phenotype of lymphocyte. (A) Flow-cytometry dot plots showed the strategy for gating peripheral blood lymphocyte cells with CD69+ and Annexin V+ phenotype. (B-E) The expression of CD69 and Annexin V were analyzed on CD45+CD3+CD4+ T cells (B), CD45+CD3+CD8+ T cells (C), CD45+CD3-CD56+ NK cells (D) and CD45+CD3-CD19+ B cells (E). Student’s paired t-test, * P < 0.05, ** P < 0.01, *** P < 0.001.
Discussion
In this study, we found that the dysregulated mRNA expression (extremely high or low level) of three SUMO machinery components (SUMO1, SUMO3 and UBE2I) in PBMC was related to the resistance to anti-PD-1 immunotherapy. The mRNA expression of SUMO genes (either SUMO1 or SUMO3) plus the only SUMO E2 conjugating enzyme gene (UBE2I) was necessary for SUMOylation, and the abnormal change of their expression had a significant impact on SUMOylation level in PBMC (35). Therefore, we speculated that dysregulated SUMOylation (extremely high or low level) in PBMC may promote the occurrence of resistance to anti-PD-1 immunotherapy in lung cancer. It had been shown that up-regulation of certain SUMO machinery components correlated with the resistance in lung cancer (36). For example, UBA2 was highly expressed in lung cancer and it knockdown increased the sensitivity of cancer cell to etoposide and cisplatin (37). SENP1 had been reported to overexpressed in patients with lung cancer, and had a negative correlation with treatment response and could potentially predict chemosensitivity (38, 39). In spite of this, up-regulation of certain SUMO machinery components associated with favorable treatment response in some cases such as UBE2I 10920CG genotype enhances sensitivity to irinotecan chemotherapy in lung cancer through upregulation of SUMO1 in tumor cells (40). These findings suggested that SUMOylation might play a complex and crucial role in the resistance of lung cancer. However, previous researchers mainly focused on the SUMOylation level during the development of resistance in tumor cells, rather than lymphocytes, which is the main effector cell in anti-PD-1 immunotherapy (41, 42). The main subpopulation of PBMC analyzed in this study is lymphocyte (43, 44). It was reported that the homeostasis and function of lymphocytes could be used to predict the response to anti-PD-1 immunotherapy in lung cancer (25). Although the steady-state level of SUMOylation played an essential role in the homeostasis and function of lymphocytes, the relationship between dysregulated SUMOylation in lymphocytes and the resistance to anti-PD-1 immunotherapy in lung cancer was still unclear (45–48).
By analyzing the immunotherapy cohort of lung cancer, we found that dysregulated gene expression of SUMO machinery components were associated with decreased peripheral blood lymphocytes counts. It was reported that the dynamically monitoring with peripheral blood lymphocytes count had great value in assessing treatment efficacy and predicting prognosis of anti-PD-1 immunotherapy (25, 49, 50). Interestingly, some researchers had found that decreased peripheral lymphocyte counts before treatment was not associated with poorer survival in patients, but persistence of decreased peripheral lymphocyte counts after 12 weeks of immunotherapy might be a poor prognostic marker of patient survival (51). However, the reason for a decreased of peripheral blood lymphocytes during therapy is unclear. Our findings evidenced that dysregulated SUMOylation is an inducing factor of decreased peripheral blood lymphocytes during anti-PD-1 immunotherapy. In in vitro experiments, we found that lightly low SUMOylation level (PBMC was treated with less than 5 μM 2-D08) was associated with the decreased death rates of lymphocyte, but extremely low SUMOylation level (PBMC was treated with greater than 10μM 2-D08) was associated with the increased death rates of lymphocyte. The results indicated that the steady-state level of SUMOylation might be promoting effective therapeutic response of anti-PD-1 immunotherapy by maintaining peripheral blood lymphocyte number, but dysregulated SUMOylation might be detrimental to treatment by promoting peripheral blood lymphocyte death. According to the reports, accumulation of SUMOylated STAT5 and SUMOylated Daxx resulted in growth suppression of lymphocyte (52, 53). Furthermore, in a mouse model of pancreatic ductal adenocarcinoma (PDAC), the SUMOylation inhibitor treated mice demonstrated a decrease in absolute numbers of peripheral lymphocytes. Although the SUMOylation inhibitor efficiently limited tumor growth by inhibiting cancer cell cycle progression and activating interferon signaling in lymphocytes, mouse were only well tolerated during short term treatment (54). We speculate that a strong decrease of peripheral blood lymphocyte induced by the SUMOylation inhibitor may be the main reason for the interruption of later experiments. Remarkably, the number of total lymphocyte was significantly reduced in the highest/lowest percentage change group, but there was no significant difference in the proportion of various lymphocyte subsets among five percentage change groups. This seemed to be related to the similar decreased degree of different lymphocyte subsets induced by dysregulated SUMOylation. In in vitro experiments, we found that SUMOylation inhibitors induced death of different lymphocyte subsets in a dose-dependent manner. Similarly, researchers found that both T and B cell development exhibited severe defects in SUMO specific protease 1 gene knockout mice (52). The above evidence suggested that dysregulated SUMOylation might lead to decrease the number of various lymphocyte subsets in a similar degree. This will result in a decrease in the number of total lymphocytes, but the proportions of various lymphocyte subsets remain unchanged.
Base on the mRNA expression of three SUMO machinery components (SUMO1, SUMO3 and UBE2I), we displayed a predictive method to distinguish the resistance to anti-PD-1 immunotherapy. Among these three genes, UBE2I had the best predictive ability (AUC = 0.733) for the resistance to anti-PD-1 immunotherapy. It may be that UBE2I, the only SUMO conjugating enzyme gene found so far, plays a crucial role in the rate of the SUMOylation cycle (55, 56). ROC curve analysis revealed that the combined expression of SUMO1/SUMO3/UBE2I showed moderate prediction performance for therapeutic responses (AUC = 0.791). Recently, many biomarkers, such as single-cell RNA sequencing or non-targeted metabolomics from tumor samples, and immune infiltration in the tumor microenvironment, had been shown to be potentially useful to predict therapeutic response in lung cancer patients treated with anti-PD-1 immunotherapy (57, 58). However, local analysis of tumor tissue might be severely limited by the amount of available samples, especially since tissue would be consumed by routine molecular analyses (59). Our predictive model, either a single gene or a combination of three genes, can serve as an easily accessible circulating biomarker to evaluate anti-PD-1 treatment response, which could help clinicians to intuitively analyze initial treatment expectation.
In conclusion, our research developed a predictive method, based on the gene expression of three SUMO machinery components (SUMO1, SUMO3 and UBE2I), to distinguish the resistance to anti-PD-1 immunotherapy. Furthermore, our findings implied that the decreased peripheral blood lymphocytes induced by dysregulated SUMOylation might be an inducing factor for the resistance to anti-PD-1 immunotherapy in lung cancer. These data might provide effective circulating biomarkers for predicting the efficacy of anti-PD-1 immunotherapy, and uncovered a novel regulatory mechanism of resistance to anti-PD-1 immunotherapy.
Data availability statement
The raw data supporting the conclusions of this article will be made available by the authors, without undue reservation.
Ethics statement
The studies involving humans were approved by The Human Ethics Committee of the First Hospital of Jilin University. The studies were conducted in accordance with the local legislation and institutional requirements. The participants provided their written informed consent to participate in this study.
Author contributions
YW: Conceptualization, Formal analysis, Funding acquisition, Investigation, Methodology, Writing – original draft. CS: Data curation, Formal analysis, Resources, Writing – review & editing. ML: Methodology, Resources, Visualization, Writing – review & editing. PX: Data curation, Validation, Writing – review & editing. YL: Project administration, Supervision, Writing – review & editing. YZ: Conceptualization, Funding acquisition, Writing – review & editing. JH: Supervision, Writing – review & editing.
Funding
The author(s) declare financial support was received for the research, authorship, and/or publication of this article. This work was supported by The Science and Technology Innovation Platform of Jilin Province (YDZJ202202CXJD050), The 12th Youth Found of The First Hospital of Jilin University (JDYY11202134) and The Training Program for Excellent Young Teacher of Jilin University (419080500583).
Acknowledgments
We would like to thank all participants involved in this study.
Conflict of interest
The authors declare that the research was conducted in the absence of any commercial or financial relationships that could be construed as a potential conflict of interest.
Publisher’s note
All claims expressed in this article are solely those of the authors and do not necessarily represent those of their affiliated organizations, or those of the publisher, the editors and the reviewers. Any product that may be evaluated in this article, or claim that may be made by its manufacturer, is not guaranteed or endorsed by the publisher.
Supplementary material
The Supplementary Material for this article can be found online at: https://www.frontiersin.org/articles/10.3389/fimmu.2024.1424393/full#supplementary-material
References
1. Chen P, Liu Y, Wen Y, Zhou C. Non-small cell lung cancer in China. Cancer Commun (Lond). (2022) 42:937–70. doi: 10.1002/cac2.12359
2. Lahiri A, Maji A, Potdar PD, Singh N, Parikh P, Bisht B, et al. Lung cancer immunotherapy: progress, pitfalls, and promises. Mol Cancer. (2023) 22:40. doi: 10.1186/s12943-023-01740-y
3. Yin J, Wu Y, Yang X, Gan L, Xue J. Checkpoint inhibitor pneumonitis induced by anti-PD-1/PD-L1 therapy in non-small-cell lung cancer: occurrence and mechanism. Front Immunol. (2022) 13:830631. doi: 10.3389/fimmu.2022.830631
4. Marei HE, Hasan A, Pozzoli G, Cenciarelli C. Cancer immunotherapy with immune checkpoint inhibitors (ICIs): potential, mechanisms of resistance, and strategies for reinvigorating T cell responsiveness when resistance is acquired. Cancer Cell Int. (2023) 23:64. doi: 10.1186/s12935-023-02902-0
5. Bai X, Kim M, Kasumova G, Si L, Tang B, Cui C, et al. Radiological dynamics and SITC-defined resistance types of advanced melanoma during anti-PD-1 monotherapy: an independent single-blind observational study on an international cohort. J Immunother Cancer. (2021) 9:e002092. doi: 10.1136/jitc-2020-002092
6. Liu Z, Li X, Gao Y, Liu J, Feng Y, Liu Y, et al. Epigenetic reprogramming of Runx3 reinforces CD8+ T-cell function and improves the clinical response to immunotherapy. Mol Cancer. (2023) 22:84. doi: 10.1186/s12943-023-01768-0
7. Dai M, Liu M, Yang H, Küçük C, You H. New insights into epigenetic regulation of resistance to PD-1/PD-L1 blockade cancer immunotherapy: mechanisms and therapeutic opportunities. Exp Hematol Oncol. (2022) 11:101. doi: 10.1186/s40164-022-00356-0
8. Chen Y. A new immuno-oncology target - SUMOylation. Trends Cancer. (2023) 9:606–8. doi: 10.1016/j.trecan.2023.04.010
9. Gu Y, Fang Y, Wu X, Xu T, Hu T, Xu Y, et al. The emerging roles of SUMOylation in the tumor microenvironment and therapeutic implications. Exp Hematol Oncol. (2023) 12:58. doi: 10.1186/s40164-023-00420-3
10. Chang HM, Yeh E. SUMO: from bench to bedside. Physiol Rev. (2020) 100:1599–619. doi: 10.1152/physrev.00025.2019
11. Tokarz P, Woźniak K. SENP proteases as potential targets for cancer therapy. Cancers (Basel). (2021) 13:2059. doi: 10.3390/cancers13092059
12. ST K, Joshi G, Arya P, Mahajan V, Chaturvedi A, Mishra RK. SUMO and SUMOylation pathway at the forefront of host immune response. Front Cell Dev Biol. (2021) 9:681057. doi: 10.3389/fcell.2021.681057
13. Du L, Liu W, Rosen ST. Targeting SUMOylation in cancer. Curr Opin Oncol. (2021) 33:520–5. doi: 10.1097/CCO.0000000000000765
14. Kunadis E, Lakiotaki E, Korkolopoulou P, Piperi C. Targeting post-translational histone modifying enzymes in glioblastoma. Pharmacol Ther. (2021) 220:107721. doi: 10.1016/j.pharmthera.2020.107721
15. Hung PF, Hong TM, Chang CC, Hung CL, Hsu YL, Chang YL, et al. Hypoxia-induced Slug SUMOylation enhances lung cancer metastasis. J Exp Clin Cancer Res. (2019) 38:5. doi: 10.1186/s13046-018-0996-8
16. Gracia-Hernandez M, Munoz Z, Villagra A. Enhancing therapeutic approaches for melanoma patients targeting epigenetic modifiers. Cancers (Basel). (2021) 13:6180. doi: 10.3390/cancers13246180
17. Ma S, Zhao Y, Lee WC, Ong LT, Lee PL, Jiang Z, et al. Hypoxia induces HIF1α-dependent epigenetic vulnerability in triple negative breast cancer to confer immune effector dysfunction and resistance to anti-PD-1 immunotherapy. Nat Commun. (2022) 13:4118. doi: 10.1038/s41467-022-31764-9
18. Amaro A, Reggiani F, Fenoglio D, Gangemi R, Tosi A, Parodi A, et al. Guadecitabine increases response to combined anti-CTLA-4 and anti-PD-1 treatment in mouse melanoma in vivo by controlling T-cells, myeloid derived suppressor and NK cells. J Exp Clin Cancer Res. (2023) 42:67. doi: 10.1186/s13046-023-02628-x
19. Borst J, Busselaar J, Bosma D, Ossendorp F. Mechanism of action of PD-1 receptor/ligand targeted cancer immunotherapy. Eur J Immunol. (2021) 51:1911–20. doi: 10.1002/eji.202048994
20. Sun C, Nagaoka K, Kobayashi Y, Maejima K, Nakagawa H, Nakajima J, et al. Immunotherapies targeting neoantigens are effective in PD-1 blockade-resistant tumors. Int J Cancer. (2023) 152:1463–75. doi: 10.1002/ijc.34382
21. Simoni Y, Becht E, Fehlings M, Loh CY, Koo SL, Teng KWW, et al. Bystander CD8(+) T cells are abundant and phenotypically distinct in human tumor infiltrates. Nature. (2018) 557:575–9. doi: 10.1038/s41586-018-0130-2
22. Luoma AM, Suo S, Wang Y, Gunasti L, Porter CBM, Nabilsi N, et al. Tissue-resident memory and circulating T cells are early responders to pre-surgical cancer immunotherapy. Cell. (2022) 185:2918–35.e29. doi: 10.1016/j.cell.2022.06.018
23. Liu B, Hu X, Feng K, Gao R, Xue Z, Zhang S, et al. Temporal single-cell tracing reveals clonal revival and expansion of precursor exhausted T cells during anti-PD-1 therapy in lung cancer. Nat Cancer. (2022) 3:108–21. doi: 10.1038/s43018-021-00292-8
24. Du X, Wen S, Shi R, Xia J, Wang R, Zhang Y, et al. Peripheral blood lymphocytes differentiation patterns in responses/outcomes to immune checkpoint blockade therapies in non-small cell lung cancer: a retrospective study. BMC Cancer. (2023) 23:83. doi: 10.1186/s12885-023-10502-4
25. Kamphorst AO, Pillai RN, Yang S, Nasti TH, Akondy RS, Wieland A, et al. Proliferation of PD-1+ CD8 T cells in peripheral blood after PD-1-targeted therapy in lung cancer patients. Proc Natl Acad Sci U.S.A. (2017) 114:4993–8. doi: 10.1073/pnas.1705327114
26. Brahmer JR, Govindan R, Anders RA, Antonia SJ, Sagorsky S, Davies MJ, et al. The Society for Immunotherapy of Cancer consensus statement on immunotherapy for the treatment of non-small cell lung cancer (NSCLC). J Immunother Cancer. (2018) 6:75. doi: 10.1186/s40425-018-0382-2
27. Wolchok JD, Hoos A, O’Day S, Weber JS, Hamid O, Lebbé C, et al. Guidelines for the evaluation of immune therapy activity in solid tumors: immune-related response criteria. Clin Cancer Res. (2009) 15:7412–20. doi: 10.1158/1078-0432.CCR-09-1624
28. Seymour L, Bogaerts J, Perrone A, Ford R, Schwartz LH, Mandrekar S, et al. iRECIST: guidelines for response criteria for use in trials testing immunotherapeutics. Lancet Oncol. (2017) 18:e143–52. doi: 10.1016/S1470-2045(17)30074-8
29. Fowler NH, Davis RE, Rawal S, Nastoupil L, Hagemeister FB, McLaughlin P, et al. Safety, activity, and immune effects of lenalidomide and rituximab in untreated indolent lymphoma. Lancet Oncol. (2014) 15:1311–8. doi: 10.1016/S1470-2045(14)70455-3
30. Boland JL, Zhou Q, Iasonos AE, O’Cearbhaill RE, Konner J, Callahan M, et al. Utility of serum CA-125 monitoring in patients with ovarian cancer undergoing immune checkpoint inhibitor therapy. Gynecol Oncol. (2020) 158:303–8. doi: 10.1016/j.ygyno.2020.04.710
31. Lin YJ, Kang YM, Wu YH, Chen YW, Hu YW. Lymphocytopenia and survival after whole-brain radiotherapy in patients with small-cell lung cancer. Thorac Cancer. (2023) 14:1268–75. doi: 10.1111/1759-7714.14868
32. Yood RA, Ottery FD, Irish W, Wolfson M. Effect of pegloticase on renal function in patients with chronic kidney disease: a post hoc subgroup analysis of 2 randomized, placebo-controlled, phase 3 clinical trials. BMC Res Notes. (2014) 7:54. doi: 10.1186/1756-0500-7-54
33. Middleton PG, Mall MA, Dřevínek P, Lands LC, McKone EF, Polineni D, et al. Elexacaftor-tezacaftor-ivacaftor for cystic fibrosis with a single phe508del allele. N Engl J Med. (2019) 381:1809–19. doi: 10.1056/NEJMoa1908639
34. Banerji A, Bernstein JA, Johnston DT, Lumry WR, Magerl M, Maurer M, et al. Long-term prevention of hereditary angioedema attacks with lanadelumab: The HELP OLE Study. Allergy. (2022) 77:979–90. doi: 10.1111/all.15011
35. Wu W, Huang C. SUMOylation and DeSUMOylation: Prospective therapeutic targets in cancer. Life Sci. (2023) 332:122085. doi: 10.1016/j.lfs.2023.122085
36. Yu L, Lin N, Ye Y, Zhuang H, Zou S, Song Y, et al. The prognosis, chemotherapy and immunotherapy efficacy of the SUMOylation pathway signature and the role of UBA2 in lung adenocarcinoma. Aging (Albany NY). (2024) 16:4378–95. doi: 10.18632/aging.205594
37. Liu X, Xu Y, Pang Z, Guo F, Qin Q, Yin T, et al. Knockdown of SUMO-activating enzyme subunit 2 (SAE2) suppresses cancer Malignancy and enhances chemotherapy sensitivity in small cell lung cancer. J Hematol Oncol. (2015) 8:67. doi: 10.1186/s13045-015-0164-y
38. Liu K, Zhang J, Wang H. Small ubiquitin-like modifier/sentrin-specific peptidase 1 associates with chemotherapy and is a risk factor for poor prognosis of non-small cell lung cancer. J Clin Lab Anal. (2018) 32:e22611. doi: 10.1002/jcla.22611
39. Mu J, Zuo Y, Yang W, Chen Z, Liu Z, Tu J, et al. Over-expression of small ubiquitin-like modifier proteases 1 predicts chemo-sensitivity and poor survival in non-small cell lung cancer. Chin Med J (Engl). (2014) 127:4060–5. doi: 10.3760/cma.j.issn.0366-6999.20141013
40. Han JY, Lee GK, Yoo SY, Yoon SJ, Cho EY, Kim HT, et al. Association of SUMO1 and UBC9 genotypes with tumor response in non-small-cell lung cancer treated with irinotecan-based chemotherapy. Pharmacogenomics J. (2010) 10:86–93. doi: 10.1038/tpj.2009.46
41. Yuan H, Lu Y, Chan YT, Zhang C, Wang N, Feng Y. The role of protein SUMOylation in human hepatocellular carcinoma: A potential target of new drug discovery and development. Cancers (Basel). (2021) 13:5700. doi: 10.3390/cancers13225700
42. Zhao Q, Ma Y, Li Z, Zhang K, Zheng M, Zhang S. The function of SUMOylation and its role in the development of cancer cells under stress conditions: A systematic review. Stem Cells Int. (2020) 2020:8835714. doi: 10.1155/2020/8835714
43. Borsci G, Barbieri S, Guardamagna I, Lonati L, Ottolenghi A, Ivaldi GB, et al. Immunophenotyping reveals no significant perturbation to PBMC subsets when co-cultured with colorectal adenocarcinoma caco-2 cells exposed to X-rays. Front Immunol. (2020) 11:1077. doi: 10.3389/fimmu.2020.01077
44. Wei SC, Levine JH, Cogdill AP, Zhao Y, Anang NAS, Andrews MC, et al. Distinct cellular mechanisms underlie anti-CTLA-4 and anti-PD-1 checkpoint blockade. Cell. (2017) 170:1120–33.e17. doi: 10.1016/j.cell.2017.07.024
45. Sun F, Wang FX, Zhu H, Yue TT, Yang CL, Luo JH, et al. SUMOylation of PDPK1 Is required to maintain glycolysis-dependent CD4 T-cell homeostasis. Cell Death Dis. (2022) 13:181. doi: 10.1038/s41419-022-04622-1
46. Huang CH, Yang TT, Lin KI. Mechanisms and functions of SUMOylation in health and disease: a review focusing on immune cells. J BioMed Sci. (2024) 31:16. doi: 10.1186/s12929-024-01003-y
47. Ding X, Wang A, Ma X, Demarque M, Jin W, Xin H, et al. Protein SUMOylation is required for regulatory T cell expansion and function. Cell Rep. (2016) 16:1055–66. doi: 10.1016/j.celrep.2016.06.056
48. Garaude J, Farrás R, Bossis G, Charni S, Piechaczyk M, Hipskind RA, et al. SUMOylation regulates the transcriptional activity of JunB in T lymphocytes. J Immunol. (2008) 180:5983–90. doi: 10.4049/jimmunol.180.9.5983
49. Kagamu H, Kitano S, Yamaguchi O, Yoshimura K, Horimoto K, Kitazawa M, et al. CD4(+) T-cell immunity in the peripheral blood correlates with response to anti-PD-1 therapy. Cancer Immunol Res. (2020) 8:334–44. doi: 10.1158/2326-6066.CIR-19-0574
50. Lao J, Xu H, Liang Z, Luo C, Shu L, Xie Y, et al. Peripheral changes in T cells predict efficacy of anti-PD-1 immunotherapy in non-small cell lung cancer. Immunobiology. (2023) 228:152391. doi: 10.1016/j.imbio.2023.152391
51. Chen Y, Wen S, Xia J, Du X, Wu Y, Pan B, et al. Association of dynamic changes in peripheral blood indexes with response to PD-1 inhibitor-based combination therapy and survival among patients with advanced non-small cell lung cancer. Front Immunol. (2021) 12:672271. doi: 10.3389/fimmu.2021.672271
52. Muromoto R, Ishida M, Sugiyama K, Sekine Y, Oritani K, Shimoda K, et al. Sumoylation of Daxx regulates IFN-induced growth suppression of B lymphocytes and the hormone receptor-mediated transactivation. J Immunol. (2006) 177:1160–70. doi: 10.4049/jimmunol.177.2.1160
53. Van Nguyen T, Angkasekwinai P, Dou H, Lin FM, Lu LS, Cheng J, et al. SUMO-specific protease 1 is critical for early lymphoid development through regulation of STAT5 activation. Mol Cell. (2012) 45:210–21. doi: 10.1016/j.molcel.2011.12.026
54. Kumar S, Schoonderwoerd M, Kroonen JS, de Graaf IJ, Sluijter M, Ruano D, et al. Targeting pancreatic cancer by TAK-981: a SUMOylation inhibitor that activates the immune system and blocks cancer cell cycle progression in a preclinical model. Gut. (2022) 71:2266–83. doi: 10.1136/gutjnl-2021-324834
55. Huang J, Tan X, Liu Y, Jiang K, Luo J. Knockdown of UBE2I inhibits tumorigenesis and enhances chemosensitivity of cholangiocarcinoma via modulating p27kip1 nuclear export. Mol Carcinog. (2023) 62:700–15. doi: 10.1002/mc.23518
56. Han ZJ, Feng YH, Gu BH, Li YM, Chen H. The post-translational modification, SUMOylation, and cancer (Review). Int J Oncol. (2018) 52:1081–94. doi: 10.3892/ijo.2018.4280
57. Hu J, Zhang L, Xia H, Yan Y, Zhu X, Sun F, et al. Tumor microenvironment remodeling after neoadjuvant immunotherapy in non-small cell lung cancer revealed by single-cell RNA sequencing. Genome Med. (2023) 15:14. doi: 10.1186/s13073-023-01164-9
58. Ricciuti B, Lamberti G, Puchala SR, Mahadevan NR, Lin JR, Alessi JV, et al. Genomic and immunophenotypic landscape of acquired resistance to PD-(L)1 blockade in non-small-cell lung cancer. J Clin Oncol. (2024) 42:1311–21. doi: 10.1200/JCO.23.00580
Keywords: PD-1, resistance, Sumoylation, lymphocyte, peripheral blood
Citation: Wang Y, Sun C, Liu M, Xu P, Li Y, Zhang Y and Huang J (2024) Dysregulated gene expression of SUMO machinery components induces the resistance to anti-PD-1 immunotherapy in lung cancer by upregulating the death of peripheral blood lymphocytes. Front. Immunol. 15:1424393. doi: 10.3389/fimmu.2024.1424393
Received: 28 April 2024; Accepted: 30 July 2024;
Published: 15 August 2024.
Edited by:
Raquel Tarazona, University of Extremadura, SpainReviewed by:
Katarina Mirjacic Martinovic, Institute of Oncology and Radiology of Serbia, SerbiaDanay Saavedra, Center of Molecular Immunology, Cuba
Copyright © 2024 Wang, Sun, Liu, Xu, Li, Zhang and Huang. This is an open-access article distributed under the terms of the Creative Commons Attribution License (CC BY). The use, distribution or reproduction in other forums is permitted, provided the original author(s) and the copyright owner(s) are credited and that the original publication in this journal is cited, in accordance with accepted academic practice. No use, distribution or reproduction is permitted which does not comply with these terms.
*Correspondence: Yongsheng Zhang, emhhbmd5czE5ODVAamx1LmVkdS5jbg==; Jing Huang, aHVhbmdqQGpsdS5lZHUuY24=