- 1Department of Rheumatology, Peking Union Medical College Hospital, Peking Union Medical College & Chinese Academy of Medical Sciences, Key Laboratory of Rheumatology & Clinical Immunology, Ministry of Education, Beijing, China
- 2Department of Rheumatology, National Clinical Research Center for Dermatologic and Immunologic Diseases (NCRC-DID), Beijing, China
- 3Department of Pharmacology and Molecular Sciences, Johns Hopkins University School of Medicine, Baltimore, MD, United States
- 4Department of Rheumatology, Aerospace Center Hospital, Aerospace, Clinical Medical College, Peking University, Beijing, China
Dysregulated autoantibodies and cytokines were deemed to provide important cues for potential illnesses, such as various carcinomas and autoimmune diseases. Increasing biotechnological approaches have been applied to screen and identify the specific alterations of these biomolecules as distinctive biomarkers in diseases, especially autoimmune diseases. As a versatile and robust platform, protein microarray technology allows researchers to easily profile dysregulated autoantibodies and cytokines associated with autoimmune diseases using various biological specimens, mainly serum samples. Here, we summarize the applications of protein microarrays in biomarker discovery for autoimmune diseases. In addition, the key issues in the process of using this approach are presented for improving future studies.
Introduction
Autoimmune diseases (AIDs) are a group of diseases characterized by disordered stimulation of self-reactive immune response attacking one's own organ or tissues. Autoantibodies play a vital role in the pathogenesis of AIDs and have been tested in clinic as biomarkers for diagnosis, prognosis, as well as patient stratification (1). In addition, alteration of cytokines, chemokines, protein glycosylation, and other molecules also provides bundles of valuable information to evaluate the progress of AIDs. However, the sensitivity and specificity of some routine tests (e.g., Antinuclear antibodies) cannot satisfy the diagnosis requirement for some common AIDs such as systemic lupus erythematosus (SLE), systemic sclerosis (SS), and rheumatoid arthritis (RA). Furthermore, there are no specific biomarkers for some AIDs, especially the ones with low incidence. More recently, protein microarray technology has attracted increasing attention from scientists working to discover novel biomarkers in various diseases. Compared to traditional techniques such as mass spectrometry, protein microarray technology is a robust and versatile platform that has the benefit of requiring only small quantities of protein to simultaneously survey a large variety of analytes in crude samples (2). For this reason, protein microarrays are a highly useful tool in biomarker discovery.
Protein microarray technology was inspired by the previously developed DNA microarray technology, which immobilizes hundreds of thousands of oligonucleosides onto a small glass slide and provides a high-throughput method to study the alteration of gene expression in cells or tissues. Similarly, protein microarrays are constructed by immobilizing large numbers of various proteins, peptides, antibodies, lectins, cell or tissue lysates. Based on the application, protein microarrays can be categorized as either an analytical array or a functional array (3). Usually, an analytical array is constructed with well-characterized affinity reagents such as antibodies to detect and quantify specific proteins (4). Functional arrays are assembled with purified recombinant proteins or peptide fragments and can be used in discovery-based studies, including studying protein-protein, protein-lipid, protein-DNA, protein-drug, and protein-peptide interactions (5). Alternatively, protein probes could also be printed onto a planar or bead-based platform (6). Based on the probes utilized on the array, protein microarrays can be categorized into antigen arrays, antibody arrays, peptide arrays, lectin array, etc. In addition to the precast recombinant proteins or cell lysates, protein probes could be synthesized in situ using cell-free expression systems based on printed plasmid DNA. Protein arrays assembled by this strategy are called Nucleic Acid-Programmable Protein Arrays (NAPPAs) (7). To date, a variety of both lab-made and commercial microarrays have been widely used to identify potential biomarkers for various diseases. In this review, we will focus on the application of protein microarrays in AID biomarker discovery, as well as the key issues that are often encountered in the process of using protein microarrays. An overview of the various protein microarrays that are commonly utilized is listed in Table 1 and their applications with various AIDs are summarized in Table 2.
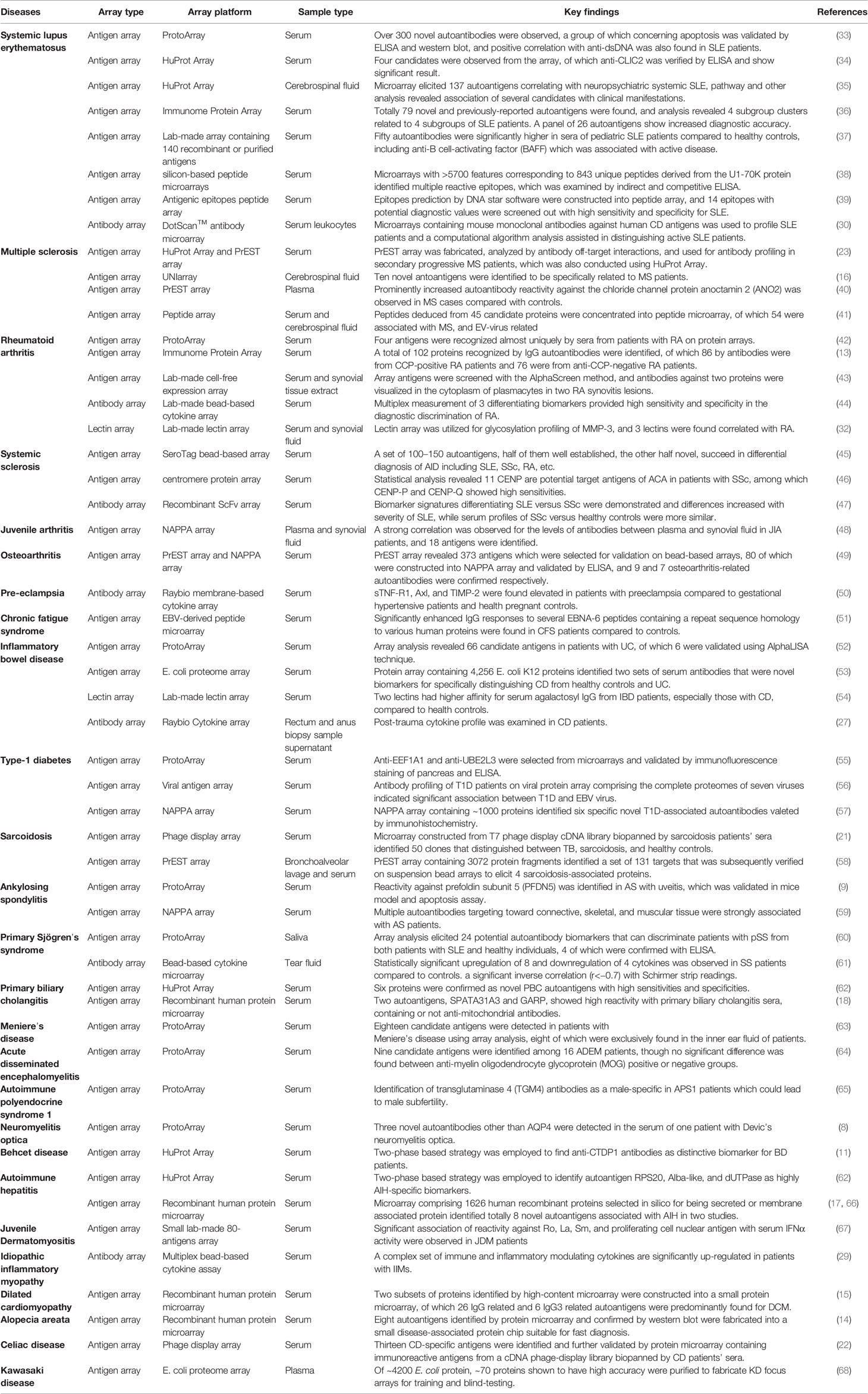
Table 2 Selective application of protein microarrays in biomarker discovery for autoimmune diseases.
Development of Antigen Arrays
Antigen arrays are very popular and widely used in autoantibody screening, especially for AIDs. Various types of antigens, from a focused selection of disease associated candidate proteins/peptides to the proteome of some organelle or even species, could be immobilized on slides to prepare the antigen array and screen the autoantibodies in diverse patients. Joos et al. first introduced the idea of antigen microarray in research of AIDs, where eighteen well-known autoantigens related to SLE and other AIDs were deposited onto nitrocellulose membranes and examined in reaction with patient sera (69). Later, Robinson et al. built a 1152-feature Connective Tissue Disease (CTD) array including 196 putative autoantigens spotted onto glass slides (70). Both studies confirmed that protein microarrays are capable of higher sensitivity than ELISA. In recent years, similar multiplex approaches were developed to screen specific autoantibody biomarkers for various AIDs, such as rheumatoid diseases (71, 72), type 1 diabetes (73), lupus nephritis (74), multiple sclerosis (75, 76), systemic sclerosis (46), SLE (37, 77, 78), juvenile dermatomyositis (67), and Sjogren's syndrome (79). Importantly, most of these studies included dozens to several hundreds of autoantigens. In addition, a multiplexed, bead-based system was developed that is also suitable for array construction and detection for this application (79). However, only a few of the most well-studied autoantigens were used to construct this detection system for screening autoantibodies in patients' sera.
Proteome Microarrays
In order to comprehensively analyze the interactions of biomolecules, such as protein-protein interactions, protein-small molecules interactions, and kinase-substrate interactions, MacBeath et al. developed the functional proteome array (80). Following the advent of this technology, Zhu et al. subsequently fabricated a yeast proteome chip containing 5,800 GST-tagged recombinant yeast proteins, and subjected it to screening of protein-drug interactions and detection of posttranslational modifications (81). The idea was later commercialized as ProtoArray® Human Protein Microarrays by Invitrogen™ (now under Thermo Fisher Scientific) and contained over 9,000 full-length human proteins. To date, the ProtoArray® Human Protein Microarrays have been widely used in identification of new biomarkers for many AIDs, including neuromyelitis optica (8), RA (42), primary Sjögren's syndrome (60), chronic renal disease (82), inflammatory bowel disease (52), type 1 diabetes (55), Meniere's Disease (63), autoimmune polyendocrine syndrome type 1 (65), acute disseminated encephalomyelitis (64), ulcerative colitis (83), SLE (33), and ankylosing spondylitis (9). In addition to the ProtoArray® Human Protein Microarrays, several new commercial proteome arrays have become available with additional protein probes using different expression systems. The HuProt™ Human Proteome Microarray V1.0 was designed by Zhu lab at Johns Hopkins School of Medicine and produced by CDI Laboratories around 2012, containing 16,368 recombinant GST-His-tagged human proteins expressed in S. cerevisiae (10), and the current v4.0 contains more than 21,000 unique proteins. To date, the HuProt array has been widely used to screen novel autoantigen biomarkers of AIDs, such as autoimmune hepatitis (84), SLE (34), primary biliary cirrhosis (62), neuropsychiatric lupus (35), multiple sclerosis (23), and Behcet disease (11). Another high-density commercial array is the IMMUNOME™ v4 Discovery Array, which was designed by Sengenics using more than 1,600 full-length human proteins, including kinases, signaling molecules, cytokines, interleukins, and chemokines. On the IMMUNOME™ v4 Discovery Array, all proteins are tagged with biotin carboxyl carrier protein (BCCP), which acts as a folding marker and solubility enhancer (12). McAndrew et al. applied the IMMUNOME™ array in autoantibody profiling with SLE serum samples (36, 85). Similarly, Poulsen et al. investigated the global reactivity of autoantibodies in RA patients (13). Additionally, Horn et al. constructed a special array constructed with large cohorts of disease-associated proteins, consisting of around 37,200 redundant, recombinant human proteins derived the E. coli expression system (14, 15). Then, serum profiling of alopecia areata and dilated cardiomyopathy patients were conducted by Beyer et al. to identify novel disease biomarkers. This approach was later commercialized into UNIarray® by Protagen Diagnostics and used to profile biomarkers in the cerebrospinal fluid of multiple sclerosis patients (16). Grifantini et al. selected 1,626 membrane-associated and secreted proteins from public resources to assemble a special microarray and screened biomarkers in autoimmune hepatitis and primary biliary cholangitis patients (17, 18, 66). The latest type of proteome array is SeroTag from Luminex Corporation. In this array, over 7,000 human proteins as antigens are immobilized onto bead-based suspension arrays, and it was used to screen novel biomarkers in SLE, SSc, and RA patients with promising results (45).
Nucleic Acid Programmable Protein Arrays
An alternative way to produce protein probes on protein microarrays is in situ expression using cDNAs coding tagged recombinant proteins which are adjacently printed with the tag specific antibodies on a slide. Incubating with the In Vitro Transcription and Translation (IVTT)-coupled cell lysates allows cDNAs to synthesize the target proteins, followed by immobilization by the tag specific antibodies. For example, Sawasaki et al. developed a cell-free protein synthesis system using the eukaryotic translation apparatus of wheat seeds (86), and generated a protein microarray containing over 2,000 proteins. They used this array to screen autoantigens associated with rheumatoid synovitis and lupus nephritis (43, 87). To date, Nucleic Acid Programmable Protein Array (NAPPA) is perhaps the most famous protein microarray adopting this strategy, on which all captured proteins were C-terminal GST tagged (19). The popular commercial NAPPA array was designed and generated by the Protein Array Core of the BioDesign Institute, and it contains more than 12,000 human proteins (88). It can also be prepared according to a customer's requirements. NAPPA arrays have been employed to identify autoantibody biomarkers for several AIDs, including ankylosing spondylitis (59), juvenile arthritis (48), osteoarthritis (49), and type 1 diabetes (20, 57). In addition, because all the protein probes on the NAPPA array were translated by the IVTT-coupled cell lysates system instead of being expressed and subjected to high-throughput purification prior to immobilization on the array, this allows the NAPPA array to be quickly and easily produced, especially when using protein probes that are more difficult to express and purify with traditional methods. Also, NAPPA arrays provide a rapid method to prepare a pathogenic proteome array for analyzing the outbreak of infectious diseases or some AIDs where infection may contribute to their pathogenesis. Bian et al. developed a viral microarray containing 761 antigens from 25 viruses based on NAPPA and used it to study anti-virus antibodies in juvenile idiopathic arthritis and type 1 diabetes patients (56, 89).
Pathogenic Proteome Arrays
Aside from NAPPA, pathogenic proteome arrays can be constructed using traditional expression systems and applied in the screening of the reactive antibodies in pathogen induced diseases. For instance, Chen et al. constructed a bacterial proteome microarray composed of 4,256 proteins encoded by the E. coli K12 strain and subjected it to the investigation of biomarkers for inflammatory bowel disease (53). Meanwhile, Kuo et al. created an E. coli proteome microarray with around 4,200 proteins and analyzed the antibody spectrum in pre-eclamptic women (90) and patients with Kawasaki disease (68). Loebel et al. also employed an EBV-derived peptide microarray to profile the serological response in chronic fatigue syndrome (51). Furthermore, phage display libraries can provide an alternative method for protein microarray construction. Talwar et al. created an T7 phage display immune-cell cDNA array that was bio-panned to obtain 1152 potential sarcoidosis antigens, and a microarray was constructed to test the sera from sarcoidosis and tuberculosis patients (21). A similar method was adopted by D'Angelo et al. in profiling celiac disease antibodies (22).
HPA Protein Fragment Arrays
The Human Protein Atlas (HPA) is an antibody-based proteomics database containing abundant of information about protein expression and localization in various cells and tissues, under both normal and diseased conditions (24). In order to select each protein's unique fragments, Protein Epitope Signature Tags (PrESTs) were developed by Berglund et al. (91). The HPA protein fragment arrays were constructed using these PrEST fragments, including over 42,000 unique purified fragments deriving from ~20,000 human proteins (23). Compared with the HuProt™ Human proteome microarray, the HPA protein fragment array has demonstrated better performance because they have much fewer off-target interactions. Therefore, the HPA protein fragment array is a valuable method to identify autoantibody targets with higher specificity in various autoimmune disease (23). Using similar strategy, Uhlen et al. produced an array containing 11,520 protein fragments with planar or bead-base methods and subjected them to antibody screening in multiple sclerosis (40, 92). Additionally, PrEST arrays of different sizes were also applied for osteoarthritis, sarcoidosis, and SLE patients (25, 49, 58).
Peptide Microarrays
In addition to protein microarrays constructed using full-length or truncated proteins, peptide arrays have also been developed using hundreds to thousands of peptides that were pre-synthesized or in situ synthesized directly on a surface. To date, peptide arrays have been applied in screening autoantibodies in AIDs, various cancers, and other diseases. Compared with full-length proteins, peptides are much cheaper, more stable, and allow for a higher capability of modification, such as acetylation or methylation. Generally, the high density peptides on the array are in situ synthesized using a semiconducting photolithography approach (93). The RepliTope™ Peptide Microarray, containing large random-sequences, was reported to identify potential peptide biomarkers in patients with lupus and determine its central nervous system (CNS) manifestations (26). In addition, peptides derived from the specific epitopes of disease-related proteins could be used to fabricate a disease-specific microarray to investigate antibody response at up to single-amino acid resolution. For instance, Haddon et al. developed a silicon-based peptide microarray, composed of over 5,700 features corresponding to 843 unique peptides derived from the U1-70K protein, and applied it in screening specific autoantibodies in SLE (38). Hecker et al. employed 3,747 peptides deduced from 45 candidate proteins to construct a high-density peptide microarray, and performed the analysis of IgG autoantibody reactivity in serum and cerebrospinal fluid of multiple sclerosis (41). Recently, Li et al. constructed a peptide array, including 73 potential antigenic epitopes in 14 autoantigens and subjected it to evaluate potential diagnostic values for each epitope in SLE patients (39).
Antibody Microarrays
In addition to autoantibodies, some other dysregulated biomolecules in disease progression, such as cytokines, chemokines, growth factors, and mRNAs, are considered reliable sensors of AIDs. Sandwich ELISA is a well-developed and popular tool in semi-quantitative or quantitative measurement of target molecules using high quality antibodies. Following need for more monoclonal antibodies with high-affinity and specificity, antibody arrays were constructed to test hundreds of targets simultaneously and greatly expanded the detection of target objects. In particular, the cytokine antibody arrays are extensively used in the field of AIDs, because the cytokines are not only indicators but also therapeutic targets of these diseases (94). One of these earliest arrays was designed by Kader et al., and it was used to investigate 78 different cytokines and growth factors in pediatric patients with IBD (95). A similar approach was also adopted for use in other diseases such as SLE, RA, and MS (96). In recent years, a series of commercial antibody arrays was developed by RayBiotech, including human biomarkers, cytokines, inflammatory factors, and growth factors. For AIDs, the RayBio arrays were successfully applied in various diseases, including Crohn's disease (27), preeclampsia (50), and SLE (28, 97, 98). More recently, incorporation of anti-cytokines antibodies into multiplexed bead-based assays is becoming increasing popular, and this type of analysis has been performed in several different AIDs such as idiopathic inflammatory myopathy, RA, and Sjogren's Syndrome (29, 44, 61, 99). Currently, most of antibodies on the array are well-characterized monoclonal antibodies. However, recombinant antibodies are also explored and applied to construction of antibody microarrays. Carlsson et al. fabricated scFv microarrays targeting immune regulatory proteins and applied them in serum profiling of SLE and SS patients (47). Apart from studying cytokines, antibody arrays could also be used to measure different antigens involved in immune dysregulation. For instance, Lin et al. utilized an antibody array that consists of 82 mouse monoclonal antibodies against human cluster of differentiation (CD) antigens to investigate leucocyte reaction for SLE diagnosis (30). A potential disadvantage of antibody microarrays is the specificity of the antibodies with more abundant off-target proteins (100). Thus, acquisition of high-quality antibodies with high affinity and specificity are essential for biomarker identification.
Lectin Arrays
Considering over half of proteins are glycosylated in vivo, the study of glycoproteomic alteration under different physio-pathological states may contribute to the discovery of disease associated biomarkers (31). Lectins, a group of carbohydrate-binding proteins specific binding different glycans could be used to assemble the lectin microarrays to profile glycosylation in tissues. Regarding AIDs, lectin arrays have been applied to identify and monitor glyco-biomarkers in IBD and RA (32, 54). Hopefully in the future, the progress of lectin microarray technology may contribute to its broader applications in AIDs.
Key Issues of Protein Microarrays in Aid Biomarker Discovery
Although a number of review papers have been published on protein microarrays, where general illustrations of their classifications, experimental procedures, and applications could be found (5, 7, 24, 101, 102), it still seems to be rare to find a comprehensive discussion on the key issues when using protein microarrays in AID biomarker discovery. Such issues can include biosample collection, optimization of experimental design with consideration for minimized cost, choice of different protein arrays, data analysis, novel biomarker validation, and even the technical transference to clinic lab tests. Addressing each of the challenges would be helpful in improving the application of protein microarrays in future studies.
Bio-Sample Collection
Sample collection is the first and most critical step in the identification of AID biomarkers using protein microarrays. Ideally, patients with clinical manifestations specific to one AID would be recommended for protein microarray experiments. However, AIDs could be complicated in terms of pathogenesis and progression, and some patients may encounter more than one disease. Moreover, age, gender, population, and other demographic variables may further contribute to the heterogeneity of patient groups, which might elicit contradictory results across different studies.
For some well-characterized AIDs, a key consideration is sample stratification. Patients could be grouped by multiplexed AID manifestations, primary or secondary pathogenesis, distinct immunophenotypes, etc. based on previous literature, and the discovery of protein microarrays may in turn assist in disease subclassification (103, 104). Another consideration is the sampling time. Longitudinal prospective studies using certain biomarker panels could be applied in disease prevention, activity monitoring, as well as prognosis following treatments (105–107). Furthermore, additional large cohorts of samples should be collected to prove any novel discoveries. For some AIDs with low incidence rates, on the other hand, sample collection is often driven by availability. Thus, collaboration between several large medical institutes or hospitals is strongly recommended to obtain sufficient samples under consistent criteria. Usually, researchers are inclined to make use of all available patients, which means the samples used in the development stages were recruited in validation assays.
In addition to serum samples, which are the most popular sources used to screen auto-antibodies for AIDs, other samples such as urine, cerebral fluid, or other body fluids could be collected, as distinct sets of biomarkers might exist corresponding to differentially impaired organs (35, 43, 58, 61).
Experimental Design for Cost-Effective Protein Microarray Assays
Considering the high cost of a commercial protein microarray, especially a proteome microarray containing tens of thousands of recombinant protein probes, experimental design with consideration for cost-effectiveness would be necessary for a screening assay with a large cohort of samples. A design with two-phases has more recently attracted researchers' attention (84). In phase I of this design, a small collection of samples was randomly selected for a screening assay with a high-density proteome array to identify candidate biomarkers with relative loose criteria. While in phase II, these candidate probes were used to construct a disease focused mini array, which contains more than ten blocks on one slide and can be a cost-effective way to evaluate samples in large cohorts. To date, this strategy has been adapted to a variety of different studies (11, 35, 62, 108). In addition, some scientists have performed this screening assay using mixtures of several samples with similar conditions on limited proteome arrays (70). However, the results from mixture samples might produce a higher possibility of losing information on targets with lower affinity or titers.
Considerations in Design of Protein Microarray Experiments
Various aspects of protein microarrays, such as the protein probes utilized, the chemical modifications on the microarray surface, and the fabrication and detection methods, could impact the result and even the transition to clinical experiments regardless of whether the array is lab-made or commercial (109–112). As mentioned above, both full-length proteins and peptides can be supplied as the primary materials for protein microarrays. Generally, full-length proteins are expected to remain in their original in vivo structure in order to mimic the authentic interaction with autoantibodies or other molecules (113–115), while peptides could provide much more information to elucidate specific binding epitopes (116–120). Thus, it might be reasonable to use full-length protein arrays for initial narrowing (phase I) of the target antigens, and then adopt a more cost-effective synthetic peptide arras to further evaluate the binding sites (phase II), though this strategy might require collaboration between several institutes or companies. On the other hand, a self-developed array could focus the antigens associated with the specific disease [e.g. and choose some pathogen antigens, especially in the assay with microbiome-related AIDs (89)]. In addition to antigen arrays, antibody arrays are more attractive in profiling dysregulated cytokines or other known disease-related molecules in serum or other samples in a quantitative fashion (121–124). Meanwhile, when considering glycosylated target molecules, lectin arrays could bring the knowledge of autoantibody-autoantigen interactions to a new level (125, 126). However, extending the scope of the probes, including proteins or peptides with various post-translational modifications or aptamers, could refine and increase the range of identified biomarkers (24). For instance, the affinity-based slow off-rate modified aptamer (SOMAmer) technology, which has been applied in proteomic profiling across various diseases and health states, could also be viable for AIDs (127).
In addition, the properties of slide surface are critical to the performance of protein probes. Achieving a high-density, robust immobilization method while retaining proper folding and orientation of the protein are the common requirements for various slides. Covalent (i.e., SuperEpoxy Slide) or noncovalent (i.e., FAST® and PATH® Slide) chemistries are both available for commercial protein microarray slides. Besides the planar or bead-based surface, a 3-dimensional matrix on a glass surface would help to maintain the proteins structure and also support additional space for immobilizing more proteins (102).
Fluorescent dyes and radioisotopes are two common regents in detection of binding activities on a protein microarray. Fluorescent dye labeled reagents enable reliable detection with high signals and low background. However, fluorescent labeling may interrupt protein structure and even its activities, which could impact the results of the array. Though radioisotope labeling has overcome this fault, this kind of labeling results in much higher background signals. Novel label-free detection approaches such as surface plasmon resonance, carbon nanotubes, reflective phantom interface, etc. are expected to address the problem with greater sensitivity (101).
Data Analysis and Novel Biomarker Validation
A general outline of data analysis for identifying biomarkers using protein microarray involves data acquisition, pre-processing, bioinformatic analysis, and differential target selection (128). To narrow down the scope of targets for further validation, data processing and mining are crucial in identifying biomarker candidates, especially when dealing with large amounts of raw data from high-density proteome microarrays. Commercial human proteome microarrays usually entail a respective processing method, such as ProtoArray Prospector Software for ProtoArray®. Most methods have been adopted from a similar one that is used in DNA microarray technology. A consensus bioinformatics procedure has still not been achieved among researchers working on protein microarrays. With regard to biomarker discovery, retrieving a suitable amount of biologically meaningful biomarkers demands special feature selection methods (129). Computational algorithm analysis could facilitate selection of appropriate biomarker profiles with better predictive accuracy (30). For data sharing, online databases such as Protein Microarray Database (130), AAgMarker 1.0 (131), and AAgAtlas 1.0 (132) offer a comprehensive platform to overview data from protein microarrays as well as various candidate autoantibody biomarkers in different diseases. To validate the identified novel biomarkers, traditional methods such as ELISA and immunoblotting would be preferred because of their higher compatibility with the current popular methods in lab tests in hospitals. Success with validation means there would be a much higher probability of technology conversion and the following industrial manufacture in the downstream.
Conclusion
Autoimmune diseases are characterized by elevated local or circulatory autoantibodies, cytokines, chemokines, etc., which are usually present before the onset of disease and might be associated with various clinical manifestations. Thus, screening and identifying disease indicators is critical for diagnosis, evaluation, as well as prediction of the disease risks. Generally, the identification of more biomarkers would contribute to increased accuracy in determination of patient diagnosis. The application of proteome microarrays has drawn growing interest in identification of new biomarkers for AIDs because of its outstanding performance in numerous studies. Protein microarrays are quick, cost-effective, high-throughput, and high-sensitivity, proving them to be a powerful technology in basic and translation research. With the rapid technical developments and accumulation of various biomaterials, increasing types of protein arrays, including lab-made disease focused antigen microarray, versatile cytokine microarray, and high-density proteome microarrays, were applied to screen and identify biomarkers for various AIDs. In the foreseeable future, more less-studied AIDs are likely to be examined for novel biomarkers using protein microarray approaches.
Despite numerous successful application of protein microarrays in AID biomarker discovery, there are still challenges that need to be overcome in order to improve the efficiency of these assays, including, but not limited to, the establishment of standard data analysis pipelines and the transition of novel discoveries to clinical lab tests. Hopefully, integration of new computer methods, new biomaterials and artificial intelligence with the protein microarray technology will shed light on biomarker discovery for AIDs in the future.
Author Contributions
JZ and CH designed the research. SL, YB, and NS collected and analyzed the data. SL and GS wrote the paper. JL edited the manuscript. All authors contributed to the article and approved the submitted version.
Funding
This study was supported by the National Natural Science Foundation of China (81771780), the National Key Research and Development Program of China (2019YFC0840603, 2017YFC0907601, and 2017YFC0907602), and the CAMS Initiative for Innovative Medicine (2017-I2M-3-001 and 2019-I2M-2-008).
Conflict of Interest
The authors declare that the research was conducted in the absence of any commercial or financial relationships that could be construed as a potential conflict of interest.
Acknowledgments
We thank Dr. Jessica Dunn for editorial assistance.
References
1. Rose NR. Prediction and Prevention of Autoimmune Disease in the 21st Century: A Review and Preview. Am J Epidemiol (2016) 183(5):403–6. doi: 10.1093/aje/kwv292
2. Syu GD, Dunn J, Zhu H. Developments and Applications of Functional Protein Microarrays. Mol Cell Proteomics MCP (2020) 19(6):916–27. doi: 10.1074/mcp.R120.001936
3. Chen CS, Zhu H. Protein Microarrays. BioTechniques (2006) 40(4):423. 5, 7 passim. doi: 10.2144/06404TE01
4. Haab BB, Dunham MJ, Brown PO. Protein Microarrays for Highly Parallel Detection and Quantitation of Specific Proteins and Antibodies in Complex Solutions. Genome Biol (2001) 2(2):Research0004. doi: 10.1186/gb-2001-2-2-research0004
5. Sutandy FX, Qian J, Chen CS, Zhu H. Overview of Protein Microarrays. Curr Protoc Protein Sci (2013) 27(1):Unit 27.1. doi: 10.1002/0471140864.ps2701s72
6. Abel L, Kutschki S, Turewicz M, Eisenacher M, Stoutjesdijk J, Meyer HE, et al. Autoimmune Profiling With Protein Microarrays in Clinical Applications. Biochim Biophys Acta (BBA) - Proteins Proteomics (2014) 1844(5):977–87. doi: 10.1016/j.bbapap.2014.02.023
7. Ayoglu B, Schwenk JM, Nilsson P. Antigen Arrays for Profiling Autoantibody Repertoires. Bioanalysis (2016) 8(10):1105–26. doi: 10.4155/bio.16.31
8. Lalive PH, Menge T, Barman I, Cree BA, Genain CP. Identification of New Serum Autoantibodies in Neuromyelitis Optica Using Protein Microarrays. Neurology (2006) 67(1):176–7. doi: 10.1212/01.wnl.0000223346.09426.34
9. Kwon OC, Lee EJ, Lee JY, Youn J, Kim TH, Hong S, et al. Prefoldin 5 and Anti-prefoldin 5 Antibodies as Biomarkers for Uveitis in Ankylosing Spondylitis. Front Immunol (2019) 10:384. doi: 10.3389/fimmu.2019.00384
10. Jeong JS, Jiang L, Albino E, Marrero J, Rho HS, Hu J, et al. Rapid Identification of Monospecific Monoclonal Antibodies Using a Human Proteome Microarray. Mol Cell Proteomics MCP (2012) 11(6):O111.016253. doi: 10.1074/mcp.O111.016253
11. Hu CJ, Pan JB, Song G, Wen XT, Wu ZY, Chen S, et al. Identification of Novel Biomarkers for Behcet Disease Diagnosis Using Human Proteome Microarray Approach. Mol Cell Proteomics MCP (2017) 16(2):147–56. doi: 10.1074/mcp.M116.061002
12. Blackburn JM, Hart DJ. Fabrication of Protein Function Microarrays for Systems-Oriented Proteomic Analysis. Methods Mol Biol (Clifton NJ) (2005) 310:197–216. doi: 10.1007/978-1-59259-948-6_14
13. Poulsen TBG, Damgaard D, Jørgensen MM, Senolt L, Blackburn JM, Nielsen CH, et al. Identification of Novel Native Autoantigens in Rheumatoid Arthritis. Biomedicines (2020) 8(6):141. doi: 10.3390/biomedicines8060141
14. Lueking A, Huber O, Wirths C, Schulte K, Stieler KM, Blume-Peytavi U, et al. Profiling of Alopecia Areata Autoantigens Based on Protein Microarray Technology. Mol Cell Proteomics MCP (2005) 4(9):1382–90. doi: 10.1074/mcp.T500004-MCP200
15. Horn S, Lueking A, Murphy D, Staudt A, Gutjahr C, Schulte K, et al. Profiling Humoral Autoimmune Repertoire of Dilated Cardiomyopathy (DCM) Patients and Development of a Disease-Associated Protein Chip. Proteomics (2006) 6(2):605–13. doi: 10.1002/pmic.200401293
16. Beyer NH, Lueking A, Kowald A, Frederiksen JL, Heegaard NH. Investigation of Autoantibody Profiles for Cerebrospinal Fluid Biomarker Discovery in Patients With Relapsing-Remitting Multiple Sclerosis. J Neuroimmunol (2012) 242(1-2):26–32. doi: 10.1016/j.jneuroim.2011.08.013
17. Zingaretti C, Arigò M, Cardaci A, Moro M, Crosti M, Sinisi A, et al. Identification of New Autoantigens by Protein Array Indicates a Role for IL4 Neutralization in Autoimmune Hepatitis. Mol Cell Proteomics MCP (2012) 11(12):1885–97. doi: 10.1074/mcp.M112.018713
18. Bombaci M, Pesce E, Torri A, Carpi D, Crosti M, Lanzafame M, et al. Novel Biomarkers for Primary Biliary Cholangitis to Improve Diagnosis and Understand Underlying Regulatory Mechanisms. Liver Int Off J Int Assoc Study Liver (2019) 39(11):2124–35. doi: 10.1111/liv.14128
19. Miersch S, LaBaer J. Nucleic Acid Programmable Protein Arrays: Versatile Tools for Array-Based Functional Protein Studies. Curr Protoc Protein Sci (2011) 64:27.2.1–27.2.26. doi: 10.1002/0471140864.ps2702s64
20. Miersch S, Bian X, Wallstrom G, Sibani S, Logvinenko T, Wasserfall CH, et al. Serological Autoantibody Profiling of Type 1 Diabetes by Protein Arrays. J Proteomics (2013) 94:486–96. doi: 10.1016/j.jprot.2013.10.018
21. Talwar H, Rosati R, Li J, Kissner D, Ghosh S, Madrid FF, et al. Development of a T7 Phage Display Library to Detect Sarcoidosis and Tuberculosis by a Panel of Novel Antigens. EBioMedicine (2015) 2(4):341–50. doi: 10.1016/j.ebiom.2015.03.007
22. D’Angelo S, Mignone F, Deantonio C, Di Niro R, Bordoni R, Marzari R, et al. Profiling Celiac Disease Antibody Repertoire. Clin Immunol (Orlando Fla) (2013) 148(1):99–109. doi: 10.1016/j.clim.2013.04.009
23. Sjöberg R, Mattsson C, Andersson E, Hellström C, Uhlen M, Schwenk JM, et al. Exploration of High-Density Protein Microarrays for Antibody Validation and Autoimmunity Profiling. New Biotechnol (2016) 33(5, Part A):582–92. doi: 10.1016/j.nbt.2015.09.002
24. Duarte JG, Blackburn JM. Advances in the Development of Human Protein Microarrays. Expert Rev Proteomics (2017) 14(7):627–41. doi: 10.1080/14789450.2017.1347042
25. Idborg H, Zandian A, Ossipova E, Wigren E, Preger C, Mobarrez F, et al. Circulating Levels of Interferon Regulatory Factor-5 Associates With Subgroups of Systemic Lupus Erythematosus Patients. Front Immunol (2019) 10:1029. doi: 10.3389/fimmu.2019.01029
26. Williams S, Stafford P, Hoffman SA. Diagnosis and Early Detection of CNS-SLE in MRL/lpr Mice Using Peptide Microarrays. BMC Immunol (2014) 15:23. doi: 10.1186/1471-2172-15-23
27. Marks DJ, Harbord MW, MacAllister R, Rahman FZ, Young J, Al-Lazikani B, et al. Defective Acute Inflammation in Crohn’s Disease: A Clinical Investigation. Lancet (London England) (2006) 367(9511):668–78. doi: 10.1016/S0140-6736(06)68265-2
28. Vanarsa K, Soomro S, Zhang T, Strachan B, Pedroza C, Nidhi M, et al. Quantitative Planar Array Screen of 1000 Proteins Uncovers Novel Urinary Protein Biomarkers of Lupus Nephritis. Ann Rheum Dis (2020) 79(10):1349–61. doi: 10.1136/annrheumdis-2019-216312
29. Szodoray P, Alex P, Knowlton N, Centola M, Dozmorov I, Csipo I, et al. Idiopathic Inflammatory Myopathies, Signified by Distinctive Peripheral Cytokines, Chemokines and the TNF Family Members B-cell Activating Factor and a Proliferation Inducing Ligand. Rheumatol (Oxford England) (2010) 49(10):1867–77. doi: 10.1093/rheumatology/keq151
30. Lin MW, Ho JW, Harrison LC, dos Remedios CG, Adelstein S. An Antibody-Based Leukocyte-Capture Microarray for the Diagnosis of Systemic Lupus Erythematosus. PloS One (2013) 8(3):e58199. doi: 10.1371/journal.pone.0058199
31. Dang K, Zhang W, Jiang S, Lin X, Qian A. Application of Lectin Microarrays for Biomarker Discovery. ChemistryOpen (2020) 9(3):285–300. doi: 10.1002/open.201900326
32. Takeshita M, Kuno A, Suzuki K, Matsuda A, Shimazaki H, Nakagawa T, et al. Alteration of Matrix Metalloproteinase-3 O-glycan Structure as a Biomarker for Disease Activity of Rheumatoid Arthritis. Arthritis Res Ther (2016) 18(1):112. doi: 10.1186/s13075-016-1013-2
33. Luo H, Wang L, Bao D, Wang L, Zhao H, Lian Y, et al. Novel Autoantibodies Related to Cell Death and DNA Repair Pathways in Systemic Lupus Erythematosus. Genomics Proteomics Bioinf (2019) 17(3):248–59. doi: 10.1016/j.gpb.2018.11.004
34. Huang W, Hu C, Zeng H, Li P, Guo L, Zeng X, et al. Novel Systemic Lupus Erythematosus Autoantigens Identified by Human Protein Microarray Technology. Biochem Biophys Res Commun (2012) 418(2):241–6. doi: 10.1016/j.bbrc.2012.01.001
35. Hu C, Huang W, Chen H, Song G, Li P, Shan Q, et al. Autoantibody Profiling on Human Proteome Microarray for Biomarker Discovery in Cerebrospinal Fluid and Sera of Neuropsychiatric Lupus. PloS One (2015) 10(5):e0126643. doi: 10.1371/journal.pone.0126643
36. McAndrew M, Wheeler C, Koopmann J, Uddin E, Lewis M, Vyse T. Sat0528 Novel Autoantibody Biomarkers for the Improved Diagnosis of Systemic Lupus Erythematosus. Ann Rheum Dis (2013) 72:A760–A. doi: 10.1136/annrheumdis-2013-eular.2252
37. Price JV, Haddon DJ, Kemmer D, Delepine G, Mandelbaum G, Jarrell JA, et al. Protein Microarray Analysis Reveals BAFF-binding Autoantibodies in Systemic Lupus Erythematosus. J Clin Invest (2013) 123(12):5135–45. doi: 10.1172/JCI70231
38. Haddon DJ, Jarrell JA, Diep VK, Wand HE, Price JV, Tangsombatvisit S, et al. Mapping Epitopes of U1-70K Autoantibodies At Single-Amino Acid Resolution. Autoimmunity (2015) 48(8):513–23. doi: 10.3109/08916934.2015.1077233
39. Li X, Li H, Hu Q, Lin J, Zhang Q, Li Y, et al. Detection Of Epitopes in Systemic Lupus Erythematosus Using Peptide Microarray. Mol Med Rep (2018) 17(5):6533–41. doi: 10.3892/mmr.2018.8710
40. Ayoglu B, Mitsios N, Kockum I, Khademi M, Zandian A, Sjöberg R, et al. Anoctamin 2 Identified as an Autoimmune Target in Multiple Sclerosis. Proc Natl Acad Sci USA (2016) 113(8):2188–93. doi: 10.1073/pnas.1518553113
41. Hecker M, Fitzner B, Wendt M, Lorenz P, Flechtner K, Steinbeck F, et al. High-Density Peptide Microarray Analysis of IgG Autoantibody Reactivities in Serum and Cerebrospinal Fluid of Multiple Sclerosis Patients. Mol Cell Proteomics MCP (2016) 15(4):1360–80. doi: 10.1074/mcp.M115.051664
42. Auger I, Balandraud N, Rak J, Lambert N, Martin M, Roudier J. New Autoantigens in Rheumatoid Arthritis (RA): Screening 8268 Protein Arrays With Sera From Patients With RA. Ann Rheum Dis (2009) 68(4):591–4. doi: 10.1136/ard.2008.096917
43. Mizutani Y, Matsuoka K, Takeda H, Shiogama K, Inada K, Hayakawa K, et al. Novel Approach to Identifying Autoantibodies in Rheumatoid Synovitis With a Biotinylated Human Autoantigen Library and the Enzyme-Labeled Antigen Method. J Immunol Methods (2013) 387(1-2):57–70. doi: 10.1016/j.jim.2012.09.011
44. Chandra PE, Sokolove J, Hipp BG, Lindstrom TM, Elder JT, Reveille JD, et al. Novel Multiplex Technology for Diagnostic Characterization of Rheumatoid Arthritis. Arthritis Res Ther (2011) 13(3):R102. doi: 10.1186/ar3383
45. Schulz-Knappe P, Budde P, Zucht HD. PS1:6 Differential Diagnosis of Autoimmune Diseases, Outlier Detection Plus Subgrouping in Clinical Trials by High Content Autoantibody Profiling. Lupus Sci Med (2018) 5(Suppl 1):A35. doi: 10.1136/lupus-2018-abstract.55.
46. Song G, Hu C, Zhu H, Wang L, Zhang F, Li Y, et al. New Centromere Autoantigens Identified in Systemic Sclerosis Using Centromere Protein Microarrays. J Rheumatol (2013) 40(4):461–8. doi: 10.3899/jrheum.120264
47. Carlsson A, Wuttge DM, Ingvarsson J, Bengtsson AA, Sturfelt G, Borrebaeck CA, et al. Serum Protein Profiling of Systemic Lupus Erythematosus and Systemic Sclerosis Using Recombinant Antibody Microarrays. Mol Cell Proteomics MCP (2011) 10(5):M110.005033. doi: 10.1074/mcp.M110.005033
48. Gibson DS, Qiu J, Mendoza EA, Barker K, Rooney ME, LaBaer J. Circulating and Synovial Antibody Profiling of Juvenile Arthritis Patients by Nucleic Acid Programmable Protein Arrays. Arthritis Res Ther (2012) 14(2):R77. doi: 10.1186/ar3800
49. Henjes F, Lourido L, Ruiz-Romero C, Fernández-Tajes J, Schwenk JM, Gonzalez-Gonzalez M, et al. Analysis of Autoantibody Profiles in Osteoarthritis Using Comprehensive Protein Array Concepts. J Proteome Res (2014) 13(11):5218–29. doi: 10.1021/pr500775a
50. Hou L, Zhu Y, Ma X, Li J, Zhang W. Serum Protein Microarray Analysis of Patients With Preeclampsia. Mol Med Rep (2012) 6(1):83–7. doi: 10.3892/mmr.2012.864
51. Loebel M, Eckey M, Sotzny F, Hahn E, Bauer S, Grabowski P, et al. Serological Profiling of the EBV Immune Response in Chronic Fatigue Syndrome Using a Peptide Microarray. PloS One (2017) 12(6):e0179124. doi: 10.1371/journal.pone.0179124
52. Vermeulen N, de Béeck KO, Vermeire S, Van Steen K, Michiels G, Ballet V, et al. Identification of a Novel Autoantigen in Inflammatory Bowel Disease by Protein Microarray. Inflamm bowel Dis (2011) 17(6):1291–300. doi: 10.1002/ibd.21508
53. Chen CS, Sullivan S, Anderson T, Tan AC, Alex PJ, Brant SR, et al. Identification of Novel Serological Biomarkers for Inflammatory Bowel Disease Using Escherichia Coli Proteome Chip. Mol Cell Proteomics MCP (2009) 8(8):1765–76. doi: 10.1074/mcp.M800593-MCP200
54. Shinzaki S, Kuroki E, Iijima H, Tatsunaka N, Ishii M, Fujii H, et al. Lectin-Based Immunoassay for Aberrant IgG Glycosylation as the Biomarker for Crohn’s Disease. Inflamm bowel Dis (2013) 19(2):321–31. doi: 10.1097/MIB.0b013e318280eade
55. Koo BK, Chae S, Kim KM, Kang MJ, Kim EG, Kwak SH, et al. Identification of Novel Autoantibodies in Type 1 Diabetic Patients Using a High-Density Protein Microarray. Diabetes (2014) 63(9):3022–32. doi: 10.2337/db13-1566
56. Bian X, Wallstrom G, Davis A, Wang J, Park J, Throop A, et al. Immunoproteomic Profiling of Antiviral Antibodies in New-Onset Type 1 Diabetes Using Protein Arrays. Diabetes (2016) 65(1):285–96. doi: 10.2337/db15-0179
57. Bian X, Wasserfall C, Wallstrom G, Wang J, Wang H, Barker K, et al. Tracking the Antibody Immunome in Type 1 Diabetes Using Protein Arrays. J Proteome Res (2017) 16(1):195–203. doi: 10.1021/acs.jproteome.6b00354
58. Häggmark A, Hamsten C, Wiklundh E, Lindskog C, Mattsson C, Andersson E, et al. Proteomic Profiling Reveals Autoimmune Targets in Sarcoidosis. Am J Respir Crit Care Med (2015) 191(5):574–83. doi: 10.1164/rccm.201407-1341OC
59. Wright C, Sibani S, Trudgian D, Fischer R, Kessler B, LaBaer J, et al. Detection of Multiple Autoantibodies in Patients With Ankylosing Spondylitis Using Nucleic Acid Programmable Protein Arrays. Mol Cell Proteomics MCP (2012) 11(2):M9.00384. doi: 10.1074/mcp.M9.00384
60. Hu S, Vissink A, Arellano M, Roozendaal C, Zhou H, Kallenberg CG, et al. Identification of Autoantibody Biomarkers for Primary Sjögren’s Syndrome Using Protein Microarrays. Proteomics (2011) 11(8):1499–507. doi: 10.1002/pmic.201000206
61. Willems B, Tong L, Minh TDT, Pham ND, Nguyen XH, Zumbansen M. Novel Cytokine Multiplex Assay for Tear Fluid Analysis in Sjogren’s Syndrome. Ocular Immunol Inflammation (2020) 28:1–6. doi: 10.1080/09273948.2020.1767792
62. Hu CJ, Song G, Huang W, Liu GZ, Deng CW, Zeng HP, et al. Identification of New Autoantigens for Primary Biliary Cirrhosis Using Human Proteome Microarrays. Mol Cell Proteomics MCP (2012) 11(9):669–80. doi: 10.1074/mcp.M111.015529
63. Kim SH, Kim JY, Lee HJ, Gi M, Kim BG, Choi JY. Autoimmunity as a Candidate for the Etiopathogenesis of Meniere’s Disease: Detection of Autoimmune Reactions and Diagnostic Biomarker Candidate. PloS One (2014) 9(10):e111039. doi: 10.1371/journal.pone.0111039
64. Peschl P, Ramberger M, Höftberger R, Jöhrer K, Baumann M, Rostásy K, et al. Methodological Challenges in Protein Microarray and Immunohistochemistry for the Discovery of Novel Autoantibodies in Paediatric Acute Disseminated Encephalomyelitis. Int J Mol Sci (2017) 18(3):679. doi: 10.3390/ijms18030679
65. Landegren N, Sharon D, Shum AK, Khan IS, Fasano KJ, Hallgren Å, et al. Transglutaminase 4 as a Prostate Autoantigen in Male Subfertility. Sci Trans Med (2015) 7(292):292ra101. doi: 10.1126/scitranslmed.aaa9186
66. Mazzara S, Sinisi A, Cardaci A, Rossi RL, Muratori L, Abrignani S, et al. Two of Them do It Better: Novel Serum Biomarkers Improve Autoimmune Hepatitis Diagnosis. PloS One (2015) 10(9):e0137927. doi: 10.1371/journal.pone.0137927
67. Balboni I, Niewold TB, Morgan G, Limb C, Eloranta ML, Rönnblom L, et al. Interferon-α Induction and Detection of Anti-Ro, Anti-La, Anti-Sm, and Anti-Rnp Autoantibodies by Autoantigen Microarray Analysis in Juvenile Dermatomyositis. Arthritis Rheum (2013) 65(9):2424–9. doi: 10.1002/art.38038
68. Kuo HC, Huang YH, Chung FH, Chen PC, Sung TC, Chen YW, et al. Antibody Profiling of Kawasaki Disease Using Escherichia Coli Proteome Microarrays. Mol Cell Proteomics MCP (2018) 17(3):472–81. doi: 10.1074/mcp.RA117.000198
69. Joos TO, Schrenk M, Höpfl P, Kröger K, Chowdhury U, Stoll D, et al. A Microarray Enzyme-Linked Immunosorbent Assay for Autoimmune Diagnostics. Electrophoresis (2000) 21(13):2641–50. doi: 10.1002/1522-2683(20000701)21:13<2641::AID-ELPS2641>3.0.CO;2-5
70. Robinson WH, DiGennaro C, Hueber W, Haab BB, Kamachi M, Dean EJ, et al. Autoantigen Microarrays for Multiplex Characterization of Autoantibody Responses. Nat Med (2002) 8(3):295–301. doi: 10.1038/nm0302-295
71. Feng Y, Ke X, Ma R, Chen Y, Hu G, Liu F. Parallel Detection of Autoantibodies With Microarrays in Rheumatoid Diseases. Clin Chem (2004) 50(2):416–22. doi: 10.1373/clinchem.2003.023994
72. Hueber W, Kidd BA, Tomooka BH, Lee BJ, Bruce B, Fries JF, et al. Antigen Microarray Profiling of Autoantibodies in Rheumatoid Arthritis. Arthritis Rheum (2005) 52(9):2645–55. doi: 10.1002/art.21269
73. Quintana FJ, Hagedorn PH, Elizur G, Merbl Y, Domany E, Cohen IR. Functional Immunomics: Microarray Analysis of IgG Autoantibody Repertoires Predicts the Future Response of Mice to Induced Diabetes. Proc Natl Acad Sci USA (2004) 101 Suppl 2(Suppl 2):14615–21. doi: 10.1073/pnas.0404848101
74. Li QZ, Xie C, Wu T, Mackay M, Aranow C, Putterman C, et al. Identification of Autoantibody Clusters That Best Predict Lupus Disease Activity Using Glomerular Proteome Arrays. J Clin Invest (2005) 115(12):3428–39. doi: 10.1172/JCI23587
75. Quintana FJ, Farez MF, Viglietta V, Iglesias AH, Merbl Y, Izquierdo G, et al. Antigen Microarrays Identify Unique Serum Autoantibody Signatures in Clinical and Pathologic Subtypes of Multiple Sclerosis. Proc Natl Acad Sci USA (2008) 105(48):18889–94. doi: 10.1073/pnas.0806310105
76. Quintana FJ, Farez MF, Izquierdo G, Lucas M, Cohen IR, Weiner HL. Antigen Microarrays Identify CNS-produced Autoantibodies in RRMS. Neurology (2012) 78(8):532–9. doi: 10.1212/WNL.0b013e318247f9f3
77. Haddon DJ, Diep VK, Price JV, Limb C, Utz PJ, Balboni I. Autoantigen Microarrays Reveal Autoantibodies Associated With Proliferative Nephritis and Active Disease in Pediatric Systemic Lupus Erythematosus. Arthritis Res Ther (2015) 17(1):162. doi: 10.1186/s13075-015-0682-6
78. Fattal I, Shental N, Mevorach D, Anaya JM, Livneh A, Langevitz P, et al. An Antibody Profile of Systemic Lupus Erythematosus Detected by Antigen Microarray. Immunology (2010) 130(3):337–43. doi: 10.1111/j.1365-2567.2010.03245.x
79. James JA, Guthridge JM, Chen H, Lu R, Bourn RL, Bean K, et al. Unique Sjögren’s Syndrome Patient Subsets Defined by Molecular Features. Rheumatol (Oxford England) (2020) 59(4):860–8. doi: 10.1093/rheumatology/kez335
80. MacBeath G, Schreiber SL. Printing Proteins as Microarrays for High-Throughput Function Determination. Sci (New York NY) (2000) 289(5485):1760–3. doi: 10.1126/science.289.5485.1760
81. Zhu H, Bilgin M, Bangham R, Hall D, Casamayor A, Bertone P, et al. Global Analysis of Protein Activities Using Proteome Chips. Sci (New York NY) (2001) 293(5537):2101–5. doi: 10.1126/science.1062191
82. Butte AJ, Sigdel TK, Wadia PP, Miklos DB, Sarwal MM. Protein Microarrays Discover Angiotensinogen and PRKRIP1 as Novel Targets for Autoantibodies in Chronic Renal Disease. Mol Cell Proteomics MCP (2011) 10(3):M110.000497. doi: 10.1074/mcp.M110.000497
83. Hamanaka S, Nakagawa T, Hiwasa T, Ohta Y, Kasamatsu S, Ishigami H, et al. Investigation of Novel Biomarkers for Predicting the Clinical Course in Patients With Ulcerative Colitis. J Gastroenterol Hepatol (2018) 33(12):1975–83. doi: 10.1111/jgh.14297
84. Song Q, Liu G, Hu S, Zhang Y, Tao Y, Han Y, et al. Novel Autoimmune Hepatitis-Specific Autoantigens Identified Using Protein Microarray Technology. J Proteome Res (2010) 9(1):30–9. doi: 10.1021/pr900131e
85. Lewis MJ, McAndrew MB, Wheeler C, Workman N, Agashe P, Koopmann J, et al. Autoantibodies Targeting TLR and SMAD Pathways Define New Subgroups in Systemic Lupus Erythematosus. J Autoimmun (2018) 91:1–12. doi: 10.1016/j.jaut.2018.02.009
86. Sawasaki T, Ogasawara T, Morishita R, Endo Y. A cell-free protein synthesis system for high-throughput proteomics. Proc Natl Acad Sci USA (2002) 99(23):14652–7. doi: 10.1073/pnas.232580399
87. Onishi S, Adnan E, Ishizaki J, Miyazaki T, Tanaka Y, Matsumoto T, et al. Novel Autoantigens Associated With Lupus Nephritis. PloS One (2015) 10(6):e0126564. doi: 10.1371/journal.pone.0126564
88. Díez P, González-González M, Lourido L, Dégano RM, Ibarrola N, Casado-Vela J, et al. NAPPA as a Real New Method for Protein Microarray Generation. Microarrays (Basel Switzerland) (2015) 4(2):214–27. doi: 10.3390/microarrays4020214
89. Bian X, Wiktor P, Kahn P, Brunner A, Khela A, Karthikeyan K, et al. Antiviral Antibody Profiling by High-Density Protein Arrays. Proteomics (2015) 15(12):2136–45. doi: 10.1002/pmic.201400612
90. Hsu TY, Lin JM, Nguyen MT, Chung FH, Tsai CC, Cheng HH, et al. Antigen Analysis of Pre-Eclamptic Plasma Antibodies Using Escherichia Coli Proteome Chips. Mol Cell Proteomics MCP (2018) 17(8):1457–69. doi: 10.1074/mcp.RA117.000139
91. Berglund L, Björling E, Jonasson K, Rockberg J, Fagerberg L, Al-Khalili Szigyarto C, et al. A Whole-Genome Bioinformatics Approach to Selection of Antigens for Systematic Antibody Generation. Proteomics (2008) 8(14):2832–9. doi: 10.1002/pmic.200800203
92. Ayoglu B, Häggmark A, Khademi M, Olsson T, Uhlén M, Schwenk JM, et al. Autoantibody Profiling in Multiple Sclerosis Using Arrays of Human Protein Fragments. Mol Cell Proteomics MCP (2013) 12(9):2657–72. doi: 10.1074/mcp.M112.026757
93. Price JV, Tangsombatvisit S, Xu G, Yu J, Levy D, Baechler EC, et al. On Silico Peptide Microarrays for High-Resolution Mapping of Antibody Epitopes and Diverse Protein-Protein Interactions. Nat Med (2012) 18(9):1434–40. doi: 10.1038/nm.2913
94. Vilcek J, Feldmann M. Historical Review: Cytokines as Therapeutics and Targets of Therapeutics. Trends Pharmacol Sci (2004) 25(4):201–9. doi: 10.1016/j.tips.2004.02.011
95. Kader HA, Tchernev VT, Satyaraj E, Lejnine S, Kotler G, Kingsmore SF, et al. Protein Microarray Analysis of Disease Activity in Pediatric Inflammatory Bowel Disease Demonstrates Elevated Serum PLGF, Il-7, TGF-beta1, and IL-12p40 Levels in Crohn’s Disease and Ulcerative Colitis Patients in Remission Versus Active Disease. Am J Gastroenterol (2005) 100(2):414–23. doi: 10.1111/j.1572-0241.2005.40819.x
96. Wilson JJ, Burgess R, Mao YQ, Luo S, Tang H, Jones VS, et al. Antibody Arrays in Biomarker Discovery. Adv Clin Chem (2015) 69:255–324. doi: 10.1016/bs.acc.2015.01.002
97. Wu T, Du Y, Han J, Singh S, Xie C, Guo Y, et al. Urinary Angiostatin–a Novel Putative Marker of Renal Pathology Chronicity in Lupus Nephritis. Mol Cell Proteomics MCP (2013) 12(5):1170–9. doi: 10.1074/mcp.M112.021667
98. Chen H, Shi B, Feng X, Kong W, Chen W, Geng L, et al. Leptin and Neutrophil-Activating Peptide 2 Promote Mesenchymal Stem Cell Senescence Through Activation of the Phosphatidylinositol 3-Kinase/Akt Pathway in Patients With Systemic Lupus Erythematosus. Arthritis Rheumatol (Hoboken NJ) (2015) 67(9):2383–93. doi: 10.1002/art.39196
99. Hughes-Austin JM, Deane KD, Derber LA, Kolfenbach JR, Zerbe GO, Sokolove J, et al. Multiple Cytokines and Chemokines are Associated With Rheumatoid Arthritis-Related Autoimmunity in First-Degree Relatives Without Rheumatoid Arthritis: Studies of the Aetiology of Rheumatoid Arthritis (Sera). Ann Rheum Dis (2013) 72(6):901–7. doi: 10.1136/annrheumdis-2012-201505
100. Chen Z, Dodig-Crnković T, Schwenk JM, Tao SC. Current Applications of Antibody Microarrays. Clin Proteomics (2018) 15:7. doi: 10.1186/s12014-018-9184-2
101. Qi H, Wang F, Tao SC. Proteome Microarray Technology and Application: Higher, Wider, and Deeper. Expert Rev Proteomics (2019) 16(10):815–27. doi: 10.1080/14789450.2019.1662303
102. Moore CD, Ajala OZ, Zhu H. Applications in High-Content Functional Protein Microarrays. Curr Opin Chem Biol (2016) 30:21–7. doi: 10.1016/j.cbpa.2015.10.013
103. Laborde CM, Castro-Santos P, Díaz-Peña R. Contribution of Multiplex Immunoassays to Rheumatoid Arthritis Management: From Biomarker Discovery to Personalized Medicine. J Personalized Med (2020) 10(4):202. doi: 10.3390/jpm10040202
104. Idborg H, Zandian A, Sandberg AS, Nilsson B, Elvin K, Truedsson L, et al. Two Subgroups in Systemic Lupus Erythematosus With Features of Antiphospholipid or Sjögren’s Syndrome Differ in Molecular Signatures and Treatment Perspectives. Arthritis Res Ther (2019) 21(1):62. doi: 10.1186/s13075-019-1836-8
105. Tasaki S, Suzuki K, Kassai Y, Takeshita M, Murota A, Kondo Y, et al. Multi-Omics Monitoring of Drug Response in Rheumatoid Arthritis in Pursuit of Molecular Remission. Nat Commun (2018) 9(1):2755. doi: 10.1038/s41467-018-05044-4
106. Vordenbäumen S, Brinks R, Hoyer A, Fischer-Betz R, Pongratz G, Lowin T, et al. Comprehensive Longitudinal Surveillance of the IgG Autoantibody Repertoire in Established Systemic Lupus Erythematosus. Arthritis Rheumatol (Hoboken NJ) (2019) 71(5):736–43. doi: 10.1002/art.40788
107. Chase Huizar C, Raphael I, Forsthuber TG. Genomic, Proteomic, and Systems Biology Approaches in Biomarker Discovery for Multiple Sclerosis. Cell Immunol (2020) 358:104219. doi: 10.1016/j.cellimm.2020.104219
108. Pan J, Song G, Chen D, Li Y, Liu S, Hu S, et al. Identification of Serological Biomarkers for Early Diagnosis of Lung Cancer Using a Protein Array-Based Approach. Mol Cell Proteomics MCP (2017) 16(12):2069–78. doi: 10.1074/mcp.RA117.000212
109. Balboni I, Limb C, Tenenbaum JD, Utz PJ. Evaluation of Microarray Surfaces and Arraying Parameters for Autoantibody Profiling. Proteomics (2008) 8(17):3443–9. doi: 10.1002/pmic.200800146
110. Sonawane MD, Nimse SB. Surface Modification Chemistries of Materials Used in Diagnostic Platforms With Biomolecules. J Chem (2016) 2016:9241378. doi: 10.1155/2016/9241378
111. Brittain WJ, Brandsetter T, Prucker O, Ruhe J. The Surface Science of Microarray Generation-a Critical Inventory. ACS Appl Mater Interfaces (2019) 11(43):39397–409. doi: 10.1021/acsami.9b06838
112. Kodadek T. Protein Microarrays: Prospects and Problems. Chem Biol (2001) 8(2):105–15. doi: 10.1016/S1074-5521(00)90067-X
113. Hu S, Xie Z, Onishi A, Yu X, Jiang L, Lin J, et al. Profiling the Human protein-DNA Interactome Reveals ERK2 as a Transcriptional Repressor of Interferon Signaling. Cell (2009) 139(3):610–22. doi: 10.1016/j.cell.2009.08.037
114. Uzoma I, Zhu H. Interactome Mapping: Using Protein Microarray Technology to Reconstruct Diverse Protein Networks. Genomics Proteomics Bioinf (2013) 11(1):18–28. doi: 10.1016/j.gpb.2012.12.005
115. Viejo-Borbolla A, Schildberg FA, Burgert HG. Mechanisms of Extracellular Immunomodulation Mediated by Infectious Agents. J Immunol Res (2017) 2017:5107527. doi: 10.1155/2017/5107527
116. Szymczak LC, Kuo HY, Mrksich M. Peptide Arrays: Development and Application. Analytical Chem (2018) 90(1):266–82. doi: 10.1021/acs.analchem.7b04380
117. Kuhne Y, Reese G, Ballmer-Weber BK, Niggemann B, Hanschmann KM, Vieths S, et al. A Novel Multipeptide Microarray for the Specific and Sensitive Mapping of Linear Ige-Binding Epitopes of Food Allergens. Int Arch Allergy Immunol (2015) 166(3):213–24. doi: 10.1159/000381344
118. Whittemore K, Johnston SA, Sykes K, Shen L. A General Method to Discover Epitopes from Sera. PloS One (2016) 11(6):e0157462. doi: 10.1371/journal.pone.0157462
119. Hansen LB, Buus S, Schafer-Nielsen C. Identification and Mapping of Linear Antibody Epitopes in Human Serum Albumin Using High-Density Peptide Arrays. PloS One (2013) 8(7):e68902. doi: 10.1371/journal.pone.0068902
120. Buus S, Rockberg J, Forsstrom B, Nilsson P, Uhlen M, Schafer-Nielsen C. High-Resolution Mapping of Linear Antibody Epitopes Using Ultrahigh-Density Peptide Microarrays. Mol Cell Proteomics MCP (2012) 11(12):1790–800. doi: 10.1074/mcp.M112.020800
121. Straussman R, Morikawa T, Shee K, Barzily-Rokni M, Qian ZR, Du J, et al. Tumour Micro-Environment Elicits Innate Resistance to RAF Inhibitors Through HGF Secretion. Nature (2012) 487(7408):500–4. doi: 10.1038/nature11183
122. Scheel C, Eaton EN, Li SH, Chaffer CL, Reinhardt F, Kah KJ, et al. Paracrine and Autocrine Signals Induce and Maintain Mesenchymal and Stem Cell States in the Breast. Cell (2011) 145(6):926–40. doi: 10.1016/j.cell.2011.04.029
123. Vargas DL, Nascimbene C, Krishnan C, Zimmerman AW, Pardo CA. Neuroglial Activation and Neuroinflammation in the Brain of Patients With Autism. Ann Neurol (2005) 57(1):67–81. doi: 10.1002/ana.20315
124. Coppe JP, Patil CK, Rodier F, Sun Y, Munoz DP, Goldstein J, et al. Senescence-Associated Secretory Phenotypes Reveal Cell-Nonautonomous Functions of Oncogenic RAS and the p53 Tumor Suppressor. PloS Biol (2008) 6(12):2853–68. doi: 10.1371/journal.pbio.0060301
125. Purohit S, Li T, Guan W, Song X, Song J, Tian Y, et al. Multiplex Glycan Bead Array for High Throughput and High Content Analyses of Glycan Binding Proteins. Nat Commun (2018) 9(1):258. doi: 10.1038/s41467-017-02747-y
126. Geissner A, Reinhardt A, Rademacher C, Johannssen T, Monteiro J, Lepenies B, et al. Microbe-Focused Glycan Array Screening Platform. Proc Natl Acad Sci USA (2019) 116(6):1958–67. doi: 10.1073/pnas.1800853116
127. Williams SA, Kivimaki M, Langenberg C, Hingorani AD, Casas JP, Bouchard C, et al. Plasma Protein Patterns as Comprehensive Indicators of Health. Nat Med (2019) 25(12):1851–7. doi: 10.1038/s41591-019-0665-2
128. Gupta S, Manubhai KP, Kulkarni V, Srivastava S. An Overview of Innovations and Industrial Solutions in Protein Microarray Technology. Proteomics (2016) 16(8):1297–308. doi: 10.1002/pmic.201500429
129. Suppers A, van Gool AJ, Wessels H. Integrated Chemometrics and Statistics to Drive Successful Proteomics Biomarker Discovery. Proteomes (2018) 6(2):20. doi: 10.3390/proteomes6020020
130. Xu Z, Huang L, Zhang H, Li Y, Guo S, Wang N, et al. Pmd: A Resource for Archiving and Analyzing Protein Microarray Data. Sci Rep (2016) 6:19956. doi: 10.1038/srep19956
131. Pan J, Liu S, Zhu H, Qian J. AagMarker 1.0: A Resource of Serological Autoantigen Biomarkers for Clinical Diagnosis and Prognosis of Various Human Diseases. Nucleic Acids Res (2018) 46(D1):D886–93. doi: 10.1093/nar/gkx770
Keywords: protein microarray, proteome, biomarker discovery, autoimmune disease, experimental design
Citation: Li S, Song G, Bai Y, Song N, Zhao J, Liu J and Hu C (2021) Applications of Protein Microarrays in Biomarker Discovery for Autoimmune Diseases. Front. Immunol. 12:645632. doi: 10.3389/fimmu.2021.645632
Received: 23 December 2020; Accepted: 13 April 2021;
Published: 03 May 2021.
Edited by:
James A. Lederer, Brigham and Women's Hospital and Harvard Medical School, United StatesReviewed by:
Richard David Leslie, Queen Mary University of London, United KingdomRobert Nagele, Rowan University, United States
Copyright © 2021 Li, Song, Bai, Song, Zhao, Liu and Hu. This is an open-access article distributed under the terms of the Creative Commons Attribution License (CC BY). The use, distribution or reproduction in other forums is permitted, provided the original author(s) and the copyright owner(s) are credited and that the original publication in this journal is cited, in accordance with accepted academic practice. No use, distribution or reproduction is permitted which does not comply with these terms.
*Correspondence: Jian Liu, 2120044180@qq.com; Chaojun Hu, huchaojun818@qq.com
†These authors have contributed equally to this work and share first authorship