- 1Wenzhou Collaborative Innovation Center of Gastrointestinal Cancer in Basic Research and Precision Medicine, Wenzhou Key Laboratory of Cancer-related Pathogens and Immunity, Department of Microbiology and Immunology, Institute of Molecular Virology and Immunology, Institute of Tropical Medicine, School of Basic Medical Sciences, Wenzhou Medical University, Wenzhou, China
- 2Department of Gynecologic Oncology, Women’s Hospital, School of Medicine, Zhejiang University, Hangzhou, China
- 3First Clinical College, Wenzhou Medical University, Wenzhou, China
- 4Department of Gynecologic Oncology, Wenzhou Central Hospital, Wenzhou, China
- 5Department of Obstetrics and Gynecology, The First Affiliated Hospital, Wenzhou Medical University, Wenzhou, China
- 6Department of Obstetrics and Gynecology, The Second Affiliated Hospital and Yuying Children’s Hospital of Wenzhou Medical University, Wenzhou, China
Serous ovarian carcinoma (SOC) is a gynecological malignancy with high mortality rates. Currently, there is a lack of reliable biomarkers for accurate SOC patient prognosis. Here, we analyzed SOC RNA-Seq data from The Cancer Genome Atlas (TCGA) to identify prognostic biomarkers. Through the pearson correlation analysis, univariate Cox regression analysis, and LASSO-penalized Cox regression analysis, we identified nine lncRNAs significantly associated with four types of RNA modification writers (m6A, m1A, APA, and A-I) and with the prognosis of SOC patients (P <0.05). Six writer-related lncRNAs were ultimately selected following multivariate Cox analysis. We established a risk prediction model based on these six lncRNAs and evaluated its prognostic value in multiple groups (training set, testing set, and entire set). Our risk prediction model could effectively predict the prognosis of SOC patients with different clinical characteristics and their responses to immunotherapy. Lastly, we validated the predictive reliability and sensitivity of the lncRNA-based model via a nomogram. This study explored the association between RNA modification writer-related lncRNAs and SOC prognosis, providing a potential complement for the clinical management of SOC patients.
Introduction
Ovarian cancer (OC) is among the deadliest gynecological malignancies. In 2020, there were more than 313,000 new cases of OC globally, in addition to more than 207,000 deaths, and these numbers continue to rise (1, 2). Serous ovarian carcinoma (SOC) accounts for approximately 75% of the OC cases, representing the most common histological OC subtype (3). Approximately 70% of OC patients already have advanced-stage disease at the time of diagnosis, and a large proportion experience disease relapse due to the lack of effective screening tools for early diagnosis (4). Although treatment methods have improved recently, the prognosis remains far from optimal (5). Due to the limitations of available SOC treatment, there is an urgent need for the identification of sensitive prognostic markers and the introduction of new predictive models for treatment response to guide personalized therapy.
RNA modification is a key epigenetic process that regulates post-transcriptional gene expression (6), with more than 170 types of post-transcriptional RNA modifications identified at present, namely, N6-methyladenosine (m6A), N1-methyladenosine (m1A), alternative polyadenylation (APA), adenosine-to-inosine (A-I), and others (7). Adenine is the most heavily modified nucleotide in RNA (8, 9). Currently, research on adenine modifications is mainly focused on m6A, m1A, APA, and A-I. At present, known m6A writers include methyltransferase-like protein 3/14 (METTL3/14), Wilms’ tumor-associated protein (WTAP), RNA-binding motif protein 15/15B (RBM15/15B), zinc finger CCCH-Type containing 13 (ZC3H13), and KIAA1429 (VIRMA, vir-like m6A methyltransferase associated) (10); m1A writers include tRNA methyltransferase 6/61A/61B/10C (TRMT6/61A/61B/10C) (11); APA writers include cleavage and polyadenylation specificity factor 1–4 (CPSF1–4), cleavage stimulation factor 1–3 (CSTF 1–3), cleavage factor I (CFI), PCF11 (protein 1 of CFI), cleavage factor polyribonucleotide kinase subunit 1 (CLP1), and nuclear poly(A)-binding protein 1 (PABPN1) (12); A-I writers include adenosine deaminases acting on RNA (ADARs, such as ADAR, ADARB1, and ADARB2) (13). Multiple studies have shown that these four RNA modifications and their respective writer enzymes play an important role in the incidence and development of various cancer types, including SOC (8, 10). Through the analysis of 11,552 samples derived from 39 tissue and cell types, Ali et al. discovered that changes in the mitochondrial RNA N1-methyladenosine and N1-methylguanine (m1A/G) modification levels affected mutations in nuclear DNA, thereby promoting the progression of breast cancer (14). Bi et al. found that METTL3 mediated the maturation of microRNA-126-5p through m6A modification, resulting in miRNA binding to phosphatase and tensin homolog and, thereby, activating the P13K/Akt/mTOR pathway, which in turn promoted OC incidence and progression (15). Loss of CPSF1 suppressed OC cell viability, induced cell cycle arrest in the G0/G1 phase and promoted cellular apoptosis (16). Amin et al. found that ADAR upregulation is an independent predictor of lung adenocarcinoma relapse and that ADAR increases FAK expression by catalyzing the A-I modification on RNA, thus promoting the migration and invasion of lung adenocarcinoma cells (17). Taken together, the dysregulation of multiple types of RNA modifications may contribute to the development of cancer. Additionally, interactions have been reported between different modifications. Xiang et al. showed that m6A modifications could suppress the binding of A-I writer ADAR to RNA, downregulating of A-I modification levels in methylated transcripts (18). Dai et al. used an unbiased quantitative proteomic method and confirmed that m6A reader YTH domain-containing family 2 can bind to m1A with low affinity, accelerating the degradation of m1A-modified transcripts (19), thus suggesting functional crosstalk between m6A and m1A modifications. Molinie et al. found that the distribution of m6A modification on transcripts may be related to that of APA modification sites (20). Taken together, these findings indicate that different types of adenine modifications, particularly m6A, m1A, APA, and A-I, may have complicated regulatory networks (9). There is growing evidence that RNA modification writers play an essential role in inflammation and innate immunity by interacting with various writers (9). Chen et al. revealed crosstalk among m6A, m1A, APA, and A-I writers in colorectal cancer and demonstrated their potential therapeutic value in colorectal cancer (9). However, no studies have explored the combined effects of m6A, m1A, APA, and A-I modifications on the pathogenesis and treatment response of SOC. Hence, we focused our research on the writer enzymes of these four RNA modifications (m6A, m1A, APA, and A-I).
Long non-coding RNAs (lncRNAs) are transcripts with a length of more than 200 nucleotides that have no or only limited protein-coding ability and influence cancer progression through their interaction with DNA, protein, or RNA, to regulate signal transduction (21, 22). Multiple studies have shown that m6A writers are involved in the regulation of the biological functions of lncRNAs (22). For instance, Xue et al. found that METTL3 enhanced the stability of the lncRNA ABHD11-AS1 by catalyzing its m6A modification, thus promoting the proliferation of non-small cell lung cancer (23). With respect to other adenine RNA modification types (such as m1A and A-I), few studies have explored the roles of their writer enzymes in lncRNA regulation. Most available research only used sequencing technology and bioinformatic analysis to preliminarily explore the distribution of these modifications on lncRNAs in cancer cells (24–26). Interestingly, studies have also shown that lncRNA could influence the function of RNA modifications. For example, Zhu et al. found that the RNA-binding regulatory peptide encoded by the lncRNA LINC00266-1 is the regulatory subunit of insulin-like growth factor 2 mRNA-binding protein 1 (IGF2BP1). Further, this regulatory subunit regulated the recognition of m6A RNA by IGF2BP1 and mediated the stabilization of c-Myc and other mRNA transcripts, thereby promoting tumor incidence and development (27). There are still relatively few studies on lncRNAs related to RNA modification writers in SOC. A comprehensive understanding of the effects of writer-related lncRNAs on the prognosis and immune response in SOC will help us better understand the SOC tumor microenvironment and thus guide immunotherapy strategies.
Previous studies have validated lncRNAs related to RNA modification writers in multiple cancers such as breast cancer (28), bladder cancer (29), and lung adenocarcinoma (30), but not in SOC. Here, we screened for lncRNAs related to RNA modification writers based on the transcriptomic data of SOC patients obtained from The Cancer Genome Atlas (TCGA) database, with the aim to identify prognostic lncRNA biomarkers. We obtained six lncRNAs related to the four types of RNA modification writers, which were significantly associated with the prognosis of SOC. Subsequently, we established a prognostic risk score model (m6A/m1A/A-I/APA-LPR) based on these six lncRNAs and validated its prognostic accuracy for SOC. Finally, we explored the correlation between our risk model and the tumor microenvironment as well as immunotherapy response. The current study provides potential biomarkers for SOC prognosis and management.
Materials and Methods
Gene Expression Profiles and Clinical Data of Patients With SOC
RNA sequencing and mutation data of patients with SOC (N = 375) from the TCGA database were downloaded using “TCGAbiolinks” (R package), and the corresponding clinical information was downloaded from the GDC database (https://cancergenome.nih.gov/). SOC patients with missing survival information were excluded. Patients were randomly separated into two cohorts at a 4:6 ratio, named the training set and the testing set, respectively, for the establishment and validation of the risk model. The total TCGA patient dataset is referred to as the “entire set”.
The RNA modification writers consisted of seven m6A modification enzymes (METTL3, METTL14, WTAP, RBM15, RBM15B, ZC3H13, and KIAA1429), four m1A modification enzymes (TRMT61A, TRMT61B, TRMT10C, and TRMT6), 12 APA modification enzymes (CPSF1-4, CSTF1/2/3, PCF11, CFI, CLP1, NUDT21, and PABPN1), and three A-I modification enzymes (ADAR, ADARB1, and ADARB2). The expression profiles for lncRNA, mRNA, and adenosine RNA modification writer genes were separately acquired for subsequent analyses.
Correlation Analysis
We screened four types of RNA modification writer-related lncRNAs via pearson correlation analysis in entire set using the “rcorr” function from “Hmisc” (R package), with the criteria of |Pearson R| >0.3 and P <0.001 (30).
Reverse Transcription Quantitative Polymerase Chain Reaction of m6A/m1A/A-I/APA-LPR Model-Associated lncRNAs
The human OC cell lines CAOV3, OVCAR3, and SKOV3 were purchased from American Type Cell Culture (ATCC, Manassas, VT, USA), and A2780 was purchased from Sigma-Aldrich (Cat#93112519, St Louis, Missouri, USA). Cisplatin-resistant cell lines (SKOV3-CIS and A2780-CIS) were established in our lab. The normal ovarian epithelial cell line IOSE-80 was purchased from MeisenCTCC (Zhejiang Meisen Cell Technology Co., Ltd., Hangzhou, China). All cell lines were cultured in Dulbecco’s modified Eagle’s medium (Gibco, Thermo Fisher Scientific Inc., Thermo Fisher Scientific Inc., Waltham, MA, USA) containing 10% fetal bovine serum at 37°C with 5% CO2. For RNA purification, the isolated cells were lysed in TRIzol reagent (Invitrogen Life Technologies, Grand Island, NY, USA). The extracted RNA was further digested using DNase I (Invitrogen, Waltham, MA, USA) to remove residual DNA and subsequently separated from each sample using TRIzol reagent/RNeasy Mini kit (Qiagen, Hilden, Germany). The total extracted RNA was stored at −80°C for future use.
The lncRNA expression levels in both the OC cell lines and normal ovarian epithelial cell lines were measured by performing a reverse transcription quantitative polymerase chain reaction (qRT-PCR) using an Applied Biosystems QuantStudioTM 6 real-time PCR instrument (Thermo Fisher Scientific Inc., Waltham, MA, USA). All qRT-PCR experiments were performed using the QuantiNova SYBR Green PCR kit (Qiagen, Hilden, Germany). For each reaction, 1 µl of diluted cDNA was mixed with 18.2 µl of 1× SYBR Green PCR Master Mix. A final volume of 20 µl was achieved by adding 0.4 µl each of the forward and reverse primers (10 µmol). The conditions for PCR amplification were as follows: 95°C for 5 min, followed by 40 cycles each of 95°C for 10 s and 60°C for 30 s. All samples were tested in triplicate. The data were analyzed using the comparative threshold cycle (Ct) method. GAPDH was used as the control, and the relative quantification of lncRNAs in cells was calculated using the following equation: amount of target = 2−ΔCt, where ΔCt = CtlncRNA − CtGAPDH. The gene-specific primers for lncRNA and GAPDH used for qRT-PCR are listed in Supplementary Table 1.
m6A/m1A/A-I/APA-LPR Model Construction and Validation
As previously reported (30, 31), the training set was used to construct a writer-related lncRNA model, and the lncRNAs were selected based on univariate Cox regression and LASSO Cox (10-fold cross-validation) analyses using “survival” and “glmnet” (R packages) and visualized via “ROCR,” “survminer,” “ComplexHeatmap,” and “ggplot2” (R packages). The risk score was calculated as the sum of the prognostic coefficients multiplied by the expression profiles of writer-related lncRNAs. Six writer-related lncRNAs (AC142528.1, PCAT29, RP11-508M8.1, MYCNOS, RP11-327F22.2, and RP11-665C16.5) were identified for establishing the risk model. The following formula was used to calculate the risk score: m6A/m1A/A-I/APA score = h0(t) × [0.010174 × expression(RP11-508M8.1) + 0.003821 × expression(RP11-665C16.5) − 0.136630 × expression(AC142528.1) − 0.081020 × expression(MYCNOS) − 0.028803 × expression(PCAT29) − 0.016343 × expression(RP11-327F22.2)], where h0(t) is the baseline risk of m6A/m1A/A-I/APA score when all variables are 0, as per a previous report (30). Patients were divided into low- and high-risk groups based on their risk scores in each cohort (training, testing, and entire sets). The latter two sets were used to validate the prognostic value of our established model, with the median risk score obtained in the training set used as the cut-off value.
Principal Component Analysis
Principal component analysis (PCA) was used for reducing the effective dimensionality, identifying the model, grouping via the “prcomp” function in R, and visualized using “scatterplot3d” (R package).
Mutation Analysis
The mutation profile was analyzed and visualized using “maftools” (R package).
Functional and Pathway Enrichment Analyses and Exploration of the Risk Model for Immunotherapy Response Prediction
The immune scores of SOC patients were downloaded from the ESTIMATE database (Estimation of STromal and Immune cells in MAlignant Tumor tissues using Expression data; https://bioinformatics.mdanderson.org/estimate/). Immune-related gene sets used for GESA in this study were downloaded from the MSigDB database (https://www.gsea-msigdb.org/gsea/index.jsp), including “IMMUNE RESPONSE.gmt,” “29immunesets.gmt,” “h.all.v7.4.symbols.gmt,” “c2.cp.kegg.v7.4.symbols.gmt,” and analyzed using “GSVA” (R package). We used the TIDE algorithm (http://tide.dfci.harvard.edu) to predict the likelihood of an immunotherapeutic response. Therapeutic responses to various drugs were predicted using “oncoPredict” (R package). LncRNA-related drugs were predicted using the LncMAP database (http://bio-bigdata.hrbmu.edu.cn/LncMAP/) and visualized via Cytoscape (version 3.9.0, http://www.cytoscape.org/).
Nomogram Construction and Evaluation
Nomogram and calibration curves were constructed and visualized using the “survival” and “rms” (R packages). Receiver operating characteristic (ROC) curves were analyzed and visualized using “ROCR,” “pROC,” and “timeROC” (R packages).
Statistical Analysis
Continuous variables were analyzed using Student’s t-tests or non-parametric Wilcoxon tests. Prognostic analyses were performed via Kaplan–Meier and Cox regression analyses using “survminer” and “survival” (R packages). R 4.0.1 (http://www.r-project.org/) was used to analyze all data. The results with P <0.05 were considered statistically significant.
Results
Identification of lncRNAs Related to RNA Modification Writers in Patients With SOC
We have summarized the process of biomarker identification in a flowchart (Supplementary Figure 1). We obtained the full transcriptome data of 375 SOC patients from the TCGA database. We identified 15,900 lncRNAs and 26 writer genes (wirters) (Figure 1A). We screened 2,460 writer-related lncRNAs through pearson correlation analysis (|R| >0.3 and P <0.001, Supplementary Table 2).
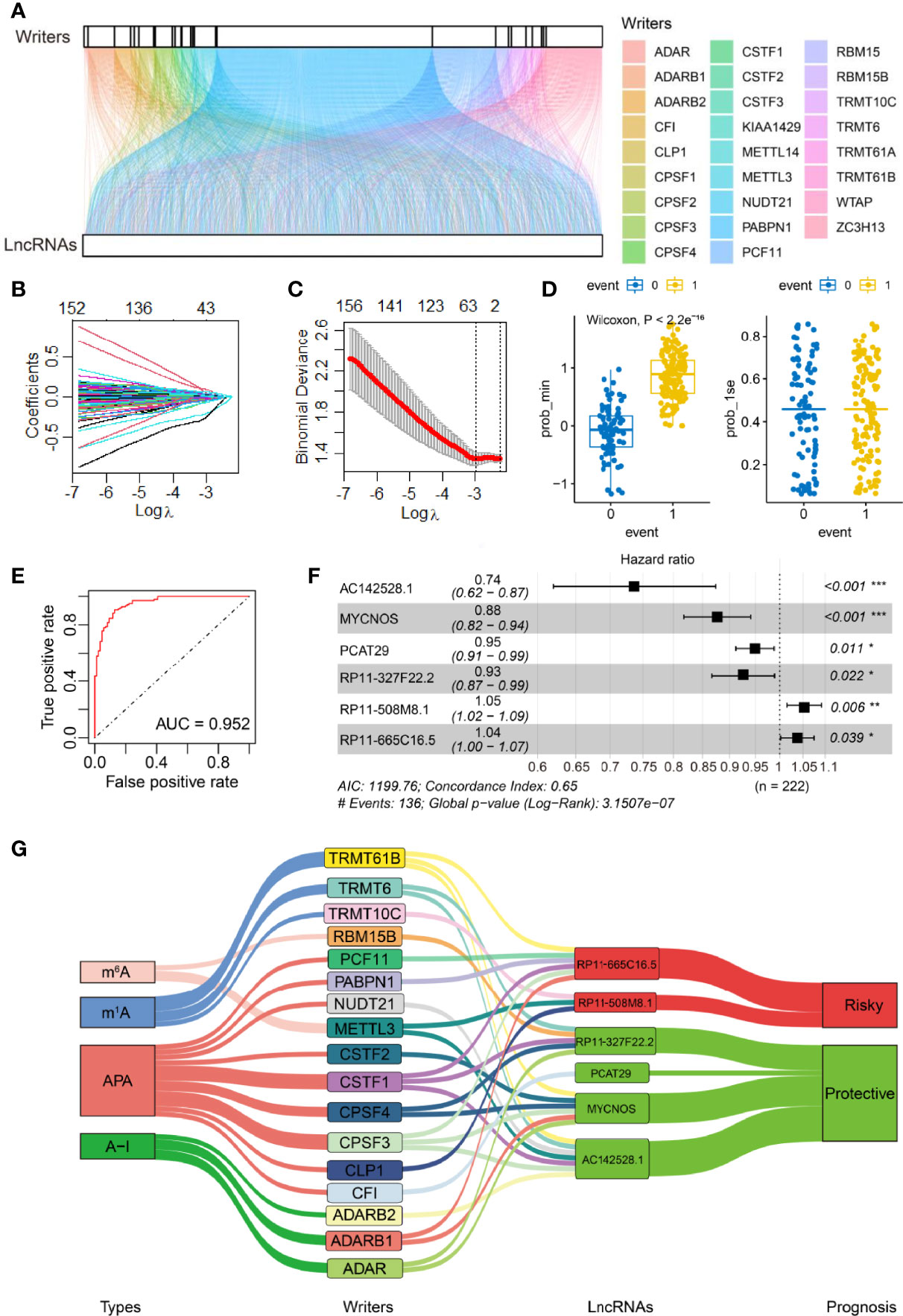
Figure 1 Identification of RNA adenosine modification writer-related lncRNAs and establishment of the lncRNA-based risk model. (A) Alluvial diagram for 26 writer genes and writer-related lncRNAs. (B) The LASSO coefficient profile of OS-related lncRNAs was drawn via 10-fold cross-validation. (C) The tuning parameters (log λ) of OS-related proteins were selected to cross-verify the error curve. Of the two dotted lines in the figure, the left is λ Min, and the right is λ 1se. λ Min is the value of λ that gives the minimum mean cross-validated error, whereas the other λ saved is λ 1se, which gives the most regularized model such that error is within one standard error of the minimum. (D) Self-prediction based on the minimal criterion and 1se criterion (0 and 1 represent the states where events are predicted to occur and not to occur, respectively, according to the model). (E) ROC curves of the model via internal validation. (F) Multivariate Cox regression analysis yielded six independent prognostic lncRNAs. PR11-508M8.1 and PR11-665C16.5 were risk factors, and the other four lncRNAs were protective factors for SOC. *P <0.05, **P <0.01, ***P <0.001. (G) Relational Sankey diagram for significant correlations between 17 writer genes and six prognostic writer-related lncRNAs.
Establishment of a Risk Model Based on lncRNAs Related to RNA Modification Writers in SOC Patients
First, 163 writer-related lncRNAs were significantly correlated with OC survival based on Cox univariate analysis in our training set (P <0.05, Supplementary Table 3). We then performed LASSO Cox analysis to further narrow down prognosis-related lncRNAs. The coefficients of candidate lncRNAs were obtained (Figure 1B), and 40 writer-related lncRNAs were selected via the λ minimization method (Figure 1C). Concurrently, we carried out a model self-rating, which indicated that the lncRNA-based risk model could easily differentiate between patients based on survival status (Figure 1D). An ROC analysis was performed to evaluate the prognostic value of candidate writer-related lncRNAs. The area under the ROC curve was 0.952, which suggested that these lncRNAs could effectively predict prognosis (Figure 1E). Next, six writer-related lncRNAs were obtained via multivariate Cox analysis (Figure 1F). The expression of these six lncRNAs was visualized in this study and checked in the TANRIC database (32) (Supplementary Figures 2A, B). Based on four lncRNA-databases, namely, Lnc2Cancer 3.0 (33), LncCAR (34), Immlnc (35), and LncMAP (36), we also found that these lncRNAs were expressed in OC. Additionally, we performed qRT-PCR to detect and validate the expression of the six lncRNAs in six OC cell lines and one ovarian epithelial cell line (Supplementary Figure 2C).
These lncRNAs were independently correlated with OC survival (Figure 1F). We established a prognostic risk model based on the expression profiles and the regression coefficients of these lncRNAs in the training set, and the C-index of our risk model was 0.646 (se = 0.024) (Figure 1F). We also visualized the significant association between lncRNAs and the 17 associated writers (out of the above mentioned 26 writers). We found that 2/17 (m6A), 3/17 (m1A), 9/17 (APA), and 3/17 (A-I) writers were significantly associated with these candidate lncRNAs (Figure 1G). Of the six candidate lncRNAs, AC142528.1, MYCNOS, PCAT29, and RP11-327F22.2 were protective factors in SOC (hazard ratio (HR) <1), while PR11-508M8.1 and PR11-665C16.5 were risk factors (HR >1) (Figure 1G; Supplementary Figure 3).
Risk scores were calculated for the training set, and patients were then grouped into low- and high-risk groups with the median risk score (0.99681) as a cutoff value. The risk score distribution, survival time, survival status, and expression level of the six writer-related lncRNAs for each patient in the training set are shown in Supplementary Figure 3. Survival analysis indicated that the overall survival (OS) of the patients in the low-risk group was greater than that of the patients in the high-risk group (P <0.0001, Figure 2A).
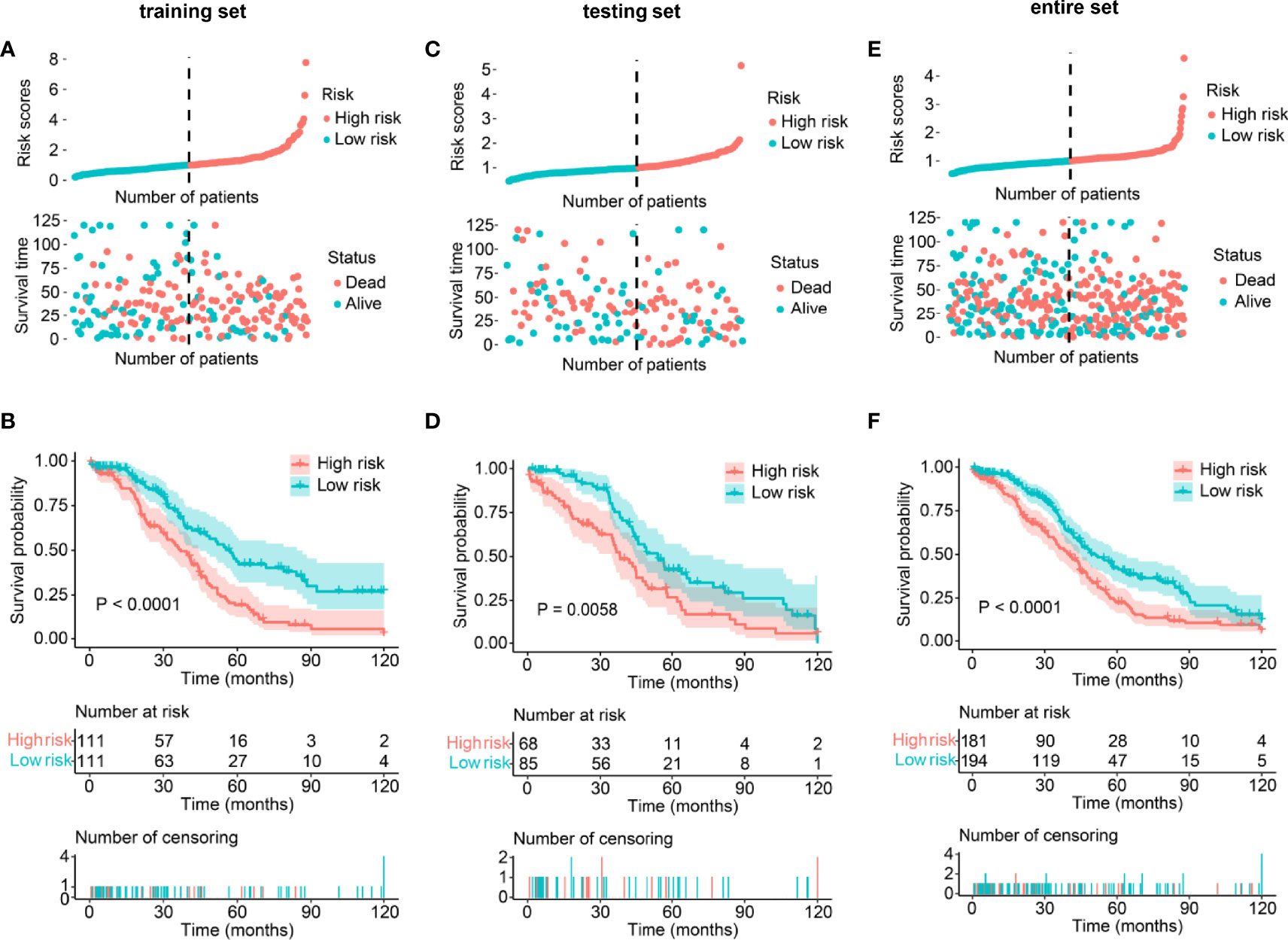
Figure 2 Validation of the lncRNA-based prognostic risk model in the training set, testing set, and entire set. (A, C, E) Distribution of risk score and survival status between low/high-risk SOC patients in the training set (A), testing set (C), and entire set (E). The blue color represents patients with a low risk score, and the red color represents patients with a high risk score. Distribution of risk score based on the writer-related lncRNA model (Upper panel). Survival status and survival time between the high- and low-risk subgroups (Lower panel). (B, D, F) Kaplan–Meier survival analysis between low- and high-risk subgroups of patients in the training set (B), testing set (D), and entire set (F).
Validation of Our Risk Model in SOC Patients
To validate the prognostic value of the above-established risk model, risk scores were calculated for every patient in the testing and entire sets. Patients were again divided into low- and high-risk groups. The distribution of risk scores, survival status, and survival time was visualized (Figure 2). As expected, Kaplan–Meier survival analysis also suggested that patients with a high-risk score had a worse OS than those with low-risk scores (Ptesting set = 0.0058, Pentire set <0.0001, Figures 2B, C). The above results indicated that the risk model could be used to predict SOC prognosis accurately.
Additionally, we stratified low- and high-risk patients in the entire set according to their clinicopathological features and analyzed the differences in OS. In the subgroups classified by age and tumor grade, the OS of low-risk patients was significantly longer than that of high-risk patients (Figure 3). Moreover, although there was no statistically significant difference, we found discrepancies in the OS between low- and high-risk SOC patients with FIGO stage IV or with tumors (Figure 3).
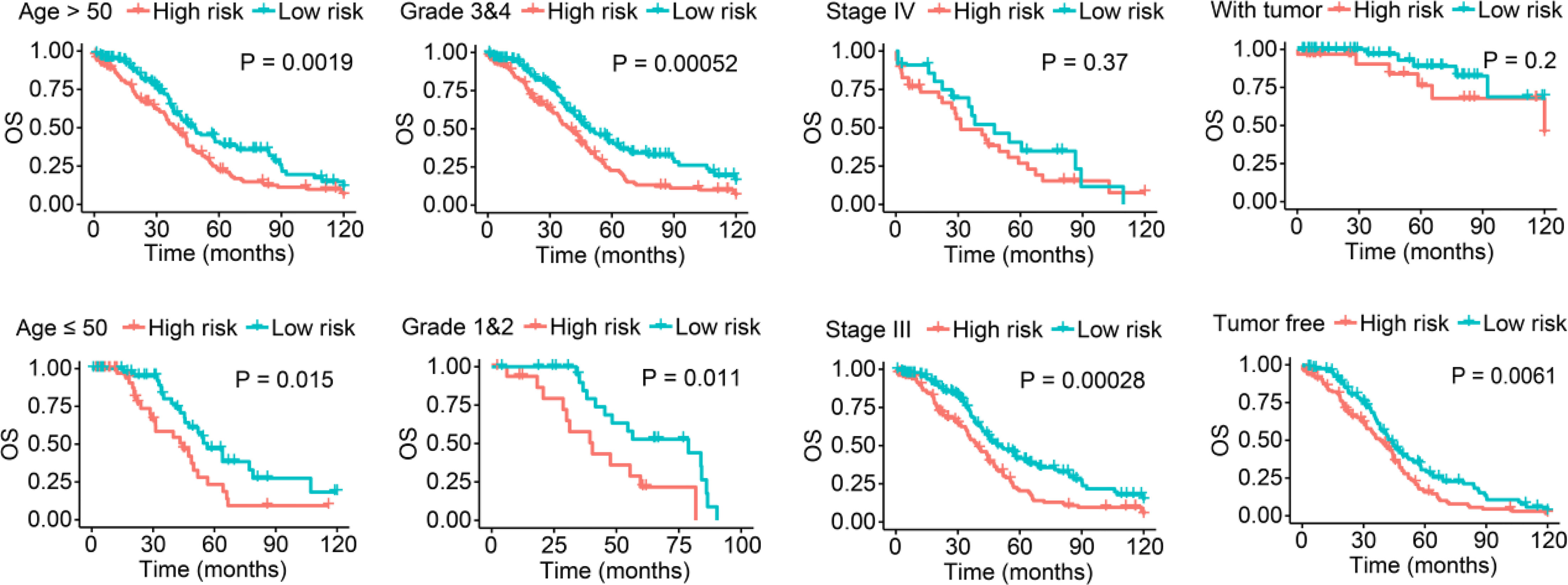
Figure 3 Survival analysis stratified by age, tumor grade, FIGO stage, and tumor status between the low- and high-risk groups in the entire set.
Principal Component Analysis Further Verified the Prognostic Value of our m6A/m1A/A-I/APA-LPR Model
PCA was performed to evaluate the ability of our risk model to discriminate between low- and high-risk patients based on gene expression profiles of 1) all RNA-seq data (Figure 4A); 2) coding genes (Figure 4B); 3) 26 writer genes (Figure 4C); 4) six writer-related lncRNAs (Figure 4D); and 5) risk model classified by the expression profiles of the six writer-related lncRNAs (Figure 4E). The gene expression profiles of the six writer-related lncRNAs could effectively distinguish patients (Figure 4D), especially for the risk model (Figure 4E). However, we did not obtain similar results based on other data (Figures 4A–C). These findings suggest that the model established based on writer-related lncRNAs could be a potential prognostic signature.
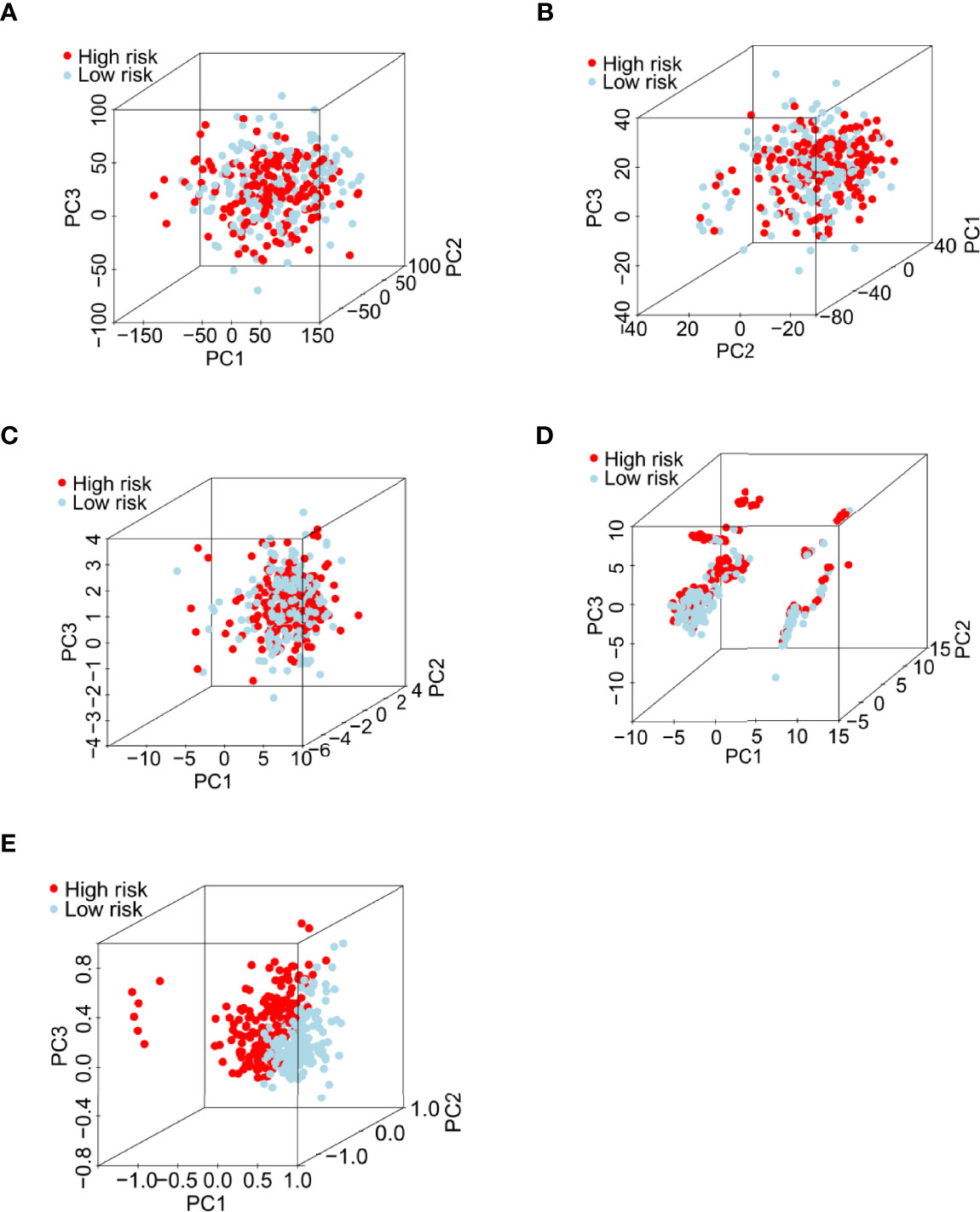
Figure 4 Principal component analysis between the low- and high-risk groups in the entire set. (A) All RNA-seq data from the TCGA database. (B) Expression profiles of all coding genes. (C) Expression profiles of 26 writer-related genes. (D) Expression profiles of six writer-related lncRNAs. (E) Risk model based on the profiles of the six writer-related lncRNAs.
The Prognostic Value of m6A/m1A/A-I/APA-LPR Was Greater Than That of TP53 Mutation Status
We visualized the top 20 most frequently mutated genes in the low- and high-risk patient groups, and our results indicated that TP53 had the highest mutation frequency in both groups (low-risk: 92%; high-risk: 89%; Figures 5A, B). TP53 mutations are present in various human cancers (pancreatic adenocarcinoma, liver hepatocellular carcinoma, chromophobe renal cell carcinoma, acute myeloid leukemia, thymoma, etc.) and represent potential prognostic markers (37). Thus, we explored whether the m6A/m1A/A-I/APA-LPR model could predict OS better than TP53 mutation status. Surprisingly, the survival results of high-/low-risk patients with TP53 mutation were similar to those of high-/low-risk patients with wild-type TP53, indicating that the TP53 mutation status failed to prognostically distinguish SOC patients. Interestingly, the low-risk patients had an apparently longer OS than those with high-risk scores, regardless of TP53 mutation status (Figure 5C). These results indicated that our risk model was a better predictor of SOC prognosis than TP53 mutation status.
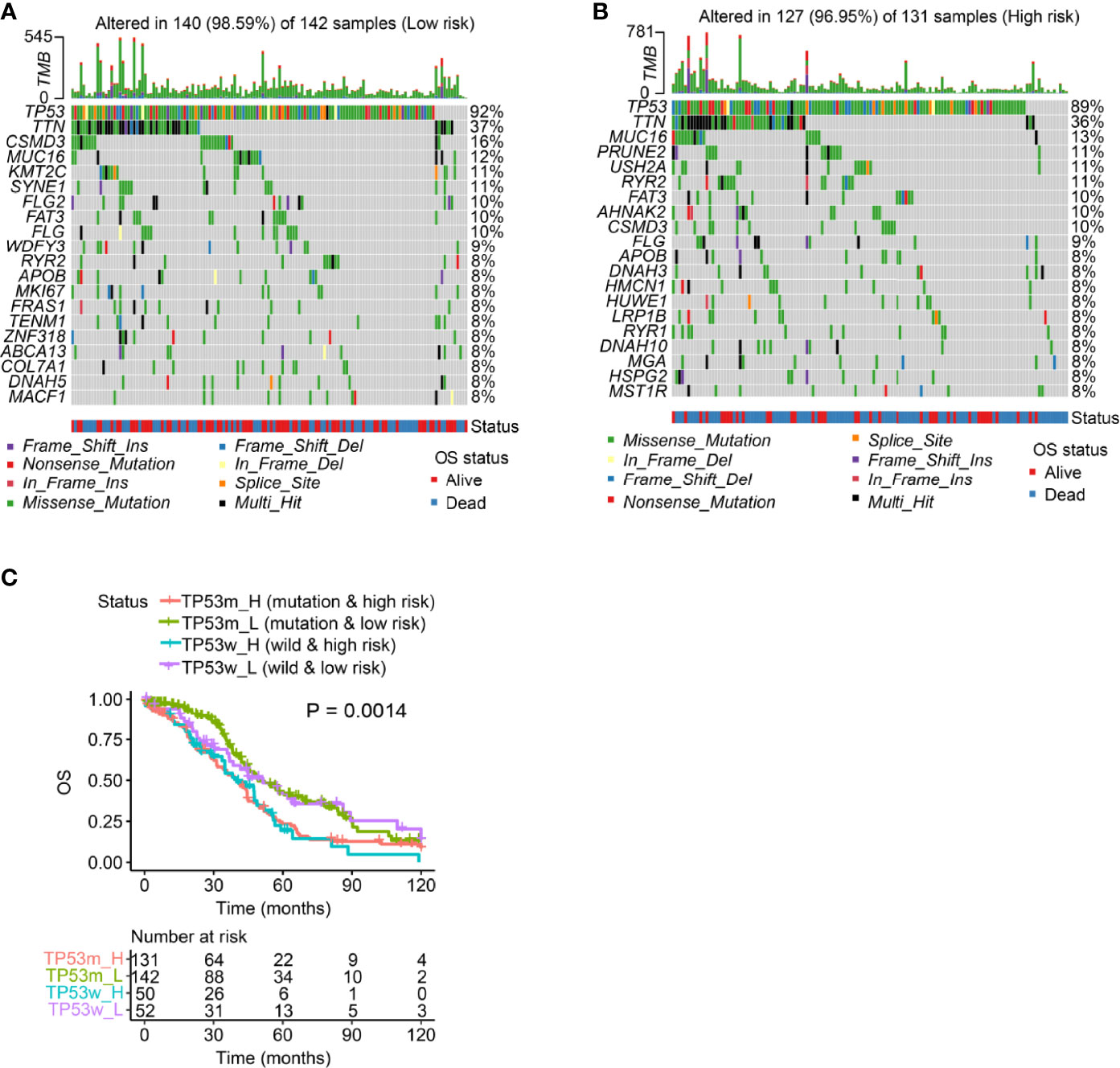
Figure 5 Mutation analysis using the risk model in the entire set. (A, B) Waterfall plot displays mutation information of the genes with high mutation frequencies in the patients with low-risk scores (A) and those with high-risk scores (B). (C) Overall survival analysis of patients classified according to the m6A/m1A/A-I/APA-LPR score and TP53 mutation status in the entire set.
Stratification Analysis of the m6A/m1A/A-I/APA-LPR Model With Regard to Tumor Immune Microenvironment and Cancer Immunotherapy Response
We performed subsequent analyses (Figure 6A) to explore differences in tumor immune microenvironment between low- and high-risk patients. As expected, SOC patients with high-risk scores had higher immune and stromal cell scores than low-risk patients did. Furthermore, the tumor purity of high-risk patients was higher (Figure 6B). High-risk patients exhibited high expression of immune factors (such as CCR and APC co-inhibition) and tumor-infiltrating immune cells (such as interdigitating dendritic cells, macrophages, mast cells, and neutrophils) (Figure 6C). We then analyzed the difference in immune responses between low- and high-risk SOC patients, with the latter having higher immune response scores (Figure 6D). To explore the molecular mechanisms underlying SOC progression, we performed hallmark gene signature and Kyoto Encyclopedia of Genes and Genomes (KEGG) pathway enrichment analyses, which revealed significant discrepancies in various immune-related biological processes between the low- and high-risk groups. For example, the high-risk group had higher scores for IL2-STAT5 signaling, IL6-JAK-STAT3 signaling, and B-cell receptor signaling than the low-risk group (Supplementary Figures 4A, B). Along with the above-described results, we explored the correlation between the risk model and immunotherapy response. As expected, we found that low-risk patients were more likely to respond to immunotherapy than high-risk ones, indicating that this risk model based on immune indexes (i.e., cluster of differentiation 274/programmed cell death ligand 1 (CD274/PD-L1) carcinoma-associated fibroblasts (CAFs)) might serve as an indicator for predicting tumor immune dysfunction and exclusion (TIDE), excluding tumor-associated macrophages (Figure 6E).
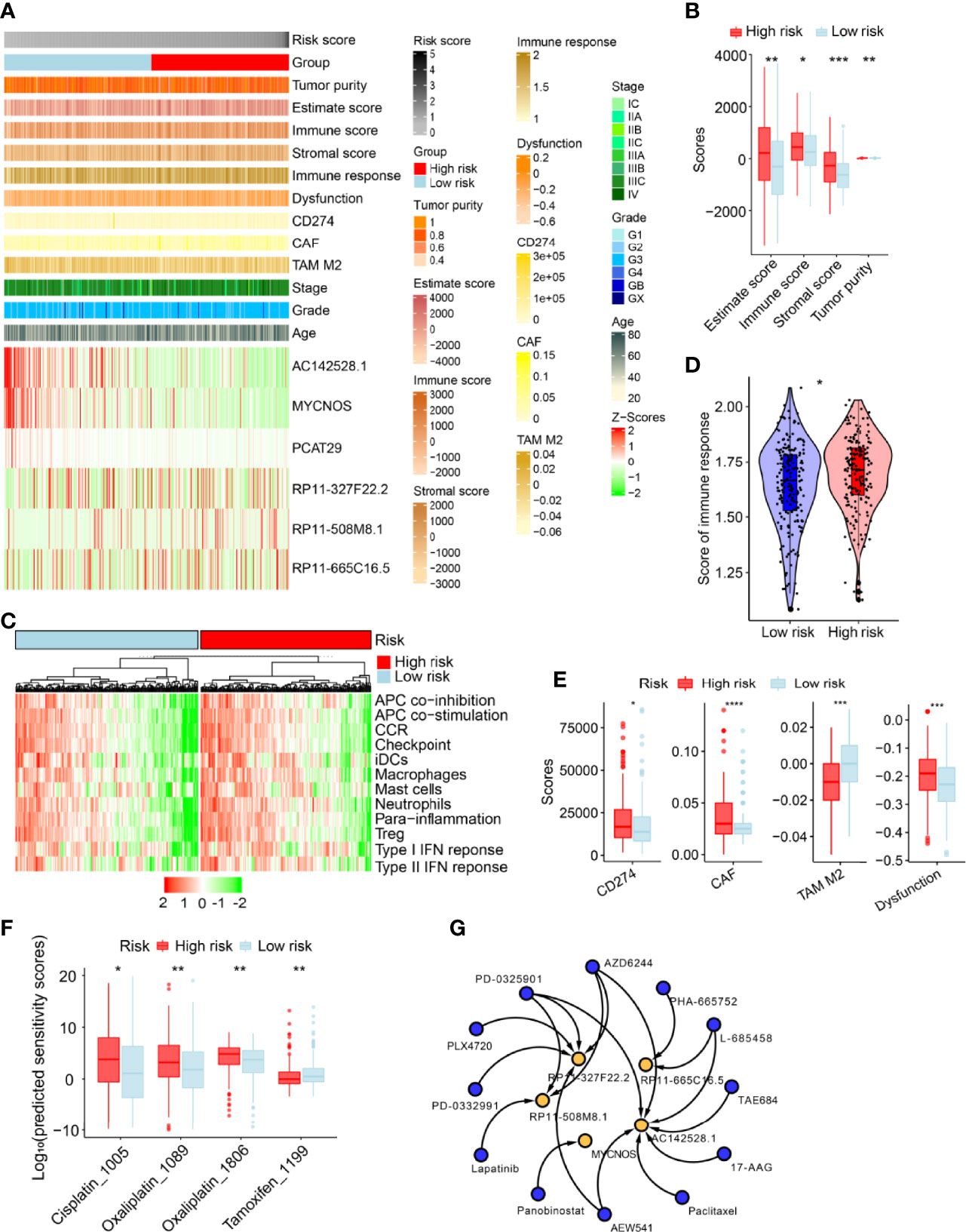
Figure 6 Estimation of immune-related factors using the risk model in the entire set. (A) Heatmap of associations between the expression levels of the six m6A/m1A/A-I/APA-related lncRNAs and clinicopathological features. (B) The differences in stromal and immune cell scores between low- and high-risk patients were analyzed. (C) The indicated standards of the immunity index for each patient were visualized via heatmaps, with red representing high expression, and green representing relatively low expression. (D) The differences in immune response between low- and high-risk SOC patients. (E) Estimation of cancer immunotherapy response. (F) Differences in sensitivity against clinical applied drugs. (G) Twelve potential drugs (blue) were screened based on interactions of the RNA adenosine modification writer-related lncRNAs (yellow) in the drug–lncRNA module of LncMAP database. Only statistically significant results are shown (P <0.05). *P <0.01, **P <0.05, ***P <0.001, ****P <0.0001.
Identification of Novel Potential Drugs for the Treatment of Patients With High m6A/m1A/A-I/APA-LPR Risk Scores
We further evaluated the therapeutic response for every patient in the entire set based on the half-maximal inhibitory concentration (IC50) of various drugs available in the Genomics of Drug Sensitivity in Cancer (GDSC) database. Therapeutic score prediction analysis revealed that 35 drugs had significantly different efficacy between the two groups (Supplementary Table 4). As expected, low-risk SOC patients were more sensitive to Cisplatin_1005 and Oxalipatin_1089/1086, but not to Tamoxifen_1199 (Figure 6F). We performed lncRNA–drug prediction analysis, as described in the Materials and Methods section. Predicted were 120 paired lncRNA-drug interactions, which included the five lncRNAs (AC142528.1, MYCNOS, RP11-327F22.2, PR11-508M8.1, and PR11-665C16.5) and 24 drugs (Supplementary Table 5). We screened and constructed a network of 18 lncRNA–drug pairs (P <0.05) out of the 120 lncRNA–drug pair interactions (Figure 6G).
Evaluation of the lncRNA-Based Prognostic Risk Score Model Together With Clinical Features in SOC Patients
Combining the risk score, FIGO stage, grade, and age of patients, we conducted univariate and multivariate Cox regression analyses to evaluate prognostic value in SOC patients. Only the risk score was an independent factor for OS (Supplementary Figure 5A, P <0.001). In univariate cox regression analysis, the risk score had an HR and a 95% confidence interval (CI) of 1.57 and 1.25–1.97, respectively. In multivariate cox regression analysis, the HR was 1.54, and the 95% CI was 1.22–1.94. These results highlighted our risk model as the only independent prognostic factor in SOC patients (Supplementary Figure 5A). The area under the ROC curve (AUC) was assessed, with the risk score model showing a larger AUC than other clinicopathological characteristics (AUCRisk model = 0.638, AUCFIGOstage = 0.566, AUCGrade = 0.499, AUCAge = 0.561; Supplementary Figure 5B). The m6A/m1A/A-I/APA-LPR risk model also performed well at differentiating follow-up time, and its concordance index was larger than that of other clinical factors over time (Supplementary Figures 5C, D). These results indicated that the prognostic capacity of m6A/m1A/A-I/APA-LPR in SOC patients was robust.
Establishment and Evaluation of a Prognostic Risk Score-Based Nomogram
To further evaluate the potential of our risk model in predicting SOC patient outcomes, we established a risk score-based nomogram. More specifically, the nomogram included clinical characteristics and the risk model. We then used it to predict the 1-, 2-, and 3-year OS. In comparison with clinical characteristics alone, the nomogram exhibited greater predictive ability (Figure 7A). Moreover, calibration analysis revealed a coherence between the prediction curves of the risk model and the actual 1-, 2-, and 3-year survival curves (Figures 7B–D), further highlighting the prognostic accuracy of the nomogram.
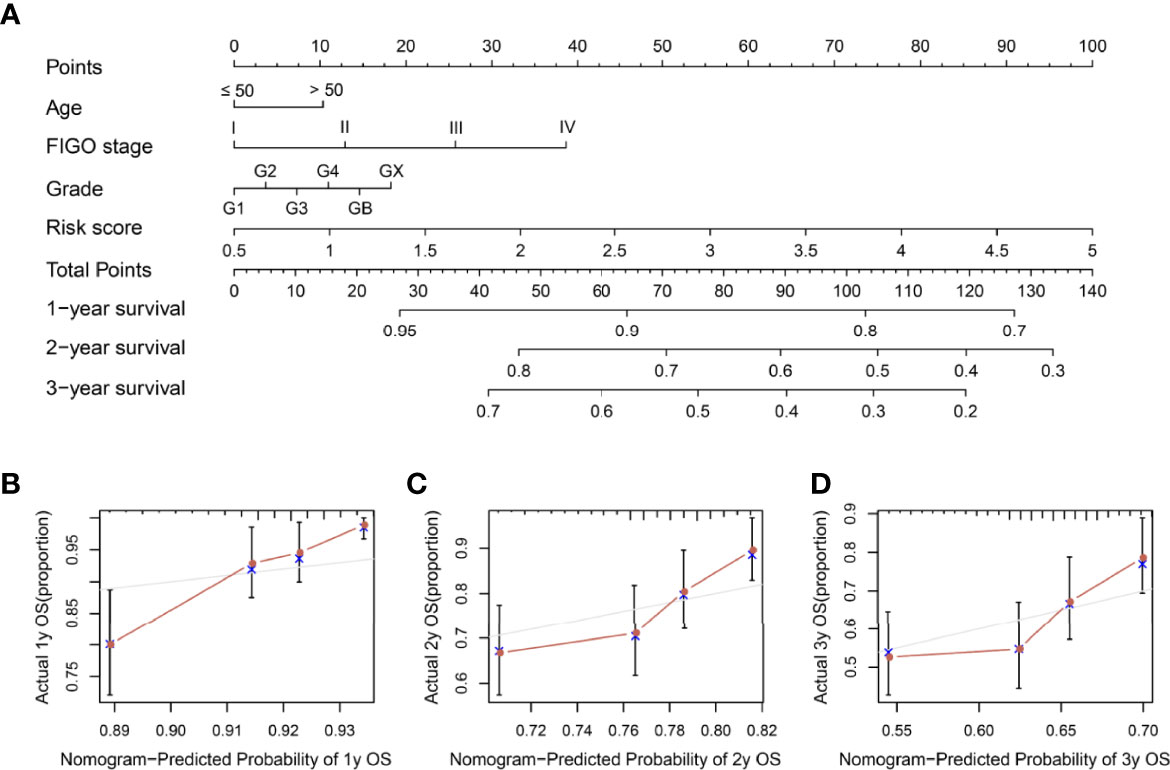
Figure 7 Nomogram construction and visualization. (A) A nomogram constructed using risk score and clinical characteristics in SOC patients within 1-, 2-, and 3-year OS data. (B–D) Calibration plots of actual and predicted 1-, 2-, and 3-year OS in the entire set.
Discussion
Studies have shown that the interaction of different writers mediates abnormal RNA modifications, which promote tumor proliferation, migration, and invasion, as well as immune regulation (8, 38). Through their regulatory effects on gene expression and signaling pathways, lncRNAs influence tumor progression and even contribute to treatment resistance in various tumors, including OC (39, 40). Numerous studies have explored the significance of RNA modifications, especially the association between m6A and lncRNA, in different tumors. METTL3 mediates the m6A modification of the lncRNA THAP7-AS1, enhancing its expression and thereby, promoting the interaction between its nuclear localization signal and importin α1. This allows the CUL4B protein to enter the nucleus and inhibit miR-22-3p and miR-320a transcription, thus promoting gastric tumorigenesis (41). The stability of lncRNA RMRP is enhanced through m6A modification, regulating the TGFBR1/SMAD2/SMAD3 pathway and the proliferation and progression of non-small cell lung cancer (42). While these studies highlight the role of RNA modification writer-related lncRNAs in human cancers, the study of these lncRNAs is still in its infancy (43–45). We believe that exploring the interactions between lncRNAs and RNA modification writers will lead to the identification of new prognostic markers or therapeutic targets for malignant tumors.
Through bioinformatics analysis of SOC RNA-Seq data from the TCGA database, we obtained six RNA modification writer-related lncRNAs (AC142528.1, MYCNOS, PCAT29, PR11-327F22.2, PR11-508M8.1, and PR11-665C16.5) that were significantly related to the prognosis of SOC (Supplementary Figure 5A and Figure 1F). Based on the expression profiles of these lncRNAs and their regression coefficients, we established the m6A/m1A/A-I/APA-LPR prognostic model. Among the six lncRNAs, MYCNOS promotes tumorigenesis in various cancers. It is upregulated in glioblastoma where it might promote tumor cell proliferation via the MYCNOS/miR-216B/FOXM1 axis (46). Additionally, MYCNOS was closely related to the poor prognosis of hepatocellular carcinoma based on bioinformatics analysis (47). Although available research on MYCNOS is still limited, its biological function in SOC is yet to be explored, considering that some lncRNAs play opposite roles in different cancer types, as previously described for metastasis-associated lung adenocarcinoma transcript 1 (MALAT1) (48). Various studies have shown that MALAT1 exerts tumor-promoting effects in several cancers, including non-small cell lung cancer, osteosarcoma, cervical cancer, and pancreatic cancer (49). However, MALAT1 was downregulated in glioma and endometrioid endometrial carcinoma, where it exerted tumor-suppressive effects (50, 51). Recently, MALAT1 was reported to bind and inactivate TEAD (TEA/ATTS domain), inhibiting breast cancer metastasis in transgenic, xenograft, and syngeneic mouse models (52). Interestingly, previous bioinformatics analysis studies suggested that MALAT1 was associated with a poor prognosis of breast cancer (53, 54). These findings highlight the complexity of lncRNA involvement in different cancers. Our group established a model for predicting SOC prognosis and immunotherapy response based on m6A effector-related lncRNAs (unpublished data). Similarly, we identified MYCNOS as a protective factor in SOC, with a potentially important role in its incidence and development. Nevertheless, whether MYCNOS exerts a tumor-suppressive or tumor-promoting effect in SOC remains to be further investigated. PCAT29 acts as a tumor suppressor and downregulates the proliferation and migration of prostate cancer cells (55). Moreover, Bao et al. found that PCAT29 was expressed in OC and the positive rate of PCAT29 was 82/116; they also identified PCAT29 as a signature associated with prognosis in pan-cancer (including OC) (56). PR11-508M8.1 was proposed as a biomarker for predicting the risk of papillary thyroid carcinoma relapse (57). Data regarding the cancer-related functions of the remaining three lncRNAs in our model, namely, AC142528.1, PR11-327F22.2, and PR11-665C16.5, are scarce. Validation in our training set (n = 153) and the entire set (n = 375) confirmed the prognostic value of the lncRNA-based model in SOC. To further explore the significance of our model with respect to the tumor microenvironment, we analyzed the differences in the expression of CD274/PD-L1 as well as the infiltration of CAFs and tumor-associated macrophages (TAMs) in high-risk and low-risk patient groups. The low-risk patient group had lower CD274/PD-L1 and CAF scores than the high-risk group, while the TAM score was greater than in the high-risk group. Research has shown that various cancers use the PD-L1 and programmed cell death-1 (PD-1) immune checkpoints to evade T cell immunity, and blocking their interaction has significant anti-tumor effects in patients with advanced cancer (58). Furthermore, the combination of PARP inhibitors with anti-PD-1/PD-L1 drugs was reported to have a synergistic anti-OC activity (59). CAFs are activated by various cytokines, which promote tumorigenesis, accelerate tumor invasion and metastasis, induce angiogenesis, and promote drug resistance (60). Thus, CAFs are therapeutic targets, and research has indicated that the miR-630/KLF6/NF-kB signaling pathway in CAFs may be targeted for treating OC (61). Previous studies have shown that TAMs release anti-inflammatory mediators and angiogenic factors, which suppress anti-tumor immune responses and promote tumor growth (62, 63). However, our findings were not in line with this notion. TAMs are considered M2-like macrophages that exert a tumor-promoting effect. They were recently shown to be in a state of constant transition between M1 and M2 polarization states (64). M1 macrophages participate in the anti-tumor immune response during the early stages of cancer development, whereas M2 macrophages suppress adaptive immunity in advanced tumors, thereby promoting tumorigenesis (64). The proportion of various macrophage phenotypes in the TAM population is regulated by various signaling factors within the tumor microenvironment (65, 66). However, the detailed mechanisms of M1–M2 dynamic transitions remain unclear, necessitating further research into the specific role of TAMs in SOC. Gene set enrichment analysis (GSEA) and KEGG pathway enrichment analysis yielded immune-related molecular mechanisms potentially implicated in SOC. The IL2-STAT5 signaling pathway was previously reported to be involved in the inhibition of T cell proliferation in OC (67). The combined use of an IL6-JAK-STAT3 signaling pathway inhibitor and paclitaxel reduced OC stem cell viability and suppressed tumor growth (68). Further, it has been shown that B-cell receptor signaling plays an important role in the pathogenesis and development of chronic lymphocytic leukemia (69). In-depth exploration of these pathways in SOC will help identify biomarkers and drug targets.
We also investigated the differences in drug sensitivity between the high-risk and low-risk groups, with the results showing that patients in the latter group were more sensitive to cisplatin and oxaliplatin (Figure 6F). In contrast, high-risk group patients tended to be more sensitive to tamoxifen. Cisplatin is currently used as a first-line chemotherapy drug for SOC. Unfortunately, with the increase in cisplatin chemotherapy cycles, the risk of platinum resistance or allergies also increases (70). Oxaliplatin is a third-generation platinum derivative that is mainly used alone or along with other platinum drugs for treating SOC relapse. Only partial cross-resistance is observed between it and cisplatin, and thus combination therapy can reduce chemotherapy resistance (71). The estrogen receptor (ER) is upregulated in many patients with OC and is a potential target for endocrine therapy. Tamoxifen is a selective ER modulator that is well-tolerated and has low toxicity (72, 73). Many long-term studies have proven its efficacy for SOC. However, it is still debatable whether tamoxifen can be used as the first-line therapy for treating SOC (73). In this study, we identified potential drugs for treating high-risk patients based on the m6A/m1A/A-I/APA-LPR model by using the LncMAP database. Among 18 drugs, we found that PD-0325901 and AZD6244 had the most interactions with RNA modification writer-related lncRNAs. Clinical studies have shown the efficacy of both drugs for treating SOC (74, 75). Finally, we established a nomogram involving our model and validated its predictive potential for SOC patient prognosis.
A recent study established a risk model based on four lncRNAs that are involved in m6A regulation (AC010894.3, ACAP2-IT1, CACNA1G-AS1, and UBA6-AS1). The model successfully predicted the OS and treatment response in OC patients (45). We checked the expression of those lncRNAs using our data (Supplementary Figure 6A) and performed the relationship of those lncRNAs with RNA modification enzymes (Supplementary Figure 6B). Similar to a previous study (45), we found that lncRNAs were related to many RNA-modification enzymes. Additionally, we found that ACAP2-IT1 had a significantly positive correlation with RBM15, consistent with previous research (45). Interestingly, we discovered that AC010894.3 was associated with ADARB1 (A-I writer) in addition to m6A (METTL5). Moreover, these four lncRNAs were also correlated with different types of RNA modification writers (Supplementary Figure 6B), which indicated that lncRNAs may be regulated by multi-RNA modification and the biological functions of lncRNAs may be the result of cross-talk of various RNA modification enzymes. This further suggests that more modification types and related modification enzymes should be included in future studies to determine the relationship between RNA modification and lncRNA regulation more comprehensively. Considering that the interactions of multiple RNA modifications are involved in the incidence and development of SOC (76, 77), we established a risk model based on lncRNAs related to writers of four RNA modification types (m6A, m1A, APA, and A-I). Therefore, we believe that our model is more reliable and accurate. Nevertheless, the current study has certain limitations. First, the number of SOC samples in TCGA data was not enough, necessitating the use of more datasets to validate the prognostic value of our m6A/m1A/A-I/APA-LPR model. Second, some of the selected lncRNAs have not yet been explored in the context of cancer, warranting research into their biological function in SOC. Third, several other types of RNA modifications exist, and their effector proteins are not just writers. It is becoming increasingly evident that cross-talk exists among different modification types. Thus, analyzing the incorporation of more RNA modifications, such as m5C and m7G, will further reveal the regulatory role of different RNA modifications in genes. In a future study, we will further explore the crosstalk of other RNA modification types (such as m5C and m7G) and other effector (readers and erasers) in SOC. In summary, we established a lncRNA-based risk model that could accurately predict the prognosis of SOC patients and analyzed its association with the tumor microenvironment of SOC. The m6A/m1A/A-I/APA-LPR model might be a promising prognostic tool for guiding the personalized treatment of SOC.
Data Availability Statement
The datasets presented in this study can be found in online repositories. The names of the repositories can be found in the article/Supplementary Material.
Author Contributions
GG and XX conceived, designed, and supervised the study. LY performed bioinformatic analyses. LY, KP, SuF, and GG wrote the manuscript. GG and XX revised the manuscript. SW, SC, ST, NW, HZ, XT, XS, ShF, DX, RZ, and YH, collected the data. All authors listed have made a substantial, direct, and intellectual contribution to the work and approved it for publication.
Funding
This work was supported by grants from the Natural Science Foundation of Zhejiang (Grant No: LQ20C060003), the Health Commission of Zhejiang (Grant No: 2019KY458), and the Wenzhou Public Welfare Science and Technology Project (Grant No: Y20170013).
Conflict of Interest
The authors declare that the research was conducted in the absence of any commercial or financial relationships that could be construed as a potential conflict of interest.
Publisher’s Note
All claims expressed in this article are solely those of the authors and do not necessarily represent those of their affiliated organizations, or those of the publisher, the editors and the reviewers. Any product that may be evaluated in this article, or claim that may be made by its manufacturer, is not guaranteed or endorsed by the publisher.
Supplementary Material
The Supplementary Material for this article can be found online at: https://www.frontiersin.org/articles/10.3389/fimmu.2022.863484/full#supplementary-material
Supplementary Figure 1 | Flowchart of this study.
Supplementary Figure 2 | Expression of m6A/m1A/A-I/APA-LPR model-associated lncRNAs in SOC. (A) The expression level (fragments per kilobase of exon model per million mapped fragments, FPKM) of six lncRNAs in this study were showed based on our data. (B) The expression level of these six lncRNAs were further checked in the TANRIC database (an open-access webapp for interactive exploration of lncRNAs in cancer) based on the normalization data of reads per kilobase per million mapped reads (RPKM). (C) qRT-PCR was conducted on RNA samples from six OC cell lines (A2780, A2780-CIS, SKOV3, SKOV3-CIS, CAOV3, and OVCAR3) and one ovarian epithelial cell line (IOSE-80).
Supplementary Figure 3 | Kaplan–Meier survival analysis. Survival analysis of SOC patients grouped based on the expression of RNA modification writer-related lncRNAs in the training set.
Supplementary Figure 4 | Difference in hallmark gene signatures, KEGG pathway enrichment, and the tumor immune microenvironment between low- and high-risk patient groups in the entire set. (A) GSEA. (B) KEGG pathway enrichment analysis.
Supplementary Figure 5 | Assessment of the writer-related lncRNA model and clinical features in the entire set. (A) Univariate and multivariate Cox regression analyses of the clinical characteristics and risk model with regard to OS. (B) ROC curves of the model and clinical characteristics. (C) Time-dependent ROC curves to evaluate the predictive accuracy of the m6A/m1A/A-I/APA-LPR score and other clinicopathological parameters for 1-, 2- and 3-year OS of SOC patients in the entire set. (D) Concordance indexes of model and clinical characteristics.
Supplementary Figure 6 | The correlation between four lncRNAs from a previous study and RNA modification writers of m6A, m1A, APA, and A-I. (A) The expression level (FPKM) of four lncRNAs based on our data. (B) Correlation analysis of these four lncRNAs with writers of m6A, m1A, APA, and A-I.
References
1. Sung H, Ferlay J, Siegel RL, Laversanne M, Soerjomataram I, Jemal A, et al. Global Cancer Statistics 2020: Globocan Estimates of Incidence and Mortality Worldwide for 36 Cancers in 185 Countries. CA Cancer J Clin (2021) 71(3):209–49. doi: 10.3322/caac.21660
2. Bray F, Ferlay J, Soerjomataram I, Siegel RL, Torre LA, Jemal A. Global Cancer Statistics 2018: Globocan Estimates of Incidence and Mortality Worldwide for 36 Cancers in 185 Countries. CA Cancer J Clin (2018) 68(6):394–424. doi: 10.3322/caac.21492
3. Matulonis UA, Sood AK, Fallowfield L, Howitt BE, Sehouli J, Karlan BY. Ovarian Cancer. Nat Rev Dis Primers (2016) 2:16061. doi: 10.1038/nrdp.2016.61
4. Cortez AJ, Tudrej P, Kujawa KA, Lisowska KM. Advances in Ovarian Cancer Therapy. Cancer Chemother Pharmacol (2018) 81(1):17–38. doi: 10.1007/s00280-017-3501-8
5. Lheureux S, Braunstein M, Oza AM. Epithelial Ovarian Cancer: Evolution of Management in the Era of Precision Medicine. CA Cancer J Clin (2019) 69(4):280–304. doi: 10.3322/caac.21559
6. Nombela P, Miguel-Lopez B, Blanco S. The Role of M(6)a, M(5)C and Psi Rna Modifications in Cancer: Novel Therapeutic Opportunities. Mol Cancer (2021) 20(1):18. doi: 10.1186/s12943-020-01263-w
7. Boccaletto P, Machnicka MA, Purta E, Piatkowski P, Baginski B, Wirecki TK, et al. Modomics: A Database of Rna Modification Pathways. 2017 Update. Nucleic Acids Res (2018) 46(D1):D303–D7. doi: 10.1093/nar/gkx1030
8. Barbieri I, Kouzarides T. Role of Rna Modifications in Cancer. Nat Rev Cancer (2020) 20(6):303–22. doi: 10.1038/s41568-020-0253-2
9. Chen H, Yao J, Bao R, Dong Y, Zhang T, Du Y, et al. Cross-Talk of Four Types of Rna Modification Writers Defines Tumor Microenvironment and Pharmacogenomic Landscape in Colorectal Cancer. Mol Cancer (2021) 20(1):29. doi: 10.1186/s12943-021-01322-w
10. Zaccara S, Ries RJ, Jaffrey SR. Reading, Writing and Erasing Mrna Methylation. Nat Rev Mol Cell Biol (2019) 20(10):608–24. doi: 10.1038/s41580-019-0168-5
11. Zhang C, Jia G. Reversible Rna Modification N(1)-Methyladenosine (M(1)a) in Mrna and Trna. Genomics Proteomics Bioinf (2018) 16(3):155–61. doi: 10.1016/j.gpb.2018.03.003
12. Tian B, Manley JL. Alternative Polyadenylation of Mrna Precursors. Nat Rev Mol Cell Biol (2017) 18(1):18–30. doi: 10.1038/nrm.2016.116
13. Nishikura K. A-To-I Editing of Coding and Non-Coding Rnas by Adars. Nat Rev Mol Cell Biol (2016) 17(2):83–96. doi: 10.1038/nrm.2015.4
14. Ali AT, Idaghdour Y, Hodgkinson A. Analysis of Mitochondrial M1a/G Rna Modification Reveals Links to Nuclear Genetic Variants and Associated Disease Processes. Commun Biol (2020) 3(1):147. doi: 10.1038/s42003-020-0879-3
15. Bi X, Lv X, Liu D, Guo H, Yao G, Wang L, et al. Mettl3-Mediated Maturation of Mir-126-5p Promotes Ovarian Cancer Progression Via Pten-Mediated Pi3k/Akt/Mtor Pathway. Cancer Gene Ther (2021) 28(3-4):335–49. doi: 10.1038/s41417-020-00222-3
16. Zhang B, Liu Y, Liu D, Yang L. Targeting Cleavage and Polyadenylation Specific Factor 1 Via Shrna Inhibits Cell Proliferation in Human Ovarian Cancer. J Biosci (2017) 42(3):417–25. doi: 10.1007/s12038-017-9701-x
17. Amin EM, Liu Y, Deng S, Tan KS, Chudgar N, Mayo MW, et al. The Rna-Editing Enzyme Adar Promotes Lung Adenocarcinoma Migration and Invasion by Stabilizing Fak. Sci Signal (2017) 10(497):eaah3941. doi: 10.1126/scisignal.aah3941
18. Xiang JF, Yang Q, Liu CX, Wu M, Chen LL, Yang L. N(6)-Methyladenosines Modulate a-to-I Rna Editing. Mol Cell (2018) 69(1):126–35.e6. doi: 10.1016/j.molcel.2017.12.006
19. Dai X, Wang T, Gonzalez G, Wang Y. Identification of Yth Domain-Containing Proteins as the Readers for N1-Methyladenosine in Rna. Anal Chem (2018) 90(11):6380–4. doi: 10.1021/acs.analchem.8b01703
20. Molinie B, Wang J, Lim KS, Hillebrand R, Lu ZX, Van Wittenberghe N, et al. M(6)a-Laic-Seq Reveals the Census and Complexity of the M(6)a Epitranscriptome. Nat Methods (2016) 13(8):692–8. doi: 10.1038/nmeth.3898
21. Bhan A, Soleimani M, Mandal SS. Long Noncoding Rna and Cancer: A New Paradigm. Cancer Res (2017) 77(15):3965–81. doi: 10.1158/0008-5472.CAN-16-2634
22. Lan Y, Liu B, Guo H. The Role of M(6)a Modification in the Regulation of Tumor-Related Lncrnas. Mol Ther Nucleic Acids (2021) 24:768–79. doi: 10.1016/j.omtn.2021.04.002
23. Xue L, Li J, Lin Y, Liu D, Yang Q, Jian J, et al. M(6)a Transferase Mettl3-Induced Lncrna Abhd11-As1 Promotes the Warburg Effect of Non-Small-Cell Lung Cancer. J Cell Physiol (2021) 236(4):2649–58. doi: 10.1002/jcp.30023
24. Shi L, Chen W, Zhang Z, Chen J, Xue M. N1-Methyladenosine Profiling of Long Non-Coding Rna in Colorectal Cancer. IUBMB Life (2021) 73(10):1235–43. doi: 10.1002/iub.2534
25. Silvestris DA, Scopa C, Hanchi S, Locatelli F, Gallo A. De Novo a-to-I Rna Editing Discovery in Lncrna. Cancers (Basel) (2020) 12(10):2959. doi: 10.3390/cancers12102959
26. Liao Y, Jung SH, Kim T. A-To-I Rna Editing as a Tuner of Noncoding Rnas in Cancer. Cancer Lett (2020) 494:88–93. doi: 10.1016/j.canlet.2020.08.004
27. Zhu S, Wang JZ, Chen D, He YT, Meng N, Chen M, et al. An Oncopeptide Regulates M(6)a Recognition by the M(6)a Reader Igf2bp1 and Tumorigenesis. Nat Commun (2020) 11(1):1685. doi: 10.1038/s41467-020-15403-9
28. Zhang X, Shen L, Cai R, Yu X, Yang J, Wu X, et al. Comprehensive Analysis of the Immune-Oncology Targets and Immune Infiltrates of N(6)-Methyladenosine-Related Long Noncoding Rna Regulators in Breast Cancer. Front Cell Dev Biol (2021) 9:686675. doi: 10.3389/fcell.2021.686675
29. Zhang Y, Zhu B, He M, Cai Y, Ying X, Jiang C, et al. N6-Methylandenosine-Related Lncrnas Predict Prognosis and Immunotherapy Response in Bladder Cancer. Front Oncol (2021) 11:710767. doi: 10.3389/fonc.2021.710767
30. Xu F, Huang X, Li Y, Chen Y, Lin L. M(6)a-Related Lncrnas Are Potential Biomarkers for Predicting Prognoses and Immune Responses in Patients With Luad. Mol Ther Nucleic Acids (2021) 24:780–91. doi: 10.1016/j.omtn.2021.04.003
31. Zhang Z, Zhang C, Yang Z, Zhang G, Wu P, Luo Y, et al. M(6)a Regulators as Predictive Biomarkers for Chemotherapy Benefit and Potential Therapeutic Targets for Overcoming Chemotherapy Resistance in Small-Cell Lung Cancer. J Hematol Oncol (2021) 14(1):190. doi: 10.1186/s13045-021-01173-4
32. Li J, Han L, Roebuck P, Diao L, Liu L, Yuan Y, et al. Tanric: An Interactive Open Platform to Explore the Function of Lncrnas in Cancer. Cancer Res (2015) 75(18):3728–37. doi: 10.1158/0008-5472.CAN-15-0273
33. Gao Y, Shang S, Guo S, Li X, Zhou H, Liu H, et al. Lnc2cancer 3.0: An Updated Resource for Experimentally Supported Lncrna/Circrna Cancer Associations and Web Tools Based on Rna-Seq and Scrna-Seq Data. Nucleic Acids Res (2021) 49(D1):D1251–D8. doi: 10.1093/nar/gkaa1006
34. Zheng Y, Xu Q, Liu M, Hu H, Xie Y, Zuo Z, et al. Lncar: A Comprehensive Resource for Lncrnas From Cancer Arrays. Cancer Res (2019) 79(8):2076–83. doi: 10.1158/0008-5472.CAN-18-2169
35. Li Y, Jiang T, Zhou W, Li J, Li X, Wang Q, et al. Pan-Cancer Characterization of Immune-Related Lncrnas Identifies Potential Oncogenic Biomarkers. Nat Commun (2020) 11(1):1000. doi: 10.1038/s41467-020-14802-2
36. Li Y, Li L, Wang Z, Pan T, Sahni N, Jin X, et al. Lncmap: Pan-Cancer Atlas of Long Noncoding Rna-Mediated Transcriptional Network Perturbations. Nucleic Acids Res (2018) 46(3):1113–23. doi: 10.1093/nar/gkx1311
37. Li VD, Li KH, Li JT. Tp53 Mutations as Potential Prognostic Markers for Specific Cancers: Analysis of Data From the Cancer Genome Atlas and the International Agency for Research on Cancer Tp53 Database. J Cancer Res Clin Oncol (2019) 145(3):625–36. doi: 10.1007/s00432-018-2817-z
38. Yang C, Hu Y, Zhou B, Bao Y, Li Z, Gong C, et al. The Role of M(6)a Modification in Physiology and Disease. Cell Death Dis (2020) 11(11):960. doi: 10.1038/s41419-020-03143-z
39. Statello L, Guo CJ, Chen LL, Huarte M. Gene Regulation by Long Non-Coding Rnas and Its Biological Functions. Nat Rev Mol Cell Biol (2021) 22(2):96–118. doi: 10.1038/s41580-020-00315-9
40. Salamini-Montemurri M, Lamas-Maceiras M, Barreiro-Alonso A, Vizoso-Vazquez A, Rodriguez-Belmonte E, Quindos-Varela M, et al. The Challenges and Opportunities of Lncrnas in Ovarian Cancer Research and Clinical Use. Cancers (Basel) (2020) 12(4):1020. doi: 10.3390/cancers12041020
41. Liu HT, Zou YX, Zhu WJ, Sen L, Zhang GH, Ma RR, et al. Lncrna Thap7-As1, Transcriptionally Activated by Sp1 and Post-Transcriptionally Stabilized by Mettl3-Mediated M6a Modification, Exerts Oncogenic Properties by Improving Cul4b Entry Into the Nucleus. Cell Death Differ (2021) 29(3):627–41. doi: 10.1038/s41418-021-00879-9
42. Yin H, Chen L, Piao S, Wang Y, Li Z, Lin Y, et al. M6a Rna Methylation-Mediated Rmrp Stability Renders Proliferation and Progression of Non-Small Cell Lung Cancer Through Regulating Tgfbr1/Smad2/Smad3 Pathway. Cell Death Differ (2021). doi: 10.1038/s41418-021-00888-8
43. Jin D, Guo J, Wu Y, Du J, Yang L, Wang X, et al. M(6)a Mrna Methylation Initiated by Mettl3 Directly Promotes Yap Translation and Increases Yap Activity by Regulating the Malat1-Mir-1914-3p-Yap Axis to Induce Nsclc Drug Resistance and Metastasis. J Hematol Oncol (2021) 14(1):32. doi: 10.1186/s13045-021-01048-8
44. Sun T, Wu Z, Wang X, Wang Y, Hu X, Qin W, et al. Lnc942 Promoting Mettl14-Mediated M(6)a Methylation in Breast Cancer Cell Proliferation and Progression. Oncogene (2020) 39(31):5358–72. doi: 10.1038/s41388-020-1338-9
45. Zheng J, Guo J, Cao B, Zhou Y, Tong J. Identification and Validation of Lncrnas Involved in M6a Regulation for Patients With Ovarian Cancer. Cancer Cell Int (2021) 21(1):363. doi: 10.1186/s12935-021-02076-7
46. Zhao P, Li T, Wang Y, Wang Y, Gu Q, Li Z. Lncrna Mycnos Promotes Glioblastoma Cell Proliferation by Regulating Mir-216b/Foxm1 Axis. Metab Brain Dis (2021) 36(6):1185–9. doi: 10.1007/s11011-021-00729-0
47. Long J, Bai Y, Yang X, Lin J, Yang X, Wang D, et al. Construction and Comprehensive Analysis of a Cerna Network to Reveal Potential Prognostic Biomarkers for Hepatocellular Carcinoma. Cancer Cell Int (2019) 19:90. doi: 10.1186/s12935-019-0817-y
48. Goodall GJ, Wickramasinghe VO. Rna in Cancer. Nat Rev Cancer (2021) 21(1):22–36. doi: 10.1038/s41568-020-00306-0
49. Goyal B, Yadav SRM, Awasthee N, Gupta S, Kunnumakkara AB, Gupta SC. Diagnostic, Prognostic, and Therapeutic Significance of Long Non-Coding Rna Malat1 in Cancer. Biochim Biophys Acta Rev Cancer (2021) 1875(2):188502. doi: 10.1016/j.bbcan.2021.188502
50. Cao S, Wang Y, Li J, Lv M, Niu H, Tian Y. Tumor-Suppressive Function of Long Noncoding Rna Malat1 in Glioma Cells by Suppressing Mir-155 Expression and Activating Fbxw7 Function. Am J Cancer Res (2016) 6(11):2561–74.
51. Li Q, Zhang C, Chen R, Xiong H, Qiu F, Liu S, et al. Disrupting Malat1/Mir-200c Sponge Decreases Invasion and Migration in Endometrioid Endometrial Carcinoma. Cancer Lett (2016) 383(1):28–40. doi: 10.1016/j.canlet.2016.09.019
52. Kim J, Piao HL, Kim BJ, Yao F, Han Z, Wang Y, et al. Long Noncoding Rna Malat1 Suppresses Breast Cancer Metastasis. Nat Genet (2018) 50(12):1705–15. doi: 10.1038/s41588-018-0252-3
53. Ou X, Gao G, Bazhabayi M, Zhang K, Liu F, Xiao X. Malat1 and Bach1 Are Prognostic Biomarkers for Triple-Negative Breast Cancer. J Cancer Res Ther (2019) 15(7):1597–602. doi: 10.4103/jcrt.JCRT_282_19
54. Zheng L, Zhang Y, Fu Y, Gong H, Guo J, Wu K, et al. Long Non-Coding Rna Malat1 Regulates Blcap Mrna Expression Through Binding to Mir-339-5p and Promotes Poor Prognosis in Breast Cancer. Biosci Rep (2019) 39(2):BSR20181284. doi: 10.1042/BSR20181284
55. Malik R, Patel L, Prensner JR, Shi Y, Iyer MK, Subramaniyan S, et al. The Lncrna Pcat29 Inhibits Oncogenic Phenotypes in Prostate Cancer. Mol Cancer Res (2014) 12(8):1081–7. doi: 10.1158/1541-7786.MCR-14-0257
56. Bao G, Xu R, Wang X, Ji J, Wang L, Li W, et al. Identification of Lncrna Signature Associated With Pan-Cancer Prognosis. IEEE J BioMed Health Inform (2021) 25(6):2317–28. doi: 10.1109/JBHI.2020.3027680
57. Li Q, Li H, Zhang L, Zhang C, Yan W, Wang C. Identification of Novel Long Non-Coding Rna Biomarkers for Prognosis Prediction of Papillary Thyroid Cancer. Oncotarget (2017) 8(28):46136–44. doi: 10.18632/oncotarget.17556
58. Cha JH, Chan LC, Li CW, Hsu JL, Hung MC. Mechanisms Controlling Pd-L1 Expression in Cancer. Mol Cell (2019) 76(3):359–70. doi: 10.1016/j.molcel.2019.09.030
59. Farkkila A, Gulhan DC, Casado J, Jacobson CA, Nguyen H, Kochupurakkal B, et al. Immunogenomic Profiling Determines Responses to Combined Parp and Pd-1 Inhibition in Ovarian Cancer. Nat Commun (2020) 11(1):1459. doi: 10.1038/s41467-020-15315-8
60. Gascard P, Tlsty TD. Carcinoma-Associated Fibroblasts: Orchestrating the Composition of Malignancy. Genes Dev (2016) 30(9):1002–19. doi: 10.1101/gad.279737.116
61. Cui Y, Wang D, Xie M. Tumor-Derived Extracellular Vesicles Promote Activation of Carcinoma-Associated Fibroblasts and Facilitate Invasion and Metastasis of Ovarian Cancer by Carrying Mir-630. Front Cell Dev Biol (2021) 9:652322. doi: 10.3389/fcell.2021.652322
62. Chanmee T, Ontong P, Konno K, Itano N. Tumor-Associated Macrophages as Major Players in the Tumor Microenvironment. Cancers (Basel) (2014) 6(3):1670–90. doi: 10.3390/cancers6031670
63. Riabov V, Gudima A, Wang N, Mickley A, Orekhov A, Kzhyshkowska J. Role of Tumor Associated Macrophages in Tumor Angiogenesis and Lymphangiogenesis. Front Physiol (2014) 5:75. doi: 10.3389/fphys.2014.00075
64. Allavena P, Sica A, Garlanda C, Mantovani A. The Yin-Yang of Tumor-Associated Macrophages in Neoplastic Progression and Immune Surveillance. Immunol Rev (2008) 222:155–61. doi: 10.1111/j.1600-065X.2008.00607.x
66. Zhu P, Baek SH, Bourk EM, Ohgi KA, Garcia-Bassets I, Sanjo H, et al. Macrophage/Cancer Cell Interactions Mediate Hormone Resistance by a Nuclear Receptor Derepression Pathway. Cell (2006) 124(3):615–29. doi: 10.1016/j.cell.2005.12.032
67. Wang H, Xie X, Lu WG, Ye DF, Chen HZ, Li X, et al. Ovarian Carcinoma Cells Inhibit T Cell Proliferation: Suppression of Il-2 Receptor Beta and Gamma Expression and Their Jak-Stat Signaling Pathway. Life Sci (2004) 74(14):1739–49. doi: 10.1016/j.lfs.2003.07.051
68. Abubaker K, Luwor RB, Escalona R, McNally O, Quinn MA, Thompson EW, et al. Targeted Disruption of the Jak2/Stat3 Pathway in Combination With Systemic Administration of Paclitaxel Inhibits the Priming of Ovarian Cancer Stem Cells Leading to a Reduced Tumor Burden. Front Oncol (2014) 4:75. doi: 10.3389/fonc.2014.00075
69. Woyach JA, Johnson AJ, Byrd JC. The B-Cell Receptor Signaling Pathway as a Therapeutic Target in Cll. Blood (2012) 120(6):1175–84. doi: 10.1182/blood-2012-02-362624
70. Tsibulak I, Zeimet AG, Marth C. Hopes and Failures in Front-Line Ovarian Cancer Therapy. Crit Rev Oncol Hematol (2019) 143:14–9. doi: 10.1016/j.critrevonc.2019.08.002
71. Bogliolo S, Cassani C, Gardella B, Musacchi V, Babilonti L, Venturini PL, et al. Oxaliplatin for the Treatment of Ovarian Cancer. Expert Opin Investig Drugs (2015) 24(9):1275–86. doi: 10.1517/13543784.2015.1062874
72. Chan KKL, Ngu SF, Chu MMY, Tse KY, Ngan HYS. Tamoxifen Use in Recurrent Ovarian Cancer in a Chinese Population: A 15 -Year Clinical Experience in a Tertiary Referral Center. Asia Pac J Clin Oncol (2021) 17(4):338–42. doi: 10.1111/ajco.13478
73. Langdon SP, Gourley C, Gabra H, Stanley B. Endocrine Therapy in Epithelial Ovarian Cancer. Expert Rev Anticancer Ther (2017) 17(2):109–17. doi: 10.1080/14737140.2017.1272414
74. Wainberg ZA, Alsina M, Soares HP, Brana I, Britten CD, Del Conte G, et al. A Multi-Arm Phase I Study of the Pi3k/Mtor Inhibitors Pf-04691502 and Gedatolisib (Pf-05212384) Plus Irinotecan or the Mek Inhibitor Pd-0325901 in Advanced Cancer. Targeted Oncol (2017) 12(6):775–85. doi: 10.1007/s11523-017-0530-5
75. Hew KE, Miller PC, El-Ashry D, Sun J, Besser AH, Ince TA, et al. Mapk Activation Predicts Poor Outcome and the Mek Inhibitor, Selumetinib, Reverses Antiestrogen Resistance in Er-Positive High-Grade Serous Ovarian Cancer. Clin Cancer Res (2016) 22(4):935–47. doi: 10.1158/1078-0432.CCR-15-0534
76. Liu T, Wei Q, Jin J, Luo Q, Liu Y, Yang Y, et al. The M6a Reader Ythdf1 Promotes Ovarian Cancer Progression Via Augmenting Eif3c Translation. Nucleic Acids Res (2020) 48(7):3816–31. doi: 10.1093/nar/gkaa048
Keywords: serous ovarian carcinoma, RNA modification writers, lncRNA, prognosis, immune microenvironment
Citation: Ye L, Pan K, Fang S, Wu S-N, Chen S, Tang S, Wang N, Zhang H, Tong X, Shi X, Feng S, Xiang D, Zou R, Hu Y, Xue X and Guo G (2022) Four Types of RNA Modification Writer-Related lncRNAs Are Effective Predictors of Prognosis and Immunotherapy Response in Serous Ovarian Carcinoma. Front. Immunol. 13:863484. doi: 10.3389/fimmu.2022.863484
Received: 27 January 2022; Accepted: 31 March 2022;
Published: 02 May 2022.
Edited by:
Eleni Anastasiadou, Sapienza University of Rome, ItalyReviewed by:
Zengjun Wang, Nanjing Medical University, ChinaSudhanshu K. Shukla, Indian Institute of Technology Dharwad, India
Copyright © 2022 Ye, Pan, Fang, Wu, Chen, Tang, Wang, Zhang, Tong, Shi, Feng, Xiang, Zou, Hu, Xue and Guo. This is an open-access article distributed under the terms of the Creative Commons Attribution License (CC BY). The use, distribution or reproduction in other forums is permitted, provided the original author(s) and the copyright owner(s) are credited and that the original publication in this journal is cited, in accordance with accepted academic practice. No use, distribution or reproduction is permitted which does not comply with these terms.
*Correspondence: Xiangyang Xue, d3p4eHkwMDFAMTYzLmNvbQ==; Gangqiang Guo, Z2FuZ3FpYW5nZ0B5ZWFoLm5ldA==
†These authors have contributed equally to this work and share first authorship