- 1School of Biology and Biological Engineering, South China University of Technology, Guangzhou, China
- 2Translational Medicine Collaborative Innovation Center, Shenzhen People’s Hospital, Shenzhen, China
T-cell exhaustion is one of the main reasons of tumor immune escape. Using single-cell transcriptome data of CD8+ T cells in multiple cancers, we identified different cell types, in which Pre_exhaust and exhausted T cells participated in negative regulation of immune system process. By analyzing the coexpression network patterns and differentially expressed genes of Pre_exhaust, exhausted, and effector T cells, we identified 35 genes related to T-cell exhaustion, whose high GSVA scores were associated with significantly poor prognosis in various cancers. In the differentially expressed genes, RGS1 showed the greatest fold change in Pre_exhaust and exhausted cells of three cancers compared with effector T cells, and high expression of RGS1 was also associated with poor prognosis in various cancers. Additionally, RGS1 protein was upregulated significantly in tumor tissues in the immunohistochemistry verification. Furthermore, RGS1 displayed positive correlation with the 35 genes, especially highly correlated with PDCD1, CTLA4, HAVCR2, and TNFRSF9 in CD8+ T cells and cancer tissues, indicating the important roles of RGS1 in CD8+ T-cell exhaustion. Considering the GTP-hydrolysis activity of RGS1 and significantly high mRNA and protein expression in cancer tissues, we speculated that RGS1 potentially mediate the T-cell retention to lead to the persistent antigen stimulation, resulting in T-cell exhaustion. In conclusion, our findings suggest that RGS1 is a new marker and promoting factor for CD8+ T-cell exhaustion and provide theoretical basis for research and immunotherapy of exhausted cells.
Introduction
T-cell exhaustion (Tex), a hyporesponsive state of T cells with increased inhibitory receptors, decreased effector cytokines, and impaired cytotoxicity, was originally described in CD8+ T cells during chronic lymphocytic choriomeningitis virus (LCMV) of mice (1). In recent years, the phenomenon of Tex has also been found in cancers (2, 3), which is one of the main reasons of tumor immune escape (4). It has been reported that exhausted T cells in cancers share many similarities with that in chronic infection (5) and play a significant role in tumorigenesis (6). Studies show that exhausted T cells can be used as one of the main targets of immunosuppression therapy to save T cell from exhaustion and reactivate the cytotoxicity of T cells, providing a new opportunity for clinical immunotherapy (7). Nevertheless, due to the complexity and heterogeneity of cancers, the concrete mechanisms and molecules of T-cell exhaustion in cancers have not been fully elucidated.
Currently, single-cell RNA sequencing (scRNA-seq) has clearly revealed some new mechanisms and phenomena of cancer with the advantages of high accuracy and reproducibility (8–10). Using single-cell transcriptome profiling, we can identify new types of immune cells which cannot be revealed at the original tissue level and can construct a developmental trajectory for immune cells which can reveal the heterogeneity (11). These new findings are useful to better understand the immune system and its mechanism of action on tumors. Notably, this technology makes it possible to explore complicated tumor microenvironment including tumor-infiltrating lymphocytes (TILs) in melanoma, head and neck cancer, breast cancer, and glioblastoma cancer (12–15). Thus, using advantage of scRNA-seq to analyze T cells and obtain the hallmarks of exhausted T cells can bring a new therapeutic strategy on clinical cancer treatment.
Due to the vital role of CD8+ T cells in eliciting antitumor responses (16), we integrated single-cell transcriptome data from colorectal cancer (CRC), hepatocellular cancer (HCC), and nonsmall cell lung cancer (NSCLC) to analyze CD8+ T cells in various cancers in the present study. Focusing on CD8+ T-cell exhaustion-associated clusters, we identified RGS1 as a new marker and promoting factor for T-cell exhaustion in multiple cancers with poor prognosis and showed highly positive correlation with the well-known genes associated with T-cell exhaustion. RGS1 protein highly expressed in tumor tissues was also verified in the immunohistochemistry (IHC) experiment. Our findings could facilitate in understanding the mechanism during the formation and development of T-cell exhaustion and provide theoretical basis for research and immunotherapy of exhausted cells.
Materials and Methods
Data Acquisition
The single-cell gene expression matrices including raw count and TPM data were obtained from the GEO database: GSE108989 (CRC), GSE99254(HCC), and GSE98638(NSCLC), and we isolated CD8+ T cells from peripheral blood (P), adjacent normal (N), and tumor tissues (T).
Quality Control and Data Processing
The raw count expression matrices were processed by R package Seurat v3.2.0 (http://satijalab.org/seurat/). To filter out the low-quality cells, we excluded cells with <600 and >10,000 detected genes (17). Counts were log normalized and scaled by linear regression against the number of reads with function NormalizeData and ScaleData. The highly variable genes (HVGs) were generated with FindVariableFeatures. Principal component analysis (PCA) was performed on the top 2,000 HVGs using function RunPCA. The appropriate PCs were selected for graph-based clustering with functions FindNeighbors and FindClusters. For visualization of clustering analysis, we performed uniform manifold approximation and projection for dimension reduction (UMAP) using RunUMAP function in Seurat. To eliminate the obvious effect from different patients, we performed standard normalization and variable feature selection after acquiring the data. Next, the function FindIntegrationAnchors was performed to find a set of anchors, which is used to integrate the data by the function IntegrateData. Also, the function AddModuleScore was used to calculate scores of gene list in different cell types.
Cell Type Annotation
Differentially expressed genes (DEGs) of each cluster were identified based on Wilcoxon rank-sum test using function FindAllMarkers compared with the rest of the clusters. In brief, for each cluster, only genes that met these criteria were considered cluster-specific DEGs (1): log2FC >0.25; (2) expressed >25% in either of the two groups of cells; (3) adjusted p-value <0.05. The top DEGs were selected to annotate each cluster based on the canonical markers from previous studies; also, the CellMarker databases (18) and R package SingleR v1.4.0 (19) were performed to further improve the accuracy of the annotation.
Trajectory Analysis
To explore the potential functional changes of CD8+ T cell of different clusters for each cancer, we performed development trajectory analysis by R package Monocle v2.18.0 (20) with the cluster-specific genes of each cluster. Dimensional reduction and cell ordering were performed using reduceDimension and orderCells functions with default parameter.
Gene Ontology Enrichment Analysis of DEGs
Biological significance was explored by Gene Ontology (GO) term enrichment analysis by R package clusterProfiler v3.18.0 (21) including biological process, cellular component, and molecular function. Adjusted p-value <0.05 was considered statistically significant. Visualization is realized by R package ggplot2 v3.3.3 and ggalluvial v0.12.3 (22).
Weighted Gene Coexpression Network Analysis
In order to identify the highly linked genes in specific clusters, weighted gene coexpression network analysis (WGCNA) was performed with functions in the R package WGCNA (23). To attenuate the effects of noise and outliers, we constructed pseudocells (24) which were calculated as averages of 10 cells randomly chosen within each cluster. The function pickSoftThreshold was used to calculate the soft power parameter and blockwiseModules to construct coexpression network. Finally, corPvalueStudent was used to mine modules related to specific cell types.
Survival Analysis
According to the median of gene expression values, cells were divided into high and low groups, then the survival curve was shown using the Kapla–Meier curve with a log-rank test by GEPIA2 (http://gepia2.cancer-pku.cn/) to illustrate the relationship between differential genes and overall patient survival. The terms with p-value <0.05 were identified as significant. Additionally, multiple hypothesis testing (FDR) was performed to the significant p-value using the function p.adjust.
Gene Set Variation Analysis
For generated gene set, we performed gene set variation analysis (GSVA) to the data in the present study and validation dataset using R package GSVA (25) with the default parameter, to evaluate the effect of distinguishing Tex cells.
The Basic Expression of mRNA and Protein in Normal and Cancer Tissues
The RNA-seq data were downloaded from the TCGA database (https://portal.gdc.cancer.gov/) and then calculated into TPM value. According to the annotation, samples were grouped into normal and four stages of cancer groups. The differential expression of mRNA in the different groups was performed by Wilcoxon test with p-value <0.05.
The protein expression level in normal and cancer tissues was analyzed using the Human Protein Atlas (HPA) database (https://www.proteinatlas.org). Immunohistochemistry pictures were downloaded from the Tissue Atlas and Pathology Atlas.
Immunohistochemistry
Immunohistochemistry was carried out on human liver cancer tissue microarray (Shanghai Outdo Biotech Co., Ltd., Shanghai, China). The tissue sections were first dried at 63°C for 1 h, dewaxed, and rehydrated before epitope retrieval by heating at 100°C in 10 mM sodium citrate (pH 6.0) for 5 min in EDTA solution for 20 min. The sections were cooled down to room temperature for 30 min. The tissue sections were treated with 3% hydrogen peroxide for 20 min to eliminate the endogenous peroxidase and alkaline phosphatase activity in the tissue. After cooling down to room temperature, the sections were treated by blocking agents for 10 min. The sections were incubated with individual primary antibody (RGS1, Invitrogen, Waltham, MA, USA; Product #PA5-86730) diluted 1:1,000,800 overnight at 4°C, followed by secondary antibodies at room temperature for 30 min. 3,3′-Diaminobenzidine (DAB) was then applied as a substrate to reveal the antigen. Hematoxylin was used for counterstaining. The stained images were counted by ImageJ software (26), and the optical density (OD) value was used for quantification.
Results
Single CD8+ T-Cell Transcriptome Landscape
We obtained single-cell transcriptome data of human T cells from the GEO database, including 12 patients from CRC (27), six patients from HCC (28), and 14 patients from NSCLC (29). After strict quality control and filtration, we collected 4,010, 1,752, and 4,439 CD8+ T cells from peripheral blood (P), adjacent normal (N), and tumor tissues (T) (Supplementary Figures S1A, B; Supplementary Table S1).
We divided the cells of each cancer into different cell types annotated with cluster-specific gene expression (Figure 1A; Supplementary Table S2). Specifically, in CRC, cells were identified as naïve T cells (Tn, cluster 4, marked with selectin L (SELL), lymphoid enhancer binding factor 1 (LEF1), C–C motif chemokine receptor 7 (CCR7), transcription factor 7 (TCF7), effector T cells (Teff, cluster 2 and cluster 5, marked with C-X3-C motif chemokine receptor 1 (CX3CR1), killer cell lectin-like receptor F1 (KLRF1)), fibroblast growth factor-binding protein 2 (FGFBP2), Fc fragment of IgG receptor IIIa (FCGR3A), exhausted T cells (Tex, cluster 3 and cluster 6, marked with hepatitis A virus cellular receptor 2 (HAVCR2), programmed cell death 1 (PDCD1), lymphocyte activating 3 (LAG3), TOX, CXCL13, tissue-resident memory T cells (Trm, cluster 1, marked with CD69, integrin subunit alpha E (ITGAE)), mucosal-associated invariant T cells (MAIT, cluster 7, marked with solute carrier family 4 member 10 (SLC4A10)), RAR-related orphan receptor C (RORC) (Figures 1B, C). Cluster 0 was located between cluster 2 and cluster 3, which was represented as Teff and Tex cells, besides, the genes marked Teff and Tex cells showed relative high expression levels in this cluster, therefore, we identified it as Pre_exhaust T cells. Similarly, cells in HCC were identified as Tn (cluster 4), Teff (cluster 0), Pre_exhaust (cluster 1), Tex (cluster 3 and cluster5), MAIT (cluster 2), and cells in NSCLC that were identified as Tn (cluster 4), Teff (cluster 0 and cluster 6), Pre_exhaust (cluster 1 and cluster 7), Tex (cluster 3), Trm (cluster 2), and MAIT (cluster 5). Different cell types showed preference in different tissues. In general, Tn and Teff cells were mainly enriched in peripheral blood, Pre_exhaust and Tex cells were mainly enriched in tumor tissues, and Trm cells existed more in adjacent normal tissues of CRC and HCC, and in tumor tissues of NSCLC, which may be related to tissue specificity. Also, the number of MAIT cells was relative less than the others. Additionally, we performed exhausted cell scoring by the function AddModuleScore in R package Seurat using the exhaustion gene list including HAVCR2, T-cell immunoreceptor with Ig and ITIM domains (TIGIT), LAG3, PDCD1, CXCL13, layilin (LAYN), TOX, cytotoxic T-lymphocyte associated protein 4 (CTLA4), and B and T lymphocyte associated (BTLA) and visualized in Supplementary Figure S1C, showing the accuracy of Tex cell classification (Supplementary Figure S1C).
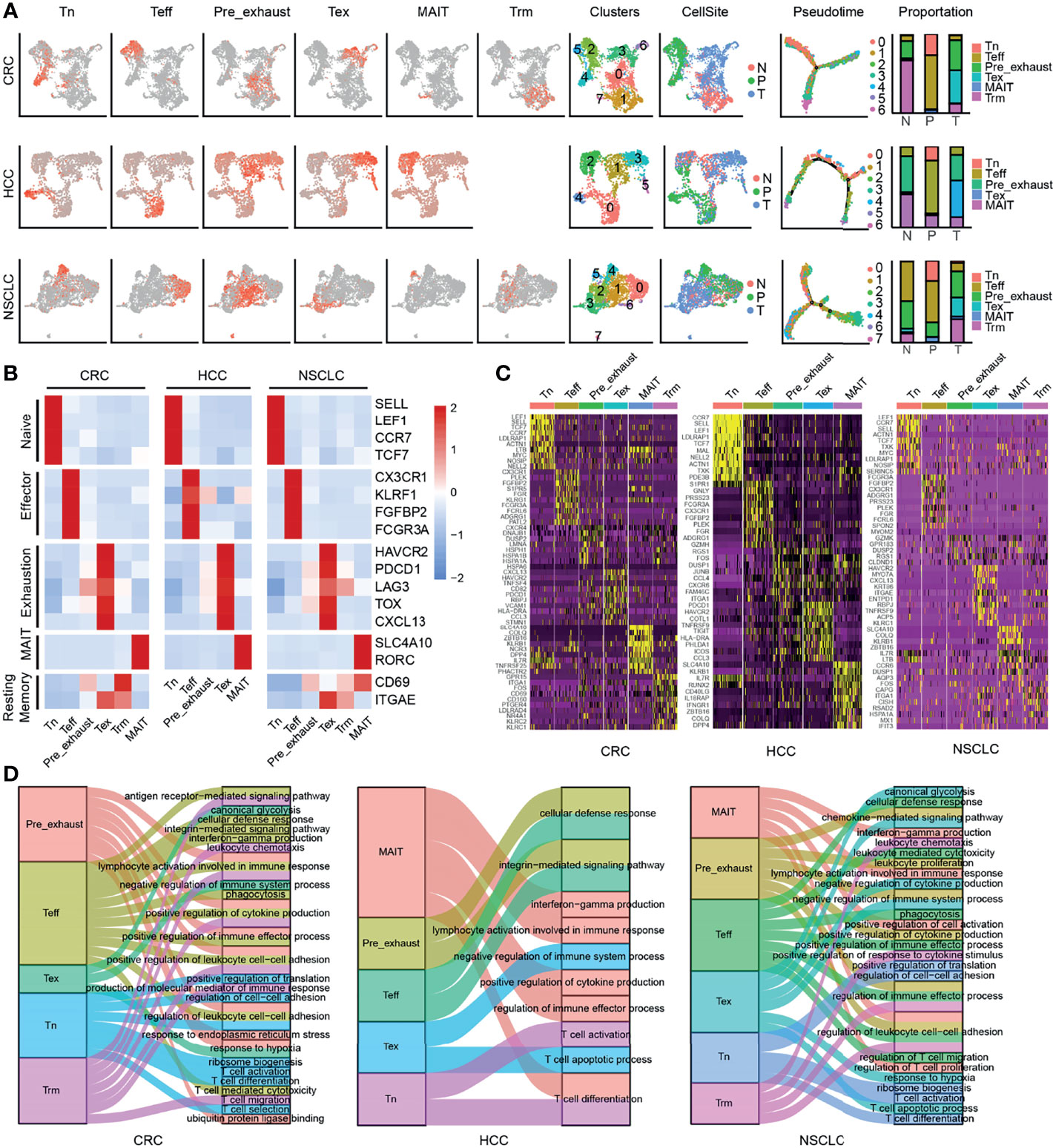
Figure 1 Clustering of CD8+ T cells in three cancers. (A) UMAP of single cells to visualize cell-type clusters (left), Pseudo-time trajectory graph (middle), and the proportion of different cell types in different sources (right). (B) Heatmap showing marker genes for CD8+ cell types. (C) The top 10 DEGs in each cell type in three cancers. (D) The GO enrichment analysis of different cell types of CD8+ T cells in three cancers.
To further explore the function of each cluster, we performed GO enrichment analysis using the cluster-specific genes (Figure 1D). In all three cancers, Tn cells were enriched in T-cell activation, T-cell differentiation, and ribosome biogenesis; Teff cells were enriched in cellular defense response, positive regulation of cytokine production, and T-cell-mediated cytotoxicity; Tex cells were enriched in negative regulation of immune system process, T-cell apoptotic process, and response to hypoxia; Trm cells were enriched in antigen receptor-mediated signaling pathway and leukocyte chemotaxis; MAIT cells were enriched in interferon-gamma production; and Pre_exhaust cells were enriched in terms associated with Teff and Tex cells, including cellular defense response and negative regulation of immune system process, showing the transitional characteristics during T-cell exhaustion.
Establishment of Coexpression Network
Tex cells, as shown above, played a negative role in immune system process, and it was suggested that Pre_exhaust cells were a transitional stage from Teff to Tex cells. To find out the highly linked genes associated with T-cell exhaustion, we used the R package WGCNA to construct the weighted coexpression network in Teff, Pre_exhaust, and Tex cells (Figure 2A). The blue module (R-value 0.96, p-value 2e−145), turquoise module (R-value 0.96, p-value 7e−67), and blue module (R-value 0.96, p-value 2e−172) represented Tex cells in CRC, HCC, and NSCLC, respectively. Combining with the differentially expressed genes (DEGs) upregulated in the Tex vs. Teff cell comparison in three cancers (Supplementary Table S3), 35 genes were found in three Tex cell-related modules and overexpressed in Tex cells (Figure 2B; Table 1), which were defined as “Candidate” gene set, including exhaustion marker PDCD1, CTLA4, HAVCR2, TOX, and TIGIT. It is noteworthy that the housekeeping gene glyceraldehyde-3-phosphate dehydrogenase (GAPDH), which can catalyze an important energy-yielding step in glycolysis metabolism, was also upregulated and enriched in the highly linked DEGs, thus we speculated that the glycolysis progress was disordered in Tex cells.
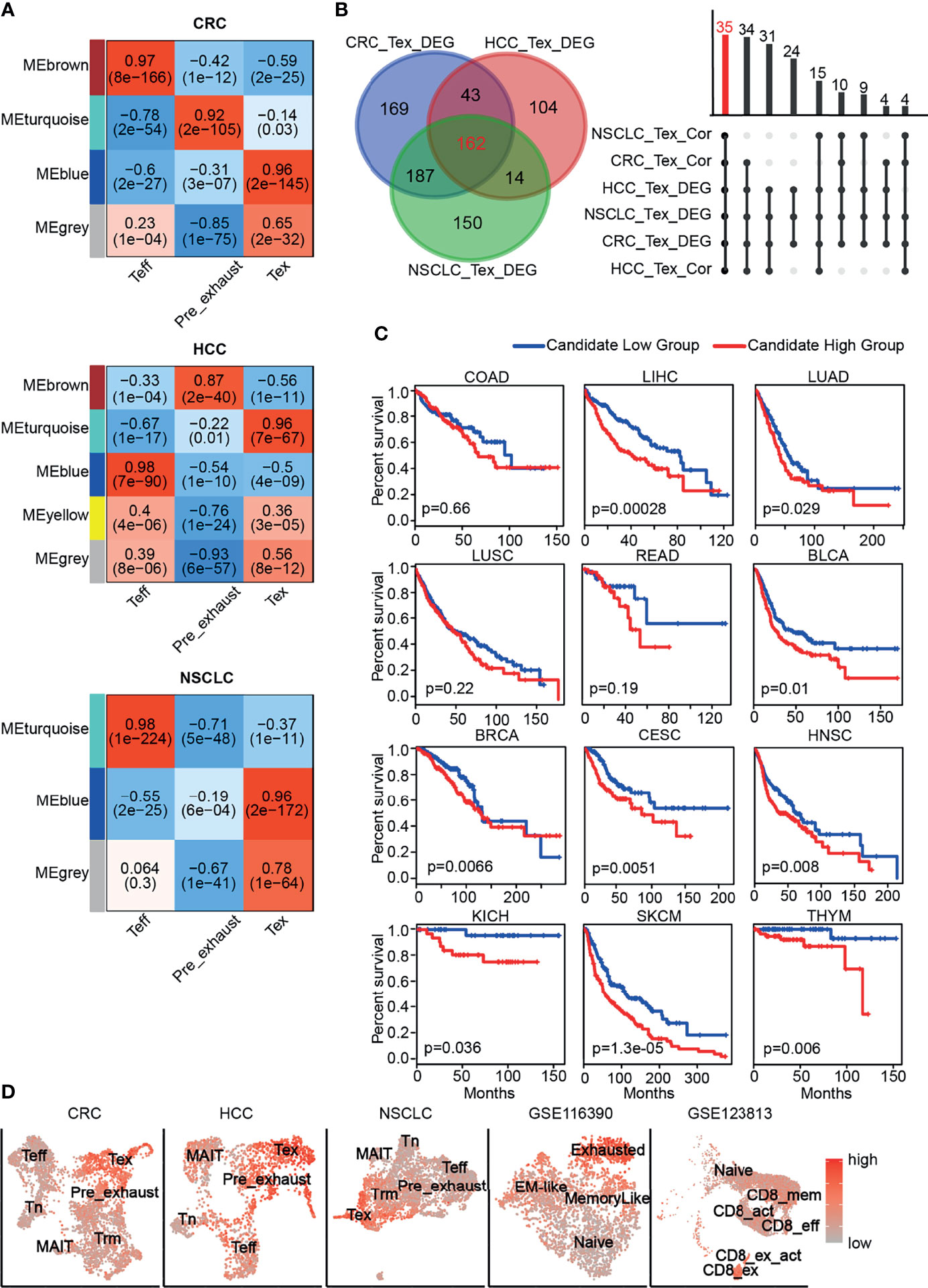
Figure 2 Candidate gene set associated with CD8+ Tex cells. (A) The gene coexpression network modules of CD8+ T cells with correlation coefficient and p-value. (B) The number of genes with differential expression (left) and coexpression (right). (C) The Kaplan-Meier overall survival curves of TCGA patients grouped by the middle expression value of Candidate gene set. The red and blue lines denote higher and lower expression group, respectively. (D) Distinguishing Tex cells from the other CD8+ T cells effectively in different cancers by GSVA score of Candidate gene set.
As shown in Figure 2C, poor overall survival was correlated with higher Candidate gene set expression in multiple cancers including liver hepatocellular carcinoma (LIHC), lung adenocarcinoma (LUAD), bladder urothelial carcinoma (BLCA), breast invasive carcinoma (BRCA), cervical squamous cell carcinoma and endocervical adenocarcinoma (CESC), head and neck squamous cell carcinoma (HNSC), kidney chromophobe (KICH), skin cutaneous melanoma (SKCM), thymoma (THYM). In addition, the GSVA scores of Candidate gene set in Tex cells were much higher than those of the other clusters in CRC, HCC, and NSCLC, so were in the other independent validation datasets (Figure 2D). GSE116390 dataset (30) was composed of four types of tumor-infiltrating CD8+ T cells, which were exhausted, memory-like, naïve, and effector memory-like (EM-like) subsets, from B16 melanoma tumor-bearing mice, and GSE123813 dataset (31) contained six types of tumor-infiltrating CD8+ T cells, which were from 11 patients with advanced basal cell carcinoma, showing higher score in the exhausted related clusters.
As indicated above, the Candidate gene set was enriched in exhausted CD8+ T cells with poor prognosis and was able to distinguish Tex cells from the other CD8+ T cells in different cancers, indicating that the GSVA score of these 35 genes might be an effective prognostic marker or a marker to identify Tex cells.
Differentially Expressed Genes Associated With T-Cell ExhaustionV
The exhaustion of T cells was gradually formed, so we performed differential expression analysis of Pre_exhaust and Tex cells compared with Teff cells, in order to further explore the critical genes associated with the formation and development of T-cell exhaustion. In the Pre_exhaust vs. Teff cell comparison, 119 DEGs existed in all three cancers, 57 of whom were upregulated and the others were downregulated. Correspondingly, there were 162 up-regulated and 88 down-regulated DEGs in the Tex vs. Teff cells comparison. Furthermore, there were 40 DEGs overexpressed in both Pre_exhaust and Tex cells compared with Teff cells (Figure 3A), which may contribute to the origin of T-cell exhaustion, including the canonical exhaustion marker PDCD1 which encodes an inhibitory receptor (32), CD69 with the capability to mediate the cell retention (33), Cbl Proto-Oncogene B(CBLB) whose deletion can inhibit CD8+ T-cell exhaustion (34), hypoxia-inducible factor-1 (HIF1A) which can stably be expressed in hypoxia condition, consistent with the biological process of Tex cells (Figure 1D).
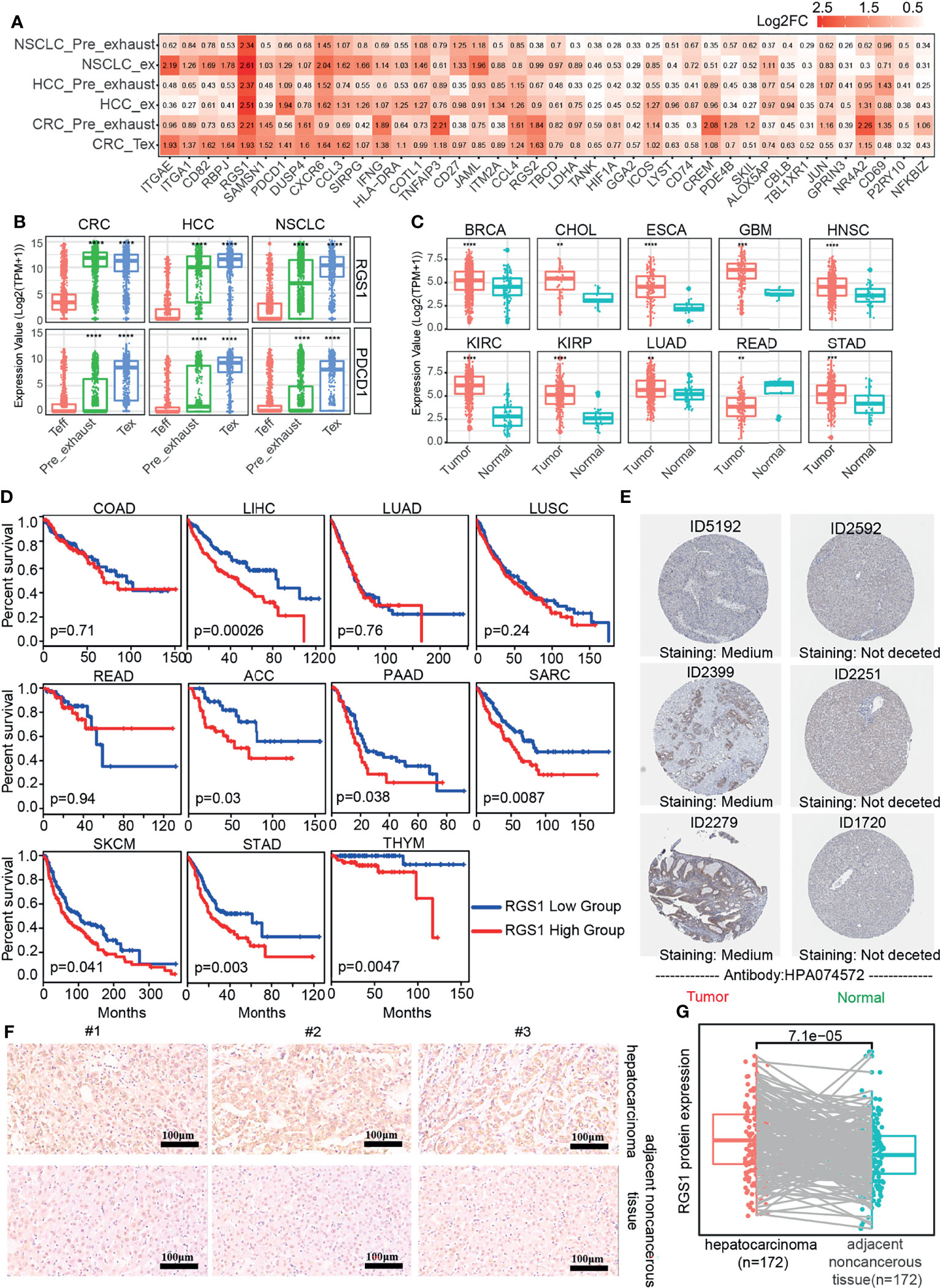
Figure 3 DEGs in Pre_exhasuted and Tex cells compared with Teff cells. (A) The common upregulated genes in Pre_exhasuted and Tex cells compared with Teff cells. (B) The mRNA expression value of RGS1 in single-cell dataset and TCGA database (C). (D) The Kaplan-Meier overall survival curves of TCGA patients grouped by the middle expression value of RGS1. The red and blue lines denote higher and lower expression group, respectively. (E) Representative IHC images of RGS1 protein in tumor and normal tissues of liver derived from the HPA database and verification experiment (F, scale bar 100 µm, magnification ×20). (G) The protein expression value of RGS1 in hepatocarcinoma and adjacent noncancerous tissues in the IHC verification experiment. **p <= 0.01, ***p <= 0.001, ****p <= 0.0001.
Obviously, regulator of G protein signaling 1 (RGS1) showed the greatest fold change in Tex cells across three cancers and also across different patients, showing the greatest fold change in Pre_exhaust cell in HCC and NSCLC (Figure 3A; Supplementary Figure S2), illustrating its potential roles during T-cell exhaustion. RGS1 was expressed highly in whole Tex cells compared with Teff cells (Figure 3B), eliminating that the high fold change of RGS1 in Pre_exhaust and Tex cells was not caused by partial cells with abnormally high expression value but high expression in whole cells. In order to evaluate the role of RGS1 in tumorigenesis, we analyzed the expression levels of RGS1 between tumor and normal tissues in the TCGA database (Figure 3C). RGS1 expression was significantly upregulated in multiple cancers including BRCA, cholangiocarcinoma (CHOL), esophageal carcinoma (ESCA), glioblastoma multiforme (GBM), HNSC, kidney renal clear cell carcinoma (KIRC), kidney renal papillary cell carcinoma (KIRP), LUAD, rectal carcinoma (READ), and stomach adenocarcinoma (STAD) when compared with the normal samples. We also added and compared the expression levels of RGS1 across different cancer stages and found that RGS1 expression was associated with stage in some cancer types, such as RGS1 expression was higher in stages II, III, and IV vs. stage I in STAD. This result revealed that RGS1 was likely a key tumorigenesis regulator in multiple cancers and may be associated with prognosis. Conspicuously, the prognosis analysis was analyzed in 33 TCGA cancer types (Supplementary Table S4). RGS1 expression was significantly correlated to poor prognosis in seven cancers, including LIHC, adrenocortical carcinoma (ACC), pancreatic adenocarcinoma (PAAD), sarcoma (SARC), SKCM, STAD, and THYM (Figure 3D), suggesting that RGS1 was a potential prognostic factor in the survival of the above cancers. Apart from that, the protein level of RGS1 in HPA database showed the immunohistochemical (IHC) staining of RGS1 was negative staining in normal tissues and positive in liver cancer tissues, demonstrating that RGS1 was significantly expressed in cancer tissues than in normal liver tissues (Figure 3E). Additionally, we performed IHC verification to quantify the protein expression of RGS1 using the local clinical samples of liver cancer and normal tissues (Figures 3F, G), similarly, RGS1 protein displayed stronger staining in hepatocarcinoma, in line with the statistical result (p = 7.1e−5). The clinicopathological information of the patient samples and protein expression value are provided in Supplementary Table S5. These results showed that RGS1 was highly expressed in Tex cells in cancers, upregulated in tumor tissues in mRNA and protein level, and with poor prognosis in multiple cancers, which indicated its potential key role in T-cell exhaustion or cancer progress, and RGS1 might be an effective prognostic marker or a marker to identify Tex cells.
Correlation Between RGS1 and Candidate Gene Set
To discover the relationship between RGS1 and Candidate gene set as their potential roles in exhausted T cells, we calculated the correlation coefficient in single cell and tissue level (Figure 4). In three-cancer single-cell datasets, it was obvious that RGS1 showed positive correlation with 35 genes (up to 0.3~0.8), indicating that consistency of coexpression patterns between these genes. In the bulk RNA sequencing datasets, the correlation coefficients were almost positive, especially high with PDCD1, CTLA4, TNF receptor superfamily member 9 (TNFRSF9), HAVCR2, TOX, and TIGIT, which further confirmed the potential key roles of RGS1 in Tex cells. The negative correlations were mainly with the genes involved in cell cycle and DNA replication, including minichromosome maintenance complex component 7 (MCM7), enolase superfamily member 1 (ENOSF1), minichromosome maintenance complex component 5 (MCM5), minichromosome maintenance complex component 3 (MCM3), proliferating cell nuclear antigen (PCNA), which pointed out the different results observed between single cell and bulk RNA sequencing analysis, suggesting we could obtain deeper understanding about T-cell exhaustion by single-cell sequencing. One interpretation of the above inconsistent result is that the bulk transcriptome change (which mixes many immune and nonimmune cells together) likely reflects an overall expression in these cells and does not discriminate specific T-cell states, which further highlights the advantages in defining and studying T-cell exhaustion state by using single-cell sequencing data.
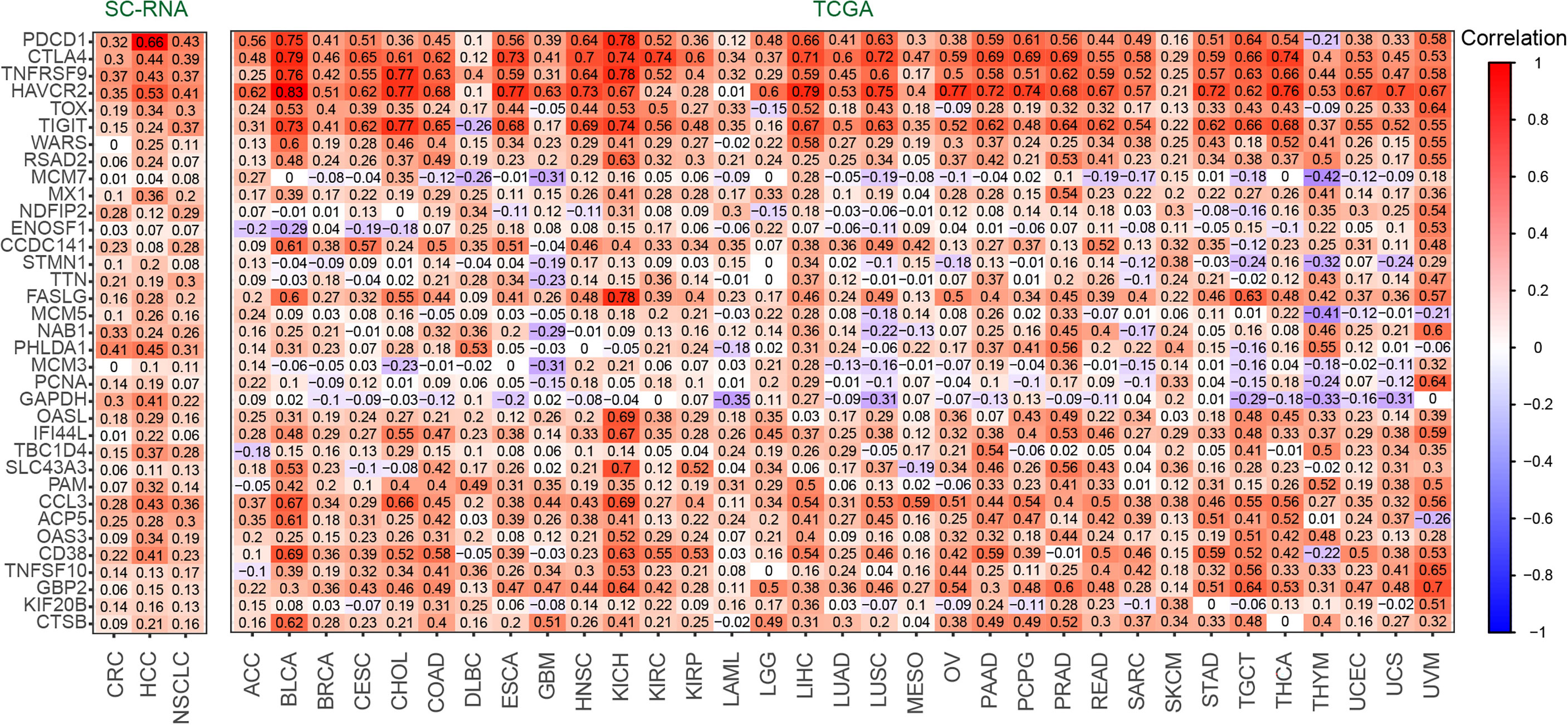
Figure 4 The correlation coefficient between RGS1 and Candidate gene set of Tex cells in CD8+ T cells (left) and TCGA database(right).
Discussion
T-cell exhaustion is characterized by loss of effector functions, continuously high expression of numerous inhibitory receptors, epigenetic and transcription profile changes, and dysregulated metabolism. Exhaustion CD8+ T cells are associated with suppressive immune microenvironment and poor overall survival in various cancer types, such as, in invasive bladder cancer (35) and clear-cell renal cell carcinoma (36). In addition, increased exhausted CD8+ T-cell subpopulations predict PD-1 blockade resistance response in melanoma (37). Accumulating evidences support exhausted T cells are possible to be rescued in cancer immunotherapy. Anti-PD1 antibodies, including atezolizumab and nivolumab, can renew the activity of exhausted CD8 T cells through preventing PD-1-mediated attenuation of proximal TCR cascades (38, 39) and can affect metabolic reprogramming to reinvigorate T cells (40). However, one study found an association between increased accumulation of one CD8+ T-cell exhaustion phenotype and clinical benefit, suggesting exhausted T cells may comprise heterogenous cell population with distinct responsiveness to intervention and the standard definition of exhaustion cells is unclear in the context of treatment (41). Thus, understanding molecular mechanism of T-cell exhaustion and comprehensively exploring potential markers associated with T-cell exhaustion is essential to precisely define T-cell exhaustion and establish rational immunotherapeutic interventions.
In this study, combining with single-cell RNA sequencing, which can facilitate to detect the transcriptome on the level of single cell (42), we can shed light on the complication of tumor-infiltrating T cells. In order to explore the key genes associated with T-cell exhaustion in multiple cancers, we performed transcriptomic analysis of single CD8+ T cells isolated from three cancers, including CRC, HCC, and NSCLC and identified different cell types, thereunto, Pre_exhaust and Tex cells overexpressed exhaustion markers and enriched in the negative regulation of immune progress. In the comparison with Teff cells, RGS1 showed almost the greatest fold change in Pre_exhaust and Tex cells of three cancers with poor prognosis and displayed highly positive correlation with the well-known genes associated with T-cell exhaustion.
In the WGCNA analysis, we identified a Candidate gene set consisting of 35 DEGs, including exhaustion markers such as PDCD1, CTLA4, HAVCR2, TOX, and TIGIT. Apart from that, genes involved in cell cycle and DNA replication also included, MCM7, MCM5, MCM3, PCNA, stathmin 1 (STMN1), titin (TTN), and TBC1 domain family member 4 (TBC1D4), suggesting that exhausted cells still retained the ability of proliferation, which was also observed in chronically infected models (43). Functionally, the Candidate gene set was able to distinguish Tex cells from the other subtypes of CD8+ T cells in different cancers, and higher GSVA scores of Candidate gene set showed poor prognosis in multiple cancers.
In addition, several DEGs appeared in Pre_exhaust and Tex cells, suggesting the role in the formation and development of T-cell exhaustion, in which RGS1 showed almost the greatest fold change. RGS1 encodes a member of the regulator of G-protein signaling family, which can act as a GTPase-activating protein (GAP), increasing the rate of conversion of the GTP to GDP, driving G-protein into its inactive GDP-bound form, hence attenuating or turning off G-protein-coupled receptor signaling (44). RGS1 is highly expressed in immune cells including T cells (45), B cells (46), natural killer (NK) cells (47), dendritic cells (48), and monocytes (49), suggesting a role for RGS1 in immune cell regulation. RGS1 inhibits the chemokine-induced lymphocyte migration (50) because chemokine-dependent activation of G-protein-coupled receptors can cause the activation of heterotrimeric G-protein subunits resulting in enhanced cell migration and adhesion (51), which has been found in Treg cells (45). In the present study, RGS1 was highly expressed in tumor tissues and correlated with shorter overall survival, which also appeared in several previous studies, including multiple myeloma (52), melanoma (53, 54), nonsmall cell lung cancer (55), gastric cancer (56), diffuse large B-cell lymphoma (57), and so on. However, the role of RGS1 in CD8+T cells especially in Tex cells has not been reported. In addition, RGS1 protein, located at the cytoplasm and membrane, is enriched in tumor tissues compared with normal tissues according to the IHC staining in the HPA database and verification experiment, further verifying its pathogenicity. Considering the ability to block cell migration of RGS1, we speculate that RGS1 can mediate the cell retention to lead to the persistent antigen stimulation of T cells, which resulted in T cell exhaustion with the overexpression of inhibitory genes such as PDCD1 and HAVCR2 (58). Additionally, RGS1 was identified as a HIF-dependent hypoxia target that dampens cell migration and signal transduction (59), indicating its role in exhausted T cells might be caused by hypoxia condition (Figure 1B).
RGS1, the most upregulated gene in Pre_exhaust and Tex cells and a potential marker for T-cell exhaustion, was excluded from the Candidate gene set. This phenomenon happened in other T cell-exhaustion-related genes as well, such as CD69 and CBLB. CD69, upregulated in Pre_exhaust and Tex cells, is an early activation marker of T cells (60). It can mediate the cell retention via the interaction with sphingosine-1-phosphate receptor 1 (S1PR1) which acts as a central mediator of lymphocyte output (61), leading to the persistent antigen stimulation of T cells, which resulted in T-cell exhaustion by overexpression of PDCD1 and HAVCR2 (62). CBLB, also upregulated in Pre_exhausted and Tex cells, whereby its deletion can inhibit CD8+ T cell exhaustion and promote chimeric antigen receptor T-cell function (34). Considering the positive correlation between RGS1 with Candidate gene set in single cells and tissues and its high expression in Tex cells, it is important and necessary to further study RGS1 mechanism in T-cell exhaustion.
In summary, our findings suggest that the GSVA score of the 35 Candidate gene set could be an effective prognostic marker or a marker to identify Tex cells. RGS1, as the most upregulated gene in Pre_exhaust and Tex cells, might play key roles in T-cell exhaustion or cancer progress. As a HIF-dependent hypoxia target, RGS1 might be upregulated by hypoxia, and further mediate the cell retention by inhibiting chemokine-induced lymphocyte migration. The current study could provide theoretical basis for research and immunotherapy of exhausted cells, while further studies are essential to fully elucidate the concrete mechanism of RGS1 during CD8+ T-cell exhaustion.
Data Availability Statement
The original contributions presented in the study are included in the article/Supplementary Material. Further inquiries can be directed to the corresponding authors.
Author Contributions
All authors contributed significantly to the work and the preparation of the manuscript. HD conceived the study. YB analyzed the data with assistance from ZC and wrote the manuscript. MH collected the data and performed IHC experiment. JW reviewed the manuscript. All authors contributed to the article and approved the submitted version.
Funding
This research was funded by the National Key R&D Program of China (2018YFC0910200), the Key R&D Program of Guangdong Province (2019B020226001).
Conflict of Interest
The authors declare that the research was conducted in the absence of any commercial or financial relationships that could be construed as a potential conflict of interest.
Publisher’s Note
All claims expressed in this article are solely those of the authors and do not necessarily represent those of their affiliated organizations, or those of the publisher, the editors and the reviewers. Any product that may be evaluated in this article, or claim that may be made by its manufacturer, is not guaranteed or endorsed by the publisher.
Supplementary Material
The Supplementary Material for this article can be found online at: https://www.frontiersin.org/articles/10.3389/fimmu.2021.767070/full#supplementary-material
Supplementary Figure 1 | Clustering of CD8+ T cells in three cancers. A. UMAP visualization of T cells in three cancers. B. The number of detected genes in each cell before filter in three cancers. C. The exhaustion scores of different cell types using the exhaustion gene list including HAVCR2, TIGIT, LAG3, PDCD1, CXCL13, LAYN, TOX, CTLA4, BTLA.
Supplementary Figure 2 | The correlation coefficient between RGS1 and Candidate gene set of Tex cells in CD8+ T cells of different patients.
Supplementary Figure 3 | The mRNA expression value of RGS1 of different stages in TCGA database.
Supplementary Table 1 | The patient ID and numbers of cell in three cancers.
Supplementary Table 2 | Differentially expressed genes of each cluster in three cancers.
Supplementary Table 3 | Differentially expressed genes of Pre_exhaust and Tex cells compared with Teff cells in three cancers.
Supplementary Table 4 | Survival analysis results of.RGS1 in different cancer types. Adj.p value referred to the adjusted p value corrected by multiple hypothesis testing (FDR).
Supplementary Table 5 | The clinicopathological information of the patient samples and RGS1 protein expression value.
References
1. Moskophidis D, Lechner F, Pircher H, Zinkernagel RM. Virus Persistence in Acutely Infected Immunocompetent Mice by Exhaustion of Antiviral Cytotoxic Effector T Cells. Nature (1993) 362(6434):758–61. doi: 10.1038/362758a0
2. Catakovic K, Klieser E, Neureiter D, Geisberger R. T Cell Exhaustion: From Pathophysiological Basics to Tumor Immunotherapy. Cell Communication Signaling Ccs (2017) 15(1):1. doi: 10.1186/s12964-016-0160-z
3. Lee PP, Yee C, Savage PA, Fong L, Brockstedt D, Weber JS, et al. Characterization of Circulating T Cells Specific for Tumor-Associated Antigens in Melanoma Patients. Nat Med (1999) 5(6):677–85. doi: 10.1038/9525
4. Dunn GP, Old LJ, Schreiber RD. The Immunobiology of Cancer Immunosurveillance and Immunoediting. Immunity (2004) 21(2):137–48. doi: 10.1016/j.immuni.2004.07.017
5. Pauken KE, Wherry EJ. Overcoming T Cell Exhaustion in Infection and Cancer. Trends Immunol (2015) 36(4):265–76. doi: 10.1016/j.it.2015.02.008
6. Schietinger A, Philip M, Krisnawan VE, Chiu EY, Delrow JJ, Basom RS, et al. Tumor-Specific T Cell Dysfunction Is a Dynamic Antigen-Driven Differentiation Program Initiated Early During Tumorigenesis. Immunity (2016) 45(2):389–401. doi: 10.1016/j.immuni.2016.07.011
7. Blackburn SD, Shin H, Haining WN, Zou T, Workman CJ, Polley A, et al. Coregulation of CD8+ T Cell Exhaustion by Multiple Inhibitory Receptors During Chronic Viral Infection. Nat Immunol (2009) 10(1):29. doi: 10.1038/ni.1679
8. Wagner A, Regev A, Yosef N. Revealing the Vectors of Cellular Identity With Single-Cell Genomics. Nat Biotechnol (2016) 34(11):1145. doi: 10.1038/nbt.3711
9. Winterhoff BJ, Maile M, Mitra AK, Sebe A, Bazzaro M, Geller MA, et al. Single Cell Sequencing Reveals Heterogeneity Within Ovarian Cancer Epithelium and Cancer Associated Stromal Cells. Gynecol Oncol (2017) 144(3):598–606. doi: 10.1016/j.ygyno.2017.01.015
10. Wu H, Yu J, Li Y, Hou Q, Zhou R, Zhang N, et al. Single-Cell RNA Sequencing Reveals Diverse Intratumoral Heterogeneities and Gene Signatures of Two Types of Esophageal Cancers. Cancer Lett (2018) 438:133–43. doi: 10.1016/j.canlet.2018.09.017
11. Papalexi E, Satija R. Single-Cell RNA Sequencing to Explore Immune Cell Heterogeneity. Nat Rev Immunol (2017) 18(1):35. doi: 10.1038/nri.2017.76
12. Darmanis S, Sloan SA, Croote D, Mignardi M, Chernikova S, Samghababi P, et al. Single-Cell RNA-Seq Analysis of Infiltrating Neoplastic Cells at the Migrating Front of Human Glioblastoma. Cell Rep (2017) 21(5):1399. doi: 10.1016/j.celrep.2017.10.030
13. Puram SV, Tirosh I, Parikh AS, Patel AP, Yizhak K, Gillespie S, et al. Single-Cell Transcriptomic Analysis of Primary and Metastatic Tumor Ecosystems in Head and Neck Cancer. Cell (2017) 171(7):1611–24 e24. doi: 10.1016/j.cell.2017.10.044
14. Tirosh I, Izar B, Prakadan SM, Treacy D, Trombetta JJ, Rotem A, et al. Dissecting the Multicellular Ecosystem of Metastatic Melanoma by Single-Cell RNA-Seq. Science (2016) 352(6282):189–96. doi: 10.1126/science.aad0501
15. Azizi E, Carr AJ, Plitas G, Cornish AE, Konopacki C, Prabhakaran S, et al. Single-Cell Map of Diverse Immune Phenotypes in the Breast Tumor Microenvironment. Cell (2018) 174(5):1293–308 e36. doi: 10.1016/j.cell.2018.05.060
16. Huang AC, Postow MA, Orlowski RJ, Mick R, Bengsch B, Manne S, et al. T-Cell Invigoration to Tumour Burden Ratio Associated With Anti-PD-1 Response. Nature (2017) 545(7652):60–5. doi: 10.1038/nature22079
17. Ziegenhain C, Vieth B, Parekh S, Reinius B, Guillaumet-Adkins A, Smets M, et al. Comparative Analysis of Single-Cell RNA Sequencing Methods. Mol Cell (2017) 65(4):631–43 e4. doi: 10.1016/j.molcel.2017.01.023
18. Zhang X, Lan Y, Xu J, Quan F, Zhao E, Deng C, et al. CellMarker: A Manually Curated Resource of Cell Markers in Human and Mouse. Nucleic Acids Res (2018) 47(D1):D721–D8. doi: 10.1093/nar/gky900
19. Aran D, Looney AP, Liu L, Wu E, Fong V, Hsu A, et al. Reference-Based Analysis of Lung Single-Cell Sequencing Reveals a Transitional Profibrotic Macrophage. Nat Immunol (2019) 20(2):163–72. doi: 10.1038/s41590-018-0276-y
20. Trapnell C, Cacchiarelli D, Grimsby J, Pokharel P, Li S, Morse M, et al. The Dynamics and Regulators of Cell Fate Decisions are Revealed by Pseudotemporal Ordering of Single Cells. Nat Biotechnol (2014) 32(4):381–6. doi: 10.1038/nbt.2859
21. Yu G, Wang LG, Han Y, He QY. Clusterprofiler: An R Package for Comparing Biological Themes Among Gene Clusters. Omics J Integr Biol (2012) 16(5):284–7. doi: 10.1089/omi.2011.0118
22. Brunson JC. Ggalluvial: Layered Grammar for Alluvial Plot. J Open Source Software (2020) 5(49):2017–23. doi: 10.21105/joss.02017
23. Langfelder P, Horvath S. WGCNA: An R Package for Weighted Correlation Network Analysis. BMC Bioinf (2008) 9:559. doi: 10.1186/1471-2105-9-559
24. Tosches MA, Yamawaki TM, Naumann RK, Jacobi AA, Tushev G, Laurent G. Evolution of Pallium, Hippocampus, and Cortical Cell Types Revealed by Single-Cell Transcriptomics in Reptiles. Science (2018) 360(6391):881–8. doi: 10.1126/science.aar4237
25. Hänzelmann S, Castelo R, Guinney J. GSVA: Gene Set Variation Analysis for Microarray and RNA-Seq Data. BMC Bioinf (2013) 14:7. doi: 10.1186/1471-2105-14-7
26. Jensen E. Quantitative Analysis of Histological Staining and Fluorescence Using ImageJ. Anatomical Rec (Hoboken NJ 2007) (2013) 296:378–81. doi: 10.1002/ar.22641
27. Zhang L, Yu X, Zheng L, Zhang Y, Li Y, Fang Q, et al. Lineage Tracking Reveals Dynamic Relationships of T Cells in Colorectal Cancer. Nature (2018) 564(7735):268–72. doi: 10.1038/s41586-018-0694-x
28. Zheng C, Zheng L, Yoo JK, Guo H, Zhang Y, Guo X, et al. Landscape of Infiltrating T Cells in Liver Cancer Revealed by Single-Cell Sequencing. Cell (2017) 169(7):1342–56 e16. doi: 10.1016/j.cell.2017.05.035
29. Guo X, Zhang Y, Zheng L, Zheng C, Song J, Zhang Q, et al. Global Characterization of T Cells in non-Small-Cell Lung Cancer by Single-Cell Sequencing. Nat Med (2018) 24(7):978–85. doi: 10.1038/s41591-018-0045-3
30. Carmona SJ, Siddiqui I, Bilous M, Held W, Gfeller D. Deciphering the Transcriptomic Landscape of Tumor-Infiltrating CD8 Lymphocytes in B16 Melanoma Tumors With Single-Cell RNA-Seq. Oncoimmunology (2020) 9(1):1737369. doi: 10.1080/2162402X.2020.1737369
31. Yost KE, Satpathy AT, Wells DK, Qi Y, Wang C, Kageyama R, et al. Clonal Replacement of Tumor-Specific T Cells Following PD-1 Blockade. Nat Med (2019) 25(8):1251–9. doi: 10.1038/s41591-019-0522-3
33. Mita Y, Kimura MY, Hayashizaki K, Koyama-Nasu R, Ito T, Motohashi S, et al. Crucial Role of CD69 in Anti-Tumor Immunity Through Regulating the Exhaustion of Tumor-Infiltrating T Cells. Int Immunol (2018) 30(12):559–67. doi: 10.1093/intimm/dxy050
34. Kumar J, Kumar R, Kumar Singh A, Tsakem EL, Kathania M, Riese MJ, et al. Deletion of Cbl-B Inhibits CD8(+) T-Cell Exhaustion and Promotes CAR T-Cell Function. J immunotherapy Cancer (2021) 9(1):e001688. doi: 10.1136/jitc-2020-001688
35. Liu Z, Zhou Q, Wang Z, Zhang H, Zeng H, Huang Q, et al. Intratumoral TIGIT(+) CD8(+) T-Cell Infiltration Determines Poor Prognosis and Immune Evasion in Patients With Muscle-Invasive Bladder Cancer. J Immunother Cancer (2020) 8(2):e000978. doi: 10.1136/jitc-2020-000978
36. Hu J, Chen Z, Bao L, Zhou L, Hou Y, Liu L, et al. Single-Cell Transcriptome Analysis Reveals Intratumoral Heterogeneity in ccRCC, Which Results in Different Clinical Outcomes. Mol Ther (2020) 28(7):1658–72. doi: 10.1016/j.ymthe.2020.04.023
37. Peng DH, Rodriguez BL, Diao L, Chen L, Wang J, Byers LA, et al. Collagen Promotes Anti-PD-1/PD-L1 Resistance in Cancer Through LAIR1-Dependent CD8+ T Cell Exhaustion. Nat Commun (2020) 11(1):4520. doi: 10.1038/s41467-020-18298-8
38. Herbst RS, Soria JC, Kowanetz M, Fine GD, Hamid O, Gordon MS, et al. Predictive Correlates of Response to the Anti-PD-L1 Antibody MPDL3280A in Cancer Patients. Nature (2014) 515(7528):563–7. doi: 10.1038/nature14011
39. Herbst RS, Soria JC, Kowanetz M, Fine GD, Hamid O, Gordon MS., et al PD-1 Blockade Induces Responses by Inhibiting Adaptive Immune Resistance. Nature (2014) 515(7528):568. doi: 10.1038/nature14011
40. Bengsch B, Johnson AL, Kurachi M, Odorizzi PM, Pauken KE, Attanasio J, et al. Bioenergetic Insufficiencies Due to Metabolic Alterations Regulated by the Inhibitory Receptor PD-1 Are an Early Driver of CD8(+) T Cell Exhaustion. Immunity (2016) 25(3):358–73. doi: 10.1016/j.immuni.2016.07.008
41. Huang AC, Orlowski RJ, Xu X, Mick R, George SM, Yan PK, et al. A Single Dose of Neoadjuvant PD-1 Blockade Predicts Clinical Outcomes in Resectable Melanoma. Nat Med (2019) 25(3):454–61. doi: 10.1038/s41591-019-0357-y
42. Pollen AA, Nowakowski TJ, Shuga J, Wang X, Leyrat AA, Lui JH, et al. Low-Coverage Single-Cell mRNA Sequencing Reveals Cellular Heterogeneity and Activated Signaling Pathways in Developing Cerebral Cortex. Nat Biotechnol (2014) 32(10):1053–8. doi: 10.1038/nbt.2967
43. Paley MA, Kroy DC, Odorizzi PM, Johnnidis JB, Dolfi DV, Barnett BE, et al. Progenitor and Terminal Subsets of CD8+ T Cells Cooperate to Contain Chronic Viral Infection. Science (2012) 338(6111):1220–5. doi: 10.1126/science.1229620
44. Watson N, Linder ME, Druey KM, Kehrl JH, Blumer KJ. RGS Family Members: GTPase-Activating Proteins for Heterotrimeric G-Protein Alpha-Subunits. Nature (1996) 383(6596):172–5. doi: 10.1038/383172a0
45. Agenes F, Bosco N, Mascarell L, Fritah S, Ceredig R. Differential Expression of Regulator of G-Protein Signalling Transcripts and In Vivo Migration of CD4+ Naive and Regulatory T Cells. Immunology (2005) 115(2):179–88. doi: 10.1111/j.1365-2567.2005.02146.x
46. Moratz C, Kang VH, Druey KM, Shi CS, Scheschonka A, Murphy PM, et al. Regulator of G Protein Signaling 1 (RGS1) Markedly Impairs Gi Alpha Signaling Responses of B Lymphocytes. J Immunol (2000) 164(4):1829–38. doi: 10.4049/jimmunol.164.4.1829
47. Kveberg L, Ryan JC, Rolstad B, Inngjerdingen M. Expression of Regulator of G Protein Signalling Proteins in Natural Killer Cells, and Their Modulation by Ly49A and Ly49D. Immunology (2005) 115(3):358–65. doi: 10.1111/j.1365-2567.2005.02174.x
48. Shi GX, Harrison K, Han SB, Moratz C, Kehrl JH. Toll-Like Receptor Signaling Alters the Expression of Regulator of G Protein Signaling Proteins in Dendritic Cells: Implications for G Protein-Coupled Receptor Signaling. J Immunol (2004) 172(9):5175–84. doi: 10.4049/jimmunol.172.9.5175
49. Denecke B, Meyerdierks A, Bottger EC. RGS1 is Expressed in Monocytes and Acts as a GTPase-Activating Protein for G-Protein-Coupled Chemoattractant Receptors. J Biol Chem (1999) 274(38):26860–8. doi: 10.1074/jbc.274.38.26860
50. Han JI, Huang NN, Kim DU, Kehrl JH. RGS1 and RGS13 mRNA Silencing in a Human B Lymphoma Line Enhances Responsiveness to Chemoattractants and Impairs Desensitization. J Leukoc Biol (2006) 79(6):1357–68. doi: 10.1189/jlb.1105693
51. Offermanns S, Simon MI. Organization of Transmembrane Signalling by Heterotrimeric G Proteins. Cancer Surv (1996) 27:177–98. doi: 10.3109/07357909609076911
52. Roh J, Shin SJ, Lee AN, Yoon DH, Suh C, Park CJ, et al. RGS1 Expression is Associated With Poor Prognosis in Multiple Myeloma. J Clin Pathol (2017) 70(3):202–7. doi: 10.1136/jclinpath-2016-203713
53. Kashani-Sabet M, Nosrati M, Miller JR, Sagebiel RW, Leong SPL, Lesniak A, et al. Prospective Validation of Molecular Prognostic Markers in Cutaneous Melanoma: A Correlative Analysis of E1690. Clin Cancer Res (2017) 23(22):6888–92. doi: 10.1158/1078-0432.ccr-17-1317
54. Rangel J, Nosrati M, Leong SP, Haqq C, Miller JR 3rd, Sagebiel RW, et al. Novel Role for RGS1 in Melanoma Progression. Am J Surg Pathol (2008) 32(8):1207–12. doi: 10.1097/PAS.0b013e31816fd53c
55. Dai J, Gu J, Lu C, Lin J, Stewart D, Chang D, et al. Genetic Variations in the Regulator of G-Protein Signaling Genes are Associated With Survival in Late-Stage non-Small Cell Lung Cancer. PloS One (2011) 6(6):e21120. doi: 10.1371/journal.pone.0021120
56. Li S, Yang H, Li S, Zhao Z, Wang D, Fu W. High Expression of Regulator of G-Protein Signalling 1 is Associated With the Poor Differentiation and Prognosis of Gastric Cancer. Oncol Lett (2021) 21(4):322. doi: 10.3892/ol.2021.12584
57. Carreras J, Kikuti YY, Beà S, Miyaoka M, Hiraiwa S, Ikoma H, et al. Clinicopathological Characteristics and Genomic Profile of Primary Sinonasal Tract Diffuse Large B Cell Lymphoma (DLBCL) Reveals Gain at 1q31 and RGS1 Encoding Protein; High RGS1 Immunohistochemical Expression Associates With Poor Overall Survival in DLBCL Not Otherwise Specified (NOS). Histopathology (2017) 70(4):595–621. doi: 10.1111/his.13106
58. Blank CU, Haining WN, Held W, Hogan PG, Kallies A, Lugli E, et al. Defining 'T Cell Exhaustion'. Nat Rev Immunol (2019) 19(11):665–74. doi: 10.1038/s41577-019-0221-9
59. Wierenga AT, Vellenga E, Schuringa JJ. Convergence of Hypoxia and Tgfβ Pathways on Cell Cycle Regulation in Human Hematopoietic Stem/Progenitor Cells. PloS One (2014) 9(3):e93494. doi: 10.1371/journal.pone.0093494
60. Ziegler SF, Ramsdell F, Hjerrild KA, Armitage RJ, Grabstein KH, Hennen KB, et al. Molecular Characterization of the Early Activation Antigen CD69: A Type II Membrane Glycoprotein Related to a Family of Natural Killer Cell Activation Antigens. Eur J Immunol (1993) 23(7):1643–8. doi: 10.1002/eji.1830230737
61. Cyster JG, Schwab SR. Sphingosine-1-Phosphate and Lymphocyte Egress From Lymphoid Organs. Annu Rev Immunol (2012) 30:69–94. doi: 10.1146/annurev-immunol-020711-075011
Keywords: single-cell transcriptome, T-cell exhaustion, multiple cancers, RGS1, poor prognosis
Citation: Bai Y, Hu M, Chen Z, Wei J and Du H (2021) Single-Cell Transcriptome Analysis Reveals RGS1 as a New Marker and Promoting Factor for T-Cell Exhaustion in Multiple Cancers. Front. Immunol. 12:767070. doi: 10.3389/fimmu.2021.767070
Received: 30 August 2021; Accepted: 15 November 2021;
Published: 08 December 2021.
Edited by:
Jin S. Im, University of Texas MD Anderson Cancer Center, United StatesReviewed by:
Hussein A. Abbas, MD Anderson Cancer Center, United StatesWilliam J. Magner, University at Buffalo, United States
Copyright © 2021 Bai, Hu, Chen, Wei and Du. This is an open-access article distributed under the terms of the Creative Commons Attribution License (CC BY). The use, distribution or reproduction in other forums is permitted, provided the original author(s) and the copyright owner(s) are credited and that the original publication in this journal is cited, in accordance with accepted academic practice. No use, distribution or reproduction is permitted which does not comply with these terms.
*Correspondence: Jinfen Wei, weijinfen@scut.edu.cn; Hongli Du, hldu@scut.edu.cn
†These authors have contributed equally to this work